This chapter reviews the current landscape of urban passenger transport, including a number of technological and business developments that disrupt the sector in the future. It develops two main disruptive scenarios: one that portrays a future in which policy adjustments are made to manage the disruptive effects of these developments and one in which no such adjustments are made. The results indicate that disruptive developments may lead to modal shifts that increase congestion and emissions by 2050, and that targeted policies will be necessary in order to steer these developments in directions that minimise their negative externalities and maximise their co-benefits.
ITF Transport Outlook 2019
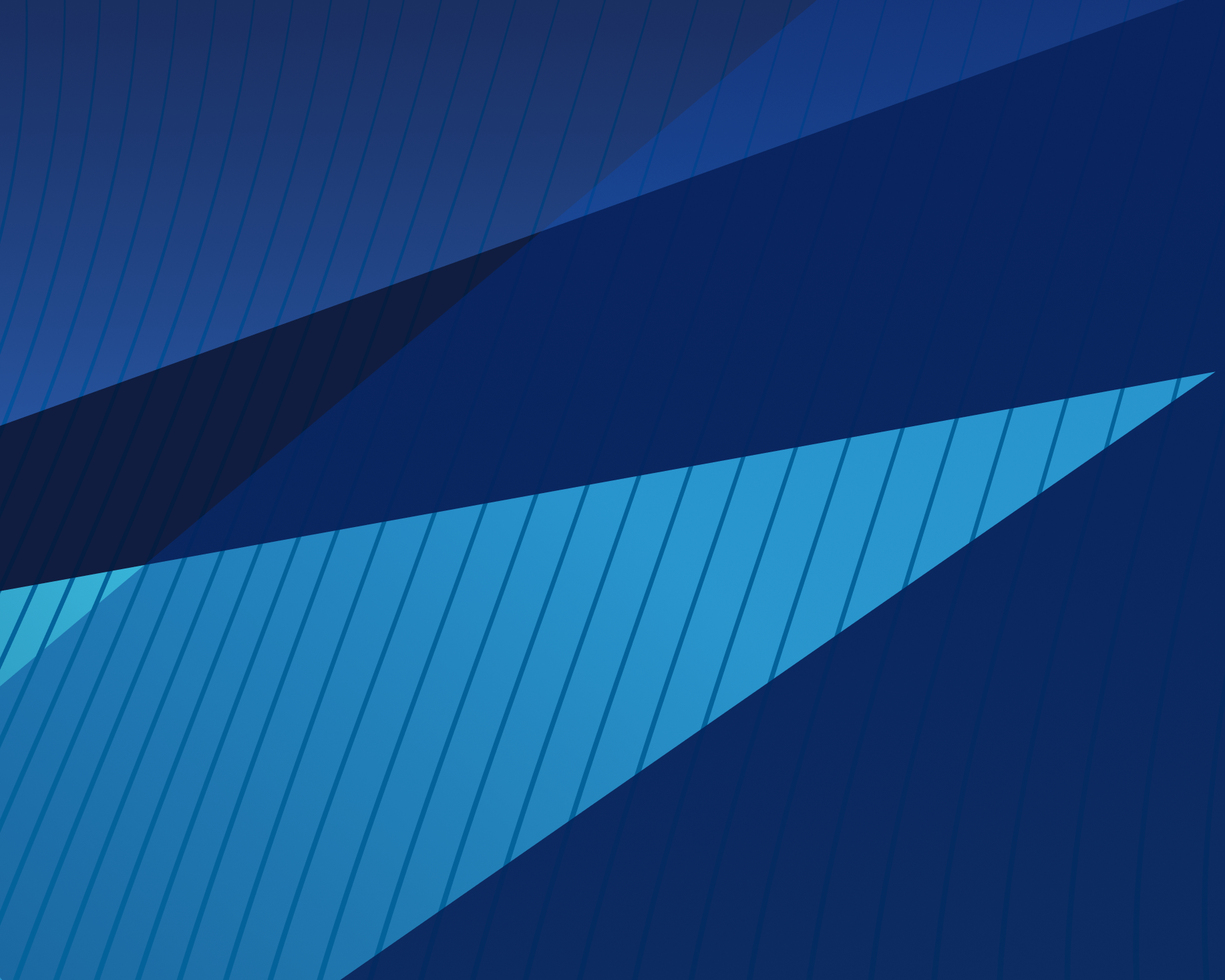
Chapter 3. Disruptions in urban passenger transport
Abstract
The urban mobility landscape is rapidly changing
Most trips today take place in urban regions, and their number is projected to continue to grow in line with cities’ population and gross domestic product (GDP). Worldwide, urban regions face both constraints and opportunities with respect to mobility. The limits of the current mix of transport solutions will be most strongly felt in cities. On the other hand, urban areas will be fertile ground for game-changing transport innovations. This push and pull is not new. Innovation under constraints has always been a force for systemic change, and the transport sector is no exception. Today, the innovation cycle is accelerating again, with a rapid convergence of disruptive technologies, business models and services, notably in urban areas. This convergence is particularly visible in urban mobility, but extends across many other areas of the economy and society.
Will these disruptive developments take hold and, more importantly, will they reach a sufficient scale? If they do, tomorrow’s transport may well operate under a different socio-technical regime from the one that characterises urban mobility today. For cities, it will be critically important to understand the conditions that would bring about such disruptions identify their potential impacts and reflect on how policy can guide and manage them.
Figure 3.1. Share of population residing in urban areas by region, 1950‑2050
Change across the wide range of urban settings worldwide will not be uniform. Different policy, economic, social or geographical contexts mean innovations will play out differently in different urban settings. What may prove to be disruptive in Beijing or Los Angeles may be less impactful in Brussels or Lagos. Likewise, the timing and duration of disruptive effects may differ from city to city and from country to country.
Finally, these disruptions will generally confer immediate benefits to those that take advantage of them – and especially to those that do so early. However, from the societal perspective their aggregate impact may be positive, negative or inconsequential. There is a clear role in proactive policy to ensure that the action of public authorities guide disruption in order to maximise benefits and minimise negative outcomes. This, of course, is difficult to do when the disruptive trends first emerge as weak outlier signals before they gain traction and scale up.
The context-specific characteristics of different urban areas and the very nature of disruptions themselves make it difficult to predict the pathway of disruptive developments. This Transport Outlook examines three plausible and potentially disruptive developments in isolation, as well as in combination with each other, in various scenarios.
The three disruptive developments for urban mobility examined in this chapter are:
1. A widespread adoption of teleworking and telepresence more broadly;
2. A significant increase of automated and autonomous driving in cities;
3. Massive uptake of shared and optimised mobility services.
Leaving aside telework, these disruptive developments do not rely on a fundamental change in the basic vehicle technology underpinning the urban mobility eco-system. Autonomous driving and widespread shared mobility will continue to rely on vehicles operating on city streets and on rails. These vehicles more or less resemble today’s fleets of cars, vans, buses, trains, bicycles and various forms of motorised single-person vehicles. The scaling-up of these two disruptive developments may be made easier by the fact that they do not call into question the current socio-technical system built around the construction, maintenance, fuelling, insuring and licensing of vehicles. Of course, both disruptions, individually and taken together, imply a significant shift in the ways in which vehicles are used and in the business models and travel behaviours that may emerge with their development. Telework and telepresence, on the other hand, could eliminate some transport activity and replace the socio-technical system that supports it with another configuration based on virtual presence.
A common feature of the three disruptions is their reliance on computer and communication technology, information and data – and the emergence of new business models enabled by digitalisation. With regard to teleworking, the meaningful and productive virtual presence of workers requires ubiquitous network technologies and systems.
With respect to autonomous driving, replacing human drivers with algorithm-led and artificial intelligence-based control systems requires the development and deployment of skilful sensing-processing-actuator technologies and opens up new fields for industrial production and commercial service development. This will also induce changes in support activities such as law, insurance, coding and design. Autonomous vehicles may improve safety and reduce cost for fleet operators, potentially leading to new service delivery models that could be tied to shared mobility services or Mobility as a Service (MaaS) packages.
Likewise, these disruptions will improve supply-demand matching and seamless integration across modes enabling the widespread uptake of new mobility services. These have the potential to affect travel costs, thereby reshape users’ mode choices and ultimately lead to new travel patterns. A massive uptake of shared mobility services, supported by MaaS, holds particular potential for shifting two fundamental and persistent paradigms in the provision of current urban mobility services: the ownership of private transport and “scheduling” of public transport. New models of mobility would enable on-demand, optimised sharing of vehicles and seamless mixing of modes and allow mobility providers to better target the needs and desires of individual travellers.
All three disruptions face a formidable hurdle: the ever-compelling model of private, car-based mobility. How far the disruptive trends will be able, alone or in combination, to drastically shift the status quo will depend on the constraints travellers face in the current mobility system, the extent to which regulatory frameworks encourage or hinder new mobility models, and finally the relative costs of new mobility services compared to those currently available (and how these costs evolve over time).
The mitigation potential of urban transport policies
The urban passenger transport model developed by the International Transport Forum (ITF) assesses transport activity, mode shares and related emissions under various policy scenarios for all urban areas with a population above 50 000 inhabitants across all world regions up to the year 2050.1 This model framework is constantly evolving as new data sources are incorporated and analytic methods improved. Box 3.1 highlights the most recent enhancements to this model.
Box 3.1. Recent enhancements to the ITF urban passenger model
The ITF’s urban passenger model was presented in 2017 (ITF, 2017[2]). Since then, the model has been enhanced in several ways. The main model updates were:
1. Improved representation of different transport modes and their interaction: now included are shared mobility services (i.e. services with a driver, where people share the same vehicle for at least a part of their trip, such as shared taxis and mini-buses) as well as shared vehicle systems (i.e. shared cars, shared bikes, shared motorbikes, and shared scooters, where users do not necessarily share their trip with anyone else). Also now included is an integration parameter that reflects the ease of changing between different means of transport, e.g. between new and conventional transport modes.
2. Refined GDP and car ownership estimates: these are now city-specific instead of country-specific.
3. More detailed representation of small urban areas: (50‑300 000 inhabitants) is made possible by introducing a new category of “small cities”. Mode share patterns for small cities are different from those of larger cities, reflecting for example the fact that small cities often do not have mass transit services
Two urban mobility scenarios were developed for this Transport Outlook, a current ambition scenario and a high ambition scenario (see Table 3.1 for scenario specifications). Ambition in this context reflects the effort undertaken by stakeholders to reduce CO2 emissions and introduce respective CO2 mitigation policies. Each scenario delivers expected increases in the demand for urban mobility in different ways (Figure 3.2), which has a significant impact on resulting CO2 emissions in the sector across scenarios (Figure 3.3). Later on in this Chapter, additional scenarios that assess the impact of potential disruptive developments on travel demand and related CO2 emissions are explored.
The current ambition scenario for urban passenger transport
The current ambition scenario is characterised by a continuation of current urban transport policy portfolios, including announced policies that are set to take effect in future years (Table 3.1). In the current ambition scenario, technological advancements and the uptake of autonomous and shared mobility occur at a moderate pace. The electrification rate of urban vehicle fleets is in line with the New Policies Scenario (NPS) of the International Energy Agency (IEA, 2018[3]). Transport modes are integrated only to a limited degree; there are still significant barriers to seamless travel across different transport modes for urban travellers. The supply of public transport develops in line with historical trends. The density of urban areas does not change dramatically, although urban sprawl continues in some regions.
Based on recent trends, some cities in the current ambition scenario implement car restriction policies in the urban core supported by corresponding parking policies to tackle increased congestion and pollution. The policy measures assumed in this scenario are already more stringent than those in the baseline scenario presented in the 2017 Transport Outlook (ITF, 2017[2]). This reflects an increased awareness of the challenges related to urban passenger transport and the impact of recent and forthcoming mitigation measures that cities have been taking as a result. Overall travel activity grows in line with the GDP and population projections. The increased uptake of teleworking somewhat slows the growth of passenger-kilometres travelled, resulting in a growth of total passenger-kilometres in urban areas by 38% by 2030 and by 104% to 2050 compared with 2015.
The share of urban passenger-kilometres travelled in private vehicles (including individual taxi services) declines from around 70% in 2015 to 40% by 2050 in the current ambition scenario. Shared modes2 that include shared vehicles systems (e.g. free-floating or non-free-floating shared cars, bikes or scooters)3 and optimised shared mobility services (e.g. a shared taxi, van or minibus with a driver) grow to account for over 20% of the total demand in cities by 2050. This is mainly through optimised shared services, where travellers share the same vehicle with a driver for at least a part of their trip. The share of more traditional public transport (bus, rail, metro) grows modestly from 30% in 2015 to over 35% of all urban passenger-kilometres travelled by 2050. Growth in demand for shared modes and public transit is mainly due to a gradual increase in the provision of new shared mobility services that better fit the customers’ needs and continued improvements in mass public transport services.
CO2 emissions from urban travel fall by 20% in 2050 compared to 2015 in the current ambition scenario, even though total passenger-kilometres double. This is largely due to increased vehicle load factors (due to the increase of the share of optimised shared mobility services and public transport) and improved vehicle fuel economy. Average CO2 emissions per passenger-kilometre (gCO2/p-km) fall from around 126 g CO2/p-km in 2015 to 50 g CO2/p-km in 2050. The CO2 emissions from an average car (i.e. g CO2 per vehicle-kilometre) fall by 48% in North America, by 54% in the People’s Republic of China and India and by 43% globally, in line with IEA’s New Policies Scenario.
The high ambition scenario for urban passenger transport
In the high ambition scenario, policy makers implement a set of ambitious policy measures that aim to optimise the use of scarce public space and reduce negative externalities from urban transport. The uptake of electric vehicles is accelerated, following the EV30@30 scenario (IEA, 2018[4]). Transport modes are better integrated than in the current ambition scenario, resulting in a higher mode share of public transport and active transport modes (walking and cycling). More money is invested in mass public transport, and integrated policies for transport and land use result in more densely populated urban areas.
Policy makers also seek to manage car use and regulate parking more actively to incentivise more space-efficient transport, reduce congestion and decrease greenhouse gas emissions. Teleworking is encouraged, which reduces travel activity compared to the current ambition scenario. Given uncertainty around the effect of shared and autonomous mobility on overall travel activity (and hence CO2 emissions), the high ambition scenario does not include policies that could accelerate the uptake of these potential disruptions, and thus the provision of shared modes and autonomous mobility is the same as in the current ambition scenario.
Projections based on the high ambition scenario see overall travel distance in urban areas fall in the coming decades. Urban passenger-kilometres travelled in 2050 under these assumptions are 15% lower than in the current ambition scenario. This is due to shorter travel distances in cities that are more densely populated as result of land use policies. A higher share of travel by public transport and higher use of shared modes also translate into shorter travel distances on average than compared to private vehicle use. Finally, more teleworking leads to a moderate decrease in overall travel activity.
The share of private modes of all urban travel falls to 30% of the urban total passenger-kilometres by 2050, compared to around 40% in the current ambition scenario and around 70% in 2015. This is the result of more targeted policies for car use and parking, combined with strong support for integrating the different transport services. Increased average vehicle occupancy and high uptake of electric vehicles help cut CO2 emissions further. Both of these metrics decline to around 20% of 2015 levels by 2050 in the high ambition scenario, around 70% lower than in the current ambition scenario. The average level of CO2 emissions per passenger-kilometre drops to 17g across all world regions. By 2050, the fuel economy of an average car improves by around 75% compared to 2015 with high ambition policies.
Table 3.1. Current and high ambition scenario specifications for urban transport
Assumption |
Current ambition |
High ambition |
||
---|---|---|---|---|
Mitigation measures |
||||
|
Efficiency improvements and electric vehicles |
The percentage of electric vehicles in use varies across regions: e.g. for cars 1‑22% by 2050 (based on (IEA, 2018[3])– NPS) |
The percentage of electric vehicles in use varies across regions: e.g. for cars 42‑64% by 2050 (based on (IEA, 2018[4]) - EV30@30) |
|
|
Mobility as a service (MaaS) |
By 2050, 20% of travellers use MaaS solutions to plan their journeys |
By 2050, 50% of travellers use MaaS solutions to plan their journeys |
|
|
Public transit integration and expansion |
Past trends continue to 2050 |
Past European trends continue to 2050 for all world regions |
|
|
Land-use policies to increase urban density |
Depending on the region, either stable or slight urban sprawl to 2050 |
Depending on the region, urban densification of 5‑10% to 2050 |
|
|
Car access restrictions |
By 2050, 20% of car trips are affected by constraints (e.g. low emission zones) |
By 2050, 40% of car trips are affected by constraints |
|
|
Parking pricing |
Depending on the region, by 2050, parking prices are 0‑20% higher than the expected purchase power of travelers |
Depending on the region, by 2050, parking prices are 10‑40% higher than the expected purchase power of travelers |
|
Potentially disruptive developments |
||||
|
Autonomous vehicles |
0‑2.5% of car trips are autonomous by 2050, depending on the region |
Same as current ambition scenario |
|
|
Shared mobility |
Past trends in the supply of shared modes continue to 2050 (50‑150% of annual growth rate of shared fleet, depending on the region) |
Same as current ambition scenario |
|
|
Telework |
Depending on the region, 2‑20% of trips are affected by 2050 |
Depending on the region, 3‑25% of trips are affected by 2050 |
Figure 3.2. Projected mode shares for urban mobility, 2015-50

Note: Private refers to private motorised vehicles or taxis. Public refers to bus, metro, tram, and rail; Shared refers to motorised and non-motorised shared vehicles, including shared vehicle systems (i.e. free-floating or non-free-floating shared cars, bikes or other) and optimised shared mobility services (i.e. shared taxis, vans or minibuses with a driver); Active comprises travel undertaken by foot, bicycle, or other human-powered mode (where a vehicle is not publically shared).
Figure 3.3. Projected CO2 emissions by mode, 2015-50
Urban mobility by region
The biggest relative increase in urban mobility demand in coming decades will occur in Africa. By 2050, Africa’s urban transport will almost quadruple compared to 2015 in the current ambition scenario and the continent’s share of global urban passenger-kilometres travelled will double from 5% to 10%. Other fast-developing regions such as China and India, the Middle East and other parts of Asia will see urban mobility demand more than double by 2050 (see Figure 3.4). In China and India, mobility demand in cities will increase by around 7 000 billion passenger-kilometres from 2015 to 2050 under the current ambition scenario. This is the largest absolute increase of urban mobility demand across the globe. The volume of urban travel in China and India will increase from around one quarter of total global urban passenger-kilometres in 2015 to around a third in 2050.
Even in regions where the growth in urban passenger-kilometres is expected to be lowest, the increase will still be significant: the increase for the OECD Pacific region is projected at 30% and for transition countries at 40%.4
Figure 3.4. Projected urban mobility shares by world region, 2015-50
Local pollutants
Urban transport is an important contributor to local air pollution, principally through the emission of oxides of nitrogen (NOx), sulphates (SO4) and particulate matter measuring 2.5 microns or less (PM2.5). These pollutants contribute to severe health problems including cardiovascular and respiratory diseases and numerous cancers. The World Health Organization estimates that more than 90% of the world population lives in areas where air pollution is above the limits for healthy living (WHO, 2016[5]).
There is no necessary correlation between the contribution of urban transport activity to CO2 levels and to local air pollution. Emissions of CO2 are strictly proportional to fuel consumption of vehicles, while the quantity of local pollutants per unit of fuel in exhaust fumes can vary greatly. This Transport Outlook uses emission factors from the Roadmap model of the International Council on Clean Transportation (ICCT, 2019[6]) to estimate the emission of local pollutants resulting from the urban mobility levels of the two scenarios examined. The ICCT Roadmap includes expected improvements in vehicle efficiency standards and their probable penetration in vehicle fleets until 2050.
In the current ambition scenario, the total PM and SO4 decrease over the coming decades, while urban transport NOx emissions stay relatively stable up to 2050 (see Figure 3.5). However, cities in some world regions will still experience significant increased air pollution if policies do not focus on addressing them. The Middle East will see NOx grow by 73%, SO4 by 197% and PM2.5 by 185%. In Africa, the projected increases are 78% for NOx, 136% for SO4 and 136% for PM2.5. The rise of air pollution from transport in these regions is related to the overall growth in the size of these cities, but also to the increasing rate of motorised private transport in the respective regions. Pollutant emissions fall especially where further increases in the use of cars is limited and where vehicles are increasingly electrified, especially in the European Economic Area (EEA) and in Turkey.
The high ambition scenario demonstrates how mitigation measures could attenuate the growth of pollutants from urban mobility. The main factor that would improve the situation is the increased penetration of zero-emission vehicles compared to the current ambition scenario. Yet urban air pollution caused by transport would still increase in some cities even under the high ambition scenario. Figure 3.6 shows the difference in the urban air pollution from transport between 2050 of the high ambition scenario and the base year 2015, expressed in percent. NOx emissions would still increase in 5% of cities around the globe. The increases of SO4 and PM2.5 are geographically more limited, mainly to Africa and the Middle East.
Figure 3.5. Pollutant emissions from transport by region
Figure 3.6. Pollutant emissions from transport

Predicting the health impacts of these scenarios is difficult. The provided estimates only account for tailpipe emissions; for example, they do not include non-exhaust emissions of PM from tyre- and brake-wear. Also, transport is of course not the only contributor to local pollutants. Several other factors, such as the topography, climate and the presence of industry enter into the equation. That said, the projections for this Transport Outlook show that much of this increase will occur in cities that are already suffering from air pollution today, and in which additional emissions are likely to cause even more significant health issues. Extra effort is required to develop no- or low-emission public transport, especially in medium-sized cities where investing in rail transport may not be an option.
Box 3.2. The International Transport Forum urban access framework
Accessibility is a growing policy priority. Improving the ease with which citizens can reach goods, services or activities is increasingly recognised as the ultimate goal of transport policies, and more relevant than enhancing speed or reducing congestion. However, metrics that capture accessibility are rarely used in decision making.
Figure 3.7. Urban population in Europe without access to a hospital within 30 minutes by car

Note: Circle size corresponds to total population.
The ITF urban access framework provides a set of indicators, computing methods and databases that make possible large-scale accessibility studies (ITF, 2017[2]) .It allows for an assessment of accessibility with regard to a number of different destinations types, such as jobs, schools and hospitals. The framework also allows for analyses that isolate the influence of speed and proximity on accessibility.
In a forthcoming ITF report, Benchmarking Accessibility in Cities: Measuring the impact of proximity and transport performance (ITF, forthcoming[7]), the framework has been applied to all European cities of more than 500 000 inhabitants. The analysis showed that average accessibility to goods and services in these cities is high, but that this average value masks important disparities. For example, more than 97% of the population in the cities examined has access to a hospital in less than 30 minutes by car.
Yet in many Eastern European cities (e.g. Sofia in Bulgaria, Budapest in Hungary, or Lublin in Poland) the share is around 90%. It falls to less than 70% when considering only the residents of commuting zones. In these areas, a significant share of the urban population would need to travel for more than 30 minutes to reach a hospital. This has obvious implications for policy, considering that access to quality essential health services is an objective under the United Nations Sustainable Development Goals (SDG).
The ITF urban access framework was developed as part of a project funded by the European Commission and carried out in collaboration with the OECD’s Centre for Entrepreneurship, SMEs, Regions and Cities.
Disruption through telework
Telework and other forms of remote presence can improve accessibility, increase productivity and enhance competitiveness. Working remotely can create jobs, foster smart growth while adding to the overall well-being of employees. In terms of impact on the transport sector, telework helps to reduce the number of commuting work trips, thus alleviating traffic on transport networks during the busiest periods. To the extent it can reduce motorised trips, teleworking reduces CO2 emissions. Encouraging teleworking thus has a potential role in travel demand management strategies that aim to decarbonise transport.
Telework is broadly defined as carrying out work at a location that is remote from the employer’s site while staying connected to the office via network technologies. Telework can also encompass flexible working arrangements that shift commuting activities to off-peak hours. In the context of this analysis, however, teleworking is considered as working arrangements that reduce the total number of trips to the office.5
The concept of teleworking was first proposed as an official arrangement in the United States in 1973 as a reaction to high oil prices and in response to the Clean Air Act of 1970. Telework was initially expected to revolutionise the workplace and eventually be adopted by a significant portion of the workforce. Yet teleworking was still the exception a quarter century later, with only 7% of workers working from home at least once a week in the United States in 1997 (ILO-Eurofound, 2017[8]). In the European Union (EU), only 5% of the employed population were working remotely at least a quarter of their working hours in 2000 (Eurofound, 2010[9]).
The prevalence of telework has since increased, largely due to the rise of the internet and mobile technology and the increasing social acceptability among both employers and employees. In 2010, nearly 10% of the employed population in the United States worked remotely at least once a week, and teleworking constitutes the fastest-growing commuting pattern. Growth in telework activity has been highest among urban populations (Mateyka, Rapino and Landivar, 2012[10]).
The lack of a harmonised definition and data collection practices regarding teleworking make cross-country comparisons somewhat difficult. Nevertheless it is possible to identify some general patterns and trends from the available data.
First, large variations in the prevalence of teleworking exist. Telework shares worldwide range from 2% to 40%, depending on the region and the sector (Gschwind et al., 2017[11]). In Europe, teleworking rates are highest in Denmark, Finland, and the Netherlands, where approximately 34%, 32%, and 29% of the population report teleworking. Across all countries, the prevalence of teleworking tends to be highest among highly-skilled employees such as managers, professionals, technicians (ILO-Eurofound, 2017[8]). Figure 3.8 reports the teleworking rates within the EU.
Figure 3.8. Workforce working at home several times per month, 2015
Relatively low rates of teleworking are found in southern Europe, with 10.1% teleworkers in Greece, 11.3% in Spain and 14% in Italy (ILO-Eurofound, 2017[8]). Germany also has a comparatively low proportion of teleworkers, with only 10.8% of the employed population working from home several times a month. Self-reports from a global survey of knowledge workers in the Asia-Pacific and Africa-Middle East regions suggest low rates of teleworking relative to most high-income countries (PGi, 2015[12]).6
Table 3.2. Teleworking rates in selected non-EU countries
Country |
Group |
Year |
Teleworking rate (%) |
---|---|---|---|
Argentina |
All workers |
2011 |
2.0 |
India |
Workers in non-agricultural organised sector |
2015 |
19 |
Japan |
All workers |
2010 |
16.5 |
United States |
All workers |
2015 |
24.1 |
Canada |
All workers |
2015 |
12.8 |
Note: In India, the non-agricultural organised sector represents approximately 15% of all workers in the country ILO-Eurofound, (2017[8]). As a result, the estimated teleworking rate among all workers in India is likely to be lower than the figure reported.
Source: National reports compiled in Eurofound (2017[8]), unless otherwise noted; Data for Japan obtained from the Ministry of Internal Affairs and Communications (2011[13]); Data for the U.S. Bureau of Labor Statistics (2016[14]) ; Data for Canada obtained from Statistics Canada (2016[15]); Data for Australia obtained from MIAESR (2013[16]).
What drives the decision to telework?
Teleworking requires a compatible assignment, i.e. tasks that can be executed using remote technologies. It also requires the availability of the relevant equipment, the literacy to operate it and an adequate working environment at the remote location (with internet access and a physical space to work). Finally, a formal or informal agreement between employee and employer regarding teleworking activity is essential.
A number of additional factors influence employees’ propensity to telework and the frequency with which they operate remotely. Sociodemographic characteristics, attitudes, and geographical accessibility are further determinants of the propensity to telework. Women, workers on high incomes and employees with advanced education levels tend to telework more than other groups; another one is employees in households with children telework (Walls, Safirova and Jiang, 2006[17]; Singh et al., 2013[18]; Loo and Wang, 2018[19]).
The propensity to telework correlates positively and consistently with the length of commutes (Helminen and Ristimäki, 2007[20]; Melo and de Abreu e Silva, 2017[21]). A causal relationship is not clear, however. Employees who have longer commutes may be more likely to telework, but equally the opportunity to telework might lead employees to choose their residence farther away from their place of work (Melo and de Abreu e Silva, 2017[21]). In reality, this association is likely to reflect a combination of these two effects.
Teleworking is subject to regulations set by governments. It is also informed by cultural norms. National differences in the legal framework and societal attitudes partly explain the wide range in teleworking rates across countries. A culture of “presenteeism” – the pressure to be physically present at work – can constitute a formidable disincentive to telework (Wilton and Scott, 2011[22]).
The attitudes of management play an important role in the shaping of acceptance of teleworking within an organisation (Haddad and Chatterjee, 2009[23]; Mayo et al., 2016[24]). Managers may be reticent to support teleworking for fear of a detrimental impact on employee engagement and company culture. They may also be averse to losing direct managerial control over the time of teleworking employees. This cautious attitude characterises managerial perspectives on telework in China and India, for instance – countries where the potential benefits from higher level of teleworking are quite significant. Some analyses suggest that these concerns are, on average, unfounded (Gajendran and Harrison, 2007[25]).
A recent study of Chinese workers also found that teleworking increased productivity and thus constitutes a profitable management strategy (Bloom et al., 2015[26]). Evidence also suggests that increasing the visibility of public sector involvement in teleworking can have a positive effect on telework activity (Mokhtarian, 1991[27]). A number of governments around the world have introduced legislation or resolutions aiming to make teleworking more feasible for their citizens, including China, the Czech Republic, Colombia, Japan, and Romania.
A specific aspect in this context is the emergence of the so-called gig economy: independent workers who string together short-term contracts that they fulfil via offline or online activities. Approximately 31% of US workers are self-employed in the gig economy, most of them in addition to other forms of employment, according to US Federal Reserve estimates. About half of these (16%) are involved in on-line gig work such as fulfilling task-based contracts remotely.7 If the latter group increasingly substitutes full-time office jobs, telepresence could reach levels that reduce the amount of work-related travel. Linked to this development is the rise in off-site office spaces such as WeWork that cater to gig-workers. Few statistics exist on the size and nature of the gig economy, but such work arrangements may in the future have a growing impact on travel demand, especially where they involve tasks being completed remotely.
How does teleworking change travel behaviour?
The main impact of teleworking on transport is to reduce travel demand during peak hours. This alleviates congestion and reduces air pollution, helps to avoid traffic crashes and eases pressure on public transport infrastructure.8
Empirical evidence indicates that teleworking can reduce traffic volume by as much as 2.7% (Choo, Mokhtarian and Salomon, 2005[28]; O’Keefe et al., 2016[29]; Giovanis, 2018[30]). The magnitude of any impact of teleworking is highly dependent on the context. The degree to which teleworking reduces emissions, for instance, depends on factors such as commuting, climate, and induced energy use patterns, as well as the characteristics of the office and remote/home space, as well as the dominant electricity mix (Kitou and Horvath, 2003[31]). In areas with high levels of public transport use, for example, CO2 reductions from teleworking will likely be lower compared to areas where vehicles with internal combustion engines are the dominant choice for commuting.
The net impacts of telework can be ambiguous. This is especially the case when its indirect, often behavioural impacts are taken into account. Evidence indicates that teleworking may go along with an increase in the number of trips that are not work-related (Kitou and Horvath, 2003[31]; Glogger, Zängler and Karg, 2008[32]; Falch, 2012[33]; Zhu et al., 2018[34]). Other studies show that telework is positively associated with increased frequency of non-urban trips and increased household energy use (Kitou and Horvath, 2003[31]).
Such indirect effects of teleworking taken together can create rebound effects, i.e. actually increase total travel volume and limit any overall positive impact of teleworking on transport demand and emissions e.g. (Melo and de Abreu e Silva, 2017[21]). Telework could even contribute to more urban sprawl, to the extent that it prompts workers to locate their homes farther away from the office (Nilles, 1991[35]). Evidence regarding the impacts of teleworking in the literature is also highly influenced by the type and intensity of teleworking activity considered (e.g. one day per week vs. three days per week), and as such, should also be taken into account when reviewing the empirical evidence for its impacts (Ben-Elia, Lyons and Mokhtarian, 2018[36]).9
Attitudes towards teleworking are increasingly positive, according to survey results. More than 50% of teleworkers polled in all regions said they would like to work remotely more often (PGi, 2015[12]). At the same time, the further evolution of various technologies will make it easier to telework and could thus stimulate the mainstreaming of telework in significant ways.
On the other hand, improved public transport could reduce the appeal of teleworking by shortening commutes and making them more agreeable. Greater fuel efficiency of internal combustion engines and advances with electric vehicles (such as better charging infrastructure, greater range of cars) could also reduce cost-related incentives to telework. Although the value of teleworking in terms of reducing CO2 would decrease as the transport sector decarbonises, cities would continue to benefit from reduced congestion.
Simulation results
The ITF urban passenger model was used to run a scenario simulating the impact of increased telework activity on transport demand. The effects of teleworking are estimated according to the impacts documented in the available literature. They are assessed in comparison to current ambition scenario, in which teleworking takes up only at a very moderate pace. In the scenarios that simulate a disruptive development pathway for transport, telework affects between 3% and 30% of urban trips by 2050, depending on the region. The simulation results indicate that the increased uptake of teleworking leads to a decrease in global urban passenger-kilometres and related CO2 emissions of around 2% compared to the current ambition scenario in 2050, indicating that the rebound effect does not lead to an overall increase in transport demand.
Massive shared mobility
The rise of the sharing economy is one of the most remarkable disruptions in recent years, both inside and outside of the transport sector. In the sharing economy, people seek to maximise the utility of under-used assets by linking supply and demand directly, usually via an online platform. The sharing economy leverages ubiquitous digitalisation for better service, more efficiency and new business models that have the potential to drastically change conventional transport systems.
A wide range of shared and quasi-shared mobility services are already available today. They include round-trip or free-floating carsharing, private short-term car rentals managed through app-based platforms, ridesourcing services for single-occupancy or shared trips, on-demand mini-bus services with flexible routes, peer-to-peer ride-sharing services, as well as bicycle and micromobility sharing (see Table 3.3).
Table 3.3. The evolving urban passenger mobility landscape
Travel modes |
Mobility applications |
Service models |
Operational models |
Business models |
---|---|---|---|---|
Self-owned car/bike/other |
Business-to-consumer sharing apps |
Ownership |
Station-based roundtrip |
Business-to-consumer services |
Taxi |
Mobility tracker apps |
Membership-based service models |
Station-based one-way |
Government-to-consumer services |
Rental car |
Peer-to-peer sharing apps |
Non-membership service models |
Free-floating one-way |
Business-to-government services |
Public transport |
Public transport apps |
Peer-to-peer service models |
Business-to-business services |
|
Bikesharing |
Real-time information apps |
For-hire service models |
Peer-to-peer mobility marketplace |
|
Carsharing |
Ridesourcing apps |
Public transport services |
Fractional ownership |
|
Microtransit |
Taxi e-hail apps |
|||
Rickshaws |
Trip aggregator apps |
|||
Personal vehicle sharing |
||||
Ride-sharing |
||||
Ridesourcing |
||||
Micromobility sharing |
||||
Scooter sharing |
||||
Shuttles |
Note: Bikesharing refers to on-demand access to bicycles at a variety of docked or free-floating pick-up and drop-off locations for one-way (point-to-point) or roundtrip travel; Carsharing offers users access to vehicles by joining an organisation that provides and maintains a fleet of cars and/or light trucks; Microtransit refers to privately or publicly operated, technology-enabled transit service that typically uses multi-passenger/pooled shuttles or vans to provide on-demand or fixed-schedule services with either dynamic or fixed routing; Rickshaws refer to for-hire services in which a driver transports users in a motorised or human-powered light vehicle containing three or more wheels and a passenger compartment; Personal vehicle sharing refers to the sharing of privately-owned vehicles, where companies broker transactions between vehicle hosts and guests; Ride-sharing refers to the formal or informal sharing of rides between drivers and passengers with similar origin-destination pairings (e.g. carpooling and vanpooling); Ridesourcing refers to prearranged and on-demand paid transportation services in which drivers and passengers connect via digital applications; Micromobility sharing provides individuals paid access to a fleet of (mostly) electric micromobility devices such as push scooters deployed and maintained by an operator; Scooter sharing provides individuals paid access to a fleet of moped/scooters deployed and maintained by an operator; Shuttles refers to shared vehicles (typically vans or busses) that connect passengers from a common origin or destination to public transit, retail, hospitality, or employment centres.
Source: Adapted from SAE (2018[37]).
These services exploit current technologies to expand the spectrum of mobility options available to individuals. Propelled by the development of Mobility as a Service (MaaS) platforms, they are also becoming more integrated with standard public transport. This is the case especially when shared mobility can feed efficient, high-capacity public transit services. At the heart of this trend is the continued growth and infiltration of digital technology in all aspects of the economy and human lives.
Why do people choose shared mobility?
Understanding the differences in land use patterns, transport supply and culture is important to appreciate how travel patterns may change with the massive adoption of shared mobility services and the replacement of private mobility by them. The layout of land use in cities determines the need for motorised mobility and average travel distances. Density and land use mixtures but also the commuting structure of a metropolitan area may set the city mobility profile. Another key element is transport infrastructure and public transport services provision.
A variety of elements shape the mobility and accessibility ecosystems in cities, and these ecosystems in turn influence car ownership rates and resulting transport mode choices. A higher density and smaller size can lead to higher shares of non-motorised travel in a city, but poor public transport and urban sprawl can also create conditions that encourage car-oriented mobility. The significant presence of bus networks in some cities indicates that some travellers do currently use this alternative over private vehicles, due to either financial constraints or personal choices regarding car ownership. In this way, a number of city characteristics (e.g. mode shares, the quality and extent of public transit services, socio-economic characteristics) are important in assessing the comparative advantage of shared mobility relative to existing transport options. For example, focus group studies carried out by the ITF show that citizens in Finland’s capital Helsinki are specifically looking for services that connect different outer areas of the city with each other (ITF, 2017[38]). In the Irish capital, Dublin, shared services could be useful as feeder services to public transport for residents in suburban areas (ITF, 2018[39]).
The choice of ridesourcing is often linked to parking prices and availability and a desire to avoid drink-driving. In the United States, the choice of ridesourcing versus public transport, cycling or walking is often linked to greater convenience and comfort. The use of ridesourcing services tends to peak in the morning and (even more so) in the (late) evening. These peaks coincide with increased traffic and therefore contribute further to already existing congestion. Trust, cost and ease of use (encompassing payment, waiting time, software interface, etc.) are also factors that conceivably influence the uptake of various shared mobility options. By building trust and enhancing the ease of use, mobile apps and MaaS platforms play a fundamental role in promoting the uptake of shared mobility.
What are the implications of shared mobility use for urban transport?
The rise of shared mobility services has led to a debate in cities around the world about how they should be regulated and how cities should interact with the players (e.g. taxis). The discussion also revolves around safety impacts of shared mobility services and their influence on travel behaviour. Some studies suggest that shared services help reduce vehicle ownership and increase use of public transit. Others find that the early adopters of these services are unsatisfied public transport users who add traffic to already congested streets by switching to shared vehicles. Furthermore, the short- and medium-term effects of shared mobility on mode choice and car ownership may not compensate for the possible long-term effect of greater urban sprawl if counteracting policies are not put in place.
A number of studies have explored the impact of shared mobility penetration on the market for urban mobility. Many focus on the observed effects of transport network companies (TNCs) and on environmental performance indicators (Shaheen et al., 2017[40]). Others are more prospective in nature, either using simulation-based experiments to assess the future adoption of shared mobility solutions at a large scale (Ciari, Schuessler and Axhausen, 2013[41]; Spieser et al., 2014[42]; Liu et al., 2017[43]; Zachariah et al., 2014[44]; Fagnant and Kockelman, 2016[45]), or by offering expert assessment of aggregate impacts of massive shared mobility adoption in different urban contexts (Shaheen et al., 2015[46]; Clewlow and Mishra, 2017[47]; Ronald et al., 2017[48]; Fulton, 2018[49]).
Most studies have examined the observed impacts of carsharing, ride-sharing and new TNC services on mode choice, changes in total motorised mobility (e.g. vehicle-kilometres travelled) and changes in car ownership. Some positive effects have been noted, such as modest shifts away from private car use, lower car ownership rates and increased public transport use (Shaheen et al., 2017[40]). Yet a significant share of users switch to shared mobility from public transport or active transport modes for some of their medium-distance trips. Additionally, the greater accessibility engendered by shared mobility services may aggravate urban sprawl if leads residents and business owners to locate farther away from city centres. Shared mobility systems have also been shown to lead to additional travel in some cities with large vehicle fleets (Bliss, 2017[50]; Bliss, 2017[51]). In these cases, policies may need to be put in place to ensure that shared mobility provides benefit (Karim, 2017[52]).
The impact of shared mobility on total vehicle-kilometres travelled, congestion, and emissions depends on average occupancy rates and the efficiency of the vehicle fleets in operation (ITF, 2016[53]). If larger shared vehicles are used, producing average occupancy rates greater than six passengers, the benefits of shared mobility are much greater (Alonso-Mora et al., 2017[54]; ITF, 2017[55]).
A number of simulation-based studies on the potential impacts of shared mobility services with high occupancy rates have been carried out by the ITF for a number of different cities including Lisbon and its metropolitan area in Portugal (ITF, 2015[56]; ITF, 2016[53]; ITF, 2017[55]), the metropolitan area of Helsinki in Finland (ITF, 2017[38]), the metropolitan area of Auckland in New Zealand (ITF, 2017[57]), the Greater Dublin Area in Ireland (ITF, 2018[39]) and the metropolitan area of Lyon, France (ITF, forthcoming[58]). These studies explored the impact of different levels of shared mobility uptake that displaces private vehicle trips and low-frequency bus services. Overall, the results show that the extent of the positive impacts of shared mobility in urban centres depends in large part on the characteristics of the cities studied, as well as on the specific aspects of the design of the shared mobility systems considered.
The results also indicate that densely populated cities that are well-connected by public transport are likely to be more fertile ground for the development of shared mobility solutions than cities characterised by sprawl and lower public transport connectivity. However, less dense cities arguably stand to gain significantly from affordable shared mobility services that feed public transport lines. In a scenario in which all private car use is replaced by the massive uptake of shared mobility in conjunction with existing public transport systems, vehicle-kilometres and CO2 emissions are reduced by 30% to 60% compared to current mobility patterns. Lower uptake of shared mobility, such as that which replaces 20% of private car use in some cases reduces vehicle-kilometres by more than 10%. Such relatively modest adoption levels seem plausible, based on feedback received from potential users participating in stated preference surveys and focus groups in some of the cities studied.
Overall, the existing evidence indicates that current, decentralised shared mobility providers do not significantly reduce total vehicle-kilometres travelled in cities. Indeed, the current trend in shared mobility has, by and large, only changed the face of personal transport and even reduced public transit ridership (Graehler, Mucci and Erhardt, 2018[59]). Shared mobility solutions have three observed benefits: Firstly, they can encourage the use of more efficient vehicle technologies. Secondly, they can reduce the fleet of private cars that consume urban space when parked. Finally, shared mobility services can also provide inter-urban transport. In some markets, these solutions by operators such as France’s BlaBla Car make up 5% of interurban travel. However, shared inter-urban trips are unlikely to replace private trips entirely; more likely is that their share will stay below 50% (Shaheen, Stocker and Mundler, 2017[60]).
How shared mobility continues to develop will depend on regulatory frameworks and on its relationship with public transport. Existing public transport operators may see shared mobility as a potential substitute for their own services, rather than a complement, although this is changing rapidly. Another challenge from the introduction of shared mobility is a potential fall in mobility costs and a related increase in urban accessibility. Urban and regional planning will need to address such effects of shared mobility in order to avoid urban sprawl. Policies that favour densification and extensive coordination with public transport networks are paramount (ITF, 2017[55]).
The significant potential gains of shared mobility will not materialise unless appropriate regulations are in place regarding empty trips, fare structure, integration with public transport and also the design of the dispatch algorithm that allocates rides to riders. Failing these, increased congestion and additional CO2 emissions, not less, may be the outcome (Shaheen et al., 2015[61]; Santi and Ratti, 2017[62]).). The interaction of shared mobility with electric mobility and self-driving technologies can even potentiate the penetration of services at lower costs and accelerate the decrease in car ownership rates (Fulton, 2018[49]).
Box 3.3. Mobility as a Service
Urban mobility is typically provided by a patchwork of poorly-optimised and disconnected service providers operating with little coordination on both public and private infrastructure. Urban mobility ecosystems are evolving with respect to new forms of transport modes. But the ways in which users’ access and pay for mobility are also changing, as are the service models, operational approaches and business cases models that deliver it (Table 3.3).
The large-scale diffusion of ubiquitous sensing devices, portable, remote and edge computing capabilities, IT infrastructure, new data treatment and analysis protocols, data-fed algorithms and wide-spread, fast, reliable and robust communication networks all lead to an unprecedented revolution in the way in which transport stakeholders can optimise multiple and converging goals and outcomes. At its core, the concept of MaaS supports the digital joining-up of different transport, information and payment services into a smooth and reliable customer-facing experience.
Digitalisation holds great promise to break independent silos of separately regulated services in the transport sector and deliver mobility not as a discrete transaction based on a single operator or mode but as a continuum of services that reliably allow travellers to meet their access needs and desires – in other words, Mobility as a Service (MaaS). Globally, non-OECD regions account for the dominant share of these new services, with China accounting for 68% of the global on-demand mobility market (with bikesharing slightly dominating ridesourcing in that country). A main driver for the uptake of new mobility services and MaaS will be the enabling legislation and standardised data protocols that ensure seamless linking of service operators, trips, payment options and regulatory reporting (Yanocha, 2018[63]).
The potential impacts of MaaS depend on the business models that are embedded into MaaS offers, as well as the policies that public authorities put in place (or not) to influence the way in which people modify their behaviour (or not) in response to the new offer. Overall, transport-related impacts are also likely to evolve in intensity and at different levels of MaaS penetration and uptake with transitional effects potentially higher than at advanced adoption levels. Potential impacts could be seen on congestion, energy use, CO2 and traditional pollutant emissions, safety/health, and land use/real-estate market effects.
Insofar as MaaS systems facilitate the adoption of shared mobility services, they have had significant impacts on urban transport systems and will continue to do so. There is common ground among existing studies that the early uptake of ridesourcing services leads to modest substitution effects for car travel with more riders coming from public transport, walking and cycling. This finding is, however, variable between and within urban areas and is likely linked to the quality and frequency of existing public transport services and lack of safe cycling environments. Car-trip substitution effects appear to be greater in medium-sized cities and peripheral areas. The role of centralised dispatch in reducing or eliminating these impacts has been demonstrated in a series of modelling studies, although such a system has not yet been commercially deployed (ITF, 2015[56]; ITF, 2016[53]; ITF, 2017[38]; ITF, 2017[57]; ITF, 2017[55]). Other evidence suggests that carsharing households tend to own fewer cars than other like households, use public transport more often, and live in urban areas where alternatives to car travel exist (Shaheen et al., 2017[40]).
The impact of other forms of shared mobility has not been studied as extensively. Bikesharing and micromobility-sharing may lead to a switch from certain short-distance car trips in some contexts, especially where car use dominates. Where high-quality and cost-effective public transport is available, bikesharing and micromobility-sharing can serve as feeders to public transport, but often replacing walking. Where public transport is infrequent or of low quality, these modes may substitute for public transport.
These early findings may evolve – especially in an environment where many of these services are actively linked to provide low-latency, affordable, convenient and highly reliable trips. They may also be influenced by public policies in support of public transport use and active mobility.
Simulation results
The impact of massive shared mobility will heavily depend on the regulatory framework that accompanies it. As such, two scenarios were developed and tested:
In a first scenario, shared modes develop at twice the speed of past trends and the regulatory framework is loose. This leads to the increased uptake of shared vehicle systems, such as shared cars or bikes that are often used by single individuals. This encourages low vehicle occupancy rates and does not incentivise the use of public transport. To the contrary, such shared vehicle systems encourage public transport users to switch to individual means of transport. The use of private cars may decrease, but low occupancy rates of shared vehicles would not lead to a drop in overall vehicle-kilometres and the associated externalities.
In a second scenario, shared modes also develop at twice the speed of past trends, but strong regulations ensure that shared modes are optimised through supporting MaaS solutions. These encourage the use of shared mobility as feeder services for more traditional public transport systems, such as bus or rail systems. Vehicles such as minibuses or vans with drivers operate on fixed routes with fixes schedules, feeding higher capacity public transport services. They run only a very limited amount of empty vehicle-kilometres. In this scenario, people would give up the use of their private car to opt for such more efficient transport options, defined by high vehicle occupancy rates.Table 3.4 summarises the simulation results for both scenarios in comparison to the current ambition scenario (where shared modes develop more moderately, i.e. in line with past trends). Where an increased uptake of shared mobility modes is accompanied by loose regulations, vehicle-kilometres would lead to an increase of 6% by 2050. Shared vehicle systems (such as shared cars or bikes) would take mode share from two- and three-wheelers. A lack of integration of conventional public transport with other modes would result in an increase in private car use. As a result, transport CO2 emissions increase by 18% by 2050 compared to the current ambition scenario.
Table 3.4. Projected impact of two shared mobility scenarios
Percentage change compared to current ambition scenario
Shared mobility scenario |
Passenger-kilometres |
Vehicle-kilometres |
CO2 emissions |
|||
---|---|---|---|---|---|---|
2030 |
2050 |
2030 |
2050 |
2030 |
2050 |
|
Loose regulation (Further uptake of shared mobility dominated by traditional shared modes with low occupancy rates) |
6 |
5 |
5 |
6 |
15 |
18 |
Strong regulation (Increased uptake of optimised shared services with high occupancy rates, supported by Mobility as a Service solutions] |
1 |
-4 |
-24 |
-51 |
-3 |
-34 |
On the other hand, in a scenario where an accelerated uptake of shared modes is accompanied by strong regulation, the increased shared mobility demand is met by optimised shared mobility services that rely on MaaS solutions and are well-integrated with public transport. This leads to significant reductions in vehicle-kilometres and hence CO2 emissions. Total vehicle-kilometres could be reduced by more than 50% thanks to high vehicle occupancy rates and a decrease in the use of private cars, resulting in CO2 reductions of more than 30% by 2050 compared to the current ambition scenario.
Autonomous vehicles
Automated driving systems that either assist or replace humans in the driving task are rapidly being designed, tested and, in many cases, deployed in pilots around the world.10 Vehicle automation can be partial, when the automated system of the vehicle can conduct some parts of the driving tasks. It can also be full, when the vehicle can perform all driving tasks under all conditions (geographic area, roadway type, traffic, weather, events/incidents) that a human driver could. In this latter case, the vehicle is said to be autonomous.
Some low-level and context-specific automation functions such as self-parking, lane-keeping and automated traffic jam driving are already deployed in commercial vehicles, both passenger and freight. All major automotive original equipment manufacturers (OEM) are working on integrating higher and higher levels of automation into their products.
Though there are clear industrial policy and market leadership outcomes with the deployment of successful automation technologies, much of the stated motivation for introducing automation is related to the potential road safety benefits this might deliver. The safety performance of automated vehicles, especially in relation to human-driving will both drive and condition its uptake.
The potential for automated vehicles to remove common and pernicious human errors and misjudgements from the driving task is significant (Fagnant and Kockelman, 2015[64]; Anderson et al., 2016[65]). However, it is reductionist to believe that human error has been properly identified as a contributory factor by those responsible for post-crash forensic investigation, or that all crashes involving human error could have been otherwise avoided by addressing that error.
A second aspect to consider when assessing the scope for automation to improve safety outcomes by removing “human errors” in crash causation is that it does not follow that all crashes attributed to human error could have been reasonably avoided by drivers (Noy, Shinar and Horrey, 2018[66]). How much automation will improve road safety ultimately depends on how safely automated driving systems can carry out the parts of the driving task they are assigned. The technical skill with which these systems are able to handle the driver task without errors, glitches or unintended outcomes will matter here.
When considering the impacts of vehicle automation, it is important to bear in mind that not all automated systems share, or are targeting, the same performance. The capabilities of these systems vary from simply assisting drivers in certain contexts and in limited capacities to fully replacing the human driver in specific contexts. The former form the basis of a number of technologies already included in commercially available cars and trucks (e.g. lane assist, self-parking functions, limited autopilot function) whereas the latter only comprise vehicles that are being tested in various trials.
Figure 3.9 sets out the five levels of automated vehicle performance as defined by the International Society of Automotive Engineers (SAE, 2018). It categorises vehicle automation functions according to the level and scope of driving task responsibilities allocated to the human driver versus the automated system – or to both in some instances. A core issue with regards to safety is how well the handover from automated systems to human drivers occurs at SAE levels 2 and 3 when the system cannot interpret its environment satisfactorily. Machine-to-human handovers also are triggered at SAE level 4 when the driving context changes to one that is beyond the capabilities of the automated system. The SAE levels are useful in developing a complete taxonomy of automated driving system capabilities and functional boundaries. Yet, fundamentally, only two dimensions matter (ITF, 2015[67]; Noy, Shinar and Horrey, 2018[66]):
Does the automated system seek to assist or replace the driver, i.e. is automation partial or complete?
Does the automated system operate part of the time in some contexts or everywhere at all times?
Figure 3.9. Society of Automotive Engineer’s five levels of automated driving performance
Figure 3.10 depicts the categorisation of automated driving systems according to the degree and duration of the automated functions.
Figure 3.10. Two-dimensional categorisation of automated driving systems
The ability for advanced driver assistance systems to offer partial automation part of the time is already quite advanced at present (e.g. quadrant 1: lane-keeping, automatic speed control) and the capability for systems to provide partial automation in all contexts is developing (e.g. quadrant 2: lane keeping assist, autonomous cruise control and lane-change capability, etc.). The technologies that allow full automation part of the time and in certain contexts is nascent but developing (e.g. quadrant 3: motorway autopilot or traffic jam assist). A significant gap exists, however, between those systems and ones that could completely replace human drivers in all contexts and at all times. In quadrants 1 through 3 just as in SAE levels 1‑3, humans retain a significant role as driver, back-up driver and/or supervisor of the automated system. This hybridisation of roles poses inherent safety challenges (ITF, 2018[69]).
At the core of all levels of automation is the ability for automated vehicles to perceive (“sense”) their environment, process this information to determine what is relevant to safely carrying out the allocated driving task (“plan”), decide on a course of action, successfully carry out this action by triggering actuating systems such as steering, braking, signalling (“act”) and then assess the result of the action carried out (“assess”) (Parasuraman, Sheridan and Wickens, 2000[70]). Each of these steps mimics how humans drive but, with different capabilities and at different execution speeds.
Technology is now reaching the point where the fusion of different sensors, processing systems and actuators can replicate and in some cases improve on human driving performance. This convergence is at the heart of the incipient revolution promised by highly- and fully-automated driving. But the convergence is not complete and there remain key areas where automated driving systems still lag behind the capabilities of the average human driver.
Humans still retain an advantage over single sensor-based automated systems when it comes to reasoning and anticipation, perception and sensing when driving. Overcoming this gap (and only in certain conditions) requires multi-sensor fusion on the part of the automated system. This strategy is commonly employed on various vehicle testbeds deployed in current trials. Even in the case of multiple sensor fusion, human capabilities still outperform that of automated systems in certain problematic and complex contexts. Some common traffic scenarios still confound automated driving system capabilities. Correct identification of bicycle orientation and anticipation of cyclist trajectories are also problematic. (NHSTA, 2017[71]; Schoettle, 2017[72]).
The risk stemming from these and other dangerous scenarios can potentially be mitigated by augmenting embarked sensing capabilities with inputs from other vehicles and infrastructure. The need to move from a “reactive” safety paradigm where vehicles rely solely on their embarked capabilities to a “proactive” safety framework where vehicles are embedded in a communicative network to deliver better safety outcomes is actively debated. Connected automated systems that can “see” what humans cannot (e.g. beyond line of sight) and relay this information to each other show promise for surpassing human driving capabilities. But the communicative car strategy is one that is not void of new risks and challenges – especially as concerns cyber-security risks (ITF, 2018[69]). Further, beyond correct perception and decision-making functions, the issue of the regulatory validation of system performance to determine legal road-worthiness remains challenging (Stolte et al., 2016[73]).
What makes people choose to use autonomous vehicles?
There are a number of drivers for increased automation, not all of which are aligned to support rapid deployment trajectories. Furthermore, these factors will impact not only the overall uptake of highly automated, and eventually fully autonomous, vehicles, but whether individually owned or fleet-owned and operated deployment pathways will dominate.
Safety performance, as noted, is a strong driver and one that is likely to increase if future automated driving systems are successfully able to handle a broader and broader range of operational contexts. The few automated vehicle crashes that have occurred to date, however, have tempered the attractiveness of automated vehicles in certain regions. In other regions with lower levels of motorisation (and of personal driving experience), acceptance rates for automated vehicles are much higher, for instance in China.
Acceptance may be linked to the perception and expectation for driving performance. In individually-owned automated car scenarios, passengers may be wary of systems that they perceive to drive differently than they themselves do – even if these are demonstrably safer. If, on the other hand, fleet-based commercial deployment dominate, expectations and acceptance of safe driving behaviour may be greater. Much as passengers may wish to be driven by “safe” taxi drivers, they may also wish to be driven by “safe” automated driving systems.
Increased accessibility may be an important driver of growth – especially for fleet-based systems that improve overall accessibility options. Accessibility can be especially improved for disabled, elderly and young passengers who do not have driving license and currently are using conventional public transport. Robustness to cyber-security threats will matter – especially if deployment pathways are conditioned on greater and greater connectivity.
Cost will condition the uptake of automated driving systems. The potential reduction of parking costs and the ability for former drivers to engage in other activities while being driven may increase the attraction and uptake of highly automated vehicles, especially for better-off households who already disproportionately contribute to overall vehicle-kilometres travelled. However, higher unit technology costs will likely act to contain the deployment rate of individually-owned automated vehicles – at least at the outset.
Partly because of this cost-constraint, many companies planning to deploy highly automated vehicles are planning to do so in the form of ridesourcing fleets (e.g. Waymo, Renault-Nissan, Ford). The potential of automation to reduce heavy goods vehicle and delivery van operating costs is also high and suggests the road freight sector may be one of the first to fully automate (ITF, 2015[67]; ITF, 2017[74]). Uptake in this sector may be accelerated by the scarcity of qualified human drivers against a backdrop of increased road freight demand.
Perhaps the greatest driver will be the regulatory framework deployed around automated driving. This is an area of great uncertainty, as it is itself linked to the still-developing understanding of the safety and traffic impacts of the technology. Permissive testing regimes as enacted by many jurisdictions today do not necessarily pre-figure permissive homologation and licensing regimes. Further, a number of regional and city transport authorities have indicated willingness to put in place constraining regulatory regimes given the potential for automated driving systems to exacerbate traffic congestion and city sprawl. If, how and under what form these regimes are put into place, and their robustness to legal challenges, remains unknown at present.
What impacts do autonomous vehicles have on urban transport?
As with any technology, vehicle automation will bring benefits but will also have negative impacts. Many benefits and negative impacts are foreseeable and the former should dominate the latter in any deployment scenario. However, the deployment of such a new and disruptive technology will also have unforeseeable benefits and negative impacts – the balance of which is uncertain but likely in favour of the former.
One of the key uncertainties in relation to the large-scale deployment of automated vehicles relates to the impact on overall vehicle-kilometres travelled, congestion and substitution effects with public transport and active mobility.
In uptake scenarios that are characterised by individual use of highly automated vehicles, the vehicle-kilometres travelled may well increase – significantly in certain cases. Wadud, MacKenzie and Leiby (2016[75]) estimate that the increase in annual vehicle-kilometres due to induced demand from underserved user groups (youth, elderly, disabled) will be between 2% and 10%. Harper, Hendrickson and Samaras (2016[76]) suggest that the upper bound of that increase will be equal to 14%, while Brown, Gonder and Repac (2014[77]) estimate a much higher increase of 40%. Childress et al. (2015[78]) arrive at a 20% increase in vehicle-kilometres travelled, assuming 30% larger road capacity, 65% lower value of travel time, and 50% decrease in parking costs. Schoettle and Sivak (2015[79]) put the increase in annual vehicle-kilometres at 75% and consider a 43% reduction in vehicle ownership. For Fagnant and Kockelman (2015[64]), the increase in vehicle-kilometres travelled depends on the market penetration rate of autonomous vehicles. At a 10% market penetration rate, they calculate a 2% increase in vehicle-kilometres, while at a 90% penetration rate vehicle-kilometres grow also by 90%.
Gruel and Stanford (2016[80]), using a system dynamics approach showed that in all the scenarios they considered, v-km is likely to increase, leading to a potential increase in energy consumption and emissions in total. The order of magnitude of the increase differs significantly across the scenarios. In the scenario assuming that the mode choice is not affected autonomous vehicles bring mostly benefits. In the scenario of highly increased attractiveness of travelling by car the v-km travelled grow significantly, with corresponding growth of congestion level and emissions, and urban sprawl. In the sharing scenario car ownership decreases but the vehicle-kilometres grow even more due to the reallocation trips. Other studies also point to vehicle-kilometre increases in fleet operation of fully automated ridesourcing services (WEF/BCG, 2018[81]). Fleet-based deployment of automated vehicles may lead to impacts similar to those seen in conjunction with the uptake of ridesourcing services – generally an increase in vehicle-kilometres travelled in early phases of deployment (ITF, 2018[39]). This impact may be mitigated over time if a large share of individual car users switches to these systems – especially in conjunction with public transport or active transport.
However, the ITF (2015[56]; 2016[53]; 2017[55]) in its simulation studies on shared mobility showed that if there is a centralised dispatcher optimising the reallocation of the shared vehicles, the number of vehicle-kilometres travelled may not grow. Parking spots and depots for idle vehicles will be needed across the city in this case.
The link between vehicle-kilometres and congestion impacts of highly automated driving depend on three main factors (Anderson et al., 2016[65]). All else equal, an increase in vehicle-kilometres might mechanically increase congestion levels. The potential reduction in road crashes and their severity will lead to lane closures and delays, increasing overall traffic flow reliability. Congestion may be further reduced, all else held equal, due to more even traffic flow and optimised speed performance (Simonite, 2013[82]). Tientrakool, Ho and Maxemchuk (2011[83]) estimated in their study that use of autonomous vehicles can increase road capacity by 273%. The potential for improved junction throughput with automation is also significant (Tientrakool, Ho and Maxemchuk, 2011[83]). As with traditional congestion-reduction efforts, this potential is only realised if it is locked-in with demand management techniques such as pricing that reduce or eliminate the induced traffic effect (ITF, 2018[39]).
Wide scale deployment of autonomous vehicles can release parking spaces in city centres for other needs. However, improved accessibility and possibility for the driver to perform other activities instead of driving might stimulate urban sprawl and growth of suburbs with decrease of the population density of metropolitan areas. This, in turn, will lead to more vehicle-kilometres travelled and an increase in related CO2 emissions, pollution, and energy use. Increased sprawl-related vehicle-kilometres might lead to higher congestion levels.
Adoption of full or even partial automation may lead to more efficient driving in terms of speed, smoother acceleration and deceleration, which, in turn, will reduce fuel consumption. In the case of congestion reduction the speed will be even more stable. This can lead to up to 10% of fuel economy (NRC, 2010[84]). The increased level of safety might also allow to the manufacturers to produce lighter vehicles and, due to that, the fuel consumption can be additionally reduced up to 14% (Bagloee et al., 2016[85]). However, increased vehicle-kilometres will have the opposite effect. Fuel consumption can grow 10‑40% according to different studies presented above (assuming that the fuel consumption increases in proportion with vehicle-kilometres).
Simulation results
The ITF’s modelling framework was also used to test an autonomous vehicle scenario to assess the possible impact of autonomous private and shared cars, as well as autonomous public transport modes (e.g. buses), on urban transport demand and CO2 emissions. In this scenario assumes that 25%-40% of car trips are autonomous by 2050, depending on the region (in contrast to the current ambition scenario, which assumes that 0%-2.5% of car trips are autonomous by 2050).
The assumptions made in this scenario account for changes in the usage costs of the respective modes, for gains in productive time of vehicle passengers (that would have been drivers in a non-automation scenario), and for potential increases of empty vehicle-kilometres where parking restrictions or charges apply (i.e. vehicles cruising without passenger). The changes in the usage costs of the different transport modes have varied impact on travel and mode choice around the globe, and depend on the relative cost structures of the different modes of transport in the base year and the current ambition scenario.
In most regions, vehicle automation is likely to increase passenger-kilometres. This is mainly due to a decrease in the cost of automated shared mobility or public transport (compared to a scenario without automation) which results in higher uptake rates of these modes that often entail detours for the travellers. The high occupancy rates of these services allow congestion levels to decrease, and CO2 emissions to decline, despite the higher number of total passenger-kilometres travelled.
Table 3.5. Projected impact of an autonomous vehicle scenario
Percentage change compared to current ambition scenario
Region |
Passenger-kilometres |
CO2 emissions |
||
---|---|---|---|---|
2030 |
2050 |
2030 |
2050 |
|
Africa |
-1 |
5 |
-2 |
0 |
Asia |
-1 |
-1 |
-3 |
-10 |
China and India |
1 |
5 |
0 |
0 |
Middle East |
-2 |
-6 |
-3 |
-11 |
Transition |
0 |
2 |
0 |
0 |
Latin America |
-3 |
0 |
3 |
-4 |
OECD Pacific |
-1 |
1 |
-2 |
-7 |
EEA and Turkey |
1 |
7 |
0 |
0 |
North America |
-1 |
-3 |
-1 |
-7 |
Box 3.4. Drones in the transport system
Drones are already being deployed in the transport sector to survey and monitor the condition of infrastructure. In the near future they will also offer innovative services in freight delivery and passenger transport. With the sector developing at a rapid pace, transport policy makers need to create frameworks for drone use that allow innovation while ensuring that society benefits as a whole. The general public may not be ready to fly on pilotless aircrafts (though much of current flight is already automated), but as with advances in self-driving cars, buses and trucks, drone technologies are quickly moving from science fiction to providing services in the real world.
Technological advances have enabled the manufacture of new types of airborne vehicles and the integration of these vehicles within existing (air) transport systems (Schwab, 2016[86]). Although demand for freight and passenger transport is increasing worldwide, many regions lack adequate surface access to potential markets. Particularly in developing and emerging countries, the introduction of reliable and efficient drone services could significantly improve regional connectivity. Aiming to take advantage of such economic opportunities, the private sector has been the main driving force behind the development of drones and it is currently experimenting with novel service applications for a variety of uses.
The potential impacts of large commercial drone fleets are as yet not fully understood. Assessment of their potential impact on aviation has begun, but appraisals are rarely addressed from a cross-sectoral perspective. Freight drones for urban goods deliveries and, eventually, drones for passenger travel, may have both positive impacts (e.g. improved connectivity in remote regions, alleviation of traffic congestion, reduced travel times) and negative impacts (e.g. issues related to safety, privacy, noise, energy consumption, land use and visual amenities) (Schechtner et al., 2018[87]). Policy makers also need to focus on the impact of the potentially millions of “drone ports” and their integration into the transport system and society as a whole.
Drone market forecasts vary considerably, but consensus generally exists regarding the fact that drones will constitute a multi-billion dollar market within the next five to ten years. One global report estimated USD 127 billion for a civil-drone powered solutions market for addressable industries (PwC, 2017[88]). A 2018 global survey by Blyenburgh (2018[89]) expected a three-fold increase in freight drone missions for 2017‑2018, and global players such as Alphabet and Amazon are already trialling both passenger and freight drone operations across the globe.
How far citizens are willing to go to accept the deployment of drone fleets will depend on their understanding of the balance of benefits and disbenefits and on the successful mitigation of potentially adverse effects. Research to quantify impacts is still scarce; therefore ITFs ongoing working group on Drones in the Transport System of the Future is collating global expertise to advance the understanding of the impact of drones.
Disruptive scenarios for urban passenger transport
This section analyses the combination of three possible future disruptions of transport: teleworking, massive shared mobility and autonomous vehicles. The potential impacts of disruptions in urban passenger transport are uncertain at best. This uncertainty relates to their effect on travel behaviour and transport demand, on people’s destination and route choices, on mode shares on spatial accessibility, and on externalities of the transport system such as emissions or congestion. Also uncertain is the future structure of the urban transport industry ecosystem and the arrival and regulation of new actors and services, and the role for incumbent service providers and manufacturers. The overall impact of these disruptive developments will depend on if, and to what degree, they happen independently from each other (e.g. some disruptions may not develop to a significant degree at all), whether they happen in a phased manner (e.g. some disruptions happen before or after other disruptions), or whether they happen simultaneously and may reinforce each other, whether for better or worse.
The impact of disruptions will also depend on policy measures in place as the disruptions evolve and gain importance. Poorly aligned policies may exacerbate potential negative impacts such as congestion or emissions. Adapted policies can ensure that negative impacts are reduced or eliminated as new services and technologies scale up to meet future urban transport demand.
Two illustrative disruption scenarios for urban areas that assess the impact of policy measures on the future of urban transport systems are presented below. Both assume that automated driving, massive shared mobility and teleworking are taken up simultaneously.
However, in the first scenario the disruptive developments play out in an environment where policies do not seek to guide outcomes relating to car use and access (e.g. parking pricing, curb pricing or urban vehicle access regulations), public transport use or the uptake of active modes. Explicit policy guiding the evolvement of disruptions and basic data syntax are absent; interoperability requirements and open access requirements are not met, and hence Mobility as a Service (MaaS) is poorly deployed and exists only in a few niche areas. This is the unmanaged disruption scenario.
In contrast, the managed disruption scenario assumes guiding policies are in place. A broad, supportive and open Mobility as a Service (MaaS) ecosystem helps citizens adopt new travel behaviours. In this scenario, seamless multi-modal trips are so convenient, reliable and affordable that in many instances they become more compelling than single-occupancy car trips.
Table 3.6 summarises the specifications for the managed and unmanaged disruption scenarios, in the context of the high ambition scenario. Both assume other developments that favour transport CO2 reduction, such as the electrification of vehicle fleets (in line with the IEA’s EV30@30 scenario). This is to reflect that such CO2 reduction ambitions will likely progress independently of other technological developments that may disrupt the transport sector.
The simulation results show that disruption without guiding policy action leads to unwanted outcomes. Disruption does not do away with the need for policy. Rather, it necessitates a recalibration of regulatory frameworks to deliver what citizens expect and public authorities are tasked to ensure. The lack of adequate restrictions on single-occupancy vehicles in the unmanaged disruption scenario, for example, results in a significant shift from shared services to private vehicles compared with the managed disruptions scenario for urban areas. This is because vehicle automation reduces travel costs for the users and increases the experienced utility of travel (since travel time can be used for other activities than driving).
The importance of integrating shared mobility options with other transport services is another important insight of the unmanaged disruption simulation. Integration allows travellers seamless interchanges between different transport modes, while a lack of integration means that single-occupancy car trips remain more attractive and reliable than multi-modal trip chains.
Strong access restrictions for single-occupancy vehicles in dense urban areas combined with a robust and compelling MaaS ecosystem have the potential to reverse the trend of increasing private car use. This would benefit society by reducing many of the negative externalities associated with single car use in dense urban environments and, more generally, the emission of pollutants and CO2. It would also benefit individual citizens by putting more reliable, convenient, comfortable, and affordable travel options at their disposal.
Table 3.6. Disruptive scenario specifications for urban passenger transport
Variables |
High ambition |
Unmanaged disruption |
Managed disruption |
|||
---|---|---|---|---|---|---|
Disruptive developments |
||||||
|
Telework |
3‑25% of trips are affected by 2050, depending on the region |
3‑30% of trips are affected by 2050, depending on the region |
3‑30% of trips are affected by 2050, depending on the region |
||
|
Shared mobility |
Provision of shared modes follows past trends |
Provision of shared modes at twice the speed of past trends |
Provision of shared modes at twice the speed of past trends |
||
|
Autonomous driving |
0%-2.5% of car trips are autonomous by 2050, depending on the region |
25%-40% of car trips are autonomous by 2050, depending on the region |
25%-40% of car trips are autonomous by 2050, depending on the region |
||
Mitigation measures |
||||||
|
Transport integration/MaaS |
50% of travellers use MaaS solutions to plan their journeys by 2050, |
20% of travellers use MaaS solutions to plan their journeys by 2050 |
100% of travellers use MaaS solutions to plan their journeys by 2050 |
||
|
Access restrictions for cars |
40% of car trips affected by 2050 |
20% of car trips affected by 2050 |
60% of car trips affected by 2050 |
||
|
Parking pricing |
Parking prices increase 10‑40% relative to expected purchasing power, depending on the region |
Parking prices increase 0‑20% relative to expected purchasing power, depending on the region |
Parking prices increase 10‑40% relative to expected purchasing power, depending on the region |
Note: For all scenarios, electric vehicle uptake follows the EV30@30 scenario. Land-use measures result in a densification of urban regions by 5‑10% by 2050. The supply of mass public transit in all regions follows past trends in Europe.
The managed disruption scenario illustrates that policies have a major impact on future urban modal shares (Figure 3.11). An optimal integration of different transport options in a MaaS ecosystem can result in a significant increase in the share of public transport-like services and meet mobility demand with significantly fewer vehicles. In the managed disruption scenario, public transport modes (bus and rail) cover almost 50% of all passenger-kilometres by 2050, while total vehicle-kilometres decrease by 19% compared to the current ambition scenario. That said, the simulation is agnostic as to how the public transport-like travel is provided – many models are possible and it is likely that what is today called “public transport” will comprise a growing diversity of actors, ideally operating under more flexible and performance-based rules.
Relative energy and operating prices will also play a role, in sometimes unexpected ways. The share of private modes in urban areas drops significantly between 2015 and 2050 in the current ambition, high ambition and managed disruption scenarios, but not in the unmanaged disruption scenario Table 3.7.
Figure 3.11. Global urban mobility by mode of transport and scenario, 2015-50
CO2 emissions from urban transport in 2050 are substantially lower in all scenarios considered when compared to the current ambition scenario. This is an encouraging – albeit partial – result, as it suggests that even unmanaged disruption on top of likely future policies will lead to a drop in the amount of CO2 emissions from urban transport. CO2 emissions in the unmanaged disruption scenario lie between those under the current and high ambition scenarios (see Figure 3.12 and Table 3.8).
This is largely the result of an increased mode share of single-occupancy cars due to the increased uptake of automated driving in the unmanaged disruption scenario. When autonomous driving takes market share away from available shared mobility services, CO2 emissions rise, all else held equal. Thus, overall urban transport-related CO2 emissions grow by almost 50% in the unmanaged disruption scenario when compared to the high ambition scenario. However, CO2 emissions in the unmanaged disruption scenario remain well below those in the current ambition scenario.
The importance of guiding policies becomes evident when comparing urban transport CO2 emissions in the managed disruption and unmanaged disruption scenarios. The creation of a comprehensive MaaS ecosystem that integrates public transport and other forms of shared mobility helps to rein in the potential CO2 emissions increase from the uptake of automated vehicles. CO2 emissions from urban travel are similar in the high ambition and managed disruption scenarios precisely because policies are in place that restrain the growth in the total distance travelled by automated vehicles with a single occupant.
Table 3.7. Projected urban mode shares by world region and scenario, 2015 and 2050
Percent of total passenger-kilometres
Regions |
Private modes |
Public transport |
||||||||
---|---|---|---|---|---|---|---|---|---|---|
2015 |
2050 |
2015 |
2050 |
|||||||
CA |
HA |
UD |
MD |
CA |
HA |
UD |
MD |
|||
Africa |
61 |
46 |
39 |
56 |
29 |
36 |
43 |
41 |
33 |
60 |
Asia |
63 |
44 |
32 |
45 |
21 |
34 |
40 |
39 |
40 |
62 |
China and India |
59 |
34 |
29 |
46 |
21 |
38 |
35 |
25 |
28 |
46 |
EEA and Turkey |
65 |
25 |
30 |
65 |
20 |
30 |
44 |
25 |
20 |
42 |
Latin America |
52 |
30 |
30 |
51 |
21 |
46 |
51 |
40 |
36 |
60 |
Middle East |
78 |
59 |
45 |
63 |
30 |
20 |
31 |
36 |
28 |
57 |
North America |
94 |
66 |
41 |
82 |
39 |
3 |
9 |
7 |
6 |
15 |
OECD Pacific |
62 |
35 |
27 |
52 |
18 |
33 |
40 |
30 |
29 |
51 |
Transition |
59 |
39 |
35 |
53 |
22 |
38 |
47 |
43 |
37 |
64 |
Regions |
Shared transport |
Active modes |
||||||||
2015 |
2050 |
2015 |
2050 |
|||||||
CA |
HA |
UD |
MD |
CA |
HA |
UD |
MD |
|||
Africa |
0 |
9 |
17 |
9 |
9 |
2 |
3 |
2 |
2 |
1 |
Asia |
0 |
14 |
26 |
14 |
16 |
2 |
2 |
2 |
1 |
2 |
China and India |
1 |
29 |
44 |
25 |
32 |
2 |
2 |
2 |
1 |
2 |
EEA and Turkey |
1 |
26 |
42 |
14 |
34 |
3 |
5 |
3 |
2 |
4 |
Latin America |
1 |
18 |
28 |
12 |
18 |
1 |
2 |
1 |
1 |
1 |
Middle East |
1 |
8 |
17 |
7 |
12 |
1 |
2 |
2 |
1 |
1 |
North America |
3 |
24 |
51 |
12 |
45 |
0 |
1 |
1 |
0 |
1 |
OECD Pacific |
3 |
24 |
41 |
18 |
30 |
1 |
2 |
2 |
1 |
1 |
Transition |
1 |
11 |
20 |
9 |
12 |
2 |
3 |
2 |
1 |
2 |
Note: CA: current ambition scenario; HA: high ambition scenario; UD: unmanaged disruption scenario; MD: managed disruption scenario.
Table 3.8. Total urban transport CO2 emissions by world region
Four alternative scenarios, million tonnes
2015 |
2050 |
||||
---|---|---|---|---|---|
Region |
Base year |
Current ambition scenario (CA) |
High ambition scenario compared to CA |
Unmanaged disruption scenario compared to CA |
Managed disruption scenario compared to CA |
Africa |
86 |
186 |
-62 |
-52 |
-66 |
Asia |
150 |
211 |
-67 |
-56 |
-69 |
China and India |
409 |
319 |
-69 |
-48 |
-74 |
EEA and Turkey |
187 |
84 |
-66 |
-34 |
-70 |
Latin America |
197 |
193 |
-61 |
-47 |
-61 |
Middle East |
91 |
142 |
-63 |
-53 |
-68 |
North America |
853 |
547 |
-81 |
-69 |
-84 |
OECD Pacific |
247 |
111 |
-73 |
-61 |
-73 |
Transition |
61 |
46 |
-57 |
-43 |
-63 |
Global |
2281 |
1839 |
-70 |
-56 |
-73 |
The congestion effects of travel activity also underline the need for policy guidance where disruptive developments occur. Congestion effects are measured here by comparing the modelled on-street traffic flows with the capacity of the available street network. The closer the ratio between these two measures, the more congested is the road network. Figure 3.13 and Table 3.9 compare congestion levels of each alternative scenario with the current ambition scenario. In the unmanaged disruption scenario, congestion levels in urban areas increase by 38% globally. The marked increase in projected congestion in Europe in the unmanaged disruption scenario results from increasing distances travelled by autonomous vehicles and from high levels of private car use facilitated by increasingly affordable electric vehicles. In the absence of infrastructure changes and more efficient shared mobility services, these factors result in significantly increased congestion in Europe. However, the guiding policies implemented in the managed disruption scenario effectively reduce congestion levels below the current ambition scenario, and even below the high ambition scenario. So while any disruption scenario can lead to substantial CO2 reductions up to 2050 - more so when guiding policies are put in place – congestion levels can be expected to increase if adequate guiding policies are missed.
Figure 3.12. CO2 emissions from urban transport by mode and scenario
Table 3.9. Projected urban congestion levels by world region, 2050
Percentage change compared to current ambition scenario
Region |
High ambition scenario |
Unmanaged disruptions scenario |
Managed disruptions scenario |
---|---|---|---|
non-OECD |
-16 |
36 |
-24 |
OECD |
-20 |
43 |
-21 |
Africa |
-16 |
20 |
-31 |
Asia |
-26 |
8 |
-43 |
China and India |
-20 |
52 |
-17 |
EEA and Turkey |
7 |
113 |
7 |
Latin America |
3 |
55 |
-5 |
Middle East |
-22 |
3 |
-42 |
North America |
-39 |
5 |
-36 |
OECD Pacific |
-36 |
7 |
-42 |
Transition |
-9 |
36 |
-28 |
Global |
-17 |
38 |
-24 |
Figure 3.13. Projected global urban congestion levels, 2050
Table 3.10. Urban mobility by world region, 2050
Four alternative scenarios, billion passenger-kilometres
2015 |
2050 |
||||
---|---|---|---|---|---|
Region |
Base year |
Current ambition scenario (CA) |
High ambition scenario (% change from CA) |
Unmanaged disruptions scenario (% change from CA) |
Managed disruptions scenario (% change from CA) |
Africa |
982 |
3 787 |
1 |
7 |
-2 |
Asia |
1 546 |
3 825 |
-11 |
-8 |
-17 |
China and India |
4 865 |
11 833 |
-13 |
-7 |
-17 |
EEA and Turkey |
1 733 |
2 695 |
-14 |
-8 |
-18 |
Latin America |
2 180 |
3 924 |
-13 |
-8 |
-16 |
Middle East |
619 |
1 446 |
-12 |
-9 |
-17 |
North America |
3 504 |
5 920 |
-30 |
-28 |
-34 |
OECD Pacific |
2 164 |
2 803 |
-29 |
-27 |
-32 |
Transition |
571 |
808 |
-1 |
2 |
-8 |
Global |
18 164 |
37 040 |
-15 |
-10 |
-19 |
References
[54] Alonso-Mora, J. et al. (2017), “On-demand high-capacity ride-sharing via dynamic trip-vehicle assignment”, Proceedings of the National Academy of Sciences, Vol. 114/3, pp. 462-467, http://dx.doi.org/10.1073/pnas.1611675114.
[65] Anderson, J. et al. (2016), Autonomous Vehicle Technology: A Guide for Policymakers, https://www.rand.org/content/dam/rand/pubs/research_reports/RR400/RR443-2/RAND_RR443-2.pdf (accessed on 29 January 2019).
[85] Bagloee, S. et al. (2016), “Autonomous vehicles: challenges, opportunities, and future implications for transportation policies”, Journal of Modern Transportation, Vol. 24/4, pp. 284-303, http://dx.doi.org/10.1007/s40534-016-0117-3.
[36] Ben-Elia, E., G. Lyons and P. Mokhtarian (2018), “Epilogue: the new frontiers of behavioral research on the interrelationships between ICT, activities, time use and mobility”, Transportation, Vol. 45/2, pp. 479-497, http://dx.doi.org/10.1007/s11116-018-9871-x.
[50] Bliss, L. (2017), Stop Asking Whether Uber Is Transit’s Enemy, CityLab, https://www.citylab.com/transportation/2017/02/uber-lyft-transportation-network-companies-effect-on-transit-ridership-new-york-city/517932/ (accessed on 15 September 2017).
[51] Bliss, L. (2017), The Ride-Hailing Effect: More Cars, More Trips, More Miles, CityLab, https://www.citylab.com/transportation/2017/10/the-ride-hailing-effect-more-cars-more-trips-more-miles/542592/ (accessed on 15 September 2017).
[26] Bloom, N. et al. (2015), “Does Working from Home Work? Evidence from a Chinese Experiment *”, The Quarterly Journal of Economics, Vol. 130/1, pp. 165-218, http://dx.doi.org/10.1093/qje/qju032.
[89] Blyenburgh (2018), Drone Operations: Today & Tomorrow.
[77] Brown, A., J. Gonder and B. Repac (2014), “An Analysis of Possible Energy Impacts of Automated Vehicles”, Springer, Cham, http://dx.doi.org/10.1007/978-3-319-05990-7_13.
[15] Canada, S. (2016), General Social Survey - Canadians at Work and Home.
[78] Childress, S. et al. (2015), “Using an Activity-Based Model to Explore the Potential Impacts of Automated Vehicles”, Transportation Research Record: Journal of the Transportation Research Board, Vol. 2493/1, pp. 99-106, http://dx.doi.org/10.3141/2493-11.
[28] Choo, S., P. Mokhtarian and I. Salomon (2005), “Does telecommuting reduce vehicle-miles traveled? An aggregate time series analysis for the U.S.”, Transportation, Vol. 32/1, pp. 37-64, http://dx.doi.org/10.1007/s11116-004-3046-7.
[41] Ciari, F., N. Schuessler and K. Axhausen (2013), “Estimation of Carsharing Demand Using an Activity-Based Microsimulation Approach: Model Discussion and Some Results”, International Journal of Sustainable Transportation, 965IM<br/>Times Cited:1<br/>Cited References Count:17, pp. 70-84, http://dx.doi.org/Doi 10.1080/15568318.2012.660113.
[47] Clewlow, R. and G. Mishra (2017), “Disruptive Transportation: The Adoption, Utilization, and Impacts of Ride-Hailing in the United States”, Ucd-Its-Rr-17-07 October, https://itspubs.ucdavis.edu/wp-content/themes/ucdavis/pubs/download_pdf.php?id=2752.
[9] Eurofound (2010), Telework in the European Union, European Foundations for the Improvement of Living and Working Conditions, https://www.eurofound.europa.eu/publications/report/2010/telework-in-the-european-union (accessed on 28 January 2019).
[45] Fagnant, D. and K. Kockelman (2016), “Dynamic ride-sharing and fleet sizing for a system of shared autonomous vehicles in Austin, Texas”, Transportation, pp. 1-16, http://dx.doi.org/10.1007/s11116-016-9729-z.
[64] Fagnant, D. and K. Kockelman (2015), “Preparing a nation for autonomous vehicles: opportunities, barriers and policy recommendations”, Transportation Research Part A: Policy and Practice, Vol. 77, pp. 167-181, http://dx.doi.org/10.1016/J.TRA.2015.04.003.
[33] Falch, M. (2012), “Environmental impact of ICT on the transport sector”, Telecommunication Economics, pp. 126-137, http://dx.doi.org/10.1007/978-3-642-30382-1_17.
[49] Fulton, L. (2018), “Three Revolutions in Urban Passenger Travel”, Joule, http://dx.doi.org/10.1016/j.joule.2018.03.005.
[25] Gajendran, R. and D. Harrison (2007), “The Good, the Bad, and the Unknown About Telecommuting: Meta-Analysis of Psychological Mediators and Individual Consequences”, http://dx.doi.org/10.1037/0021-9010.92.6.1524.
[30] Giovanis, E. (2018), “The relationship between teleworking, traffic and air pollution”, Atmospheric Pollution Research, Vol. 9/1, pp. 1-14, http://dx.doi.org/10.1016/j.apr.2017.06.004.
[32] Glogger, A., T. Zängler and G. Karg (2008), “The Impact of Telecommuting on Households’ Travel Behaviour, Expenditures and Emissions”, in Road Pricing, the Economy and the Environment, Springer Berlin Heidelberg, Berlin, Heidelberg, http://dx.doi.org/10.1007/978-3-540-77150-0_21.
[59] Graehler, M., R. Mucci and G. Erhardt (2018), Understanding the Recent Transit Ridership Decline in Major US Cities: Service Cuts or 1 Emerging Modes?, 98th Annual Meeting of the Transportation Research Board, https://www.researchgate.net/publication/330599129_Understanding_the_Recent_Transit_Ridership_Decline_in_Major_US_Cities_Service_Cuts_or_Emerging_Modes (accessed on 1 March 2019).
[80] Gruel, W. and J. Stanford (2016), “Assessing the Long-term Effects of Autonomous Vehicles: A Speculative Approach”, Transportation Research Procedia, Vol. 13, pp. 18-29, http://dx.doi.org/10.1016/J.TRPRO.2016.05.003.
[11] Gschwind, L. et al. (2017), Working anytime, anywhere : the effects on the world of work., https://www.eurofound.europa.eu/publications/report/2017/working-anytime-anywhere-the-effects-on-the-world-of-work (accessed on 29 January 2019).
[23] Haddad, H. and K. Chatterjee (2009), “An examination of determinants influencing the desire for and frequency of part-day and whole-day homeworking”, Journal of Transport Geography, Vol. 17/2, pp. 124-133, http://dx.doi.org/10.1016/J.JTRANGEO.2008.11.008.
[76] Harper, C., C. Hendrickson and C. Samaras (2016), “Cost and benefit estimates of partially-automated vehicle collision avoidance technologies”, Accident Analysis & Prevention, Vol. 95, pp. 104-115, http://dx.doi.org/10.1016/J.AAP.2016.06.017.
[20] Helminen, V. and M. Ristimäki (2007), “Relationships between commuting distance, frequency and telework in Finland”, Journal of Transport Geography, Vol. 15/5, pp. 331-342, http://dx.doi.org/10.1016/J.JTRANGEO.2006.12.004.
[6] ICCT (2019), Transportation Roadmap, https://www.theicct.org/transportation-roadmap (accessed on 13 March 2019).
[4] IEA (2018), Global EV Outlook 2018: Towards cross-modal electrification, International Energy Agency, Paris, https://dx.doi.org/10.1787/9789264302365-en.
[3] IEA (2018), World Energy Outlook 2018, International Energy Agency, Paris, https://dx.doi.org/10.1787/weo-2018-en.
[8] ILO-Eurofound (2017), “Sixth European Working Conditions Survey”, https://www.eurofound.europa.eu/surveys/european-working-conditions-surveys/sixth-european-working-conditions-survey-2015.
[69] ITF (2018), Safer Roads with Automated Vehicles?, OECD Publishing, https://doi.org/10.1787/b2881ccb-en (accessed on 29 January 2019).
[39] ITF (2018), “Shared Mobility Simulations for Dublin”, International Transport Forum Policy Papers 72, https://doi.org/10.1787/e7b26d59-en.
[2] ITF (2017), ITF Transport Outlook 2017, OECD Publishing, Paris, https://dx.doi.org/10.1787/9789282108000-en.
[74] ITF (2017), Managing the Transition to Driverless Road Freight Transport, International Transport Forum, Paris, https://doi.org/10.1787/0f240722-en (accessed on 2 October 2018).
[57] ITF (2017), “Shared Mobility Simulations for Auckland”, International Transport Forum Policy Papers, No. 41, OECD Publishing, Paris, https://dx.doi.org/10.1787/5423af87-en.
[38] ITF (2017), “Shared Mobility Simulations for Helsinki”, International Transport Forum Policy Papers 64, https://doi.org/10.1787/3d340a2a-en.
[55] ITF (2017), “Transition to Shared Mobility: How large cities can deliver inclusive transport services”, International Transport Forum Policy Papers, No. 33, OECD Publishing, Paris, https://doi.org/10.1787/b1d47e43-en.
[53] ITF (2016), “Shared mobility: innovation for liveable cities”, International Transport Forum Policy Papers Nº 21, https://doi.org/10.1787/5jlwvz8bd4mx-en.
[67] ITF (2015), Automated and Autonomous Driving Regulation under uncertainty, https://doi.org/10.1787/5jlwvzdfk640-en (accessed on 29 January 2019).
[56] ITF (2015), Urban Mobility System Upgrade: How shared self-driving cars could change city traffic, OECD Publishing, https://doi.org/10.1787/5jlwvzdk29g5-en.
[7] ITF (forthcoming), “Benchmarking Accessibility in Cities: Measuring the impact of proximity and transport performance”.
[58] ITF (forthcoming), “Shared Mobility Simulations for Lyon”, International Transport Forum Policy Papers.
[52] Karim, D. (2017), “Creating an Innovative Mobility Ecosystem for Urban Planning Areas”, in Disrupting Mobility, Springer.
[31] Kitou, E. and A. Horvath (2003), Energy-related emissions from telework, American Chemical Society, http://dx.doi.org/10.1021/es025849p.
[43] Liu, J. et al. (2017), “Tracking a system of shared autonomous vehicles across the Austin, Texas network using agent-based simulation”, Transportation, Vol. 44/6, pp. 1261-1278, http://dx.doi.org/10.1007/s11116-017-9811-1.
[19] Loo, B. and B. Wang (2018), “Factors associated with home-based e-working and e-shopping in Nanjing, China”, Transportation, Vol. 45/2, pp. 365-384, http://dx.doi.org/10.1007/s11116-017-9792-0.
[10] Mateyka, P., M. Rapino and L. Landivar (2012), Home-Based Workers in the United States: 2010, https://www.census.gov/prod/2012pubs/p70-132.pdf (accessed on 29 January 2019).
[24] Mayo, M. et al. (2016), “Leader beliefs and CSR for employees: the case of telework provision”, Leadership & Organization Development Journal, Vol. 37/5, pp. 609-634, http://dx.doi.org/10.1108/LODJ-09-2014-0177.
[21] Melo, P. and J. de Abreu e Silva (2017), “Home telework and household commuting patterns in Great Britain”, Transportation Research Part A: Policy and Practice, Vol. 103, pp. 1-24, http://dx.doi.org/10.1016/J.TRA.2017.05.011.
[16] MIAESR (2013), A Statistical Report on Waves 1 to 10 of the Household, Income and Labour Dynamics in Australia Survey, http://library.bsl.org.au/jspui/bitstream/1/3517/1/statreport-v8-2013.pdf (accessed on 29 April 2019).
[13] Ministry of Internal Affairs and Communications (2011), Efforts to Promote Telework in Japan, http://www.soumu.go.jp/main_sosiki/joho_tsusin/eng/presentation/pdf/110908_1.pdf (accessed on 29 April 2019).
[27] Mokhtarian, P. (1991), “Telecommuting and travel: state of the practice, state of the art”, Transportation, Vol. 18/4, pp. 319-342, http://dx.doi.org/10.1007/BF00186563.
[71] NHSTA (2017), Automated Driving Systems 2.0: A Vision for Safety, https://www.nhtsa.gov/sites/nhtsa.dot.gov/files/documents/13069a-ads2.0_090617_v9a_tag.pdf (accessed on 30 January 2019).
[35] Nilles, J. (1991), “Telecommuting and urban sprawl: mitigator or inciter?”, Transportation, Vol. 18/4, pp. 411-432, http://dx.doi.org/10.1007/BF00186567.
[66] Noy, I., D. Shinar and W. Horrey (2018), “Automated driving: Safety blind spots”, Safety Science, Vol. 102, pp. 68-78, http://dx.doi.org/10.1016/J.SSCI.2017.07.018.
[84] NRC (2010), Hidden Costs of Energy, http://dx.doi.org/10.17226/12794.
[29] O’Keefe, P. et al. (2016), “The impacts of telecommuting in Dublin”, Research in Transportation Economics, Vol. 57, pp. 13-20, http://dx.doi.org/10.1016/J.RETREC.2016.06.010.
[70] Parasuraman, R., T. Sheridan and C. Wickens (2000), A Model for Types and Levels of Human Interaction with Automation, http://hci.cs.uwaterloo.ca/faculty/elaw/cs889/reading/automation/sheridan.pdf (accessed on 29 January 2019).
[12] PGi (2015), The State of Telecommuting Around the World, http://go.pgi.com/gen-genspec-15telesur-SC1129 (accessed on 29 January 2019).
[88] PwC (2017), Clarity from above: transport infrastructure The commercial applications of drone technology in the road and rail sectors, http://www.dronepoweredsolutions.com (accessed on 29 January 2019).
[48] Ronald, N. et al. (2017), “Disrupting Mobility”, Disrupting Mobility, pp. 275-290, http://dx.doi.org/10.1007/978-3-319-51602-8.
[68] SAE (2018), Taxonomy and Definitions for Terms Related to Driving Automation Systems for On-Road Motor Vehicles, https://saemobilus.sae.org/content/j3016_201806 (accessed on 29 January 2019).
[37] SAE (2018), Taxonomy and Definitions for Terms Related to Shared Mobility and Enabling Technologies, https://saemobilus.sae.org/content/J3163_201809/ (accessed on 29 April 2019).
[62] Santi, P. and C. Ratti (2017), “A future of shared mobility”, Journal of Urban Regeneration and Renewal, Vol. 10/4, pp. 328-333.
[87] Schechtner, K. et al. (2018), (Un)certain Skies? Drones in the World of Tomorrow, International Transport Forum, Paris, http://www.itf-oecd.org (accessed on 29 January 2019).
[72] Schoettle, B. (2017), Sensor Fusion: A Comparison of Sensing Capabilities of Human Drivers and Highly Automated Vehicles, http://umich.edu/~umtriswt/PDF/SWT-2017-12.pdf (accessed on 30 January 2019).
[79] Schoettle, B. and M. Sivak (2015), Potential Impact of Self-driving Vehicles on Household Vehicle Demand and Usage, http://www.umich.edu/~umtriswt (accessed on 30 January 2019).
[86] Schwab, K. (2016), The Fourth Industrial Revolution, http://www.weforum.org (accessed on 29 January 2019).
[46] Shaheen, S. et al. (2015), “Shared Mobility a Sustainablity and Technology Workshop: Definition, Industry Development and Early Understanding”, University of California Berkeley Transportation Sustainability Research Center, p. 30, http://innovativemobility.org/wp-content/uploads/2015/11/SharedMobility_WhitePaper_FINAL.pdf.
[61] Shaheen, S. et al. (2015), “Shared Mobility. Definitions, Industry Developments, and Early Understanding”, University of California Berkeley Transportation Sustainability Research Center, p. 30, http://innovativemobility.org/wp-content/uploads/2015/11/SharedMobility_WhitePaper_FINAL.pdf.
[40] Shaheen, S. et al. (2017), Shared mobility : current practices and guiding principles, This report is disseminated under the sponsorship oU.S. Department of Transportation, http://www.ops.fhwa.dot.gov/publications/fhwahop16022/index.htm.
[60] Shaheen, S., A. Stocker and M. Mundler (2017), “Online and App-Based Carpooling in France: Analyzing Users and Practices—A Study of BlaBlaCar”, in Disrupting Mobility. Impacts of Sharing Economy and Innovative Transportation on Cities, http://dx.doi.org/10.1007/978-3-319-51602-8_12.
[82] Simonite, T. (2013), Data Shows Google’s Robot Cars Are Smoother, Safer Drivers Than You or I - MIT Technology Review, MIT Technology Review, https://www.technologyreview.com/s/520746/data-shows-googles-robot-cars-are-smoother-safer-drivers-than-you-or-i/ (accessed on 30 January 2019).
[18] Singh, P. et al. (2013), “On modeling telecommuting behavior: option, choice, and frequency”, Transportation, Vol. 40/2, pp. 373-396, http://dx.doi.org/10.1007/s11116-012-9429-2.
[42] Spieser, K. et al. (2014), “Toward a systematic approach to the design and evaluation of automated mobility-on-demand systems: A case study in Singapore”, in Road Vehicle Automation, pp. 0-16, Springer,, http://dx.doi.org/10.1007/978-3-319-05990-7_20.
[73] Stolte, T. et al. (2016), “On Functional Safety of Vehicle Actuation Systems in the Context of Automated Driving”, IFAC-PapersOnLine, Vol. 49/11, pp. 576-581, http://dx.doi.org/10.1016/J.IFACOL.2016.08.084.
[83] Tientrakool, P., Y. Ho and N. Maxemchuk (2011), Highway Capacity Benefits from Using Vehicle-to-Vehicle Communication and Sensors for Collision Avoidance, IEEE, http://dx.doi.org/10.1109/VETECF.2011.6093130.
[14] U.S. Bureau of Labor Statistics (2016), 24 percent of employed people did some or all of their work at home in 2015, https://www.bls.gov/opub/ted/2016/24-percent-of-employed-people-did-some-or-all-of-their-work-at-home-in-2015.htm (accessed on 29 April 2019).
[1] UN DESA (2018), World Urbanization Prospects: The 2018 Revision, Key Facts, United Nations, Department of Economic and Social Affairs, Pupulation Division, http://dx.doi.org/(ST/ESA/SER.A/366).
[75] Wadud, Z., D. MacKenzie and P. Leiby (2016), “Help or hindrance? The travel, energy and carbon impacts of highly automated vehicles”, Transportation Research Part A: Policy and Practice, Vol. 86, pp. 1-18, http://dx.doi.org/10.1016/J.TRA.2015.12.001.
[17] Walls, M., E. Safirova and Y. Jiang (2006), What Drives Telecommuting? What Drives Telecommuting? The Relative Impact of Worker Demographics, Employer Characteristics, and Job Types, Resource for the Future, https://pdfs.semanticscholar.org/5555/609f5a39c2b7aa5320c87f573356fca2bded.pdf (accessed on 29 January 2019).
[81] WEF/BCG (2018), System Initiative on Shaping the Future of Mobility Reshaping Urban Mobility with Autonomous Vehicles Lessons from the City of Boston, http://www3.weforum.org/docs/WEF_Reshaping_Urban_Mobility_with_Autonomous_Vehicles_2018.pdf (accessed on 30 January 2019).
[5] WHO (2016), WHO releases country estimates on air pollution exposure and health impact, https://www.who.int/news-room/detail/27-09-2016-who-releases-country-estimates-on-air-pollution-exposure-and-health-impact (accessed on 13 March 2019).
[22] Wilton, R. and D. Scott (2011), “Why do you care what other people think? A qualitative investigation of social influence and telecommuting”, Transportation Research Part A: Policy and Practice, Vol. 45/4, pp. 269-282, http://dx.doi.org/10.1016/J.TRA.2011.01.002.
[63] Yanocha, D. (2018), Optimising New Mobility Services, International Transport Forum Discussion Papers, http://www.itf-oecd.org (accessed on 29 January 2019).
[44] Zachariah, J. et al. (2014), Uncongested Mobility for All: A Proposal for an Area Wide Autonomous Taxi System in New Jersey.
[34] Zhu, P. et al. (2018), “Metropolitan size and the impacts of telecommuting on personal travel”, Transportation, Vol. 45/2, pp. 385-414, http://dx.doi.org/10.1007/s11116-017-9846-3.
Notes
← 1. See www.itf-oecd.org/outlook for model details.
← 2. Excluding public transit; see glossary for more details.
← 3. See glossary for a definition of free-floating.
← 4. OECD Pacific countries include Australia, Japan, New Zealand and South Korea; transition economies include Former Soviet Union countries and non-EU south-eastern European countries.
← 5. Although flexible working arrangements involving teleworking that do not reduce trips to the office can also have an impact on travel demand patterns and congestion, the emissions reductions resulting from these arrangements is assumed to be lower than teleworking activity that reduces total trips to the office.
← 6. Since the PGi survey sample targets digitally-enabled workers, it likely overestimates the proportion of teleworkers in the total employed population.
← 7. https://www.federalreserve.gov/publications/2018-economic-well-being-of-us-households-in-2017-employment.htm
← 8. Non-transport related impacts are numerous, ranging from increased productivity, reduced exposure to air pollution, increased accessibility to employment, and greater employee well-being.
← 9. Ben-Elia et al. (2018) also call for future research on the relationship between ICT (including teleworking activity) and travel behaviour that addresses the possible simultaneity between the two as well as the possibility that unobserved confounding factors may be responsible for the direct impacts that have been documented thus far.
← 10. In 2018, the ITF published a report entitled Safer Roads with Automated Vehicles? (ITF, 2018a), on which much of this section is heavily based.