The artificial intelligence (AI) landscape has evolved significantly since 1950 when Alan Turing first posed the question of whether machines can think. Today, AI is transforming societies and economies. It promises to generate productivity gains, improve well-being and help address global challenges, such as climate change, resource scarcity and health crises. Yet, the global adoption of AI raises questions related to trust, fairness, privacy, safety and accountability, among others. Advanced AI is prompting reflection on the future of work, leisure and society. This chapter examines current and expected AI technological developments, reflects on the opportunities and risks foresight experts anticipate, and provides a snapshot of how countries are implementing the OECD AI Principles. In so doing, it helps build a shared understanding of key opportunities and risks to ensure AI is trustworthy and used to benefit humanity and the planet.
OECD Digital Economy Outlook 2024 (Volume 1)
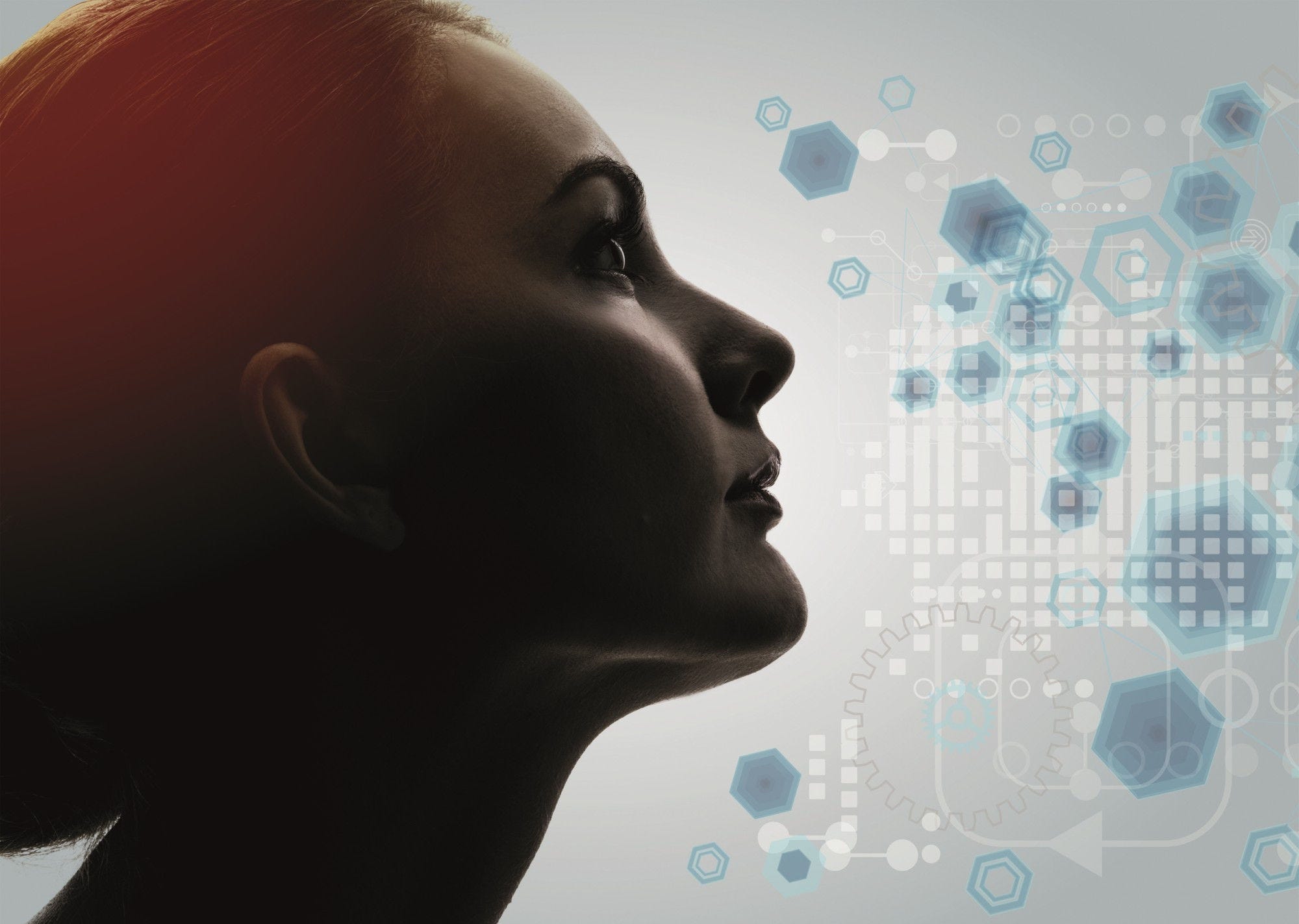
Chapter 2. The future of artificial intelligence
Copy link to Chapter 2. The future of artificial intelligenceAbstract
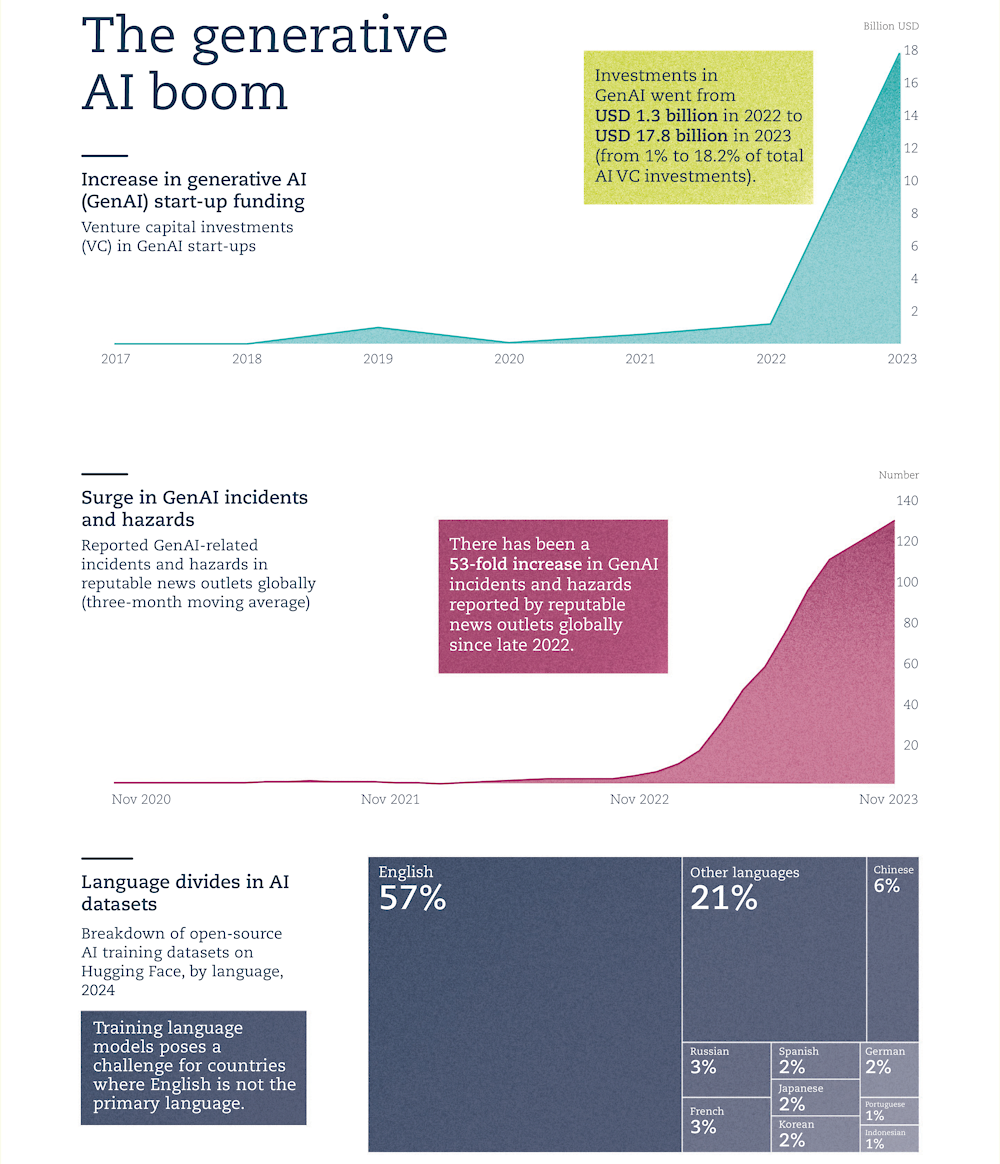
Source: OECD.AI (accessed on 10 March 2024).
Key findings
Copy link to Key findingsTechnical breakthroughs have enabled generative AI that is so advanced users may be unable to distinguish between human and AI-generated content
In late 2022, generative AI advances took many by surprise, despite some researchers anticipating such developments. Collaboration and interdisciplinarity between policy makers, AI developers and researchers are key to helping keep pace with AI progress and close knowledge gaps.
Research and expert opinions suggest that future impacts of AI could vary widely, promising considerable socio-economic benefits but also presenting substantial risks that need addressing
The future of AI may yield tremendous benefits, including enhanced productivity gains, accelerating scientific progress and helping address climate change. However, AI advances also present critical risks, including spreading mis- and dis-information, and threats to human rights.
The long-term trajectories and risks of AI are often discussed and widely debated
Most AI today can be considered “narrow” (designed to perform a specific task), but some experts argue that foundation models are an early form of more “general” AI. This includes progress towards artificial general intelligence (AGI) – a controversial concept that can be described as machines with human-level or greater intelligence across a broad spectrum of contexts.
Some experts argue that challenges in ensuring alignment of machine outputs with human preferences could result in humans losing control of AGI. However, the plausibility and potential nature of AGI are disputed. Many future risks do not require AGI, leading others to argue that focusing on hypothetical AGI distracts from near-term risks.
AI research and development and venture capital (VC) investments are poised to increase
Since mid-2019, the People’s Republic of China (hereafter “China”) published more AI research than the United States or the European Union. India is also making strides, more than doubling its AI research publications since 2015.
Between 2015 and 2023, global VC investments in AI start-ups tripled (from USD 31 billion to USD 98 billion), with investments in generative AI specifically growing from 1% of total AI VC investments in 2022 (USD 1.3 billion) to 18.2% (USD 17.8 billion) in 2023, despite cooling capital markets.
AI development and use is expected to continue to depend on access to computing infrastructure
Compute divides may worsen between and within countries. Increasingly, within countries, public sector entities lack the computing resources to train advanced AI.
AI benefits and risks have global reach, making international co-operation critical to ensure AI policies and laws are complementary, effective and interoperable
AI systems around the world can use the same underlying AI inputs and tools, such as AI algorithms, models and training datasets. This makes countries and organisations vulnerable to similar risks like bias, human rights infringements, and security vulnerabilities or failures.
Artificial intelligence (AI) is transforming economies and societies, but is the world ready? AI promises to generate productivity gains, improve well-being and help address global challenges, such as climate change, resource scarcity and health crises. Yet, as AI is adopted around the world, its use raises questions and challenges. AI has advanced significantly, prompting reflection on the future of work, education, leisure and society. This chapter looks to potential futures to help build an understanding of key opportunities and risks in ensuring AI is trustworthy and used for good.
This chapter examines current and expected AI technological developments, reflects on the opportunities and risks foresight experts anticipate may lie ahead, and provides a snapshot of how countries are implementing the OECD AI Principles. It examines the interrelated factors that will likely shape AI governance in the decades to come (Figure 2.1), to help build a shared understanding of key opportunities and risks to ensure AI is trustworthy and used to benefit humanity and the planet.
Figure 2.1. Examples of interrelated factors that will likely shape AI governance in future decades
Copy link to Figure 2.1. Examples of interrelated factors that will likely shape AI governance in future decades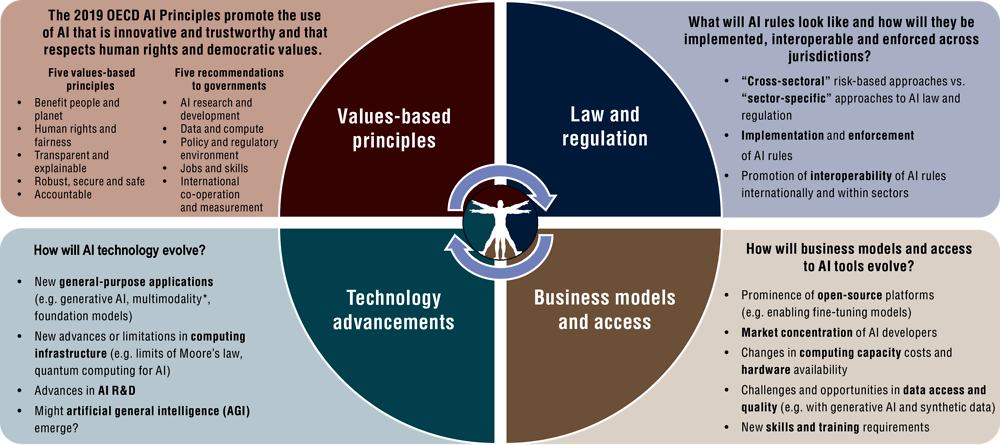
Note: *Multimodal AI combines multiple types of data – such as data from text, image or audio – via machine-learning models and algorithms. It is key for AI research and applications such as in manufacturing and robotics (The Alan Turing Institute, 2023[1]).
Source: Adapted from Figure 1.S.2. of the Spotlight “Next generation wireless networks and the connectivity ecosystem” in this volume.
The AI technological landscape today and tomorrow
Copy link to The AI technological landscape today and tomorrowThe AI technological landscape has evolved significantly from the 1950s when British mathematician Alan Turing first posed the question of whether machines can think (Turing, 2007[2]). Coined as a term in 1956, AI has evolved from symbolic AI where humans built logic-based systems, through the AI “winter” of the 1970s, to the chess-playing computer Deep Blue in the 1990s. The 21st century saw breakthroughs in the branch of AI called machine learning that improved the ability of machines to make predictions from historical data (OECD, 2019[3]). Recent years have witnessed the emergence of generative AI, including large language models, that can generate novel content and enable consumer-facing applications like advanced chatbots at people’s fingertips (OECD, 2023[4]). For many, AI became “real” in 2022 – the year that OpenAI’s ChatGPT became the fastest-growing consumer application in history (Hu, 2023[5]). To understand the evolution of AI technological developments to date, and how they might develop in future years, policy makers need a shared understanding of key AI terms (Box 2.1), as well as of the basic AI production function, described by three enablers: algorithms, data and computing resources (“compute”).
Advances in neural networks and deep learning are resulting in larger, more advanced and more compute-intensive AI models and systems
Copy link to Advances in neural networks and deep learning are resulting in larger, more advanced and more compute-intensive AI models and systemsThe application of machine-learning techniques, the availability of large datasets, and faster and more powerful computing hardware have converged. Together, they are dramatically increasing the capabilities, impact and availability of AI models and systems, moving from academic discussions into remarkable real-world applications. Inspired by the human brain, neural networks are made up of layers of “neurons”, known as “nodes”, that process inputs with weights and biases to give specific outputs (Russell and Norvig, 2016[11]). A subset of algorithms in the area of neural networks – called deep neural networks (in the field of study and set of techniques called deep learning) – allows machine-based systems to “learn” from examples to make predictions or “inferences”, based on the large amount of data processed during their training phase. Deep neural networks are distinct mostly in that they are general and require little adaptation (or “cleaning”) of input data to make accurate predictions.
In 2017, a group of researchers introduced a type of neural network architecture called “transformers”. It became a key conceptual breakthrough, unleashing major progress in AI language models. Transformers learn to detect how data elements – such as the words in this sentence – influence and depend on each other (Vaswani et al., 2023[12]). Unlike previous neural networks, transformers can process inputs from a sequence, such as words of text, in parallel. This enabled AI developers to design larger-scale language models with significantly more parameters – the numerical weights that define the model – and greater efficiency (OECD, 2023[4]; Vaswani et al., 2023[12]). Transformers have unlocked significant advances across language recognition (such as chatbots) and science (such as protein folding). Vision transformers are also becoming popular for various computer vision tasks (Islam, 2022[13]). Notable transformer models include AlphaFold2 (DeepMind), GPT-4 (OpenAI), LaMDA (Google) and BLOOM (Hugging Face) (Collins and Ghahramani, 2021[14]; Hugging Face, 2022[15]; Merritt, 2022[16]).
Box 2.1. “A” is for artificial intelligence
Copy link to Box 2.1. “A” is for artificial intelligenceAI has received notable attention in the media and in policy circles. Amid the flurry of headlines and analysis, policy makers require a shared understanding of key AI terms to help keep up with rapid AI advancements, and to respond with AI policies that can stand the test of time. This chapter uses the following terms:
Artificial intelligence: “An AI system is a machine-based system that, for explicit or implicit objectives, infers, from the input it receives, how to generate outputs such as predictions, content, recommendations or decisions that can influence physical or virtual environments. Different AI systems vary in their levels of autonomy and adaptiveness after deployment” (OECD, 2024[6]).
Algorithm: “[A] set of step-by-step instructions to solve a problem (e.g. not including data). [It] can be abstract and implemented in different programming languages and software libraries” (EU-US Trade and Technology Council, 2023[7]).
AI compute: “AI computing resources (‘AI compute’) include one or more stacks of hardware and software used to support specialised AI workloads and applications in an efficient manner” (OECD, 2023[8]).
Foundation model: A model that is trained on large amounts of data – generally using self-supervision at scale – that can be adapted (e.g. fine-tuned) to a wide range of downstream tasks. Examples include BERT (Google) and GPT-3 (OpenAI) (Bommasani et al., 2021[9]).
Generative AI: AI systems capable of creating new content – including text, image, audio and video – based on their training data, and usually in response to prompts. The recent growth and media coverage of generative AI, notably in the areas of text and image generation, has spotlighted AI’s capabilities, leading to significant public, academic and political discussion (Lorenz, Perset and Berryhill, 2023[10]).
AI language model: A model that can process, analyse or generate natural language text trained on vast amounts of data, using techniques ranging from rule-based approaches to statistical models and deep learning. Chatbots, machine translation and virtual assistants that recognise speech are all applications of language models. Not all language models are generative in nature, and they can be large or small (OECD, 2023[4]).
Machine learning: “[A] branch of artificial intelligence (AI) and computer science which focuses on development of systems that are able to learn and adapt without following explicit instructions imitating the way that humans learn, gradually improving its accuracy, by using algorithms and statistical models to analyse and draw inferences from patterns in data” (EU-US Trade and Technology Council, 2023[7]). The “learning” process using machine-learning techniques is known as “training”. A machine-learning technique called “neural networks”, and its further subset technique called “deep learning”, has enabled leaps in technological AI developments (OECD, 2019[3]).
Multimodal AI: AI that combines multiple types of data – such as data from text, image or audio – such as through machine-learning models and algorithms. Multimodal AI is key for AI research and applications such as in manufacturing and robotics (The Alan Turing Institute, 2023[1]).
Notes: This is an inexhaustive list of terms selected for this chapter. The EU-US Trade and Technology Council released a second edition of Terminology and Taxonomy for Artificial Intelligence in April 2024. For further information, please visit: https://digital-strategy.ec.europa.eu/en/library/eu-us-terminology-and-taxonomy-artificial-intelligence-second-edition.
Researchers are exploring whether transformers can create “generalist” and “multimodal” AI systems that can perform multiple of different tasks across robotics, computer vision, simulated environments, natural language and more. For example, Google DeepMind’s Gato (2022) can perform tasks such as stacking blocks with a robot arm, playing video games, captioning images and chatting with users. Unlike previous AI models that could only learn one task at a time, some new transformer models can learn multiple different tasks simultaneously, enabling them to switch between tasks and learn new skills without forgetting previous skills (Heikkilä, 2022[17]; Reed et al., 2022[18]). However, AI models and systems today still exhibit factual inaccuracy; “hallucinations” (making up facts in a credible way, often when a correct answer is not found in the training data); inconsistency; and misunderstanding when used in new contexts, often requiring human assistance and oversight for correct functioning. Some experts argue that advanced AI systems and models represent a step towards more capable and general forms of AI. Some even claim progress towards the hypothetical advent of artificial general intelligence (AGI) that would usher in significant benefits and risks. Such discussions are actively debated in the AI expert community (Box 2.2).
Box 2.2. Increasingly general AI systems
Copy link to Box 2.2. Increasingly general AI systemsSome experts believe the latest large language and other generative AI models can apply outputs in a general way across many contexts. However, the extent to which these models can generalise versus simply mimicking information from their datasets is actively debated.
Since their origin in the 1950s, AI systems have been “narrow” and context-specific. However, due to recent advances, some experts argue that state-of-the-art “foundation models” are moving to capabilities that are more general in nature.
Foundation models are trained on large amounts of data and can be adapted and built upon for a wide range of downstream tasks. Some experts argue that such models represent a significant step towards the hypothetical advent of artificial general intelligence (AGI), the timeline, definition and premise of which is intensely debated. AGI is a controversial concept that can be described as machines with human-level or greater intelligence across a broad spectrum of domains and contexts.
Many experts argue that a focus on speculative notions of AGI obfuscates potentially significant benefits and risks posed by existing and near- to medium-term AI systems. To move away from this debate, some AI experts have begun using the phrase artificial capable intelligence (ACI). This is meant to describe potentially transformative AI systems that require minimal human supervision but that may not achieve the hypothesised state of AGI.
Researchers at DeepMind (Google) have proposed a framework for classifying progress in capabilities based on AI systems’ levels of performance, generality and autonomy. They aim to provide a common language for comparing AI models, assessing risks and measuring progress in AI advancements.
AGI is the focus of active debates and media reporting. While an increasing number of experts from a wide array of disciplines are engaging in the debate around achieving more general AI systems, their views, forecasts and understanding of key related terms vary greatly.
Foundation models are enabling increasing AI generality across application domains, industries and tasks
Copy link to Foundation models are enabling increasing AI generality across application domains, industries and tasksRecent AI breakthroughs have shifted capabilities from task-specific models and systems to those that are more flexible and applicable across domains, industries and tasks. These advances centre on “foundation models” – models trained on large amounts of data that can be adapted to a wide range of downstream tasks such as OpenAI’s Generative Pretrained Transformers (GPT) series (Bommasani et al., 2021[9]; Jones, 2023[23]; Lorenz, Perset and Berryhill, 2023[10]). Foundation models can be further trained or “fine-tuned”, for example, with specific data to gain insights relevant to a specific sector or adapted to a wide range of distinct tasks (Bommasani et al., 2021[9]).
Today’s foundation models share several common characteristics. First, they often include billions of parameters, making them very large in size. Second, their outputs can be difficult to explain due to their complex computational processes. This has resulted in impressive performance and outputs, even beyond their developers’ expectations. However, the model’s steps or process to arrive at an output often cannot be explained. Third, training today’s foundation models requires massive amounts of AI compute, data and specialised AI talent, making them expensive to develop (Dunlop, Moës and Küspert, 2023[24]). However, as the technology, hardware/software and training methods advance, it is unclear whether future foundation models will require similarly large datasets and compute, and retain such large numbers of parameters.
Many have called the recent emergence of foundation models a major “paradigm shift” and technological advancement. Enabled by foundation models, some AI models can transfer capabilities from one domain to another. For example, they can combine multiple types of data across domains (e.g. image, text, audio). Foundation models, especially those available as open-source, can also allow AI developers and researchers with limited resources to fine-tune and deploy AI in their specific domains. Using foundation models, developers do not necessarily require access to advanced compute hardware and large datasets, or need to incur significant training costs to train the foundation model to begin with.
Foundation models will likely continue to drive advancement of AI capabilities, unlocking promising opportunities for innovation and productivity gains across domains and sectors. However, they also raise several challenges. A reliance on foundation models – for example by smaller and less well-capitalised downstream users like start-ups – could create dependency dynamics between the users and producers of such models. The cost and complexity of foundation models have limited their development to well-capitalised firms, such as models like GPT-4 (OpenAI/Microsoft), BERT (Google) or LlaMA 2 (Meta), or to organisations and governments that can afford to fund their training.
It is often difficult and expensive to understand what foundation model training datasets contain, and verifying the validity of outcomes sometimes requires specialised expertise. Moreover, foundation model developers may not disclose key information about such models, for a variety of business or technical reasons. Distribution mechanisms by developers – such as application programming interfaces (APIs) – can mask certain model properties. This makes it difficult to assess how models align with certain principles, or to use models in downstream contexts in ways that promote transparency, explainability and accountability (Dunlop, Moës and Küspert, 2023[24]).
Such issues illustrate the possible increasing imbalance between those who can perform the most resource-intensive first steps of building such models and those who rely on pretrained models (i.e. foundation models). Access to the most advanced AI models could be limited by those who own them. This would pose challenges for policy makers and governments as they seek to create a level playing field that allows smaller and less-resourced groups to innovate (OECD, 2023[25]). Policy challenges could include questions around access to tools for innovation and market dynamics concerning competition.
AI development and use is expected to continue to depend on access to computing infrastructure
Copy link to AI development and use is expected to continue to depend on access to computing infrastructureComputing infrastructure (“AI compute”) is a key component needed for AI development and expected to be a continued driver of AI’s improved capabilities over time. Unlike other AI inputs like data or algorithms, AI compute is grounded in “stacks” (layers) of physical infrastructure and hardware, along with software specialised for AI (OECD, 2023[8]). Advancements in AI compute have enabled a transition from general-purpose processors, such as Central Processing Units (CPUs), to specialised hardware requiring less energy for more computations per unit of time. Today, advanced AI is predominantly trained on specialised hardware optimised for certain types of operations, such as Graphics Processing Units (GPUs), Tensor Processing Units, Neural Processing Units and others. Training AI on general-purpose hardware is less efficient (OECD, 2023[8]). The high volume of AI-focused computing infrastructure has also enabled AI advances, in addition to technological advances in AI hardware itself.
The demand for AI compute has grown dramatically, especially for deep learning neural networks (OECD, 2023[8]). Securing specialised hardware purpose-built for AI can be challenging due to complex supply chains, as illustrated by bottlenecks in the semiconductor industry (Khan, Mann and Peterson, 2021[26]). Integrated circuits or computer chips made of semiconductors are a critical input for AI compute. They are called the “brains of modern electronic equipment, storing information and performing the logic operations that enable devices such as smartphones, computers and servers to operate” (OECD, 2019[27]). Any electronic device can have multiple integrated circuits fulfilling specific functions, such as CPUs or chips designed for power management, memory, graphics (e.g. GPUs used for AI). Demands on semiconductor supply chains have grown in recent years, especially as digital and AI-enabled technologies become more commonplace, such as Internet of Things devices, smart energy grids and autonomous vehicles. The semiconductor supply chain is also highly concentrated, making it more vulnerable to shocks (OECD, 2019[27]).
Training and inference related to advanced AI requires significant compute resources, leading to environmental impacts: energy and water use, carbon emissions, e-waste and natural resource extraction like rare mineral mining. Experts have raised concerns around the direct environmental impacts of AI (i.e. those created from training or inference). This is especially relevant as generative AI becomes more accessible, with applications like chatbots increasing demand for server time and AI inference. Some compute providers have given AI-specific estimates, but such standardised measures remain underreported and scarce (OECD, 2022[28]).
AI has advanced significantly thanks to increases in computer speeds and to Moore’s Law (i.e. computer speed and capability are expected to double every two years). However, experts have warned of potentially reaching the limits of such scaling, posing challenges for future leaps in computer performance (OpenAI, 2018[29]; Sevilla et al., 2022[30]). Researchers are looking at new ways to enable continued improvements. These include more powerful processors; faster data transmission; larger memory and storage; next-generation networks like 6G; and expanded and faster edge and distributed computing devices and quantum computing capabilities. Experts are also researching neuromorphic computing modelled after human cognition. This aims to make processors more efficient using orders of magnitude less power than traditional computing systems (Schuman et al., 2022[31]). Computing methods like optical computing – technology harnessing the unique properties of photons – are also being explored for AI applications (Wu et al., 2023[32]).
The availability of vast amounts of data for training AI has greatly enhanced systems’ capabilities, including their ability to generate realistic content
Copy link to The availability of vast amounts of data for training AI has greatly enhanced systems’ capabilities, including their ability to generate realistic contentAccess to data is crucial for AI, including for training, testing and validation. The demand for data to train AI (“AI input data”) has increased significantly, particularly with the rise of generative AI and large language models. Today, data used to train AI are aggregated from a variety of sources, such as through curated datasets, data-sharing agreements, collecting user data, using existing stored data and “data scraping” of publicly available data from the Internet.
The availability and collection of data raise policy questions. This is especially true when data contain personally identifiable information (i.e. personal data) or protected material, such as that under licence or copyright (e.g. software, text, images, audio or video). The limited availability of digitally readable text for non-English languages could also limit the benefits of AI for linguistic groups, including those using minority languages. Based on open-source AI training datasets available on Hugging Face, English represents more than half of all languages. This points to a potential diversity gap in terms of training dataset languages for AI (OECD.AI, 2024[33]) (Figure 2.2).
Figure 2.2. More than half of open-source AI training datasets are in English
Copy link to Figure 2.2. More than half of open-source AI training datasets are in EnglishPercentage breakdown of languages for open-source AI training datasets on Hugging Face from a list of 225 languages, 2024
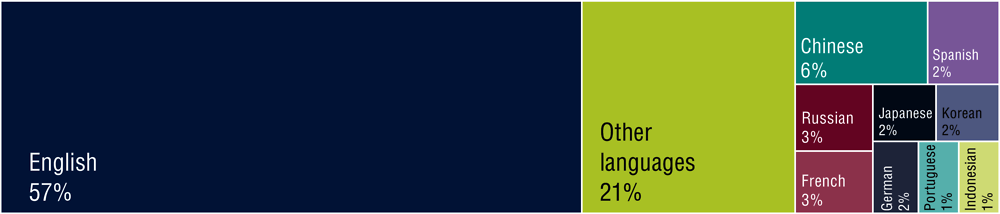
Notes: This chart represents the language distribution of all datasets. Multilingual and translation datasets on Hugging Face contain more than one language and are thus double counted. More methodological information available at: https://oecd.ai/huggingface (accessed on 10 March 2024).
Source: OECD.AI (2024[33]), using data from Hugging Face. For more information, please see: https://oecd.ai/en/data?selectedArea=ai-models-and-datasets.
Privacy-enhancing technologies are emerging, including confidential computing methods and federated learning
Copy link to Privacy-enhancing technologies are emerging, including confidential computing methods and federated learningThe use of vast amounts of personal data in some AI training data raises policy questions around the protection of privacy rights. Several techniques are emerging to help preserve privacy when using large datasets (e.g. for machine learning) (OECD, 2023[34]). These “privacy-enhancing technologies” (PETs) can help implement privacy principles such as data minimisation, use limitation and security safeguards. For example, “confidential computing” methods help ensure that companies hosting or accessing data in the cloud or through edge devices cannot view the underlying data without unlocking it with controlled encryption methods (O’Brien, 2020[35]; Mulligan et al., 2021[36]). Another example is “federated learning”, where users can each train a model using data on their own device. The data are then transferred to a central server and combined into an improved model that is shared back with all the users (Zewe, 2022[37]). Researchers are also developing methods of training machine-learning models over encrypted data. For example, in homomorphic encryption, the underlying data remains undisclosed during the entire training process. This strengthens privacy protections, while allowing for data to be used effectively (OECD, 2019[3]).
The potential of PETs to protect confidentiality of personal and non-personal data is recognised and applications are maturing. However, many PETs are still at the research stage and not yet scaled-up and used in production for major consumer-facing AI systems. It is also generally recognised that use of PETs can still be associated with privacy breaches and thus should not be regarded as a “silver bullet” solution.
The use of synthetic data has attracted significant interest as a PET approach. Synthetic data are generated via computer simulations, machine-learning algorithms, and statistical or rules-based methods, while preserving the statistical properties of the original dataset.1 Synthetic data have been used to train AI when data are scarce or contain confidential or personally identifiable information. These can include datasets on minority languages; training computer vision models to recognise objects that are rarely found in training datasets; or data on different types of possible accidents in autonomous driving systems (OECD, 2023[25]). However, challenges remain. For example, “[r]e-identification is still possible if records in the source data appear in the synthetic data” (OPC, 2022[38]). Furthermore, similar to anonymisation and pseudonymisation, synthetic data can also be susceptible to re-identification attacks (Stadler, Oprisanu and Troncoso, 2020[39]).
Generative AI could lead to more representative datasets but also raises concerns about manipulation
Copy link to Generative AI could lead to more representative datasets but also raises concerns about manipulationSome types of AI, like generative AI, can also produce new data, creative works and inferences or predictions about individuals or a topic that could serve as AI input data (i.e. “AI output data” for training) (Staab et al., 2023[40]). Such AI-generated data may not preserve the statistical properties of the original data and therefore should not be conflated with synthetic data. Nevertheless, the ability of AI to generate new data and content has been highlighted as an opportunity for generating larger and eventually more representative datasets that could be used for training. However, emerging research finds that using AI-generated content can degrade AI models over time. This leads to “model collapse” where the use of model-generated content in training causes “irreversible defects” in the model’s results, causing models to “forget” (Shumailov et al., 2023[41]). This is particularly worrisome if training data are collected by “scraping” the Internet without the ability to verify whether a given data sample was AI-generated.
AI-generated content can also be manipulated for various harms, such as for mis- and dis-information campaigns and influencing public opinion (OECD, 2023[25]). Current research suggests there is no reliable way to detect AI-generated content, even by using watermarking schemes or neural network-based detectors. This could allow for AI-generated content to be spread widely without detection, enabling sophisticated spamming methods, mis- and dis-information like manipulative fake news, inaccurate document summaries and plagiarism (Sadasivan et al., 2023[42]). Bad actors could engage in large-scale and low-cost “data poisoning”. In these cases, training datasets are “poisoned” by inaccurate or false data, changing a model’s behaviour or reducing its accuracy. For example, datasets could be poisoned to deliver false results in all or specific situations.
Regulation around generative AI is emerging in response to such tools becoming widely available at the end of 2022. However, the lag in policy, implementation, and enforcement of such responses around the world, may result in damage to the quality of public discourse online and the quality of information itself on the Internet. This damage may be difficult, if not impossible, to rectify in the years ahead (OECD, 2023[43]).
Open-source resources can make progress more broadly accessible but introduce other challenges
Copy link to Open-source resources can make progress more broadly accessible but introduce other challengesMany resources and tools for AI development are available as open-source resources. This facilitates their widespread adoption and allows for crowdsourcing answers to questions. Tools include TensorFlow (Google) and PyTorch (Meta) (open-source libraries for the programming language Python). Such tools can be used to train neural networks in computer vision and object detection applications. Some companies and researchers also publicly share curated training datasets and tools to promote AI diffusion (OECD, 2019[3]). Open-source developers have adapted and built on notable AI models with impressive speed in recent years (Benaich et al., 2022[44]).
The open-source AI community uses platforms like GitHub and Hugging Face to access open-source datasets, code and AI model repositories, and to exchange information on AI developments. Between 2012 and 2022, the number of open-source AI projects worldwide (measured by AI-related GitHub “repositories” or “projects”), grew by over 100 times, showcasing the increasing popularity of open-source software platforms (OECD.AI, 2023[45]). Training AI has become more accessible through low or no-code paradigms, where user-friendly interfaces substantially lower the barrier to entry for training and using AI (Marr, 2022[46]).
Although several AI firms operate proprietary AI models and systems and commercialise their access, some – such as Meta’s language model LLaMA 2 (Meta, 2023[47]) – make them available as open-source. The emergence of several open-source AI models contributes to more rapid innovation and development. This could mitigate “winner-take-all dynamics” that lead a few firms to seize significant market share (Dickson, 2023[48]). Yet open-sourcing entails other potentially significant risks, including non-existent or weak safeguards against use by bad actors.
Computer vision capabilities continue to develop, but applications like facial recognition raise concerns
Copy link to Computer vision capabilities continue to develop, but applications like facial recognition raise concernsSince its emergence in the 1960s, computer vision has been key to developing AI that can perceive and react to the world (Russell and Norvig, 2016[11]). Advances in face detection and recognition or image classification underpin significant technological progress in areas like image captioning and image translation (the process of recognising characters in an image to extract text contained in the image). Some computer vision technologies are mature, like face detection and recognition, or image classification.
Facial recognition, a key application enabled by computer vision, has received significant attention in public debate and in policy circles as countries move to create laws to govern AI. In many jurisdictions, facial recognition has been central to discussions on “high-risk” applications of AI, including the question of its use by law enforcement. Risks of algorithmic bias and data privacy concerns have resulted in various calls and actions to limit certain uses of facial recognition technology, such as in public spheres (OECD, 2021[49]). Previous analysis of commercial AI-enabled facial-recognition technologies revealed their greater accuracy when identifying light-skinned and male faces compared to darker-skinned and female faces (Buolamwini and Gebru, 2018[50]; Anderson, 2023[51]). In many cases, this bias is caused by using large training datasets that lack representative population samples from diverse groups. This highlights the important role that synthetic data can play in generating more representative datasets for training. Facial recognition technology has also been involved in mistaken identifications and wrongful arrests, predominantly in communities of colour (Benedict, 2022[52]). For example, a facial recognition system in China mistook a face on a bus for someone illegally crossing the street (“jaywalking”) (Shen, 2018[53]). These examples point to the potential for this powerful technology to be misused to harm specific population groups. Jurisdictions are considering stronger regulation, including prohibiting public authorities to use AI for biometric recognition.
Experts have identified key considerations for policy makers in ensuring trustworthy computer vision technology. First, policy makers should consider the data used for training the model, which have recently led to privacy, bias or copyright issues. Second, AI models themselves often require large computational architectures that consume significant amounts of energy and are complex. This raises issues like explainability because they are too complex for people to understand. Some researchers are attempting to improve explainability through methods such as “mechanistic interpretability” by “reverse-engineering” model elements to be understandable to humans (Conmy et al., 2023[54]). Third, policy makers should consider the impact of deploying AI in the real world, particularly around societal and economic impacts like fairness (OECD, 2023[25]).
Robots are getting better and smarter thanks to AI
Copy link to Robots are getting better and smarter thanks to AIRobots can be defined as “physical agents that perform tasks by manipulating the physical world” where mechanical components such as arms, joints, wheels and sensors, such as cameras and lasers, allow perception of an environment (Russell and Norvig, 2016[11]). AI can be “embodied” into robots to perform a wide range of tasks, including manufacturing and assembly, transportation and warehousing. It can also be used for various applications where human interaction might be dangerous or physically impossible, for example in emergency rescue contexts. Robotics can also be combined with image, audio and video generation models to produce advanced systems with multimodal capabilities combining these functions (Lorenz, Perset and Berryhill, 2023[10]).
Pending more advanced technology, the market for robotics may grow rapidly. Further technological developments are still needed for robotics to reach wide commercial markets. Some estimated the market for autonomous mobile robots at USD 2.7 billion in 2020. Projections put the market as high as USD 12.4 billion by 2030 (Allied Market Research, 2022[55]).
Researchers are also advancing with “neuro-morphic” robots, machines controlled by human brain waves rather than a voice command, for example. This could include brain chip implants and wearable robotic “exoskeletons” that use machine learning and sensors to gather and process data in real time (Lim, 2019[56]). AI-enabled digital twins, copies of systems or networks for modelling purposes, have also been explored in remote surgery applications alongside robots and virtual reality. Such environments need low network latency (i.e. fast networks) and high levels of security and reliability (Laaki, Miche and Tammi, 2019[57]). These developments could significantly advance the strength and reliability of prosthetic limbs, bring precision to remote surgery applications and even to robot-assisted surgery in space and remote areas, and other cutting-edge health care applications (Bryant, 2019[58]; Newton, 2023[59]).
Will scaling-up current AI models continue to drive advancements in AI capabilities?
Copy link to Will scaling-up current AI models continue to drive advancements in AI capabilities?In deep learning, research around “scaling laws” offers insights into predictable patterns for “scaling up” AI (i.e. advancing AI by training models with more parameters, compute and data, often bringing significant training costs). These approximate laws describe the relationship between an AI model’s performance and the scale of key inputs. For example, some researchers have observed an increase in the performance of language models with increases in compute, dataset size and parameter numbers (Kaplan et al., 2020[60]). Typically, larger language models perform better, pointing to a relationship between performance and scale.
However, models may not be able to scale in perpetuity if AI developers become limited by access to compute and data. As AI models scale with an increasing number of parameters, trade-offs may emerge, such as the cost of compute and increasing memory requirements. Some also argue that large language models based on probabilistic inference cannot reason and thus might never achieve completely accurate outputs (LeCun, 2022[61]). Consequently, some hypothesise that current model architectures may never allow for complete accuracy and generalisation.
Some experts also question whether AI chat-based search models – such as ChatGPT – will benefit from scaling laws, given the significant cost required to retrain their underlying models to keep them up to date so users can search for the most recent information (Marcus, 2023[62]). In addition, some research shows the scaling of models may outpace data availability in the next few years (Villalobos et al., 2022[63]).
Experts offer varying predictions for the future trajectories and implications of AI
Copy link to Experts offer varying predictions for the future trajectories and implications of AIAI research and policy discussions often cover existing AI challenges. Yet the long-term implications of rapidly advancing AI systems remain largely uncertain and fiercely debated. Experts raise a range of potential future risks from AI, some of which are already manifesting in various ways. At the same time, experts and others expect AI to deliver significant or even revolutionary benefits. Future-focused activities are critical to better understand AI’s possible long-term impacts and to begin shaping them in the present to seize benefits while mitigating risks (OECD, forthcoming[64]).
Strategic foresight and other future-oriented activities can support policy makers to anticipate possible futures and begin to actively shape them in the present. Strategic foresight is a structured and systematic approach to using ideas about the future to anticipate and prepare for change. It involves exploring different plausible futures, and associated opportunities and risks. It reveals implicit assumptions, challenges dominant perspectives and considers possible outcomes that might otherwise be ignored. Strategic foresight uses a range of methodologies, such as horizon scanning to identify emerging changes, analysing “megatrends” to reveal and discuss directions and scenario exploration to help engage with alternative futures (OECD, forthcoming[64]).
In November 2023, recognising that future AI developments could present both enormous global opportunities and significant risks, 28 governments and the European Union signed the Bletchley Declaration. In so doing, they committed to co-operation on AI, ensuring it is designed, developed, deployed and used in a manner that is safe, human-centric, trustworthy and responsible (AI Safety Summit, 2023[65]). The Declaration also committed signatories to convene a series of future AI Safety Summits. This gave further impetus to ensuring that potential future AI benefits and risks remain a topic of international importance and dialogue.
AI is expected to yield significant future benefits
Copy link to AI is expected to yield significant future benefitsWhile many headlines focus on AI risk, tremendous benefits are also expected to accrue. Through the G7 Hiroshima Process on Generative AI, G7 members unanimously saw productivity gains, promoting innovation and entrepreneurship, and unlocking solutions to global challenges as some of the greatest opportunities of AI technologies worldwide, including for emerging and developing economies. G7 members also emphasised the potential role of generative AI to help address pressing societal challenges. These include improving health care, helping solve the climate crisis and supporting progress towards achieving the United Nations Sustainable Development Goals (SDGs) (OECD, 2023[66]). The benefits AI may generate include:
Enhancing productivity and economic growth. Projections vary for how much AI may contribute to gross domestic product (GDP) growth. Some market research estimates the global AI market, including hardware, software and services, will have a compound annual growth rate of 18.6% between 2022 and 2026, resulting in a USD 900 billion market for AI by 2026 (IDC, 2022[67]). Others estimate the AI market could grow to more than USD 1.5 trillion by 2030 (Thormundsson, 2022[68]). For generative AI specifically, estimates include raising global GDP by 7% over a ten-year period (Goldman Sachs, 2023[69]).
Accelerating scientific progress. AI could spur scientific breakthroughs and speed up the rate of scientific progress (OECD, 2023[70]). AI can increase the productivity of scientists and overcome previously intractable resource bottlenecks, saving time and increasing resource efficiency (Ghosh, 2023[71]). Impacts in this area can already be seen, with AI driving progress in areas like nuclear fusion and generating new life-saving antibodies to disease (Stanford, 2023[72]; Yang, 2023[73]).
Strengthening education. AI may be able to provide personalised tutoring and other individualised learning opportunities that are currently only available to those who can afford them, increasing access to quality education for all (Molenaar, 2021[74]). On the teaching side, AI can potentially help teachers create materials and lesson plans tailored to students’ needs (Fariani, Junus and Santoso, 2023[75]).
Improving health care. Advances in AI technologies could transform many aspects of health care service and delivery. These include interventions at the individual level to provide people with information about their personal health, as well as those that improve diagnoses and alleviate workload for health care providers (Anderson and Rainie, 2018[76]; Davenport and Kalakota, 2019[77]).
Potential benefits of AI come with risks and uncertain future trajectories
Copy link to Potential benefits of AI come with risks and uncertain future trajectoriesExperts, civil society and governments have all identified many potential risks from AI. The Bletchley Declaration, for example, notes the potential for unforeseen risks, such as those stemming from dis-information and manipulated content. It also points to potential safety risks from highly capable narrow and general-purpose AI models. These could result in substantial or even “catastrophic harm” stemming from the most significant capabilities of leading-edge models.
Although attention has been placed on preventing negative outcomes from advanced AI systems in the medium- to long-term, some risks are already apparent. Early efforts to track AI incidents found that generative AI-related incidents and hazards reported in the press have increased steeply since 2022 (Figure 2.3) (OECD, 2023[66]). G7 members see risks of mis- and dis-information, intellectual property rights infringement, and privacy breaches as major threats stemming from generative AI in the near term (OECD, 2023[66]).
Figure 2.3. Generative AI-related incidents and hazards reported by reputable news outlets have increased steeply since 2022
Copy link to Figure 2.3. Generative AI-related incidents and hazards reported by reputable news outlets have increased steeply since 2022Number of generative AI-related incidents and hazards, three-month moving average 2019-23
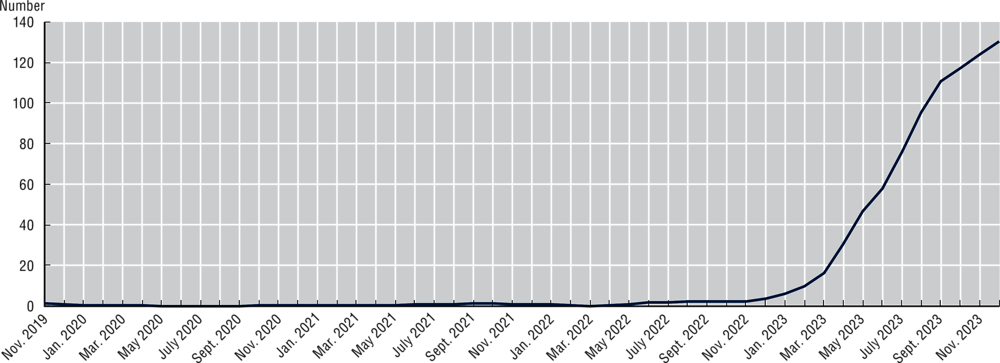
Notes: This chart shows the three-month moving average of real count of incidents and hazards reported. Results might differ from previous analysis due to modifications in the methodology of clustering articles into incidents.
Source: OECD.AI (2024[78]), AI Incidents Monitor (AIM), using data from Event Registry. Please see: www.oecd.ai/incidents for more information.
AI could amplify mis- and dis-information on a considerable scale. Generative AI can produce novel images or video from text prompts or produce audio in various tones and pitches with relatively limited input (such as a short recording of a human voice). Already today, generative AI outputs can be challenging to distinguish from human creation (Kreps, Mccain and Brundage, 2022[79]), raising policy implications about the ease of using AI to produce mis- and dis-information – fake news, deep fakes and other convincing manipulated content (Sessa, 2022[80]). Challenges may be particularly acute on issues related to science, such as vaccine effectiveness and climate change, and in polarised political contexts. Mitigation measures are emerging, such as watermarking and AI-enabled deep fake detection, but current approaches have limitations and may be insufficient to address future challenges (Lorenz, Perset and Berryhill, 2023[10]). Over time, some experts argue that increasingly advanced AI systems could fuel mass persuasion and manipulation of humans (Anderson and Rainie, 2018[76]). This, in turn, could cause material harm at the individual and societal levels, eroding societal trust and the fact-based exchange of information that underpins science, evidence-based decision making and democracy (OECD, 2022[81]).
AI hallucinations, information pollution and data poisoning harm data quality and erode public trust. AI outputs may decrease the quality of data online, as they exhibit hallucinations (making up facts in a credible way, often when a correct answer is not found in the training data). This can contribute to information pollution, allowing AI-produced data to harm the online “commons” and produce a vicious cycle whereby AI is trained on lower quality data produced by AI (Lorenz, Perset and Berryhill, 2023[10]). Bad actors could also use AI to sabotage or ruin robust data (i.e. data poisoning) (OECD, 2023[43]).
AI could significantly disrupt labour markets. Advanced AI could help people find jobs but also displace highly skilled professionals, or at the very least, change the nature of some jobs. Advanced AI can be designed to assist humans in the labour market, including with “collaborative robots” or “cobots”. However, some research and experts have raised concerns that increasingly capable AI, such as AI that can act as autonomous agents, could in some cases replace high-skilled and high-wage tasks (e.g. programmers, lawyers or doctors), leading to economic and social disruption (Russell, 2021[82]; Clarke and Whittlestone, 2022[83]; Metz, 2023[84]). Even if AI does not result in net job losses the task composition of jobs is likely to evolve significantly, changing skills needs (OECD, 2023[85]; 2023[86]).
Significant risks could emerge by embedding AI in critical infrastructure. AI is increasingly embedded in critical infrastructure because it can improve timeliness and efficiency, safety and/or reliability, or reduce costs (Laplante et al., 2020[87]). However, some argue this could enable bad actors using AI to cause physical or virtual harm (Zwetsloot and Dafoe, 2019[88]; OECD, 2022[89]). AI deployed in critical infrastructure, like in chemical or nuclear plants, could pose serious risks to safety and security if AI models and systems prove unreliable or unsafe.
AI models and systems can exacerbate bias and inequality. AI can echo, automate and perpetuate social prejudices, stereotypes and discrimination by replicating biases, including those contained in training data, in their outputs. This could further marginalise or exclude specific groups (Bender et al., 2021[90]; NIST, 2022[91]). For example, generative AI tools have been shown to produce sexualised digital avatars or images of women, while portraying men as more professional and career oriented (Heikkilä, 2022[92]). Biases involving specific religions have also been found (Abid, Farooqi and Zou, 2021[93]). As AI becomes more complex, it could also exacerbate divides between advanced and emerging economies, creating inequalities in access to opportunities and resources. “Automation bias” – the propensity for people to trust AI outputs because they appear rational and neutral – can contribute to this risk when people accept AI results with little or no scrutiny (Alon-Barkat and Busuioc, 2022[94]; Horowitz, 2023[95]). At the same time, AI tools can help human operators interpret and question complex AI decisions, discouraging overreliance. More generally, inequal access to AI resources risks creating and deepening inequality both within and between countries (e.g. between developed and emerging economies).
Generative AI raises data protection and privacy concerns. The vast amounts of data used to train AI, especially generative AI, raise data protection and privacy concerns. The processing of personal data for training purposes or its use for automated decision making may conflict with data protection regulations. For example, where data are used for automated decision-making, the European Union General Data Protection Regulation requires the operator to provide “meaningful information about the logic involved”. This may pose challenges with AI trained by processing information in “black box” neural networks that humans cannot understand or replicate.
Intellectual property rights represent another area of challenges and unknowns. Generative AI in particular raises issues around intellectual property rights. This could include unlicensed content in training data; potential copyright, patent and trademark infringement of AI creations; and ownership questions around AI-generated works. Generative AI is trained on massive amounts of data from the Internet that include copyrighted data, often without authorisation of the rights-owners. Whether this is permissible is being discussed across jurisdictions, with numerous court cases under way (Zirpoli, 2023[96]). Legal decisions will set precedents and affect the ability of the generative AI industry to train models. Because legal systems around the world differ in their treatment of intellectual property rights, the treatment of AI-generated works also varies internationally (Murray, 2023[97]). Although most jurisdictions agree that works generated by AI are not copyrightable (Craig, 2021[98]), views could shift as AI becomes ubiquitous.
Many solutions are being proposed to help yield AI’s benefits and mitigate its challenges
Copy link to Many solutions are being proposed to help yield AI’s benefits and mitigate its challengesActions are needed to answer unknowns and shape potential AI futures. AI researchers, experts and philosophers have proposed many potential solutions to enable future benefits, while mitigating risks. The OECD, through its Expert Group on AI Futures, has identified nearly 70 potential solutions being explored and analysed.2 Surveyed expert group members agreed on the most important potential solutions listed below:
Liability rules for AI-caused harms;
Requirements that AI discloses that it is an AI when interacting with humans;
Research and development (R&D) on approaches to evaluating the capabilities and limitations of AI systems and preventing accidents, misuse or other harmful consequences;
AI red-lines, such as regulation prohibiting certain AI use cases or outputs; and
Controlled training and deployment of high-risk AI models and systems.
Other solutions include moratoriums or bans on advanced AI, and international statements or declarations on managing potential risks from AGI. These proposals have gathered less widespread agreement among expert group members.
Demystifying debates on maintaining human control of AI systems and on the alignment of AI systems and human values
Copy link to Demystifying debates on maintaining human control of AI systems and on the alignment of AI systems and human valuesThe risk that humans lose control of AI systems received significant attention in 2023 both in news media and at the AI Safety Summit hosted by the United Kingdom. However, the topic is controversial. In a 2023 survey of the OECD Expert Group on AI Futures, respondents rated loss of control as both one of the most important and least important risks of AI.
As AI systems become more capable and are assigned more responsibility over important tasks, some experts believe that a lack or misalignment of shared values and goals between humans and machines could lead AI systems to act against the interests of humans. This concern has spawned “AI alignment” work to ensure the behaviour of AI systems aligns consistently with human preferences, values and intent (Dietterich and Horvitz, 2015[99]; Russell, 2019[100]; Bekenova et al., 2022[101]; Dung, 2023[102]).
It can be difficult to discern whether an AI system is pursuing objectives that align with its creators’ intent and goals. For example, such issues have begun to emerge through “reward hacking”. In these cases, a model finds unforeseen and potentially harmful ways of achieving its objective while exploiting reward signals (Cohen, Hutter and Osborne, 2022[103]; Skalse et al., 2022[104]). In trying to increase user engagement, for example, AI systems managing content on social media could promote articles that spread extreme views and keep users engaged. However, humans may benefit most from balanced and non-polarised content, and real human preferences may reflect this belief (Grallet and Pons, 2023[105]).
A number of experts believe that sufficiently robust methods are not available today to ensure that AI systems align with human values (OECD, 2022[89]). They consider this perceived gap to be one of the top “unsolved problems” in AI governance (Hendrycks et al., 2022[106]). In the 2023 survey of members of the OECD Expert Group on AI Futures, respondents ranked the absence of robust methods to ensure alignment between AI systems’ outputs and human values as one of the most important potential future risks. In connecting the concepts of alignment and control, some AI experts believe the negative consequences already witnessed in relatively basic AI systems could be taken to extremes. They argue this could happen if highly capable AI systems were developed in ways that unfold so quickly that humans cannot maintain control, for example if machines gained the ability to improve themselves independently (Cotton-Barratt and Ord, 2014[107]).
As another underlying issue, AI systems can be highly efficient at pursuing human-defined objectives but in ways their developers did not always anticipate. To achieve these aims, AI systems could develop subgoals (i.e. means to an end) that differ dramatically from the values and intent that underpin human-defined objectives. Some argue this could even lead to machines resisting being switched off since this could be considered counter-productive to the achievement of objectives (Russell, 2019[100]). However, as mentioned in Box 2.2, achieving AGI – the level of advancement hypothesised as needed for such scenarios – is hypothetical and highly controversial. Some experts argue that achieving AGI itself might be an exceedingly complex and challenging task. This would make the notion of misalignment a premature concern lacking agreed-upon definitions and clear boundaries (LeCun, 2022[109]). Research on such topics can be challenging, including a limited number of peer-reviewed articles, lack of appropriate modelling techniques and lack of specific definitions and standardised terminology (McLean et al., 2021[110]).
Policy considerations for a trustworthy AI future
Copy link to Policy considerations for a trustworthy AI futureThe rapid progress in AI capabilities has not yet been matched by assurances that AI is trustworthy and safe. AI is advancing rapidly and could produce unsafe outputs, especially as AI-enabled products are transferred from research contexts to consumer use before the full consequences and risks of their deployment are understood. Advanced AI techniques such as deep learning pose specific safety and assurance challenges: technologists and policy makers alike do not fully understand their functioning and therefore cannot use traditional guardrails to ensure reliability. Lack of explainability inhibits understanding of how AI generates outputs in the form of predictions, content, recommendations or decisions. This, in turn, creates issues related to transparency, robustness and accountability. “Narrow” AI focuses on optimising outcomes for well-defined contexts. However, the increasingly generality of AI applications makes it difficult to train advanced models and systems to produce an appropriate response for every relevant scenario.
There is little understanding or agreement on how significant future AI risks may be. Experts should build awareness and understanding of AI risks, particularly among policy makers, and identify key sources of these risks. To help mitigate some AI risks, improved methods to interpret and assure AI are needed. In addition, policy makers need to ensure that developers can deploy models and systems that operate reliably as intended, even in novel contexts.
Where are countries in the race to implement trustworthy AI?
Copy link to Where are countries in the race to implement trustworthy AI?AI research and development priorities feature prominently on national policy agendas, with investments poised to continue in the years ahead
Copy link to AI research and development priorities feature prominently on national policy agendas, with investments poised to continue in the years aheadAI R&D investments feature prominently in national AI strategies. Governments and firms are redoubling efforts to leverage AI for productivity gains and economic growth and such trends are poised to continue. Entirely new business models, products and industries emerge from AI-enabled R&D breakthroughs. This underscores the importance of basic research, especially as policies emerge to consolidate R&D capabilities to catch up with the leading players: China, the United States and the European Union (OECD.AI, 2023[111]).
Progress in AI R&D can be measured by examining to what extent countries publish AI research. The United States and European Union have had steady growth in the number of AI research publications over past decades. These include journal articles, books, conference proceedings and publications in academic repositories like arXiv. Meanwhile, AI publications have increased dramatically in China, with India also making strides in recent years (Figure 2.4). Since mid-2019, China has published more AI research than either the United States or the European Union. India has also made significant advances, more than doubling its number of AI research publications since 2015 (OECD.AI, 2023[111]).
Figure 2.4. China, the European Union and the United States lead in the number of AI research publications, with India recently making strides
Copy link to Figure 2.4. China, the European Union and the United States lead in the number of AI research publications, with India recently making stridesNumber of AI research publications, 2000-23
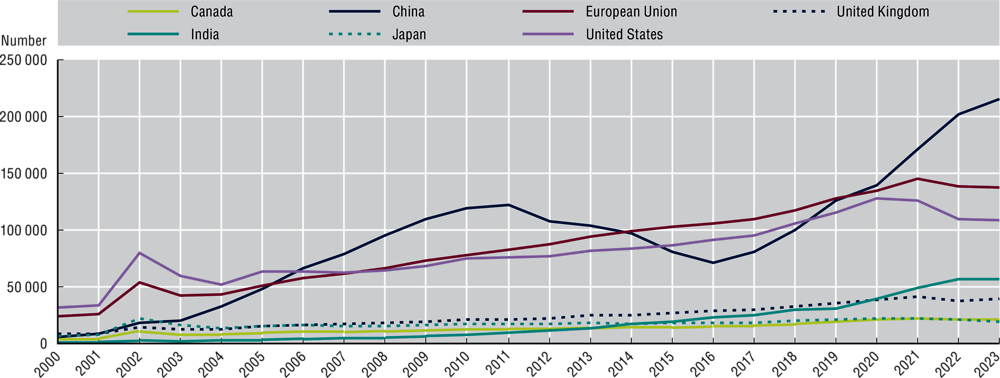
Notes: This figure shows the number of AI research publications for a sample of top countries for 2000-23. OpenAlex publications are scholarly documents such as journal articles, books, conference proceedings and dissertations.
Source: OECD.AI (2023[111]) using data from OpenAlex available at: www.oecd.ai/en/data?selectedArea=ai-research. StatLink contains more data.
The number of AI publications alone does not provide a full picture of publication quality and impact. The number of citations can be a proxy indicator for “impact”, with increased citations possibly indicating a higher impact. Since 2022, the share of total high-impact AI research publications in the United States has declined, while China’s share has steadily risen. Notably, China had overtaken the United States and European Union by 2019 (Figure 2.5). In 2023, with respect to institutions most active in publishing AI research, seven of the ten leading AI research institutions were based in China, with one institution each in France, the United States and Germany3 (OECD.AI, 2023[111]).
Figure 2.5. China’s share of “high-impact” AI publications has steadily risen since 2000, notably overtaking the United States and European Union in 2019
Copy link to Figure 2.5. China’s share of “high-impact” AI publications has steadily risen since 2000, notably overtaking the United States and European Union in 2019Percentage of high-impact AI research publications by jurisdiction, 2000-23
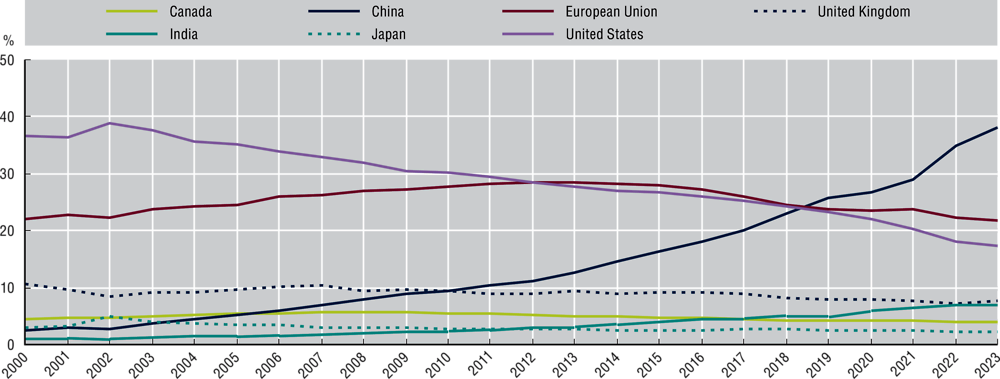
Notes: This figure shows the percentage of “high-impact” AI research publications for a sample of top countries for 2000-23. OpenAlex publications are scholarly documents such as journal articles, books, conference proceedings and dissertations. A publication’s impact is calculated by dividing the number of citations by the average citations in the subdiscipline, discounted by the number of years since the publication was published, so that older publications that have been around for longer are discounted accordingly. Publications are classified as “high impact” if its score falls in the highest quartile.
Source: OECD.AI (2023[111]) using data from OpenAlex available at: www.oecd.ai/en/data?selectedArea=ai-research.
Countries dedicate significant funding for AI R&D through different instruments. The main trends include launching AI R&D-focused policies, plans and programmes, establishing national AI research institutes and centres, and consolidating AI research networks and collaborative platforms. Highlights from France, Korea, Türkiye, the United States, the European Union and China appear below.
France established its 2018 National AI Research Programme out of its National AI Strategy. The programme represented 45% of the National AI Strategy budget (about EUR 700 million) (INRIA, 2023[114]).
In 2021, Korea announced the AI promotion plan on the theme of “AI into all regions for our people”. It focuses on creating an “AI cluster village” in Gwang-ju city to serve as a base for AI innovation as well as projects across the country considering each region’s key strengths and industries (Ministry of Science and ICT, 2021[115]).
Türkiye has been funding AI R&D projects and programmes by launching calls for proposals or awarding grants, including multidisciplinary research that encourages collaboration across relevant fields. The Scientific and Technological Research Council of Türkiye and the National AI Institute have funded more than 2 000 R&D and innovation projects. Total funding for academic R&D projects amounted to more than USD 50 million. Meanwhile, industrial R&D projects received funding of more than USD 150 million (TÜBITAK, 2023[116]).
The United States introduced initiatives to retain its leadership position in AI R&D through the United States National AI Initiative Act of 2020. In 2023, the National AI Initiative Office helped manage billions of dollars for non-defence and defence-related AI R&D (NSTC, 2023[112]), following the 2016 and 2019 National AI R&D Strategic Plan. The 2023 budget includes funding for the National Science Foundation (NSF) that has long been supporting AI research, including through dedicated AI research grants. In 2018, the Defense Advanced Research Projects Agency announced an AI R&D investment of more than USD 2 billion. The NSF and the White House Office of Science and Technology Policy (OSTP) recently proposed a National AI Research Resource (NAIRR) to provide researchers access to critical computational, data, software and training resources to support AI R&D (NAIRR Task Force, 2023[113]).
The European Union allocated EUR 1 billion per year for AI, including for R&D, within the broader EUR 100 billion budget for the Horizon Europe and Digital Europe programmes (European Commission, 2023[117]). This follows the Co-ordinated Plan on Artificial Intelligence, which was released in 2018. In this plan, member states emphasise co-ordination in AI R&D to maximise impact, including “shared agendas for industry-academia collaborative AI R&D and innovation” (OECD.AI, 2024[118]).
In 2018, China invested an estimated USD 1.7-5.7 billion in AI R&D spending. This estimate includes basic research through the National Natural Science Foundation of China and applied research through the National Key R&D Programmes. While precise estimates are challenging to validate, some researchers believe that China’s AI R&D budget is closer to the upper bound estimate. Moreover, they believe it likely to have increased in recent years (Acharya and Arnold, 2019[119]). This investment is aligned with the country’s ambitions, set in the 2017 New Generation AI Plan, to become the world’s primary innovation centre by 2030.
Countries also establish national AI research institutes and research centres:
The Australian AI Action Plan allocated USD 124 million to establish a National AI Centre to further develop AI research and commercialisation (DISR, 2021[120]).
Canada’s Pan-Canadian AI Strategy emphasises research and talent, with the Canadian Institute for Advanced Research leading the strategy for the Government of Canada and three AI Institutes.
Japan’s AI R&D Network provides opportunities to exchange information among AI researchers and promote co-operation in R&D in Japan and abroad.
The Research Institutes of Sweden combine AI research with interdisciplinary research, a wide range of test beds, innovation hubs and educational programmes.
Established in 2020, the National Artificial Intelligence Institute of Türkiye serves as a driving force in disseminating AI. Acting as a bridge between academic research and industry needs, the Institute serves as a key stakeholder in the implementation of Türkiye’s National AI Strategy (2021-2025) (Scientific and Technological Research Council of Türkiye, 2023[121]).
The United States National Science Foundation-led National AI Research Institutes Program is the nation’s largest AI research ecosystem. It is supported by a partnership of federal agencies and industry leaders (National Science Foundation, 2023[122]).
Brazil’s Ministry of Science, Technology and Innovation and partners are investing BRL 1 million annually for ten years to create up to eight AI Applied Research Centres, with partner firms matching investments.
India’s 2021 Kotak-IISC AI-Machine Learning Centre offers education on AI and machine learning research (OECD.AI, 2021[123]). Other initiatives focus on reinforcing collaborative networks of experts and researchers.
While global venture capital investments in AI and overall have declined since 2021, investments in generative AI start-ups have boomed
Copy link to While global venture capital investments in AI and overall have declined since 2021, investments in generative AI start-ups have boomedThe annual value of global venture capital (VC) investments in AI start-ups4 more than tripled between 2015 and 2023 (from more than USD 31 billion to nearly USD 98 billion) (Figure 2.6) (OECD.AI, 2024[124]). Notably, between 2020 and 2021, such investments jumped by over 2.3 times (from about USD 92 billion to USD 213 billion). Most of the funds flowed to AI firms in the United States and China. The United States has the advantage of a strong market for private sector R&D and VC funding, accounting for more than two-thirds of global private sector investment in software and computer services in 2022 (European Commission, 2023[125]).
Following trends in the global VC market, overall VC investments in AI firms experienced a significant drop of over 50% between 2021 and 2023 (from USD 213 billion to USD 98 billion (OECD.AI, 2024[124]). This reflects broader VC trends, with investors exercising caution after the technology boom of the COVID-19 pandemic, rising interest rates and inflationary pressures. Generative AI start-ups are one exception to this cooling trend in VC investments, jumping from USD 1.3 billion in 2022 to USD 17.8 billion in 2023, a large increase from 1% of total AI VC investments to 18.2%. The rise was spurred largely by Microsoft’s USD 10 billion investment in OpenAI (Figure 2.6) (OECD, 2023[66]).
VC investments in AI have focused on different areas over the past decade. In 2012 and 2013, the largest investments were made in media and marketing, and in IT infrastructure and hosting. The following years saw a VC boom in AI for mobility and autonomous vehicles, but its share of total VC investments declined significantly after 2020. Unsurprisingly, the pandemic years saw an increase in VC investments in AI for health care, drugs and biotechnology (OECD.AI, 2023[126]).
Figure 2.6. VC investments in generative AI start-ups have boomed since 2022, while VC investments overall and in AI start-ups reached a peak in 2021
Copy link to Figure 2.6. VC investments in generative AI start-ups have boomed since 2022, while VC investments overall and in AI start-ups reached a peak in 2021VC investments in AI and generative AI start-ups, 2012-23
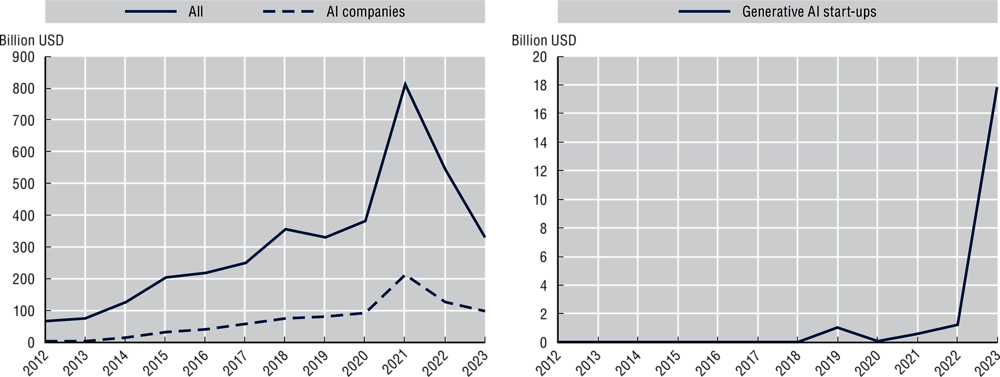
Notes: AI start-ups are identified based on Preqin’s cross-industry and vertical categorisation, as well as on OECD’s automated analysis of the keywords contained in the description of the company’s activities. AI keywords used include: generic AI keywords, such as “artificial intelligence” and “machine learning”; keywords pertaining to AI techniques, such as “neural network”, “deep learning”, “reinforcement learning”; and keywords referring to fields of AI applications, such as “computer vision”, “predictive analytics”, “natural language processing”, “autonomous vehicles”. Please see: https://oecd.ai/preqin for more information.
Source: OECD.AI (2024[124]) using data from Preqin. Also available at: www.oecd.ai/en/data?selectedArea=investments-in-ai-and-data.
Governments are building human capacity for AI as demand for AI skills grows
Copy link to Governments are building human capacity for AI as demand for AI skills growsAI is changing the nature of work. Countries have realised that both managing a fair labour market transition and leading in AI R&D and adoption requires solid policies to build human capacity and attract top talent. The AI workforce has grown considerably, almost tripling as a share of employment from less than a decade ago (Green and Lamby, 2023[127]). However, according to LinkedIn in 2023, men are about twice as likely to work in an AI occupation or report AI skills as women. This suggests a gendered skills gap in global AI labour markets (OECD.AI, 2023[128]).
AI talent is in high demand and remains a mobile workforce across economies, with countries competing for the small pool of highly skilled AI workers. For example, economies like Luxembourg, Canada, Germany and Japan attracted more AI talent than they lost in 2022 (Figure 2.7). In contrast, countries like India, Greece and Lithuania saw a net outflow of AI talent from their borders. This might indicate an AI “brain drain”, where highly specialised workers move to another country for improved employment opportunities. India is noteworthy for its considerable AI talent growth rate in recent years. This rate is ahead of the United States, United Kingdom and Canada, as measured by the year-over-year change in LinkedIn members declaring to have AI skills (OECD.AI, 2022[129]).
With industry outpacing academia in developing advanced AI, countries increasingly see AI compute capacity as a crucial resource to be managed
Copy link to With industry outpacing academia in developing advanced AI, countries increasingly see AI compute capacity as a crucial resource to be managedThe demand for AI compute has grown dramatically as shown by valuations in the AI chip market. In 2021, some called the unfolding AI compute trends an “AI chip race”; the market value for AI chips was estimated at more than USD 10 billion with revenues expected to reach nearly USD 80 billion by 2027 (Pang, 2022[130]). Large technology companies have invested significantly to join the race.
The growing focus on AI compute is driven by a desire from AI developers to obtain the specialised hardware and software needed to train advanced AI. Industry, rather than academia, increasingly provides and uses compute capacity and specialised labour for state-of-the-art machine-learning research and for training AI models with a high number of parameters (Ahmed and Wahed, 2020[131]; Ganguli et al., 2022[132]; Sevilla et al., 2022[30]). Compute divides may emerge and worsen between the public and private sectors because, increasingly, public sector entities lack resources to train advanced AI (OECD, 2023[8]).
Figure 2.7. Advanced economies are competing for AI talent
Copy link to Figure 2.7. Advanced economies are competing for AI talentBetween-country AI skills migration in 2019 and 2022
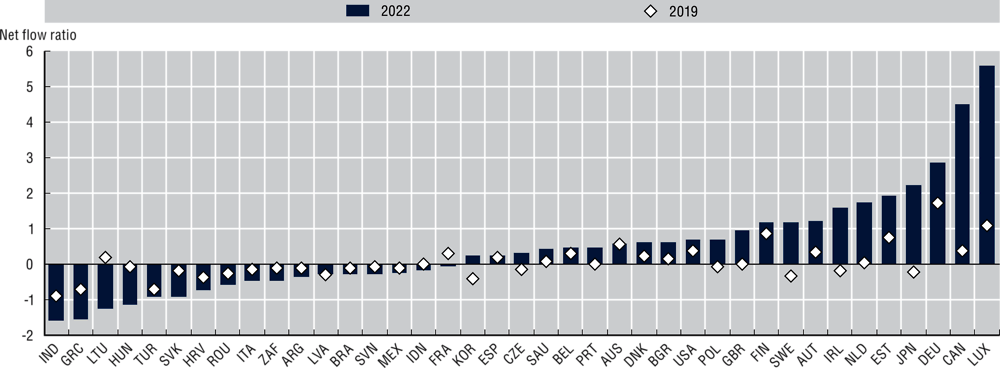
Notes: This chart displays the net migration flows per 10 000 LinkedIn members with AI skills in 2019 and 2022 for a selection of countries with 100 000 LinkedIn members or more declaring to have AI skills both in 2019 and 2022. Migration flows are normalised according to LinkedIn country membership.
Source: OECD.AI (2022[129]) using data from LinkedIn, also available at: www.oecd.ai/en/data?selectedArea=ai-jobs-and-skills.
Based on the number of “top supercomputers”, the United States and China have led in compute capacity since 2012. According to the November 2023 Top500 list,5 35 economies have a top supercomputer. The highest concentration occurs in the United States (32%), followed by China (21%), Germany (7%), Japan (6%), France (5%) and the United Kingdom (3%). The remaining 29 economies on the list represent a combined 26% of supercomputers (Top500, 2023[133]). The United States and China have led in the number of supercomputers on the Top500 list since 2012, with China increasing its number of supercomputers significantly since 2015.
Analysis of top supercomputers through the Top500 list is a proxy for measuring national compute capacity. However, not all supercomputers on the list are specialised and used for AI. Moreover, some economies have slowed or refrained from submitting data to the list in recent years, posing challenges for cross-country comparisons. In addition, counting the number of supercomputers only provides a partial picture of national compute capacity because some supercomputers are more powerful than others.
Another proxy for national compute capacity is the share of the most powerful computers6 on the Top500 list. The United States has the highest concentration of supercomputers among the world’s leading economies with 53%, followed by Japan (10%) and China (6%) (Top500, 2023[133]). Such concentration of supercomputers is a proxy measure for benefits and risks from AI hardware “economies of scale”. On the one hand, high concentration creates efficiencies by pooling resources in hyperscale computing centres. On the other, it indicates possibly worsening “compute divides” where leading economies have greater resources to invest in R&D and benefit from it, solidifying their leading positions for the foreseeable future.
Countries are striving to increase AI compute capacity available for research and academia, in addition to taking stock of their national AI compute capacity and needs:
Canada’s Pan-Canadian AI Strategy (2017, 2021) leverages a national network of AI research institutes and supports acquisition of high-performance computing capacity for AI research. In 2020, the first Canadian Digital Research Infrastructure Needs Assessment was launched to identify future digital research infrastructure and service needs (Digital Research Alliance of Canada, 2020[134]).
Korea’s K-Cloud Project aims to manufacture and deploy world-class AI chips domestically to provide improved national cloud computing infrastructure.
The Turkish National e-Science e-Infrastructure (TRUBA) in Türkiye provides high-performance computing and data storage to research institutions and researchers. TRUBA seeks to expand and strengthen computing and data storage resources for researchers. It has a national network operating throughout the country (TRUBA, 2023[137]).
In 2022, the United Kingdom conducted the Future of Compute review to examine its digital research infrastructure needs, including for AI. It called for an integrated compute ecosystem and significant investment in public AI infrastructure (The Alan Turing Institute, 2022[135]).
The United States aims to make world-class computing resources and datasets available to researchers through the proposed NAIRR, supported by the 2023 United States Executive Order on AI (The White House, 2023[136]).
The European High-Performance Computing Joint Undertaking (EuroHPC), established in 2018, co-ordinates and shares compute resources among EU members and partners with a 2021-27 budget of EUR 7 billion (EuroHPC, 2022[138]). At the end of 2023, the European Commission committed to widening access to EuroHPC’s infrastructure for European AI start-ups, SMEs and the broader AI community as part of the EU AI Start-Up Initiative (European Commission, 2023[139]). EuroHPC also launched a new Research and Innovation call to establish a European support centre to assist European AI users in finding supercomputing capacity (EuroHPC, 2023[140]).
Countries are embedding values-based principles into AI legislation, regulation and standards, moving towards future-fit policies for trustworthy AI
Copy link to Countries are embedding values-based principles into AI legislation, regulation and standards, moving towards future-fit policies for trustworthy AIAs the first intergovernmental standard on AI, the OECD AI Principles cast a light on what OECD countries and partner economies rightly saw as an emerging but fundamental challenge and opportunity to economies and societies. The principles formed the basis for the G20 AI Principles. Currently, 46 countries adhere to the OECD AI Principles, including economies such as Argentina, Brazil, Peru, Romania, Egypt, Malta, Singapore and Ukraine.
Since the principles were adopted in 2019, AI policy initiatives have flourished across OECD and partner economies. Many countries now have learnings from implementation of their first national AI strategies. Indeed, many jurisdictions are moving from “principles to practice” by codifying the OECD AI principles into law, regulation and standards. As implementation continues, discussions are ongoing about related AI certification, standards assessments and enforcement bodies. In addition, questions also remain about interoperability with existing privacy, data and intellectual property regimes.
The field of responsible AI has grown dramatically. AI policy makers across disciplines are rallying to ensure that AI benefits not only the “bottom line” but also societies and the environment more broadly. In 2016, only a handful of countries had national AI strategies. In 2024, as an established hub for sharing experiences and best practices, the OECD.AI Policy Observatory documents over 1 000 AI-related policy initiatives across 70 jurisdictions. Of these, more than 354 initiatives relate to AI guidance and regulation (OECD.AI, 2024[118]).
Increasingly, countries are promoting AI governance by proposing or implementing AI principles, legislation, regulation and standards. Some jurisdictions are taking a cross-sectoral approach to AI regulation (Canada, European Union, Brazil), while others consider a more sectoral approach (United Kingdom, United States).
Since 2019, through its Directive on Automated Decision-Making, Canada has implemented regulations around automated decision-making systems for public services, including the requirement for algorithmic impact assessments (Government of Canada, 2019[141]). Canada has put forward the Digital Charter Implementation Act (Bill C-27) to regulate use of AI in the private sector and economy. The proposed legislation includes new rules for AI in the Artificial Intelligence and Data Act (Parliament of Canada, 2022[142]). Ultimately, Canada wants its rules and regulations to be interoperable with other emerging AI regulations, such as those in the European Union.
In 2019, Japan published a set of Social Principles for Human-Centric AI outlining three philosophies (dignity, diversity and inclusion, and sustainability) and seven principles on AI (human-centric, education and literacy, privacy, fair competition, security, innovation and fairness, accountability and transparency) (Cabinet Office, 2019[145]). In May 2023, under the Japanese presidency of the G7, leaders established the G7 Hiroshima AI Process to examine opportunities and challenges related to generative AI. In December 2023, G7 leaders agreed to publish the Hiroshima AI Process Comprehensive Policy Framework and its future “Work Plan” to advance the process. The Comprehensive Policy Framework comprises the OECD’s Report towards a G7 Common Understanding on Generative AI; international guiding principles; an international code of conduct; and project-based co-operation on AI (G7 Hiroshima Summit, 2023[146]; OECD, 2023[66]).
In 2023, the United Kingdom put forward a context-specific regulatory framework for AI in a white paper (“A Pro-innovation Approach to AI Regulation”), which establishes outcomes-focused principles to be applied to AI in specific sectors. It proposes that regulators consider these principles when developing sector-specific definitions and policies. This approach also promotes use of regulatory experimentation in the form of testbeds and sandbox initiatives. These experiments would help AI innovators get new technologies to market, while testing possible regulatory approaches (DSIT, 2023[143]). In November 2023, the United Kingdom hosted the AI Safety Summit, which culminated in the establishment of the AI Safety Institute. The Institute will conduct advanced research, possibly with counterparts in other countries, and commission an AI “State of the Science” report (AI Safety Summit, 2023[65]).
In the United States, binding federal regulation includes sectoral or domain-specific regulations. These incorporate existing AI principles into their application, such as AI-specific initiatives to protect consumers by the Federal Trade Commission. In 2023, the White House released an Executive Order on the Safe, Secure and Trustworthy Development and Use of Artificial Intelligence. It directed various activities related to establishment of standards for AI safety and security. It also outlined various requirements for government entities and other relevant actors related to the protection of privacy, consumer, worker and civil rights, among others (The White House, 2023[136]; The White House, 2023[144]).
Since 2021, the European Union has advanced a Regulation on Artificial Intelligence (hereafter the “EU AI Act”) that is globally influential. The EU AI Act accompanies the European Coordinated Plan on AI (2018, 2021). It thus aims to position Europe as a major world player in AI innovation, embedded with values (human-centric, trustworthy, secure and sustainable) and addressing AI risks through regulation and harmonised standards. The EU AI Act seeks to avoid regulatory fragmentation across member states to mitigate high-risk uses of AI, including potential threats to European values. It proposes obligations for certain AI applications that pose high risks, bans use of AI listed as carrying unacceptable risks (such as social scoring by governments) and establishes transparency obligations for AI uses that present limited risks (such as chatbots) (European Commission, 2021[147]).
Brazil has also proposed an AI regulation, inspired by the EU AI Act and based on three central pillars: protecting the rights of those affected by AI, risk-level grading and governance measures aimed at providers of AI (Agência Senado, 2022[148]).
In 2022, Singapore’s Infocomm Media Development Authority (IMDA) launched A.I. Verify, a voluntary AI governance testing framework and toolkit. It verifies the performance of an AI system against the developer’s claims and against internationally accepted AI principles (IMDA, 2022[149]).
Technical standards will play a key role in the implementation of trustworthy AI
Copy link to Technical standards will play a key role in the implementation of trustworthy AIStandard-setting organisations play a key role in building consensus to promote interoperability between jurisdictions. They also offer market certainty for those using or developing AI in different parts of the world. Promoting wide participation from relevant parties in the establishment of such standards will be critical to ensure different perspectives are considered for effective risk management. The United Kingdom launched the AI Standards Hub in October 2022, which aims to champion use of technical standards as governance tools for AI by providing businesses, regulators and civil society organisations with the practical tools and information to apply AI standards effectively and contribute to their development (AI Standards Hub, 2022[150]). In the United States, NIST has established voluntary technical guidelines for AI risk management that have received broad support, building on the OECD Framework for the Classification of AI Systems. In the European Union, the European Committee for Electrotechnical Standardization (CEN-CENELEC) develops technical standards supporting the EU AI Act. The International Organization for Standardization (ISO) developed ISO/IEC 23053 (2022), establishing a Framework for AI Systems Using Machine Learning. This complements ISO 31000 (2009) for risk management that applies across sectors and activities (ISO, 2022[151]; 2009[152]).
Initiatives supporting international co-operation for trustworthy AI continue to grow
Copy link to Initiatives supporting international co-operation for trustworthy AI continue to growSince adoption of the OECD AI Principles in 2019, there have been numerous global initiatives and partnerships for trustworthy AI.
In addition to adoption of the OECD AI Principles by the OECD countries and partner economies, G20 members have committed to the same principles in the G20 AI Principles. Since adoption of the OECD AI Principles, the OECD has worked with the global AI community to put them into practice, launching the OECD.AI Policy Observatory and Network of Experts early in 2020. In 2022, the OECD subsequently established a new Working Party on AI Governance (AIGO). It has also continued expanding its AI Network of Experts (ONE AI), which includes hundreds of participants globally (OECD.AI, 2024[118]).
The Council of Europe’s Committee on Artificial Intelligence is tasked with developing an AI legal instrument based on its standards for human rights, democracy and the rule of law (Council of Europe, 2023[153]).
Globalpolicy.ai is a coalition of eight7 intergovernmental organisations with complementary mandates on AI (GlobalPolicy.ai, 2023[154]).
The Global Partnership on AI (GPAI) was launched in June 2020 as a multistakeholder initiative focusing on practical projects. Several expert working groups foster responsible AI development based on the OECD AI Principles (GPAI, 2023[155]).
UNESCO’s 2021 Recommendation on the Ethics of Artificial Intelligence introduced principles for trustworthy AI and areas of policy action (UNESCO, 2022[156]).
Challenges lie ahead in crafting future-fit AI policies that also spur innovation
Copy link to Challenges lie ahead in crafting future-fit AI policies that also spur innovationFrameworks for the governance of AI will continue to evolve. Many questions remain for crafting future-fit policies that can sufficiently address concerns raised by AI, while spurring innovation and standing the test of time. Several such questions are explored below, reflecting key considerations and trade-offs policy makers face in preparing for the future of AI.
How can policy makers be agile and keep pace with rapid AI advancements? The capabilities of generative AI took many policy makers by surprise in late 2022. Closing this information gap by promoting interdisciplinarity and collaboration between AI policy makers, developers and technical communities will be essential to build capacity and ensure governments keep pace with AI advancements. However, this can be challenging. AI developers often limit information disclosure to protect trade secrets, among other reasons. In future, national and regional regulations, as well as enforcement bodies, will be instrumental. They will need to protect developers and users from misuse of AI technologies, while enabling innovation and productivity gains. Nevertheless, de jure laws and regulations require time to develop. Large technology companies often race ahead and dictate de facto rules until such legislation and enforcement mechanisms are put in place.
How will jurisdictions regulate increasingly general AI, including foundation models, in an effective manner? Advanced AI, such as GPT-4 (OpenAI), can be used in and adapted to a wide range of applications for which it was not intentionally and specifically designed. This raises questions around how to create adequate rules for AI systems that can be applied generally across contexts. Concerns include how to craft regulation that ensures safe application of AI systems when used in contexts for which they were not expressly trained and tested.
How will jurisdictions avoid a patchwork of complex rules governing AI? International co-operation on AI policy is important because like the Internet, AI knows no borders. However, countries are competing to achieve global leadership in AI, leading them to adopt varying policy approaches. In a world where many firms do business internationally by default, interoperable standards for AI are critical. They provide businesses with stability and predictability, while assuring users that AI applications will not be misused. Many AI models and systems globally use the same core AI algorithms and datasets for their training. This makes many countries vulnerable to the same issues, including bias, security concerns and infringement of human rights. Some attempts at interoperability have already taken shape. For example, a potential “Brussels Effect” from the EU AI Act may be observed in other jurisdictions, namely in Canada and Brazil where a risk-based approach and other features of the EU model can be seen. International organisations like the OECD also play an important role with the OECD definition of an AI system informing the definition in the EU AI Act, helping to facilitate interoperability.
How will high-risk or unacceptable uses of AI be treated? Jurisdictions are trying to establish rules to promote trustworthy AI and to protect fundamental rights without creating undue barriers to innovation. If the treatment of high-risk or unacceptable uses of AI differs across jurisdictions, it may create loopholes and incentivise regulatory arbitrage. Creating minimum global standards to mitigate serious and unacceptable risks may help in this regard. Policy makers may wish to engage in a wider debate on how to address any long-term or broader-scale risks that prove well founded. In addition, they could explore approaches to mitigate and avoid current AI risks, such as adverse outcomes caused by bias in AI.
How will fundamental rights like privacy be upheld in a world where AI can infer undisclosed information about individuals? The considerable advancement of AI capabilities has included increased ability to make predictions and draw conclusions from vast amounts of training data – also known as “inference”. This raises the question of whether AI, including large language models, could violate individuals’ privacy rights by inferring personal information not shared with the model during its training (Staab et al., 2023[40]). Some research suggests that current large language models can infer personal data at a scale previously unseen. This calls for a broader discussion around privacy implications for language models, beyond what they “memorise” during training (Staab et al., 2023[40]). Questions also remain regarding how best to obtain individuals’ consent when using personal data for AI training.
How will intellectual property rights be upheld? Mounting legal cases and policy proposals around upholding intellectual property rights, such as using copyrighted material to train AI, will help clarify these issues in the coming years. Such litigation highlights the need to develop solutions to addressing intellectual property considerations in AI training data across jurisdictions. Policy makers can further examine tools to ensure respect for intellectual property rights, while sufficient data are made available for AI training. Tools include setting rules and codes of conduct around “data scraping” and fostering fair and equitable data-sharing agreements.
How will different sectors of the economy apply their existing regulatory schemes to the use of AI? As AI tools diffuse across nearly every facet of economies, different sectors will likely apply their existing regulatory frameworks to the use of AI. This is already apparent such as in AI-specific initiatives to protect consumers undertaken by the Federal Trade Commission in the United States. Sector-specific AI strategies and approaches to AI governance are expected to continue emerging. This raises questions around promoting interoperability between sector-specific AI schemes, as well as interoperability with existing AI laws and regulations, such as those at national or regional levels.
How will emerging rules for AI be enforced effectively? Questions remain around enforcing AI laws. The emergence of varying regulatory approaches and combinations across mandatory and voluntary regulations, technical standards and policies, both within and across countries, will be a challenge for enforcement. For example, it is not clear how regulators will decide when conformity assessments will be needed. This may lead to overlap and duplication, such as between AI and data protection requirements. Policy makers and regulators will need to overcome any gaps, overlaps and contradictions in laws and regulations as they are implemented and enforced. In addition to paying compliance costs, companies may misinterpret complex regulatory requirements. Effective enforcement may also rely on standards yet to be established.
The future of AI is uncertain and complex, posing great opportunities but also risks for society and economies
Copy link to The future of AI is uncertain and complex, posing great opportunities but also risks for society and economiesBuilding trustworthy AI and using it responsibly are key to realising a future where AI is trusted and a force for good. To better understand the long-term implications of AI, researchers should engage in dialogue and collaboration with policy makers, practitioners, industry partners and civil society groups in multistakeholder forums such as the OECD. They need to ensure their findings are relevant, accessible and actionable to help address opportunities and risks posed by AI today and in the future. Interdisciplinary collaboration between technical and policy communities at national and international levels is critical. Such collaboration can foster mutual learning, trust and co-operation among different actors and stakeholders. Together, they can facilitate the development of inclusive, responsible and human-centric AI policies and practices that stand the test of time.
References
[93] Abid, A., M. Farooqi and J. Zou (2021), “Persistent anti-Muslim bias in large language models”, arXiv, 2105.05783, http://arxiv.org/abs/2101.05783.
[119] Acharya, A. and Z. Arnold (2019), Chinese Public AI R&D Spending: Provisional Findings, Center for Security and Emerging Technology, https://doi.org/10.51593/20190031.
[148] Agência Senado (2022), “Comissão de juristas aprova texto com regras para inteligência artificial” [Committee of jurists approves the text with rules for artificial intelligence], 1 December, Agência Senado, https://www12.senado.leg.br/noticias/materias/2022/12/01/comissao-de-juristas-aprova-texto-com-regras-para-inteligencia-artificial.
[131] Ahmed, N. and M. Wahed (2020), “The de-democratization of AI: Deep learning and the compute divide in artificial intelligence research”, arXiv, 2010.15581, https://arxiv.org/abs/2010.15581.
[65] AI Safety Summit (2023), The Bletchley Declaration by countries attending the AI Safety Summit, 1-2 November 2023, AI Safety Summit, https://www.gov.uk/government/publications/ai-safety-summit-2023-the-bletchley-declaration/the-bletchley-declaration-by-countries-attending-the-ai-safety-summit-1-2-november-2023.
[150] AI Standards Hub (2022), AI Standards Hub, website, https://aistandardshub.org (accessed on 1 March 2024).
[55] Allied Market Research (2022), Autonomous Mobile Robot Market: Global Opportunity Analysis and Industry Forecast, 2020-2030, Allied Market Research, https://www.alliedmarketresearch.com/autonomous-mobile-robot-market-A16218.
[94] Alon-Barkat, S. and M. Busuioc (2022), “Human–AI interactions in public sector decision making: ‘Automation bias’ and ‘Selective adherence’ to algorithmic advice”, Journal of Public Administration Research and Theory, Vol. 33/1, pp. 153-169, https://doi.org/10.1093/jopart/muac007.
[51] Anderson, J. (2023), “You can’t code for humanity: AI, algorithms, and the bias of machine learning”, Resources for Gender and Women’s Studies: a Feminist Review, Vol. 4/1/2, pp. 18-19, https://www.proquest.com/openview/7ca3ec23bcf12fcea7b8e664041536ec/1?cbl=27053&pq-origsite=gscholar&parentSessionId=0X0ibRAd50O3XxOL4riRGVrLr81h619vlKaL6U0u58Y%3D.
[76] Anderson, J. and L. Rainie (2018), “Artificial intelligence and the future of humans”, 10 December, Pew Research Center, https://www.pewresearch.org/internet/2018/12/10/artificial-intelligence-and-the-future-of-humans.
[101] Bekenova, Z. et al. (2022), “Artificial intelligence, value alignment and rationality”, TalTech Journal of European Studies, Vol. 12/1, pp. 79-98, https://doi.org/10.2478/bjes-2022-0004.
[44] Benaich, N. et al. (2022), State of AI Report, State of AI Report, https://www.stateof.ai.
[90] Bender, E. et al. (2021), “On the dangers of stochastic parrots: Can language models be too big?”, FAccT 2021 – Proceedings of the 2021 ACM Conference on Fairness, Accountability, and Transparency, Association for Computing Machinery, Inc., https://doi.org/10.1145/3442188.3445922.
[52] Benedict, T. (2022), The Computer Got It Wrong: Facial Recognition Technology and Establishing Probable Cause To Arrest, thesis, Washington and Lee University School of Law, HeinOnline, https://heinonline.org/HOL/LandingPage?handle=hein.journals/waslee79&div=21&id=&page=.
[9] Bommasani, R. et al. (2021), “On the opportunities and risks of foundation models”, arXiv preprint, 2108.07258, https://arxiv.org/pdf/2108.07258.pdf.
[58] Bryant, M. (2019), “How AI and machine learning are changing prosthetics”, 29 March, MedTech Dive, https://www.medtechdive.com/news/how-ai-and-machine-learning-are-changing-prosthetics/550788.
[20] Bubeck, S. et al. (2023), “Sparks of artificial general intelligence: Early experiments with GPT-4”, arXiv, 2302.12712, https://arxiv.org/pdf/2303.12712.pdf.
[50] Buolamwini, J. and T. Gebru (2018), “Gender shades: Intersectional accuracy disparities in commercial gender classification”, Proceedings of the 1st Conference on Fairness, Accountability and Transparency, Vol. 81, pp. 77-91, http://proceedings.mlr.press/v81/buolamwini18a.html?mod=article_inline.
[145] Cabinet Office (2019), Social Principles of Human-Centric AI, Cabinet Office, Government of Japan, https://www.cas.go.jp/jp/seisaku/jinkouchinou/pdf/humancentricai.pdf.
[83] Clarke, S. and J. Whittlestone (2022), “A survey of the potential long-term impacts of AI – How AI could lead to long-term changes in science, cooperation, power, epistemics and values”, AIES ’22: Proceedings of the 2022 AAAI/ACM Conference on AI, Ethics, and Society, pp. 192-202, https://doi.org/10.1145/3514094.3534131.
[103] Cohen, M., M. Hutter and M. Osborne (2022), “Advanced artificial agents intervene in the provision of reward”, AI Magazine, Vol. 43/3, pp. 282-293, https://doi.org/10.1002/aaai.12064.
[14] Collins, E. and Z. Ghahramani (18 May 2021), “LaMDA: Our breakthrough conversation technology”, Google blog, https://blog.google/technology/ai/lamda.
[54] Conmy, A. et al. (2023), “Towards automated circuit discovery for mechanistic interpretability”, arXiv, 2304.14997, https://arxiv.org/pdf/2304.14997.pdf.
[107] Cotton-Barratt, O. and T. Ord (2014), “Strategic considerations about different speeds of AI takeoff”, 12 August, Future of Humanity Institute, University of Oxford, https://www.fhi.ox.ac.uk/strategic-considerations-about-different-speeds-of-ai-takeoff.
[153] Council of Europe (2023), Revised Zero Draft [Framework] Concention on Artificial Intelligence, Human Rights, Democracy and the Rule of Law, Council of Europe Committee on Artificial Intelligence, https://rm.coe.int/cai-2023-01-revised-zero-draft-framework-convention-public/1680aa193f.
[98] Craig, C. (2021), “The AI-copyright challenge: Tech-neutrality, authorship, and the public interest”, Osgood Legal Studies Research Paper, 26 January, Osgood Hall Law School, York University, https://ssrn.com/abstract=4014811.
[77] Davenport, T. and R. Kalakota (2019), “The potential for artificial intelligence in healthcare”, Future Healthcare Journal, Vol. 6/2, pp. 94-98, https://doi.org/10.7861/futurehosp.6-2-94.
[48] Dickson, B. (8 May 2023), How Open-Source LLMs Are Challenging OpenAI, Google, and Microsoft, TechTalks blog, https://bdtechtalks.com/2023/05/08/open-source-llms-moats.
[99] Dietterich, T. and E. Horvitz (2015), “Rise of concerns about AI”, Communications of the ACM, Vol. 58/10, pp. 38-40, https://doi.org/10.1145/2770869.
[134] Digital Research Alliance of Canada (2020), Canadian Digital Research Infrastructure Needs Assessment, Digital Research Alliance of Canada, Toronto, https://alliancecan.ca/en/initiatives/canadian-digital-research-infrastructure-needs-assessment.
[120] DISR (2021), “The National Artificial Intelligence Centre is launched”, 14 December, News Release, Department of Industry, Science and Resources, Government of Australia, https://www.industry.gov.au/news/national-artificial-intelligence-centre-launched.
[143] DSIT (2023), “A pro-innovation approach to AI regulation”, Policy Paper, No. 815, UK Department for Science, Innovation and Technology, https://www.gov.uk/government/publications/ai-regulation-a-pro-innovation-approach/white-paper.
[102] Dung, L. (2023), “Current cases of AI misalignment and their implications for future risks”, Synthese, Vol. 202/138, https://doi.org/10.1007/s11229-023-04367-0.
[24] Dunlop, C., N. Moës and S. Küspert (10 February 2023), “The value chain of general-purpose AI: A closer look at the implications of API and open-source accessible GPAI for the EU AI Act”, Ada Lovelace Institute blog, https://www.adalovelaceinstitute.org/blog/value-chain-general-purpose-ai/#content.
[19] Elangovan, A., J. He and K. Verspoor (2021), “Memorization vs. generalization: Quantifying data leakage in NLP performance evaluation”, arXiv, 2012.01818, https://arxiv.org/abs/2102.01818.
[140] EuroHPC (2023), “Open call to support HPC-powered artificial intelligence (AI) applications”, 28 November, Press Release, EuroHPC, https://eurohpc-ju.europa.eu/open-call-support-hpc-powered-artificial-intelligence-ai-applications-2023-11-28_en.
[138] EuroHPC (2022), Discover EuroHPC JU, website, https://eurohpc-ju.europa.eu/about/discover-eurohpc-ju_en (accessed on 8 January 2024).
[117] European Commission (2023), “A European approach to artificial intelligence”, webpage, https://digital-strategy.ec.europa.eu/en/policies/european-approach-artificial-intelligence (accessed on 8 January 2024).
[139] European Commission (2023), “Commission opens access to EU supercomputers to speed up artificial intelligence development”, 16 November, Press Release, European Commission, Brussels, https://ec.europa.eu/commission/presscorner/detail/en/IP_23_5739.
[125] European Commission (2023), Economics of Industrial Research and Innovation (database), https://iri.jrc.ec.europa.eu/data# (accessed on 19 March 2023).
[147] European Commission (2021), Regulation of the European Parliament and of the Council Laying Down Harmonised Rules on Artificial Intelligence (Artificial Intelligence Act) and Amending Certain Union Legislative Acts, COM(2021) 206 final, European Commission, Brussels, https://eur-lex.europa.eu/legal-content/EN/TXT/?uri=celex%3A52021PC0206.
[7] EU-US Trade and Technology Council (2023), EU-U.S. Terminology and Taxonomy for Artificial Intelligence, First Edition, EU-US Trade and Technology Council, https://digital-strategy.ec.europa.eu/en/library/eu-us-terminology-and-taxonomy-artificial-intelligence.
[75] Fariani, R., K. Junus and H. Santoso (2023), “A systematic literature review on personalised learning in the higher education context”, Technology, Knowledge and Learning, Vol. 28/2, pp. 449–476, https://link.springer.com/article/10.1007/s10758-022-09628-4.
[146] G7 Hiroshima Summit (2023), Hiroshima AI Process G7 Digital & Tech Ministers’ Statement, G7 Hiroshima Summit, https://www.soumu.go.jp/hiroshimaaiprocess/pdf/document02.pdf.
[132] Ganguli, D. et al. (2022), “Predictability and surprise in large generative models”, arXiv, 2202.07785 [cs], https://arxiv.org/abs/2202.07785.
[71] Ghosh, A. (2023), “How can artificial intelligence help scientists? A (non-exhaustive) overview”, in Artificial Intelligence in Science: Challenges, Opportunities and the Future of Research, OECD Publishing, Paris, https://doi.org/10.1787/a8e6c3b6-en.
[154] GlobalPolicy.ai (2023), GlobalPolicy.ai, website, https://globalpolicy.ai/en (accessed on 8 January 2024).
[69] Goldman Sachs (2023), “Generative AI could raise global GDP by 7%”, 5 April, Goldman Sachs, https://www.goldmansachs.com/intelligence/pages/generative-ai-could-raise-global-gdp-by-7-percent.html.
[141] Government of Canada (2019), Directive on Automated Decision-Making, Treasury Board Secretariat, Government of Canada, https://www.tbs-sct.canada.ca/pol/doc-eng.aspx?id=32592.
[155] GPAI (2023), “About”, webpage, https://gpai.ai/about (accessed on 8 January 2024).
[105] Grallet, G. and H. Pons (2023), “Yuval Noah Harari (Sapiens) versus Yann Le Cun (Meta) on artificial intelligence”, 5 November, Le Point, https://www.lepoint.fr/sciences-nature/yuval-harari-sapiens-versus-yann-le-cun-meta-on-artificial-intelligence-11-05-2023-2519782_1924.php.
[127] Green, A. and L. Lamby (2023), “The supply, demand and characteristics of the AI workforce across OECD countries”, OECD Social, Employment and Migration Working Papers, No. 287, OECD Publishing, Paris, https://doi.org/10.1787/bb17314a-en.
[17] Heikkilä, M. (2022), “The hype around DeepMind’s new AI model misses what’s actually cool about it”, 23 May, MIT Technology Review, https://www.technologyreview.com/2022/05/23/1052627/deepmind-gato-ai-model-hype.
[92] Heikkilä, M. (2022), “The viral AI avatar app Lensa undressed me—without my consent”, 12 December, MIT Technology Review, https://www.technologyreview.com/2022/12/12/1064751/the-viral-ai-avatar-app-lensa-undressed-me-without-my-consent.
[106] Hendrycks, D. et al. (2022), “Unsolved problems in ML safety”, arXiv, 2109.13916, https://arxiv.org/abs/2109.13916.
[95] Horowitz, M. (2023), “Bending the automation bias curve: A study of human and AI-based decision making in national security contexts”, arXiv, 2306.16507, https://arxiv.org/abs/2306.16507.
[15] Hugging Face (2022), “BLOOM”, webpage, https://huggingface.co/docs/transformers/model_doc/bloom (accessed on 27 February 2023).
[5] Hu, K. (2023), “ChatGPT sets record for fastest-growing user base – analyst note”, 2 February, Reuters, https://www.reuters.com/technology/chatgpt-sets-record-fastest-growing-user-base-analyst-note-2023-02-01.
[67] IDC (2022), “IDC forecasts 18.6% compound annual growth for the artificial intelligence market in 2022-2026”, 29 July, International Data Corporation, https://www.idc.com/getdoc.jsp?containerId=prEUR249536522.
[149] IMDA (2022), “About artificial intelligence”, webpage, https://www.imda.gov.sg/How-We-Can-Help/AI-Verify (accessed on 8 January 2024).
[114] INRIA (2023), “French national artificial intelligence research program”, webpage, https://www.inria.fr/en/french-national-artificial-intelligence-research-program (accessed on 8 January 2024).
[13] Islam, K. (2022), “Recent advances in vision transformer: A survey and outlook of recent work”, arXiv, 2203.01536, https://arxiv.org/abs/2203.01536.
[151] ISO (2022), ISO/IEC 23053:2022 Framework for Artificial Intelligence (AI) Systems Using Machine Learning (ML), International Organization for Standardization, Geneva, https://www.iso.org/standard/74438.html.
[152] ISO (2009), “ISO 31000 risk management”, webpage, https://www.iso.org/iso-31000-risk-management.html (accessed on 8 January 2024).
[23] Jones, E. (2023), “Explainer: What is a foundation model?”, 17 July, Ada Lovelace Institute, https://www.adalovelaceinstitute.org/resource/foundation-models-explainer.
[60] Kaplan, J. et al. (2020), “Scaling laws for neural language models”, arXiv, 2001.98361, https://arxiv.org/pdf/2001.08361.pdf.
[26] Khan, S., A. Mann and D. Peterson (2021), The Semiconductor Supply Chain: Assessing National Competititveness, Center for Security and Emerging Technology, https://cset.georgetown.edu/wp-content/uploads/The-Semiconductor-Supply-Chain-Issue-Brief.pdf.
[79] Kreps, S., R. Mccain and M. Brundage (2022), “All the news that’s fit to fabricate: AI-generated text as a tool of media misinformation”, Journal of Experimental Political Science, Vol. 9/1, pp. 104–17, https://doi.org/10.7910/DVN/1XVYU3.
[57] Laaki, H., Y. Miche and K. Tammi (2019), “Prototyping a digital twin for real time remote control over mobile networks: Application of remote surgery”, IEEE, Vol. 7, https://doi.org/10.1109/ACCESS.2019.2897018.
[87] Laplante, P. et al. (2020), “Artificial intelligence and critical systems: From hype to reality”, Computer, Vol. 53/11, pp. 45-52, https://doi.org/10.1109/mc.2020.3006177.
[61] LeCun, Y. (2022), “A path towards autonomous machine intelligence”, Openreview.net, https://openreview.net/pdf?id=BZ5a1r-kVsf.
[109] LeCun, Y. (2022), “LinkedIn post”, https://www.linkedin.com/posts/yann-lecun_i-think-the-phrase-agi-should-be-retired-activity-6889610518529613824-gl2F.
[56] Lim, D. (2019), “Prosthetics controlled by brain implants in the offing, FDA says”, 25 February, Dive Brief, https://www.medtechdive.com/news/prosthetics-controlled-by-brain-implants-in-the-offing-fda-says/549042.
[10] Lorenz, P., K. Perset and J. Berryhill (2023), “Initial policy considerations for generative artificial intelligence”, OECD Artificial Intelligence Papers, No. 1, OECD Publishing, Paris, https://doi.org/10.1787/fae2d1e6-en.
[62] Marcus, G. (2023), “Babbage: is GPT-4 the dawn of true artificial intelligence”, The Economist Podcasts, https://podcasts.apple.com/ca/podcast/economist-radio/id151230264?i=1000605454954.
[46] Marr, B. (2022), “The 10 best examples of low-code and no-code AI”, Enterprise Tech, Forbes, https://www.forbes.com/sites/bernardmarr/2022/12/12/the-10-best-examples-of-low-code-and-no-code-ai/?sh=3035370574b5 (accessed on 27 February 2023).
[110] McLean, S. et al. (2021), “The risks associated with artificial general intelligence: A systematic review”, Journal of Experimental & Theoretical Artificial Intelligence, Vol. 35/5, pp. 649-663, https://doi.org/10.1080/0952813x.2021.1964003.
[16] Merritt, R. (25 March 2022), “What Is a transformer model?”, NVIDIA blog, https://blogs.nvidia.com/blog/2022/03/25/what-is-a-transformer-model.
[47] Meta (2023), “Meta and Microsoft introduce the next generation of Llama”, 18 July, Meta, https://about.fb.com/news/2023/07/llama-2.
[84] Metz, C. (2023), “What exactly are the dangers posed by A.I.?”, 7 May, The New York Times, https://www.nytimes.com/2023/05/01/technology/ai-problems-danger-chatgpt.html.
[115] Ministry of Science and ICT (2021), “Plan to spread the use of AI across all regions and industries established”, Press Release, https://www.korea.net/koreanet/fileDown?fileUrl=/upload/content/file/dc52b9c0fb0f4958812920c40ab4c164_20211102092303.pdf&fileName=211101+Plan+to+spread+the+use+of+AI+across+all+regions+and+industries+established.pdf.
[74] Molenaar, I. (2021), “Personalisation of learning: Towards hybrid human-AI learning technologies”, in OECD Digital Education Outlook 2021: Pushing the Frontiers with Artificial Intelligence, Blockchain and Robots, OECD Publishing, Paris, https://doi.org/10.1787/589b283f-en.
[21] Morris, M. et al. (2023), “Levels of AGI: Operationalizing progress on the path to AGI”, arXiv, 2311.02462, https://arxiv.org/abs/2311.02462.
[36] Mulligan, D. et al. (2021), “Confidential computing – a brave new world”, 2021 International Symposium on Secure and Private Execution Environment Design (SEED), https://doi.org/10.1109/SEED51797.2021.00025.
[97] Murray, M. (2023), “Generative and AI authored artworks and copyright law”, Hastings Communications and Entertainment Law Journal, Vol. 45, https://papers.ssrn.com/sol3/papers.cfm?abstract_id=4152484.
[113] NAIRR Task Force (2023), Strengthening and Democratizing the U.S. Artificial Intelligence Innovation Ecosystem: An Implementation Plan for a National Artificial Intelligence Research Resource, National Artificial Intelligence Research Resource Task Force, Washington, D.C.
[122] National Science Foundation (2023), “National AI research institutes”, webpage, https://nsf-gov-resources.nsf.gov/2023-08/AI_Research_Institutes_Map_2023_0.pdf?VersionId=TJXMSgV4U7Zgmad3iwYkg8Zffbm7KyNM (accessed on 1 November 2024).
[59] Newton, E. (2023), “Will neuromorphic-controlled robots soon become a reality?”, 30 January, Tech Informed, https://techinformed.com/will-neuromorphic-controlled-robots-soon-become-a-reality.
[91] NIST (2022), Towards a Standard for Identifying and Managing Bias in Artificial Intelligence, Special Publication (NIST SP), National Institute of Standards and Technology, Gaithersburg, MD, https://doi.org/10.6028/NIST.SP.1270, https://tsapps.nist.gov/publication/get_pdf.cfm?pub_id=934464.
[112] NSTC (2023), The Networking & Information Technology R&D Program and the National Artificial Intelligence Initiative Office, National Science & Technology Council, Government of the United States, https://www.nitrd.gov/pubs/FY2024-NITRD-NAIIO-Supplement.pdf.
[35] O’Brien, C. (2020), “Why Intel believes confidential computing will boost AI and machine learning”, 2 December, Venture Beat, https://venturebeat.com/ai/why-intel-believes-confidential-computing-will-boost-ai-and-machine-learning.
[64] OECD (forthcoming), “Exploring artificial intelligence futures: Prospective milestones, benefits and risks”, OECD Artificial Intelligence Papers, OECD Publishing, Paris, https://doi.org/10.1787/dee339a8-en.
[6] OECD (2024), “Explanatory memorandum on the updated OECD definition of an AI system”, OECD Artificial Intelligence Papers, No. 8, OECD Publishing, Paris, https://doi.org/10.1787/623da898-en.
[8] OECD (2023), “A blueprint for building national compute capacity for artificial intelligence”, OECD Digital Economy Papers, No. 350, OECD Publishing, Paris, https://doi.org/10.1787/876367e3-en.
[4] OECD (2023), “AI language models: Technological, socio-economic and policy considerations”, OECD Digital Economy Papers, No. 352, OECD Publishing, Paris, https://doi.org/10.1787/13d38f92-en.
[70] OECD (2023), Artificial Intelligence in Science: Challenges, Opportunities and the Future of Research, OECD Publishing, Paris, https://doi.org/10.1787/a8d820bd-en.
[34] OECD (2023), “Emerging privacy-enhancing technologies: Current regulatory and policy approaches”, OECD Digital Economy Papers, No. 351, OECD Publishing, Paris, https://doi.org/10.1787/bf121be4-en.
[66] OECD (2023), G7 Hiroshima Process on Generative Artificial Intelligence (AI): Towards a G7 Common Understanding on Generative AI, OECD Publishing, Paris, https://doi.org/10.1787/bf3c0c60-en.
[86] OECD (2023), OECD Employment Outlook 2023: Artificial Intelligence and the Labour Market, OECD Publishing, Paris, https://doi.org/10.1787/08785bba-en.
[43] OECD (2023), “Security risks in artificial intelligence”, OECD Global Forum on Digital Security for Prosperity, OECD, Paris, https://www.oecd.org/digital/global-forum-digital-security/GFDSP-2023-agenda.pdf.
[25] OECD (2023), “Summary of the OECD-MIT virtual roundtable on the future of artificial intelligence (AI)”, OECD, Paris, https://wp.oecd.ai/app/uploads/2023/03/OECD-MIT-Workshop-1.pdf.
[85] OECD (2023), “The shifting landscape of AI”, 27 March, presentation, Stuart Russell, 2023 International Conference on AI in Work, Innovation, Productivity, and Skills (AI-WIPS), https://www.oecd-events.org/ai-wips-2023.
[81] OECD (2022), Building Trust and Reinforcing Democracy, OECD Public Governance Reviews, OECD Publishing, Paris, https://doi.org/10.1787/76972a4a-en.
[28] OECD (2022), “Measuring the environmental impact of AI compute and applications: The AI footprint”, OECD Digital Economy Papers, No. 341, OECD Publishing, Paris, https://doi.org/10.1787/7babf571-en.
[89] OECD (2022), “Summary of OECD expert discussion on future risks from artificial intelligence of 20 October 2022”, OECD, Paris, https://wp.oecd.ai/app/uploads/2023/03/OECD-Foresight-workshop-notes-1.pdf.
[49] OECD (2021), “State of implementation of the OECD AI Principles: Insights from national AI policies”, OECD Digital Economy Papers, No. 311, OECD Publishing, Paris, https://doi.org/10.1787/1cd40c44-en.
[3] OECD (2019), Artificial Intelligence in Society, OECD Publishing, Paris, https://doi.org/10.1787/eedfee77-en.
[27] OECD (2019), “Measuring distortions in international markets: The semiconductor value chain”, OECD Trade Policy Papers, No. 234, OECD Publishing, Paris, https://doi.org/10.1787/8fe4491d-en.
[78] OECD.AI (2024), “AI Incidence Monitor (AIM)”, OECD.AI Policy Observatory (database), https://oecd.ai/en/incidents (accessed on 14 February 2024).
[33] OECD.AI (2024), “Hugging Face training datasets by language”, OECD.AI Policy Observatory (database), https://oecd.ai/en/data?selectedArea=ai-models-and-datasets (accessed on 6 February 2024).
[124] OECD.AI (2024), “Live data: Investments in AI and data”, OECD.AI Policy Observatory (database), https://oecd.ai/en/data?selectedArea=investments-in-ai-and-data, https://oecd.ai/en/data?selectedArea=investments-in-ai-and-data (accessed on 14 February 2024).
[118] OECD.AI (2024), “National AI policies & strategies”, OECD. AI Policy Observatory (database), https://oecd.ai/en/dashboards/overview (accessed on 10 March 2024).
[111] OECD.AI (2023), “Live data: AI research”, OECD.AI Policy Observatory (database), https://oecd.ai/en/data?selectedArea=ai-research&selectedVisualization=ai-publications-by-country-over-time and https://oecd.ai/en/data?selectedArea=ai-research&selectedVisualization=ai-publication-time-series-by-institution (accessed on 13 March 2023).
[128] OECD.AI (2023), “Live data: AI talent concentration by country and gender”, OECD.AI Policy Observatory (database), https://oecd.ai/en/data?selectedArea=ai-jobs-and-skills&selectedVisualization=ai-talent-concentration-by-country-and-gender (accessed on 1 November 2023).
[45] OECD.AI (2023), “Trends & data overview”, OECD.AI Policy Observatory (database), https://oecd.ai/en/trends-and-data (accessed on 27 February 2023).
[126] OECD.AI (2023), “VC investments in AI by country”, OECD.AI Policy Observatory (database), https://oecd.ai/en/data?selectedArea=investments-in-ai-and-data&selectedVisualization=vc-investments-in-ai-by-country (accessed on 19 March 2023).
[129] OECD.AI (2022), “Live data: AI Jobs and Skills”, OECD.AI Policy Observatory (database), https://oecd.ai/en/data?selectedArea=ai-jobs-and-skills&selectedVisualization=ai-talent-concentration-by-country (accessed on 14 February 2024).
[123] OECD.AI (2021), OECD.AI Policy Observatory, website, https://oecd.ai (accessed on 30 September 2022).
[38] OPC (12 October 2022), “When what is old is new again – the reality of synthetic data”, OPC Privacy Tech-know blog, https://priv.gc.ca/en/blog/20221012/?id=7777-6-493564.
[29] OpenAI (16 May 2018), “AI and compute”, OpenAI blog, https://openai.com/research/ai-and-compute.
[130] Pang, G. (2022), “The AI chip race”, IEEE Intelligent Systems, Vol. 37/2, https://ieeexplore.ieee.org/document/9779606.
[142] Parliament of Canada (2022), Digital Charter Implementation Act, Parliament of Canada, https://www.parl.ca/legisinfo/en/bill/44-1/c-27.
[18] Reed, S. et al. (12 May 2022), “A generalist agent”, DeepMind blog, https://www.deepmind.com/publications/a-generalist-agent.
[82] Russell, S. (2021), “Living with artificial intelligence”, The Reith Lectures, BBC Radio 4, https://www.bbc.co.uk/programmes/m001216k.
[100] Russell, S. (2019), Human Compatible: Artificial Intelligence and the Problem of Control, Viking.
[11] Russell, S. and P. Norvig (2016), Artificial Intelligence: A Modern Approach, Pearson Education, Inc.
[42] Sadasivan, V. et al. (2023), “Can AI-generated text be reliably detected?”, arxiv, 2303.11156, https://doi.org/10.48550/arXiv.2303.11156.
[31] Schuman, C. et al. (2022), “Opportunities for neuromorphic computing algorithms and applications”, Nature, Vol. 2, https://doi.org/10.1038/s43588-021-00184-y.
[121] Scientific and Technological Research Council of Türkiye (2023), “Who we are”, webpage, https://bilgem.tubitak.gov.tr/en/yze-corporate (accessed on 8 January 2024).
[80] Sessa, M. (2022), “What is gendered disinformation?”, 27 January, Israel Public Policy Institute, https://www.ippi.org.il/what-is-gendered-disinformation.
[30] Sevilla, J. et al. (2022), “Compute trends across three eras of machine learning”, arXiv, 2202.05924, https://arxiv.org/abs/2202.05924.
[53] Shen, X. (2018), “Facial recognition camera catches top businesswoman “jaywalking” because her face was on a bus”, 22 November, Abacus, https://www.scmp.com/abacus/culture/article/3028995/facial-recognition-camera-catches-top-businesswoman-jaywalking.
[41] Shumailov, I. et al. (2023), “The curse of recursion: Training on generated data makes models forget”, arXiv pre-print, 2305.17493, https://arxiv.org/abs/2305.17493.
[104] Skalse, J. et al. (2022), “Defining and characterizing reward hacking”, arXiv, 2209.13085, https://arxiv.org/abs/2209.13085.
[40] Staab, R. et al. (2023), “Beyond memorization: Violating privacy via inference with large language models”, arXiv, 2310.07298v1, https://arxiv.org/pdf/2310.07298v1.pdf.
[39] Stadler, T., B. Oprisanu and C. Troncoso (2020), “Synthetic data – Anonymisation Groundhog Day”, Proceedings of the 31st USENIX Security Symposium, Security 2022, pp. 1451-1468, https://doi.org/10.48550/arxiv.2011.07018.
[72] Stanford (2023), Artificial Intelligence Index Report 2023, Stanford University, Stanford, CA, https://aiindex.stanford.edu/report.
[22] Suleyman, M. (2023), “Mustafa Suleyman: My new Turing test would see if AI can make $1 million”, 14 July, MIT Review, https://www.technologyreview.com/2023/07/14/1076296/mustafa-suleyman-my-new-turing-test-would-see-if-ai-can-make-1-million.
[1] The Alan Turing Institute (2023), “First workshop on multimodal AI”, webpage, https://www.turing.ac.uk/events/first-workshop-multimodal-ai#:~:text=Multimodal%20AI%20combines%20multiple%20types,finance%2C%20robotics%2C%20and%20manufacturing (accessed on 8 January 2024).
[135] The Alan Turing Institute (2022), UK AI Research Infrastructure Requirements Review, https://www.turing.ac.uk/work-turing/uk-ai-research-infrastructure-requirements-review.
[136] The White House (2023), Executive Order on the Safe, Secure, and Trustworthy Development and Use of Artificial Intelligence, https://www.whitehouse.gov/briefing-room/presidential-actions/2023/10/30/executive-order-on-the-safe-secure-and-trustworthy-development-and-use-of-artificial-intelligence.
[144] The White House (2023), “President Biden issues Executive Order on safe, secure, and trustworthy artificial intelligence”, 30 October (fact sheet), The White House, https://www.whitehouse.gov/briefing-room/statements-releases/2023/10/30/fact-sheet-president-biden-issues-executive-order-on-safe-secure-and-trustworthy-artificial-intelligence.
[68] Thormundsson, B. (2022), “Artificial intelligence (AI) market size/revenue comparisons 2018-2030”, 26 October, Statista, https://www.statista.com/statistics/941835/artificial-intelligence-market-size-revenue-comparisons.
[133] Top500 (2023), November 2023 List, website, http://www.top500.org (accessed on 8 January 2024).
[137] TRUBA (2023), TRUBA, website, https://www.truba.gov.tr/index.php/en/main-page (accessed on 8 January 2024).
[116] TÜBITAK (2023), “Centre of Excellence support program”, webpage, https://www.tubitak.gov.tr/en/funds/academy/national-support-programmes (accessed on 8 January 2024).
[2] Turing, A. (2007), “Computing machinery and intelligence”, in Parsing the Turing Test, Springer, https://link.springer.com/chapter/10.1007/978-1-4020-6710-5_3.
[156] UNESCO (2022), Recommendation on the Ethics of Artificial Intelligence, UNESCO, Paris, https://unesdoc.unesco.org/ark:/48223/pf0000381137.
[12] Vaswani, A. et al. (2023), “Attention is all you need”, arXiv, 1706.03762, https://arxiv.org/abs/1706.03762.
[63] Villalobos, P. et al. (2022), “Will we run out of data? An analysis of the limits of scaling datasets in machine learning”, arXiv, 2211.04325, https://arxiv.org/abs/2211.04325.
[32] Wu, J. et al. (2023), “Analog optical computing for artificial intelligence”, Engineering, Vol. 10, pp. 133-145, https://doi.org/doi.org/10.1016/j.eng.2021.06.021.
[73] Yang, M. (2023), “Scientists use AI to discover new antibiotic to treat deadly superbug”, 25 May, The Guardian, https://www.theguardian.com/technology/2023/may/25/artificial-intelligence-antibiotic-deadly-superbug-hospital.
[37] Zewe, A. (2022), “Collaborative machine learning that preserves privacy”, 7 September, MIT News, https://news.mit.edu/2022/collaborative-machine-learning-privacy-0907.
[96] Zirpoli, C. (2023), Generative Artificial Intelligence and Copyright Law, Congress, United States, https://crsreports.congress.gov.
[88] Zwetsloot, R. and A. Dafoe (2019), “Thinking about risks from AI: Accidents, misuse and structure”, 11 February, Lawfare, https://www.lawfaremedia.org/article/thinking-about-risks-ai-accidents-misuse-and-structure.
Notes
Copy link to Notes← 1. “Synthetic data is generated from data/processes and a model that is trained to reproduce the characteristics and structure of the original data aiming for similar distribution. The degree to which synthetic data is an accurate proxy for the original data is a measure of the utility of the method and the model.” (EU-US Trade and Technology Council, 2023[7]).
← 2. The initial set of potential future AI solutions can be found at: https://easyretro.io/publicboard/Lg97hwaJe8MJWJTe5uKGfjjtInh1/f63b59a0-0531-456e-82f2-d9bea1463fb9.
← 3. At the time of writing, top research institutions in 2023 according to data from OpenAlex available on OECD.AI include Chinese Academy of Sciences (China), French National Centre for Scientific Research (France), Tsinghua University (China), Shanghai Jiao Tong University (China), Zhejiang University (China), Harbin Institute of Technology (China), Beihang University (China), Huazhong University of Science (China), Stanford University (United States) and the Max Planck Society (Germany). Publications are in multiple languages, not just in English. Data available at https://oecd.ai/en/data?selectedArea=ai-research&selectedVisualization=ai-publication-time-series-by-institution.
← 4. AI keywords used include: generic AI keywords, such as “artificial intelligence” and “machine learning”; keywords pertaining to AI techniques, such as “neural network”, “deep learning”, “reinforcement learning”; and keywords referring to fields of AI applications, such as “computer vision”, “predictive analytics”, “natural language processing”, “autonomous vehicles”.
← 5. In recent years, supercomputer systems have been increasingly updated to also run AI-specific workloads. However, the list does not distinguish supercomputers according to workload capacity specialised for AI. Drawing conclusions from the list should thus be made cautiously: the list does not define “supercomputers” for AI but uses a benchmark methodology (Linpack). This means any supercomputer can make it into the Top500 list if it can solve a set of linear equations using floating point arithmetic. As submitting to the list is voluntary, some countries have reportedly slowed or stopped submissions of top supercomputers to the list in recent years. Thus, country comparisons using this data are limited and should be considered with this caveat. Analysis of the Top500 list can serve as a proxy measure to observe emerging or deepening compute divides between economies. However, such figures should be supplemented by AI-specific analysis as countries reflect on their specific national AI compute needs.
← 6. Maximum achieved performance measured by Rmax in tera floating-point operations per second (TFLOPS).
← 7. Members include the Council of Europe, the European Commission, the European Union Agency for Fundamental Rights, the Inter-American Development Bank, the OECD, the United Nations, UNESCO and the World Bank.