The previous chapters outlined the role of tradable sectors and of cities as sources and catalysts of productivity growth. This chapter considers two concrete aspects of global trade. The first aspect is the clustering of related traded activities within regions and the role of concentration and diversification in productivity growth. The second is the integration of regions in global trade relationships. Rather than exports themselves, “global value chains” measure the overall contribution of a region’s economy to products that are comprised of parts made in different countries and regions before being assembled into a final good.
Productivity and Jobs in a Globalised World
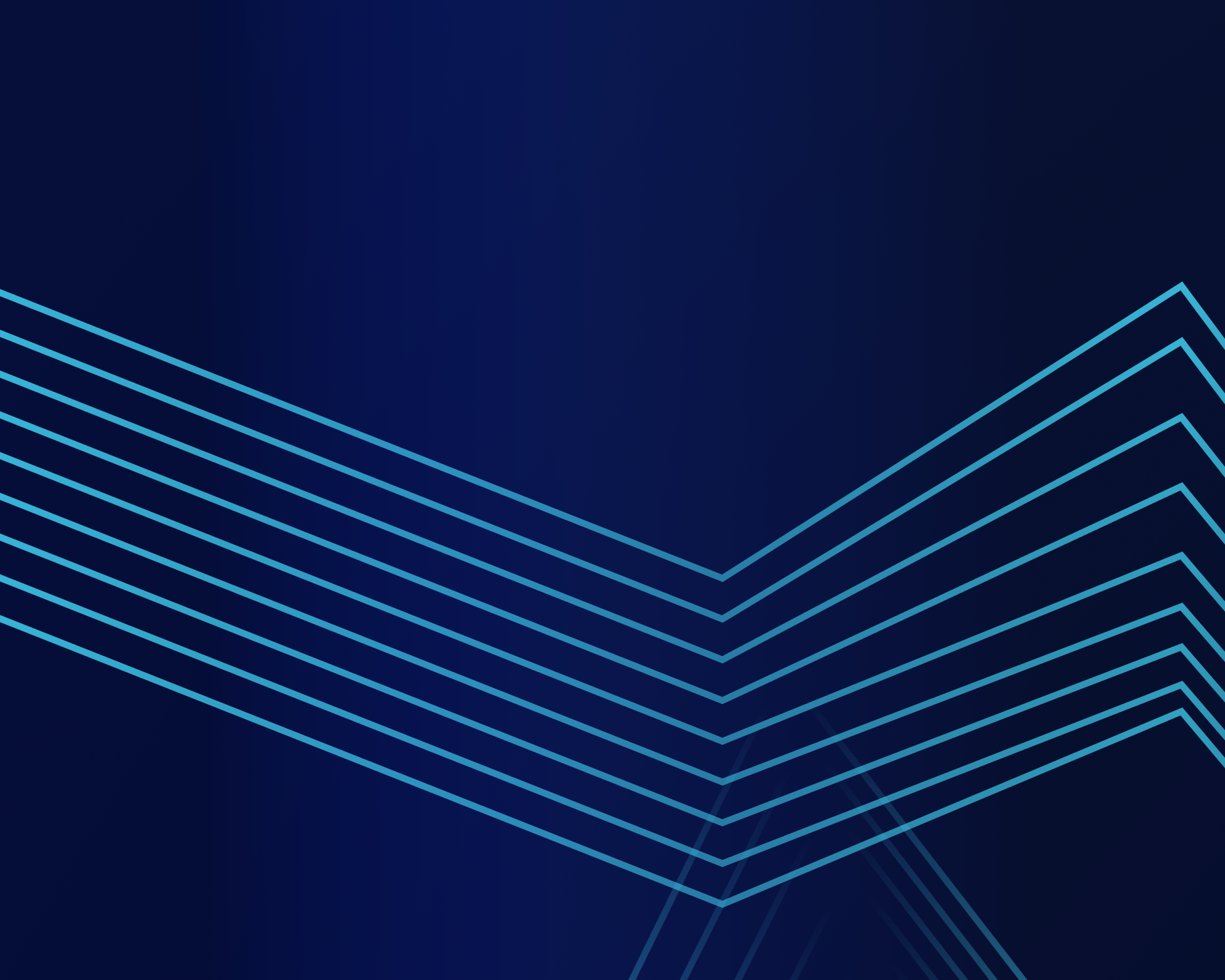
Chapter 3. Global trends and regional links: Jobs, clusters and global value chains
Abstract
Chapter synopsis
Clusters of economic activity are important sources of innovations and productivity growth. Yet, the importance of individual clusters for regional economies varies strongly. In some European regions, the largest cluster employs less than 5% of the workforce, whereas in others it employs more than 40% of the workforce. At the same time, there appears to be no statistical link between the size of the largest cluster in a region and the total share of regional economic activity that occurs within clusters. This indicates that greater specialisation in a few clusters does not lead to a greater overall importance of clusters and raises the question to what degree regions should try to specialise in just a few, potentially very large clusters, or diversify across many smaller clusters.
While highly specialised regions tend to have higher levels of per capita gross domestic product (GDP) than regions whose economy is more evenly distributed across many clusters, their per capita GDP growth rate is lower than in more diversified regions. This implies that specialisation is increasing when regions become richer, but this effect can limit their future growth potential.
Policy makers should therefore consider counteracting excessive specialisation by encouraging diversification of the regional economy. In this context, optimal degrees of diversification differ from region to region. Dense urban economies can generate greater economic diversity than economies in sparsely-populated, rural areas. Not all forms of diversification are likely to have the same positive effects. Evidence suggests that diversification into so-called “related varieties” (economic activities that are characterised by similar, but not identical processes) is most beneficial. Through such diversification, innovations can spread from one cluster to another without restricting opportunities for future growth through excessive concentration in a single economic activity.
The integration of regions in global production process as part of a “global value chain” (GVC) can also create benefits in terms of innovation and productivity. Global value chains is a term used to describe supply chains that divide production processes into different stages distributed across several countries. After growing rapidly in importance throughout the 1990s, GVC integration in most regions in Europe remained stable from 2000 to 2010 except for an intense but brief dip in the aftermath of the global financial crisis in 2008. As of 2010, an average of approximately 18% of all value-added in European regions was created within GVCs.
Although GVC integration coincides with higher GDP levels, the effect is not uniform. Regions with either particularly low or particularly high productivity levels have GVC participation rates that are below average. In low productivity regions, this is due to a weak tradable sector. In contrast, the low share of GVC integration in high productivity regions is due to a strong service sector, for which trade might not always be fully captured in the underlying data. Highly productive regions often include large cities, whose economy is dominated by services that are less tradable than manufactured goods.
Moreover, not all types of GVC integration yield the same benefits. The greater the amount of value-added produced in a region, the higher the economic benefits. Labour intensive low-skilled manufacturing that creates little value-added can bring important jobs to regions with high unemployment rates, but it offers little potential to diversify the economy. Furthermore, such production will only stay in a region while wage levels remain low. Instead of focusing on these activities, regions should try to attract production activities at the beginning and at the end of a GVC that are likely to add more value, such as product development, marketing and after-sales services.
Regional clusters
Cluster policies have long been policy instruments in many OECD countries. They have been identified as a well-established mainstay of regional development policy in the OECD (2007[1]) and their on-going importance as a policy instrument has been confirmed in the OECD (2016[2]). In particular, cluster policies are frequently used to promote economic development in lagging regions.
The rationale behind cluster policies is based on agglomeration benefits and economies of scale that are created by clusters. These mechanisms make it beneficial for firms to be located within very close proximity of one another. However, clusters do not always emerge naturally because of friction and co-ordination failures that prevent firms from coordinating with each other about where to locate. In such situations, public intervention can provide the impetus for clusters to emerge and to reap the associated benefits.
Furthermore, cluster policies can also been beneficial if they contribute to a process of learning and self-discovery at the regional level. By bringing together different regional stakeholders, cluster policies and the processes related to their design and implementation can help regional governments to develop a better understanding of the strengths and weaknesses of a region. Through this mechanism, cluster policies can also help to improve other economic policies at the regional level.
Despite these potential advantages, there are risks associated with cluster policies. They can lead to insufficient diversification, lock-in into unsuccessful investment strategies and over-reliance on key firms (OECD, 2007[1]). Furthermore, cluster policies have often been driven by wishful thinking in the sense that policy makers try to promote clusters for which no realistic basis exists in the region. Lastly, cluster policies can also lead to an overreliance on a few or even a single cluster. As discussed in the following, this can stifle innovation and increase susceptibility to economic shocks.
This section does not intend to assess cluster policy in all its dimensions. Instead, it focuses on two angles. First, it analyses how regional specialisation in a few clusters - compared to broad diversification - is related to regional GDP growth and economic convergence. Second, it analyses the growth performance of regions depending on how their clusters are distributed within the tradable and non-tradable sector. Subsequently, it connects the findings to existing literature and develops policy implications.
Cluster data
Traded clusters are defined as groups of firms that are connected through one or more linkages, such as co-location, use of similar skills, and direct input-output relationships. Based on these linkages, the European Cluster Observatory (Ketels and Protsiv, 2016[3]) defines 51 clusters in traded sectors with all remaining firms (and their employment) falling into non‑traded “local” clusters (for simplicity this chapter refers to traded clusters as “clusters”). The clusters follow the definition of Delgado, Porter and Stern (2016[4]), but have been adapted to account for differences in the economic structure between Europe and the United States. The focus on the tradable sector is explained by the fact that economic activity in the non-tradable sector is focused on the local market. If goods or services are not traded across regional borders, a region produces by definition the amounts that are demanded within the region. Therefore, the potential for regions to specialise in the non-tradable sector is limited.
Box 3.1. A method for defining traded clusters
The cluster definition developed by Delgado, Porter and Stern (2016[4]) uses data for the United States on co-location patterns of employment and the number of establishments in detailed (3‑digit) industry groups, together with national-level information from input-output matrices that calculate flows of products and services across industries and similarity in occupational structure using employment shares in 3‑digit occupational categories. These linkages are used to group industries into a set of defined clusters using a hierarchical cluster function for continuous data. The resulting clusters are regionally comparable as the same industries belong to the same cluster in all regions. In that sense the definition is not region‑specific, but allows for interregional comparisons.
Source: Delgado, Porter and Stern (2016[4]).
The cluster data of the European Cluster Observatory has several advantages for analysing both the effect of specialisation and the role of the tradable sector on regional economic growth and productivity. Compared to more common data that divides economic activities by sectors, cluster data provides a better representation of the connections between businesses. For example, cluster data would group a service activity and a manufacturing activity within the same cluster if they contribute to the production of the same product. In contrast, such activities would show up in different sectors in sectoral data where the economic links between the two activities are invisible.
While the importance of clusters can be measured in different ways, this report focuses on full-time equivalent employment as the key measure for the size of a cluster. Due to its clarity, this measure provides the best data quality. Other measures, such as gross value-added, depend on accounting methods and are potentially less reliable.
The prevalence of clusters varies significantly across regions
Traded clusters’ contribution to employment varies widely across regions. On average, 46% of the total full-time equivalent employment of a region takes place within clusters, with 25% as the lowest share in a region and 86% as the highest share. Figure 3.1 shows the distribution of employment in tradable clusters across all regions. In most regions, between 40% and 60% of the working population works in clusters, but there are several regions where the share is significantly higher or lower.
Figure 3.1. Employment in traded clusters
Across all regions, 8.5%, on average, of full-time equivalent employment is provided by the largest cluster. The importance of individual clusters varies substantially. In most regions, the largest cluster in terms of jobs provides between 5% and 15% of all full time employment. But Figure 3.2 shows that in some regions the most dominant cluster accounts for a much higher percentage of employment. In 12 regions, the largest cluster provides more than 20%of all jobs. In eight out of those 12 regions, Transport and Logistics is the dominant cluster, providing up to 40% of all employment.
Figure 3.2. Share of employment provided by largest cluster
The degree of specialisation in a single cluster is unrelated to the overall share of jobs provided by clusters. However, some regions stand out because of particularly strong specialisation in single clusters. In particular, regions in Greece show an exceptional reliance on their largest cluster. On average, a Greek region relies on the largest cluster for 17% f all employment, approximately twice the average of all European regions.
More generally, low-growth regions differ from other regions due to a low prevalence of economic activity in traded clusters in combination with a strong reliance on their largest cluster.1 As can be seen in Figure 3.3, low-growth regions tend, on average, to have a much smaller share of jobs in clusters. Given that clusters are only defined for traded activities, this confirms the finding that low-growth regions tend to have underdeveloped tradable sectors.
Figure 3.3. Specialisation and employment in regional clusters

Note: The vertical and horizontal lines indicate the median share of jobs in traded clusters and the median share of FTE employment in the largest cluster, respectively. Diamonds indicate European NUTS2 regions, squares indicate low‑growth and low‑income regions as defined by the EU Lagging Regions Initiative (European Commission, 2017[5]).
Source: Calculations based on Ketels and Protsiv (2016[3]) with data provided by the authors.
Even though low-growth regions have comparatively few jobs in clusters, the relative size of their largest cluster compared to all other clusters is significantly bigger than average. The largest cluster employs approximately 2 percentage points more people than the average largest cluster in all NUTS2 regions. As a result, most lagging regions fall into the upper left quadrant of Figure 3.3.
Highly specialised regions – high per capita GDP but low growth rates
The question of whether regions benefit from being specialised or diversified has been discussed in a seminal article by Glaeser et al. (1992[6]). Studying different cities in the United States, the authors find that cities with a diversified industrial structure have higher employment growth rates than cities with a high degree of industrial specialisation.
The data on regional clusters can be used to test this hypothesis at the regional level. In order to measure the degree to which a region is specialised into selected clusters, a Herfindahl-type index can be computed. The Herfindahl index is a well-established measure that can be used to describe concentration or specialisation in a variety of settings (see Box 3.2). It takes a value of close to 0 if all clusters contribute equally to employment and a value of 1 if all employment is concentrated in a single cluster. In other words, a higher value of the Herfindahl index is associated with greater specialisation.
Box 3.2. The Herfindahl index
The Herfindahl index is a commonly used measure of concentration or specialisation. It was developed by Herfindahl (1950[7]) to measure concentration and market power of individual firms in an industry and has since been used as a measure of concentration in a variety of other settings. It is computed according to the following formula:
When used to calculate the degree of regional specialisation into clusters represents the share of employment within cluster i among total employment and N is the total number of clusters. Expressed verbally, the Herfindahl index is the sum of the squared employment shares of all clusters. A higher value on the Herfindahl index indicates greater specialisation of a region. The Herfindahl index would take its maximum value of if all employment within a region were provided by a single cluster and would take its minimum value of if all N clusters of a region were to employ the same number of people.
Two patterns appear when analysing the relation between cluster-specialisation and regional economic performance. First, regions where employment is highly concentrated in a few clusters tend to have higher per capita GDP levels than regions where employment is distributed across many clusters. Second, the opposite is true concerning per capita GDP growth. Regions that are diversified in the sense that employment is evenly distributed across many clusters have had, on average, higher growth rates since 2008 than regions where employment is concentrated in a few clusters.
Table 3.1 shows the results of regressions of log per capita GDP levels and average annual per capita GDP growth rates between 2008 and 2014. For ease of interpretation, the Herfindahl index has been normalised by its standard deviation. Thus, the coefficient on the Herfindahl index in Specification 1 implies that regions that have a 1-standard deviation higher Herfindahl index (i.e. are more specialised) and have on average a per capita GDP level that is 7.45% higher. The corresponding coefficient in Specification 2 shows, however, that those regions also had 0.14 percentage points lower annual per capita GDP growth between 2008 and 2014.
Importantly, the relationship between the Herfindahl index of cluster-specialisation and annual per capita GDP growth does not depend on whether a region initially had high or low per capita GDP levels. Specification 3 shows regression results that include log per capita GDP in 2008 as a control variable. The coefficient estimate on the normalised Herfindahl index remains virtually unchanged compared to Specification 2. In other words, a higher specialisation in a few clusters is associated with lower per capita GDP growth between 2008 and 2014, no matter whether the region had high or low per capita GDP levels in 2008.
Table 3.1. The effect of cluster specialisation on per capita GDP and per capita GDP growth
Log per capita GDP 2014 (1) |
Annual per capita GDP growth 2008-14 (2) |
Annual per capita GDP growth 2008-14 (3) |
|
---|---|---|---|
Normalised Herfindahl Index |
0.0745*** (0.0258) |
-0.0014** (0.0006) |
-0.0016*** (0.0006) |
Log per capita GDP 2008 |
- |
- |
0.0031 (0.0030) |
Country-fixed effects |
Yes |
Yes |
Yes |
Number of regions |
279 |
272 |
272 |
Note: ***,**,* indicate statistical significance at the 99%, 95%, and 90% confidence level, respectively. Heteroskedasticity robust standard errors in parenthesis.
Source: Calculations based on Ketels and Protsiv (2016[3]) with data provided by the authors and Eurostat.
The results in Table 3.1 allow for several related explanations. One possible explanation could be that it is not specialisation itself that has positive effects on growth but increasing specialisation that benefits growth. For example, regions that can shift resources from unproductive into productive clusters by specialising in them would see higher levels of per capita growth.
This explanation is consistent with the abovementioned econometric results. Since regions that have low levels of specialisation have greater potential for growing specialisation, it would explain why regions with low levels of specialisation see higher per capita GDP growth. It would also explain why regions with high levels of specialisation have high per capita GDP levels. These regions have gone through the growth-enhancing process of increasing specialisation and have consequently high per capita GDP levels, but due to their already high levels of specialisation they have little scope for further specialisation. The explanation is also in line with findings by Delgado et al. (2012[8]), who show that highly specialised clusters do not contribute to job creation. Instead, the authors find that job creation primarily occurs in related industries where the degree of specialisation is lower.
An alternative explanation that is equally consistent with the pattern above would be a natural trend towards greater specialisation as regions grow richer. More advanced industries tend to require more specialised knowledge and expertise. This may push regional economies towards greater specialisation as they become wealthier. According to this explanation, increasing specialisation does not cause growth. Rather, it would be a side effect of the greater complexity of more advanced economies. If high levels of specialisation prevent future growth, this trend could slow down growth if it is not counteracted by public policies.
Further research is required to determine the underlying causes of the abovementioned empirical regularities. Nevertheless, they have important policy implications even if their causes are not fully understood. They suggest that overly high specialisation can have detrimental effects on growth even though it is associated with high GDP levels. Thus, policy makers should refrain from encouraging excessive specialisation, especially if regions are already heavily reliant on only a few clusters. Instead, they should support continued growth by carefully encouraging diversification.
Box 3.3. Cluster data as a measure of traded activities
As discussed above, the definition of clusters by the European Cluster Observatory covers activities that are primarily or exclusively traded. Thus, the share of employment provided by the 51 defined clusters also provides an alternative measure of the importance of the traded sector in a region.
Specification 1 shows that regions with a larger share of employment in clusters tend to have higher per capita GDP levels than regions with a lower share of employment in clusters. A region with a one percentage point higher share of employment in clusters has a per capita GDP level that is approximately 2.2% higher. In contrast, Specifications 2 and 4 show that no statistically significant relation can be found between employment in tradable clusters and per capita GDP.
Table 3.2. Regional clusters and economic performance
Log per capita GDP (1) |
Per capita GDP growth 2008-14 (2) |
|
---|---|---|
Employment in tradable clusters in % |
0.0219*** (0.0041) |
0.0001 (0.0001) |
Log per capita GDP in 2008 |
- |
0.0006 (0.0032) |
Labour productivity relative to frontier in 2008 |
- |
- |
Country fixed-effects |
YES |
YES |
Number of regions |
268 |
272 |
Note: ***,**,* indicate statistical significance at the 99%, 95%, and 90% confidence level, respectively. Heteroskedasticity robust standard errors in parenthesis.
Source: Calculations based on Ketels and Protsiv (2016[3]) with data provided by the authors and Eurostat.
Diversification and catching-up
Just as specialisation affects per capita GDP levels and per capita GDP growth, it is likely that it has an effect on productivity levels and catching-up dynamics. Nevertheless, the effect might differ from effects on GDP levels, as it is possible that it affects productivity levels differently than employment levels (which determine GDP levels jointly with productivity).
In order to calculate catching-up performance, regional productivity is defined relative to productivity levels in frontier regions (i.e. regions that are ranked in the top 10% for productivity in a given country). Specification 1 in Table 3.3 shows that more specialised regions with a one standard-deviation higher Herfindahl index have productivity levels relative to the frontier that are on average 4.5 percentage points higher. The result, albeit not statistically significant, corresponds to the previous finding that more specialised regions have higher per capita GDP levels. Specification 2 confirms that the effect on catching-up performance is equivalent to the effect on per capita GDP growth, too. Highly specialised regions tend to diverge whereas diversified regions catch-up. A one standard deviation higher specialisation leads to a 0.2 percentage point lower catch-up rate in labour productivity.
Table 3.3. Specialisation and catch-up productivity growth
Labour productivity relative to frontier 2014 (1) |
Percentage point change in labour productivity frontier gap 2008-14 (2) |
Percentage point change in labour productivity frontier gap 2008-14 (3) |
|
---|---|---|---|
Normalised Herfindahl index |
0.0458 (0.0287) |
-0.0021** (0.0008) |
0.0049 (0.0031) |
(Normalised Herfindahl index) * (Labour productivity relative to frontier in 2008) |
- |
- |
-0.0084** (0.0033) |
Labour productivity relative to frontier in 2008 |
- |
-0.0025 (0.0066) |
-0.0016 (0.0065) |
Country fixed-effects |
YES |
YES |
YES |
Number of regions |
239 (excluding frontier regions) |
232 (excluding frontier regions) |
232 (excluding frontier regions) |
Note: ***,**,* indicate statistical significance at the 99%, 95%, and 90% confidence level, respectively. Heteroskedasticity robust standard errors in parenthesis.
Source: Calculations based on Ketels and Protsiv (2016[3]) with data provided by the authors and Eurostat.
Specification 3 varies from the previous estimations as it analyses whether specialisation affects lagging regions differently than those close to the frontier. For this purpose, a so-called ‘interaction-term’ for the Herfindahl index and labour productivity in 2008 is included. This interaction term can show whether regions that had low labour productivity relative to the frontier in 2008 are differently affected by specialisation than regions that had high labour productivity relative to the frontier. The coefficient on the interaction terms is statistically significant and negative. In contrast, the coefficient on the Herfindahl index changes from Specification 2 and becomes positive and statistically insignificant. This implies that the positive effect of low specialisation is strongest for regions that are strongly lagging behind the frontier.
Importantly, the effects of specialisation on catching up are largely driven by very highly-specialised regions. When excluding regions that have a Herfindahl index of more than two standard deviations above the average, coefficients in Specifications 2 and 3 become very small and statistically insignificant. Thus, specialisation appears to be an impediment to catching up in labour productivity mostly when it is very high. In contrast, the coefficient on the Herfindahl index in Specification 1 remains roughly unchanged. This implies that the general pattern (i.e. specialised regions have higher levels of labour productivity despite lower rates of productivity growth) seems to hold more widely across varying levels of specialisation and diversification.
Specialisation and patenting activity
One possible explanation why highly specialised regions have lower per capita GDP and productivity growth could be lower levels of innovation-related activity. This hypothesis is supported by the analysis of patenting activity in regions. Diversification benefits innovation, insofar as it can be measured by patents per capita. More specialised regions tend to have lower patenting activity per capita. Table 3.4 shows that regions with a one standard deviation higher Herfindahl index have approximately 15% fewer patents per capita than other regions.
Table 3.4. Specialisation and patenting activity
Log patents per capita (1) |
Log patents per capita (2) |
|
---|---|---|
Normalised Herfindahl index |
-0.146*** (0.050) |
-0.597*** (0.114) |
Labour productivity relative to frontier |
- |
4.451*** (0.510) |
Country fixed-effects |
YES |
YES |
Number of regions |
250 |
220 (excluding frontier regions) |
Note: ***,**,* indicate statistical significance at the 99%, 95%, and 90% confidence level, respectively. Heteroskedasticity robust standard errors in parenthesis.
Source: Calculations based on Ketels and Protsiv (2016[3]) with data provided by the authors and Eurostat.
Perhaps surprisingly, the magnitude of the estimated effect increases significantly if the productivity level of a region relative to the frontier is taken into account. After controlling for labour productivity relative to the frontier, the coefficient in Specification 2 indicates that regions with a one standard deviation higher Herfindahl index have almost 60% fewer patents per capita than other regions. This drastic effect on innovative activity provides a potential explanation for why per capita GDP growth rates in regions with higher specialisation tend to be lower.
In contrast to the negative relation between specialisation and productivity levels, the negative relationship between specialisation and patenting activity is not limited to highly specialised regions. In fact, it exists even among regions that are less specialised than average.
“Related varieties”
While the analysis above confirms that diversification can have positive effects on economic growth and innovation, it is likely that not any one type of diversification across clusters has the same positive effect. Frenken, van Oort and Verburg (2007[9]) and Boschma and Iammarino (2009[10]) show that diversification into so-called “related varieties” has positive effects on employment growth whereas unrelated variety across sectors has little or negative effects on employment growth.
Related variety in this context refers to economic activities that require related skills and knowledge. It does not necessarily imply that the activities are related through direct connections of their businesses or that they are located along the same value chains. In practical terms, related variety is often measured by the degree of diversification within very broadly defined economic sectors. For example, a typical economic sector defined at a high-level would be manufacturing. The greater a region’s diversification into ‘related varieties’ the greater the diversification of that region’s manufacturing output across different sub-sectors within manufacturing (e.g. manufacturing of vehicles, manufacturing of machinery, manufacturing of textiles, etc.).
The concept of related variety is linked to an academic debate about how knowledge and innovation is diffused throughout the economy. One school of thought emphasises that innovations are spread primarily within industries, whereas another school of thought argues that innovations are spread across industries. The two schools of thought highlight the importance of different types of knowledge-spillovers. So called Marshall-Arrow-Romer-(MAR)-externalities [named after Marshall (1890[11]), Arrow (1962[12]), and Romer (1986[13])] are knowledge-spillovers that occur within the same industry. In contrast, Jacobs-externalities [named after Jacobs (1969[14])] are spillovers that occur across different industries. Jacobs (1969[14]) argued that ideas and innovation in one industry will eventually be picked up by other industries within the same region. However, such knowledge spillovers are unlikely to occur randomly across different industries. Rather, it is more probable that knowledge is diffused across industries if the industries require similar kinds of knowledge.
Depending on which type of knowledge-spillover is more important, the optimal degree of specialisation would differ. If MAR-externalities dominate, regions would benefit from very high specialisation to maximise the probability of innovations and the impact of spillovers within an industry. In contrast, if Jacobs-externalities are more important, regions benefit from diversification across related varieties as described above. The empirical results presented in this report and the related literature appear to support Jacob-externalities.
Box 3.4. Small steps or great leaps – how to best support innovation?
The related varieties argument assumes implicitly that productivity growth is driven by the adoption and combination of ideas and innovations from related sectors. According to this line of reasoning, technological progress and productivity evolve gradually. Any advancement builds on existing capabilities and economic structures that are already in place. Consequently, strategies based on related varieties appear best suited for regions that already have some successful economic clusters already in place. In contrast, lagging regions that are most in need of economic development could struggle to successfully implement development strategies based on related varieties because they lack the economic base from which related varieties could emerge.
Furthermore, it is unclear to what degree public intervention is necessary to promote economic development through related varieties. It is possible that the innovation process based on related varieties occurs naturally without the intervention of public policy. If this is true, policy makers would do better to focus on overcoming coordination failures that prevent the emergence of entirely new clusters instead of supporting the evolution of existing ones. In other words, it could be possible that public policies should focus on promoting great leaps in economic development instead of small steps that would occur anyway.
At this point, too little is known about the nature of knowledge diffusion and spillovers to provide a definitive answer to the question. In any case, it is important to bear in mind that strategies focusing on great leaps to support the emergence of entirely new economic clusters have potentially significant downsides and can easily fail (OECD, 2007[1]). There is no guarantee that public policy will be able to identify coordination failures that prevent the emergence of new clusters or that it has the right tools to overcome such failures. Therefore, public support of evolutionary innovation based on related varieties appears to be more promising and less risky in most cases than big bets on great leaps.
Source: Authors elaboration and OECD (2007[1]), Competitive Regional Clusters: National Policy Approaches, OECD Publishing, Paris, http://dx.doi.org/10.1787/9789264031838-en.
The idea of related varieties is also reflected in the concept of smart specialisation, which emphasises public support for economic activities instead of sectors (OECD, 2013[15]). Smart specialisation has been a key element of the European Commission’s regional development policy since 2011 and the development of a smart specialisation strategy is a prerequisite to receive funding from the European Regional Development Fund (European Commission, 2017[16]). The focus on activities acknowledges the fact that innovations in an economic activity or process can benefit all sectors that make use of the activity or the process.
Despite the overall evidence pointing towards the importance of Jacobs-externalities and thus the benefits of related variety, important nuances need to be taken into account. Recent work by Caragliu, de Dominicis and de Groot (2016[17]) shows that different regions benefit differently from specialisation. In particular, regions with a high density of economic activity benefit from diversification in related variety as described above. In contrast, in low density regions, specialisation in a single industry can be more beneficial. This can be explained by the fact that many economic sectors require a critical mass to function well. In densely-populated areas with substantial economic activity, it is not difficult to achieve this critical mass in many sectors. However, for low density regions, this can only be achieved by specialising in a few selected sectors.
The policy implications of this result go beyond the finding that less dense regions benefit from greater specialisation. More generally, it suggests that a region’s optimal degree of specialisation cannot be determined without a thorough understanding of a region’s specificities. Beyond density, other characteristics are likely to play a role, such as the degree of polycentricism, geographic characteristics, workforce characteristics and so on.
Diversity and resilience
The diversity of the economic structure of a region has consequences beyond the growth performance of a region - a sufficient degree of diversity can also strengthen a region’s resilience to economic shocks. Regions that rely on a single cluster of firms or even on a single firm are at risk of a severe negative downturn if a shock hits the cluster or the firm (OECD, forthcoming[18]). In contrast, if regions are diversified and rely on various economic sectors and many different types of firms, the likelihood that all of them are simultaneously affected by negative shocks is much smaller. As a consequence, diversification can protect regions from severe downturns that are not caused by systemic events that affect all parts of the economy.
Box 3.5. From clusters to open innovation platforms in Tampere Region, Finland
The region of Tampere has a long-standing tradition of innovation and change. It was the cradle of Finnish industrialisation in the 19th century and continued to be a manufacturing powerhouse in the 1960s. It moved towards a university-driven knowledge economy, becoming a Nokia-led global ICT hub from the 1990s to the early 2010s (City of Tampere, 2015[19]).
A technological change in the mobile phone industry obliged Nokia to change its business strategy and to downsize globally. The negative impact of this downsizing showed in the 14.4% fall in Tampere’s GDP growth rate between 2008 in 2009 (Statistics Finland, 2015[20]). On the other hand, the large companies that predominantly constitute the region’s machine-building sector (mechanical engineering, paper and pulp) continue to play a very important role, accounting for approximately 26% of total employment in the region in 2014 (City of Tampere, 2016[21]). Nonetheless, after the global financial crisis in 2008, this sector faced lower demand and competitive pressures, reducing investment and employment levels.
In light of this context, the region’s innovation system needed review. The decline of Nokia and its related industries highlighted the need for Tampere Region to develop a new innovation policy, encouraging a move away from the previous cluster-based emphasis on sectoral specialisation towards a focus on a cross-cutting strategy for innovation. Indeed, the region gradually moved away from the regional cluster specialization policy (1994-2013) towards an open innovation platform policy (2009-present). In the platform economy, co-creation processes tighten the link between research and value creation, across widely different sectors, from administrative support to ICT technologies.
The cross-sectoral innovation strategy adopted by the Tampere region has proved successful in a number of ways. It enabled to break down silos and improve the exchange of expertise, leading to more adaptive and flexible innovation processes, the so-called open platform economy. The involvement of universities and R&D centres contributed to skilled human resource retention and fostered a culture of entrepreneurship.
Sources: Adapted from: OECD (2016[22]), Resilient Cities, Preliminary Report: www.oecd.org/cfe/regional-policy/resilient-cities-report-preliminary-version.pdf. City of Tampere (2016[21]), Response to the OECD questionnaire on Resilient Cities, www.tampere.fi/en/index.html; City of Tampere (2015[19]), Tampere: Open/smart/connected: Summary of the application by the city of Tampere, Finland to the contest for the European Capital of Innovation Award”, available at: https://fl-cdn.scdn1.secure.raxcdn.com/files/11649/tampere-icapital-proposal.pdf; Statistics Finland (2015[20]) Annual National Accounts, www.stat.fi/til/index_en.html.
In contrast to the abovementioned related varieties argument, Frenken, van Oort and Verburg (2007[9]) show that broad diversification offers the best protection against external shocks. Regions whose economies are diversified across as many sectors as possible (and not just across related varieties) are the least affected by external shocks. Nevertheless, the benefits of such broad diversification as a protection against shocks have to be weighed against any possible negative effect on average growth rates. In many cases, the benefits from improved average economic growth rates due to some specialisation in related varieties will outweigh the risks related to a higher susceptibility to economic shocks.
Box 3.6. Concerted efforts to promote innovation in Tampere Region, Finland
The innovation strategy adopted by the region of Tampere (Finland) (Box 3.5) sets a good example of a collaborative, multi-level innovation strategy. The local, regional and national levels of government have a clear and aligned long-term vision to promote innovation. Universities, public institutions and businesses are closely involved in this process, too.
The city of Tampere has adopted the new Open/Smart/Connected (O/S/C) strategy. It is based on three key elements: open innovation platforms, open data and interfaces and open participation (City of Tampere, 2015[19]). It involves issues of digitalisation, smart urban living, access to information and effective public procurement. The Tampere strategy follows the priorities and activities of the European Commission Horizon 2020 Work Programme 2016-17, such as smart and sustainable cities, promotion of healthy ageing and personalised health care, and piloting of demand‑driven collaborative innovation models.
The regional and city strategies benefit from the support of the national government. Finland’s national “INKA-Innovative Cities” programme was launched in 2014 to create competitive, high-tech companies and foster innovation clusters. The programme aims to generate new business and new companies from high-quality competences, creating more jobs. It is based on close local co‑operation and pooling of resources between science, education, companies and the government. The city of Tampere also plays a key role in the national strategy “The Six City Strategy – Open and Smart Services (2014-2020)”, aimed at sustainable development for the six largest Finnish cities. Tampere leads the Six City Strategy spearhead project on open data and collaborates in the projects of open innovation platforms and open participation.
Besides the national government, the open innovation strategies for the Tampere region and city have the support of key stakeholders. The local universities and corporate R&D facilities have started to make a successful transition to a more entrepreneurial and open mode of innovation, through their involvement in various platforms. The Tampere Region Economic Development Agency (TREDEA) provides services, information and assistance to firms and individuals to invest in or start a business venture in the region. TREDEA also leads the region’s international marketing on tourism, investment and innovation, through the “Tampere – All Bright!” strategy (www.tampereallbright.fi). Lastly, the Baltic Institute of Finland (BIF) promotes inter-regional co-operation. The BIF has secured a number of European projects and developed networks that support smart specialisation agenda in the Tampere region (Newcastle University, 2015[23]).
Sources: Adapted from OECD (2016[22]), Resilient Cities, Preliminary Report: www.oecd.org/cfe/regional-policy/resilient-cities-report-preliminary-version.pdf. City of Tampere (2015[19]), Tampere: Open/smart/connected: Summary of the application by the city of Tampere, Finland to the contest for the European Capital of Innovation Award”, available at: https://fl-cdn.scdn1.secure.raxcdn.com/files/11649/tampere-icapital-proposal.pdf; Newcastle University (2015[23]), “Smart specialisation for regional innovation: WP5 regional report on Pirkanmaa (Tampere), Finland”, www.regioconeixement.catedra.urv.cat/media/upload/domain_697/arxius/carpeta%20sense%20nom/tampere%20regional%20report%20-%20full%20version%20(october%202015).docx.
Encourage labour force mobility to start new clusters
Domestic and international in-migration can provide an important impetus and the human capital basis for the development of new clusters. Hausmann and Neffke (2016[24]) show that ‘pioneer plants’ rely heavily on experienced workers hired from other parts of the country. They define pioneer plants as those that open in a region in which no similar industry has existed previously. By definition, these regions do not have a domestic workforce with experience in the industry. Instead of training local workers, firms prefer to hire experienced workers from other regions. The authors argue that this is the case because actual experience provides crucial tacit knowledge that cannot be learned by training alone. Thus, hiring experienced workers from the outside is crucial to successfully open new plants or start new clusters.
To illustrate their point, Hausmann and Neffke (2016[24]) provide a series of historical examples to illustrate how the inflow of migrants created important and long-lasting clusters across the world. The beer brewing industry in the Czech Republic was started by immigrants from Bavaria. Likewise, wine-making in South Africa was introduced by immigrants from France. More recently, high-tech clusters in the People’s Republic of China (“China” herafter), India, Israel and other countries were founded by diasporas returning from the U.S. Rhee (1990[25]) shows how Bangladeshi garment workers trained in Korea created the seeds of Bangladesh’s successful garment industry.
These examples show that the skills brought by migrants to regions can provide the seeds for new clusters to emerge. More importantly, they also offer the possibility for diversification beyond related varieties into sectors that are completely unrelated to existing clusters. Such diversification is difficult to achieve based on endogenous factors alone, but if successful it offers a large potential for regions. It creates a new economic foundation from which further innovation can evolve and new clusters can be spun off.
A regional perspective on global value chains
The emergence of global value chains
The term global value chains (GVCs) describes production processes that take place in several stages across various countries around the world. GVCs are characterised by production processes that are split into several stages and distributed across countries. Throughout each stage of the production process, value is added, for example by producing a new good out of several intermediate goods or by refining an intermediate good. As intermediate goods and traded services used in GVCs flow from one country to another, the emergence of GVCs has contributed to growing global trade.
Value chains that divide the production process into different stages have existed for centuries even though the concept was first formally described by Porter (1985[26]). By connecting different activities that are necessary to produce a final good, they create more value than the sum of all activities contained within them would create on their own. Value chains also facilitate the division of labour and specialisation within the production process. The importance of this mechanism in fostering economic productivity has long been known and has famously been discussed by Adam Smith (1776[27]) in The Wealth of Nations.
Although value chains have existed for a long time, their nature started to change in the 1980s. Different steps in the production process were increasingly located within different countries. Value chains that were previously located primarily within one country became increasingly international. Initially, the production processes were often distributed across countries in close proximity, but in the late 1990s and early 2000s, they became truly global by integrating production processes over very long distances (Los, Timmer and de Vries, 2015[28]). Over the same time period, the importance of GVCs increased further, up to the point where they now form the basis of the global economy (OECD, 2017[29]).
As value chains became more prevalent, the production of more and more goods has been distributed across GVCs. Products made through GVCs range from goods requiring traditional manufacturing techniques such as textiles to technology-intensive goods such as electronics (OECD, 2017[29]). In parallel to becoming more prevalent, GVCs have also become longer and more complex. Production processes are divided into more steps that are distributed across countries. Along with the increasing flows of goods and services the economic interconnectedness of countries has increased.
Today, GVCs form the backbone of the global economy. Nevertheless, emerging evidence suggests that the importance of GVCs might have reached a peak. Global trade flows have started to decline as a share of GDP (IMF, 2016[30]); (OECD, 2016[31]). A number of structural factors – ranging from rising trade costs to new technologies that reduce the costs of small scale production – could make it more attractive to produce closer to markets. Notwithstanding these uncertainties about the future evolution of GVCs, at this point in time they are an essential element of every advanced economy (OECD, 2017[29]).
Vertical specialisation
One of the reasons behind the growth of GVCs has been so-called vertical specialisation [see (Hummels, Ishii and Yi, 2001[32])]. The term refers to an increasing division of the production process into more and more specialised steps. As vertical specialisation implies a break-up of the production process into many small steps, it is an important factor that contributes to being able to distribute production processes across countries. Not surprisingly, trade in vertically specialised intermediate goods significantly increased during the 1990s alongside the rise of GVCs (Chen, Kondratowicz and Yi, 2005[33]).
Increasing vertical specialisation in GVCs implies that an intermediate good might cross borders into and out of a country several times during the production process. In between each border crossing, the intermediate good is augmented or refined and a small amount of value is added in the process. In trade statistics, the intermediate good is registered as an export each time it leaves the country. Thus, increasing vertical specialisation and GVCs have contributed to rising gross exports in many countries.
However, rising gross exports do not necessarily indicate that a greater share of the economy relies on exports. Increasing vertical specialisation implies that the value added to an intermediate good before it is exported decreases. As a consequence, the total exported value-added can stay constant despite rising gross exports. If this effect is not taken into account, rising gross exports due to vertical specialisation and GVC integration can be misinterpreted as an increase in the overall importance of exports for the economy (Chen, Kondratowicz and Yi, 2005[33]). UNCTAD (2013[34])estimate that approximately 28% of the value-added in global gross exports has previously been imported.
Global value chains at the regional level
In contrast to the national level, where the importance of GVCs is undisputed, their role at the regional level is less well understood. While it could be expected that a greater degree of GVC integration is strongly correlated with economic performance, this is not necessarily the case. On the one hand, it is true that lagging regions are less integrated in GVCs than the average. Yet, the same is true for frontier regions as well. The most productive regions tend to be urban economies that rely heavily on an advanced service sector. As services play a comparatively small role in GVCs, frontier regions are in fact less integrated into GVCs than the average region.
More generally, the effects of GVC integration seem to vary widely across regions. Anecdotal evidence suggests that some regions benefit from GVC integration and are able to build important regional clusters around production processes within GVCs, whereas in others few connections between GVCs and the regional economy emerge.
Box 3.7. Regional GVCs
As described above, GVCs have been primarily discussed in terms of the distribution of production across different countries. Yet, they contain an important regional dimension. First, production processes are not only distributed across countries, they can also be distributed across regions. For example, R&D might take place in one part of a country whereas manufacturing might occur in another depending on the availability of workers with the right skill set. Second, GVC integration might vary significantly across regions within a country. Using only national level data would miss these regional differences.
Figure 3.4 shows a stylised value chain across four regions. Region 1 produces intermediate goods using capital and labour that get exported to Region 2 where they and other intermediate goods are used as inputs for the production of another intermediate good. Using this and other intermediate goods, a final good is produced in Region 3, which is partly consumed in Region 3 and partly exported for consumption in Region 4.
Many value chains and almost all global value chains are much more complex than the stylised structure presented in the figure below and can involve large numbers of intermediate goods being produced across many regions. Since many intermediate goods are produced from other intermediate goods that may come from different regions, they can also branch out into complex, tree-like structures instead of following a linear path.
Figure 3.4. A stylised value chain

Source: Adapted from Los, B. and W. Chen (2016[35]), “Global Value Chain Participation Indicators for European Regions”, Report for the OECD, December 2016 and Chen, H., Kondratowicz, M., & Yi, K. M. (2005[33]), Vertical specialization and three facts about US international trade. The North American Journal of Economics and Finance, 16(1), 35-59.
Data on regional GVC integration
Data on GVCs at the regional level is derived from so-called input-output tables that document all flows of goods and services into and out of a region as well as their origins and destinations. These are large matrices with sometimes hundreds of millions of entries. By summing up inflows and outflows of goods and services and calculating the difference between the two sums, it is possible to derive several meaningful measures that describe the integration of regions into GVCs.
The first global input-output tables became available in the early 2000s, but they did not provide any information on the regional distribution of trade flows within countries. This section analyses a new dataset by Los and Chen (2016[35]) that is based on regional input-output tables and provides information on regional GVC integration.
The following measures are used as key indicators on regional GVC integration:
Value-added within GVCs indicates the total value-added that is produced within GVCs in a region.
Regional labour income within GVCs measures the labour income that is earned within GVCs in a region.
Regional gross exports are the sum of all exports from a region. It is a measure of total trade activity. As discussed above, it includes the exported value-added from within a region and the value of previously imported intermediate goods that are exported.
Regional value-added in gross exports measures the value-added within a region that is exported. It is the difference between gross exports and gross imports. It includes value-added that is produced within GVCs and exported, but also value-added that is produced and exported in more basic trade relationships outside of GVCs.
Instead of using absolute values, many measures are more meaningful if they are expressed as shares or ratios. The following ones are used in this section:
Share of value-added from GVCs in total regional value-added is the share of regional value-added that is created within GVCs. It provides a good indication of the overall importance of GVCs for the regional economy because total regional value-added is a good proxy of regional GDP.
Share of regional domestic labour from GVCs in total regional labour income is another measure of the importance of GVCs for the regional economy focusing on labour income.
Share of regional value-added in exports in regional GDP provides a measure of the overall importance of exports (within and outside of GVCs) for the regional economy.
Share of exported regional value-added in regional gross exports is a measure of the concept of vertical specialisation that has been discussed above. The lower the share of value-added as a percent of gross exports, the greater the degree of vertical specialisation of exporters. If the share is very low, the average firm adds only little value to exports and is thus likely to work on a small and specialised step within the production process. In contrast, if the share is high, the average firm is likely to work on many steps within the production process and thus has a low degree of specialisation.
Key facts on GVCs at the regional level
GVC integration varies strongly not only across countries, but also across regions within a country. Figure 3.5 shows the value-added produced within GVCs as a share of total value added. While most national averages fall between 15% and 25%, the shares across regions within a country fluctuate by approximately 10 percentage points in many countries.
Figure 3.5. Contribution of GVCs to total regional value-added in Europe, 2010

Note: Small horizontal bars denote weighted country averages, long vertical bars show the range of values across regions within a country. No regionally disaggregated data available for Romania, Bulgaria, Slovenia, Malta, Latvia, Luxembourg, Lithuania or Estonia.
Source: Calculations based on data provided by Los and Chen (2016[35]), see Thissen, Lankhuizen and Los (2017[36]) for details.
Regional GVC integration remained largely stable between 2000 and 2010
While GVC integration varies widely from region to region, few changes occur over time. Figure 3.6 shows the average contribution of value-added within GVCs to total regional value-added from 2000 to 2010. It distinguishes three groups of regions: highly integrated regions are the top 25% of regions that obtain the highest share of value-added from GVCs in 2000. Regions with low integration are the bottom 25% of regions in terms of the share of value-added from GVC participation and regions with medium integration are the 50% of regions in the middle.
Figure 3.6. Share of value-added from GVCs in total regional value-added over time (by group)

Note: Highly integrated regions are the top 25% of regions with the highest percentage of value-added from GVCs in total regional value added in 2000. Regions with low integration constitute the bottom 25%, and regions with intermediate levels of integration are the 50% of regions in between.
Source: Calculations based on data provided by Los and Chen (2016[35]), see Thissen, Lankhuizen and Los (2017[36]) for details.
The figure shows two striking facts. First, it confirms that there are large differences in GVC integration across regions. The top 25% of regions on average obtain more than 30% of their value-added from economic activities within GVCs. In contrast, the bottom 25% of regions obtains only approximately 11% of their value-added from activities that are integrated in GVCs. Second, there is little convergence occurring. The share of GDP that is obtained from GVCs has moved mostly in parallel with the GVC share of more integrated regions and declined slightly between 2000 and 2010.
The lack of convergence in GVC integration across regions is largely due to a generally high degree of persistence in the degree of GVC integration over the 2000 to 2010 period. On average, the share of value-added from GVCs that a region obtained changed by less than 2.5 percentage points during this time period.
The picture is similar for other measures of trade activity. Gross exports rose by 20% between 2000 and 2010 despite significant declines in the immediate aftermath of the financial crisis in 2008. However, GDP grew by approximately 10% over the same time, implying that the growth in gross exports as a share of GDP has only been half as much.
Exported value-added as a share of total value-added increased only marginally by one percentage point from 19.7% to 20.7% over the same time period. In parallel, the share of value-added in gross exports has declined somewhat from 54.2% to 51.8%, thus indicating greater vertical specialisation.
Thus, while there has been a slight increase in exported value-added in combination with greater vertical specialisation, the overall picture has been relatively stable. Apart from a severe, but brief shock in 2009, no major changes in the overall importance of regional trade occurred between 2000 and 2010.
The general absence of clear trends on GVC integration is also reflected in Figure 3.7. It shows how GVC integration and vertical specialisation changed between 2000 and 2010 in each region. Each arrow represents a region with the end of the arrow indicating the region’s position in 2000 and its tip indicating its position in 2010. Panels are separated depending on whether the region is a frontier region, catching-up, diverging or lagging as defined in Chapter 1. While there is some variety across regions, a faint pattern can be discerned. On average, all groups of regions experienced a slight decline in GVC participation, as has already been mentioned above. More importantly, frontier and catching-up regions have experienced a decline in vertical specialisation, which indicates that their share of exported value-added in total exports has increased. In contrast, regions that diverge or keep pace have seen a roughly constant degree of vertical specialisation, which indicates stagnating shares of exported value-added.
Figure 3.7. GVC integration and vertical specialisation

Source: Calculations based on data provided by Los and Chen (2016[35]), see Thissen, Lankhuizen and Los (2017[36]) for details.
GVCs create high value-added in the regions where they have their final production stage
GVCs can be distinguished according to where the production of the final output occurs. Perhaps surprisingly, Figure 3.8 shows that in 2010 55% of the value-added that is produced within GVCs is produced within those GVCs whose final production occurs within the same region: 14% of the value-added is produced within GVCs whose final production stage occurs in another region within the same country and 31% of value-added is produced in GVCs whose final production stage occurs in a foreign country. Since 2000, GVCs with final production stages in foreign countries have increased slightly in importance, but in general the pattern has remained largely unchanged.
Figure 3.8. Share of value-added from GVCs depending on place of final production

Note: This bar chart shows the share of value-added from GVCs depending on whether the final production process takes place in the same region, in another region within the same country or in a foreign country. The values refer to the average region (i.e. they reflect the unweighted average across all 242 regions).
Source: Calculations based on data provided by Los and Chen (2016[35]), see Thissen, Lankhuizen and Los (2017[36]) for details.
A likely reason behind the importance of GVCs that end in the same region is the production of the final good. The value-added in the last production stage tends to be disproportionally large (UNCTAD, 2013[34]). If a region produces the final good in a GVC, by definition the GVC ends in this region and the region is likely to create high value-added from that GVC.
Regional GVC participation varies by sector
The data provides a picture of GVCs across five different sectors: mining, textiles, fuels, machinery, and other manufacturing. Among them, machinery and other manufacturing are by far the most important sectors, contributing each to approximately 6-7% of regional value-added, of which fuels contributed roughly half (just over 3%). With slightly above 2% and 1%, respectively, GVCs in mining and textiles contributed the lowest share of total regional value-added in 2010.
Figure 3.9 shows the development over time. Several patterns are visible. First, the contributions of GVCs in machinery and other manufactured goods move in lockstep. Both fluctuated over time and were comparatively strongly affected by the financial crisis and the subsequent decline in trade in 2009. Nevertheless, their average contributions to total regional value-added remained virtually identical over the entire observation period. Second, the contribution of GVCs in fuels remained constant between 2000 and 2010. Third, the importance of mining- and textile-related GVCs has diverged. Whereas the average contribution from mining-related GVCs increased steadily from 1.8% in 2000 to 2.2% in 2010, the average contribution from textile-related GVCs steadily declined from 1.9% to 1.1%.
Figure 3.9. Average share of value-added of GVCs as a % of total value-added by sector

Note: The (unweighted) average contribution of GVCs to regional GDP by sector.
Source: Calculations based on data provided by Los and Chen (2016[35]), see Thissen, Lankhuizen and Los (2017[36]) for details.
Of course, averages hide significant variation across regions. In particular, regions vary considerably with respect to the importance of GVCs in the textile sector and in fuels. The coefficient-of-variation that measures the standard deviation relative to the mean of a variable is above 0.6 in both sectors in 2010. For example, the region with the lowest importance of GVCs in fuels obtains only 0.3% of its total value-added from it, whereas the region with the highest importance receives approximately 13% from it. With coefficients-of-variation of approximately 0.5, mining and machinery also show relatively strong variation across regions. In contrast, the importance of GVCs in other manufactured goods varies the least across regions with a coefficient-of-variation of approximately 0.35.
Exports’ contribution to labour income is below average
Across all regions, the labour share – the percentage of GDP earned as labour income – is 48.8%, which is in line with the OECD average.2 This is somewhat higher than the share of labour income earned from exports. Only 46.6% of the value-added that is exported is earned as labour income.
Regions with diverging labour productivity have a higher labour share in aggregate GDP and exported value-added. The labour share is 52.4% of general GDP and 49.2% of exported value-added. Assuming the return to capital equalises across regions, production in diverging regions tends to be less capital intensive than in other regions.
Figure 3.10. Labour share in GDP and exported value-added

Source: Calculations based on data provided by Los and Chen (2016[35]), see Thissen, Lankhuizen and Los (2017[36]) for details.
When the labour share in exported value-added is plotted against the labour share in GDP, another pattern emerges. Diverging regions tend to have higher labour shares in their general GDP relative to the labour share in exported value-added. In contrast, regions that are catching up tend to have a lower labour share in general GDP relative to exported value-added. In Figure 3.10, this can be seen by diverging regions lying mostly above the trend line and regions that are catching-up fall mostly below the trend line.
GVC participation increases exports
Increased GVC participation is associated with greater exports. Specification 1 in Table 3.5 shows that a 1% increase in value-added produced within GVCs in a region leads to a 0.75% increase in total exports. Given that GVCs account for, on average, only half of regional exports; this implies that increased GVC participation typically goes along with increases in other exporting activities, too. This could be seen as an indication that the same factors that foster regional GVC participation are also responsible for more general export performance and vice versa.
Table 3.5. GVCs and exports
Log regional gross exports (1) |
Log regional value-added in exports (2) |
Log regional gross exports (3) |
Log regional value-added in exports (4) |
|
---|---|---|---|---|
Log value-added from regional GVCs |
0.75*** (0.03) |
0.72*** (0.03) |
0.47*** (0.03) |
0.58*** (0.03) |
Region fixed-effects |
YES |
YES |
YES |
YES |
Year fixed-effects |
YES |
YES |
YES |
YES |
Linear regional trend-growth |
- |
- |
YES |
YES |
Number of regions |
241 |
241 |
241 |
241 |
Number of observations |
2651 |
2651 |
2651 |
2651 |
Note: ***,**,* indicate statistical significance at the 99%, 95%, and 90% confidence level, respectively. Heteroskedasticity robust standard errors in parenthesis.
Source: Calculations based on data provided by Los and Chen (2016[35]), see Thissen, Lankhuizen and Los (2017[36]) for details.
Perhaps surprisingly, a 1% increase in value-added produced within GVCs also leads to a 0.72% increase in exported value-added as shown in specification 2, Table 3.5. Thus, the estimated increase in exported value-added is only slightly smaller than the increase in gross exports. This suggests that increasing integration into GVCs has not lead to substantial increases in vertical specialisation, which would be characterised by increases in gross exports without corresponding increases in exported value-added.
On the contrary, if regional trend-growth is taken into account (specifications 3 and 4) the estimates even suggest that regional GVC participation has led to a disproportionally high increase in exported value-added. Whereas a 1% increase in value-added from GVCs is estimated to lead to a 0.47% increase in gross exports, the effect on exported value-added is estimated to be 0.58%.
Box 3.8. A note on statistical models
This box presents a brief and largely non-technical description of the statistical models that are used for the estimations presented in this section. Unless otherwise noted, all estimates are based on so-call fixed-effects models for panel data that use annual data from 2000 to 2010 for 241 European regions. This implies that the estimates describe the consequences of changes over time within regions. In other words, the estimates are not based on comparisons of different regions with each other, but on the average effects of changes within regions over time. This eliminates the general characteristics of a region that do not change over time, such as its geographic location.
Furthermore, the estimates do not capture effects from changes over time that affect all regions similarly. This is important because the data covers the 2000-2010 time period, which includes the financial crisis of 2008 and its immediate aftermath. The severe recession during this period had exceptional effects on global trade patterns. If these effects were not excluded from the estimations, they could lead to spurious results. However, insofar as the financial crisis was a global phenomenon that affected all regions in a similar way, the estimates presented in this section are not influenced by it. Only if regions were affected in unusual ways by the crisis would it influence the results shown in the following.
Some of the estimated specifications also include variables to rule out that linear trend-growth in regions affects the results. This is important if the dependent variable and the explanatory variable grow for unrelated reasons at similar rates within regions. For example, many eastern European regions had high GDP growth rates during the early 2000s. At the same time, they also experienced strong growth in GVC participation.
While the two processes are most likely connected to some degree, it cannot be ruled out that at least partially they are due to independent catch up processes that did not affect each other. By including trend-growth terms in the estimations the results are not influenced by (linear) growth processes that occur in parallel, but for unrelated reasons.
All estimations use log-levels or ratios as variables of interests. This implies that the coefficients can be interpreted as the percentage change in the dependent variable in response to a 1%change in the independent variable (in case of log-levels) or as the percentage point change in the dependent variable in response to a 1 percentage point change in the independent variable (in case of ratios).
Formally, the following Specification is estimated:
,
where indicates the outcome variable in region i and year t, is a set of explanatory variables, are the coefficients of interest, is a set of region fixed-effects, is a set of year-fixed effects, t is a set of variables capturing linear time-trends for each region, and is the error term for region i and year t. Cluster-robust standard errors are computed to allow for arbitrary correlations of the error terms within regions.
When interpreting the estimates in this shapter, the reader should keep the maxim correlation does not imply causality in mind. It is generally incorrect to infer that one variable causes a change in another variable just because the two variables are correlated with each other. Much more commonly, there are many unobserved variables that cause changes in both observed variables and are the cause of their correlation.
The estimates presented in this section employ a variety of methods that prevent many of such unobserved variables from influencing the estimates. Yet, it is unlikely that they prevent all unobserved variables from influencing the estimates. In particular, region-specific factors that fluctuate over time and affect both variables of interest in an estimation (e.g. GDP and value-added from GVCs) can create correlations between the two variables that could be misinterpreted as causal effects.
As a consequence, the estimates described throughout this chapter should be carefully interpreted. They are most likely influenced by unobserved variables and therefore cannot be interpreted as showing the causal impact of a change in one variable on another variable.
The above-mentioned pattern is confirmed if the impact of increasing GVC participation on vertical specialisation is estimated directly. Specifications 1 in Table 3.6 indicates that the share of regional value-added in exports increases by 3.8 percentage points if regional GVC participation increases by 1%. This implies decreasing vertical specialisation. Once regional growth-trends are taken into account, the estimate becomes somewhat smaller but still remains statistically significant. As specification 2 shows, an increase in value-added from GVCs of 1% leads to an 1.6 percentage points increase in exported value-added as share of regional gross exports. Thus, the evidence suggests that greater GVC participation actually reduces vertical specialisation at the regional level.
Table 3.6. GVCs and vertical specialisation
Share of exported regional value-added in regional gross exports (1) |
Share of exported regional value-added in regional gross exports (2) |
|
---|---|---|
Log value-added from regional GVCs |
3.81*** (0.53) |
1.59** (0.64) |
Region fixed-effects |
YES |
YES |
Year fixed-effects |
YES |
YES |
Linear regional trend-growth |
YES |
|
Number of regions |
242 |
242 |
Number of observations |
2662 |
2662 |
Note: ***,**,* indicate statistical significance at the 99%, 95%, and 90% confidence level, respectively. Heteroskedasticity robust standard errors in parenthesis.
Source: Calculations based on data provided by Los and Chen (2016[35]), see Thissen, Lankhuizen and Los (2017[36]) for details.
Some studies predict that greater GVC integration will lead initially to greater vertical specialisation, which is followed by a subsequent decline in vertical specialisation as regions develop and increase their exported value added [e.g. (UNCTAD, 2013[34])]. However, no evidence for such a u-shaped relationship between GVC integration and vertical specialisation can be found in the data, either.
GVC integration goes hand in hand with better economic performance
Greater GVC integration is strongly positively correlated with better economic performance at the regional level. A 1% increase in value added from GVCs is associated with a 0.52% increase in GDP levels (Table 3.7, Specification 1). Given that on average, GVCs create less than 20% of a region’s value-added, this implies that for each additional euro created within GVCs, another 1.5 euros of value-added is created outside of GVCs.
Table 3.7. GVC integration and GDP levels
Log GDP (1) |
Log GDP (2) |
|
---|---|---|
Log value-added from regional GVCs |
0.52*** (0.04) |
0.41*** (0.042) |
Log value-added in exports |
- |
0.33*** (0.094) |
Log gross exports |
- |
-0.17* (0.10) |
Region fixed-effects |
YES |
YES |
Year fixed-effects |
YES |
YES |
Linear regional trend-growth |
YES |
YES |
Number of regions |
241 |
241 |
Number of observations |
2651 |
2651 |
Note: ***,**,* indicate statistical significance at the 99%, 95%, and 90% confidence level, respectively. Heteroskedasticity robust standard errors in parenthesis.
Source: Calculations based on data provided by Los and Chen (2016[35]), see Thissen, Lankhuizen and Los (2017[36]) for details.
Specification 2 in Table 3.7 takes the effect on value-added in exports into account. This reduces the effect of a 1% increase in GVC participation to 0.41%, whereas a 1% increase in exported value-added increases GDP by 0.32%. The estimates imply that an increase in value-added that gets exported through GVCs has an impact on GDP that is more than twice as big as an increase in exported value-added that is exported outside of GVCs. Whereas a 1% increase in the former is associated with a GDP increase of 0.41% + 0.33% = 0.74%, the latter is associated with an increase of only 0.32%. Thus, while increases in exported value-added are positively associated with GDP, the effect appears much stronger when those exports occur through GVCs.
In contrast, gross exports are only very weakly and negatively correlated with GDP once the effects of value-added from GVC participation and exported value-added have been taken into account. This indicates that exports per se create little benefits. Unsurprisingly, it is the value-added contained in those exports that matters for economic performance.
Increasing labour income from GVCs is negatively correlated with GDP
Although the estimates suggest that GVCs generally have positive effects on economic performance, there are signs that not every kind of GVC integration is positive for the regional economy. In particular, an increasing share of labour income from GVCs relative to the value-added produced within GVCs is correlated to lower GDP levels. A one percentage point increase in the ratio of labour income from GVCs relative to value-added produced within GVCs is associated with a 0.2% decrease in GDP levels.
This finding provides evidence that some forms of increasing regional GVC integration can actually harm economic performance and labour productivity. GVC integration in labour intensive sectors tends to reduce productivity and GDP. This can most likely be explained by the fact that GVC participation in labour intensive sectors frequently involve activities that create low value-added. Furthermore, these activities offer little potential for innovation and are often disconnected from other parts of the economy. In contrast, no statistically significant correlation between GVC integration and the employment rate of a region can be found if regional and year fixed-effects are taken into account.
Box 3.9. Who are the losers from trade?
This chapter provides tentative evidence that trade and GVC integration can benefit regions. However, it is important to acknowledge that trade also has downsides for some regions. Regions lose from increasing trade if economic activities in which they have specialised are replaced by traded products from other regions or countries.
The estimates in this chapter do not show if and to what degree the positive effects of trade and regional GVC integration occur at the expense of other regions. This is primarily due to the difficulty in identifying where economic decline is due to trade and where it is due to other factors. Data on GVCs show in which regions trade and value-added from GVCs have grown, but that data does not show if this growth has raised overall economic output or simply displaced economic activity from one region to another. Thus, the data can show which regions are the winners from trade and how they benefit from it, but they are less useful in identifying the losers.
Recent evidence by leading scholars such as Autor, Dorn and Hansen (2016[37]) and Acemoglu et al. (2016[38]) shows that growth in international trade can indeed have strong negative effects on some economic sectors even if it benefits national economies in aggregate. Facing new competition from businesses abroad, some industries have declined strongly in OECD countries. Since these industries are often not evenly distributed across countries, but concentrated in specific regions, the negative impact from increasing trade can outweigh the benefits in these regions. Chapter 5 discusses policies to respond to trade’s downsides.
The economic benefits of GVC integration depend on the position within the GVC
Different activities within GVCs create different amounts of value-added. In particular, production steps at the beginning and the end of a GVC tend to create greater amounts of value-added than production activities in the middle of the GVC. This pattern is well documented and is sometimes called the ‘smile curve’ because of its characteristic shape when plotted on a graph.
Early stages in a GVC include research and development activities, which tend to be high in value-added. Likewise, resource extraction can create very high amounts of value-added.3 In contrast, manufacturing and fabrication processes that are in the middle of a GVC are often low in value-added, especially when they include mass production. Lastly, the final stages of the GVC often create high value-added again, which is also reflected in the previously discussed Figure 3.8. They include activities such as branding and services related to the final product.
Thus, the benefits that regions derive from GVC participation will depend on where in the value chain the activities are located. Regions that are initially only able to attract low value-added activities in the middle of the GVC should try to expand upstream or downstream along the GVC into activities that create more value-added.
Policies to maximise the returns from GVC participation
Due to the global nature of GVCs, production processes that are integrated in them can lack connections to the regional economy. Intermediate goods used in the production process might be imported, knowledge and intellectual property used at a production facility is often created outside the region and marketing and after-sales services are potentially provided from somewhere else, too. In such situations, little value is created by a GVC within the region and there is a risk that greater GVC participation will create few benefits for the broader economy operating outside of GVCs. This section provides suggestions about how to avoid such situations and maximise benefits from GVC integration.
Linking GVCs to cluster policy
Policies to integrate GVCs into the regional economy are closely linked to cluster policies. Clusters can create distinct absolute advantages for regions if they offer access to services or time-critical intermediate goods that are not available in other regions. They can also contribute to the development of particular skill sets among regional workers. Through all these factors, clusters can attract investments linked to GVCs that rely on the availability of these services. In the best case, virtuous cycles can develop in which existing regional clusters attract investments linked to production stages within GVCs, which in turn strengthen the existing clusters by increasing the demand for the goods and services produced by them.
Clusters and GVCs are governed in opposing ways. Humphrey and Schmitz (2000[39]) show that clusters are characterised by strong horizontal inter-firm co-operation and active co-ordination from public institutions. In contrast, GVCs are characterised by strong inter-firm governance along the value chain. Due to their fragmented nature across borders, national or regional institutions play only a limited role in governing GVCs. Compared to cluster policies, they have fewer possibilities to intervene directly and to shape the GVCs active in their region.
Yet, regional authorities are not powerless when facing GVCs. Regional institutions and governance structures play a crucial role in shaping how GVCs are integrated in the regional economy. They can influence the degree to which firms are willing to link their production processes with the regional economy and facilitate knowledge transfers. However, the bargaining power with respect to central firms in GVCs can vary widely, depending on aspects such as regional characteristics and the national institutional framework. This can influence the degree to which policy makers can compel firms to link their GVCs into regional economies. To negotiate effectively with large firms it is particularly important that policy makers be well-informed about characteristics related to GVCs and the regional economy (Coe et al., 2004[40]). The four following criteria are especially important.
First, policy makers need to have clear and detailed knowledge about the characteristics of their regions, in particular concerning the location-specific factors that make the region attractive for investments. Second, policy makers need to be aware of the sectoral specificities of the firms they are negotiating with. Firms in different sectors have different requirements and policies should be targeted at the specific requirements of firms. Third, policy makers need to be aware of their own institutional environments and the motivations and incentives of all relevant actors. Finally, policy makers need to understand which economic activities are most beneficial for their regions and target their policy interventions accordingly, for example by compelling firms to maximise the value-added within their regions (Coe et al., 2004[40]).
Strengthen the service sector connected to GVCs
GVCs are often portrayed as being primarily about the flow of goods from one country to another. Yet, this oversimplification risks distorting their nature and may lead to focusing on the wrong policy priorities. The OECD (2016[31]) emphasises that governments should not only focus on the core activities within a value chain (usually the manufacturing part), but pay attention also to business activities that support core activities. These activities may be located upstream (e.g. R&D, engineering) or downstream (e.g. transport, distribution, marketing, aftersales services) and often feature less prominently in the public debate on trade and global value chains, despite their growing importance over time.
Policies targeted at GVCs should take these activities into account. Some countries have succeeded in building significant clusters around these activities, whereas others rely more on offshore services. Regions that try to strengthen clusters around GVCs should not prefer manufacturing over the service sector. The value-added generated by an activity matters more for economic performance than the sector in which the activity occurs. Both the service sector and the manufacturing sector include activities that create high value-added and activities that create low value-added. Instead of focusing on the sector, policy makers should take the value-added in an activity as a yardstick when deciding whether or not to support it.
Develop complementary policies to address the downsides of increasing trade
While GVC integration offers significant potential gains to regions, these gains do not accrue automatically. The OECD (2016[31]) highlights the importance of complementary policies that can address the potential downsides of GVC integration. Trade increases specialisation and changes the structure of the economy. While this tends to be beneficial in aggregate, it can potentially create significant losses for specific groups of workers or economic sectors that are potentially concentrated in specific regions.
Several different policies are necessary to address the losses that trade and GVC integration can create. In particular, skill and education policies are crucial to reduce the impact of workers. Only when workers are able to adapt their skill profile to the changing demand for labour can they find new opportunities when economic sectors disappear. However, it is unlikely that skill and education policies alone will be enough.
Many skills policies are generic in the sense that they do not target the workers who are most affected by trade shocks. Due to the strong geographical concentration of the losses from trade and GVCs in some regions, skill and education policies are often not enough to compensate for the negative effects. Since trade can lead to a decline in the number of firms in a region, it is important to address not only labour supply but also labour demand. This includes, for example, policies to foster firm creation or to attract foreign direct investment into a region. Furthermore, workers should be supported to find jobs in other regions where unemployment rates are lower. These policies will be discussed in Chapter 5.
Regional employment shocks from trade and automation
Economists have long argued that trade is mutually beneficial for the countries involved in it. The theory of comparative advantage developed by Ricardo (1817[41]) states that by trading with each other, two countries can both increase their production and consumption because each country can focus on the production of the good it is comparatively more specialised in. Even today, gains from comparative advantage are a centrepiece of many of the most important theories of international trade, e.g. (Krugman, 1979[42]). Furthermore, economists have identified expanding markets as an important benefit from trade because it increases innovation (Grossman and Helpman, 1993[43]) and creates economies of scale. More recently, Melitz (2003[44]) argued that trade benefits more productive firms, which gain international market share at the expense of less productive firms. This process is beneficial in aggregate because it increases the average productivity level of a country by shifting resources from less productive firms to more productive firms.
Yet, trade theories also acknowledge that trade can generate losers. Theories of comparative advantage have distributional implications, which predict that trade harms the owners of the comparatively scarce production factors. Furthermore, trade creates losers among the less productive firms that cannot compete anymore (Melitz and Trefler, 2012[45]). Both effects eventually have an impact on some workers, who see their wages reduced due or their jobs disappear.
In light of the benefits from trade, the negative effects of trade on some workers were less of a concern if they occurred gradually and if the affected workers were evenly distributed across a country. In such an ideal scenario, jobs in unproductive firms that go out of business would be replaced quickly by more productive jobs in more productive firms. Workers would find new jobs in these firms and benefit from the turnover because rising productivity would be reflected in higher wages.
However, recent empirical evidence suggests that job losses from trade can be concentrated in some regions, occur rapidly and take a long time to be offset by job growth in other firms or sectors (OECD, 2017[46]). As documented by Autor, Dorn and Hanson (2016[37]), the rise of imports from China has led to a decline in employment in the manufacturing sector in many OECD countries. Since the industries that have been affected by import competition were often clustered in specific regions, the employment losses are equally concentrated in those regions [see Autor, Dorn and Hanson (2013[47]); Dauth, Findeisen and Suedekum, (2014[48]); Donoso, Martin and Minondo, (2015[49]); and Balsvik, Jensen and Salvanes, (2015[50])].
Even job losses that are located in one specific geographic location and sector would not pose a major problem if workers could easily find jobs in other sectors in the same region or in other regions. Yet, laid-off workers in affected regions struggle to find other jobs because affected regions lack dynamic sectors, which would create jobs that would offset those trade-related job losses. As a consequence, unemployment rates remain persistently high over long periods of time in regions that have suffered from negative trade shocks (Autor, Dorn and Hanson, 2016[37]).
Thus, policy makers face the conundrum that trade – while overall beneficial for national economies – can have important and lasting negative effects in some regions. This is an important point to understand. Previous theories of trade assumed that the effects of trade would be equally spread across all regions so that the aggregate benefits dominate everywhere and no specifically place-based policy response would be needed to address the downsides. However, given that the negative effects are predominantly concentrated in specific regions, policies to help those regions are much more important than previously thought.
In contrast, protectionist policies that restrict trade with other countries to help affected regions are likely to do more harm than good. They would reduce productivity gains from specialisation and slow down innovation. As a consequence, they would reduce living standards in the long run. Furthermore, they would harm consumers across the country that would have to pay higher prices on many goods that could be imported more cheaply than produced domestically if protectionist measures were not in place. While they could be beneficial for regions that are most affected by negative trade shocks, the downsides for the rest of the country would outweigh those benefits.
Automation increases wage disparities between low- and high-skilled workers
In parallel to the rise in trade, a second global trend has had strong effects on regional employment in manufacturing. Automation in manufacturing has made jobs obsolete by replacing workers by machines. While this process has been going on over centuries (e.g. weavers’ jobs were replaced by mechanic looms in the 19th century), it has been receiving increasing attention recently because of increasing computerisation. Growing use of computers and robots has made it possible to use robots for a wide range of manufacturing processes that previously had to be performed manually.
In order to estimate the impact of automation on jobs, economists distinguish between jobs that primarily involve routine tasks and those that involve primarily non-routine tasks. Routine tasks tend to be repetitive and occur in controlled environments. Compared to non-routine tasks, they can easily be performed by machines or computers. In contrast, non-routine tasks require adaptive responses to changing environments. They are difficult to automate (Autor, Levy and Murnane, 2003[51]). Not surprisingly, many routine tasks are predominantly low-skilled whereas non-routine tasks are more frequently high-skilled. Nevertheless, the routine-content and skill-level of a task are not identical and some high-skilled tasks are mostly routine, whereas some low-skilled tasks are non-routine (OECD, 2015[52]).
In contrast to trade, automation seems to have had less of an impact on unemployment up to now. Instead, it has shifted employment towards non-routine jobs (Autor, Dorn and Hanson, 2015[53]). In other words, jobs that are made obsolete due to automation tend to be replaced by jobs in other sectors. While automation has had, at most, a moderate impact on unemployment, it has probably contributed to greater wage inequality (Autor, 2015[54]). The jobs that have replaced those that disappeared due to automation are frequently service sector jobs. They tend to be either disproportionally high-paying (e.g. many intellectual services) or disproportionally poor-paying (e.g. many manual services) (OECD, 2017[55]).
Thus, the effects of trade and automation on regional employment can appear similar, but they are not identical for two reasons. First, trade shocks have increased unemployment whereas automation has led to increasing labour market polarisation and potentially to greater inequality. Second, jobs that are vulnerable to trade shocks are often clustered in different regions than jobs that are vulnerable to automation.
Why trade and automation shocks differ
A potential reason for why trade and automation affect employment patterns differently is that they have different effects on firm performance. Foreign competition is generally negative for local firms. It is well documented that it can force firms out of business and can lead to the disappearance of entire industries in extreme cases, e.g. (Underhill, 2016[56]). In contrast, the effects of automation on firms are more ambiguous. Rapid technological change creates challenges for firms and requires them to adapt quickly. Yet, it also offers important upsides for those firms that are able to use technological advances to improve the efficiency of the production process (Bharadwaj, 2000[57]). Thus, automation is less likely to homogenously harm all firms across a region.
As a consequence, automation leads to job losses among workers whose jobs get automatized, but does not destroy the underlying fabric of firms within a region. Labour demand from these firms will still exist, even though they are likely to demand a different and more advanced set of skills from their workers. This is reflected by the abovementioned fact that automation leads to labour market polarisation that tends to benefit high-skilled workers in particular.
Importantly, the evidence on the regional impact of trade and automation is very recent and is still evolving. Most of the papers cited in this section have been written by a small group of authors. Furthermore, most of the results are focused on the United States. Additional research by other authors might yield insights that add important nuances or affect the interpretation of the findings that have been presented in this section.
Looking forward, it appears likely that automation will have greater labour market impacts than trade. As mentioned previously, GVC integration and trade is stagnating and is unlikely to increase in considerable proportions in the future. In contrast, the nature of automation is changing. Where in the past it affected mostly manufacturing, there are signs that it will have stronger impacts on service sector jobs in the future (OECD, 2017[55]).
The effect of future automation on labour markets might very well differ from the effects observed in the past. It is possible that it will rapidly affect a larger group of workers than previous waves of automation. For example the ITF (2017[58]) estimates that up to 4.4 million out of a total of 6.4 million truck drivers could be replaced by 2030 if autonomous vehicles become available quickly. If this materialises, the consequences might go beyond wage polarisation and could have important employment effects. Given that future waves of automation could occur in the intermediate future, regional policy should try to anticipate any region-specific consequences and proactively plan ahead.
References
[38] Acemoglu, D. et al. (2016), “Import competition and the great US employment sag of the 2000s”, Journal of Labor Economics, Vol. 34/S1, pp. S141-S198.
[12] Arrow, K. (1962), “The economic implications of learning by doing”, Review of Economic Studies, Vol. 29, pp. 155-173.
[51] Autor, D., F. Levy and R. Murnane (2003), “The Skill Content of Recent Technological Change: An Empirical Exploration”, The Quarterly Journal of Economics, Vol. 118/4, pp. 1279-1333.
[47] Autor, D., D. Dorn and G. Hanson (2013), “The China Syndrome: Local Labor Market Effects of Import Competition in the United States”, American Economic Review, Vol. 103/6, pp. 2121-2168.
[54] Autor, D. (2015), “Why are there still so many jobs? The history and future of workplace automation”, The Journal of Economic Perspectives, Vol. 29/3, pp. 3-30.
[53] Autor, D., D. Dorn and G. Hanson (2015), “Untangling trade and technology: Evidence from local labour markets”, Economic Journal, Vol. 125/584, pp. 621-46.
[37] Autor, D., D. Dorn and G. Hanson (2016), “The China shock: Learning from labor-market adjustment to large changes in trade”, Annual Review of Economics, Vol. 8, pp. 205-240.
[50] Balsvik, R., S. Jensen and K. Salvanes (2015), “Made in China, sold in Norway: Local labor market effects of an import shock”, Journal of Public Economics, Vol. 127, pp. 137-144.
[57] Bharadwaj, A. (2000), “A resource-based perspective on information technology capability and firm performance: An empirical investigation”, MIS Quarterly, Vol. 24/1, pp. 169-196.
[10] Boschma, R. and S. Iammarino (2009), “Related variety, trade linkages, and regional growth in Italy”, Economic Geography, Vol. 85/3, pp. 289-311.
[17] Caragliu, A., L. de Dominicis and H. de Groot (2016), “Both Marshall and Jacobs were right!”, Economic Geography, Vol. 92/1, pp. 87-111.
[33] Chen, H., M. Kondratowicz and K. Yi (2005), “Vertical specialization and three facts about US international trade”, The North American Journal of Economics and Finance, Vol. 16/1, pp. 35-59.
[19] City of Tampere (2015), Tampere: Open/smart/connected: Summary of the application by the City of Tampere, Finland to the contest for the European Capital of Innovation Award, https://fl-cdn.scdn1.secure.raxcdn.com/files/11649/tampere-icapital-proposal.pdf.
[21] City of Tampere (2016), Response to the OECD Questionnaire on Resilient Cities, http://www.tampere.fi/en/index.html.
[40] Coe, N. et al. (2004), “'Globalizing' regional development: A global production networks perspective”, Transactions of the Institute of British Geographers, Vol. 29/4, pp. 468-484.
[48] Dauth, W., S. Findeisen and J. Suedekum (2014), “The rise of the East and the Far East: German labor markets and trade integration”, Journal of the European Economic Association, Vol. 12/6, pp. 1643-1675.
[8] Delgado, M. et al. (2012), “The determinants of national competitiveness”, No. w18249, National Bureau of Economic Research.
[4] Delgado, M., M. Porter and S. Stern (2016), “Defining clusters of related industries”, Journal of Economic Geography, Vol. 16/1, pp. 1-38.
[49] Donoso, V., V. Martin and A. Minondo (2015), “Do differences in the exposure to Chinese imports lead to differences in local labour market outcomes? An analysis for Spanish provinces”, Regional Studies, Vol. 49/10, pp. 1746-1764.
[16] European Commission (2017), Smart specialisation, https://ec.europa.eu/jrc/en/research-topic/smart-specialisation (accessed on 4 September 2017).
[5] European Commission (2017), “Competitiveness in Low-Income and Low-Growth Regions: The Lagging Regions Report”, European Commission Staff Working Document, European Commission.
[9] Frenken, K., F. Van Oort and T. Verburg (2007), “Related variety, unrelated variety and regional economic growth”, Regional Studies, Vol. 41/5, pp. 685-697.
[6] Glaeser, E. et al. (1992), “Growth in cities”, Journal of Political Economy, Vol. 100/6, pp. 1126-1152.
[43] Grossman, G. and E. Helpman (1993), Innovation and Growth in the Global Economy, MIT Press.
[24] Hausmann, R. and F. Neffke (2016), “The Workforce of Pioneer Plants”.
[7] Herfindahl, O. (1950), Concentration in the Steel Industry, Columbia University.
[32] Hummels, D., J. Ishii and K. Yi (2001), “The Nature and Growth of Vertical Specialization in World Trade”, Journal of International Economics, Vol. 54/1, pp. 75-96.
[39] Humphrey, J. and H. Schmitz (2000), “Governance and upgrading: Linking industrial cluster and global value chain research”, Institute of Development Studies, Vol. 120.
[59] ILO and OECD (2015), The Labour Share in G20 Economies, Report prepared for the G20 Employment Working Group, 26-27 February, http://www.oecd.org/g20/topics/employment-and-social-policy/The-Labour-Share-in-G20-Economies.pdf.
[30] IMF (2016), Global Trade: What's Behind the Global Slowdown, IMF, Washington DC.
[58] ITF (2017), “Managing the Transition to Driverless Road Freight Transport”, International Transport Forum Policy Papers, No. 32, OECD Publishing, Paris, http://dx.doi.org/10.1787/0f240722-en.
[14] Jacobs, J. (1969), The Economy of Cities, Vintage Books.
[3] Ketels, C. and S. Protsiv (2016), “European Cluster Panorama 2016”, European Cluster Observatory.
[42] Krugman, P. (1979), “Increasing Returns, Monopolistic Competition, and International Trade”, Journal of International Economics, Vol. 9/4, pp. 469-79.
[28] Los, B., M. Timmer and G. de Vries (2015), “How Global are Global Value Chains? A New Approach to Measure International Fragmentation”, Journal of Regional Science, Vol. 55, pp. 66-92.
[35] Los, B. and W. Chen (2016), “Global Value Chain Participation Indicators for European Regions”, Report for the OECD, No. December 2016.
[11] Marshall, A. (1890), Principles of Economics, MacMillan, London.
[44] Melitz, M. (2003), “The impact of trade on intra-industry reallocations and aggregate industry productivity”, Econometrica, Vol. 71/6, pp. 1695-1725.
[45] Melitz, M. and D. Trefler (2012), “Gains from trade when firms matter”, The Journal of Economic Perspectives, Vol. 26/2, pp. 91-118.
[23] Newcastle University (2015), Smart specialisation for regional innovation: WP5 regional report on Pirkanmaa (Tampere), Finland, http://www.regioconeixement.catedra.urv.cat/media/upload/domain_697/arxius/carpeta%20sense%20nom/Tampere%20Regional%20Report%20-%20full%20version%20(October%202015).docx.
[1] OECD (2007), Competitive Regional Clusters: National Policy Approaches, OECD Reviews of Regional Innovation, OECD Publishing, Paris, http://dx.doi.org/10.1787/9789264031838-en.
[15] OECD (2013), Innovation-driven Growth in Regions: The Role of Smart Specialisation, Preliminary report, OECD, http://www.oecd.org/sti/inno/smart-specialisation.pdf.
[52] OECD (2015), Data-Driven Innovation: Big Data for Growth and Well-Being, OECD Publishing, Paris, http://dx.doi.org/10.1787/9789264229358-en.
[22] OECD (2016), Resilient Cities, Preliminary Report, http://www.oecd.org/cfe/regional-policy/resilient-cities-report-preliminary-version.pdf.
[2] OECD (2016), OECD Regional Outlook 2016: Productive Regions for Inclusive Societies, OECD Publishing, Paris, http://dx.doi.org/10.1787/9789264260245-en.
[31] OECD (2016), “Global Value Chains and Trade in Value-Added: An Initial Assessment of the Impact on Jobs and Productivity”, OECD Trade Policy Papers, No. 190, OECD Publishing, Paris, http://dx.doi.org/10.1787/5jlvc7sb5s8w-en.
[29] OECD (2017), “The Future of Global Value Chains: "Business as Usual" or a "New Normal"?”, unpublished manuscript.
[55] OECD (2017), OECD Employment Outlook 2017, OECD Publishing, Paris, http://dx.doi.org/10.1787/empl_outlook-2017-en.
[46] OECD (2017), “How to make trade work for all”, in OECD Economic Outlook, Volume 2017 Issue 1, OECD Publishing, Paris, http://dx.doi.org/10.1787/eco_outlook-v2017-1-3-en.
[18] OECD (forthcoming), “Indicators for Resilient Cities”, OECD Regional Development Policy Working Papers.
[26] Porter, M. (1985), Competitive Advantage: Creating and Sustaining Superior Performance, Simon and Schuster.
[25] Rhee, Y. (1990), “The catalyst model of development: Lessons from Bangladesh's success with garment exports”, World Development, Vol. 18/2, pp. 333-346.
[41] Ricardo, D. (1817), On the Principles of Political Economy, and Taxation, London.
[13] Romer, P. (1986), “Increasing returns and long-run growth”, Journal of Political Economy, Vol. 94/5, pp. 1002-1037.
[27] Smith, A. (1776), An Inquiry into the Nature and Causes of the Wealth of Nations, Project Gutenberg.
[20] Statistics Finland (2015), Annual National Accounts, http://www.stat.fi/til/index_en.html.
[36] Thissen, M., M. Lankhuizen and B. Los (2017), Construction of a Time Series of Fine-Grained Detailed Nuts2 Regional Input-Output Tables for the EU embedded in a Global System of Country Tables, PBL Netherlands Environmental Assessment Agency, The Hague.
[34] UNCTAD (2013), Global Value Chains: Investment and Trade for Development, UNCTAD.
[56] Underhill, G. (2016), Industrial Crisis and the Open Economy: Politics, Global Trade and the Textile Industry in the Advanced Economies, Springer.
Notes
← 1. “Low‑income” and “low‑growth” regions have been defined as part of the EU Lagging Regions Initiative (European Commission, 2017[5]). Low‑income regions are those with less than 50% of the EU‑average per capita GDP in 2013 and are located in Bulgaria, Hungary, Poland and Romania. Low‑growth regions are those classified as “less‑developed” or “transition” regions, i.e. with less than 75% or 90% of EU‑average per capita GDP and with growth that was less than the EU average over the 2000‑13 period.
← 2. The labour share reported here is the so-called unadjusted labour share because it does not include income earned by the self-employed. Including the income earned by the self-employed increases the labour share by approximately eight percentage points (ILO and OECD, 2015[59]).
← 3. Though depletion of resources makes the benefits created through resource extraction temporary. See also “Not all gaps will close, but persistent and growing differences raise concerns” in Chapter 1.