This chapter looks at work carried out since the 2009 Stiglitz-Sen-Fitoussi Commission on going “Beyond GDP”. It argues that the 169 targets agreed by the international community on the Sustainable Development Goals are too many, and that countries have to select a set that corresponds to their priorities. It shows that growing inequality in income and wealth are global concerns and that, even in developed countries, data are often inadequate. It warns against over-reliance on broad averages, since these fail to reflect important inequalities across given groups (“horizontal inequalities”) and say nothing about how resources are shared and managed within households. The chapter argues that what matters are not just inequalities in outcomes but inequalities in people’s opportunity to achieve those outcomes, and that measuring this is possible. Other areas where more work is needed include subjective well-being and economic insecurity, which interact with social capital and trust, as well as sustainability across its social, economic and environmental dimensions.
Beyond GDP
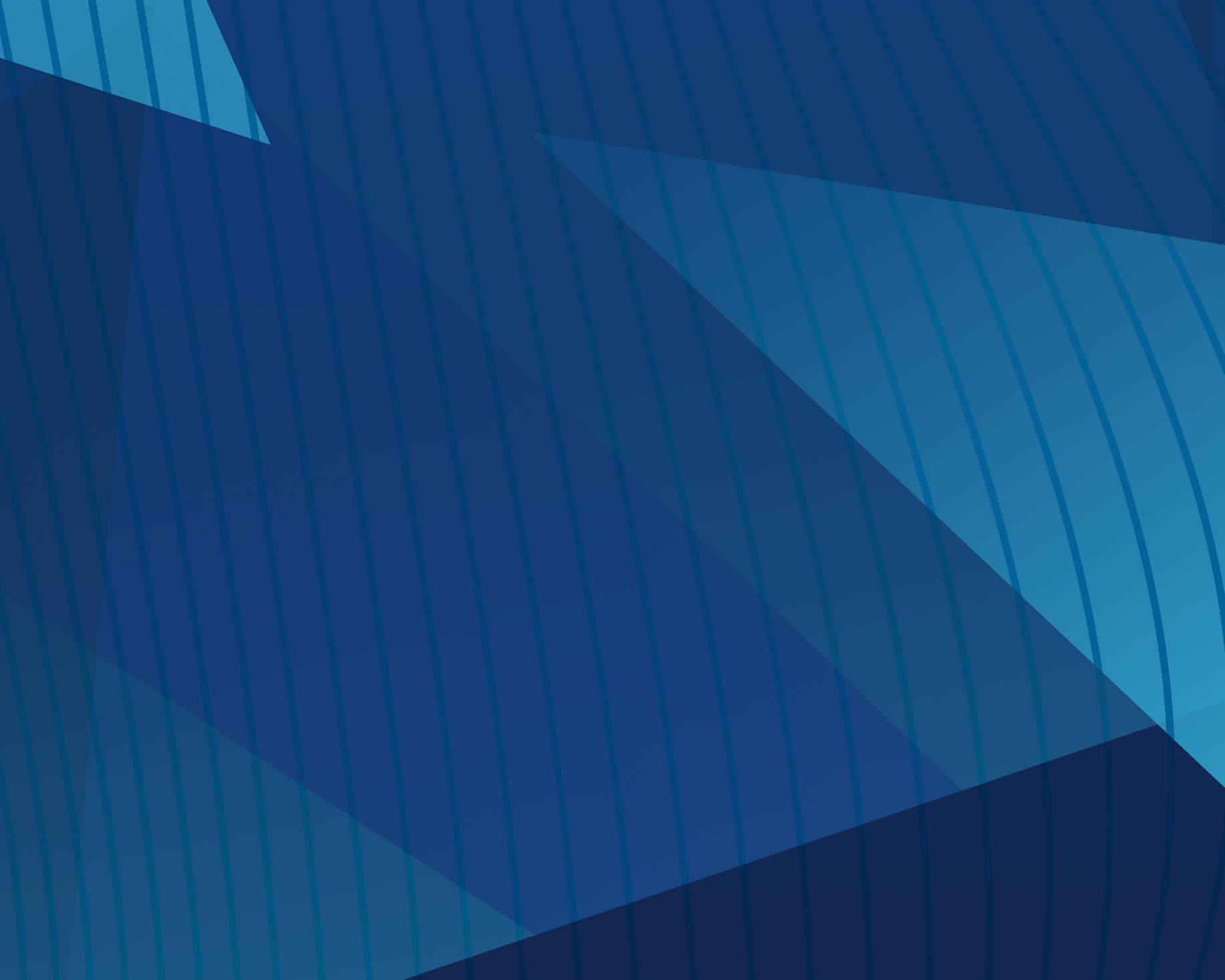
Chapter 3. The need to follow up on the Stiglitz-Sen-Fitoussi Commission
Abstract
The statistical data for Israel are supplied by and under the responsibility of the relevant Israeli authorities. The use of such data by the OECD is without prejudice to the status of the Golan Heights, East Jerusalem and Israeli settlements in the West Bank under the terms of international law.
3.1. Introduction
While much has been happening since the Stiglitz-Sen-Fitoussi Commission’s report in 2009 in terms of high-level national initiatives, statistical production and research, it was also clear that greater focus and more work were needed in a number of areas. Five years on, the decision was therefore taken to create a follow-up High-Level Expert Group (HLEG) hosted by the OECD to take stock of progress, to provide direction and maintain momentum, and to identify new areas requiring more work. The HLEG decided to focus on nine broad areas: the Sustainable Development Goals, including global and developing country perspectives; income and wealth inequalities; horizontal inequalities, i.e. inequalities among groups of people sharing the same characteristics; integrating information on economic inequalities in macro-economic statistics; inequalities of opportunities; subjective well-being; economic insecurity; sustainability and resilience; and trust. While authored chapters on each of these topics are included in the companion volume, we provide below our own perspective on these issues.
3.2. Sustainable Development Goals: How to measure progress in poorer countries
In Chapter 1, we noted that one of the major changes since the report of the Commission in 2009 was the agreement by the international community of a set of Sustainable Development Goals (SDGs), a global norm-setting process applying to developed as well as developing countries. These goals, endorsed by the UN General Assembly in 2015, expanded the range of goals well beyond the Millennium Development Goals that had been formulated in 2000 as aspirations by the development community to be achieved by 2015 (Table 3.1). The SDGs entail ensuring shared economic and social progress as well as environmental, social and economic sustainability.
Table 3.1. From the MDGs to the SDGs
Millennium Development Goals (MDGs) |
Sustainable Development Goals (SDGs) |
---|---|
1. Eradicate extreme poverty and hunger. 2. Achieve universal primary education. 3. Promote gender equality and empower. |
1. No poverty. 2. No hunger. 3. Good health and well-being. 4. Quality education. 5. Gender equality. 6. Clean water and sanitation. 7. Affordable and clean energy. 8. Decent work and economic growth. 9. Industry innovation and infrastructure. 10. Reduced inequalities. 11. Sustainable cities and communities. 12. Responsible consumption and production. 13. Climate action. 14. Life below water. 15. Life on land. 16. Peace, justice and strong institutions. 17. Partnership. |
The goals were increased from 8 to 17, with 169 associated targets. The goals focused attention of both policy-makers and citizens on issues deemed important by the international community. As the former UN Secretary Ban Ki-Moon said: “They [the MDGs] generated new and innovative partnerships, galvanized public opinion and showed the immense value of setting ambitious goals. It was hoped that the SDGs would perform a similar role” (United Nations, 2015).
The proliferation of goals reflected the inevitable tension between the pull to broaden and expand indicators for assessing and monitoring economic and social progress on the one hand, and on the other the imperative to keep a relatively small number of indicators at the “top level of the dashboard”, in order to facilitate national discourse and policy making. Of course, the more information is detailed and the greater data are disaggregated, the more complete the picture one has of what is going on. But limited human attention requires that indicators be streamlined, and this is especially so if the statistics are to influence the public debate. The Commission “squared the circle” by arguing for a dashboard of indicators. However, the 169 SDG policy targets, and 232 indicators for “global monitoring” agreed by the international community are clearly too many.
Focus is especially important for developing countries, where resources – including the human resources necessary to design and implement the policies required to achieve the goals and to develop the large number of indicators to monitor progress – are limited.
Given that different countries are in different circumstances, it is natural that they will and should focus on different objectives. Differences are likely to be especially significant between developed and developing countries. For example, while most of the former have managed to achieve relatively high rates of formal employment and low levels of extreme poverty, lack of productive employment and poverty are still of central concern to most developing countries. But there is a cost to having different goals pursued by different countries – it undermines inter-country comparability. Countries themselves need to be mindful of the benefits of comparability: to know how well one is doing, one wants to know how well other similarly situated countries are performing. Still, it is likely that many similarly situated countries will choose similar goals.
3.2.1. Metrics
Even when the set of goals is decided, the choice of metrics can make a difference – another of the messages of the Commission – and the metrics that developing countries might want to focus on may well differ from those of the more developed ones.
In the case of SDG 1 (“end poverty”), for example, the indicator agreed by the UN Statistical Commission for Target 1.1 (“By 2030, eradicate extreme poverty for all people everywhere”) is the number of people living below the USD 1.9 per person per day global poverty line.1 Most developed countries are likely to have met this target already: poverty generally means something markedly different in the developed world.2
Or consider SDG 10 (“reduce inequality”). The indicator chosen for Target 10.1 (“By 2030, progressively achieve and sustain income growth of the bottom 40 per cent of the population at a rate higher than the national average”) is the growth rate of household expenditure or income per capita among the bottom 40 per cent of the population. Jose Gabriel Palma of Cambridge University has argued that a more sensitive measure of income inequality, especially for emerging economies and developing countries, is the ratio of the income share of the top 10% of population divided by the share of the poorest 40% (Palma, 2016). He argues that the income share of the middle-class seems relatively similar across at least middle-income and developing countries, so that this ratio really identifies the differences across countries. Doyle and Stiglitz (2014) argued therefore that the Palma ratio might be the preferred measure of income inequality for the SDGs.
Measures, of course, embrace values and concerns. Target 10.1, advocated by the World Bank, is a more inclusive measure than extreme poverty, but is still in keeping with the Bank’s traditional focus on the lower part of the distribution.
3.2.2. Global Policy Issues
One important issue raised by Kanbur, Patel and Stiglitz in their chapter of the companion report relates to metrics for matters of global concern. Most importantly, we need to know what is happening to climate change (which requires measuring the global carbon emissions from the economic activities of a country, i.e. both those relating to the production within its borders and those associated with a country’s imports to satisfy its domestic demand). There is also a great deal of interest in knowing what is happening to global income inequality (the inequality that we would observe if we ranked all people in the world by their income, as if they were living in the same country), especially in assessing the offsetting effects of higher within-countries inequalities and lower income disparities across countries (Deaton, 2013; Milanovic, 2016). There is value in having National Statistical Offices collect data on a sufficiently comparable basis that we can make reasonable estimates of what is happening in both regards.
3.3. Inequalities in income and wealth
At the time of the Stiglitz, Sen and Fitoussi (2009) report, we were intensely aware that one of the reasons that GDP was not a good measure of economic and social performance was that it took no account of distribution: a society in which most people were doing poorly, but a few were doing very well was not, in a fundamental sense, a well-performing economy, even if GDP was increasing, possibly even rapidly. Indeed, that was the case for the United States and in most other advanced countries. Using GDP as a metric, the US economy seemed to be doing reasonably well. But looking at measures that took into account distribution provided a markedly different picture. The United States, as some commentators put it, was a great country in which to be born, if you knew you were going to be in the top 1%, or even better, in the top 0.1%; being born in the bottom 90% was a markedly different story.
In the years since the Stiglitz, Sen and Fitoussi report, the attention paid to income inequality has increased significantly, partly thanks to the availability of new data showing the long-term nature of this rise and the increasing share taken by the 1% (Piketty, 2014); and partly driven by the sheer size of the increase in income inequality that took place since the late 1970s. Comparative data analyses have also shown cross-country differences in the size of this increase in inequality, which suggests that country-specific factors, and in particular policies and institutions, matter considerably.
Income inequality, its causes, consequences and what should be done about it have thus become a focus of policy discussions around the world. This is a new and important development, which also reflects the contributions of several of the members of the HLEG (Fitoussi and Rosanvallon, 1996; Fitoussi and Stiglitz, 2013; López-Calva and Lustig, 2010; Bourguignon, 2012b; Stiglitz, 2012a; Piketty, 2014; Deaton, 2013; Atkinson, 2015) and international organisations (OECD, 2008, 2015; IMF, 2017; Ostry, Berg and Tsangarides, 2014). Research has highlighted a number of drivers of income inequality and of its increases over time, which include both changes in the structure of the economy (globalisation, skill-biased technological change, the more important role of the service sector, especially the financial sector, higher market power of firms in many sectors) and weaker bargaining power of workers, itself a function of some of the other changes noted. Changes in policy affect the distribution of both market and disposable income. These include changes in the rules governing labour markets, corporate governance, globalisation and anti-trust; changes in policies affecting the inter-generational transfer of advantage and disadvantage, such as inheritance taxes and public education; and policies affecting redistribution through taxes and transfers (Stiglitz, 2015; Inchauste and Lustig, 2017). In all cases, however, policies to reduce inequalities will require better data, and incorporating more systematically into policy deliberations an analysis of the effects of alternative policies on the distribution of income and wealth.
The Stiglitz, Sen and Fitoussi report emphasised not only the importance of inequalities in income and wealth, but also of every other aspect of well-being: health, education, political voice, insecurity, access to justice, opportunity. Many of these inequality indicators are correlated, with the same households or individuals experiencing disadvantage in many of these dimensions. The report argued that one should focus on the household (or even better, the individual) as the unit of analysis, looking at all the dimensions that affect well-being at the same time. Dashboards need to take into account what is happening, say, to average health outcomes, but also who is in good or bad health. The Case-Deaton study (2015) referred to earlier highlights this issue: even if longevity is increasing and mortality decreasing on average, just the opposite is happening among American whites who have only a high school education, and this is the same group that has seen their incomes plummet. (The same issues arise in making cross-country comparisons.)
3.3.1. Growing inequality in most advanced countries
For more than 30 years, while the income gap between major emerging markets (e.g. China, India and Brazil) and advanced countries was narrowing, that within most, but not all, advanced countries was rising. According to OECD data, 18 out of 23 OECD countries experienced a significant increase in income inequality between the mid-1980s and around 2013 (OECD, 2015a, p. 24). In some cases, the increase in inequalities in market income was offset by government tax and transfer programmes. In others, most notably the United States, it was not, resulting in statistics that could only be called alarming. By 2017, the median family income adjusted for inflation was barely above the level a quarter century earlier, real wages were barely above the level attained 60 years earlier, and the real median income of a full-time male worker was lower than 41 years previously.
While the United States was the most extreme case, others were not far behind. OECD studies showed that there were large increases in income inequalities even in countries like Sweden that had earlier established a reputation for egalitarianism, though even with their large increases in inequality, their income inequality was far lower than for others. The rise of income inequality was also important in most emerging countries.
3.3.2. A global perspective
Branko Milanovic combined what was happening within and between countries into a global picture (Milanovic, 2016). A single diagram, reprinted below (Figure 3.1), summarises much of the story. Two groups have been doing very well, the global top (point D in diagram), i.e. the 1%, and the emerging middle-class in China and India, around the 50 percentile (point C). These two groups have been the main beneficiaries of globalisation. There are two groups, conversely, who did not do well: those at the bottom, e.g. people in conflict-affect areas or subsistence farmers in developing countries (point A), hurt in part by European and American agricultural subsidies; and the working/middle-classes in Europe and America (point B), especially those with limited education.
Figure 3.1. Cumulative gains in real income growth around the world, 1988-2008
Source: Milanovic, B. (2016), Global Inequality: A New Approach for the Age of Globalization, Harvard University Press, Cambridge, MA.
Using the standard metrics for measuring inequality, the Gini coefficient (see the discussion below), the effect of reduced cross-country inequality and increased within-country inequality largely offset each other.3 But summarising Figure 3.1 into a single number such as the Gini coefficient misses much of the story – the discontent among the middle-class in the advanced countries and among those at the bottom of the income pyramid, and the outsized gains of the very wealthy.
Milanovic’s data may be affected by a range of assumptions and measurement problems (Kharas and Seidel, 2018)4, and do not give a full picture of the extent of variability in levels and changes in income inequality across countries. Income inequality (by most measures) is not only higher in the United States than in other advanced countries, it has also increased the most. In several OECD countries, the increase in inequality was concentrated in the early 1990s, while in Germany the increase started in the early 2000s and in France in the late 2000s (Bourguignon, 2012b). Some countries in Latin America even saw income inequality reduced, albeit from a high level. In some countries like China, a massive reduction in poverty has been achieved alongside higher income inequality – with the rich experiencing greater income gains than the poor.
3.3.3. Some Broad Data Issues
Comparative data on income distribution for OECD countries5 exist today but not when the long-term “wave” of higher inequality started (in the United Kingdom, the United States, New Zealand) in the mid-1970s and early 1980s. Had these data been available back then, policy-makers and concerned citizens could have noticed the trend and might have taken action to counter it. While the increase in economic inequality was noted earlier in individual countries, this was often dismissed as reflecting country specificities. In reality, the common pattern to higher economic inequalities became more evident and common across countries, partly the consequence of the same set of policies that had been introduced in the United Kingdom and the United States in the late 1970s and early 1980s being adopted elsewhere. Income inequality became an area of concern long after the damage was done.6
Data on economic inequalities are today often weaker in those countries where they are most needed, for instance in those emerging and developing countries that are experiencing strong economic growth unequally shared, without the cushion provided by adequate social protection programmes. As documented by Nora Lustig in the companion volume, while various data on economic inequalities for these countries exist, they are often based on estimates made up by researchers. Even when good quality official micro data exist (e.g. China), access to them is often out of reach for both researchers and international organisations. Also, most data on economic inequality in developing and emerging countries are based on surveys of consumption expenditures rather than income. When countries grow rapidly, and household savings increase (for those households that benefit from growth), consumption inequalities will increase by less than income inequalities. Similarly, as these countries undergo a process of urbanisation and demographic transition, growth of household income and consumption per capita may exceed growth of household income and consumption per consumption unit, due to lower household size. On both accounts, policy-makers in these countries may be under-estimating the increase in economic inequalities because of inadequate data.
Even in rich countries, out-of-date measures of income inequalities are a problem, with such data typically lagging by years the most recent data on GDP. Data on income inequality have little traction in policy discussions simply because, by the time the data become available, they represent “water under the bridge”, and often reflect policy decisions taken by previous administrations.
Limits to the metrics used in national official statistics on economic inequality can bias policy discussions, as some examples illustrate. While, for purposes of measuring inequality, the broader metric of income inequality is that of adjusted disposable income (which includes the individual services provided for free, or at subsidised prices, by governments to households), most of the available measures of income inequality are typically based on cash income. While there are methodological challenges in measuring in-kind services, their exclusion from the income metric often allows policy-makers to get away with the “fiction” that tax cuts improve people’s economic well-being even when they are offset by cuts in valued public services. Similarly, some developing countries have devoted significant expenditures to help the poor through better education and health care programmes. These programmes improve the well-being of poor children, but do not show up as an increase of the cash income of beneficiaries.7 Over time, they should lead to higher incomes, moving people out of poverty, but these income effects take time to show up.8
Statistics on the distribution of household economic resources remain limited in other respects.9 First, traditional surveys on the distribution of household income have come under increasing scrutiny because of their failure to capture development at the top-end of the distribution. Other sources (mainly tax records) have been increasingly used, in particular following the work by Atkinson and Piketty (2007) and their co-authors. Use of administrative data sets has prompted questions about the role of each source, their strengths and limitations, and possible ways to integrate them. Tax records typically don’t have adequate representation of those at the bottom end of the distribution; while there is some presumption that the incomes of those at the top are understated, in some cases as a result of employing legal loopholes, in other cases as a result of more questionable practices bordering on tax evasion. In some countries, the rich shelter much of their income through corporations, and so income that rightly should be attributed to them is not. In most countries, capital gains are reported in tax declarations (if reported at all) only when an asset is sold.10 Indeed, when capital gains are given tax preferences (as is often the case), rich individuals have found easy ways of converting what otherwise would be a dividend into a capital gain. At the other end of the distribution, even surveys, which do a better job than administrative data in picking up incomes at the very bottom, are inadequate (Atkinson, 2016). Surveys miss the homeless, people in institutions and jails or those with no permanent address.11
Second, there are typically large differences in levels, and sometimes changes, in income inequalities, on one side, and inequalities in consumption expenditures and wealth, on the other. Administrative data can provide good income measures, subject to the limitations discussed above, but we have to rely on surveys when it comes to measuring consumption expenditures. Measuring consumption, rather than income, has both advantages and drawbacks. On the one hand, individuals may be better able to report what they consumed (through diaries) than the income they have received: “net income”, for example for a small shopkeeper who does not keep books, is an abstract concept, and reported numbers may be inaccurate. On the other hand, individual-level data on consumption have their own limits: they are burdensome to report for survey participants, implying that results may miss the very poor; they may omit expenditures on durable goods or, when they include them (often in a separate module), record them as consumption expenditure flows that should, in theory, be distributed over several years.12 These data are also affected by their own measurement errors, due to factors such as the length of the recall period, the number of consumption items listed in the diary, and whether participants record their consumption themselves or are interviewed.13 Especially troublesome is the fact that we may have data only for income inequality in some countries and only for consumption inequality in some others, making cross-country comparisons difficult.
Third, the treatment of government-provided goods and services is especially problematic, as these items may represent a large fraction of the “effective consumption” of low-income individuals. Ignoring such goods and services may give a misleading view of levels and changes in both economic inequality and average living standards. The problem (to which the 2009 Commission report called attention) is that because these are not market transactions, it is hard to value them, and in some cases, hard even to identify who are the recipients. Medical services are especially difficult to value for non-insured people; but even for those covered by health insurance, valuing the transactions at market prices is unsatisfactory, since market prices are generally distorted. In many countries, such as the United States, the cost of providing health care services has risen much faster than the cost of living in general. The result is that, even when nominal health spending is increasing faster than the CPI (the overall measure of cost of living), real health services may be actually falling if one used a health-care specific price index. This is important: Burkhauser, Larrimore and Simon (2012) noted, using income metrics in nominal terms, that inequality in living standards in the United States was increasing by less than claimed once one takes into account the large increase in (nominal) health care expenditures. However, accounting for the higher increase in health-care prices would lead to the opposite conclusion.
Fourth, we still lack adequate data on the distribution of household wealth. This lack is especially troublesome at a time when many rich countries have been reducing taxes on wealth holdings, capital income, capital gains and inheritances (OECD, 2018b). The regressive implications of these policies are not given adequate weight in policy discussions, partly because adequate data on wealth distribution are not available.14 In addition to the problems described earlier on the measurement of income, there are two more critical limitations in the case of household wealth data. First, in some countries, significant amounts of the wealth are held abroad, including in tax heavens. While Zucman (2015) has shown the large magnitude of this hidden wealth, incorporating it into countries’ wealth distribution data is a huge challenge. Second, in some countries wealthy people may hold significant fractions of their wealth in trusts and foundations, the beneficial ownership of which is often not transparent. Since the fraction of the wealth so held can vary markedly across countries, this makes it difficult to make cross-country comparisons of wealth inequality. Similarly, the fraction of wealth so held can vary markedly over time, especially with changes in regulations and tax laws.15
Last, micro-statistics on household income, consumption and wealth (even when they exist) are only rarely collectedly in a joint way, with information of each component available for each household.16 A stronger correlation between different types of economic resources would imply higher economic inequality even when the (marginal) distribution of each type is unchanged. As noted earlier, the natural unit of analysis is the household, or the individual, and those at the bottom typically suffer deprivations in all dimensions. Evidence on the joint distribution of income, consumption and wealth for the United States suggests that their correlation has increased over time, meaning that “true” economic inequality has increased faster than when looking at each type of economic resource separately (Fisher et al., 2016). For most other countries, we simply don’t know the magnitudes of the underestimate. Recent OECD work on the joint distribution of income and wealth shows for instance that more than 40% people in OECD countries lack the liquid financial assets that would prevent them from falling into poverty if they had to forego three months of income, indicating widespread vulnerability to unforeseen economic shocks (Balestra and Tonkin, 2018).
3.3.4. Technical issues
Beyond these issues, a host of important technical issues have to be addressed when comparing economic inequality both across countries and over time. There are, for instance, many issues affecting the comparability of data, which in turn affect the ability to make cross-country comparisons. There are also differences in the nature of datasets between advanced and developing countries, and in the extent to which the data provided by respondents correspond to appropriate definitions of income or consumption. For advanced economies, economic inequality is typically measured based on equivalised income (where adjustments are made for family size and sometimes for the age of each household member) while for other countries consumption or income per capita is more commonly used. Large differences across countries and over time in household size raise questions about cross-country comparisons of income inequality. In some developing countries, the very notion of household (people sharing the same roof and meals) may be difficult to define and measure.
While in principle the income variable that should be the focus of attention when analysing inequalities is disposable income (what individuals can spend, after paying their direct taxes and receiving any current transfers) the precise income concept used by respondents in most Latin American countries (where surveys have traditionally measured income, rather than consumption) is often not clear. Likewise, while international standards in this field (UNECE, 2011) argue that both income and consumption should include goods produced within the household for their own use (services produced within the household, such as child care, are excluded due to the difficulties in measuring them) as well as imputed rents of owner-occupied housing (the rent that individuals would have had to pay if they were renting their house), in practice this is not in general the case, even among industrialised countries.17
Similarly, the impacts of consumption taxes and subsidies on household economic resources are typically neglected. As shown in the chapter by Nora Lustig in the accompanying volume, while it is generally acknowledged that household consumption possibilities are reduced/increased by consumption taxes/production subsidies passed on to the prices that households pay for the goods and services they purchase, taking this impact into account has not been part of the conventions typically used for analysing disparities in households’ economic well-being and for analysing the redistributive impacts of government taxes and spending.18 Fiscal incidence studies that take these taxes into consideration highlight significant distributive effects (Lustig, 2018).
Secondary databases differ too on whether adjustments (and which ones) are made to the underlying micro data in order to correct for under-reporting, to eliminate outliers, or to address missing responses on specific items. This situation results in inconsistencies between datasets, meaning that different datasets may produce different estimates about the level of inequality even when the underlying data source is the same. In some cases, these problems may be so large that ascertaining whether there has been convergence in levels of inequality among countries may simply depend on which secondary dataset is used.
3.3.5. Describing income inequality: the choice of indicator
Presenting (and summarising) data on inequalities in income also gives rise to a number of problems. The usual summary statistic is the Gini coefficient, an index which varies between zero (in the case of perfect equality. i.e. everyone has the same income) and 1 (in the case of perfect inequality, i.e. all the income accrues to a single person). This index is computed based on a (Lorenz) curve (Figure 3.2) that plots cumulative shares of the population against the cumulative shares of the income received (e.g. people in the bottom 10% of the population may receive 5% of total income, people in the bottom 20% may receive 12% of total income, etc.). The Gini coefficient is (twice) the area between the Lorenz curve and the diagonal, and corresponds to the average income difference between all pairs of individuals in the sample.
Figure 3.2. The Lorenz curve for income
However, only when the curve for one year is below that for another year at all points in the distribution can it be said that a higher Gini coefficient indicates an unambiguous increase in inequality, that is, all inequality-averse societies would prefer one curve to the other. Whenever these curves “cross” each other, the assessment of changes in income inequality depends on the “weight” attributed to different portions of the curve. For instance, some societies might be more concerned with the hollowing out of the middle-class, others to the 1% seizing such a large fraction of the nation’s economic pie, others to the depth of poverty. Even when these curves do not cross, for policy purposes it is important to know what is happening to each part of the income distribution – to those at the bottom (living in poverty), to those in the middle (the middle-class), and to those at the higher end (the top 1%). Inequality measures capturing developments at different points of the distribution should be routinely reported alongside measures summarising the overall distribution.
Sir Tony Atkinson introduced in 1970 a welfare-based measure that assessed, given society’s concern about inequality, the loss in societal well-being that is due to income inequality (Atkinson, 1970). This measure asks how much income people would be willing to give up if this could eliminate all inequality. With reasonable assumptions on the degree of “inequality aversion”, the welfare-loss turned out to be quite large (up to a third or more of their average income). But even this measure may underestimate the “price of inequality”, for it focuses on eliminating all inequality. The relevant question, in most cases, is how much a country would be willing to pay to get rid of some inequality, i.e. as elsewhere in economics one wants a marginal measure. Stiglitz (2009) developed one such measure, showing that it typically is much larger than the Atkinson measure; but unlike the Atkinson measure, applications have been fewer to date.
3.3.6. Using inequality metrics in policy design
In many areas of regulation, e.g. those concerning the environment and safety, it is common to require a cost-benefit analysis, to force policy-makers to assess both the benefits of the regulations and their costs. So too, it should be routine to require for any major policy an assessment of its distributional consequences, as the IMF has now started doing.19 It is conceivable that, if such procedures had been routine, there might not have been the large cuts in capital gains taxes in the United States under President Clinton, which contributed so greatly to the growth in inequality; and if there had been a cut, it might well have been designed differently.20 It is also possible that such assessments would have led to some second thoughts about the austerity policies imposed on some European countries following the financial crisis. Similarly, it would be advisable to assess the distributional impacts of changes in labour market regulations. For example, changing a labour market regulation without providing adequate social protection and active labour markets programmes might generate adverse impacts on inequality out of balance with any positive efficiency gains.
3.4. Macro- and micro-level statistics on household economic resources
The system of national accounts originated out of the need to have the information necessary to manage the economy following the Great Depression, and based on Keynes’ notion that governments could use monetary and fiscal policy to manage the economy. Both the impact of income distribution on macro-economics and the impact of macro-economic policies on distribution were often not well recognised, and even less frequently taken into account in policy discussions, especially over the past four decades.21
This has changed today. A range of studies since the crisis (mainly undertaken by researchers at the IMF and OECD), and based on cross-country datasets combining data on GDP growth and income inequality, have suggested that higher income inequalities may have reduced GDP growth (Ostry, Berg and Tsangarides, 2014; Cingano, 2014). Such negative association has some plausibility in the aftermath of the 2008 crisis, when lack of demand was a key constraint on growth, due to different propensities to spend by people at different levels of the distribution.22 But there are obviously huge data problems for any research that combines micro and macro data that are measured based on different concepts and that exhibit large differences in how they change over the same period. The difficulties are even larger when it comes to assessing the direction of causality between the two and the role of other factors in explaining their association.
At the same time, the unorthodox monetary policies (quantitative easing) used by central banks to support the economy in the aftermath of the crisis had large (adverse) distributional impacts. Retired individuals who held safe, short and medium term bonds saw their incomes hurt because of low interest rates, while wealthy individuals who owned a disproportionately large share of equities saw the value of their stocks soar as a result of markets’ positive response to quantitative easing. This distributive effect, largely or more than offsetting the distributive benefits from higher employment which had been one of the objectives of these monetary policies, may have contributed to limit the effectiveness of these policies in sustaining growth and contributed to a sense of unfairness in the design of public policy, fuelling people’s mistrust in government.
It is thus clear that macro-economic and distributional data need to be integrated. Macro- and micro-statistics on household economic well-being have historically developed on parallel tracks, sometimes leading to very different conclusions on how household living standards had, on average, changed over time. Macro- and micro-consistency is obviously important, and the disparity in numbers should call attention to the deficiencies in each source. Scholars have been aware of the large discrepancies for some time (Altimir, 1987; Deaton, 2005; Anand, Segal and Stiglitz, 2010), and suggested ideas to explain the reasons behind them. However, co-ordinated action at the international level to put macro- and micro-data on household economic resources on a consistent framework started only in 2011, when the OECD and Eurostat carried out a feasibility study on compiling distributional measures of household income, consumption and saving within the framework of national accounts on the basis of micro data (see Box 5.1 in the companion volume). By working to obtain consistency, we may get a far more accurate picture of what is going on. Research reported by Alvaredo et al. in the accompanying volume highlights the progress that has been achieved in harmonising the two sets of data, through harmonised definitions and methods, and in using national accounts, surveys and tax data together with other data sources such as rankings provided by “rich lists” compiled by the press.
While most analyses of the rise in income inequalities are based on summary inequality measures based on surveys, assessment of the pace of income growth for people at different points in the distribution have to contend with the large (and growing) differences in measured income growth that are reported in micro and macro sources. All attempts to bring together information on both sources have to confront a range of controversial methodological issues (described by Alvaredo et al.) and to make choices that are open to disagreements. That said, when these choices and assumptions are made, these estimates highlight some of the markedly different patterns across the world. For instance, the estimates reported by Alvaredo et al., which combine data from tax, survey and macro-data sources, show that between 1978 and 2015 national income per adult increased by over 800% in China, by around 60% in the United States, and by around 40% in France. In China, the average income of the bottom 50% also grew strongly, by around 400%, but less than the average, hence inequality increased. In contrast, the bottom 50% of the population in the United States experienced a small (-1%) income drop. In France, the income of adults in the bottom half of the distribution increased at the same pace as the average, and income inequality was broadly stable – with only small increases in the second half of the 2000s.
The integration of macro and micro statistics on household income, in ways that are consistent with macro-economic totals for GDP and its components, is necessary to reach firmer conclusions about the relationships between macro-economic activity, policy and distribution. An understanding of these relationships is important not just because macro-economic policy may have unintended distributional consequences, but also because economic inequalities matter for the transmission of monetary and fiscal policies, and for assessing risks of default linked to changes in asset prices. Macro-economists simply cannot ignore issues of distribution in the way they did in the past.
3.5. Horizontal inequalities
Vertical inequalities such as those discussed above describe the extent of differences between, say, the rich and the poor. Horizontal inequalities describe differences among roughly similarly situated individuals or groups, e.g. between men and woman, across races or ethnic lines, or within the family. Horizontal inequalities are often associated with discrimination, which has well-discussed moral, social, political and economic consequences. Horizontal (i.e. group) inequalities can lead to political instability when the groups on the losing side manage to build coalitions based on their shared identities (Doyle and Stiglitz, 2014). It is possible, even likely, that had more attention been paid to horizontal inequalities, conflicts based on ethnic or religious groups might have been avoided or at least lessened. At the same time, data showing the magnitude of horizontal inequalities can confirm existing beliefs about discrimination, thereby heightening tensions.
Some types of horizontal inequalities are more difficult to capture than others. Traditional settlement countries have long-established data collection that look at race and ethnicity, while in many European countries (those scarred by the experience of genocide and discrimination during WWII, or those that only recently experienced large-scale immigration) such types of data collection are either explicitly forbidden or still under-developed. A case can be made that, by not collecting these data, these countries are blinding themselves to the most severe forms of discrimination.
Some of the criteria for comparing horizontal inequalities across countries also lack well-established statistical conventions and definitions. An example is provided by disability status where, despite decade-long discussions, no generally accepted definition applied across official surveys exists yet. In other cases, no statistical criteria exist simply because these types of horizontal inequalities (for example, those linked to sexual orientation) have only recently entered public discussions: it is important that such definitions are agreed and implemented in the context of population censuses.
Other types of horizontal inequalities are better understood but raise specific issues in terms of measurement. In particular, focusing on household economic resources doesn’t tell us how economic resources are distributed within the family or household, and whether there are systematic gender biases. We know that there are inequalities in the distribution of resources within families, especially in poor societies where girls are less likely to receive education, or even food or health care, than their male siblings. In more developed countries, we also know that who in the family receives the welfare payments affects how these benefits are shared within the family (Woolley, 2004; Browning, Chiappori and Weiss, 2014).
Deere, Kanbur and Stewart, in Chapter 4 of the accompanying volume, argue that inequality is underestimated when intra-household inequality is not taken into account (see also Deaton, 1997). However, as we have noted, gathering the requisite data to assess intra-household distribution is not straightforward. Indirect indicators such as marital regimes, or information on the weight or stunting of different household members, may provide information on within-household inequalities in some contexts. In most circumstances, however, measuring intra-household inequalities will require distinguishing between those income streams that are paid to each household member (e.g. earnings) and those that accrue jointly to all (e.g. returns on family assets), who in the family takes the most important financial decisions, or the part of individual income that is not shared with other members. Collecting information on both individual and household economic resources in the context of the same survey would allow developing better (if still imperfect) measures of gender inequalities, and help to target a range of policies (e.g. whether welfare payments are paid to the male of female partner in a couple) to reduce them.
3.6. Inequality of opportunity
Inequalities in the distribution of household economic resources and other individual characteristics are not the only type of inequalities that matter to people and communities. In fact, many observers claim that what matters are not inequalities of outcomes but inequalities of opportunity. In a fair society, everyone should have equal chances to achieve good outcomes regardless of the circumstances of his or her birth. Prominent politicians have claimed, for instance, that while the United States has more inequality of incomes than other countries, it has more equality of opportunity. This is an empirical claim, one that needs to be supported. To ascertain the answer to that question, one needs a conceptual framework – how should equality of opportunity be defined – as well as metrics and data with which to make the assessment.
Inequality of opportunity may be defined as the differences in the set of options available to individuals and/or families in different circumstances. Thus, an important strand in the economics literature attempts to distinguish between an individual’s circumstances and efforts. Equality of opportunity, in this approach, means that individuals exerting the same levels of effort should have the same outcomes (or the same probability distribution of outcomes) regardless of their circumstances.23 In particular, it would mean that individuals born into poor families who exert a given level of effort would have as great a chance in succeeding in life as those born to a rich parent exerting the same level of effort.
Unfortunately, as shown by Bourguignon in Chapter 5 of the accompanying volume, we typically do not observe effort. What this means in practice is that differences in outcomes that are not explained by observable circumstances are attributed to effort. If these circumstances explain only a small fraction of outcomes, there is a presumption that there is a high level of equality of opportunity. In fact, if differences in circumstances shift significantly the probability distribution of outcomes for a given level of effort, then it is not possible to disentangle what is due to one and what is due to the other. Moreover, our ability and willingness to exert effort may itself be affected by the circumstances of birth. So the conceptual distinction between circumstances and efforts is, at best, blurred.
3.6.1. Why do we care?
People care about equality of opportunity because it is unfair, because it is inefficient, or because of both. Most people feel that children are not responsible for the circumstances of their birth, and many would find it deeply unfair if a child’s life chances were found to be determined by those circumstances. Inequality of opportunity is economically inefficient because it generates disincentives, undermines morale and is associated with a misallocation of resources. Individuals who perceive that outcomes are largely predetermined by the circumstances of their birth have little incentive to exert effort. A perception that the economic system is rigged, that those born at the top will be the winners, will also lower morale. (This is sometimes referred to as the fairness-efficiency wage effect). If part of the inequality of opportunity is associated with a lack of access to education or other resources, then it implies that those at the bottom of the scale will underinvest in it. All of these factors imply that inequality of opportunities leads to a less efficient and a more unequal society.
Indeed, inequalities of opportunity will lead to the perpetuation of inequalities of outcomes. Most of the inequalities in income and wealth of the present generations (which are an essential determinant of the set of opportunities of their children) transfer themselves to the next generation. In turn, inequalities of the latter generation become inequality of opportunities of the following generation. Thus greater inequalities in one generation are translated to the next. The process is cumulative. In the words of Alan Krueger: “the rise in inequality in the United States over the last three decades has reached the point that inequality in incomes is causing an unhealthy division in opportunities, and is a threat to economic growth. Restoring a greater degree of fairness to the US job market would be good for businesses, good for the economy, and good for the country”.24
Corak (2013) documented the systematic relationship between inequalities of opportunity and inequalities of outcomes.25 He showed that societies with greater inequality of outcomes systematically had lower levels of economic mobility. This is reflected, for instance, in data suggesting that the children of rich Americans who do poorly in school wind up having higher incomes than children of poor Americans who do well in school (Bartik and Hershbein, 2018). It is not effort (or even intelligence) that determines outcomes so much as the circumstances of birth.26
One of the important implications of this analysis is that, while conceptually clear, the practical demarcation between equalities of opportunity and equality of outcomes is fuzzy. Fortunately, as the discussion below highlights, statistics already available provide some guidance on policies that could increase equality of opportunity.
3.6.2. Metrics
While the Commission in 2009 emphasised the difficulties in measuring inequalities of income and wealth, those presented by inequality of opportunity are far greater. A standard, roughshod approach is simply to measure the correlation between the earnings of a child and that of his or her parents (typically the father) at the same age (Corak, 2013). Equality of opportunity is then measured by the size of the correlation between the two. When on average there is no correlation, earnings mobility across generations is high, and it is said that there is a high level of equality of opportunity. Knowing at least one key circumstance – the parent’s earnings – provides in this case no information about the child’s prospects. In reality, in many other countries (OECD, 2018c), the correlation is high.
But this approach does not give us the kind of information that we would need to identify the source of the failure in equality of opportunity. A society could be marked by poverty traps, even if on average there is a reasonable level of mobility. An often-used approach looks at the mobility matrix, which describes, say, the probability for someone whose parents are in the middle-income deciles of moving up or down to the top or bottom quintile. Such a mobility matrix can show the presence of a poverty trap, where someone in a low income decile has a small probability of significant upward mobility, even if someone in a middle-decile has more mobility.
With sufficient data, one can refine the correlation analysis described above, asking what is the correlation between the parent’s income (and education) and that of the child with parents in the bottom, middle, or top of the income distribution.
One of the key problems with implementing these ideas is the absence of data, and this is an especially important limitation if one wants to ascertain long-run trends. Ideally, we would like to know the relationship between the lifetime income of somebody today and that of his or her parents, and that between the parents and the grandparents, requiring data on income stretching back decades in time.27 With less data, one can ascertain the income of an individual who is, say, 40 with that of his parents when they were 40. But if the profile of earnings is changing over time, and in ways that differ at different income levels, correlations between children’ outcomes and parental characteristics will become less informative.
Of course, inter-generational advantages and disadvantages may be transmitted not just by income, but by a broader set of circumstances, and most importantly by education.
A shortcut to looking over long time-periods is to look at indicators of future performance: for example, performance in students’ test scores, such as those administered under the OECD PISA programme to 15 year-old students. Through these data, one can ask whether there are circumstances of the child, such as the education of his or her parents, the language spoken at home, or the number of books available, that significantly affect his or her performance in school. The answer to that question is that there are, and that the socio-economic conditions of households account for a significant part of the competencies gained at school by students (OECD, 2013b). Other studies show that childhood programmes, the health of the mother and exposure to adverse environmental conditions during pregnancy can have significant effects on how children develop (Currie, 2009).
3.6.3. Policy
While the available evidence shows that inequalities in child outcomes (e.g. among children with parents at different levels of education and income) are large and, in many cases, increasing over time, this evidence is limited in terms of both the outcomes considered and the age of the child. Were such evidence available, it might be possible to intervene earlier, and avoid more costly remedial interventions at later ages. Mounting evidence on these inequalities, especially for children born of parents in the bottom of the distribution, has led to a surge of interest around the world in early childhood education (Heckman and Masterov, 2004).
As individuals move through life, they experience a variety of shocks (sickness, layoffs, etc.). The ability to respond to such shocks – as well as the probability of experiencing such a shock, its depth and duration – are, to a large extent, determined by circumstances. This is probably the main source of inequality of opportunity appearing during adult life. The availability of data on these circumstances would help in evaluating the role of social policies in neutralising the long-run effects of such shocks.
While our discussion has focused on inequalities of opportunity in terms of income and wealth, just as important is the opportunity to live a long and healthy life. Especially in countries where there is inadequate public provision of health care services, a child born of poor parents faces a greater prospect of a shorter and a less healthy life. Health and economic outcomes are, of course, deeply inter-related, with low income contributing to, and resulting from, poor health. Just as there is inter-generational transmission of economic advantages and disadvantages, the same applies to health.
Gender is a key circumstance shaping outcome inequalities, and many of the indicators conventionally used to chart gender inequalities, such as earnings differentials, point to a progressive, albeit slow, reduction of these inequalities, at least in OECD countries. This conclusion is however less clear-cut when controlling for the many characteristics influencing earnings (e.g. education, job experience). Figure 3.3 (drawn from Chapter 5 by Bourguignon in the companion volume, itself sourced from Weichselbaumer and Winter-Ebmer, 2005) shows that when some of these other characteristics are controlled for, there is little or no reduction of women’s earnings gap (the continuous line in Figure 3.3). In other terms, a circumstance of birth – gender – remains a critical determinant of outcomes when controlling for observable aspects of effort such as education. There is no equality of opportunity, as the economic system treats differently a man and a woman with the same characteristics. This, in turn, implies that policies that are only aimed at raising women’s participation in school and the labour market are failing to address enduring wage discrimination.
Figure 3.3. Gender earnings gap and adjusted earnings gap in a meta-analysis of the literature
Source: Weichselbaumer, D. and R. Winter-Ebmer (2005), “A Meta-analysis of the international gender wage gap”, The Journal of Economic Surveys, Vol. 19(3), pp. 479-511.
Nothing is more important for our sense of a fair society than ensuring equality of opportunity, yet in no domain is the gap between the data we need and the data we have greater. Closing this gap will require persistent, concerted and co-ordinated action: we need standardised data sets over long periods of time.
3.7. Subjective well-being
The objective of economic and social progress is to increase people’s well-being. Who knows better than people themselves how well off they are and what affects the most their own well-being? Money isn’t everything in life, and just because an individual is richer doesn’t mean he is happier or better off.
These ideas seem almost obvious, but they suggest an approach for looking “Beyond GDP” to assess how well people and society are doing. Is GDP growth increasing the perceived (or what has come to be called subjective) well-being of most citizens? As obvious as it may seem, it is only recently that economists, working with psychologists, have attempted to pursue this approach to the assessment of economic performance and social progress. A major stimulus to this work, and to the inclusion of subjective well-being questions in official surveys, has been provided by the analysis in the 2009 Commission report and its recommendation to Statistical Offices to include such questions in their surveys. The Commission report highlighted the potential of this approach. Subjective well-being provides information that is not reflected in conventional economic statistics, although, conversely, conventional economic statistics may also provide information that is not captured by subjective well-being data.
In the words of Stone and Krueger in Chapter 7 in the companion volume: “Measures of subjective well-being... ask individuals to self-report ratings of aspects of their lives, including satisfaction with their life as a whole, their feelings at a particular moment, or the extent to which they feel that their lives have meaning or purpose. These measures focus on what people believe and report feeling, not their objective conditions”.
A case in point that has attracted some attention was the sharp decline in life evaluation measures in several Arab countries in the years preceding the Arab Spring, despite strong GDP growth and improvements in health and education experienced in these countries (Figure 3.4). Other examples, quoted by Stone and Krueger in their chapter, are the findings that life evaluation is a stronger predictor of electoral results than conventional economic measures, and that the losses in average life-evaluation associated with a decline in GDP are twice as high as the gains from a GDP increase of similar size, a result that is consistent with standard results in behavioural economics on “loss aversion” (Kahneman and Tversky, 1984).
Figure 3.4. Trends in subjective well-being in Egypt
Note: Subjective well-being data are from the Gallup World Poll. GDP per capita estimates (at purchasing power parities) are from the IMF World Economic Outlook database.
Source: Reproduced from OECD (2013c), OECD Guidelines on Measuring Subjective Well-being, OECD Publishing, Paris, http://dx.doi.org/10.1787/9789264191655-en.
Since 2009, there has been enormous progress both in addressing technical problems and in the use of measures of subjective well-being. The report released in 2015 by the US National Academy represents an important milestone (Stone and Mackie, 2015), while the OECD Guidelines on Measuring Subjective Well-Being have, since their release, been adopted by Statistical Offices in almost all OECD countries (OECD, 2013c).
Underlying the success of subjective well-being measures are several research findings. First, information obtained in this way is replicable, and the statistics produced have a high degree of reliability. Second, these measures themselves can, at least in part, be explained and the explanations make intuitive sense. For example, people who live in conflict zones, like Syria, report very low levels of subjective well-being; also, across countries, an increase in average income increases people’s subjective well-being. As noted in the previous chapter, the Great Recession lowered people’s subjective well-being in countries most affected by the crisis (OECD, 2015b), especially for those who had lost their jobs.
Explaining subjective well-being is not only of academic interest. It provides us with tools for enhancing individual and societal welfare. If being employed matters for people’s subjective well-being, then this evidence should provide impetus for stronger responses to economic downturns, and perhaps even a justification for a government jobs programme. This is even more true if economic insecurity adversely affects subjective well-being and if there are compounding effects, i.e. both unemployment and economic insecurity have a direct effect on subjective well-being, but the combination of the two may have impacts that are multiplicative rather than just additive. These measures in turn may help explain other variables of interest, though here research is still progressing, and we have to worry about causality. For example, as noted by Krueger and Stone, there is a positive correlation between people’s health status and their subjective well-being, but it may be that low subjective well-being leads to negative health consequences, rather than the other way around, where negative health consequences lead to low subjective well-being. In reality, causality runs both ways. It will also be important in the future to assess the consequences of lower subjective well-being for trust and for people’s view of how the political system functions.
Throughout this book and the companion volume, we have emphasised the inter-relations among the various threads discussed in this chapter, and this is especially true for subjective well-being. Economic downturns affect insecurity, as we just noted, but economic insecurity also affects health, and health and insecurity both affect subjective well-being. Unemployment, or even the threat of unemployment, affects economic insecurity as well as subjective well-being. Recognising these inter-relations is important for at least two reasons. First, it helps us understand the multiple channels through which changes in policy may affect economic performance and societal well-being. And, second, in many cases, it helps us understand that there may be multiplier effects, not just the positive effects upon which we are focusing (e.g. the benefits of say expansionary fiscal policy on GDP) but indirect effects as well, through the reduction of economic insecurity, the increase in human capital and the strengthening of wealth accumulation, all adding up to what we are really interested in, i.e. the impact of policy on people’s lives.
3.7.1. Technical issues
The 2009 Commission report argued that subjective well-being is in itself a multi-dimensional construct encompassing several different aspects, such as life evaluations, positive and negative experiences, and eudemonia (having a sense of purpose in life). Each of these aspects requires its own metrics, and better understanding of its drivers.
Evaluative measures require a person to reflect upon and evaluate his or her life. Survey questions can be used to elicit people’s summary evaluations of their current lives. Measures based on these questions also appear to be broadly consistent with people’s choices, as people tend to make decisions based on their projected impact on their own life valuations, which can therefore be understood in terms of the economists’ standard concept of “utility”.
Experiential measures are different: they assess an individual’s feelings, emotions and states (happiness, sadness, pain) at a given moment. These measures are sensitive to the resiliency and the capacity to adapt of individuals.
Eudemonia measures the extent to which a person believes that his or her life has meaning and purpose, and is also related to a person’s psychological functioning.
These different metrics sometimes give different assessments of an individual’s well-being. They are also affected by different variables and, in turn, have different implications for observed behaviour. Understanding the differences is an area of ongoing research.
In most self-reporting, people are reflecting on past experiences, not on the subjective well-being at the moment. The two can differ, sometimes markedly. When parents are asked to respond to questions about the experiences of raising a young child at the moment, they often respond less positively than they do when asked years later. Both of these may reflect important and different aspects of well-being, but for many purposes, including for understanding decision-making and certain other outcomes like health, it is the contemporaneous assessment that is especially relevant. To avoid some of the problems that arise as a result of these disparate subjective assessments, the collection of real-time or near-time data should be encouraged, especially for reporting of people’s experiences and feelings. However, real-time data collection is expensive and sometimes impractical, so the standard has become the use of questions that ask about experiences in the prior day.
The order in which questions are asked – a standard methodological issue in all household surveys – is especially important for surveys that ask questions on subjective well-being, requiring careful consideration in survey design. There is also a problem of inter-personal comparability. Different groups may interpret the questions differently, or have in their mind different reference points. How can one know that an Italian who rates himself at 3 on a 0-10 point scale really has a lower level of subjective well-being than a Frenchman who ranks himself as 4? Problems of inter-personal comparability of this kind have long plagued welfare economics, the branch of economics centred on making judgments about the performance of economic systems. We currently lack the evidence needed to ascertain whether inter-personal differences in the use of response scale are random (hence cancelling out in large samples) or systematic across groups and countries. Researchers on subjective well-being have explored ways to address these inter-personal comparability problems (e.g. through vignettes, i.e. concrete examples of people and their behaviours on which survey participants offer as assessment that is then used to scale their own responses), while guidance on question wording, response format, survey mode and context effects allows measurement errors to be managed and reduced. Research exploring the importance of cultural effects on people’s responses to subjective well-being questions also indicates that these effects, while significant, explain only a small fraction of country-differences in life evaluation (Exton, Smith and Vandendriessche, 2015). More generally, if one can’t be sure about comparison of levels, changes can be meaningful. We can, in other terms, assess how changes in circumstances affect people’s subjective well-being, as the discussion below illustrates.
3.7.2. Some further insights
A long-standing issue in research is the relationship between subjective well-being and income. Early “happiness” studies (Easterlin, 1974) suggested that, as countries reach a certain level of GDP per capita, their average subjective well-being (life evaluation) didn’t increase. A great deal of effort has gone into explaining this “Easterlin paradox”. For instance, if subjective well-being is related to one’s relative position, then if everyone’s income doubles one’s relative position will be unchanged, in which case subjective well-being would not change either. Recent studies have enhanced our understanding of the relationship between GDP and subjective well-being (Stevenson and Wolfers, 2012) but many aspects of the relationship remain unsettled. We know, for instance, that the shape and strength of the relation depends on which aspect of subjective well-being (evaluative, experiential, eudemonia) is considered. At lower levels of income, the association between GDP and subjective well-being is positive whatever the specific aspect considered, while at higher levels of income evaluative well-being is positively associated with per capita GDP whereas experiential well-being is not (Kahneman and Deaton, 2010).
We also know that many other factors beyond income influence subjective well-being (Helliwell, Layard and Sachs, 2018). Being employed, in better health, having stronger relationships and just being connected with others are among the most important factors, with many other factors also identified in research. For instance, environmental conditions and (lack of) air pollution not surprisingly contribute to subjective well-being. There is also strong evidence of the importance of childhood experiences for people’s subjective well-being as adults, and of the importance of people’s resilience, i.e. their capacity to bounce back when confronting shocks. Childhood subjective well-being and children’s emotional health are strong predictors of adult life satisfaction (Layard et al., 2014).
Subjective well-being studies have provided us insights into how people fare over their life. There are, of course, other indicators, such as depression and suicide, but these are indicators that might be viewed as capturing “extremes” in subjective well-being. As shown by Krueger and Stone in their chapter in the companion report, in most Western countries, there is a U-shaped pattern of life evaluations with respect to age, a pattern that does not hold for experiential well-being (which is often low for young people) and that is absent in poorer countries (Steptoe, Deaton and Stone, 2015). This relationship holds across different birth-cohorts. This suggests that people’s life priorities change as they age (away from income), implying that policies towards the elderly should focus on a range of aspects beyond income security in old age.
Differences in subjective well-being, for example between individuals at different ages or in different circumstances, can also enrich distributional analysis. Elsewhere, in this and the companion volume, we speak of “inequalities”. But perhaps the most important inequalities are those associated with subjective well-being. These should be more systematically measured and reported.
3.7.3. Further research
Our earlier discussion (and, even more so, the chapter by Krueger and Stone) has already called attention to many areas where further research is needed. For instance, we noted that there are measures for different aspects of subjective well-being. It will be important to understand better their relationship with each other, the factors that affect each, and the consequences of each.
The measurement agenda is a comprehensive and inter-related one. We already noted the importance of subjective well-being as a mediating variable. As we get better measures of economic insecurity, for instance, we will be able to ascertain better how economic insecurity affects subjective well-being; and as we get better metrics of trust, we can ascertain better how subjective well-being affects trust, thus providing a more comprehensive perspective on the determinants of societal well-being.
While there has been great progress in collecting subjective well-being data, these are still far from adequate, particularly if we are to assess changes over time (such as might be associated with an economic downturn) or across countries. A low-cost way to increase data availability about subjective well-being is to add subjective well-being questions to existing surveys. We encourage National Statistical Offices to continue regular, frequent, and standardised collection of subjective well-being data based on the OECD Guidelines.
3.8. Economic insecurity
In the late 1990s, the World Bank surveyed around 60 000 individuals living in poverty in 60 countries around the world in their project on Voices of the Poor (Deepa et al., 2000). The project asked poor people about what aspects of their lives presented the greatest hardship. After the obvious deprivation of income came their sense of economic insecurity.
Concern about economic security grew during the financial crisis that erupted in 2008. Even those who had not yet lost their job realised that their jobs were at risk, as the unemployment rate soared. More than 12 million people are estimated to have lost their jobs in OECD countries from the last quarter of 2008 to the first quarter of 2010, when the employment count reached its trough. Small businesses experienced large falls in sales, sufficient to push many to the brink of bankruptcy, and many went over the brink. Declining asset and housing wealth affected many people, especially those in or nearing retirement. Young people graduating from school worried whether they would be able to get a job, especially one commensurate with their education and talents, and in countries where tertiary education is often financed by student loans they worried about whether they would have sufficient income just to repay their debt.
The impact of the crisis on people’s sense of economic insecurity may have been all the greater because, even before the crisis, large parts of society had faced growing insecurity due to changes in the economy, society and policies. For instance, areas of the world confronting de-industrialisation saw life prospects in decline. Without adequate systems of social protection, active labour market policies, and policies ensuring near-full employment, the result was high insecurity.
It is, accordingly, natural that the topic of economic security is nowadays a greater concern than at the time when the Commission report was published in 2009, not only for citizens, but also for economists and policy-makers.
As argued by Jacob Hacker in Chapter 8 of the companion volume, economic insecurity is a major aspect of people’s well-being. One of the reasons that governments did not pay sufficient attention to it was that it was not adequately reflected in our standard statistics.
We focus here on economic security, but the boundary between economic security and other forms of security is hazy. Countries like the United States with inadequate systems of health care and disability insurance, forcing especially those with limited incomes to face high levels of health insecurity, have high levels of economic insecurity. A major determinant of personal bankruptcy in the United States is an extended period of illness or expensive health expenditures for some member of the family. In countries with high levels of physical insecurity, people also face the risk of serious losses of their assets from theft.
3.8.1. The concept of economic insecurity
Hacker defines economic insecurity as “individuals’ (or households’) degree of vulnerability to economic loss”. This broad definition includes three elements: 1) the probability of an adverse event (a shock); 2) the negative consequences from this shock (the loss); and 3) the kinds of protection to prevent or cover these losses (the buffers). All three elements are inherent in this definition: some probability of an adverse event; some negative economic consequence if this event occurs; and some set of protections (from formal insurance to informal risk sharing, to self-insurance through savings and the like) that potentially offset or reduce the impact of the losses. All of these elements of economic security are crucial when it comes to the development of measures to capture overall economic insecurity.
Within this definition, one can also distinguish between “observed” (or objective) insecurity, where analysts use economic data to determine whether an individual or household is, in some sense, at risk of experiencing large reduction in say consumption (perhaps reflected in large proportions of those within a particular group experiencing such large reductions); and “perceived” (or subjective) insecurity, reflecting the sense of vulnerability felt by individuals. With measures of perceived insecurity, individuals themselves reveal their subjective response to their economic situation, whether through surveys, experiments or some other revelation technique.
3.8.2. The drivers of growing economic insecurity
Even before the financial crisis, citizens and leaders in advanced democracies expressed concern that economic security was declining. In developing countries, governments grappled with new economic risks as people moved into wage labour and the traditional risk-spreading role of the family declined. In both developed and developing countries, public concern centred on the changing character of the economy and society with, for instance, the fast-paced changes driven by globalisation and technological advances, rising health care costs and lower pension benefits (especially in relation to previous earnings) in many countries, and on the roles of governments, markets and households in coping with the related economic risks.
Economic insecurity stems from the interaction of shocks, impacts and buffers, with changes in any of the three potentially leading to higher economic insecurity. Changes in the structure of the economy or in economic policies can increase people’s economic insecurity by increasing the probability of a negative shock or its impact or by reducing buffers available to respond to shocks. For instance, changes in family arrangements that increase the likelihood of living alone have reduced the protections provided by the family. Financial globalisation, it was argued, would enable countries to better manage risks, borrowing in bad times and repaying in good; but in fact, for most countries (and for most individuals), it has also increased volatility and insecurity, with capital flows typically being pro- rather than counter-cyclical. Digitalisation increases consumer choice and provides access to new services, but also fuels concerns about the effects of robotics and artificial intelligence in displacing jobs and entire occupations, increasing people’s fear of losing their job and income.
The risks that can lead to economic losses are many, and stretch beyond the economic realm narrowly defined. While some of them are recognised by existing social security programmes (e.g. unemployment, disability, illness, old age), others are often disregarded (as in the case of needs of long-term care for frail elderly, of mental health problems, and of frequent shifts between low-paid work and unemployment) or addressed through programmes originally devised for addressing risks that no longer exist (such as programmes for war widows, which are used today in the United States to address the needs of lone mothers).
Many reforms to social protection or health care introduced in recent years, pursued either to contain costs or to extend coverage, have reduced buffers available to people or shifted risks to those less capable of protecting themselves against them. As we discuss later, the weakening of social protection systems has had the collateral effect of increasing economic insecurity. In developing countries, the security provided by family support systems has typically weakened faster than countries have been able to provide public systems of social protection. Similarly, in advanced countries, the shift from defined benefit to defined contribution retirement systems has shifted risk from pension schemes to the elderly.
Even good systems of social protection do not fully offset the increase in economic insecurity associated with macro-economic volatility. This is especially so because individuals are more sensitive to an income loss of a given size than to a gain of the same amount (“loss aversion”, as mentioned above). This evidence suggests that macro-economic policies should pay more attention to minimising the bumps that the economy may face rather than just focusing on achieving the maximum GDP growth rate possible.
Increasing income inequality also increases economic insecurity: as the rungs of the ladder are further apart, slipping down a rung becomes more consequential. Labour market reforms aimed at increasing flexibility, while giving more discretion to the employer, may have increased workers’ economic insecurity, except in those countries where unemployment benefits were raised and policies that helped workers find a new job quickly introduced.
3.8.3. Consequences
The consequences of economic insecurity can be severe, both economically and politically, both for individuals and for society as a whole. Economic insecurity is a source of stress and misery for people around the world. When individuals worry about the future, trying to figure out how various contingencies might impact them when they are just getting by as it is, they are less able to make good decisions, including about those elements that will affect their future well-being. There is not just a present cost from economic insecurity, but a future cost as well.
An increase in economic insecurity affects well-being and economic performance through many other channels. Autonomy at work is reduced by the fear of losing one’s job. Job satisfaction falls and, with it, overall well-being. Growing economic insecurity and inadequate safety nets make people less willing to take risks, undermining economic growth and entrepreneurship.
Economic insecurity is conceptually different from poverty. It affects a much larger share of the population than poverty does. Those above the poverty level but at risk of falling into it might feel high economic insecurity. Because of its wider reach, a growing sense of economic insecurity is more likely to lead to shifts in political power than changes in poverty headcounts. Perhaps the increase of populist voting after the crisis would have been less of a surprise had the higher economic insecurity among large parts of the population prompted by the crisis and by ongoing structural and policy changes been recognised.
We noted earlier the increase in economic insecurity associated with deep downturns. But economic insecurity may also deepen and extend the downturn: individuals, worried about their future, may be hesitant to spend, just at a time when such spending could help spur recovery.
3.8.4. Market failure and policy
One might ask, if individuals value security in the same way they value the nutritional content of food, why isn’t economic security reflected in market prices? Shouldn’t the increased value of market products designed to reduce insecurity be reflected in higher GDP?
To some extent, this is already the case. The market does provide insurance that helps reduce economic insecurity, and the value of those products (the excess of the price of those products, i.e. of the benefits paid out, over their costs - the wages paid to those administering the system) is indeed included in GDP. But, due to informational asymmetries, transaction costs and other market failures, insurance markets for many important contingencies do not exist.
Social protection systems were created to address these market failures and to reduce economic insecurity associated with unemployment, illness, disability, widowhood and old age. But recent reforms have often shifted risks from governments to individuals, from firms to workers, and reduced the extent to which risks are “mutualised”. Consider for instance, the risks to economic security in old age. Before governments created public pensions (known as social security in the United States), the private market failed to provide annuities (i.e. assets paying someone a fixed amount of money for the rest of his or her life). Even today, transaction costs for annuities are large and, whether because of this or of information asymmetries or other market failures, buying a long-term bond is often more advantageous than buying an annuity. Yet, reforms of public pension systems have tended to put more reliance on the private sector. According to the EU Pension Adequacy Report, the UK partial privatisation of public pensions may have had the effect of reducing the projected pension benefits of men earning the average wage by 52% (European Commission, 2018, p. 114). More generally, the shift from defined benefit to defined contribution programmes has shifted risk from corporations to individuals, even though the former are generally in a better position to bear and manage such risks.
There is an extensive literature on the fact that market failures are widespread in risk markets. Social insurance programmes were created to make up for failures in insurance markets, and are often more efficient than private schemes, partly because they do not have advertising costs, partly because the private sector devotes large efforts at “cream skimming”, trying to make sure that they insure only those with the lowest risk. Some of these public institutions can be thought of as reflecting a social contract that arises from a democratic process. When these social contracts become more costly to maintain, policy reforms need to confront the risk that, as they try to make social protection systems financially sustainable, they may also increase the economic insecurity of groups of the population who have little capacity to bear it.
If our metrics do not capture the benefits that social insurance provides through greater economic security, there will be a tendency to focus on their costs, underestimating their benefits. The move towards a leaner welfare state is thus in part a consequence of not accounting properly for the benefits of the welfare state.
These benefits are, of course, greater than just the value of the increased individual security. If more secure individuals are more productive and/or are able to undertake higher productivity but higher risk activities because of the presence of good social insurance, then society is better off, with some of the benefits of the increased productivity captured for the public benefit through taxation.
Concerns about moral hazard (adverse incentives) imply that it is not always desirable to provide complete insurance. The attack against social insurance, however, has gone beyond the level which can be so justified, especially today when so many people feel economically insecure. It is clear that we need to improve our metrics of economic insecurity and to assess policies for their impact on economic security, in addition to other goals.
Both globalisation and technological change increase the likelihood of many of the risks mentioned above. Hence, their acceleration should be accompanied by higher and/or different (rather than lower) social protections, including systems that permit the portability of acquired rights across jobs and countries of residence, as well as investments in education and training to make people more resilient in the event of a negative shock. In all cases, however, it would be better to explicitly consider the effects of globalisation and technological change on people’s economic insecurity ex ante, rather than just coping with their effects ex post.
3.8.5. Metrics
There are two critical distinctions in designing metrics of economic insecurity. The first we referred to earlier: that between objective and subjective measures. The second distinction is between dashboards of economic security based on multiple measures, and integrated measures, which try to capture individual or household security in a single measure. For many purposes, dashboards are preferable to single indexes, which are less transparent and more sensitive to analysts’ choice of components and weights.
One of the main challenges for researchers and Statistical Offices is to identify a limited set of measures of economic security that can be used in a broader dashboard of well-being indicators. These selected measures should generally be: 1) broadly comparable across countries; 2) available over sufficient time periods to monitor changes; 3) closely linked to individuals’ actual experience; and 4) capable of informing policy-makers as they seek to address economic security.
Surveys for assessing perceived economic security have the advantage of directly capturing individuals’ perception of their personal and household economic conditions. However, they have two shortcomings: surveys have not been run for a significant time span, and questions differ among national surveys, thus hampering cross-country comparisons. Survey questions can be grouped in three categories: 1) general assessment of the economy (how one feels about the economy or the economic situation in the present or in the past); 2) perception of the buffers available to respondents (for example, the length of time respondents believe they could avoid economic hardship if faced with an adverse shock); and 3) expectations regarding future shocks (one’s likely economic situation in the future or one’s worry with regard to specific risks, such as job loss). All types of questions should be included in household surveys.
Objective measures try to quantify the shocks facing an individual (the probability of a shock and the depth and duration of the impacts) and the size of the buffers enabling individuals to cope with these shocks. One such measure, described by Jacob Hacker in his chapter in the accompanying volume, looks at the size of the population who experienced a large income loss (a fall in household-size-adjusted individual income from one year to the next of 25% or more). This measure, which relies on panel data following the same individual over time, is now available in a large number of industrialised countries; it shows large differences across OECD countries (Figure 3.5), with many countries experiencing increasing insecurity.28 Other types of objective measures discussed by Hacker look at the key features (such as coverage and generosity) of the public programmes that exist in various countries to address risks such as unemployment, disability, old age, etc.
Figure 3.5. Average, range and evolution of the incidence of large income losses across countries
Note: Based on the following panel data collections: ECHP, EU-SILC, CPS, CNEF (BHPS, SOEP, HILDA, KLIPS, SHP, SLID). For each country, the period covered is indicated on the horizontal axis. Arc-changes, unlike percentage changes, treat gains and losses symmetrically (e.g. an income gain from USD 50 to USD 100 implies a 100 per cent change but a 67 per cent arc-change; while an income loss from USD 100 to USD 50 implies a 50 per cent change but a 67 per cent arc-change); they are bound between +2 and -2.
Source: Hacker, J. (2018), “Economic security”, in Stiglitz, J.E., J.-P. Fitoussi and M. Durand (eds.) (2018), For Good Measure: Advancing Research on Well-being Metrics Beyond GDP, OECD Publishing, Paris.
3.8.6. Further research
Further work is required to select the best type of measure, to understand its properties, and to relate insecurity to other aspects of economic performance and individual well-being. The availability of reliable and cross-nationally comparable data has been a crucial constraint on the development of improved measures of economic security. Three shortcomings of existing statistics stand out: the limited pool of long-term and cross-nationally comparable panel data about the income of various individuals; the weaknesses of most administrative data for tracing individuals over time; and the lack of regular questions about economic insecurity in conventional household surveys.
Measures of complex phenomena are necessarily imperfect, as is the measure of GDP, but that should not prevent us from going ahead. The implication of the remarkable advances in the measurement of economic insecurity, described by Jacob Hacker in his chapter, is that governments should no longer ignore the effects of changes in economic structure and policy on economic insecurity. Policies and statistical practice cannot ignore something that is of first order importance for people and communities.
3.9. Sustainability
The importance of sustainability has been emphasised by the name of the goals that the world has set for itself for the coming decades: Sustainable Development Goals. GDP growth today that is at the expense of future generations is unacceptable. As we think about what we do today, we must bear in mind our legacy to the future. If we leave the world a worst place to live, then our own prosperity is at the expense of our descendants. Economists and philosophers refer to this as inter-generational equity. People used to believe that future generations would automatically be better off than the current one, the only question being by how much. This assumption no longer holds.
Assessing sustainability requires determining if the current level of well-being can be maintained for future generations. By its very nature, sustainability involves the future and, as Yogi Berra quipped (paraphrasing Niels Bohr), “It’s tough to make predictions, especially about the future.” Its assessment involves many assumptions and normative choices. If (appropriately measured) wealth is increasing, then presumably society could do in the future whatever it does today, i.e. it can sustain its per capita well-being. But, to assure that outcome, we need a comprehensive measure of wealth, and we need to use the right valuations. The Commission report in 2009 argued that measures of the sustainability of well-being are conceptually different from measures of current well-being, except to the extent that individuals’ current well-being is adversely affected by anxieties over sustainability itself.29 Well-being may be enhanced today by depleting some of the capital stocks essential for maintaining future production and future well-being.
3.9.1. Environmental, economic and social sustainability
The most immediate threat to sustainability is the environment. We live within a limited biosphere. Economists traditionally ignored these limitations, and constructed models of economic growth without end. When, some half century ago, the Club of Rome started talking about Limits to Growth (Meadows et al., 1972), the response was a wave of technological optimism: we are innovative, the argument was, and whatever the Earth’s limitations we can innovate around them. This techno-optimism has now been questioned, especially with the growing awareness of climate change brought about by the accumulation of greenhouse gases in the atmosphere, and natural scientists have quantified “safe operation boundaries” for several critical types of natural capital (Rockström et al., 2009). Of course, there may be a technological revolution, but right now, in the race between technology and humans’ destruction of the single planet on which they live, technology is losing. We are on course for disruptive changes in our ecosystem, with temperatures and sea levels rising, and disease vectors and weather variability increasing – a host of changes that, at the very least, threaten to impose costs on future generations which may well lower their standards of living, and significantly so. Major cities will be affected, and hundreds of millions, perhaps billions, of people relocated. The world faces countless environmental threats.
The 2008 crisis also showed that the economic system might itself not be sustainable. Much of the GDP growth that preceded the crisis was based on a bubble, and when the bubble burst, so did the seeming prosperity. Governments that thought that they were acting prudently (like Spain and Ireland) found that their spending was based on “bubble revenues”. Families that thought they could get by with stagnating wages because their wealth was increasing as house prices soared and they could increase their debt, were brought down to earth. They had been living beyond their means, and promises of ever-rising standards of living were shown to be false. Standards of living in large swaths of advanced countries were seen to be little better than they were decades earlier.
In 2009, the Commission emphasised the importance of looking at sustainability in all of these dimensions. It was keenly aware that standard economic measures captured none of this, and it struggled with the question of what indicators would best reflect sustainability. The Commission arrived at a consensus that if markets worked well, one could form a comprehensive measure of the “capital” of the country, i.e. the value of the resources that one generation passes on to the next. This would include all forms of capital, physical/economic capital (buildings and machines), human capital (and knowledge capital); natural capital (environmental resources); and social capital (trust and connections with each other). If we leave more capital to our descendants than we inherited, then our descendants are likely to be better off, and their standards of living will be at least as good as that of the current generation. We say then that such growth is sustainable. This is the capital approach to sustainability.
The Commission, however, recognised that there were many problems with this approach, especially with its practical implementation. The first, and most obvious, is that markets for the future often don’t work very well. There was a housing bubble because markets put an excessively high value on real estate. For fifty years, economists have studied why markets so often systematically get these prices wrong. In the case of natural resources, the market failure is even more obvious: we generally don’t impose a price on carbon emitters or on biodiversity loss.30
The obvious failures of market pricing led the Commission to suggest that within the dashboard of indicators, there ought to be some physical metrics, such as the carbon footprint or the levels of concentration of carbon in the atmosphere.
In the narrower sphere of economics, some asset metrics also need to be introduced (see below). Unfortunately, in much public discourse, attention gets centred on gross debt, and especially public debt. Chapter 2 emphasised that this is an unbalanced perspective: no one looking at a firm would consider its debt without also looking at its assets. You need to consider the overall balance sheet. Partial information is dangerous: focusing on debt alone may lead governments to take actions (like cutting productive investments) that more than offset the increased value of liabilities, leaving the country worse off.
Other aspects of wealth, such as social and human capital, present even more formidable challenges for measurement. The HLEG, as we noted, decided to focus its attention on one aspect only, trust, a key part of social capital (see below).
As argued by De Smedt, Giovannini and Radermacher in Chapter 9 of the companion volume, another problem with the capital approach is that it may suggest a straightforward trade-off: one could make up for the deterioration of environmental capital simply by adding more economic (physical) capital. This reasoning led some economists to suggest that it would be better to do nothing about climate change today; we should simply set aside enough money (economic capital) so that, should climate change occur, we had made future generations better off by leaving them more capital overall.
Even at the time of the Commission report, we cast doubt on that analysis. As a practical matter, the magnitude of the increased economic capital that would be required to offset the effects of climate change simply wouldn’t be forthcoming. Any rational discourse had to take into account risk, recognising that in the worst outcomes, when the magnitude and consequences of climate change were most severe, we would be least able to take the actions to offset those effects.
The work of the HLEG has highlighted these risks in several ways. First, it highlighted the concepts of resilience and vulnerability. Secondly, it focused on the complexity of the economic/social/environmental system as a whole. Complex systems are characterised by extensive non-linearities and interactions, which make them hard to predict. Even simple complex systems can give rise to chaotic dynamics, with the system oscillating forever without any discernible patterns. Small changes in initial conditions or in the parameter values of the system can give rise to large changes in the dynamics of the system. This inherent uncertainty should make us particularly cautious as we approach major changes in the ecosystem, and the marked increases in atmospheric greenhouse gases represent such a large change. As the debates on climate change unfolded, we already saw several examples of this, many of which were not fully anticipated: as the tundra has defrosted, methane gases have been released which have hastened its melting. As the solar ice cap melts, the Earth reflects back less energy, exacerbating global warming. There can be sudden reversals in patterns of ocean currents.
Analytically, price systems work only when the underlying technology is “well behaved”, that is it exhibits familiar patterns of diminishing returns, as seen when one adds more and more labour and capital to land in agriculture. The early pioneers in mathematical economics (Arrow and Debreu, 1954) recognised this.31 Unfortunately it was a lesson that practical followers of market economics lost sight of – a mistake that has become increasingly important as attention has centred on a host of violations of conventional assumptions of economic models, such as those associated with R&D, learning, bankruptcy, contagion, etc. (Stiglitz and Greenwald, 2016; Battiston et al., 2013). The fact that systems dynamics can be so sensitive to slight perturbations in initial conditions and parameter values means that, even if it were possible to find competitive prices that might prevail in the future, it would be extraordinarily difficult to be sure what those prices should be, with small errors in estimates of the relevant parameters resulting in large changes in prices (Roukny, Battiston and Stiglitz, 2017).
3.9.2. Vulnerability and resilience
As important as risks are, most earlier analyses paid insufficient attention to the uncertainties and variabilities. Just as the previous section focused on how risk gave rise to economic insecurity at the individual level, here we need to focus on the consequences of risk to the sustainability of systems. There are two related questions.
First, what is the risk of a negative shock to the economic or environmental system, in particular of a shock that might make the growth path unsustainable (in which case past living standards might not be attainable in the future)? For instance, there is uncertainty about the relationship between current levels of greenhouse gas emissions, the total stock of these gases in the atmosphere, and future levels of climate change. As shown by the Stern Review (2006), while stabilising concentrations of greenhouse gases at the level of 450 parts per million (the level targeted by the 2015 Paris Agreement) will most likely limit the increase in global temperature to 2 degrees Celsius, greenhouse gas concentrations at this level still imply an 18% risk of a temperature increase of 3 degrees, and a 4% risk of increases of 4 degrees of more. The system may be more fragile and vulnerable than we currently think, so that, for a given level of GHG concentration in the atmosphere, the size of temperature increase (with all the attendant knock-on effects) could be much greater than in the baseline scenario.
Second, what is the resilience of the system, i.e. how strong are its capabilities of recovering, for instance, from a large shock?
We can think of quantifying, at least in principle, the vulnerability of the economic and environmental system – taking into account its resilience – by asking (analogously to how we measure the impact of inequality) how much would a person or a society be willing to give up (in terms of their wealth) to eliminate this risk. Similarly, we could measure the value of an improvement in the resilience of the system by ascertaining by how much the “vulnerability premium” is reduced as a result. Both types of measures, however, are likely to vary across individuals and countries. The question of how much society would pay to reduce vulnerability entails judgments about the welfare across generations. In turn, any reasonable assessment of the welfare of future generations – especially taking into account the risks of very adverse scenarios on climate change and recognising that, in those contingencies, society would have limited capacity to deal with the impending disaster – implies that we are almost surely not investing enough to reduce vulnerability.
3.9.3. Metrics of environmental and human capital
In Chapter 2 we discussed the particular difficulties in measuring capital (notably human capital), especially in the aftermath of a crisis. We noted there how underestimating the impact on wealth (and thereby on sustainability) of the recession and of the accompanying austerity policies lead governments to not take strong enough policies to stimulate the economy.
We also discussed some of the flawed measures used to assess economic sustainability (looking just at the government gross debt-to-GDP ratio) and how these too may have contributed to a weaker than desirable response to the downturn. As we noted earlier, even in the lead-up to the crisis, standard metrics may have given an impression that the economy was doing better than it really was, and not just because the government’s revenues were artificially inflated as a result of capital gains associated with the bubble. Higher risks incurred by banks raised the spread between interest rates on loans and deposits, and thereby the measured output of financial intermediaries.32
Since the 2009 report, there has been significant progress in the availability of data on environmental sustainability. In 2012, the UN Statistical Commission – the guardian of official statistics – adopted as a statistical standard (the same status given to the System of National Accounts) a set of “core” economic and environmental accounts (the System of Environmental-Economic Accounting, or SEEA). The SEEA provides a framework in which environmental statistics are fully integrated with national accounts, and thus offers a measurement framework for analysing the interactions between the economy and the environment.33
SEEA accounts cover flows of natural resources, products and residuals entering in (as inputs) and exiting from (as waste) the economic system. These accounts (which include both flow and asset accounts, in both monetary and physical units) cover minerals and energy resources; land and soil resources; timber resources; water and aquatic resources; as well as other biological resources (United Nations et al., 2014).34 While these core accounts start from an economic perspective, a separate set of ecosystem accounts (whose status is recognised as “experimental”) starts from the perspective of ecosystems,35 looking at both the relation between ecosystems and human activities, and at the relation among ecosystems (for example, how wetlands depends on water flows from a contiguous river basin). While these are important developments, implementation of these accounts will spread over a long period, with different countries giving priority to different types of natural resources. So we still fall short of having adequate measures of the risks to the natural system, and scientific consensus on where the planetary boundaries and tipping points are (Steffen et al., 2015).
As we take a closer look at each of the elements of “capital”, the notion underlying sustainability, we note many unresolved measurement problems, both at the conceptual level and in implementation. Beyond the issues on measuring trust and social capital discussed below, there are also unresolved controversies over the best way of accounting for the depletion of natural resources, the degradation of the environment and the loss of biodiversity. For instance, the discovery (or the confirmation of the existence) of an economically exploitable natural resource (adding to the level of “proven reserves”) is treated in the national accounts as an addition to wealth, implying that if discoveries and depletion move in tandem, there is no reduction in capital. That would be the case if there were an infinite amount of natural resources and all that was needed was to discover them. What is scarce, in this view, is information about where the resources are, rather than the resources in themselves. A more realistic view, though, is that in the case of many non-producible and non-renewable resources, resources are finite, and when we consume those resources, our natural capital is being reduced even if this may be offset by discoveries.
The SEEA addresses this issue. While the SNA only includes mineral and energy resources that are economically exploitable given current prices and technology, SEEA includes (at least in theory) all known mineral and energy resources, exploitable and not exploitable. Nonetheless, extending the boundary of mineral and energy assets in the accounts is more easily done in physical than in monetary terms. The valuation of mineral and energy deposits, especially those that are currently non-exploited because of market prices that are below extraction costs, poses considerable challenges to Statistical Offices.36
Human capital is another important asset for individuals and communities to maintain their well-being over time. An increase in human capital, and a decrease in the inequality of its distribution, can have great positive effects on the economy and on the well-being of society. It enhances future productivity and the ability to innovate. People with higher education live longer, have higher earnings and accumulated wealth, better health conditions, denser networks of connections and are more active citizens. While well-being, both now and in the future, is enhanced when human capital increases, people’s skills and competencies decay when they are not used (e.g. in the workplace). Market and individual decisions may also lead to under-provision of human capital when the full range of its benefits is not fully recognised or doesn’t accrue to the person or firm investing in it, or when there are capital constraints limiting the resources that an individual with limited means has to invest in himself.
The problem is that there is no fully satisfactory way of measuring human capital. One approach focuses on “costs” (like the costs of constructing physical capital). But physical capital goods are bought and sold in relatively competitive markets, so that the price of a machine reflects, in some sense, its underlying “value”. Human capital is generated by formal education, most of which is publicly provided, but also by work experience and upbringing: in none of these cases do we have a good mechanism for assessing the value of the asset which has been acquired. Another approach looks at the “income generated”, say by education and work experience, over the working life of each person. But this has at least two problems. First, it is impossible to identify with any precision how much of the income generated is a result of capital investment and of the individual’s own efforts. It may be that individuals with more education, for instance, work harder, in which case some of the increased income associated with higher education is wrongly attributed to human capital, exaggerating the estimates of its magnitude and importance. Secondly, it suggests that the only value of human capital is to produce more goods and services. In this approach, when a person retires, he or she has no more human capital left. This is obviously wrong. Even then, human capital continues to yield dividends in terms of higher well-being.
While both of the approaches to human capital measurement described above aim to derive a monetary value of the stock of human capital, a more practical approach is the one used by the OECD to measure the key cognitive and workplace skills needed by adults to prosper in society, similar to that used to measure the skills and competencies of 15 year-old students. The OECD Survey of Adult Skills has so far been conducted in 40 countries, with second cycles starting in 2019. Physical measures of this type need to be continued in the future, extended to more countries and to more types of (non-cognitive) skills.
3.10. Social capital and trust
Previous sections have outlined aspects of economic performance that have typically been given short shrift in policy discussions, and suggested that one of the reasons for this is that they are inadequately reflected in our standard measures of economic performance, such as GDP, and social progress.
Here we consider one area that has only recently moved into the purview of economic discourse: social capital in general and trust in particular. The Commission report in 2009 recognised the importance of social capital, the glue that holds society together, noting the importance of social ties within the community to individual and societal well-being. The extent of connectedness with other members of society varies widely between individuals, with people with more connections having advantages over others.37 An important source of inequalities of opportunity is the unequal distribution of social capital.
Social capital allows contracts to be honoured without resorting to courts. If every contract had to be enforced through the courts, the market system could not function. Indeed, most economic relations are not based on contracts but on generalised trust. Traditionally, trust has been recognised as a fundamental factor in the history of finance and financial transactions. There is now a well-developed literature on social capital. Some of this is formalised through game theory, showing that it is rational, in repeated games, for people to act in a co-operative and trustworthy way (Dasgupta and Serageldin, 2000). More recently, a small literature has used insights from sociology and cultural psychology to explain trustworthy and co-operative behaviour, for instance to explain the success of micro-credit lending in Bangladesh and its failures in India (Haldar and Stiglitz, 2016).
The HLEG decided to focus its attention on one aspect of social capital, albeit a very important one, trust. Yann Algan, in Chapter 10 of the companion volume, defines trust as “a person’s belief that another person or institution will act consistently with their expectations of positive behaviour”. What makes the concept of trust so useful is: 1) there are a variety of relatively consistent ways by which it can be measured; 2) variations in levels of trust can be explained in ways that correspond to theory and common sense; and 3) trust metrics can be shown to be related to other measures of economic performance and individual well-being. Given the importance of trust, policy-makers should pay more attention to it, and to how their policies affect it.
The 2008 crisis reduced not just economic security but also trust. This was especially so because of the widespread perception of the unfairness in the manner in which the crisis was handled, as we showed in Chapter 2. The loss of people’s trust (both in others and in institutions) is likely to be a long-lasting legacy of the crisis. This loss was predictable, given what we know about the determinants of trust.
We should think of trust as an asset, as a key part of social capital. Trust takes time to build but can dissipate quickly when people perceive that others did not behave in a trustworthy way. There are thus important hysteresis effects, i.e. long lasting impacts of past actions on present or future circumstances and behaviours.
There are many different forms of trust. A key distinction is that between trust among individuals (inter-personal trust) and trust in institutions (institutional trust). Similarly, within a given society, there can be trust in some institutions and not in others; trust among people you know personally but not strangers (generalised trust). While trust in others and trust in institutions are different, they are also related. When institutions are dysfunctional and the rules of the game are perceived as unfair, they lower people’s willingness to co-operate with each other, creating a “society of mistrust” (Coyle, 2013). It then becomes more difficult to implement policies for the common good, which helps create the conditions for populism and can even put democracy at risk (Inglehart and Norris, 2016).
Typically, trust diminishes the more distant the relationship or the institution is, as we go from “local” to “national” or “global”, from families to clans to strangers. While each aspect of trust has its own determinants, causes and consequences, some general patterns are discussed briefly below.
3.10.1. Metrics
Good measures of trust have been lacking, and this has contributed to policy-makers’ failure to focus adequately on trust. Existing measures of trust in institutions are typically based on small-scale unofficial surveys that use a single question conflating different aspects of trust, for instance trust in different institutions, and provide only limited evidence of changes over time. Existing measures of trust in others have similar limits. Better understanding of trust and its drivers call for better metrics.
Despite these limits, existing measures show huge cross-country differences in levels of trust in others (Figure 3.6), differences in trust between different institutions in the same country, and a downward trend in many countries (e.g. the United States, other English-speaking countries, and the European countries most affected by the crisis).
Figure 3.6. Trust in others around the world
Source: Algan, Y. and P. Cahuc (2014), “Trust, growth and well-being: New evidence and policy implications”, in Aghion, P. and S. Durlauf (eds.), Handbook of Economic Growth, Vol. 2, Elsevier, North Holland, Amsterdam, pp. 49-120.
Trust is usually measured through answers to survey questions. Generalised trust, by which is meant trust in strangers, is evaluated in the World Values Survey through the question: “Generally speaking, would you say that most people can be trusted, or that you can’t be too careful when dealing with others?”. Trust is equal to 1 if the respondent answers: “Most people can be trusted” and 0 otherwise. Generalised trust is positively correlated with a range of positive phenomena: GDP per capita, subjective well-being, total factor productivity, financial development, co-operative relations between workers and management, quality of the legal system, etc. Studies have shown enormous differences in trust not just across countries but also across regions of the same country (Putnam, Leonardi and Nanetti, 1993) or across states in federal countries (Putnam, 2000).
Countries with higher levels of trust tend to have higher per capita income. (This is a correlation, not a statement about causation. As we have already noted, there may be a two-way relationship, with greater trust leading to higher GDP, and a higher GDP leading to behaviours which stimulate trust, as well as other factors that may influence both trust and GDP.) Both generalised trust and trust in institutions are higher among higher income groups and among more highly-educated people. They are lower among unemployed people and single-person households with one or more dependent child.
As one might expect, trust is negatively correlated with income inequality. And rising income inequality has also been related to lower trust in institutions. High-trusting societies have lower levels of income inequality, measured by Gini coefficients, while low-trusting societies show typically higher levels of income inequality. Trust is undermined by things that run against people’s sense of fairness. Since, at least in many countries, there is a general sense that income is inequitably distributed, it is not a surprise that economic inequality is consistently identified as one of the strongest predictors of generalised trust, and that countries with highest levels of trust rank highest on economic equality (e.g. Nordic countries, the Netherlands, Canada; OECD, 2018a).
The adverse impacts on trust are among the “hidden costs” of inequality, which deepen fault lines in societies. It is undoubtedly one of the reasons behind the argument that income inequality has adverse effects on economic performance. Societies pay a high price for inequality, including through a loss of trust in each other and in institutions (Stiglitz, 2012a).
While the patterns described here hold true within and across the majority of OECD countries, it is important to study the drivers of trust in the context of countries’ specific circumstances.
3.10.2. The role of trust in our economy and the consequences of a lack of trust
A growing literature has traced the growth of modern capitalism to trust (Greif, 1993, 1994). The modern financial system, arguably, would not have arisen without the high levels of trust that existed in the different Italian city-states, such as Florence.
For reasons that have been well described by Arrow (1972), trust matters a great deal in a market economy. It makes it unnecessary for market participants to engage in the impossible task of attempting to write contracts covering all types of future contingencies, and thus it improves the functioning of the economy. Arrow’s intuition was straightforward. In the absence of informal rules like trusting behaviour, markets are missing, gains from economic exchanges are lost and resources are misallocated. In that respect, trust and the informal rules shaping co-operation can explain differences in economic development.
Responses to survey-based questions on trust are good predictors of macro-economic outcomes, but there is very little understanding of the microeconomic mechanisms involved, save the Arrow analysis.
Not surprisingly, trust is related to subjective well-being. For instance, co-operative social relationships with others, which are facilitated by trust and give rise to trust, affect people’s health and happiness above and beyond the monetary gains derived from co-operation.
A lack of trust also has political consequences. Growing economic insecurity and higher unemployment lower trust in political institutions and this in turn leads to higher voting for populist parties. Trust is also rooted in places and closely-knit communities whose members behave in a trustworthy manner towards other members of the same community (taking into account the well-being of others). Diversity, if not supported by integration policies, may erode this sense of communal trust and, more broadly, support for redistributive policies.
In turn, low trust in institutions leads to fewer people voting (this is especially true among the less educated and those with lower income)38 or participating in policy in other forms. The growing gulf in the occupational background and economic circumstances between voters and their elected representatives also breeds mistrust. In a sample of OECD countries, manual workers and people in other elementary occupations make up 44% of the voting age population, but only around 10% of the elected members of parliament (OECD, 2017a). In the United States, the median net worth of members of Congress was above USD 1 million in 2013, while the median net worth of American households was around USD 60 000 (Sitaraman, 2017). One implication is that less than 30% of all people in OECD countries feel that they have a say in what the government does.39 The 2009 Commission report emphasised the importance of “political voice” for well-functioning democracies and for people’s well-being. Judging from the current wave of populist votes in many developed countries, this is an area where we seem to be moving backwards rather than forward.40,41
3.10.3. Policy
As trust plays a key role in explaining economic and social outcomes, it is urgent to identify the institutions and policies needed for it to develop. In particular, policy-makers need to pay attention to those actions which might erode it.
Actions and processes that are perceived to be “unfair” (for instance, in how people are treated in the processes by which decisions get made) undermine trust. In the United States, as we noted, the seemingly favourable treatment given to bankers relative to homeowners, undermined trust in government. So too in Europe, where the austerity measures imposed on crisis countries – with particularly adverse effects on the poor – were widely perceived as unfair, undermining trust in the key European institutions. At a minimum, governments engaged in policies that are so perceived have a duty to do a better job in persuading citizens that there is an underlying “just” rationale for the policies.42
More generally, measures of people’s trust in various institutions, its causes and consequences, provide a point of entry for developing metrics of “how government functions” from the perspective of the people they serve. This is an important area for future statistical work. There are some obvious candidates for important determinants: when citizens’ preferences do not get reflected in legislations, it undermines trust that the institutions are acting in any way consonant with democracy. So too actions that limit democratic participation and enhance the influence of money should be expected to undermine trust in democratic institutions. These are all empirical questions which can be tested, with results that might shape a “trust building” agenda.
There has only been limited progress in identifying policies that most effectively promote trust. As shown by Algan in his chapter, the evidence that exists is mainly limited to the importance of education, including early interventions, with a focus on social (non-cognitive) skills and teaching methods.43 If this is so, it implies that we need to routinely measure those non-cognitive skills that impact on trust through instruments like PISA and PIAAC (the OECD Survey of Adult Skills).
3.10.4. Further work
We need to improve existing measures of trust. As we noted, trust metrics (either trust in others or trust in institutions) are typically derived from household surveys, but most evidence stems from non-official surveys with small and non-representative samples that do not allow to see which parts of the population face the largest trust deficits. Advances in experimental economics and psychology have made it possible to verify correlations between trust as reported by respondents in surveys and trust and other co-operative norms observed in laboratory experiments. The results suggest that survey measures, especially those of generalised trust, produce valid measures. In the future, it will hence be important to include these questions in the routine, large-scale, data collection activities of National Statistical Offices. The OECD Guidelines on Measuring Trust (OECD, 2017b) provide information on various facets of statistical quality of existing trust measures, as well as practical recommendations on the type of questions that could be included in official statistics.
At the same time, efforts should continue to extend the complementary measures of trust that lab experiments can offer using representative population samples. Yann Algan describes in his chapter how these types of measures are already being implemented by researchers, as in the case of the Trustlab project, jointly managed by the OECD and Sciences-Po, Paris. Evidence from Trustlab can be used to assess the statistical quality of survey data (with evidence showing that people who report high trust in surveys also display more trusting behaviours in experimental games) but also to investigate what are the key drivers of trust. Results from Trustlab indicate that government integrity, its responsiveness, openness, and access to quality services are the most important determinants of people’s trust in government (Murtin et al. 2018). The same tools could also be used to explore other aspects of people’s behaviours, such as discounting of future benefits, aversion to risks and uncertainties, and how (perceived) inequalities shape behaviours. But we also need stronger evidence that how people behave in games and experiments is a good guide to how they behave in real life situations.
While research on the causes and consequences of trust is still in its infancy, it is proving to be a rich field of endeavour, with promising insights into our understandings of economic performance and social progress. This is especially important in the context of concerns about “fake news” and of the decline in trust in the media observed in many countries. There are profound consequences for any society if the sources of information cannot be trusted. The ability to have a rational discussion about alternative policies is undermined when there is no minimal agreement about what the “facts” are.
Earlier in this chapter, we identified several correlates of trust. But, to date, we still have an imperfect understanding of causality, and indeed, causality may often run in both directions. A lack of trust in public institutions may hamper their ability to engage in redistribution because there may be a fear that redistribution will go the wrong way, i.e. from the poor to the rich. But high levels of inequality, especially when they cannot be justified, also undermine trust in institutions. Similarly, a well-functioning legal system may enhance trust, while a lack of trust may lead to an overly rule-bounded legal system in which, in the process of following detailed rules, miscarriages of justice may end up undermining trust.
Because trust plays such an important role in economic and social performance, it is urgent to identify the institutions and public policies that will foster its growth. Developing better metrics would enable us to measure our successes in these endeavours.
3.11. Conclusions
All the areas discussed above will require greater research and better data in the years to come. Even for those areas that already feature prominently in ongoing statistical collection – such as vertical inequalities in household economic resources – there are important methodological challenges that stand in the way of adequately capturing underlying realities. Other areas – such as subjective well-being or environmental accounting – have only recently started to be covered by Statistical Offices, and measurement initiatives will need to be strengthened to extend the evidence base needed to improve the quality of these statistics. Other areas yet – such as economic insecurity, inequality of opportunity, trust and resilience – currently lack a foundation in countries’ statistical system. Greater investment is needed in all these areas, as arguably lack of more adequate metrics contributed to inadequate policy decisions.
Notes
← 1. USD 1.9 is the updated value of the earlier USD 1 a day standard for extreme poverty.
← 2. The OECD generally reports its measures of income poverty based on a threshold set at half of median (equivalised) disposable income, i.e. around USD 82 per day for a single mother of two children in the United States (i.e. USD 27 per day per capita). This does not imply that “extreme poverty” (based on the conventional World Bank threshold) has been eradicated in developed countries. Deaton (2018) argues, based on World Bank data, that of the 769 million people who lived on less than USD 1.9 a day in 2013, 3.2 million were in the United States, and 3.3 million in other OECD countries. Edin and Shaeffer (2016), based on data from the US Census Bureau Survey of Income and Program Participation, report that 4.3% of American households with children (and 5.1% of those without children) reported living on less than USD 2 a day per person in 2011 based on their pre-tax money income; and that 1.6% (and 4.3%) were in the same condition based on a welfare metric that included food stamps and other welfare benefits.
← 3. According to Milanovic (2016), the value of the global Gini coefficient (based on household survey data for some 120 countries and 2005 PPP) declined from around 0.72 in 1988 to 0.67 in 1988. Historical estimates of the global Gini coefficient compiled by Bourguignon and Morrison (2002) and by Moatsos et al. (2014) – based on measures of GDP per capita for countries’ mean income, on 1990s PPPs and on measures of within-country inequalities from a variety of sources – show a continuous increase from the 1820s (with Gini values of around 0.50) to the 1950s (0.67) followed by broad stability thereafter.
← 4. As noted by Milanovic, measuring global income inequality is a recent endeavour, with estimates affected by factors such as the quality of income distribution data available for individual countries, the PPP wave used, and whether national-account based corrections for differences in mean incomes between surveys and national accounts are applied or not.
← 5. See OECD Income Distribution Database www.oecd.org/social/income-distribution-database.htm.
← 6. The Stolper-Samuelson theorem (Stolper and Samuelson, 1949) predicted that opening up trade between developed and less developed countries would lower wages in the developed country, especially those of unskilled workers, even taking into account the lower prices that consumers would have to pay.
← 7. Whether these programmes directly improve the well-being of their parents is debatable. The assumptions used in conventional incidence analysis imply that they do, as they consider the costs of these programmes as an income stream accruing to the household participating in them.
← 8. The use of adjusted income as welfare metric raises its own interpretative problems. Poor households in rural areas will not benefit from health and education services that are mainly benefitting richer households in urban neighbourhoods, so that “attributing” these services to them through inadequate techniques would simply overstate their economic well-being. The same upward bias would result from analysis that attributes to end-users the higher costs of health-care services.
← 9. The problems associated with discrepancies between macro (national accounts) and micro measures of mean household income are discussed in the next section of this chapter.
← 10. Capital gains are excluded from the standard definition of household income provided by the UN Canberra Group Handbook on Household Income Statistics, 2nd edition. That said, in standard economic analysis, capital gains would count as “income” in the same way as a dividend does.
← 11. This under-coverage of some hard-to-reach population groups is one explanation of the difference between income measured from surveys and from the national accounts (see below).
← 12. In the System of National Accounts, all household expenditures on durables are recorded as consumption at the time of the purchase.
← 13. Based on experiments carried out in Tanzania (with 8 random samples of 5 000 households each drawn from the same population), Beegle et al. (2012) concluded that, relative to a benchmark household budget survey with a 14-day recall period and a long list of consumption items, reducing the recall period by a week lowered measured mean consumption by 12% percent, while combining a lower recall period and a shorter list of commodity items reduced mean consumption by 28%.
← 14. The OECD has started to collect data on wealth distribution based on its Guidelines for Micro Statistics on Household Wealth (OECD, 2013e). The first wave of the OECD Wealth Distribution Database included 17 countries, the second wave released in 2018 extended the coverage to 27 countries. See www.oecd.org/statistics/guidelines-for-micro-statistics-on-household-wealth-9789264194878-en.htm; and https://stats.oecd.org/Index.aspx?DataSetCode=WEALTH.
← 15. Even the direction of change in wealth inequality may differ according to whether one captures wealth not held directly by the individual. There is not only the problem of lack of disclosure. Sometimes, the trust documents deliberately obscure who is the beneficiary, naming several individuals who might benefit. Only the trustees know who is likely to benefit from the trust. Nevertheless, Kopczuc and Saez (2004) have argued that wealth held in trust is small relative to total household wealth and that its omission does not affect their results on wealth inequalities.
← 16. The OECD and Eurostat, in association with a number of National Statistical Offices, are currently producing experimental estimates of the joint distribution of household income, consumption and wealth, based on the OECD Framework for Statistics on the Joint Distribution of Household Income, Consumption and Wealth www.oecd.org/statistics/framework-for-statistics-on-the-distribution-of-household-income-consumption-and-wealth-9789264194830-en.htm.
← 17. While imputed rents are included in the 2011 “operational definition” of household income proposed by the Canberra Group Handbook (UNECE, 2011), several Statistical Offices in OECD countries (Canada, Korea, New Zealand and the United States) do not routinely include them in their income distribution statistics. Even in countries that do include them, methodological differences (e.g. on the residences included, methods of estimation, types of renters) are so large as to prevent meaningful comparisons across countries.
← 18. This is partly because there are complex issues associated with assessing the general equilibrium incidence of different taxes and subsidies. This is particularly relevant in assessing the distributional impacts of different policies.
← 19. Assessments conducted by the IMF (and the World Bank) are based on the fiscal incidence tools developed by the Commitment to Equity (CEQ) Institute. The CEQ, founded in 2015 at Tulane University, has so far developed tax and benefits models for 43 countries (with work in progress for other 23) based on methodologies described in the CEQ Handbook. See http://commitmentoequity.org/.
← 20. For instance, previous investments also benefitted from the tax cut, implying negative distributional effects with no positive impact on incentives.
← 21. A large group of Keynes’ followers, led by Cambridge economist Nicholas Kaldor, did give primacy to the importance of distribution in the determination of the macro-economic equilibrium. This tradition survives, and in the aftermath of the 2008 crisis, the Commission of Experts on Reforms of the International Monetary and Financial System appointed by the President of the United Nations General Assembly emphasised the role of growing inequality in the crisis. The Report is available as The Stiglitz Report: Reforming the International Monetary and Financial Systems in the Wake of the Global Crisis, New York: The New Press (2010). Published in complex Chinese by Commonwealth Magazine Company, in simplified Chinese by Xinhua, in French by Odile Jacob, in India by Orient Black Swan (2011), in Korean by Dongnyok, in Russian by International Relations Publishing, and in Spanish by RBA.
← 22. Stiglitz provides a theoretical investigation of the relationship between inequality and macro-stability (Stiglitz, 2012a and 2012b). Other empirical and theoretical studies have shown that, other things being equal, more inequality undermines economic performance, leading to lower growth and more instability. Cingano (2014), for example, shows that more inequality is associated with greater inequality of opportunity, which means that children at the bottom are less likely to get the human capital they need to live up to their potential. Of course, some countries, like China, have grown very fast in spite of high levels of inequality; indeed, the high GDP growth rate, with parts of the country “pulling ahead” of the rest, contributed to higher inequality.
← 23. Luck also plays an important role in determining people’s life beyond either “circumstances” and “effort”. Frank (2016) shows how the role of luck becomes more important in winner-takes-all societies, arguing that the tendency for “successful people… to understate luck’s role in their success” makes them less willing to invest in achieving public goods.
← 24. Krueger, statement delivered (in his capacity as Chairman of the Council of Economic Advisors to US President Obama) on 12 January 2012 at the Centre for American Progress. https://milescorak.files.wordpress.com/2012/01/34af5d01.pdf
← 25. Krueger, in his 2012 statement, labelled this the “Great Gatsby” relationship. Chetty and his co-authors have shown that a similar relationship holds when looking across countries in the United States (Chetty et al., 2018).
← 26. A simple interpretation of this relationship is that when the rungs of the ladder are further apart (as they are in societies with greater inequality of outcomes), it is harder to move up the ladder.
← 27. Such long time series are especially important if we want to establish whether income dynamics are Markovian, i.e. whether there are effects that stretch across generations. In the case of Sweden, for which there is some data, there is evidence of such effects (Adermon, Lindhal and Palme, 2016).
← 28. A major determinant of economic insecurity is economic volatility; but the effects on insecurity of a given variability in say GDP may differ, depending not just on the buffers available but also on the structure of the economy. Germany, for example, responded to the 2008 financial crisis with more work-sharing, so that the risks were more effectively shared among workers than in other countries.
← 29. In practice however, the indicators that may be used to monitor some aspects of sustainability (e.g. measures of people’s health status or education to measure human capital) may be the same as those used to monitor current well-being.
← 30. A recent international commission reviewed the evidence and noted that, if the world is to attain the goals it has set for itself of limiting global warming to between 1.5 and 2 degrees Celsius, a tax of at least USD 50 to USD 100 per ton of carbon will have to be imposed (CPLC, 2017).
← 31. One could even say that, along with the theory, they also delivered “its complete mode of use”.
← 32. Within the system of national accounts, output at current prices of financial intermediaries is measured through an indirect measure of the value of intermediation services with no explicit charge provided by banks (FISIM). In practice, this is done by applying the difference between the observed interest rates on loans and a risk-free “reference rate” to the amount of outstanding bank loans, and the difference between the observed interest rates on deposit and the risk-free rate to the amount of bank deposits (Lequiller and Blades, 2014).
← 33. By applying the SEEA framework, monetary and physical data can be combined in a consistent manner for calculating intensity and productivity ratios. Macro-level indicators can also be broken down by industry, allowing to analyse environmental pressures exerted by different industries and consumption behaviours, and to distinguish government responses from those of the business sector or private households.
← 34. See, for example, the physical supply table for air emissions that reports emissions (in tonnes) of 14 substances (ranging from carbon dioxide to ammonia and particulates) from industries (by sector), households (by activity) and landfills. Similarly, the physical asset account for mineral and energy resources reports opening stocks, additions (discoveries, upward reappraisals, reclassifications), reductions (extractions, catastrophic losses, downward reappraisals, reclassifications) and closing stocks of five types of natural resources (oil, natural gas, coal and peat, non-metallic and metallic minerals), with information separately provided for “commercially recoverable resources”, “potentially commercially recoverable resources” and “non-commercial and other known deposits” (United Nations et al., 2014).
← 35. The SEEA Ecosystem Accounts define ecosystems as a “dynamic complex of plant, animal and micro-organism communities and their living environment interacting as functional unit” (United Nations et al., 2014).
← 36. For an overview of the treatment of mineral and energy resources in the SEEA framework and of the challenges related to the valuation of these resources, see www.oecd-ilibrary.org/docserver/3fcfcd7f-en.pdf?expires=1533647411&id=id&accname=guest&checksum=5887745A4C5EC338EABCF35528EFE5B1.
← 37. In the sense of Bourdieu and Passeron (1990).
← 38. According to data from the Comparative Study of Electoral Preferences (CSES), reported in OECD (2017a), the gap in voter turnout, based on self-reports, between people in the bottom and top income quintile across 25 OECD countries, is around 15 points. These measures are likely to underestimate differentials in voting behaviour, as those who did not vote are less likely to report it in surveys.
← 39. Based on data from the OECD Survey of Adult Skills (OECD, 2013f) reported in OECD (2017a).
← 40. So too, the “Voices of the Poor” exercise, to which we referred earlier in this chapter: lacking voice in decisions that affect their lives was one of the important deprivations reported by the poor (Narayan et al., 2000).
← 41. A process is currently in place to develop metrics for monitoring progress in attaining Goal 16 of the UN 2030 agenda, which is about “effective and accountable institutions”. A UN City Group on Governance Statistics (Praia) has also been created to move forward statistical work in this field.
← 42. Some policy-makers argued that saving banks, bankers and their shareholders was necessary to keep the flow of credit going, and that this could be done even without imposing conditions of “good behavior” (e.g. lending) on the banks. Such policies – protecting bankers rather than ordinary citizens – only undermined further trust in government.
← 43. Careful attention, however, needs to be given to the mechanisms by which education builds trust. To the extent that it enhances citizens’ ability to understand the rationale for government actions – a particular policy can be understood as more than a special measure advancing the interest of some group – it is natural that more education enhances trust. But if government policies largely serve the interests of elites, moving more individuals into the elites through education may increase the number of people who support the government, but will do little to improve trust amongst the non-elites. Thus, as we have emphasized throughout this book, a “Beyond GDP” analysis requires more granular data than just “averages”. More generally, correlation is not causation, and there are often multiple interpretations for why one might expect a particular correlation, with markedly different policy implications.