Assia Elgouacem, Herwig Immervoll, Anasuya Raj, Jules Linden, Cathal O’Donoghue and Denisa Sologon
OECD Employment Outlook 2024: The Net-Zero Transition and the Labour Market
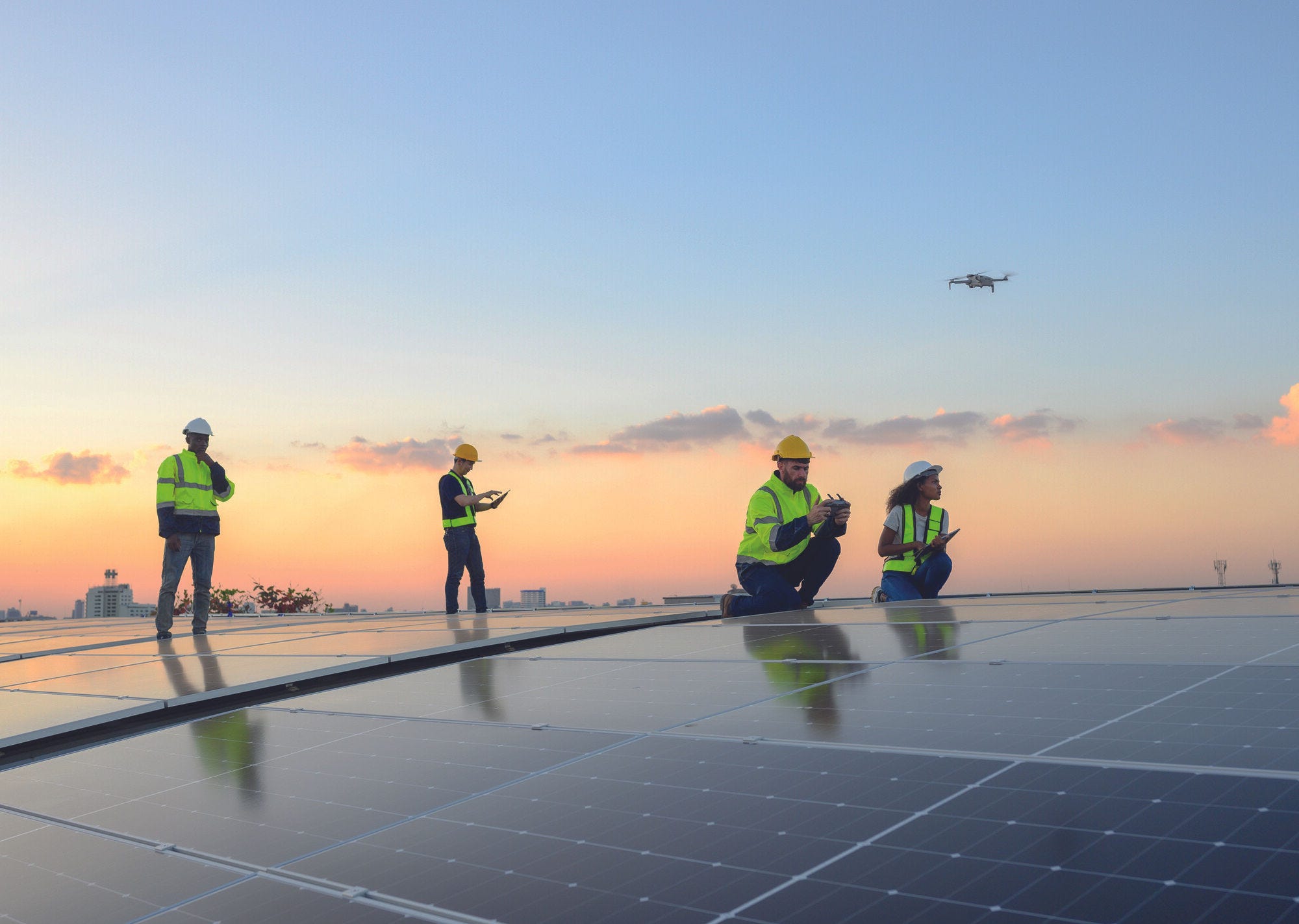
5. Who pays for higher carbon prices? Mitigating climate change and adverse distributional effects
Abstract
Carbon pricing incentivises a reduction in emissions and is one of the key climate change mitigation policies. It may raise a number of concerns, however, not least in the context of recent inflation surges and the energy crisis brought about by Russia’s war of aggression against Ukraine. A key concern is that carbon pricing measures may have adverse distributional consequences, which in turn can hinder support for necessary climate change mitigation action. This chapter estimates the carbon content of households’ consumption baskets and examines how higher carbon prices alter household budgets and consumer prices – and therefore the real value of workers’ wages. It examines whether carbon pricing measures are regressive and explores how burdens differ across groups, including disadvantaged ones. Based on the distributional impact and associated carbon price revenues, the chapter considers the scope for offsetting household burdens by channelling revenues back to households in the form of income transfers.
In Brief
To address the causes of climate change, OECD countries have implemented different climate change mitigation policy packages that include carbon pricing measures to varying degrees. These measures, whether explicit such as carbon taxes or emissions trading systems or implicit such as fuel excise taxes, incentivise a reduction in emissions. Prices for carbon emissions built into current measures are generally far from the levels that are considered in line with national and international commitments, notably the targets agreed upon in the Paris Agreement. Numerous governments are therefore considering reforms to increase these prices, broaden the share of emissions covered by such instruments or introduce new carbon pricing measures.
By charging producers and consumers for emissions, carbon pricing may result in potentially sizable burdens for households, which can differ substantially between population groups. There are concerns that this may aggravate existing disparities and worsen economic challenges for specific groups, notably in a context of recent and on-going cost-of-living crises. The size and distribution of carbon price burdens can also weaken support for more ambitious climate change mitigation policies. There can be a case for shielding vulnerable groups from adverse impacts of higher carbon prices, not only for social equity reasons, but also as a means to build or maintain public support for the required transition to a net-zero emission economy.
This chapter examines the impact of carbon pricing policies on households. It discusses different channels for distributive effects and quantifies household burdens stemming from the effects of carbon prices on consumption expenditures. The chapter also explores possible compensation measures that governments can finance with carbon pricing revenues and the extent to which they can attenuate the burdens for different income groups. The empirical analysis calculates carbon footprints for households in five OECD countries that differ in terms of carbon prices, greenhouse gas emissions and GDP levels: France, Germany, Mexico, Poland and Türkiye. Estimates account for households’ own use of fossil fuels and for emissions released in the production of all other goods and services that they consume. The resulting footprints are combined with granular data from the OECD’s Effective Carbon Rates database to approximate household burdens from carbon pricing reforms introduced over the 2012‑21 period. The analysis of distributional impacts from carbon pricing conducted in this chapter adopts the status quo as a counterfactual without factoring in the costs of policy inaction on household living standards, which are expected to be significant (see Chapter 2).
The main findings of the chapter include the following:
Large parts of households’ energy use are related to basic needs. Poorer households tend to spend large shares of their incomes on energy, giving rise to equity and affordability concerns when prices for fuel, electricity or other necessities go up. Results in this chapter mostly confirm regressive spending patterns for energy. For instance, in the years prior to the COVID‑19 pandemic, low-income households (the bottom 10%) in Poland and Türkiye spent more than one‑fifth of their incomes on energy – 3 to 10 times the shares spent by those on the highest incomes (the top 10%). But not all forms of energy are necessities. Spending shares for motor fuel increase with income in Mexico and Poland, and motor fuel spending shares in Germany vary little between income groups. In Mexico, high-income households in fact devote larger parts of their income to energy than poorer households, indicating that energy can be a luxury item in middle‑income countries.
The immediate impact of carbon pricing on households’ budgets depends on their reliance on different fuels for heating and transportation (direct effect), and on emissions embodied in all other goods that give rise to carbon emissions (indirect effect). Across the five countries, non-fuel consumption in the years preceding the COVID‑19 pandemic accounted for between 45% and 71% of all CO2 emissions linked to household spending. This result highlights that assessments of distributional impacts need to go beyond examining households’ own fuel and energy consumption, which has sometimes dominated policy debates.
Carbon footprints are very unequal both across and within countries. Before the COVID‑19 pandemic, average annual emissions related to household consumption ranged from around 1 tonne of CO2 per household in Mexico and Türkiye, to 6 tonnes in Poland, and 8 to 9 tonnes in France and Germany. Carbon footprints differ markedly between income groups. On average across the five countries, the highest-income households (top 10%) accounted for 4.5 times the emissions of those in the bottom 10%. But emissions also vary within income groups, e.g. by employment status, age, family size and between urban and rural areas. These findings can help to anticipate patterns of public support for, or resistance to, carbon pricing policies. A granular picture of emissions by demographic group is also needed for targeting support to the most impacted groups, and for anticipating future emission trends and associated policy priorities, e.g. in the context of population ageing.
Households’ carbon footprints are a primary determinant of carbon pricing burdens, but they are not the only one. Carbon pricing measures do not apply uniformly across sectors and fuels and therefore not all emissions are priced equally. For instance, excise taxes, carbon taxes and emissions trading systems can, and often do, vary substantially between industries and fuel types, and each measure can therefore affect consumers and households differently.
Increases in carbon prices and the resulting burdens on households were limited in five of the five countries over the 2012‑21 period, altering the cost of an average households’ consumption basket by 1% of income or less. This is small, relative to both recent annual inflation rates and cumulative inflation over the decade prior to the cost-of-living crisis. Average additional burdens were largest in Poland, at 2.3% of household income, but they were negligible in Türkiye.
Additional carbon price burdens linked to these reforms were sizeable for some income groups, however, and effects were mostly regressive, reflecting the reliance of low-income households on high-emitting consumption items. In France, estimated additional burdens as a share of household incomes for the bottom 10% were three times those for the top 10%, and in Germany, they were approximately twice as big. A notable exception is Mexico, where relative additional burdens were bigger among high-income households, reflecting the top-heavy pattern of energy spending.
Although lower-income households often saw the biggest burdens relative to their incomes, losses for many middle‑class households were mostly of a similar order of magnitude. Therefore, while carbon pricing impacts the living standards of the poor, it also matters for middle‑class workers.
As part of broader policy packages, channelling some or all revenues from carbon pricing back to households allows governments considerable scope to cushion losses and shape distributional outcomes. Some past studies have suggested possible trade‑offs between equity and environmental objectives, as redistribution can increase overall emissions when low-income groups spend larger shares of their incomes on carbon-intensive goods than better-off households. Results in this chapter indicate that such differences in carbon intensity between most income groups are, in fact, small overall and carbon footprints are larger for high-income groups, pointing to opportunities for compensating households without increasing emissions.
Simple compensation measures, such as a uniform lump-sum transfer to all households, are sometimes favoured among researchers and in policy debates. But results illustrate that they may be insufficient to protect all disadvantaged households. They are also not cost effective, leaving little to no room for financing other priorities such as public investment, programmes to boost household investments in energy efficiency or to help workers transition between jobs as part of a green transition. This calls for efforts to reduce the fiscal costs of direct compensation measures, by linking transfer amounts to household burdens and support needs.
As the urgency of action to mitigate the potential dramatic effects of climate change escalates, future carbon price increases may be much more sizeable and fast-paced in some countries than they have been during the past decade. The mostly regressive patterns of past reforms analysed in this chapter underscore the need to carefully consider distributional impacts of future policy changes, along with suitable compensation, both for equity reasons, and to ensure necessary public and political support.
Introduction
Climate change and climate‑change mitigation both have potentially major welfare and distributional effects.1 In the medium to long term, large sections of the global and national populations will be significantly better off with effective climate‑change mitigation that averts rapid-onset disasters (floods, hurricanes, wildfires) and slow-onset events (desertification, heat waves, rising sea levels, etc.). In the short term, however, there can be notable trade‑offs between the intended effects of mitigation policies, such as incentives from higher carbon prices, and unintended distributional effects (Baumol and Oates, 1988[1]; Baranzini, Goldemberg and Speck, 2000[2]).The specific patterns of short-term losses, in turn, have been found to be key drivers of public and political support for necessary policy action to fight climate change (Büchs, Bardsley and Duwe, 2011[3]; Tatham and Peters, 2022[4]).
Carbon pricing is frequently seen as one of several policy tools for turning national and international net-zero commitments into reality. Like other mitigation measures, carbon pricing can be controversial, not least in the context of recent cost-of-living increases.2 Unlike other abatement strategies, however, it generates revenues, which governments can employ to accelerate the net-zero transition, to make it more equitable, to adapt to consequences of climate change that can no longer be avoided (Boyce, 2018[5]), or also to lower other distortionary taxes or reduce public debt.3
The implementation of carbon pricing measures remains uneven globally and across the OECD. There are concerns among policy makers and the public about undue burdens on households, workers and firms (see also Chapters 2 and 3), with notable controversies and recent protests by specific groups in some countries. Surveys asking households directly about their concerns indicate that economic worries (such as unemployment, price growth or poverty) frequently rank more prominently than environmental ones (OECD, 2023[6]), suggesting that voters may tend to resist carbon pricing if they perceive that it will create significant costs for them. A recent large‑scale survey of 40 000 people across 20 OECD countries and emerging economies (Dechezleprêtre et al., 2022[7]) shows that public support hinges not only on respondents’ assessment of their own household’s gains and losses, but also on broader distributional impacts, such as respondents’ perception of burdens on lower-income households (Figure 5.1). There can therefore be a growing tension between the escalating need for decisive climate action and the political feasibility of agreeing and implementing it.
Current carbon prices remain well below levels that are considered in line with national and international commitments, notably the targets affirmed in the Paris Agreement (OECD, 2023[8]; OECD, 2022[9]). For instance, to reach net zero emissions by 2050, scenarios developed by a network of 127 central banks point to a globally weighted implicit price on all emissions of more than EUR 600 per tonne of CO2 (Network for Greening the Finanical System, 2023[10]), when using carbon prices as a proxy for all climate policies. Some studies warn that even carbon prices of this order of magnitude will not suffice to meet net-zero emission targets, without accompanying policies to markedly raise the responsiveness of emissions to carbon pricing, such as regulations concerning certain uses of fossil fuels or support for clean technology (D’Arcangelo et al., 2022[11]). Yet, in 72 OECD and non-OECD countries that together account for 80% of global greenhouse gas (GHG) emissions, less than half of all emissions were covered by some form of carbon pricing measure4 in 2021 (OECD, 2023[8]), and prevailing price levels are mostly much too low to incentivise deep emission cuts. In OECD countries, only a small share of emissions is priced at or above EUR 60 per tonne of CO2. Globally, sizeable fossil-fuel subsidies reduce effective carbon prices, sometimes turning them negative in countries with little or no carbon pricing measures in place (OECD, 2022[12]).
Figure 5.1. Support for climate policies hinges on perceived gains and losses
Correlation between beliefs (as listed) and support for a carbon tax package with cash transfers
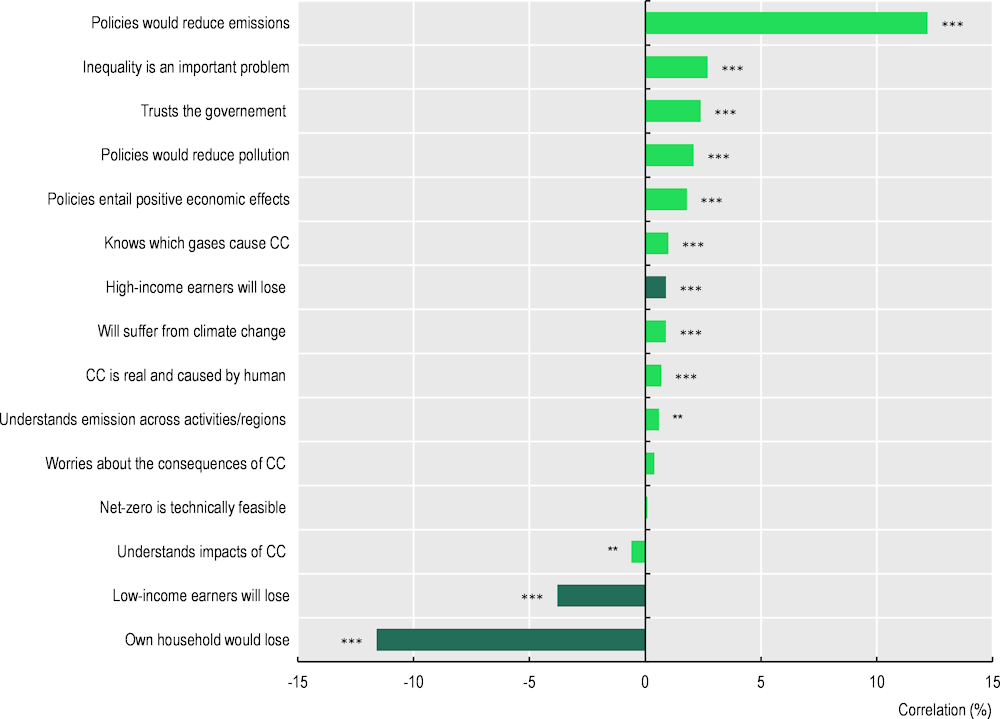
Note: Results of regressions of support on standardised variables measuring respondents’ beliefs and perceptions. Dark green indicates variables measuring expected gains and losses. Country fixed effects, treatment indicators, and individual socio‑economic characteristics are included but not displayed. The dependent variable is an indicator variable equal to 1 if the respondent (somewhat or strongly) supports each of the main climate policies. N=40 680, R2=0.378. CC: climate change.
Source: Adapted from Dechezleprêtre, A. et al. (2022[7]), “Fighting climate change: International attitudes toward climate policies”, https://doi.org/10.1787/3406f29a-en.
As the time available for bridging gaps between current and required climate change abatement efforts becomes shorter, a prospect of drastic and fast-paced policy changes carries growing risks of significant adjustment burdens for households, and of trade‑offs between carbon pricing and household living standards.5 These trade‑offs are currently not well understood, however. In particular, there is a lack of evidence on the distributional effects of policies, and their drivers, especially in a comparative context. In practice, countries have implemented a range of different carbon pricing measures (such as cap and trade emissions certificates, explicit carbon taxes and implicit measures like excise taxes) – all with different rates and bases and with potentially quite different effects on households.
This chapter estimates the carbon content of households’ consumption baskets and assesses how higher carbon prices alter household budgets and consumer prices – and therefore the real value of workers’ wages. It considers carbon pricing in the broad sense of the term, to account for explicit carbon pricing policies (carbon taxes and emissions trading systems), but also implicit carbon pricing through fuel excise taxes. It examines whether carbon pricing measures are regressive and explores differences in carbon price burdens across groups, including those that may be of particular policy interest, such as low-income, older or rural populations, or by gender. In a final step, the chapter considers the scope for offsetting household burdens by channelling carbon price revenues, or certain portions of them, back to households in the form of income transfers (“revenue recycling”). The results enable assessing the patterns of gains and losses and, hence, the feasibility of ensuring that a majority are better off than in the absence of carbon pricing and revenue recycling. The chapter builds on two companion papers. An earlier country-specific OECD analysis developed and illustrated the methodology in the context of a hypothetical reform in one OECD country (Immervoll et al., 2023[13]). A longer technical paper undertakes the comparative assessment of recent real-world policy reforms, and presents the results discussed in this chapter (Elgouacem et al., forthcoming[14]).
The empirical part of the chapter draws on granular information on different types of carbon pricing that countries have introduced over the past decade, using Effective Carbon Rates data collected by the OECD Centre for Tax Policy and Administration (OECD, 2016[15]; OECD, 2023[8]). The analysis combines granular Effective Carbon Rates data with emission factors for different fuels, and with input-output information to approximate the carbon content across fuel types and product categories and, ultimately, the carbon footprint of household consumption baskets. This makes it possible to trace carbon prices through the value chain, and to quantify carbon price burdens at the household level using household budget surveys. Although climate change mitigation impacts current and future generations for years to come, the focus in this chapter is on short-term distributional effects following carbon-pricing reforms. This choice is partly made for methodological reasons, such as the difficulty of accounting for households’ medium-term behavioural adjustments in a realistic manner. In addition, the political-economy implications of perceived gains and losses arising from climate mitigation policies suggest that evidence on short-term impacts can be critical for initiating or accelerating policy action.
As with all modelling approaches, the analysis is subject to a number of simplifying assumptions and limitations, which may be addressed in further empirical work. It draws on granular input-output and household budget data, assuming that production technologies and product demand remain as given. A choice was also made not to model consumers’ subsequent behavioural responses to the calculated price changes across goods and services, due to data and methodological limitations. A novelty of the approach is that it combines sectoral and household-level data with detailed policy information on recent carbon price changes. However, the current version of the analysis focuses on domestic policy changes and does not account for differential changes in carbon pricing across countries and the resulting impact on consumer prices through trade linkages. Finally, the analysis leaves out the effects of carbon pricing on the labour market for tractability reasons (see Chapters 2 and 3 for a discussion of the green transition’s effects on labour markets more generally). The text discusses the rationale and possible effects of simplifying assumptions in more detail and the concluding section suggests associated priorities for future work.
Section 5.1 briefly sets out the objectives of carbon pricing, discusses distributional effects of different climate change mitigation measures, and the channels through which they operate, and summarises carbon pricing policies and recent policy changes across countries. Section 5.2 describes existing evidence on the distribution of carbon price burdens, along with evidence gaps. Sections 5.3 and 5.4 present an empirical analysis of carbon price burdens across five OECD countries. Section 5.3 outlines patterns of energy spending, which are a key driver of household emissions. It then analyses carbon footprints associated with all types of household consumption, comparing them across income levels and other household characteristics. Section 5.4 calculates household burdens resulting from carbon pricing reforms between 2012 and 2021, quantifying effects on household budgets across income groups. The section also considers the effects of a simple compensation measure, simulating the extent to which full or partial revenue recycling could offset carbon pricing burdens, and discussing implications for redistribution strategies. A final section offers concluding remarks.
The distributional impacts of carbon prices matter for labour market policies on multiple fronts. These include the use of revenues raised from carbon pricing to reduce other distortionary taxes such as labour taxes, and the link between labour costs growth and carbon price growth, which affects the welfare impacts of carbon pricing and the real value of wages. The concept of a “double dividend” in the context of carbon taxes (Goulder, 1995[16]) describes the situation where carbon pricing could yield both environmental benefits (by reducing emissions) and economic benefits (through an efficient recycling of the generated revenue, such as reducing distortionary labour taxes) and has been extensively discussed in the literature. Labour costs growth rates can proxy income growth rates and compared against carbon price growth rates may better help evaluate the welfare impacts of carbon price reforms. Such a comparison also helps inform on the effect of carbon price reforms on the real value of wages. These aspects are touched upon in various parts of the chapter (in particular Sections 5.3 and 5.4). Finally, the distributional impacts of carbon pricing measures on households’ consumption may aggravate some of the labour market inequalities induced by the net-zero transition (see Chapters 2 and 3) and reinforce the need for investment in skills (see Chapter 4).
An overarching objective of the chapter is to explore key drivers of distributional outcomes, such as the type of carbon pricing measure, patterns of households’ fuel consumption and the carbon intensity of different goods and services. The presentation of results seeks to inform policy decisions about alternative carbon pricing reform paths, including strategies for providing compensation to households. As countries seek to narrow gaps between the private and social costs of GHG emissions over the coming decades, the empirical analysis may serve as one possible template for a regular monitoring of the distributional implications of carbon pricing initiatives, while also highlighting important future methodological extensions.
5.1. Carbon pricing: Objectives and policy evolution
Measures to contain carbon emissions are progressing, but so is the urgency of greater commitments and corresponding decisive and sustained action – see IEA (2022[17]). Since 2020, the Paris Agreement requires countries to outline and communicate their national climate action plans, known as nationally determined contributions (NDCs) and update them every five years. These NDCs aim to achieve deeper emission reductions, with many countries striving for net-zero targets: globally, net-zero targets have been pledged by 105 countries covering more than 80% of global GHG emissions (OECD, 2023[18]). Most of these targets are not legally binding, however, and global emissions continue to rise (IEA, 2024[19]; Climate Watch, 2024[20]). The anticipated mitigation effect of current international and national commitments is nowhere near sufficient and even full implementation of existing conditional and unconditional commitments made under the Paris Agreement for 2030 will put the world on a course of temperature increases of at least 2.5°C this century (United Nations Environment Programme, 2023[21]). By 2030, GHG emissions would need to fall by 14% and 42% relative to 2019 levels to correct course in line with the Agreement’s 2oC and 1.5oC goals, respectively (Pouille et al., 2023[22]).
The relative advantages and challenges of different climate‑change mitigation strategies remain subject to debate, including among climate scientists (Drews, Savin and van den Bergh, 2024[23]), and countries’ mitigation commitments and approaches differ. A consensus view among climate scientists is that a series of transformative step changes, involving a combination of multiple policy levers, are needed to reach net zero at a pace that is consistent with avoiding catastrophic effects of climate change (Lenton et al., 2023[24]; Jaakkola, Van der Ploeg and Venables, 2023[25]). Such policy packages may encompass measures on both the demand and the supply side, including carbon pricing, as well as regulatory measures, subsidies targeted to specific sectors and direct investments to advance technological solutions (Blanchard, Gollier and Tirole, 2023[26]; OECD, 2023[27]). Each of these mitigation approaches has distributional consequences, impacting households through numerous channels (Box 5.1).
Box 5.1. .Distributional effects of different mitigation strategies: Overview and key mechanisms
Climate mitigation policies have distributional effects that impact households economically (by altering their capacity to consume), and otherwise (through direct effects on people’s well-being and health, and through co-benefits, e.g. better air quality, of reducing CO2 emissions) (Zachmann, Frederikson and Clayes, 2018[28]; Rudolph, Beyeler and Patel, 2022[29]). Economic effects include price changes, the focus of this chapter. In addition, mitigation alters the incomes of workers and asset owners through changing returns to different production factors, including labour, natural resources, and equity in “green” or “brown” industries (Rausch, Metcalf and Reilly, 2011[30]). Several meta studies provide systematic reviews (Peñasco, Anadón and Verdolini, 2021[31]; Lamb et al., 2020[32]; Markkanen and Anger-Kraavi, 2019[33]) and Chapters 2 and 3 in this publication discuss employment effects of climate‑change mitigation. This box illustrates relevant distributional mechanisms via other channels, focussing on non-price mitigation. The distributional impact of carbon pricing is discussed in greater detail in the main text.
Energy efficient and clean technologies play a central role in the climate change‑ mitigation agenda. Demand-side policies, including subsidies and related incentives (such as preferential feed-in tariffs for solar power) tend to accelerate technology adoption and diffusion and can be politically attractive (Giraudet, Guivarch and Quirion, 2011[34]; Douenne and Fabre, 2022[35]). Yet, assessments of past measures generally show that they are regressive, and generally more so than carbon pricing, as they primarily benefit higher-income households with the necessary capital to invest in low-emitting assets (Lihtmaa, Hess and Leetmaa, 2018[36]; Lekavičius et al., 2020[37]; Winter and Schlesewsky, 2019[38]; West, 2004[39]; Levinson, 2019[40]). Findings differ, however, across technologies, with more regressive impacts of subsidies for electric vehicles than for home insulation or solar panels, and little correlation between heat pump adoption and income (Borenstein and Davis, 2016[41]; Davis, 2023[42]). Design features of subsidies or tax credits, such as refundability, timing and targeting, all shape distributional impacts (Giraudet, Bourgeois and Quirion, 2021[43]; Borenstein and Davis, 2016[41]). Outright bans on the demand side are relatively common in Europe, placing restrictions on the use of cars or certain types of residential heating (Braungardt et al., 2023[44]). Bans also raise equity issues, e.g. by creating large and possibly unaffordable asset-replacement costs for the poorest, unless bans are combined with targeted exemptions or compensation (Torné and Trutnevyte, 2024[45]).
Supply-side measures shape production processes via regulation or through subsidies, such as those provided for in the US Inflation Reduction Act (Bistline et al., 2023[46]; Bistline, Mehrotra and Wolfram, 2023[47]) and the European Union’s Net-Zero Industry Act. Comprehensive studies are not yet available but there is some initial evidence of progressive impacts of “supply-push” policies that form part of such packages (Brown et al., 2023[48]). Regulatory approaches can take the form of targeted measures, such as building energy codes, fuel economy standards and vehicle pollution-control, including outright bans of high-emission technologies, with some evidence of high burdens for lower-income households (Davis and Knittel, 2019[49]; Jacobsen, 2013[50]; West, 2009[51]; Bruegge, Deryugina and Myers, 2019[52]). Regulation can also take the form of encompassing packages involving multiple levers, such as the US Clean Air Act (Robinson, 1985[53]) and equivalent provisions in other countries. The scope of these packages varies, as do their distributional impacts, with some evidence of regressive effects (Levinson, 2019[40]).
As part of strategies to tackle the causes of climate change, different types of carbon pricing measures have been introduced to shift the marginal private cost of carbon towards its marginal social cost and to align with climate neutrality targets.6,7 Carbon pricing incentivises a reduction in emissions, including through the reduced use of fossil fuels and the substitution from dirtier to cleaner fuels and technologies. It is usually recommended for its effectiveness in reducing GHG emissions, and because it can be administratively simple, requiring less information than other types of regulation. A key argument for pricing carbon is economic efficiency, in the sense of reducing emissions where it is least costly to do so, without being technologically prescriptive. Moreover, carbon pricing does not weigh on government budgets but instead generates revenue (High-Level Commission on Carbon Prices, 2017[54]; Pigou, 1920[55]; Nordhaus, 1991[56]; Pearce, 1991[57]; Blanchard, Gollier and Tirole, 2023[26]).
Political traction of carbon pricing has increased globally, and there are currently more than 70 explicit carbon pricing initiatives, at regional, national and subnational levels.8 Available estimates suggest that significant emission reductions are possible as a result of carbon pricing, e.g. in the order of 3‑7% for a price increase of EUR 10 per tonne of CO2 applying to all emissions (Sen and Vollebergh, 2018[58]; D’Arcangelo et al., 2022[11]). For a USD 40/tCO2 tax covering only 30% of emissions in the European Union, Metcalf and Stock (2023[59]) estimate a cumulative emissions reduction of 4‑6%, with a low impact on employment and growth – see also Chapter 2. To put these values into perspective, a carbon price of USD 1/tCO2 adds about 0.3 cents to the price of one litre of petrol, or about 1 cent per gallon.
But the speed of adoption varies greatly and recent data point to a growing divide between countries with high and low prices (OECD, 2022[12]). Numerous governments are therefore considering reforms to introduce new carbon pricing measures, to increase prices in existing measures, or to expand them to cover greater shares of emissions. Crucially, and as highlighted by the Intergovernmental Panel on Climate Change (IPCC), “coverage and prices have been insufficient to achieve deep reductions” (Calvin et al., 2023, p. 53[60]). In 72 countries accounting for 80% of worldwide GHG emissions, and including 45 OECD and G20 countries, less than half of GHG emissions (42%) were priced in some form in 2021, either directly through carbon pricing instruments or indirectly through fuel excise taxes or similar. In OECD countries, only 14.6% of GHG emissions were priced at EUR 60/tCO2 or more in 2021 (and 18.5% of CO2 emissions from energy use only). Prices were lower in G20 countries,9 with only 6.6% of GHG emissions (and 8.7% of CO2 emissions from energy use) priced at EUR 60 or more.
A price of EUR 60/tCO2 corresponds to a low-end estimate of the social cost of carbon in 2030, and a mid-range estimate for 2020 (High-Level Commission on Carbon Prices, 2017[54]) and the US Government currently relies on a mean value of USD 51/tCO2 (Interagency Working Group on Social Cost of Greenhouse Gases (IWG), 2021[61]). Recent and forward-looking studies typically support significantly higher values. A 2021 report by the European Commission (2021[62]) suggests a central value of EUR 100/tCO2 already through to 2030, while a recent comprehensive review indicates a preferred mean estimate of USD 185/tCO2, at 2020 prices (Rennert et al., 2022[63]). Estimates of prices that are compatible with longer-term net-zero commitments vary but are higher still.10 Scenarios developed by a network of 127 central banks, and using carbon prices as a proxy for all climate policies, point to a Net Zero 2050 weighted global price of USD 600/tCO2, at 2010 prices (Network for Greening the Finanical System, 2023[10]).
5.1.1. Evolution of carbon prices: Effective carbon rates
The OECD’s Effective Carbon Rates (ECR) database traces the evolution of carbon price policies starting in 2012. The ECR database maps carbon prices to the emissions they cover for each country, by sector and by fuel type. It includes carbon taxes, permit prices from emissions trading systems (ETSs) as well as fuel excise taxes. Carbon taxes and emissions trading systems are explicit forms of carbon prices, as they directly price CO2 (or GHG) emissions. Fuel excise taxes are economically similar to explicit carbon prices, since their base, fuel use, is directly proportional to associated CO2 emissions. Fuel excise taxes, however, can vary across fuel types in ways that do not reflect emissions (e.g. through preferential tax treatment of diesel of heating fuel). Excise tax rates are often higher than explicit carbon prices and they exist in almost all countries. Box 5.2 describes the ECR database and measurement approach in further detail.
Box 5.2. Effective Carbon Rates: Concept, measurement, and interpretation
The OECD Effective Carbon Rates 2021 (ECRs) database covers 72 countries, collectively emitting approximately 80% of global greenhouse gas (GHG) emissions in 2021. It presents carbon prices arising from carbon taxes, emissions trading systems (ETSs) and fuel excise taxes. Effective carbon rates account for implicit fossil fuel support / subsidies when delivered through preferential excise or carbon tax rates, so total ECRs are always greater than or equal to zero. They do not account for government measures that lower pre‑tax prices of fossil fuels, i.e. for negative carbon prices.1 “Carbon taxes” include explicit taxes not only on CO2 emissions, but also on emissions of GHGs other than CO2, such as taxes on fluorinated gases (F-gases).
The pricing instruments included in the ECR dataset either set an explicit price per unit of GHG (e.g. per tonne of CO2e, as in the case of ETSs or carbon taxes), or on a base which is proportional to the resulting GHG emissions (e.g. excise taxes per unit of fuel):
Carbon taxes generally set a rate on fuel consumption based on its carbon content (e.g. on average, a EUR 30/tCO2 tax on carbon emissions from diesel use would translate into a 7.99 eurocent per litre tax on diesel) or less frequently, apply directly to GHG emissions.
Fuel excise taxes are typically set per physical unit (e.g. litre, kilogram, cubic metre) or per unit of energy (e.g. gigajoule), which can be translated into rates per tonne of CO2.
The price of tradable emission permits issued under ETSs, regardless of the permit allocation method, represent the opportunity cost of emitting an extra unit of CO2e (the CO2 equivalent of GHG including CO2 as well as other GHG, see below).2
The considerable granularity of the ECR data is key for capturing differences in emission prices across sectors and, therefore, across the consumption categories that shape carbon price burdens for households. The database covers six sectors that together span all energy uses: road transport, electricity, industry, buildings, off-road transport, agriculture and fisheries. Fuels are grouped into nine categories: coal and other solid fossil fuels, fuel oil, diesel, kerosene, gasoline, liquified petroleum gas (LPG), natural gas, other fossil fuels and non-renewable waste, and biofuels.
CO2 emissions in the ECR database are based on energy use data from the International Energy Agency’s World Energy Statistics and Balances (IEA, 2020[64]). The ECR database covers CO2 emissions from energy use from six sectors (mentioned above). Since its 2018 vintage, it also covers other GHG emissions – i.e. emissions from methane (CH4), nitrous oxide (N2O), F-gases3 and process CO2 emissions (excluding land use change and forestry, LUCF). These are sourced from the CAIT database (Climate Watch, 2024[20]).4 Due to data limitations, and to facilitate comparisons with previous ECR vintages, other GHG emissions are not allocated to the six economic sectors but are considered separately (as a seventh sector).5
In the context of this chapter, the standard ECR indicator is used as the price that is passed on to consumers. This does not account for free emissions allocations to producers. Using it as a basis for assessing consumer prices, therefore effectively assumes full marginal-cost pass-through, regardless of the permit allocation method, with any free allocations being a rent for emitting firms. There is some empirical evidence of marginal-cost pass-through, and associated “windfall profits”, in the energy sector (Fabra and Reguant, 2014[65]; Nazifi, Trück and Zhu, 2021[66]). Nevertheless, full marginal-cost pass-through is a simplifying assumption and the actual incidence will vary across countries and sectors.
Annex 5.B provides additional details on the methodology and discusses a number of core issues related to the ECR indicator and its interpretation.
1. Net Effective Carbon Rates, available from year 2018, account for a broader range of fossil fuel subsidies, i.e. those that decrease pre‑tax prices of fossil fuels, and hence include negative carbon prices (Garsous et al., 2023[67]).
2. Thus, effective carbon rates are sometimes also referred to as effective marginal carbon rates. Effective average carbon rates, which account for free allocations, are discussed in Annex 5.B.
3. HFCs, PFCs, and SF6.
4. Excluding Land-use Change and Forestry (LUCF).
5. See e.g. the International Energy Agency’s documentation on GHG emissions from energy use (IEA, 2021[68]).
Source: OECD (2023[8]), Effective Carbon Rates 2023: Pricing Greenhouse Gas Emissions through Taxes and Emissions Trading, https://doi.org/10.1787/b84d5b36-en; OECD (2022[12]), Pricing Greenhouse Gas Emissions: Turning Climate Targets into Climate Action, https://doi.org/10.1787/e9778969-en; OECD (2016[69]), Effective Carbon Rates: Pricing CO2 through Taxes and Emissions Trading Systems, https://doi.org/10.1787/9789264260115-en.
Effective carbon rates have been increasing in most OECD countries over the 2012 to 2021 period (Figure 5.2), both in nominal and in real terms. Where they decreased, this was in general due to inflation or exchange rate fluctuations. In most OECD countries, fuel excise taxes clearly make up the largest part of total ECR. EU countries as well as Iceland and Norway have been subject to the EU ETS since 2005 and permit prices substantially increased between 2018 and 2021. As part of its Fit for 55 package, the European Union plans to extend carbon pricing through emissions trading to transportation and buildings sectors. Explicit carbon taxes were first introduced in Finland in 1990 and in Norway in 1991, with numerous countries implementing or announcing them since then. In addition, countries variously commit to phasing out fossil fuel subsidies (G20 Leaders Statement, 2009[70]; OECD/IEA, 2021[71]).
Figure 5.2. Evolution of effective carbon rates in 34 OECD countries, 2012‑21
In 2021 EUR per tonne of CO2, levels for 2012 and 2021
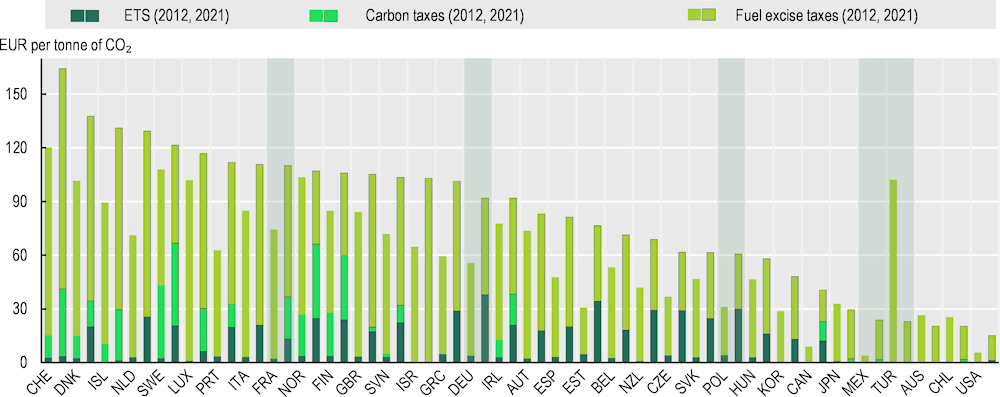
Note: For each country, the two bars refer to the two years (2012, 2021). Highlighted countries are those included in the empirical analysis of this chapter. The chart presents emissions-weighted average Effective Carbon Rates (ECR) over the whole economy, by pricing instrument, presented in 2021 constant EUR. Effective Carbon Rates are the sum of carbon taxes, permit prices from emissions trading systems (ETS) and fuel excise taxes. The emissions-weighted average effective carbon rates presented here are computed excluding emissions from the combustion of biomass.
Reading: In 2012, the average ECR in France was of EUR 74.5 per tonne of CO2, with carbon pricing mostly resulting from fuel excise taxes (with an average of EUR 72 per tonne of CO2 across the economy). In 2021, the average ECR in France was of EUR 110 per tonne of CO2, with carbon taxes and ETS prices increasing the most since 2012: the French economy-wide average carbon price resulting from the EU ETS was of EUR 13/tCO2 and from carbon taxes of EUR 23.6/tCO2.
Source: OECD Effective Carbon Rates database.
In parallel, per-capita carbon emissions from energy use declined in most OECD countries (Figure 5.3). By contrast, in spite of lower average emissions than OECD countries in 2012, non-OECD G20 countries have not all followed the decreasing trend in the OECD area. In particular, China, India and Indonesia have seen a rise in per capita emissions. In India and Indonesia, they nevertheless remained lower than in most OECD countries in 2021. These differences arise due to many factors, including the different levels of developments between most OECD and non-OECD G20 countries. Less developed countries may rely more on energy-intensive resources to foster their development.
Figure 5.3. Carbon emissions from energy use
In tonnes of CO2 per capita, 2012 and 2021
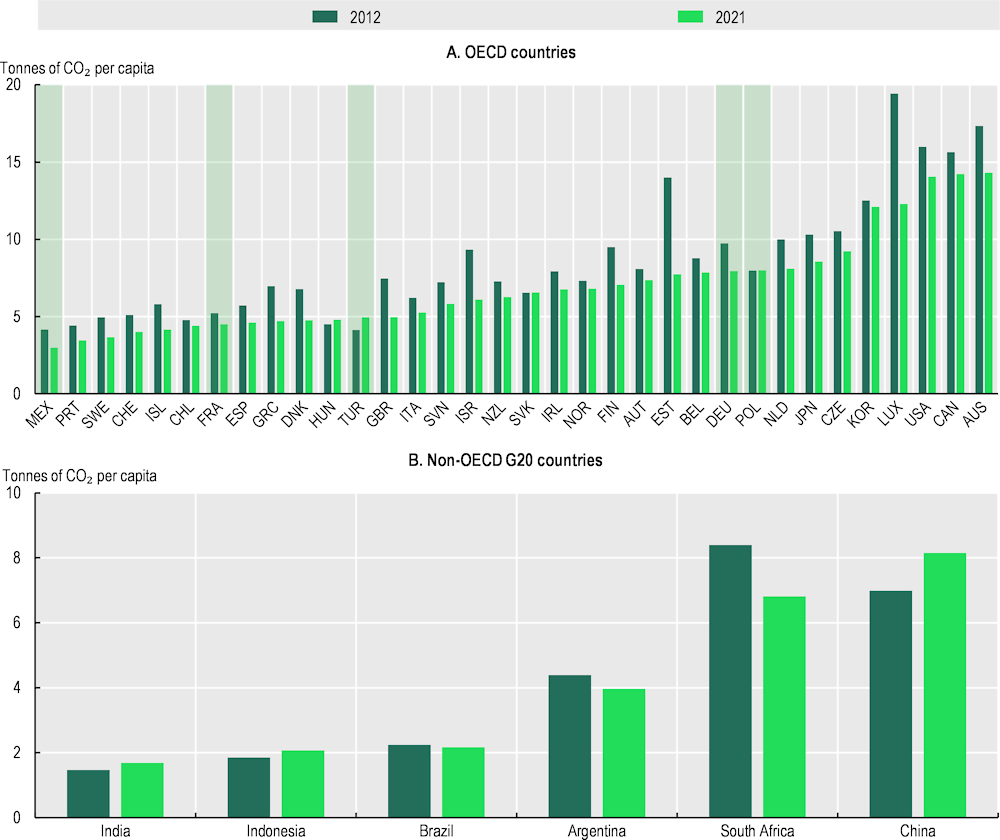
Note: Highlighted countries are those included in the empirical analysis of this chapter. Emissions are on a territorial basis generated in each country. This contrasts with average carbon footprints, as derived in the empirical part of this chapter, which are linked to domestic consumption, i.e. taking into account emissions along the value chain. The emissions presented here are carbon emissions from energy use and exclude emissions from the combustion of biomass.
Source: OECD Effective Carbon Rates database.
The main fuels responsible for CO2 emissions from energy use in OECD countries are natural gas (33%), followed by coal (25%), diesel (16%) and gasoline (13%) (Annex Figure 5.B.1). In non-OECD G20 countries, coal holds a much more important share (65%).11 Carbon prices levied on these fuels are very heterogeneous, in terms of both the rates and the type of policy measure.12
5.2. Previous results on the distribution of carbon price burdens
In part, concerns about the distributional impacts of carbon pricing stem from the fact that domestic fuels are, at the same time, necessities for many households, and a main source of their GHG emissions. When prices go up, the poorest households may be ill-equipped to draw on savings, to cut back on other expenditures, or to reduce their reliance on high-emission products (OECD, 2022[72]; Sologon et al., 2022[73]). As a result, low-income groups may bear a substantial burden from higher carbon prices. A regressive impact, in turn, risks worsening key aspects of inequality, material deprivation and social exclusion, such as energy poverty (including fuel poverty) or food insecurity. Aspects of poverty and deprivation may also worsen even if carbon pricing is not regressive. The current cost-of-living crisis has dramatically heightened concerns over the economic burdens on households from rising living costs, and higher energy prices in particular.
There are numerous drivers of distributional outcomes. Studies tend to focus on the gradient of carbon price burdens by income group and a common question is whether this overall pattern is “regressive”, in the sense that relative burdens decline with income. Other inequalities, such as difference between age groups, education levels, dwelling types or geography have received comparatively little attention in past studies, though a few have shown that such “horizontal” inequalities, even for a given income level, can be greater than “vertical” differences between income groups (Labrousse and Perdereau, 2024[74]; Missbach, Steckel and Vogt-Schilb, 2024[75]; Cronin, Fullerton and Sexton, 2019[76]). For instance, Causa et el. (2022[77]) find that energy price inflation between 2021 and 2022 had stronger impacts on rural households.
Results are available for several countries, but country coverage is far from comprehensive. The scope, objectives and approaches of studies vary greatly, making findings difficult to compare and interpret. Many studies focus on fuel expenditures but do not consider the effects of carbon prices on the affordability of everything else. Likewise, studies frequently do not account for compensation measures that can be financed from carbon-tax revenues (“revenue recycling”). A common focus is on specific hypothetical policy changes (such as the introduction of a comprehensive across-the‑board carbon tax), without considering real-world price variations due to differentiation between sectors, exemptions or linkages between different types of carbon pricing that typically exist in parallel (such as carbon taxes, excise taxes on fuel and/or ETS). To date, there is no comparative13 distributional assessment of real-world policies that governments have already implemented, including combinations of different carbon pricing measures, and associated lessons for the design of future reforms – single‑country distributional analysis of specific components of carbon pricing may nonetheless be found (Gonzalez, 2012[78]; Sajeewani, Siriwardana and McNeill, 2015[79]; Callan et al., 2009[80]).
The net effects of carbon pricing on the cost of households’ entire consumption baskets vary strongly between countries and policy measures, depending not only on spending patterns, but also on population characteristics and existing inequalities (Ohlendorf et al., 2020[81]; Andersson and Atkinson, 2020[82]). Importantly, carbon prices affect not only the cost of transportation and heating but, subject to the carbon intensity of the production process, also the prices of other goods and services (Vogt-Schilb et al., 2019[83]; Immervoll et al., 2023[13]).
There is a common conjecture that carbon taxation and other forms of carbon pricing are regressive in high-income countries (Klenert and Mattauch, 2016[84]). However, home fuel and electricity taxation tends to be more regressive than fuel taxation in the transport sector (Büchs, Ivanova and Schnepf, 2021[85]; Köppl and Schratzenstaller, 2022[86]; Pizer and Sexton, 2019[87]), which can be progressive, especially in countries with moderate car ownership and well-developed public transport systems (Wang et al., 2016[88]; Missbach, Steckel and Vogt-Schilb, 2024[75]; Flues and Thomas, 2015[89]). Constrained energy consumption among low-income households can make carbon pricing somewhat less regressive, although even small increases in energy costs are a concern for households who already consume below their needs.
In countries with lower GDP levels, including in the OECD area, households at the bottom of the income distribution tend to face significant risks of energy affordability (Flues and van Dender, 2017[90]). Outside the OECD, progressive impacts are also more likely in poorer countries, where energy can be a luxury that is unaffordable for large parts of the population, home fuels can be less important due to patchy heating provisions or climatic conditions, and car ownership is highly concentrated at the top of the income distribution (Ohlendorf et al., 2020[81]; Dorband et al., 2019[91]; Mardones, Di Capua and Vogt-Schilb, 2023[92]; Steckel et al., 2021[93]; Missbach, Steckel and Vogt-Schilb, 2024[75]; Klenert and Mattauch, 2016[84]).
Most studies do not compare distributional impacts between different types of carbon pricing. Those that do suggest that pricing direct emission through taxes on fuel consumption (excise taxes) is more regressive than pricing all emissions, including also indirect ones from the consumption of everything else (e.g. through a carbon tax) (Ohlendorf et al., 2020[81]; Immervoll et al., 2023[13]). Yet, extending pricing to GHG other than CO2 can make emissions pricing more regressive, primarily through the impacts on food prices. Exemptions of specific energy carriers or sectors also affects the distribution of carbon price burdens. For instance for a federal carbon tax in Mexico, which exempts natural gas, Renner (2018[94]) finds that burdens are relatively equal across income groups, but that it would be regressive if natural gas were included.
Behavioural responses, which are the primary purpose of carbon pricing, can also differ by population group and can therefore influence distributional outcomes. Households’ consumption decisions respond to changes in relative prices (substitution effect) but also to average price levels (which then impact available income – income effect). The resulting distributional impact depends on preferences and the ability of low and high-income households to adjust their consumption of carbon intensive goods. There is no clear evidence on whether high or low-income households respond more strongly to carbon prices, with some studies suggesting greater responses among low-income households (West and Williams, 2004[95]) and others finding the opposite (Campagnolo and De Cian, 2022[96]). Overall, the impact of households’ behavioural response on the distributional impact of carbon prices seems limited at current carbon price levels (Renner, Lay and Greve, 2018[97]; Immervoll et al., 2023[13]), though this may not hold for bigger and/or fast-paced increases in the future. The lack of any clearer income gradient of behavioural responses reflects multiple drivers of price reactions, but also significant methodological challenges and data limitations (Annex 5.B). Within a country, behavioural impacts may differ across income groups due to many factors, including their composition: for example, if higher income households tend to live in more densely populated areas, they may have more substitution options for lower-emitting transport; or if lower income households tend to commute more, they may have less possibilities to reduce their car usage (and related emissions).
The literature commonly considers revenue recycling as an appropriate tool to alleviate any undesirable distributional impact of carbon pricing (Klenert et al., 2018[98]; Immervoll et al., 2023[13]). Even simple revenue recycling schemes, such as per capita transfers, can lead to progressive impacts of carbon pricing (Feindt et al., 2021[99]; Budolfson et al., 2021[100]). Moreover, available results suggest that it may be feasible to ensure favourable distributional outcomes by redistributing less than the full amount of carbon pricing revenues, leaving some of it for other purposes (Landis, 2019[101]). As carbon price burdens are typically highly heterogeneous, however, redistributing the tax take through an across-the‑board lump-sum transfer will create both gainers and losers (Sallee, 2019[102]; Cronin, Fullerton and Sexton, 2019[76]).
Beyond the consumption and revenue‑recycling channels, carbon pricing has further distributional effects, similar to those of other climate‑mitigation measures, notably by altering the demand or supply of production factors, including labour (see Box 5.1 above).14
5.3. Carbon footprints and distributional impact of carbon pricing reforms
This section combines a range of data sources to explore the impact of carbon pricing measures in five selected OECD economies: France, Germany, Mexico, Poland and Türkiye. These five countries were chosen based on data availability and quality, and they capture a reasonable spread of geographic region, GDP levels, emissions per capita and carbon price levels (see Figure 5.2 and Figure 5.3).
A particular focus of the empirical assessment is to link information on carbon prices and emissions (which is needed for tracing carbon price burdens through the value chain) with micro-data data on consumption patterns (needed for quantifying burdens at the household level, and for assessing government policies that aim to cushion or offset those burdens). Existing studies have tended to be country-specific, or to look at simplified hypothetical reforms or a specific type of carbon pricing, such as excise taxes (see literature overview in Section 5.2). By contrast, this section compares a broad range of carbon pricing reforms that countries have implemented between 2012 and 2021, drawing on the OECD’s ECR database.
The assessment builds on a recent analysis on Lithuania (OECD, 2023[103]; Immervoll et al., 2023[13]). Annex 5.A describes the methodology. In a nutshell, it draws on granular input-output data, which capture emissions by sector and allow tracing them from source inputs to final consumer products and services. Emissions for different output categories are then matched with household spending information from available budget surveys to approximate the carbon footprint from household consumption across groups in a bottom-up manner, incorporating emissions from producing and combining relevant inputs. Apart from the comparative perspective using data sources for different countries, this approach is similar to that followed in some national studies (Pottier, 2022[104]).15
This chapter does not report households’ subsequent reactions to price changes at this stage, because of data and methodological limitations. Households do respond to price changes, and the earlier study that this chapter partly builds on illustrates an approach for estimating a full set of budget and price elasticities (Immervoll et al., 2023[13]). That study estimates that behavioural reactions, mainly in the form of shifts to less polluting goods and services, reduces carbon price burdens by less than 10% for most income groups. However, estimated responses differ significantly across studies and across and within countries. More importantly for the present study, evidence on the overall distributional impact arguably remains inconclusive. Section 5.2 and Box 5.3 provide an overview of previous studies and of the estimation difficulties in the context of carbon pricing. The considerable range of available results on behavioural responses, and the lack of an empirical consensus on the direction of the distributional impact of consumer behaviour, suggests that the effects of methodological and data choices may currently dominate the variation in responses across population groups. Studying households’ evolving responsiveness to carbon price changes is an important topic for future research (see Section 5.5).
Box 5.3. Consumer response to carbon prices: Estimates, driving factors and knowledge gaps
Behavioural responses to carbon pricing are central for effective mitigation, and households play a crucial role in lowering emissions. When emission prices go up, households adjust consumption patterns towards lower-emitting products and services in response to price increases (price elasticities). In addition, higher prices change consumption as a result of reduced overall budgets (in real terms, budget elasticities). Household responses shape the effectiveness of Pigouvian taxes in tackling negative externalities. They are potentially also relevant for estimating second-order distributional consequences of price changes.
Both own and cross-price responses are relevant when assessing behavioural reactions. But a key focus of existing studies is on the responsiveness of fuel demand to changing fuel prices. This is commonly captured in a single number, the own-price elasticity of fuel demand, with a large literature using a number of different estimation strategies and data sources (Labandeira, Labeaga and López-Otero, 2017[105]; Zhu et al., 2018[106]; Havranek, Irsova and Janda, 2012[107]; Brons et al., 2008[108]; Espey, 1998[109]; Dahl, 2012[110]).
Published estimates vary markedly across studies. Considering residential, commercial and industrial actors’ elasticities, Labandeira, Labeaga and López-Otero (2017[105]) find central estimates for energy elasticities of –.221 in the short run, and –.584 in the long-run. They highlight large differences across energy commodities, with the largest elasticities for gasoline (‑.293, ‑.773), natural gas (‑.180, ‑.684) and diesel (‑.153, ‑.443), and lower values for electricity (‑.126, ‑.365) and heating oil (-.017, -.185). Other studies, however, find markedly lower price elasticities for gasoline, providing central estimates of ‑.09 in the short run and ‑.31 over longer time horizons (Havranek, Irsova and Janda, 2012[107]). A range of country characteristics can shape these differences, for instance fuel demand is typically more elastic in densely populated areas and those well-served by public transport.
But the wide range of estimates is also driven by empirical methods and measurement choices, including time horizons. Most studies focus on short-run elasticities and assume that the responsiveness to price changes is independent of the initial price level. In reality, elasticities and price levels vary over time. Households may be unable to adjust their consumption of fuels quickly. But in the medium term, they may invest into new heating systems, improved insulation, and energy efficient transport assets. Indeed, these responses are a key policy objective of carbon pricing and ignoring them is therefore problematic. A further limitation is that demand elasticities are typically estimated linearly, without allowing them to vary depending on the magnitude of price changes (Immervoll et al., 2023[13]). In effect, this approach predominantly captures continuous changes in consumption of a given good (intensive margin), but not complete shifts between consumption categories once prices surpass specific thresholds (extensive margin, such as transitioning entirely from cars to public transport or bicycles). The latter can be particularly important in the context of efforts to lower emissions.
A comparatively small literature examines differences in price elasticities across socio-economic and income groups – see e.g. Wadud, Graham and Noland (2010[111]), who identify the number of vehicles owned, number of wage earners and household location as determinants of price elasticity. The same study assesses the importance of behavioural responses for the distributional impact of a gasoline tax, finding that the inclusion of heterogeneous behavioural responses does not alter the distributional profile of the tax, a finding also echoed in a study of carbon pricing by Renner, Lay and Greve (2018[97]). As noted in Section 5.2, there is no consensus on whether low-or high-income households are more responsive to fuel price increases, with some other studies finding stronger responses among low-income households (West and Williams, 2004[95]), while others find larger elasticities for higher incomes (Zhu et al., 2018[106]).
In summary, households’ responses to price changes in energy commodities are larger in the long run than the short run, and they differ across energy types, although differences are often small to moderate. For all energy commodities, increases in prices are associated with a less than proportional decrease in consumption. Households will therefore typically face larger energy bills when carbon prices go up, even after considering behavioural responses. Some studies suggest that behavioural responses play a limited role in determining the overall distributional impact of price changes, but evidence on the difference in behavioural responses across household types remains inconclusive, partly due to methodological and data-related challenges.
5.3.1. Energy spending is one key driver of household emissions
Carbon prices affect household budgets both directly through households’ own fuel consumption, and indirectly via the consumption of other goods and services that give rise to CO2 emissions during the production process. The direct effect is shaped by the pattern of expenditures on heating and transport (fossil-)fuel (Figure 5.4). The figure also shows spending on electricity, which is a derived good, whose production can be fuel intensive.16 Energy-related emissions embodied in the full set of derived goods, including, e.g. food and public transport, form one part of the overall carbon footprints presented in the next sub-section.
Poor households save less than the rich, or they dissave, and total consumption therefore accounts for larger portions of their income than for better-off households. In four of the five countries included in Figure 5.4, such a regressive spending pattern holds also for energy consumption. This is particularly visible in Poland and Türkiye, where low-income households spent more than one fifth of their incomes on energy.17 Spending on electricity and heating is also regressive in the other European countries. This makes them particularly vulnerable to energy poverty.18 But the share of resources devoted to some categories of energy can in fact increase with income. Spending shares for motor fuel are increasing with income in Mexico and Poland, reflecting both income inequality and unequal car ownership. In Germany, motor fuel spending is mostly flat. Mexico is the only country where richer households devote bigger portions of their income to energy overall, confirming that energy can be a luxury item in middle‑income countries.19 Average spending shares also vary strongly across countries, with plausible drivers including average incomes, climatic factors, as well as energy taxation and subsidies.
Figure 5.4. Poorer households typically spend large parts of their income on energy, but spending is “top-heavy” in some middle-income countries
Household expenditures on fuel and other energy, as a percentage of disposable income, by income decile
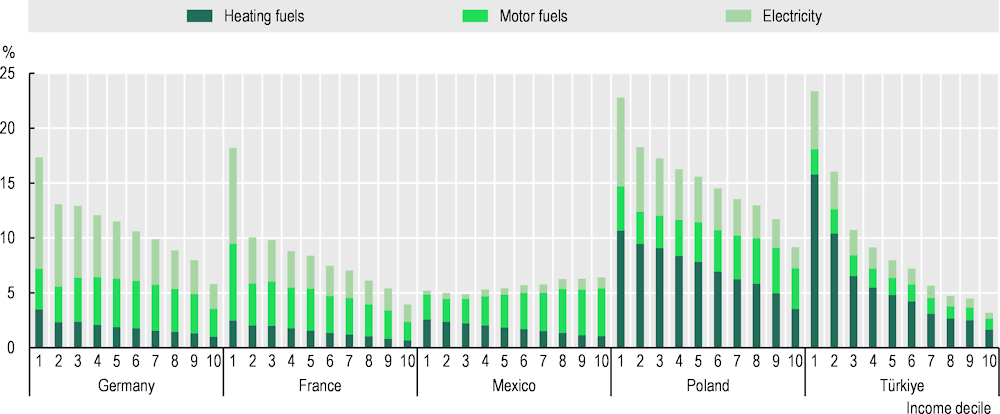
Note: Groups 1‑10 refer to income deciles (equivalised disposable household income). Heating fuel includes expenditure on gas (natural gas and town gas), liquified hydrocarbons, kerosene and other liquid fuels, coal and other solid fuels. Motor fuel includes expenditure on diesel and petrol for transportation.
Source: OECD calculations using household budget surveys (2015 for EU countries, 2016 for Mexico, 2019 for Türkiye) and WIOD input-output data (for electricity).
5.3.2. Who pollutes the most? Carbon footprints linked to household consumption
Energy consumption is a key driver of emissions, but it is not the only one. There are several reasons why energy spending is only a partial indicator of households’ carbon footprints. First, emissions from electricity generation vary enormously between countries, by a factor of 15 across the five countries considered here.20 Relatedly, for each of the main fuel consumption categories, emissions vary by type of fuel (see Annex Figure 5.B.1), as do prices before accounting for carbon pricing. For lower-income or rural households, domestic fuels can include high shares of solid fuels (coal, coke, peat, firewood), which have much higher emission factors than liquid fuel. Emission factors are lower for natural gas, an energy source that can be more common in urban areas. Motor fuels are commonly more expensive than domestic fuels and produce fewer emissions per energy unit than firewood, coal, or heating oil. Per unit of fuel expenditure, emissions – and therefore also the effect of a given carbon price – thus tend to be higher for domestic fuels than for motor fuels.
Second, non-fuel expenditures account for a large share of household spending.21 Per unit of spending, fuel use creates more emissions than other consumption, but due to the size of non-fuel spending, the production of non-fuel goods and services is a significant driver of carbon footprints. Across the five countries, direct emissions from households’ own use of fossil fuels mostly account for around half of the total emissions linked to consumption (Figure 5.5). These estimates incorporate all household consumption, following the “consumer responsibility” principle (see figure note). Fuel spending was a lesser driver of total emissions in France, partly reflecting the balance of spending on fossil fuels and electricity, but also the types of fuel (coal and other solid fuel, liquid fuel, gas) used by households and in production processes.22 Emissions linked to imported inputs or final goods (other than fuel) are also significant but comparatively small, accounting for less than 10% of emissions in each of the five countries. (This small share is reassuring in the context of the chapter’s later estimates of carbon price burdens, which do not account for differential carbon price trends in source countries.)
Figure 5.5. Fuel expenditure is not the only driver of households’ carbon footprints
Emissions from fuel (“direct”) and non-fuel (“indirect”) consumption, in percentage of total
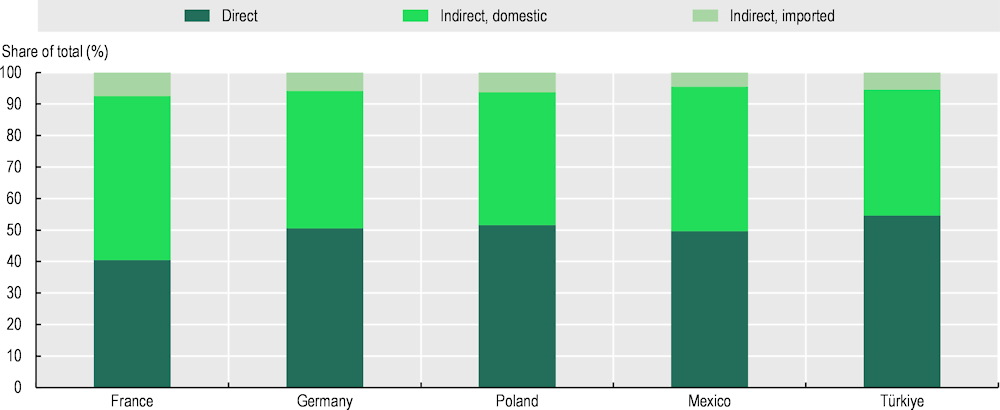
Note: “Direct” includes households’ own consumption of fossil fuels, both domestically sourced and imported. “Indirect, domestic” includes households’ own non-fuel, domestically sourced consumption. “Indirect imported” accounts for emissions linked to all other non-domestically sourced inputs and consumption goods. Estimates are based on the “consumer responsibility” principle, accounting for all household consumption. They therefore attribute emissions to the country where the final good is consumed, and this differs from the emissions per capita that are physically released in a given country. It is important to account for the consumption of imported goods in order to determine the carbon price embedded in households’ consumption baskets.
Source: OECD calculations using IEA emissions factors for different fuels, World Input-Output Database (WIOD) as well as household budget surveys (2015 for EU countries, 2016 for Mexico, 2019 for Türkiye).
Quantifying indirect emissions embodied in non-fuel consumption is thus crucial for assessing distributional effects. Embodied emissions are not observed directly. Consumption patterns, and their carbon content, vary in complex ways between countries and households, making the net effect impossible to anticipate without careful modelling. Results in the remainder of this chapter trace emissions from both direct and indirect consumption to individual households, using the modelling approach summarised in Annex 5.A.
Across countries, differences in carbon footprints are very large, reflecting various factors, including levels of development, population density, consumption patterns, and production technology. Figure 5.6 shows emissions linked to household consumption across countries, at different points of the national emissions distribution (rather than the income distribution). Average emissions (not shown) range from around 1 tonne of CO2 per household and year in Mexico and Türkiye, to 6 tonnes in Poland, and 8 to 9 tonnes in France and Germany. Median emissions of German households were nearly as high as for households at the 8th decile of the Polish emissions range. The average consumption for the top 10% emitting households in Mexico and Türkiye produced the same amount of emissions as the bottom 3rd decile in Germany. These country differences in emissions attributed to domestic household consumption can be much bigger than those in terms of per-capita emissions that are physically released in each country (compare with Figure 5.3).
Figure 5.6. Emissions from household consumption are very unequal across and within countries
Emissions from household consumption, tCO2 per household at different points in the national emissions distribution
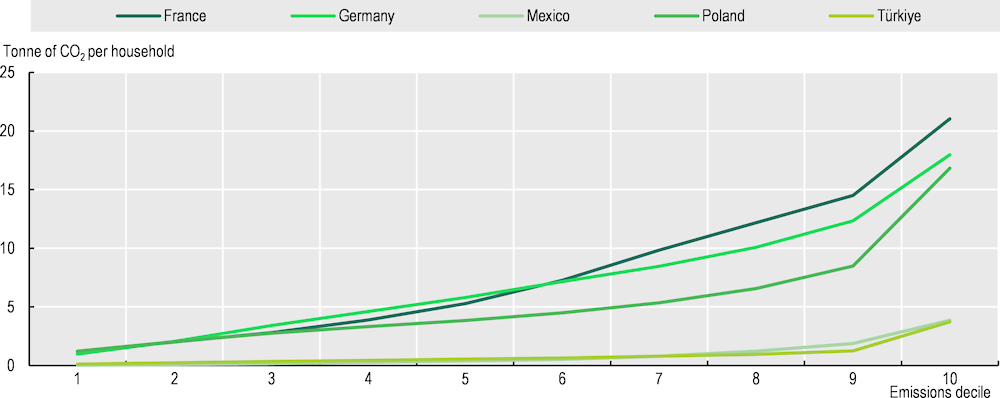
Note: Average emissions across the national emissions distribution (not income distribution), from lowest-emitting to the highest-emitting households. The ranking variable is emissions linked to household consumption, equivalised to account for household size. Estimates follow the “consumer responsibility” principle, accounting for all household consumption, including both domestically produced and imported goods. They therefore attribute emissions to the country where the final good is consumed, and this differs from the emissions per capita that are physically released in a given country.
Source: OECD calculations using IEA emissions factors for different fuels, World Input-Output Database (WIOD) as well as household budget surveys (2015 for EU countries, 2016 for Mexico, 2019 for Türkiye).
Within countries, the key driver of emissions disparities between poor and rich households is total consumption. In the five countries, on average, high-income households (top 10%) spent 4.5 times as much as the poorest 10% (Figure 5.7, top-left panel). Spending inequality was lowest in France and Poland (total spending for the top and bottom 10% differ by a factor of 3) and highest in Mexico (a factor of 9). Emissions associated with consumption therefore also rose strongly with income. On average across countries, profiles of total spending and emissions are very similar, pointing to the importance of total spending levels as a driver of carbon footprints. The findings on the income gradient of consumption-based emissions echo those found in Chancel, Bothe and Voituriez (2023[112])’s recent study, even though the results presented here are less skewed.
Figure 5.7. Carbon footprints are much bigger at high income levels, but in some countries low-income households consume greater shares of high-emission goods
Household expenditure and emissions shares of total, by (disposable) income decile
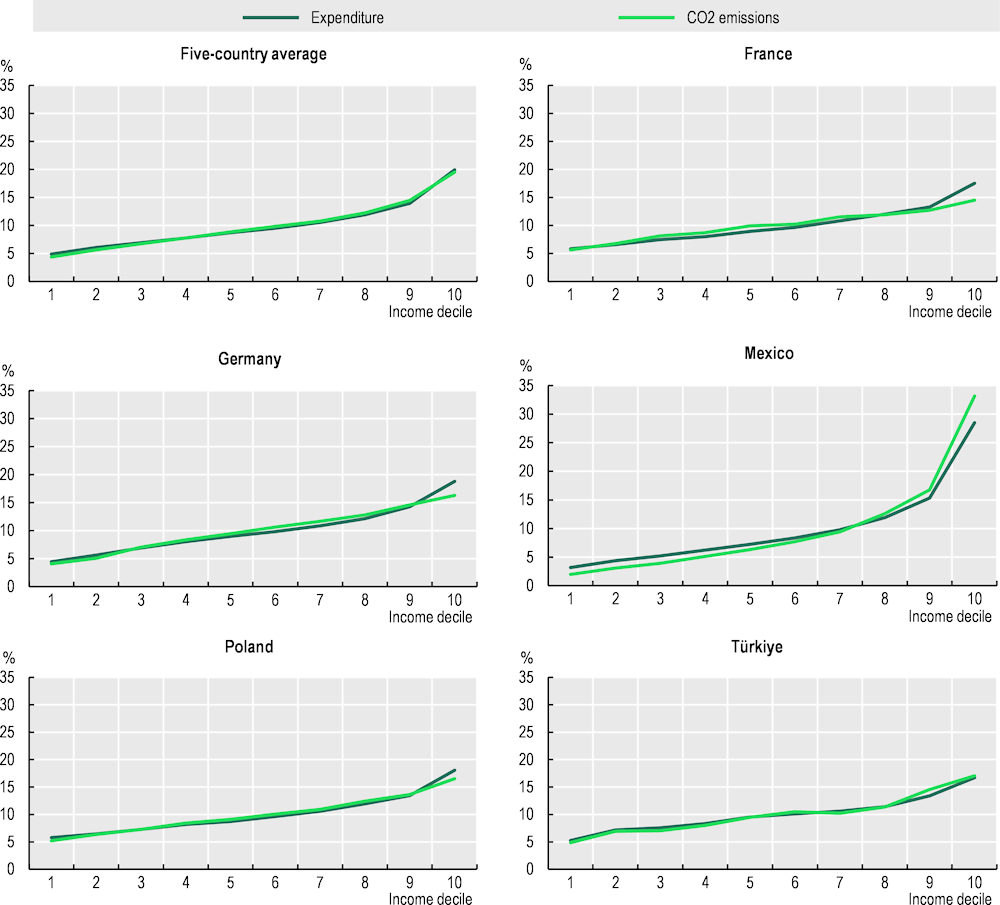
Note: Average expenditure (resp. emissions) by income group (equivalised disposable household income) as a percentage of overall expenditure (resp. emissions). Estimates follow the “consumer responsibility” principle, accounting for all household consumption, including both domestically produced and imported goods. They therefore attribute emissions to the country where the final good is consumed, and this differs from the emissions per capita that are physically released in a given country.
Reading: in Poland, expenditure from the lowest income decile accounts for about 6% of total national expenditure and expenditure from the highest income decile accounts for about 18%. About 5% of emissions accrued to the lowest income decile while 16.5% of emissions accrued to the highest income decile.
Source: OECD calculations using IEA emissions factors for different fuels, World Input-Output Database (WIOD) as well as household budget surveys (2015 for EU countries, 2016 for Mexico, 2019 for Türkiye).
But results for individual countries highlight that emissions can be reduced not only by spending less, but also by spending differently.23 In addition to total spending, the share of spending on particularly carbon-intensive items differs across income groups as well. Due to carbon-intensive necessities, including categories of energy and food, emissions per spending unit can be higher at the bottom of the distribution. Consumption among high-income households, although much larger than for poorer households in total, is then less carbon intensive. Figure 5.7 shows such a pattern in several high-income countries (France, Germany, Poland): for instance, in France, similar shares of total expenditure and carbon emissions accrue to the lowest income decile (resp. 5.8% and 5.6%) whereas the share of total expenditure was higher than that of emissions for the highest income decile (17.5% versus 14.5%). But differences are largely driven by consumption of the highest-income groups (top 10%) and differences in the incidence of emissions and total spending are small or very small in most individual countries and in the five countries on average. Calculations for Mexico indicate that the richest households not only consume more, but that their consumption is also associated with higher carbon emissions per spending unit: 3.2% of total expenditure and 1.9% of total carbon emissions accrue to the lowest income decile, while 28.5% of total expenditure and 33.2% of total carbon emissions accrue to the highest income decile. Section 5.4 discusses implications of these results for strategies to compensate households for carbon price burdens.
An exclusive focus on emissions differences by income misses many other characteristics that may drive the reliance on high-emitting products. As noted in the literature review, past research has often focussed on income‑emission gradients. Yet, a number of studies have documented large carbon-footprints disparities within income groups, pointing to the importance of drivers other than income. These may, for instance, include age, household size, or living in a rural area rather than a city – for the latter, see e.g. recent findings by the Swedish National Institute of Economic Research (Konjunktur Institutet, 2023[113]) – but also individuals’ consumption habits and environmental considerations. The broader social stratification of GHG emissions vary by country and can drive patterns of public support for, or resistance to, carbon pricing policies.24 These patterns need to be understood to design policy packages that do not disproportionately harm disadvantaged groups. A granular picture of emissions by demographic group is also needed for anticipating future emission trends and policy priorities, notably in the context of population ageing (Tian et al., 2023[114]).
Figure 5.8 compares a range of household characteristics between high-emitting and low-emitting households (see figure notes). Emissions in some EU countries are strongly related to the number of earners in the household, likely reflecting the importance of motor fuel for commuting purposes. Relatedly, in several countries, high emissions are more likely in rural areas, reflecting longer commuting times and typically different heating provisions and older housing stock with less insulation (urban/rural flags are not available from the Mexican and Turkish budget survey). There is also a notable gender dimension to carbon emissions with more male-headed‑ households among the top emitters, consistent with prevailing gender income gaps. The education gradient is also notable, and tertiary education is a particularly strong correlate of high emissions in Mexico and Poland. In practice, these and other characteristics are correlated, potentially strongly so. Future work should further analyse carbon footprints for a range of household characteristics, while controlling for others.25
Figure 5.8. Various household characteristics shape carbon footprints
Household characteristics at different emission levels (see figure note)
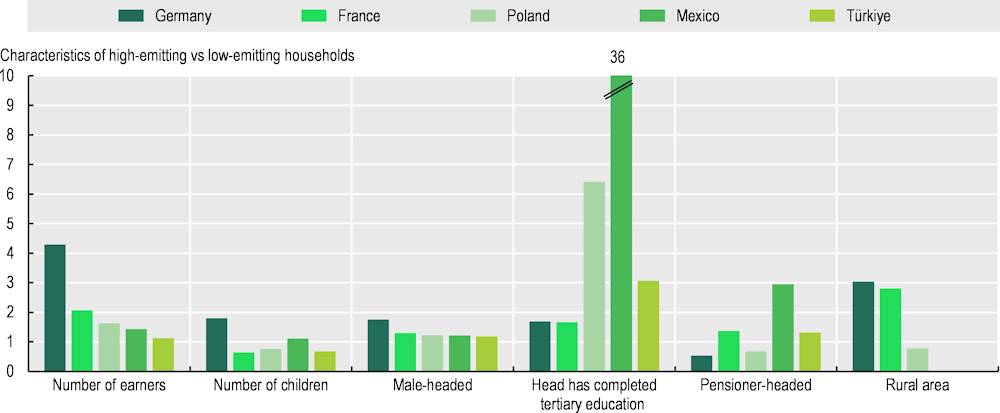
Note: Ratios compare socio‑economic characteristics between high-emitting households (the top 10% of emissions) and low-emitting households (the bottom 10% of emissions) as follows. Number of earners and number of children in the household: Ratio of average numbers of earners and children per household; Other categories: Ratio of number of households in a rural area, or headed by a male, by a person with completed tertiary education, or by a pensioner. Urban/rural indicators are not available from the Mexican and Turkish household budget surveys.
Reading, using the example of Germany: The average number of earners in the top 10% emitting households is 4.3 times that in the bottom 10% emitting households (note that the reason for this large relative difference is that almost 80% of the bottom 10% emitting households in Germany had no earner – and the average number of earners in the top 10% emitting households is 1.4). Reading is equivalent for “number of children”. For the other categories, ratios relate to the number of households in the top 10% and bottom 10% emitting households: the number of households located in rural areas, and the number headed by a male, by somebody with tertiary education, and by a pensioner. In other (less technical) terms, in Germany, high-emitting households were three times more likely to live in a rural area than low-emitting households.
Source: OECD calculations using IEA emissions factors for different fuels, World Input-Output Database (WIOD) as well as household budget surveys (2015 for EU countries, 2016 for Mexico, 2019 for Türkiye).
5.3.3. Policy changes: Who paid for recent carbon pricing measures?
Households’ carbon footprints are a primary determinant of carbon pricing burdens. But they are not the only one, as real-world carbon pricing measures do not apply uniformly, and not all emissions are therefore priced equally. For instance, excise taxes, carbon taxes and emissions trading systems can, and often do, vary substantially between industry and fuel type. For instance, in the 72 countries covered by the OECD ECR database, the road transport sector faces the highest carbon rates (rates above EUR 60 and EUR 120 per tonne of CO2 mostly occur in that sector), followed by the electricity and off-road transport sectors. In the industry and building sectors 72% and 64%, respectively, of emissions remain unpriced, while almost three‑quarters of emissions in the electricity sector face a positive carbon price (OECD, 2023[8]). The correspondence between household emissions and their carbon price burden is thus neither perfect nor straightforward, and depends on the specific design of carbon pricing measures.
The period between 2012 and 2021 saw considerable policy innovation in the area of carbon pricing. While carbon prices mostly fall far short of those needed for meeting key mitigation commitments, OECD countries often raised them significantly over the period. Initial carbon prices in 2012 and the pace of subsequent changes varied, however, as did policy levers (Section 5.1 and Figure 5.2 above). Elgouacem et al. (forthcoming[14]) gives further details on specific reforms in the five countries. In four of the five countries included in the empirical analysis effective carbon rates increased in (constant) Euro terms – in most of them substantially so. ETS prices and coverage increased in all EU countries, approximately doubling ECRs in Germany and Poland and increasing them by a smaller proportion in France. Mexico raised excise taxes by a large margin compared to 2012 values. France introduced a carbon tax. The ECR in Türkiye fell by about 80% of the 2012 value in EUR terms, though carbon prices in fact increased in national currency, which is relevant when considering the impact relative to household incomes. France had the highest ECR in 2021 among the five countries. They were the lowest in Mexico and Türkiye in absolute EUR terms, though with potentially significant impacts relative to household living standards.
Over the 2012‑21 period, household burdens resulting from higher carbon prices were mostly muted overall. Figure 5.9 reports household burdens from recent reforms across the income spectrum. In four of the five countries, the additional cost for an average household’s consumption basket was 1% of income or less. This is small, both relative to recent annual inflation rates, and relative to cumulative inflation over the decade prior to the cost-of-living crisis.26 Average additional burdens were the largest in Poland, at 2.3% of household income, but they were negligible in Türkiye (see figure notes).
Carbon price burdens were more sizeable for some income groups, however, and effects were mostly regressive. In four of the countries, carbon price increases had a regressive impact overall, with consumption baskets of low-income households impacted more heavily. In addition to consumption patterns, initial (pre-reform) fuel prices also play a role, as they can differ significantly between fuel type. Where cheaper and higher-emitting fuels are disproportionately used by lower-income groups, they will see a greater impact of carbon prices on the prices they pay, both in absolute and in relative terms.
While lower-income households mostly saw the biggest impact relative to their incomes, losses for (lower) middleclass households can be of a similar order of magnitude. The regressive impact is most notable in Türkiye, where burdens were small on average but significant in the bottom third of the income distribution, and in Poland, where the bottom decile group saw costs rise by more than 4% of their income. In France, estimated burdens for the bottom decile were three times those for the top decile, while in Germany, they were approximately twice as big. An exception to the regressive patterns is Mexico, with bigger relative burdens among high-income households, reflecting the very top-heavy pattern of energy spending that has been documented for some middle income countries as discussed earlier, and may also be related to geographic / climatic factors (Figure 5.4).
The specific impact on household budgets depends on the type and design of carbon pricing:
Since excise taxes are levied directly on energy spending, the incidence of an increase partly mirrors patterns of households’ energy consumption (compare Figure 5.9 and Figure 5.4). But excise taxes can also drive prices of non-fuel items. In Türkiye, increases were biggest in sectors affecting food prices (agriculture and fisheries), which account for a big share of spending by low-income households. Similarly, excise taxes in Poland changed little for households’ fuel purchases, but they went up substantially in agriculture and fisheries, exerting upwards pressure on food prices.
In four of the five countries, new or increased explicit carbon taxes played no role for overall carbon prices during the 2012‑21 period. The exception, with notable regressive impact, is France, where the Contribution Climat Énergie was introduced in 2014. It was subsequently increased but then kept unchanged since 2018 following a moratorium on previously planned increases in the wake of the so-called “yellow vest” protests.
In EU countries, sizeable changes in household burdens were due to rising prices for emissions certificates (ETS) and/or their extension to additional sectors. Depending on their design, ETS can impact fuel prices for final consumers. For instance, a national ETS introduced in 2021 in Germany (Nationales Emissionshandelssystem) applies specifically to fuel suppliers and therefore affects fuel prices charged to consumers (“direct” impact). “Indirect” effects of ETS on the prices of non-fuel items tend to be larger, however (see Elgouacem et al. (forthcoming[14]) for breakdowns of household burdens into direct and indirect impacts). Indeed, during the 2012‑21 period, increased EU ETS permit prices had little impact on fuel prices for final consumers but exerted upwards pressure on household budgets through the price of non-fuel items, notably in Poland.
Overall, household burdens depend strongly on the specific patterns of carbon pricing across sectors. There is a huge spread of prices in practice, both in any given year, and when considering carbon price changes over time (OECD, 2023[8]). This chapter’s mapping from sectors to consumption goods captures this heterogeneity. By contrast, reform assessments based on simple “stylised” carbon pricing scenarios, such as a uniform rate applying to all emissions, are likely to miss key aspects of the distributional impacts of real-world policy trajectories. Moreover, as illustrated in some of the results, concurrent or sequential carbon pricing reforms in different policy areas can produce complex distributional effects, amplifying, or offsetting each other in terms of their impact on households.
Figure 5.9. Carbon pricing measures during the past decade: Burdens for households
Percentage of disposable income, 2012‑21
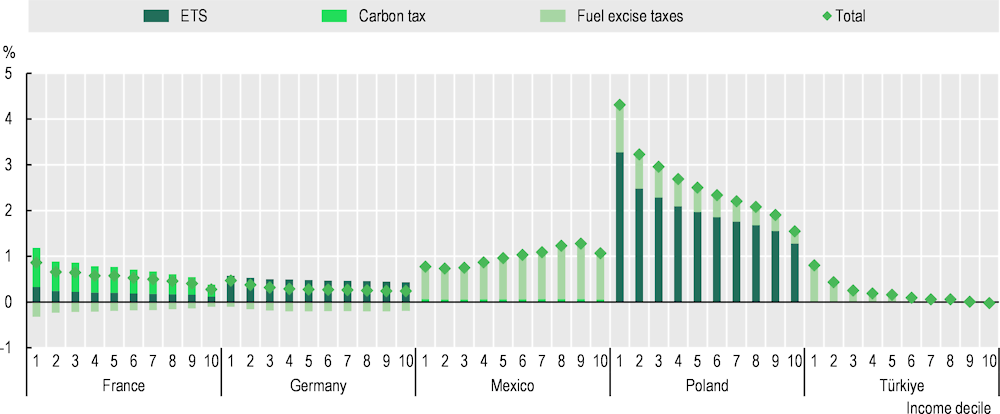
Note: Change in the cost of household-specific consumption baskets, as a share of disposable household incomes, taking consumption baskets (2015 in EU countries, 2016 in Mexico and 2019 in Türkiye) and the fuel mix and carbon intensity of consumption (using the 2016 vintage of the environmentally extended World Input-Output Database – WIOD) as fixed. The changes in carbon pricing burdens are thus only driven by changes in carbon pricing and neither by changes in consumption behaviour nor by changes in the fuel mix and carbon intensity of consumption (see Section 5.2 and Box 5.3 for more details on this assumption). Effective carbon rates for 2012 and 2021 are converted to real terms to match the corresponding household budget survey vintage. Averages by income decile (equivalised disposable household income). For the average household, burdens from carbon pricing reforms were as follows. France: +0.53% of total household income, Germany: +0.50%, Mexico: +1.03%, Poland: +2.34%, Türkiye: +0.09%. ETS: Emission Trading Systems. Changes are computed against the status quo, and do not account for the distributional impacts of inaction.
Source: OECD calculations using OECD Effective Carbon Rates data, IEA emissions factors for different fuels, household budget surveys (2015 for EU countries, 2016 for Mexico, 2019 for Türkiye) and World Input-Output Database (WIOD).
The welfare impact of carbon price changes depends not only on the carbon price, but also on the growth of labour earnings, which may inform the rate of growth of individual and, hence, household labour income.27 Indeed, if carbon prices increase at a faster (resp. lower) rate than earnings, then purchasing power (conditional on interactions with other policies such as taxation and benefits) is likely to fall (resp. increase). This can also help inform the impact of carbon prices on the real value of wages. While combining earnings changes and carbon price changes at the individual level is beyond the scope of the analysis conducted in this chapter, Box 5.4 presents some results when comparing the distribution of carbon price changes relative to the mean sectoral labour earnings growth (as proxied by labour cost growth) over the 2012 to 2021 period.
Box 5.4. Evolution of labour costs and carbon prices
Figure 5.10 below reports the growth rate of (nominal) effective carbon rates (ECRs) for households compared with inflation (the growth rate of prices, using the consumer price index – CPI) and labour costs (in the sectors with the highest and lowest growth rates). For almost all countries, carbon prices in households’ budgets grew faster than inflation for all income deciles, indicating the increasing share of purchasing power subject to carbon prices. However, average labour costs also grew over that period, and at a rate faster than inflation. While the data used in the analysis for this chapter prevents the disaggregation of labour cost changes for individual households, Eurostat labour cost data enables the disaggregation of labour costs by (broadly-defined) sector, which enables the calculation of growth rates of labour costs for the sector with the highest increase and the sector with the lowest increase. For Germany, France and Poland, the growth of ECRs in household budgets was higher than labour costs growth in the sector with the highest growth rate. Türkiye experienced the opposite, with the ECR growth rate for households lower than the labour costs growth rate in the highest growth sector. This indicates that in France, Germany and Poland, the welfare effects of increase in carbon prices possibly tended to be negative (i.e. earnings increased less than prices), while welfare effects in Türkiye were possibly positive for individuals in the sectors with the highest earnings growth.
Figure 5.10. Growth rates of carbon prices (ECR), of the consumer price index and of labour costs 2012‑21, by country
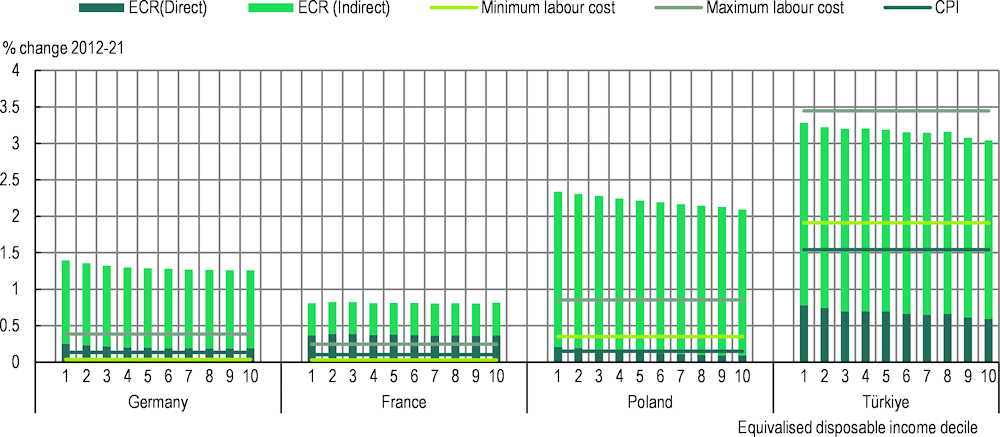
Note: Mexico does not appear in the graph for lack of comparable labour costs evolution data. This figure reports the average labour cost growth of the lowest growing sector, the highest growing sector, the average CPI growth, and the relative change in nominal ECRs between 2012 and 2021 by household (equivalised disposable) income decile. ECR: Effective Carbon Rates. LC: Labour Cost. CPI: Consumer Price Index.
Reading: for instance, for the first decile in Germany, the relative change in nominal ECRs is the difference between the average ECR paid by households in the first decile in Germany in 2021 and in 2012 divided by the average ECR paid by households in Germany in 2012.
Source: Eurostat labour costs data, https://ec.europa.eu/eurostat/web/labour-market/information-data/labour-costs and OECD calculations using OECD Effective Carbon Rates data, IEA emissions factors for different fuels, household budget surveys (2015 for EU countries and 2019 for Türkiye) and World Input-Output Database (WIOD)
5.4. Recycling carbon pricing revenues to limit losses for households
Revenues from carbon pricing are substantial, even if current carbon prices are well below the rates that would be in line with agreed mitigation commitments. Already in 2018, total carbon price revenues averaged around 1.3% of GDP across OECD and G20 countries.28 In a number of OECD countries, this is a similar order of magnitude as key categories of social spending, such as working-age income support or social services other than health.29 As part of broader policy packages, channelling some or all revenues from carbon pricing back to households allows governments considerable scope for cushioning losses and shaping the distributional profile. Such compensation can reduce the regressive impacts of carbon pricing. Moreover, it can also have a role when burdens are not regressive, as carbon pricing can pose affordability challenges for some households, even when its impact across households is uniform or progressive.
Figure 5.11 illustrates the potential of revenue recycling for limiting household losses, and for making parts of the population better off than without the introduction of the 2012‑21 carbon pricing measures. It focuses on the four countries where estimated burdens are significant, without Türkiye (where additional burdens from policy changes were very small). For tractability reasons, estimates are based on the simplest of the revenue recycling scenarios, an equal lump-sum transfer to all households.30,31 The scenario is hypothetical and intended to inform discussions about more tailored compensation strategies. Akin to a universal basic income, uniform lump-sum payments are often less redistributive than targeted social transfers. When conceived as a standalone benefit that replaces other transfers, a basic income is difficult to finance without substantial tax increases and may be regressive in the sense that many vulnerable groups could be worse off by losing the targeted support they used to receive in exchange for the flat basic income (Browne and Immervoll, 2017[115]). However, in the context of a carbon tax, lump-sum compensation is an option that is frequently discussed. It builds on a novel revenue source and can be introduced “on top of” existing transfers, without needing to substitute for them. It is also simple to communicate and, as everyone receives a recurring payment, it can act as a signal that the carbon tax aims to alleviate climate change, without raising household burdens overall. Universal lump-sum payments to all households are sometimes argued to be a suitable revenue recycling option (Klenert et al., 2018[98]).32
Panel A of Figure 5.11 shows the shares of losers in each income group when the entire carbon pricing revenue is redistributed in the form of uniform lump-sum payments.33 Across the four countries, a majority towards the top of the income spectrum lose out, because, for households spending large amounts on fuel and other goods, carbon price burdens are likely to exceed the lump sum. By contrast, most (70% or more) of households in the bottom income decile benefit or have no additional burden. These households have low expenditures (in absolute terms), and the flat-rate transfer therefore offsets or exceeds the effect of carbon prices for many of them.34 Even among low-income groups, the lump-sum transfer leaves some losers, however, highlighting the limitations of simple across-the‑board compensation and the potential horizontal inequality this could lead to.
There may be scope for compensation strategies that achieve short-term distributional objectives at a lower fiscal cost.35 One way of doing so is simply to reduce the per-capita transfer. Indeed, in practice, less than the full revenue from carbon pricing may be available for financing compensation measures. The link between the size of the lump-sum and the number of losers is shown in Panel B. For each spending share, the figure shows how many would lose out. With no compensation at all, household members see unchanged incomes while higher carbon prices translate into higher expenditures, making all of them worse off. As transfers increase, the share of losers eventually declines. For some of the policy changes analysed here, ensuring that a majority are better off appears possible with less than the full carbon price revenues dedicated to income transfers. In Mexico, a very unequal income distribution and the comparatively small burdens of low and middle‑income households mean that a majority are better off, even with only 20% of revenues channelled back to households via lump-sum transfers. In Mexico, there is therefore significant budgetary space for other purposes, such as scaling up programmes that support and accelerate the net-zero transition, by tackling households’ underinvestment in energy efficiency, or facilitating the reallocation of jobs towards less carbon-intensive production – for example through unemployment insurance and active labour-market policies – see D’Arcangelo et al. (2022[116]) and Chapters 3 and 4.36
Fiscal, equity, efficiency and effectiveness considerations can call for carefully tailored compensation strategies, however. For carbon pricing reforms in France and Germany, a uniform lump-sum transfer that prevents a majority from losing out would use up almost the entire carbon pricing revenue. And as noted above, a majority (albeit small) of Poles would be worse off even with full revenue recycling. This suggests that care needs to be taken when committing resources from carbon pricing: where household compensation consumes all or most of additional revenues, the potential for financing other programmes may be limited. This, as well as horizontal inequality (as highlighted by the non-negligeable share of losers within income deciles – see Figure 5.11, Panel A), calls for efforts to reduce the fiscal costs from direct compensation measures, by linking transfer amounts to households’ support needs (which may be income‑related or related to other factors, such as rural versus urban considerations), while maintaining the price signals consistent with the objective of lowering emissions.37 Many countries that have introduced some form of recycling of carbon price revenues have indeed targeted transfers to those most in need (Box 5.5). The importance of well-targeted support measures has become all the more salient with the recent energy crisis (Hemmerlé et al., 2023[117]). Informing tailored and, therefore, cost-effective support strategies is indeed one argument for a detailed and regular monitoring of the distribution of carbon price burdens (Douenne and Fabre, 2020[118]).
Box 5.5. Recycling carbon revenues to compensate households: Policy examples
Marten and Van Dender (2019[119]) take stock of the use of revenues from different carbon pricing measures across 40 OECD and G20 economies – see also World Bank (2019[120]). As other “Pigouvian” taxes, carbon pricing is typically not intended as a stable source of financing and will subside as it achieves relevant emissions-reduction objectives. And similar to other government revenues, those from carbon pricing are subject to competing demands, and this may limit the scope for earmarking them for income transfers. Yet, there are several reasons why carbon revenues could play a significant redistributive role. First, at commonly discussed carbon price trajectories, prospective revenues are sizeable (see main text). Second, although rising carbon prices are designed to narrow the tax base eventually, this is a gradual process and, initially, the negative impact on government revenues can and should be compensated by increasing rates further. Carbon price revenues are set to decline at some point, but this is a matter of decades, not years; Third, redistribution and associated social protection has a key enabling role by cushioning adjustment costs for affected households and facilitating voter support. The associated resource needs are therefore arguably temporary, rather than permanent, and can thus be financed through a temporary revenue source.
There are several examples of carbon pricing earmarked for income transfers and related social protection programmes:
New Zealand: The 2022 budget established a Climate Emergency Response Fund based on proceeds from its emissions trading system. Initiatives that are eligible for funding include those that reduce vulnerability or exposure to climate change or addresses distributional consequences of climate change and mitigation policies.
Austria: The 32.50 EUR/t carbon tax measure enacted in 2022 recycles all revenue in the form of cash payments. The system uses location-based targeting, whereby residents in regions with greater dependence on carbon-heavy activities (e.g. private transport if public transport is comparatively difficult to access) receive more support (OECD, 2022[72]).
Switzerland: A carbon tax of 12 CHF/t was introduced in 2008 and raised in steps to 120 CHF/t in 2022. It currently raises annual revenues of about CHF 1.2 billion, with two‑thirds distributed as a lump-sum transfer, in the form of reduced health insurance rates (FOEN (Swiss Federal Office for the Environment), 2023[121]).
Ireland: A 48.50 EUR/t carbon tax was put in place in 2010, employing a “soft” type of earmarking, with a political commitment to use a share of revenues for raising social assistance benefits for households with children, and to provide retraining for workers in carbon-intensive sectors. The 2020 budget raised carbon taxes by 6 EUR/t and ring-fenced the proceeds, including for protecting vulnerable households and workers.
British Columbia, Canada: British Columbia has a Climate Action Tax Credit, which is a quarterly payment that helps offset the impact of the carbon taxes paid by individuals and families. In April 2024, British Columbia’s carbon tax rate rose from CAD 65 to CAD 80 per tonne of CO2e – to protect affordability, revenues generated by this increase are to be directed to carbon tax relief for British Columbians through enhancements to the Climate Action Tax Credit.
Canada (eight provinces): Eight Canadian provinces (Alberta, Manitoba, New Brunswick, Newfoundland and Labrador, Nova Scotia, Ontario, Prince Edward Island and Saskatchewan) redistribute carbon tax revenues back to households through the Canada Carbon Rebate – formerly known as the Climate action incentive payment (CAIP).
Source: Immervoll (forthcoming[122]) and ELSAC Policy Questionnaire. Canadian support measures: www.canada.ca/en/revenue-agency/services/child-family-benefits/canada-carbon-rebate.html and www2.gov.bc.ca/gov/content/taxes/income-taxes/personal/credits/climate-action, accessed 3 June 2024.
As for redistribution policies more generally, there can be trade‑offs between environmental goals, equity objectives and others, including simplicity, strengthening work incentives, or addressing other pre‑existing challenges. In general, adding additional objectives (such as reducing unrelated tax burdens), tends to leave less fiscal space for redistribution or for furthering climate‑change mitigation. For instance, a common argument for environmental tax reform is that it may create a “double dividend”, by simultaneously improving environmental and economic conditions, through lowering harmful emissions, and creating fiscal space for reducing distortionary taxes, e.g. on labour (Pearce, 1991[57]; Ekins et al., 2011[123]; Antosiewicz et al., 2022[124]; García-Muros, Morris and Paltsev, 2022[125]). Such a policy approach is sometimes considered on efficiency grounds (Guillemette and Château, 2023[126]). But, unlike redistributive cash transfers, lowering progressive income taxes provides little to no support to the poorest and may render carbon price packages more regressive than alternative compensation schemes (Rausch, Metcalf and Reilly, 2011[30]; Immervoll et al., 2023[13]). Bundling carbon pricing with labour-tax reductions can nevertheless be an attractive option from a political economy point of view, notably if there is empirical evidence that employment gains would be sizeable, including among groups who are strongly affected by higher emission charges – see Chapter 3.
In practice, revenue recycling options require careful policy design taking into account several considerations, such as the time‑lag between revenue collection and redistribution or legal constraints on earmarking of revenues. A recent report by Cardenas Monar (2024[127]) highlights some of these.
Figure 5.11. 2012‑21 carbon pricing measures with revenue recycling (lumps-sum payments)
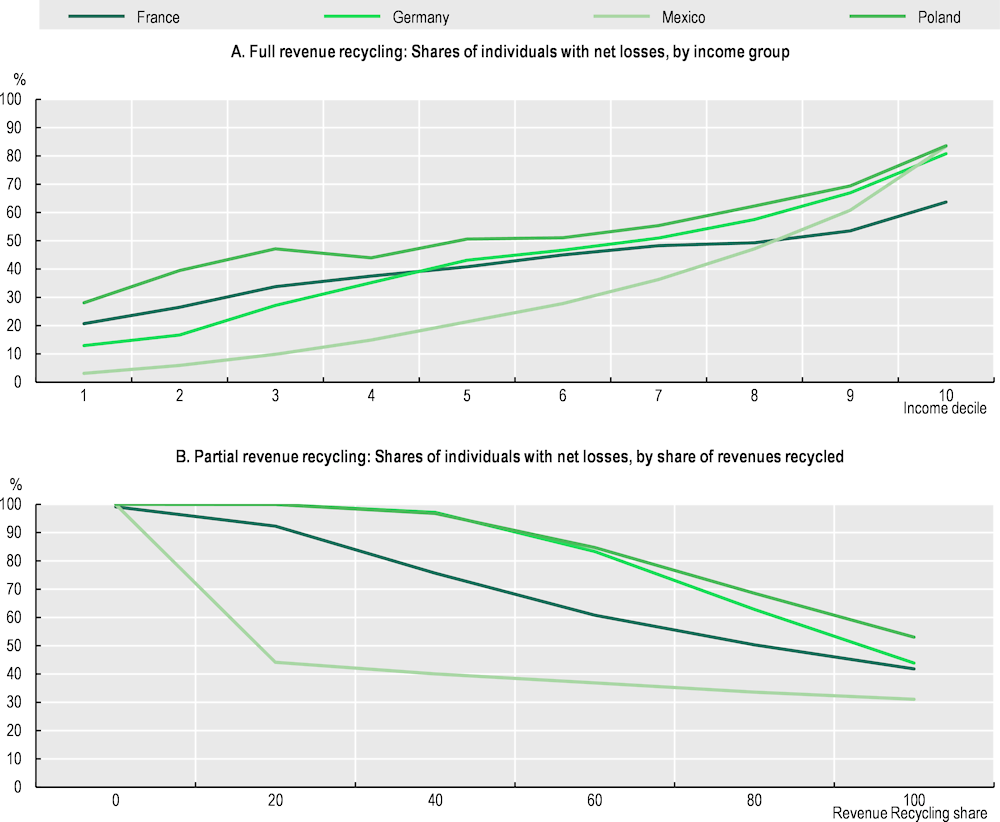
Note: Household compensation takes the form of uniform lump-sum transfers to each individual. Income deciles in Panel A refer to equivalised disposable household income. In order to remain agnostic as to the use of the remaining revenue, the modelling does not include the distributional impacts that the share of revenue not used for revenue recycling may have.
Source: OECD calculations using OECD Effective Carbon Rates data, IEA emissions factors for different fuels, World Input-Output Database (WIOD) and household budget surveys (2015 for EU countries and 2016 for Mexico).
5.5. Concluding remarks
This chapter assesses the impact of carbon pricing policies on households, discussing different channels for distributive effects and outlining an approach for quantifying household burdens stemming from higher consumption expenditures. The empirical assessment estimates carbon footprints for individual households in five countries and traces carbon prices from inputs to consumers across complex value chains. It employs granular data from the OECD’s Effective Carbon Rates database to estimate burdens from a wide range of carbon pricing reforms introduced during the 2012‑21 period.
Results largely confirm commonly held views about carbon pricing as impacting low-income and middle‑income groups in particular, even though emissions attributed to their consumption are only a fraction of those linked to high-income households. In the period under study, carbon pricing reforms often imposed only small burdens on households. After 2021, governments have sought to provide support to households and firms (e.g. through reduced carbon prices in the road transport sector) and inflation also reduced the real value of effective carbon rates in some cases. As current carbon prices remain well below levels that are considered in line with national and international climate commitments, future increases may be sizeable and rapid in some countries. The mostly regressive nature of reforms examined in this chapter show that the distributional impacts of future policy changes need to be scrutinised closely, both for reasons of equity and to ensure that reforms have the backing of voters.
The comparative results highlight big differences in distributional impacts between countries and policy measures, suggesting a number of policy levers for avoiding or limiting detrimental distributional impacts. Governments have employed a range of carbon pricing measures. The design of these matters not only for achieving environmental objectives but also for distributional outcomes. Carbon pricing revenues also provide leeway to compensate households. Uniform lump-sum compensation to all households is straightforward to implement, but it is, by definition, poorly targeted, which increases the cost of compensation measures and may limit the capacity to finance other priority programmes from carbon-pricing revenues. Untargeted compensation also fails to ensure adequate protection for some vulnerable households.
One prominent debate in the literature on recycling carbon pricing revenues relates to the so-called “equity-pollution dilemma” (Scruggs, 1998[128]; Heerink, Mulatu and Bulte, 2001[129]; Beiser‐McGrath and Busemeyer, 2023[130]). This refers to a situation where low-income households spend larger portions of their resources on carbon-intensive goods and services than the rich, and redistributing to the poor can be expected to increase total emission levels (an example of what is sometimes also referred to as the “rebound effect” of climate change mitigation). Any trade‑offs between equity and environmental objectives can present a formidable challenge, as households in poverty or material deprivation consume too little, rather than too much, and redistribution presents one key strategy for improving their living standards. Some country-specific studies have documented such a dilemma (Sager, 2019[131]). Results in this chapter confirm that total emissions from consumption are much higher at the top of the income distribution, but that emissions intensities (emissions relative to spending) can indeed be greater at lower income levels.
The results nuance earlier ones in three ways, however. First, the dilemma does not apply universally for all types of carbon pricing. For instance, fuel and energy do not always behave like necessities and data presented in this chapter shows that middle‑income and high-income households sometimes do spend larger shares of their income on transport fuel. Second, apart from the highest income group (top 10%) overall emissions intensities appear to differ only marginally between income groups, and carbon footprints are increasing in income and much higher for the top income group. In one country (Mexico), emissions intensities are significantly greater for high-income households, indicating that redistribution can simultaneously strengthen equity and environmental goals. Moreover, emissions vary not only by income but by other household characteristics as well. This points to opportunities to side‑step possible trade‑offs between reducing emissions and tackling inequality, e.g. by tailoring compensation strategies to characteristics such as region or age rather than to income as such. Finally, the results suggest that even partial revenue recycling can make many lower-income households better off. Retaining the remaining revenues to tackle underinvestment in energy efficiency (potentially complemented by the general budget), notably among disadvantaged groups, can help low-income households to reduce their reliance on carbon-intensive consumption and therefore address the underlying driver of any equity-pollution dilemmas.
The urgency of the climate change mitigation agenda suggests that one priority would be the regular monitoring of distributional impacts of past and prospective carbon pricing reforms and associated compensation measures, and the systematic communication of results to voters and stakeholders.38 Future research by the OECD could extend the applications in other country contexts than those presented in this chapter to deepen understanding the drivers of gains and losses and better assess the resulting policy challenges and the political economy of carbon pricing.
Moreover, further analysis could consider the distributional impact of trade‑related carbon leakage (whereby the introduction or intensification of domestic climate policies potentially leads to increased imports of more carbon-intensive products) and policy measures to tackle it, drawing on multi-region input-output data from the OECD Inter-Country Input-Output (ICIO) database (Smith et al., forthcoming[132]). Future work should also explore relaxing some of the assumptions made in the present analysis (Elgouacem et al., forthcoming[14]). One such assumption is the proportionality of carbon content and spending in a given spending category, as in reality emissions per spending unit can be expected to differ by price level, e.g. between budget and luxury restaurants or between discount and premium holiday travel. Explicit modelling of behavioural responses to carbon price changes also represents an interesting avenue for future research, and the recent period of large price swings represents an opportunity to study this more closely. Finally, carbon pricing is one instrument in the portfolio of climate change mitigation policies and understanding the distributional impacts of other mitigation policies is equally important.
The research agenda for assessments of distributional impacts is also closely linked to the broader evolving evidence on the economic impact of climate change, and of policies to avert it. A key question concerns the counterfactual that is adopted in distributional studies. While the status quo, as adopted in this chapter, can be a natural starting point, any losses also need to be compared with the cost of policy inaction or, vice versa, the benefits of mitigation that are the very rationale for climate‑change mitigation (Tovar Reaños and Lynch, 2022[133]) – see also Chapter 2. The scale of economic damages from climate change remains uncertain (Auffhammer, 2018[134]; Howard and Sterner, 2022[135]), though recent studies tend to find they are very large and significant (Bilal and Känzig, 2024[136]). In the long run, however, they are by definition of the same order of magnitude as carbon prices that internalise all the negative externalities of GHG emissions.
References
[82] Andersson, J. and G. Atkinson (2020), “The distributional effects of a carbon tax: The role of income inequality”, Centre for Climate Change Economics and Policy Working Paper 378/Grantham Research Institute on Climate Change and the Environment Working Paper 349, London School of Economics and Political Science.
[163] André, M. et al. (forthcoming), “Challenges in measuring the distribution of carbon footprints: the role of product and price heterogeneity”, Documents de travail INSEE.
[124] Antosiewicz, M. et al. (2022), “Distributional effects of emission pricing in a carbon-intensive economy: The case of Poland”, Energy Policy, Vol. 160, p. 112678, https://doi.org/10.1016/j.enpol.2021.112678.
[60] Arias, P. et al. (eds.) (2023), IPCC, 2023: Climate Change 2023: Synthesis Report. Contribution of Working Groups I, II and III to the Sixth Assessment Report of the Intergovernmental Panel on Climate Change [Core Writing Team, H. Lee and J. Romero (eds.)]. IPCC, Geneva, Switzerland., Intergovernmental Panel on Climate Change (IPCC), https://doi.org/10.59327/ipcc/ar6-9789291691647.
[134] Auffhammer, M. (2018), “Quantifying Economic Damages from Climate Change”, Journal of Economic Perspectives, Vol. 32/4, pp. 33-52, https://doi.org/10.1257/jep.32.4.33.
[2] Baranzini, A., J. Goldemberg and S. Speck (2000), “A future for carbon taxes”, Ecological Economics, Vol. 32/3, pp. 395-412, https://doi.org/10.1016/s0921-8009(99)00122-6.
[1] Baumol, W. and W. Oates (1988), The theory of environmental policy, Cambridge University Press.
[130] Beiser‐McGrath, L. and M. Busemeyer (2023), “Carbon inequality and support for carbon taxation”, European Journal of Political Research, https://doi.org/10.1111/1475-6765.12647.
[136] Bilal, A. and D. Känzig (2024), “The Macroeconomic Impact of Climate Change: Global vs. Local Temperature”, NBER Working Papers, No. 32450, National Bureau of Economic Research, Cambridge, MA, https://doi.org/10.3386/w32450.
[46] Bistline, J. et al. (2023), “Emissions and energy impacts of the Inflation Reduction Act”, Science, Vol. 380/6652, pp. 1324-1327, https://doi.org/10.1126/science.adg3781.
[47] Bistline, J., N. Mehrotra and C. Wolfram (2023), “Economic implications of the climate provisions of the Inflation Reduction Act”, NBER Working Paper, Vol. 31267.
[26] Blanchard, O., C. Gollier and J. Tirole (2023), “The Portfolio of Economic Policies Needed to Fight Climate Change”, Annual Review of Economics, Vol. 15/1, pp. 689-722, https://doi.org/10.1146/annurev-economics-051520-015113.
[41] Borenstein, S. and L. Davis (2016), “The Distributional Effects of US Clean Energy Tax Credits”, Tax Policy and the Economy, Vol. 30/1, pp. 191-234, https://doi.org/10.1086/685597.
[151] Bosman, J. (2008), “Unlikely allies campaign for a gas-tax holiday”, New York Times, p. A18.
[5] Boyce, J. (2018), “Carbon Pricing: Effectiveness and Equity”, Ecological Economics, Vol. 150, pp. 52-61, https://doi.org/10.1016/j.ecolecon.2018.03.030.
[44] Braungardt, S. et al. (2023), “Banning boilers: An analysis of existing regulations to phase out fossil fuel heating in the EU”, Renewable and Sustainable Energy Reviews, Vol. 183, p. 113442, https://doi.org/10.1016/j.rser.2023.113442.
[162] Brewer, M., B. Etheridge and C. O’Dea (2017), “Why are Households that Report the Lowest Incomes So Well-off?”, The Economic Journal, Vol. 127/605, pp. F24-F49, https://doi.org/10.1111/ecoj.12334.
[108] Brons, M. et al. (2008), “A meta-analysis of the price elasticity of gasoline demand. A SUR approach”, Energy Economics, Vol. 30/5, pp. 2105-2122, https://doi.org/10.1016/j.eneco.2007.08.004.
[115] Browne, J. and H. Immervoll (2017), “Mechanics of replacing benefit systems with a basic income: comparative results from a microsimulation approach”, The Journal of Economic Inequality, Vol. 15/4, pp. 325-344, https://doi.org/10.1007/s10888-017-9366-6.
[48] Brown, M. et al. (2023), Tax Credits for Clean Electricity: The Distributional Impacts of Supply-Push Policies in the Power Sector, National Bureau of Economic Research, Cambridge, MA, https://doi.org/10.3386/w31621.
[52] Bruegge, C., T. Deryugina and E. Myers (2019), “The Distributional Effects of Building Energy Codes”, Journal of the Association of Environmental and Resource Economists, Vol. 6/S1, pp. S95-S127, https://doi.org/10.1086/701189.
[3] Büchs, M., N. Bardsley and S. Duwe (2011), “Who bears the brunt? Distributional effects of climate change mitigation policies”, Critical Social Policy, Vol. 31/2, pp. 285-307, https://doi.org/10.1177/0261018310396036.
[85] Büchs, M., D. Ivanova and S. Schnepf (2021), “Fairness, effectiveness, and needs satisfaction: new options for designing climate policies”, Environmental Research Letters, Vol. 16/12, p. 124026, https://doi.org/10.1088/1748-9326/ac2cb1.
[100] Budolfson, M. et al. (2021), “Climate action with revenue recycling has benefits for poverty, inequality and well-being”, Nature Climate Change, Vol. 11/12, pp. 1111-1116, https://doi.org/10.1038/s41558-021-01217-0.
[142] Cai, M. and T. Vandyck (2020), “Bridging between economy-wide activity and household-level consumption data: Matrices for European countries.”, Data in brief, Vol. 30/105395, https://doi.org/10.1016/j.dib.2020.105395.
[80] Callan, T. et al. (2009), “The distributional implications of a carbon tax in Ireland”, Energy Policy, Vol. 37/2, pp. 407-412, https://doi.org/10.1016/j.enpol.2008.08.034.
[96] Campagnolo, L. and E. De Cian (2022), “Distributional consequences of climate change impacts on residential energy demand across Italian households”, Energy Economics, Vol. 110, p. 106020, https://doi.org/10.1016/j.eneco.2022.106020.
[127] Cardenas Monar, D. (2024), “Maximising benefits of carbon pricing through carbon revenue use: A review of international experiences”, I4CE, Institute for Climate Economics, https://www.i4ce.org/wp-content/uploads/2024/05/240515-i4ce-EUCDs-use-of-revenues-66pA4-Web.pdf.
[77] Causa, O. et al. (2022), “A cost-of-living squeeze? Distributional implications of rising inflation”, OECD Economics Department Working Papers, No. 1744, OECD Publishing, Paris, https://doi.org/10.1787/4b7539a3-en.
[141] Cazcarro, I. et al. (2020), “Linking multisectoral economic models and consumption surveys for the European Union”, Economic Systems Research, Vol. 34/1, pp. 22-40, https://doi.org/10.1080/09535314.2020.1856044.
[112] Chancel, L., P. Bothe and T. Voituriez (2023), “Climate Inequality Report 2023 - Fair taxes for a sustainable future in the Global South”, World Inequality Lab Study 2023/1, https://wid.world/wp-content/uploads/2023/01/CBV2023-ClimateInequalityReport-3.pdf.
[20] Climate Watch (2024), Climate Watch, https://www.climatewatchdata.org.
[76] Cronin, J., D. Fullerton and S. Sexton (2019), “Vertical and Horizontal Redistributions from a Carbon Tax and Rebate”, Journal of the Association of Environmental and Resource Economists, Vol. 6/S1, pp. S169-S208, https://doi.org/10.1086/701191.
[116] D’Arcangelo, F. et al. (2022), “A framework to decarbonise the economy”, OECD Economic Policy Papers, No. 31, OECD Publishing, Paris, https://doi.org/10.1787/4e4d973d-en.
[11] D’Arcangelo, F. et al. (2022), “Estimating the CO2 emission and revenue effects of carbon pricing: New evidence from a large cross-country dataset”, OECD Economics Department Working Papers, No. 1732, OECD Publishing, Paris, https://doi.org/10.1787/39aa16d4-en.
[110] Dahl, C. (2012), “Measuring global gasoline and diesel price and income elasticities”, Energy Policy, Vol. 41, pp. 2-13, https://doi.org/10.1016/j.enpol.2010.11.055.
[42] Davis, L. (2023), The Economic Determinants of Heat Pump Adoption, National Bureau of Economic Research, Cambridge, MA, https://doi.org/10.3386/w31344.
[167] Davis, L. and P. Gertler (2015), “Contribution of air conditioning adoption to future energy use under global warming”, Proceedings of the National Academy of Sciences, Vol. 112/19, pp. 5962-5967, https://doi.org/10.1073/pnas.1423558112.
[49] Davis, L. and C. Knittel (2019), “Are Fuel Economy Standards Regressive?”, Journal of the Association of Environmental and Resource Economists, Vol. 6/S1, pp. S37-S63, https://doi.org/10.1086/701187.
[7] Dechezleprêtre, A. et al. (2022), “Fighting climate change: International attitudes toward climate policies”, OECD Economics Department Working Papers, No. 1714, OECD Publishing, Paris, https://doi.org/10.1787/3406f29a-en.
[91] Dorband, I. et al. (2019), “Poverty and distributional effects of carbon pricing in low- and middle-income countries – A global comparative analysis”, World Development, Vol. 115, pp. 246-257, https://doi.org/10.1016/j.worlddev.2018.11.015.
[35] Douenne, T. and A. Fabre (2022), “Yellow Vests, Pessimistic Beliefs, and Carbon Tax Aversion”, American Economic Journal: Economic Policy, Vol. 14/1, pp. 81-110, https://doi.org/10.1257/pol.20200092.
[118] Douenne, T. and A. Fabre (2020), “French attitudes on climate change, carbon taxation and other climate policies”, Ecological Economics, Vol. 169, p. 106496, https://doi.org/10.1016/j.ecolecon.2019.106496.
[23] Drews, S., I. Savin and J. van den Bergh (2024), “A Global Survey of Scientific Consensus and Controversy on Instruments of Climate Policy”, Ecological Economics, Vol. 218, https://doi.org/10.1016/j.ecolecon.2023.108098.
[123] Ekins, P. et al. (2011), “The implications for households of environmental tax reform (ETR) in Europe”, Ecological Economics, Vol. 70/12, pp. 2472-2485, https://doi.org/10.1016/j.ecolecon.2011.08.004.
[14] Elgouacem, A. et al. (forthcoming), “A just transition? Carbon pricing reforms and household burdens”, OECD Taxation Working Papers, OECD Publishing, Paris.
[155] Emmerling, J., P. Andreoni and M. Tavoni (2024), “Global inequality consequences of climate policies when accounting for avoided climate impacts”, Cell Reports Sustainability, Vol. 1/1, p. 100008, https://doi.org/10.1016/j.crsus.2023.100008.
[109] Espey, M. (1998), “Gasoline demand revisited: an international meta-analysis of elasticities”, Energy Economics, Vol. 20/3, pp. 273-295, https://doi.org/10.1016/s0140-9883(97)00013-3.
[62] European Commission (2021), Green taxation and other economic instruments - Internalising environmental costs to make the polluter pay, https://environment.ec.europa.eu/publications/green-taxation-and-other-economic-instruments-internalising-environmental-costs-make-polluter-pay_en.
[168] Eurostat (2023), 9% of EU population unable to keep home warm in 2022, https://ec.europa.eu/eurostat/web/products-eurostat-news/w/ddn-20230911-1.
[65] Fabra, N. and M. Reguant (2014), “Pass-Through of Emissions Costs in Electricity Markets”, American Economic Review, Vol. 104/9, pp. 2872-2899, https://doi.org/10.1257/aer.104.9.2872.
[99] Feindt, S. et al. (2021), “Understanding regressivity: Challenges and opportunities of European carbon pricing”, Energy Economics, Vol. 103, p. 105550, https://doi.org/10.1016/j.eneco.2021.105550.
[89] Flues, F. and A. Thomas (2015), “The distributional effects of energy taxes”, OECD Taxation Working Papers, No. 23, OECD Publishing, Paris, https://doi.org/10.1787/5js1qwkqqrbv-en.
[148] Flues, F. and K. Van Dender (2017), “Permit allocation rules and investment incentives in emissions trading systems”, OECD Taxation Working Papers, No. 33, OECD Publishing, Paris, https://doi.org/10.1787/c3acf05e-en.
[90] Flues, F. and K. van Dender (2017), “The impact of energy taxes on the affordability of domestic energy”, OECD Taxation Working Papers, No. 30, OECD Publishing, Paris, https://doi.org/10.1787/08705547-en.
[121] FOEN (Swiss Federal Office for the Environment) (2023), Redistribution of the CO2 levy, https://www.bafu.admin.ch/bafu/en/home/topics/climate/info-specialists/reduction-measures/co2-levy/redistribution.html.
[70] G20 Leaders Statement (2009), G20 Leaders Statement: The Pittsburgh Summit, https://www.oecd.org/g20/summits/pittsburgh/G20-Pittsburgh-Leaders-Declaration.pdf.
[125] García-Muros, X., J. Morris and S. Paltsev (2022), “Toward a just energy transition: A distributional analysis of low-carbon policies in the USA”, Energy Economics, Vol. 105, p. 105769, https://doi.org/10.1016/j.eneco.2021.105769.
[67] Garsous, G. et al. (2023), “Net effective carbon rates”, OECD Taxation Working Papers, No. 61, OECD Publishing, Paris, https://doi.org/10.1787/279e049e-en.
[43] Giraudet, L., C. Bourgeois and P. Quirion (2021), “Policies for low-carbon and affordable home heating: A French outlook”, Energy Policy, Vol. 151, p. 112140, https://doi.org/10.1016/j.enpol.2021.112140.
[34] Giraudet, L., C. Guivarch and P. Quirion (2011), “Comparing and Combining Energy Saving Policies: Will Proposed Residential Sector Policies Meet French Official Targets?”, The Energy Journal, Vol. 32/1_suppl, pp. 213-242, https://doi.org/10.5547/issn0195-6574-ej-vol32-si1-12.
[78] Gonzalez, F. (2012), “Distributional effects of carbon taxes: The case of Mexico”, Energy Economics, Vol. 34/6, pp. 2102-2115, https://doi.org/10.1016/j.eneco.2012.03.007.
[16] Goulder, L. (1995), “Environmental taxation and the double dividend: A reader’s guide”, International Tax and Public Finance, Vol. 2/2, pp. 157-183, https://doi.org/10.1007/bf00877495.
[160] Grasso, M. (ed.) (2023), “Income-based U.S. household carbon footprints (1990–2019) offer new insights on emissions inequality and climate finance”, PLOS Climate, Vol. 2/8, p. e0000190, https://doi.org/10.1371/journal.pclm.0000190.
[126] Guillemette, Y. and J. Château (2023), “Long-term scenarios: incorporating the energy transition”, OECD Economic Policy Papers, No. 33, OECD Publishing, Paris, https://doi.org/10.1787/153ab87c-en.
[107] Havranek, T., Z. Irsova and K. Janda (2012), “Demand for gasoline is more price-inelastic than commonly thought”, Energy Economics, Vol. 34/1, pp. 201-207, https://doi.org/10.1016/j.eneco.2011.09.003.
[129] Heerink, N., A. Mulatu and E. Bulte (2001), “Income inequality and the environment: aggregation bias in environmental Kuznets curves”, Ecological Economics, Vol. 38, pp. 359–367.
[117] Hemmerlé, Y. et al. (2023), “Aiming better: Government support for households and firms during the energy crisis”, OECD Economic Policy Papers, No. 32, OECD Publishing, Paris, https://doi.org/10.1787/839e3ae1-en.
[54] High-Level Commission on Carbon Prices (2017), Report of the High-Level Commission on Carbon Prices, World Bank, Washington, DC, https://doi.org/10.7916/d8-w2nc-4103.
[150] Hobbie, H., M. Schmidt and D. Möst (2019), “Windfall profits in the power sector during phase III of the EU ETS: Interplay and effects of renewables and carbon prices”, Journal of Cleaner Production, Vol. 240, p. 118066, https://doi.org/10.1016/j.jclepro.2019.118066.
[135] Howard, P. and T. Sterner (2022), “Between Two Worlds: Methodological and Subjective Differences in Climate Impact Meta-Analyses”, Resources for the Future Working Paper, Vol. 22/10.
[152] Howard, P. and T. Sterner (2017), “Few and Not So Far Between: A Meta-analysis of Climate Damage Estimates”, Environmental and Resource Economics, Vol. 68/1, pp. 197-225, https://doi.org/10.1007/s10640-017-0166-z.
[19] IEA (2024), CO2 Emissions in 2023 - A new record high, but is there light at the end of the tunnel?, International Energy Agency, Paris, https://www.iea.org/reports/co2-emissions-in-2023.
[144] IEA (2024), “Extended world energy balances”, IEA World Energy Statistics and Balances (database), https://doi.org/10.1787/data-00513-en (accessed on 26 June 2024).
[17] IEA (2022), “World Energy Outlook 2022”, IEA, Paris, License: CC BY 4.0 (report); CC BY NC.
[68] IEA (2021), Database documentation - Greenhouse gas emissions from energy - 2021 Edition, https://iea.blob.core.windows.net/assets/d82f9e09-9080-4dcf-9100-0ba686536341/WORLD_GHG_Documentation.pdf.
[64] IEA (2020), “World Energy Balances - 2020 Edition - Database documentation”, https://www.iea.org/subscribe-to-data-services/world-energy-balances-and-statistics.
[122] Immervoll, H. (forthcoming), “Financing social protection: The role of earmarking”.
[13] Immervoll, H. et al. (2023), “Who pays for higher carbon prices?: Illustration for Lithuania and a research agenda”, OECD Social, Employment and Migration Working Papers, No. 283, OECD Publishing, Paris, https://doi.org/10.1787/8f16f3d8-en.
[61] Interagency Working Group on Social Cost of Greenhouse Gases (IWG) (2021), Technical Support Document: Social Cost of Carbon, Methane, and Nitrious Oxide: Interim Estimates under Executive Order 13990.
[156] Inter-American Development Bank (2023), “Social protection and climate change: How can we protect the most vulnerable households against new climate threats?”, Policy Brief, Vol. IDB-PB-00375.
[145] IPCC (2006), Guidelines for National Greenhouse Gas Inventories, http://www.ipcc-nggip.iges.or.jp/public/2006gl/vol2.html.
[158] Irfany, M. and S. Klasen (2017), “Affluence and emission tradeoffs: evidence from Indonesian households’ carbon footprint”, Environment and Development Economics, Vol. 22/5, pp. 546-570, https://doi.org/10.1017/s1355770x17000262.
[25] Jaakkola, N., F. Van der Ploeg and A. Venables (2023), ““Big Push” Green Industrial Policy”, EconPol Forum, Vol. 24.
[50] Jacobsen, M. (2013), “Evaluating US fuel economy standards in a model with producer and household heterogeneity”, American Economic Journal: Economic Policy, Vol. 5/2, pp. 148-187, https://doi.org/10.1257/pol.5.2.148.
[139] Kitzes, J. (2013), “An Introduction to Environmentally-Extended Input-Output Analysis”, Resources, Vol. 2/4, pp. 489-503, https://doi.org/10.3390/resources2040489.
[84] Klenert, D. and L. Mattauch (2016), “How to make a carbon tax reform progressive: The role of subsistence consumption”, Economics Letters, Vol. 138, pp. 100-103, https://doi.org/10.1016/j.econlet.2015.11.019.
[98] Klenert, D. et al. (2018), “Making carbon pricing work for citizens”, Nature Climate Change, Vol. 8/8, pp. 669-677, https://doi.org/10.1038/s41558-018-0201-2.
[113] Konjunktur Institutet (2023), “Miljö, ekonomi och politik 2023 (Environmental economic report 2023)”, https://www.konj.se/publikationer/miljoekonomisk-rapport/miljoekonomisk-rapport/2023-12-06-fordelningseffekter-av-klimatpolitiken-hanteras-bast-med-annan-politik.html.
[86] Köppl, A. and M. Schratzenstaller (2022), “Carbon taxation: A review of the empirical literature”, Journal of Economic Surveys, Vol. 37/4, pp. 1353-1388, https://doi.org/10.1111/joes.12531.
[105] Labandeira, X., J. Labeaga and X. López-Otero (2017), “A meta-analysis on the price elasticity of energy demand”, Energy Policy, Vol. 102, pp. 549-568, https://doi.org/10.1016/j.enpol.2017.01.002.
[74] Labrousse, C. and Y. Perdereau (2024), “Geography versus income: the heterogeneous effects of carbon taxation”, Paris School of Economics Working Paper, https://pse.hal.science/halshs-04464900v1.
[32] Lamb, W. et al. (2020), “What are the social outcomes of climate policies? A systematic map and review of the ex-post literature”, Environmental Research Letters, Vol. 15/11, p. 113006, https://doi.org/10.1088/1748-9326/abc11f.
[101] Landis, F. (2019), “Cost distribution and equity of climate policy in Switzerland”, Swiss Journal of Economics and Statistics, Vol. 155/1, https://doi.org/10.1186/s41937-019-0038-2.
[37] Lekavičius, V. et al. (2020), “Distributional impacts of investment subsidies for residential energy technologies”, Renewable and Sustainable Energy Reviews, Vol. 130, p. 109961, https://doi.org/10.1016/j.rser.2020.109961.
[24] Lenton, T. et al. (2023), The Global Tipping Points Report 2023, University of Exeter.
[137] Leontieff, W. (1951), “Input-output economics”, Scientific American, Vol. 185, pp. 15-21.
[165] Lévay, P., T. Goedemé and G. Verbist (2023), “Income and expenditure elasticity of household carbon footprints. Some methodological considerations”, Ecological Economics, Vol. 212, p. 107893, https://doi.org/10.1016/j.ecolecon.2023.107893.
[40] Levinson, A. (2019), “Energy Efficiency Standards Are More Regressive Than Energy Taxes: Theory and Evidence”, Journal of the Association of Environmental and Resource Economists, Vol. 6/S1, pp. S7-S36, https://doi.org/10.1086/701186.
[36] Lihtmaa, L., D. Hess and K. Leetmaa (2018), “Intersection of the global climate agenda with regional development: Unequal distribution of energy efficiency-based renovation subsidies for apartment buildings”, Energy Policy, Vol. 119, pp. 327-338, https://doi.org/10.1016/j.enpol.2018.04.013.
[92] Mardones, C., L. Di Capua and A. Vogt-Schilb (2023), “Improving the design of cash transfers to reduce the regressive effects of a carbon tax in Latin American countries-a look at territorial heterogeneity”, Applied Economics, pp. 1-12, https://doi.org/10.1080/00036846.2023.2288062.
[33] Markkanen, S. and A. Anger-Kraavi (2019), “Social impacts of climate change mitigation policies and their implications for inequality”, Climate Policy, Vol. 19/7, pp. 827-844, https://doi.org/10.1080/14693062.2019.1596873.
[119] Marten, M. and K. van Dender (2019), “The use of revenues from carbon pricing”, OECD Taxation Working Papers, No. 43, OECD Publishing, Paris, https://doi.org/10.1787/3cb265e4-en.
[153] Metcalf, G. (2023), Five Myths About Carbon Pricing, National Bureau of Economic Research, Cambridge, MA, https://doi.org/10.3386/w31104.
[143] Metcalf, G. (2021), “Carbon Taxes in Theory and Practice”, Annual Review of Resource Economics, Vol. 13/1, pp. 245-265, https://doi.org/10.1146/annurev-resource-102519-113630.
[59] Metcalf, G. and J. Stock (2023), “The Macroeconomic Impact of Europe’s Carbon Taxes”, American Economic Journal: Macroeconomics, Vol. 15/3, pp. 265-286, https://doi.org/10.1257/mac.20210052.
[138] Miller, R. and P. Blair (2009), Input-Output Analysis, Cambridge University Press, https://doi.org/10.1017/cbo9780511626982.
[75] Missbach, L., J. Steckel and A. Vogt-Schilb (2024), “Cash transfers in the context of carbon pricing reforms in Latin America and the Caribbean”, World Development, Vol. 173, https://doi.org/10.1016/j.worlddev.2023.106406.
[140] Mongelli, I., F. Neuwahl and J. Rueda-Cantuche (2010), “Integrating a household demand system in the input–output framework. Methodological aspects and modelling implications”, Economic Systems Research, Vol. 22/3, pp. 201-222, https://doi.org/10.1080/09535314.2010.501428.
[114] Muris, C. (ed.) (2023), “Implementation of carbon pricing in an aging world calls for targeted protection schemes”, PNAS Nexus, Vol. 2/7, https://doi.org/10.1093/pnasnexus/pgad209.
[66] Nazifi, F., S. Trück and L. Zhu (2021), “Carbon pass-through rates on spot electricity prices in Australia”, Energy Economics, Vol. 96, p. 105178, https://doi.org/10.1016/j.eneco.2021.105178.
[10] Network for Greening the Finanical System (2023), NGFS Scenarios for central banks and supervisors, NGFS.
[56] Nordhaus, W. (1991), “A sketch of the economics of the greenhouse effect”, Amercian Economic Review, Vol. 81/2, pp. 146-150.
[8] OECD (2023), Effective Carbon Rates 2023: Pricing Greenhouse Gas Emissions through Taxes and Emissions Trading, OECD Series on Carbon Pricing and Energy Taxation, OECD Publishing, Paris, https://doi.org/10.1787/b84d5b36-en.
[6] OECD (2023), How Green is Household Behaviour?: Sustainable Choices in a Time of Interlocking Crises, OECD Studies on Environmental Policy and Household Behaviour, OECD Publishing, Paris, https://doi.org/10.1787/2bbbb663-en.
[27] OECD (2023), Net Zero+: Climate and Economic Resilience in a Changing World, OECD Publishing, Paris, https://doi.org/10.1787/da477dda-en.
[164] OECD (2023), OECD Economic Surveys: European Union and Euro Area 2023, OECD Publishing, Paris, https://doi.org/10.1787/7ebe8cc3-en.
[103] OECD (2023), Reform Options for Lithuanian Climate Neutrality by 2050, OECD Publishing, Paris, https://doi.org/10.1787/0d570e99-en.
[18] OECD (2023), The Climate Action Monitor 2023: Providing Information to Monitor Progress Towards Net-Zero, OECD Publishing, Paris, https://doi.org/10.1787/60e338a2-en.
[72] OECD (2022), “Coping with the cost-of-living crisis: Income support for working-age individuals and their families”, OECD, Paris, https://www.oecd.org/social/Income-support-for-working-age-individuals-and-their-families.pdf.
[12] OECD (2022), Pricing Greenhouse Gas Emissions: Turning Climate Targets into Climate Action, OECD Series on Carbon Pricing and Energy Taxation, OECD Publishing, Paris, https://doi.org/10.1787/e9778969-en.
[9] OECD (2022), “Towards a sustainable recovery? Carbon pricing policy changes during COVID-19”, OECD Policy Responses to Coronavirus (COVID-19), OECD Publishing, Paris, https://doi.org/10.1787/92464d20-en.
[166] OECD (2021), OECD Companion to the Inventory of Support Measures for Fossil Fuels 2021, OECD Publishing, Paris, https://doi.org/10.1787/e670c620-en.
[146] OECD (2019), Taxing Energy Use 2019: Using Taxes for Climate Action, OECD Publishing, Paris, https://doi.org/10.1787/058ca239-en.
[15] OECD (2016), Effective Carbon Rates: Pricing CO2 through Taxes and Emissions Trading Systems, OECD Series on Carbon Pricing and Energy Taxation, OECD Publishing, Paris, https://doi.org/10.1787/9789264260115-en.
[69] OECD (2016), Effective Carbon Rates: Pricing CO2 through Taxes and Emissions Trading Systems, OECD Series on Carbon Pricing and Energy Taxation, OECD Publishing, Paris, https://doi.org/10.1787/9789264260115-en.
[147] OECD (2015), Taxing Energy Use 2015: OECD and Selected Partner Economies, OECD Publishing, Paris, https://doi.org/10.1787/9789264232334-en.
[71] OECD/IEA (2021), Update on recent progress in reform of inefficient fossil-fuel subsidies that encourage wasteful consumption, http://www.oecd.org/fossil-fuels/publicationsandfurtherreading/OECD-IEA-G20-Fossil-Fuel-Subsidies-Reform-Update-2021.pdf.
[81] Ohlendorf, N. et al. (2020), “Distributional Impacts of Carbon Pricing: A Meta-Analysis”, Environmental and Resource Economics, Vol. 78/1, pp. 1-42, https://doi.org/10.1007/s10640-020-00521-1.
[157] Pachauri, S. (2004), “An analysis of cross-sectional variations in total household energy requirements in India using micro survey data”, Energy Policy, Vol. 32/15, pp. 1723-1735, https://doi.org/10.1016/s0301-4215(03)00162-9.
[57] Pearce, D. (1991), “The role of carbon taxes in adjusting to global warming”, Economic Journal, Vol. 101/407, pp. 938-948, https://doi.org/10.2307/2233865.
[31] Peñasco, C., L. Anadón and E. Verdolini (2021), “Systematic review of the outcomes and trade-offs of ten types of decarbonization policy instruments”, Nature Climate Change, Vol. 11/3, pp. 257-265, https://doi.org/10.1038/s41558-020-00971-x.
[55] Pigou, A. (1920), The economics of welfare, Macmillan.
[87] Pizer, W. and S. Sexton (2019), “The Distributional Impacts of Energy Taxes”, Review of Environmental Economics and Policy, Vol. 13/1, pp. 104-123, https://doi.org/10.1093/reep/rey021.
[104] Pottier, A. (2022), “Expenditure elasticity and income elasticity of GHG emissions: A survey of literature on household carbon footprint”, Ecological Economics, Vol. 192, p. 107251, https://doi.org/10.1016/j.ecolecon.2021.107251.
[159] Pottier, A. et al. (2021), “Who Emits CO2? Landscape of Ecological Inequalities in France from a Critical Perspective”, Fondazione Eni Enrico Mattei (FEEM) Working Paper, Vol. 2021/014.
[22] Pouille, C. et al. (2023), “Paris-consistent climate change mitigation scenarios: A framework for emissions pathway classification in line with global mitigation objectives”, OECD Environment Working Papers, No. 222, OECD Publishing, Paris, https://doi.org/10.1787/0de87ef8-en.
[149] Quirion, P. (2007), “Comment faut-il distribuer les quotas échangeables de gaz à effet de serre ?”, Revue française d’économie, Vol. 22/2, pp. 129-164, https://doi.org/10.3406/rfeco.2007.1651.
[30] Rausch, S., G. Metcalf and J. Reilly (2011), “Distributional impacts of carbon pricing: A general equilibrium approach with micro-data for households”, Energy Economics, Vol. 33, pp. S20-S33, https://doi.org/10.1016/j.eneco.2011.07.023.
[154] Rausch, S. and G. Schwarz (2016), “Household heterogeneity, aggregation, and the distributional impacts of environmental taxes”, Journal of Public Economics, Vol. 138, pp. 43-57, https://doi.org/10.1016/j.jpubeco.2016.04.004.
[94] Renner, S. (2018), “Poverty and distributional effects of a carbon tax in Mexico”, Energy Policy, Vol. 112, pp. 98-110, https://doi.org/10.1016/j.enpol.2017.10.011.
[97] Renner, S., J. Lay and H. Greve (2018), “Household welfare and CO2 emission impacts of energy and carbon taxes in Mexico”, Energy Economics, Vol. 72, pp. 222-235, https://doi.org/10.1016/j.eneco.2018.04.009.
[63] Rennert, K. et al. (2022), “Comprehensive evidence implies a higher social cost of CO2”, Nature, Vol. 610/7933, pp. 687-692, https://doi.org/10.1038/s41586-022-05224-9.
[53] Robinson, H. (1985), “Who pays for industrial pollution abatement?”, The Review of Economics and Statistics, pp. 702-706.
[29] Rudolph, L., N. Beyeler and L. Patel (2022), “The Inflation Reduction Act - a Historic Piece of Climate and Health Legislation”, The Journal of Climate Change and Health, Vol. 7, p. 100172, https://doi.org/10.1016/j.joclim.2022.100172.
[131] Sager, L. (2019), “Income inequality and carbon consumption: Evidence from Environmental Engel curves”, Energy Economics, Vol. 84, p. 104507, https://doi.org/10.1016/j.eneco.2019.104507.
[79] Sajeewani, D., M. Siriwardana and J. McNeill (2015), “Household distributional and revenue recycling effects of the carbon price in Australia”, Climate Change Economics, Vol. 06/03, p. 1550012, https://doi.org/10.1142/s2010007815500128.
[102] Sallee, J. (2019), Pigou Creates Losers: On the Implausibility of Achieving Pareto Improvements from Efficiency-Enhancing Policies, National Bureau of Economic Research, Cambridge, MA, https://doi.org/10.3386/w25831.
[128] Scruggs, L. (1998), “Political and economic inequality and the environment”, Ecological Economics, Vol. 26/3, pp. 259–275, https://doi.org/10.1016/S0921-8009(97)00118-3.
[58] Sen, S. and H. Vollebergh (2018), “The effectiveness of taxing the carbon content of energy consumption”, Journal of Environmental Economics and Management, Vol. 92, pp. 74-99, https://doi.org/10.1016/j.jeem.2018.08.017.
[132] Smith, J. et al. (forthcoming), “Carbon rates embodied in trade”, OECD Taxation Working Papers.
[73] Sologon, D. et al. (2022), Welfare and Distributional Impact of Soaring Prices in Europe, IZA Discussion Paper Series.
[161] Starr, J. et al. (2023), “Assessing U.S. consumers’ carbon footprints reveals outsized impact of the top 1%”, Ecological Economics, Vol. 205, p. 107698, https://doi.org/10.1016/j.ecolecon.2022.107698.
[93] Steckel, J. et al. (2021), “Distributional impacts of carbon pricing in developing Asia”, Nature Sustainability, Vol. 4/11, pp. 1005-1014, https://doi.org/10.1038/s41893-021-00758-8.
[4] Tatham, M. and Y. Peters (2022), “Fueling opposition? Yellow vests, urban elites, and fuel taxation”, Journal of European Public Policy, pp. 1-25, https://doi.org/10.1080/13501763.2022.2148172.
[45] Torné, A. and E. Trutnevyte (2024), “Banning fossil fuel cars and boilers in Switzerland: Mitigation potential, justice, and the social structure of the vulnerable”, Energy Research & Social Science, Vol. 108, p. 103377, https://doi.org/10.1016/j.erss.2023.103377.
[133] Tovar Reaños, M. and M. Lynch (2022), “The benefits of action on implementing carbon taxation in Ireland: a demand system approach”, Journal of Environmental Planning and Management, pp. 1-25, https://doi.org/10.1080/09640568.2021.2006157.
[21] United Nations Environment Programme (2023), Emissions Gap Report 2023: Broken Record – Temperatures hit new highs, yet world fails to cut emissions (again), United Nations Environment Programme, https://doi.org/10.59117/20.500.11822/43922.
[83] Vogt-Schilb, A. et al. (2019), “Cash Transfers for Pro-Poor Carbon Taxes in Latin America and the Caribbean.”, Nature Sustainability, Vol. 2/10, pp. 941–948., https://doi.org/10.1038/s41893-019-0385-.
[111] Wadud, Z., D. Graham and R. Noland (2010), “Gasoline Demand with Heterogeneity in Household Responses”, he Energy Journal, Vol. 31/1, pp. 47-74, http://www.jstor.org/stable/41323270.
[88] Wang, Q. et al. (2016), “Distributional effects of carbon taxation”, Applied Energy, Vol. 184, pp. 1123-1131, https://doi.org/10.1016/j.apenergy.2016.06.083.
[51] West, S. (2009), Distributional effects of alternative vehicle pollution control policies, Routledge, London, https://doi.org/10.4324/9781315257570.
[39] West, S. (2004), “Distributional effects of alternative vehicle pollution control policies”, Journal of Public Economics, Vol. 88/3-4, pp. 735-757, https://doi.org/10.1016/s0047-2727(02)00186-x.
[95] West, S. and R. Williams (2004), “Estimates from a consumer demand system: implications for the incidence of environmental taxes”, Journal of Environmental Economics and Management, Vol. 47/3, pp. 535-558, https://doi.org/10.1016/j.jeem.2003.11.004.
[38] Winter, S. and L. Schlesewsky (2019), “The German feed-in tariff revisited - an empirical investigation on its distributional effects”, Energy Policy, Vol. 132, pp. 344-356, https://doi.org/10.1016/j.enpol.2019.05.043.
[169] World Bank (2023), State and trends of carbon pricing 2023, http://hdl.handle.net/10986/39796.
[120] World Bank (2019), Using Carbon Tax Revenues, World Bank, Washington, DC.
[28] Zachmann, G., G. Frederikson and G. Clayes (2018), Distributional effects of climate policies, Bruegel, Brussels.
[106] Zhu, X. et al. (2018), “A meta-analysis on the price elasticity and income elasticity of residential electricity demand”, Journal of Cleaner Production, Vol. 201, pp. 169-177, https://doi.org/10.1016/j.jclepro.2018.08.027.
Annex 5.A. Methodology: Carbon emissions from household consumption and resulting carbon price burdens
A household’s overall carbon price burden is a function of their consumption patterns (what and how much they consume) and the respective carbon prices assigned to these consumed goods and services. Estimating carbon price burdens at the household level involves assessing both direct emissions from fuel consumption and indirect emissions from the production of goods and services (including energy related expenditures such as electricity or public transport). This requires information on the impact of carbon prices on fuel prices, on the price of all other goods and services, and information on the structure of households’ consumption baskets. The impact of carbon prices on fuels is directly related to their carbon content and can be calculated using the carbon content of fuels per monetary unit. The impact of carbon prices on all other products requires information on energy consumed in the production process of goods and services.
A companion paper provides details on each of the key steps of the modelling framework, and how it was implemented in the context of this chapter (Elgouacem et al., forthcoming[14]). It describes:
the effective carbon rate (ECR) data;
the Input-Output (IO) model, which captures carbon emissions by sector and allows quantifying the pass-through of carbon taxes from inputs to the price of final consumer products and services;
the matching of input-output data with a household budget survey (HBS), which is needed to compute the carbon footprint from household consumption.
The ECR, IO and HBS data are a suitable basis for distributional analysis, as they are very granular – for instance, WIOD data differentiates between 56 industries and HBS data includes information for 301 (Eurostat data), 282 (Turkish data) and 745 (Mexican data) expenditure categories. Yet, as each data source uses different classification systems, several transformations are needed to enable links between them.
Input-Output modelling
Modelling the level of CO2 emissions from household consumption requires economy-wide data that capture carbon emissions by sector, and production linkages between sectors. This chapter employs the World Input Output Data (WIOD). The IO methodology, introduced by Leontieff (1951[137]) and discussed extensively by Miller and Blair (2009[138]) employs a matrix mapping the monetary flows between sectors and regions. A technology matrix contains input coefficients for all sectors in all regions and enables the calculation of economy-wide input requirements, which gives the input needed by a sector in one region from every other sector in all regions to produce one (monetary) unit of output.
The chapter employs an environmentally extended IO model, linking products to the indirect carbon emissions that occur in the course of production (Kitzes, 2013[139]). For each household, direct and indirect emissions are added to get final CO2 emissions from household consumption. Dividing emissions entry-wise by each sector’s output gives the level of CO2 emissions per monetary unit of the sector’s output vector. Direct emissions are released through households’ consumption of motor and domestic fuels. They are computedfor one euro worth of fuels using fuel-price data from the IEA (to calculate the carbon content of fuels per monetary unit) and carbon content per kWh per fuel sourced from the IPCC 2006 Guidelines for National Greenhouse Gas Inventories.
Matching ECR and input-output data with household expenditures
To approximate the carbon footprint from households’ consumption, it is necessary to match IO data with information on expenditure as reported in household budget surveys (HBS). HBS data commonly reports households’ consumption expenditure across different consumption purposes (COICOP), while WIOD tables report household final consumption expenditure in industry outputs terms (here, NACE rev 2). The integration of HBS data into multi-sectoral models is described in Mongelli, Neuwahl and Rueda-Cantuche (2010[140]) and Cazcarro et al. (2020[141]). Matching information from WIOD tables to HBS data involves translating goods by expenditure purpose into industry outputs using a bridging matrix, which maps the use of a product to satisfy a consumption purpose, so that the 𝑖𝑡ℎ element of matrix represents the use share of industry product j for consumption purpose i. As COICOP categories from HBS do not correspond directly to NACE sectors as recorded in WIOD, the matching procedure involves a crosswalk from COICOP to CPA (Classification of products by activity) to NACE using a bridging matrix (Cai and Vandyck, 2020[142]) and involving four main steps; (i) transforming COICOP to CPA; (ii) matching budget shares to CPA categories by aggregating COICOP categories into budget shares and calculating the weighted sum of CPA contributions to budget shares; (iii) matching CPA categories to WIOD using national supply tables that link CPA inputs to each industry output; (iv) assigning the relative contribution of each sector in the country to the appropriate consumption good / budget share.
Challenges and limitations
There is a growing literature on modelling the distributive impact of environmental taxes. All models abstract from the full complexity of the real world. This chapter makes a number of modelling choices to keep the empirical illustration transparent and tractable, and they should be kept in mind when interpreting results:
Scope of GHG emissions data. The current approach approximates the emissions from energy use that can be traced to consumer spending, via fuel use recorded in household consumption data and via input-output data. This scope is consistent with the historical ECR data, which covers levies on emissions from energy use. Process-related CO2 emissions, and emissions of other GHG, including methane, account for substantial shares of overall emissions, notably in food production. These are currently not accounted for. But have been added in recent ECR data and could be included in distributional analysis if and when granular emissions data become available.
Data quality and consistency. When combining data from various sources such as the OECD Effective Carbon Rates, Household Budget Survey, and International Energy Agency fuel price data, inconsistencies in data quality and classification can arise. These discrepancies can affect the accuracy of the estimations of carbon price burdens at the household level. Ensuring data compatibility and rectifying any mismatches is crucial, yet challenging, given the varied nature of these datasets. This limitation highlights the importance of continuous efforts to improve data quality and harmonisation in environmental economic research.
Need for accurate translation between data classification systems. The integration of diverse datasets necessitates precise alignment of classifications and terminologies used across sources. Misalignment can lead to errors in estimating carbon price burdens at the household level. Meticulous attention to detail and careful translation processes are vital to ensure the integrity and reliability of our findings.
The reference period of the simulations is relevant, as household circumstances, consumption patterns and prices change over time, as do preferences, including for consumption. Both the Input/Output data and household budget surveys refer to 2015, which represents an intermediate year during the 2012‑21 period. It therefore relates to prices and consumption prior to the recent cost-of-living crisis, and sidesteps the COVID-related distortions of consumption and production patterns that affects the most recent available household consumption data.
Impact of traded inputs and final goods. Multi-region input-output models allow accounting for the differential carbon prices across countries, and their potential impact on domestic consumer prices in global value chains. The companion paper illustrates this approach in the context of a single‑country study (Immervoll et al., 2023[13]). By contrast, calculations in this chapter currently assume that price changes are the same for domestically produced inputs and consumer products, and for imported ones. Following a hike in carbon prices, consumer prices will in practice tend to rise by less than the full increase in the short term when carbon prices in source countries are lower, and this is the rationale for balancing provisions such as carbon border carbon adjustment schemes. But consumer prices can also rise by more than the domestic carbon price increase, if carbon charges in source countries increase faster than they do domestically. Related OECD work is ongoing, using multi-region input-output data from the OECD Inter-country Input-Output (ICIO) database (Smith et al., forthcoming[132]). Building on this work, future extensions can analyse distributional effects accounting for the impact of differential carbon pricing across countries.
Beyond their immediate effect on consumption expenditures, carbon taxes and other climate‑change mitigation measures also alter the incomes of the owners of the different factors of production, including natural resources, “brown industry” equity and labour (Rausch, Metcalf and Reilly, 2011[30]; Metcalf, 2021[143]). Relatedly, changing input prices and consumer demand trigger labour-market adjustments through a reallocation of jobs from high-carbon to low-carbon industries and activities and employment effects can be a specific focus in public debates. The medium-term gains and losses from labour-market adjustments can be difficult to quantify and are not reflected in the approach presented here but may be sizeable for some groups (see Chapters 2 and 3 in this publication).
Beyond their effect on consumption expenditures, carbon pricing and other climate‑change mitigation measures also alter the incomes of the owners of the different factors of production (see Box 5.1). These are not accounted for in the present analysis.
Annex 5.B. Effective Carbon Rates methodology and further considerations
Sectors, users and fuels
This section provides further details on sectoral, user and fuel breakdown in the database.
The Effective Carbon Rates database covers CO2 emissions from energy use from six sectors that together span all energy uses and also covers other GHG emissions (i.e. emissions from methane (CH4), nitrous oxide (N2O), F-gases39 and process CO2 emissions) excluding Land use change and Forestry (LUCF)40 (Annex Table 5.B.1). Fuels are grouped into nine categories (Annex Table 5.B.2), effective carbon rates and emissions by fuel type are shown in Annex Figure 5.B.1.
Annex Figure 5.B.1. Effective carbon rates and share of CO2 emissions
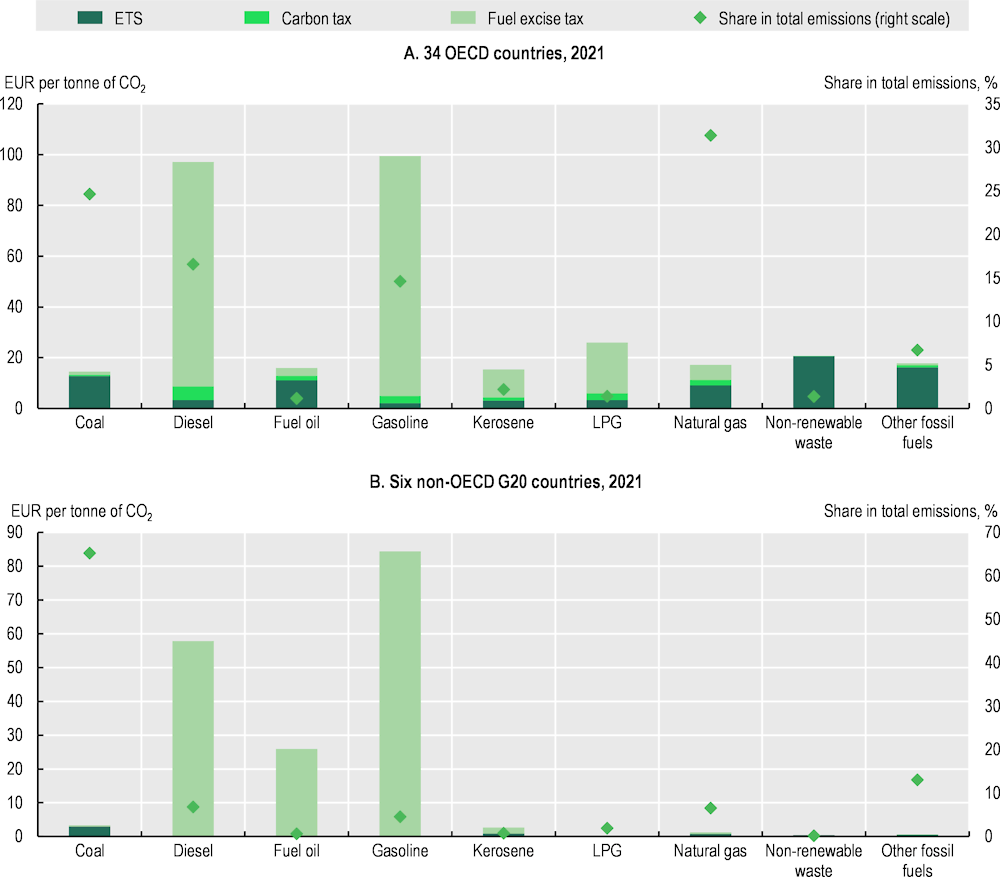
Note: Effective carbon rate averages are emissions weighted. ETS: Emission Trading Systems. LPG: Liquefied Petroleum Gas.
Source: Effective Carbon Rates database.
Annex Table 5.B.1. ECR sectors and users
Sector |
Definition |
Energy users |
---|---|---|
Road transport |
Fossil fuel CO2 emissions from all primary energy used in road transport. |
Road |
Electricity |
Fossil fuel CO2 emissions from primary energy used to generate electricity (excl. auto-producer electricity plants which are assigned to industry), including for electricity exports. Electricity imports are excluded. |
Main activity producer electricity plants |
Industry |
Fossil fuel CO2 emissions from primary energy used in industrial facilities (incl. district heating and auto-producer electricity plants). |
Adjusted losses in energy distribution, transmission and transport; Adjusted energy industry own use; Adjusted transformation processes; Auto-generation of electricity; Chemical and petrochemical; Construction; Food and tobacco; Industry not elsewhere specified; Iron and steel; Machinery; Mining and quarrying; Non-ferrous metals; Non-metallic minerals; Paper, pulp and print; Sold heat; Textile and leather; Transport equipment; Wood and wood products |
Buildings(*) |
Fossil fuel CO2 emissions from primary energy used by households, commercial and public services for activities other than electricity generation and transport. |
Commercial and public services; Final consumption not elsewhere specified; Residential |
Off-road transport |
Fossil fuel CO2 emissions from all primary energy used in off-road transport (incl. pipelines, rail transport, aviation and maritime transport). Fuels used in international aviation and maritime transport are not included. |
Domestic aviation; Domestic navigation; Pipeline transport; Rail; Transport not elsewhere specified |
Agriculture & fisheries |
Fossil fuel CO2 emissions from primary energy used in agriculture, fisheries and forestry for activities other than electricity generation and transport. |
Agriculture; Fishing |
Other GHG (excl. LUCF) |
All other GHG emissions include methane, nitrous oxide from agriculture; fugitive emissions from oil, gas and coal mining activities; waste; non-fuel combustion CO2 emissions from industrial processes (mainly cement production), N20 and CH4 emissions from industrial processes and F-gas emissions. Excludes LUCF emissions. Excludes CO2 emissions from fuel combustion which are already reported in the agriculture & fisheries sector. |
n.a. |
Note: Estimates of primary energy use are based on the territoriality principle, and include energy sold in the territory of a country but potentially used elsewhere (e.g. because of fuel tourism in road transport). Own classification based on information on energy flows contained in the IEA’s extended world energy balances (IEA, 2020[64]) and “other GHG” reported in the Climate Watch dataset (2024[20]). GHG: Greenhouse Gases. ECR: Effective Carbon Rates. LUCF: Land Use Change and Forestry.
(*) In Effective Carbon Rates editions prior to the 2023 edition (OECD, 2023[8]), this sector was referred to as “Residential and Commercial”.
Source: OECD (2016[15]), Effective Carbon Rates: Pricing CO2 through Taxes and Emissions Trading Systems, https://doi.org/10.1787/9789264260115-en and OECD (2022[12]), Pricing Greenhouse Gas Emissions: Turning Climate Targets into Climate Action, https://doi.org/10.1787/e9778969-en.
Annex Table 5.B.2. Fuel category breakdown
Energy type |
Fuel category |
Energy Products |
---|---|---|
Fossil fuels |
Coal and other solid fossil fuels |
Anthracite; Bitumen; Bituminous coal; Brown coal briquettes; Oven coke; Coking coal; Gas coke; Lignite; Oil shale; Patent fuel; Peat; Peat products; Petroleum coke; Sub-bituminous coal |
Fuel oil |
Fuel oil |
|
Diesel |
Gas/diesel oil excluding biofuels |
|
Kerosene |
Jet kerosene; Other kerosene |
|
Gasoline |
Aviation gasoline; Jet gasoline; Motor gasoline |
|
LPG |
Liquefied Petroleum Gas |
|
Natural gas |
Natural gas |
|
Other fossil fuels and non-renewable waste |
Additives; Blast furnace gas; Coal tar; Coke oven gas; Converter gas; Crude oil; Ethane; Gas works gas; Lubricants; Naphtha; Natural gas liquids; Other hydrocarbons; Other oil products; Paraffin waxes; Refinery feedstocks; Refinery gas; White and industrial spirit; Industrial waste; Non-renewable municipal waste |
|
Biofuels |
Biofuels |
Bio jet kerosene; Biodiesels; Biogases; Biogasoline; Charcoal; Municipal waste (renewable); non-specified primary biofuels and waste; Other liquid biofuels; Primary solid biofuels |
Note: Energy products are defined as in IEA (2020[64]). Emissions from the combustion of biofuels are not included in this analysis (see discussion in OECD (2023[8])).
Source: OECD (2022[12]), Pricing Greenhouse Gas Emissions: Turning Climate Targets into Climate Action, https://doi.org/10.1787/e9778969-en.
Carbon taxes, fuel excise taxes and ETSs
This subsection provides further details on estimation of rates and prices for taxes and emissions trading as well as coverage – based on Annex A of OECD (2016[15]). Indeed, data on ETS permit prices and coverage is originally gathered for the Effective Carbon Rates (ECR) database. The Effective Carbon Rates database then builds on the Taxing Energy Use (TEU) database, which gathers fuel excise tax and carbon tax data.
Carbon tax rates
Carbon taxes may be set following two approaches: a fuel-based approach, in which case the tax is explicitly linked to fuels, or a direct emissions approach, in which case the tax is directly levied on GHG emissions.
If established following a fuel-based approach, carbon tax rates are usually set for each fuel on the basis of their CO2 content. The carbon content of a fuel can be calculated as follows: common commercial units of fuels (e.g. kilogram for solid fuels, litre for liquid fuels, cubic metre for gaseous fuels) can be converted into energy units (e.g. GJ or MWh) using calorific factors from the IEA World Energy Statistics and Balances (IEA, 2024[144]). Energy units can then be converted into tonnes of CO2 using IPCC emissions conversion factors (IPCC, 2006[145]), volume 2).
In case there is a fixed rate per unit of CO2, the result is a uniform carbon tax, a desirable feature from a cost-effectiveness point of view. However, some carbon taxes may specify different rates for different fuels or users even in carbon terms. For administrative purposes, for each fuel, the tax can be translated into tax rates per litre, kilogram, cubic metre or gigajoule of energy. For instance, Annex Table 5.B.3 illustrates what a carbon tax rate of EUR 30/tCO2 would translate into for different energy categories. These taxes can only apply to CO2 emissions from energy use, but are relatively straightforward to administer.
Annex Table 5.B.3. From tax rates per tonne of CO2 to rates per common commercial unit of fuel
Energy category |
Rate per tonne of CO2 |
Equivalent rate per commercial unit of fuel |
---|---|---|
Coal and other solid fossil fuels |
EUR 30/tCO2 |
6.24 eurocent per kilogramme |
Fuel oil |
EUR 30/tCO2 |
8.94 eurocent per litre |
Diesel |
EUR 30/tCO2 |
7.99 eurocent per litre |
Kerosene |
EUR 30/tCO2 |
7.58 eurocent per litre |
Gasoline |
EUR 30/tCO2 |
6.86 eurocent per litre |
LPG |
EUR 30/tCO2 |
4.75 eurocent per litre |
Natural gas |
EUR 30/tCO2 |
5.13 eurocent per cubic metre |
Note: OECD calculations based on IEA (2024[144]), World Energy Statistics and Balances. The values shown are based on average carbon content of these energy categories across the 44 countries covered in the 2018 Effective Carbon Rates database. Actual carbon emissions associated with combusting the respective fuel may vary depending on local fuel characteristics. LPG: Liquefied Petroleum Gas.
Source: Table 3.1 of OECD (2019[146]), Taxing Energy Use 2019: Using Taxes for Climate Action, https://doi.org/10.1787/058ca239-en.
If established following a direct emissions approach, the tax is levied on GHG emissions directly. These taxes can apply beyond CO2 emissions from energy use, e.g. also to the other GHG category (i.e. CH4, N2O, F-gases and CO2 from industrial processes, see Annex Table 5.B.1). However, these require monitoring, reporting and verification systems, which may provide challenges, including emissions measurement and administrative complexity.
Carbon tax rates are gathered by user and fuel as of 1 April of the year under consideration (or the latest available date before that if not available) – e.g. 1 April 2021 for ECR 2021.
Fuel excise tax rates
Fuel excise taxes are the most significant component of ECRs – still in 2021. These taxes are typically levied per physical unit (e.g. litres in the case of liquid fuels, kilograms in the case of solid fuels, cubic metres in the case of gaseous fuels), or per energy content (e.g. GJ or kWh), and not by reference to the carbon content of the fuel. However, using the reverse process to that described for carbon tax rates, fuel excise tax rates can be translated into effective tax rates on the carbon content of the fuel due to the proportional relationship between fuels and their carbon content (Annex Table 5.B.4). Hence, in terms of their behavioural impact they are similar to a fuel-based carbon tax, albeit less consistent from an environmental perspective in the approach to the rates applied (since rates are generally not designed to align with CO2 content).
Support measures for fossil fuel consumption that are delivered through the tax code, such as excise or carbon tax exemptions, rate reductions and refunds are included.
Fuel excise tax rates are gathered by user and fuel as of 1 April of the year under consideration (or the latest available date before that if not available) – e.g. 1 April 2021 for ECR 2021.
Annex Table 5.B.4. From tax rates per common commercial unit of fuel to tax rates per tonne of CO2
Fuel |
Rate per common commercial unit |
Equivalent rate in EUR per tonne of CO2 |
---|---|---|
Coal and other solid fossil fuels |
10 eurocent/kg |
48.1 |
Fuel oil |
10 eurocent/L |
33.6 |
Diesel |
10 eurocent/L |
37.5 |
Kerosene |
10 eurocent/L |
39.6 |
Gasoline |
10 eurocent/L |
43.7 |
LPG |
10 eurocent/L |
63.2 |
Natural gas |
10 eurocent/m3 |
58.5 |
Note: OECD calculations based on IEA (2024[144]), World Energy Statistics and Balances. The values shown are based on average carbon content of these energy categories across the 44 countries covered in the 2018 Effective Carbon Rates database. Actual carbon emissions associated with combusting the respective fuel may vary depending on local fuel characteristics. LPG: Liquefied Petroleum Gas.
Source: Adapted from Table 3.1 of OECD (2019[146]), Taxing Energy Use 2019: Using Taxes for Climate Action, https://doi.org/10.1787/058ca239-en.
Emissions trading systems permit prices
The Effective Carbon Rates 2023 report covers permit prices from emissions trading systems in the 72 countries of the database, encompassing supra-national, national and subnational jurisdictions. Emissions trading systems exist in 34 of the countries studied.
Average permit prices at auctions are calculated across a year if the data is available. An average is taken to smooth price fluctuations, where possible. For some emissions trading systems, price information is only available for part of the year, in which case an average across the available dates is calculated, or for a single auction or date, in which case this price is used. Due to data availability issues, secondary market prices rather than auction prices are used in the calculation for certain systems.
Permit prices are gathered for the year under consideration (e.g. over 2021 for ECR 2021).
Estimation of coverage of carbon pricing instruments
Tax rates are gathered by energy user (see Annex Table 5.B.1) and fuel (see Annex Table 5.B.2), which then directly translates into the base covered by these taxes.
ETS coverage is an estimate as it applies to emissions of a facility subject to an ETS and does not distinguish between fuels. For most systems, ETS coverage is estimated by reference to verified emissions data at facility level or at aggregated facility level (e.g. firm). Where this is not available, broader measures are used such as the share of sectoral emissions covered. These emissions covered by ETSs are then matched where possible at a user-level, else, at a sector-level.
Finally, carbon taxes are often entirely or partially alleviated if the energy user is subject to an ETS. This is reflected once the TEU database is merged with the ETS information to generate the Effective Carbon Rates.
Further considerations
The ECR database covers pricing instruments that apply to a base that is directly proportional to energy use or GHG emissions. It therefore excludes taxes and fees that are only partially correlated with energy use or GHG emissions. These include vehicle purchase taxes, registration or circulation taxes, and taxes that are directly levied on air pollution emissions (e.g. the Danish tax on SOX or the Swedish NOx fee). Production taxes on the extraction or exploitation of energy resources (e.g. severance taxes on oil extraction) are not within the scope of instruments covered either, as supply-side measures are not directly linked to domestic energy use or emissions.
The database covers specific taxes (i.e. taxes that apply per unit of good as opposed to ad-valorem taxes, which depend on the good’s price) and taxes that affect the relative price of carbon-intensive goods. In line with these two criteria, value added taxes (VAT) or sales taxes are not accounted for. Indeed, in principle VAT applies equally to a wide range of goods, so does not change the relative prices of products and services (i.e. it does not make carbon-intensive goods and services more expensive relative to cleaner alternatives). In practice, differential VAT treatment and concessionary rates may target certain forms of energy use, thereby changing their relative price (OECD, 2015[147]). However, quantifying the effects of differential VAT treatment is beyond the scope of the database. One reason is that such an exercise would require extensive price information, which is generally not available for all energy products. In addition, fully accounting for VAT would require information on the sellers and purchasers of energy because the way that VAT is designed means that no net VAT is charged on taxable supplies between VAT-registered businesses. Also, electricity excise taxes do not treat fossil fuels in a differential manner as compared to clean sources and are therefore not part of the ECR indicator.
The ECR database includes support measures for fossil fuel consumption that are delivered through the tax code, such as excise or carbon tax exemptions, rate reductions and refunds, which are pervasive in energy tax and carbon pricing systems. This is different from the Net ECR (nECR) database, which includes also fossil fuel subsidies that lower pre‑tax prices. The availability of preferential treatment varies substantially across countries, and even within a country such preferential treatment frequently changes over time. As a result, simply comparing statutory rates (also sometimes referred to as standard or advertised rates) across countries and time would be misleading. More precisely, certain energy users or GHG emitters frequently enjoy preferential treatment that effectively reduces prices on energy or emissions. Therefore, effective tax rates measured by the database are adjusted accordingly irrespective of whether countries report such policy measures as tax expenditures (OECD, 2022[12]).41
Interpreting Effective Carbon Rates
Comparisons across countries and sectors
Mitigation policy packages vary with many factors, including country circumstances, policy objectives and targeted sectors. Carbon pricing is a core mitigation policy in some countries, while others rely more on non-carbon price‑based instruments, e.g. regulation or technology support. This may be due to many factors, including administrative capacity, historical context, the technical and methodological challenges of pricing emissions, and political constraints. The approach to carbon pricing instruments itself also varies with these factors. For instance, fuel excise taxes are more common than carbon taxes and ETSs and in general were initially introduced to raise revenue (so that aligning them better with climate goals often requires reform). Carbon taxes may require less administrative capacity to implement than ETSs, as they are generally based on the carbon content of fuels. On the other hand, ETSs, while generally requiring sophisticated monitoring, reporting and verification mechanisms, can face fewer political barriers to implementation.
Effective carbon rates depend on the sectoral composition of a country’s emissions.
In particular, in 2021, the road transport sector faces the highest rates, while the majority of other GHG emissions aside CO2 from energy generation (CH4, N2O, F-gases and CO2 from process) face no carbon price. The difference of ECRs between sectors may have various explanations. High taxation rates in the road transport sector may also reflect the pricing of other externalities caused by road transport, such as air pollution, accidents, congestion and noise,42 or can reflect revenue raising objectives. Indeed, the different externalities present in each sector provide a clear economic rationale for effective carbon rates to vary by sector, even though the greenhouse gas externality is equal everywhere. On this point, it can be worth noting that transport externalities may also be priced using different instruments such as congestion charges or vehicle taxes. A country using those instruments could then have lower fuel excise tax rates in the road transport sector because it addresses externalities in that sector through different instruments. This would then lead to a lower ECR. Low carbon pricing of other GHG emissions may come from the challenges of measuring these emissions, making it a challenge to price them directly. Given political constraints facing carbon pricing and different abatement opportunities in different sectors, setting higher prices on larger emissions bases can be more challenging than for narrower emissions bases.
Sectoral shares can substantially vary across countries and this variation influences average ECRs at the country level. Countries with a high share of road transport sector emissions tend to have higher average ECRs.
On one end of the spectrum, diesel and gasoline, which are primarily used in the road transport sector, are subject to the highest fuel excise tax rates (translating to respectively EUR 70 and EUR 85 per tonne of CO2 on average in 2021), which also relates to their historically broad tax base used by countries to raise revenue. On the other end of the spectrum, coal and other solid fossil fuels, which are mostly used in the industry and electricity sectors face relatively low effective carbon tax rates (at an average of EUR 5.4 per tonne of CO2 in 2021). Fuels such as LPG and natural gas, which are used in the buildings sector, stand in the middle, with average ECRs at about EUR 8/tCO2 and EUR 10.6/tCO2 respectively. These fuels often face reduced tax rates or exemptions, particularly when applying in the residential sector.
Effective Marginal Carbon Rates versus Effective Average Carbon Rates
Most emissions trading systems distribute part or all of emissions allowances for free, at least during the inception phase. Auctioning or fixed price selling of allowances is generally gradually introduced into systems as they become more mature. In 2021, the share of free allocation of allowances varies widely across systems, ranging from a 100% in Japanese subnational ETSs (Tokyo and Saitama), for instance, to almost 0% in RGGI and the Massachusetts Limits on Emissions from Electricity Generators (310 CMR 7.74).
While free allocation of allowances does not affect the marginal price signal, it does affect the average price signal, which in turn affect economic rents and thus can influence investment decisions. Free allocations do not change the marginal price signal faced by firms because even if entities receive free allocations, reducing their emissions allows them to sell extra permits while emitting more requires them to buy additional permits. And even if they emit exactly what they have been allocated, they face an opportunity cost as they forgo the income they would have gotten from reducing their emissions and selling those extra permits. However, the average43 price paid by entities for permits does depend on the level of free allocation received. Flues and Van Dender (2017[148]) show that permit allocation rules affect economic rents and that in practice they tend to do so in a way that favours more carbon-intensive technologies.
The wedge created by free allocation of allowances between the marginal and average carbon prices may be captured by using the share of free allocations incurred by an installation, subsector, sector or country but it may also be captured by the Effective Average Carbon Rates (EACR) and Effective Marginal Carbon Rates (EMCR) indicators. The EMCR is the main indicator used in this report: the ECR summarises the marginal carbon rates faced by subsectors, sectors or countries. The EACR, on the other hand, summarises the average carbon rates faced by subsectors.
Free allocation can result in windfall profits in certain sectors. The mechanism is that even when receiving free allocation of allowances, firms still face opportunity costs, i.e. the marginal cost of carbon. If they can adjust pricing and then pass-through this cost to consumers, the free allocation becomes a rent. In practice, this depends on many factors, including the allocation regime, the competition in the sector, demand and supply elasticities, carbon intensity of production, and international trade exposure of the sector (Quirion, 2007[149]; Hobbie, Schmidt and Möst, 2019[150]). These are all factors that impact pass-through of carbon costs to consumer prices.
Hence, the underlying assumption of using EMCRs (i.e. ECRs) to infer prices on consumers is that there is full marginal cost pass-through regardless of the permit allocation method, and free allocation is a rent for all firms. Using EACRs would imply the assumption that there are no windfall profits.
Notes
← 1. The scale of economic damages from climate change and, hence, from the absence of mitigation efforts, remains uncertain (Auffhammer, 2018[134]; Howard and Sterner, 2022[135]). But potential welfare losses are very large – for example, Bilal and Känzig (2024[136]) find that the macroeconomic damages from climate change could be six times larger than previously estimated and estimate that world GDP per capita would be 37% higher today had no warming occurred since 1960. Distributional implications are also large, since higher-income groups are more likely to cope with, or to shield themselves from, the effects of rising temperatures (Davis and Gertler, 2015[167]; Calvin et al., 2023[60]; Emmerling, Andreoni and Tavoni, 2024[155]). For instance, for Latin America and the Caribbean, a recent IBRD study suggests that 78 million poor people or more live in areas that are highly exposed to climate‑related shocks (Inter-American Development Bank, 2023[156]) – see also Chapter 2.
← 2. These concerns are not new, however. For instance, during the 2008 US presidential campaign, both Hillary Clinton and John McCain supported carbon cap-and-trade legislation but nonetheless called for temporary suspension of the federal gasoline tax (Bosman, 2008[151]).
← 3. For instance, revenues from fuel excise taxes, carbon taxes and permit prices in 2018 averaged around 1.3% of GDP across OECD and G20 countries. Using disaggregated data by sector and fuel type, and accounting for emission reductions in response to higher prices, D’Arcangelo et al. (2022[11]) find that a moderate carbon price floor of EUR 60/tCO2 would roughly double revenues to 2.5% of GDP, with much larger increases in countries with high emission intensities but low current carbon prices.
← 4. Carbon taxes and emissions trading systems (explicit carbon pricing) but also implicit carbon pricing through fuel excise taxes.
← 5. According to the Intergovernmental Panel on Climate Change (IPCC), maintaining the current emission levels until 2030 will irremediably compromise the chances to keep global warming below 1.5 degrees above the average for the pre‑industrial era (Calvin et al., 2023[60]).
← 6. Price‑based measures can co‑exist with other mitigation approaches, for instance, by combining hard emissions limits with carbon pricing in cap-and-trade (emissions-trading) schemes. While there are downsides to cap-and-trade schemes, the explicit ceiling on emissions side‑steps uncertainties surrounding emissions reductions that can be achieved with price increases alone, while a market-based price mechanism facilitates cost-effectiveness (by allowing firms with higher abatement costs to purchase from those with lower abatement costs).
← 7. The social cost of carbon is the economic cost caused by an additional tonne of CO2 emissions or its equivalent. It rests on the concept of internalising externalities, considering both inter- and intra-generation equity (Nordhaus, 1991[56]).
← 8. The World Bank estimates that 23% of global emissions were covered by a carbon price in early 2023, up from just 5% in 2010 (World Bank, 2023[169]).
← 9. All G20 countries excluding Saudi Arabia.
← 10. Calculating the social cost of carbon is fraught with difficulties, including estimating GDP losses resulting from global temperature increases, weighing catastrophic risks whose probability and magnitude are inherently uncertain, and choosing a suitable discount rate for possibly catastrophic climate‑related costs (Howard and Sterner, 2017[152]).
← 11. These numbers are for 34 OECD countries (Australia, Austria, Belgium, Canada, Chile, Czechia, Denmark, Estonia, Finland, France, Germany, Greece, Hungary, Iceland, Ireland, Israel, Italy, Japan, Korea, Luxembourg, Mexico, the Netherlands, Norway, New Zealand, Poland, Portugal, the Slovak Republic, Slovenia, Spain, Sweden, Switzerland, Türkiye, the United Kingdom and the United States), and for 6 non-OECD G20 countries (Argentina, Brazil, China, India, Indonesia), in 2021.
← 12. In OECD and G20 countries, coal is predominantly used in the electricity and industry sectors, where carbon prices stem mostly from emissions trading systems. The average ECR on coal is EUR 11 per tonne of CO2 in OECD countries and 3.4 in non-OECD G20 countries. Diesel and gasoline are principally used in the road transport sector (gasoline almost entirely so), with carbon prices mostly due to fuel excise taxes. ECR rates are much higher, at respectively EUR 84 and 89 per tonne of CO2 in OECD countries and respectively EUR 58 and 84 in non-OECD G20 countries (High tax rates in this sector may also reflect revenue raising objectives or to a certain extent, the pricing of other externalities caused by road transport, such as air pollution, accidents, congestion and noise). Natural gas is principally used in the electricity, industry and buildings sectors. It is covered by fuel excise taxes and emissions trading systems, and rates are similar to coal in OECD countries. Its use in the buildings sector (commercial and residential heating) frequently presents reduced fuel excise tax rates, especially when applying to household consumption. Average ECRs are EUR 12.6 per tonne of CO2 in OECD countries and EUR 1.3 per tonne in non-OECD G20 countries.
← 13. i.e. comparative across countries for comprehensive carbon pricing reforms, using the same methodology.
← 14. See also Rausch, Metcalf and Reilly (2011[30]). Some studies suggest that accounting for firms’ behavioural response can reduce any regressivity of carbon pricing and other environmental taxes. This is primarily through any lower returns to equity in some sectors, which predominantly accrue to higher-income households (Metcalf, 2023[153]; Rausch and Schwarz, 2016[154]), though these households also stand to gain from any increasing returns to “green” capital.
← 15. Household emissions sum up to less than the full carbon footprint of final demand a country, with government consumption and investment being the remaining components. These could be attributed to households in principle, including by attributing emissions to household savings that finance investment. This is not attempted here, but see, e.g. Starr et al. (2023[160]) for the United States.
← 16. IEA reports the following 2021 emission factors for electricity, in kgCO2e per kWh. France: 0.05; Germany: 0.34; Mexico: 0.43; Poland: 0.76; Türkiye: 0.38.
← 17. While drawing down wealth can be one factor behind very high spending shares among low-income groups, under-reporting of income has also been shown to play a role in household expenditure surveys, which are not designed to prioritise income measurement (Brewer, Etheridge and O’Dea, 2017[162]).
← 18. For instance, in 2022, 9.3% of the EU population declared that they were not able to keep their home adequately warm (Eurostat, 2023[168]). This means that poor households do not only spend a relatively high share of their budget on energy, but that they still face considerable budget constraints as their energy needs may be even higher than the effective expenditure levels.
← 19. A progressive pattern of energy spending is increasingly uncommon among high-income countries, but it has been documented for some OECD members with lower GDP levels (Immervoll et al., 2023[13]). Spending can be substantially more top-heavy in emerging economies and lower-middle‑income countries outside the OECD area. Patterns vary between middle‑income countries, with studies pointing to climatic factors, inequality in housing and access to utility grids, as well as sizable energy subsidies. In addition, low-income households may source substantial shares of energy outside the market, e.g. in the form of peat or firewood. See Steckel et al. (2021[93]) and, e.g. Pachauri (2004[157]) for India, Irfany and Klasen (2017[158]) for Indonesia.
← 20. See endnote 17 for the different emission factors for electricity (as reported by the IEA).
← 21. Non-fuel related expenditures may be broken down into categories according to the Classification of Individual Consumption According to Purpose (COICOP). These include food, beverages, tobacco, clothing and footwear, housing, gas and water, furnishings, household equipment, health, transport, information and communication, recreation, sport, culture, education services, restaurants and accommodation services, insurance and financial services, personal care and social protection, individual consumption expenditure of non-profit institutions serving households and of general government. Households’ indirect carbon emissions are driven by both the carbon intensity of these consumption categories but also by the expenditure devoted to these categories. In most countries, and across income deciles, the housing, gas and water category represented the main source of emissions across income deciles. Food and transport are also generally important. Services such as education and recreation have low energy intensities and generally represent the lowest household indirect emissions. The share of emissions from each category is reasonably consistent across the income distribution.
← 22. See Figure 5.4 and Annex Figure 5.B.1.
← 23. These estimates are in line with those found in national studies for the countries included, e.g. for France (André et al., forthcoming[163]). For the United States, Starr et al. (2023[161]) report emission shares of 4% and 24%, respectively, for the bottom and top deciles, with large increases of emissions at the very top, e.g. 6% of the total for the top 1% and 2.3% of the total for the top 0.1%.
← 24. For instance, in an analysis of emission inequalities in France, Pottier et al. (2021[159]) note that “the size of the dwelling increases with income and distance from urban centres […] the first factor to account for variability of emissions is the heating system: this has little to do with income but more to do with settlement patterns, which constrain access to the various energy carriers”.
← 25. See Lévay, Goedemé and Verbist (2023[165]) for references to (the small number of) multi-variate studies. Sager (2019[131]) undertakes such an exercise for the United States and also calculates the contribution of different population characteristics / groups to the evolution of carbon emissions over time.
← 26. These results are by and large explained by the fact that the carbon price increases in this period were much smaller than what would be needed to successfully transition to a net-zero emission economy (see e.g. the Introduction). It is easy to conjecture that much larger carbon price hikes would have a more significant impact even on average household burdens.
← 27. This holds in particular in countries where the majority of the labour market is formal.
← 28. See the Introduction of this chapter. In addition, carbon pricing instruments are often themselves subject to value added tax (VAT) at full or reduced rates, and this has both budgetary and distributional consequences. VAT is not currently accounted for in the results reported here. Incorporating such interaction effects with other tax instruments is a topic for future extensions.
← 29. Across OECD countries, 2019 expenditures on income support for the working-age population and on non-health social services average, respectively, 3.6% and 2.3% of GDP. 8 countries spent less than 2% of GDP on working-age income support, and 18 spent less than 2% on non-health social services. See OECD Social Expenditure Database, www.oecd.org/social/expenditure.htm.
← 30. The available information in the analysis conducted in this chapter – i.e. income and other information in household budget surveys – is not sufficiently granular for simulating more targeted social benefits or, e.g. labour-tax reductions. The required matching with income data is beyond the scope of this comparative study. See, however, Immervoll et al. (2023[13]) for an example of such an approach in a country-specific context.
← 31. The lump-sum transfers in these cases are therefore the same as the average carbon price burden. Results account for carbon price burdens at the household level, and therefore capture the variability of gains and losses across and within income groups, which remain hidden when assessing average burdens by decile.
← 32. Lump-sum redistribution, however, also comes with many drawbacks, which are further discussed below. These include the importance of targeting transfers due to fiscal, equity, efficiency, and effectiveness reasons.
← 33. Across populations as a whole, recycling all the revenue from 2012‑21 carbon pricing reforms mostly creates more gainers than losers, with a smaller share of reform losers than winners in Mexico (31%), followed by France (42%) and Germany (44%). In Poland, carbon price burdens were highly concentrated at the bottom of the income distribution, and a lump-sum transfer would offset the burdens of slightly less than half of the population, leaving 53% worse off.
← 34. The particular shape of the curves reflects the incidence of carbon price burdens shown earlier. They are also driven by inequalities within income groups. For instance, greater disparities of spending on fuel and other high-emission consumption items in the lower parts of the income distribution can translate into significant numbers of people with sizeable burdens, who may then be net losers even after a lump-sum transfer.
← 35. For instance, the recent OECD survey of the European Union assumes that 30% of carbon pricing revenues are returned to households (OECD, 2023[164]).
← 36. Behavioural responses to price changes in subsequent periods are not accounted for here. They would alter the patterns of gains and losses, with results depending on the specific price and budget elasticities across population groups. Past work suggests that behavioural adjustments are modest in the short term and evidence is mixed on their distributional profile (see Box 5.3). Moreover, on average, a rebalancing of household consumption towards lower-emitting goods reduces household burdens and carbon price revenues by the same proportion. In combination, this makes it unlikely that behavioural responses would substantively alter the shares of gains and losses reported here. The country-specific behavioural modelling reported in Immervoll et al. (2023[13]) supports such a conjecture.
← 37. Recent findings, however, highlight equity-efficiency trade‑offs when seeking to address horizontal equity concerns such as rural-urban gaps (Labrousse and Perdereau, 2024[74]).
← 38. Surveys suggest that one factor behind weak support for carbon pricing measures is limited public knowledge about their environmental effectiveness (which tends to be underestimated), and the likely burdens for households and their regressivity (which tends to be overestimated). See Dechezleprêtre et al. (2022[7]) for a summary of that evidence.
← 39. HFCs, PFCs, and SF6.
← 40. The abbreviation LUCF is used (as opposed to the term LULUCF, i.e. land use, land-use change, and forestry) to emphasise that the underlying GHG emissions data is sourced from the CAIT dataset (Climate Watch, 2024[20]), which does not rely on countries’ official inventories reported to the UNFCCC (OECD, 2022[12]).
← 41. This represents a different approach from the OECD’s Inventory of Fossil Fuel Support (OECD, 2021[166]). See Box 1.2 of OECD (2022[12]) for additional details on the difference in approaches.
← 42. While the former externality would not be present if a full switch to electric vehicles (EVs) took place, the other three externalities would remain.
← 43. i.e. overall price divided by the amount of emissions.