The new data presented in the previous chapter allow for an empirical analysis of the effects of concentration in seed markets. An analysis of determinants of seed prices does not find strong evidence of a harmful impact of market concentration. Using data for the European Union on the introduction of new varieties, an empirical analysis similarly does not find strong evidence of a harmful effect of concentration on innovation.
Concentration in Seed Markets
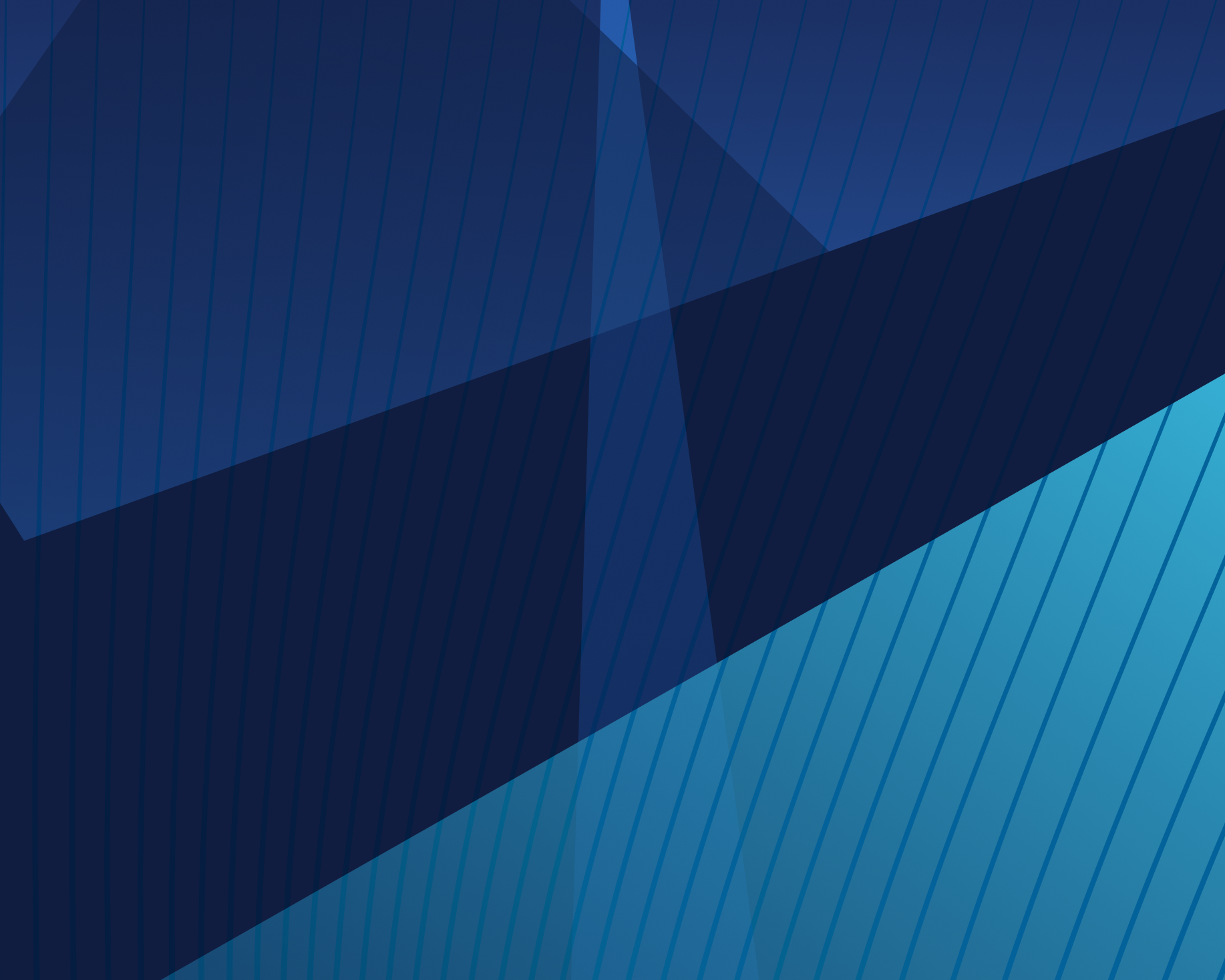
6. New evidence on the effects of concentration in seed markets
Abstract
Chapter 5 presented disaggregated data on market concentration in seed for different crops and countries. This dataset allows an empirical exploration of the effects of market concentration in seed on prices and innovation.
6.1. Market concentration and seed prices
Does market concentration lead to higher prices? At first sight, the data seems to suggest this is the case. Figure 6.1 shows average maize seed prices and market concentration levels for maize seed. There indeed appears to be a positive correlation, with more concentrated markets showing a higher seed price. In addition, price levels appear to be systematically lower in countries where public sector plant breeding institutes are active (Belarus, the Russian Federation, Serbia, and, to a lesser extent, Romania, Ukraine, and Croatia).1
However, when looking at other crops, the picture is more complicated. For rapeseed, there is no clear link between market concentration and seed prices (Figure 6.1). Among the other markets, there is also no clear link between market concentration levels and average prices. This example demonstrates that prices and market concentration do not necessarily correlate in all markets.
A more rigorous analysis is possible through a regression of seed prices on different possible determinants, including market concentration. Table 6.1 shows the outcome of six linear specifications where the average seed price in each market is regressed on measures of market size, market concentration, and a set of other characteristics. All specifications include crop and country fixed effects, which filter out any price effects common to a crop or country (only the crop effects are shown explicitly).
Figure 6.1. Market concentration and seed prices
Note: Countries with public research institutes active in the maize seed market (Belarus, Russian Federation, Serbia, Romania, Ukraine, Croatia) highlighted in grey. The seed price index measures the relative price of seed (per kg) converted at average 2016 exchange rates. Because of data confidentiality, seed prices have been rescaled by a random factor. Hence, absolute levels are not meaningful, but relative comparisons (within a crop) can be made.
Source: OECD analysis using the Kleffmann amis® AgriGlobe® database.
Table 6.1. Determinants of seed prices (linear specification)
Dependent variable: average seed price (USD per kg)
Explanatory variables |
(1) |
(2) |
(3) |
(4) |
(5) |
(6) |
|||||
---|---|---|---|---|---|---|---|---|---|---|---|
Market size (USD mln) |
0.001*** |
0.001*** |
0.001** |
0.001** |
0.0003 |
0.0003 |
|||||
|
(0.0003) |
(0.0003) |
(0.0003) |
(0.0002) |
(0.0003) |
(0.0003) |
|||||
HHI (Value) |
3.335 |
-42.952** |
-39.757** |
||||||||
|
(6.745) |
(19.08) |
(19.579) |
||||||||
C4 (Value) |
-2.56 |
-75.952*** |
-69.082*** |
||||||||
|
(4.786) |
(18.092) |
(21.835) |
||||||||
(HHI)² |
88.269*** |
79.052** |
|||||||||
|
(34.057) |
(34.907) |
|||||||||
(C4)² |
52.447*** |
47.154*** |
|||||||||
|
(12.238) |
(14.216) |
|||||||||
GM used? (Yes = 1, No = 0) |
3.740** |
2.706* |
|||||||||
|
(1.535) |
(1.579) |
|||||||||
Volume share of public breeders |
1.646 |
0.687 |
|||||||||
|
(2.93) |
(3.339) |
|||||||||
Volume share of farm-saved seed |
-0.328 |
-2.53 |
|||||||||
|
(2.35) |
(2.896) |
|||||||||
Crop fixed effects |
(Reference category: wheat and barley) |
||||||||||
Cotton |
11.444*** |
12.669*** |
8.000*** |
10.455*** |
6.303*** |
8.506*** |
|||||
|
(1.78) |
(1.468) |
(2.132) |
(1.105) |
(2.431) |
(1.984) |
|||||
Maize |
5.350*** |
6.006*** |
6.172*** |
6.615*** |
6.233*** |
6.556*** |
|||||
|
(1.006) |
(1.066) |
(0.921) |
(0.931) |
(0.946) |
(0.971) |
|||||
Potato |
-3.386** |
-3.396* |
-2.651* |
-1.617 |
-2.696* |
-1.854 |
|||||
|
(1.708) |
(1.964) |
(1.565) |
(1.804) |
(1.63) |
(1.889) |
|||||
Rapeseed |
15.912*** |
16.500*** |
16.864*** |
17.552*** |
17.089*** |
17.499*** |
|||||
|
(1.111) |
(1.058) |
(0.971) |
(0.937) |
(1.103) |
(1.041) |
|||||
Soybean |
2.579** |
2.776** |
2.802** |
2.779** |
1.09 |
1.524 |
|||||
|
(1.261) |
(1.2) |
(1.158) |
(1.135) |
(1.129) |
(1.291) |
|||||
Sugar beet |
62.360*** |
63.771*** |
62.590*** |
63.832*** |
63.199*** |
63.994*** |
|||||
|
(2.615) |
(2.92) |
(2.145) |
(2.384) |
(2.127) |
(2.282) |
|||||
Sunflower |
11.753*** |
12.858*** |
12.948*** |
12.776*** |
13.863*** |
13.301*** |
|||||
|
(1.283) |
(1.539) |
(1.302) |
(1.387) |
(1.443) |
(1.524) |
|||||
Country fixed effects |
Yes |
Yes |
Yes |
Yes |
Yes |
Yes |
|||||
Constant |
-2.509 |
0.058 |
3.043 |
24.589*** |
0.216 |
20.689** |
|||||
|
(1.984) |
(3.606) |
(2.971) |
(6.861) |
(3.541) |
(9.186) |
|||||
Observations |
87 |
87 |
87 |
87 |
87 |
87 |
|||||
Adjusted R2 |
0.92 |
0.92 |
0.93 |
0.94 |
0.93 |
0.93 |
|||||
F Statistic |
24.346*** (df = 45; 41) |
24.348*** (df = 45; 41) |
25.912*** (df = 46; 40) |
27.884*** (df = 46; 40) |
23.742*** (df = 49; 37) |
25.124*** (df = 49; 37) |
Note: Output of OLS regressions with crop and country fixed effects. Numbers in brackets denote heteroscedasticity-robust standard errors. Significance levels: *p<0.1; **p<0.05; ***p<0.01. HHI (Value), C4 Value, Volume share of public breeders, and Volume share of farm-saved seed all range from 0 to 1.
Source: OECD analysis using the Kleffmann amis®AgriGlobe® database.
The first and second column of Table 6.1 use two different measures of market concentration: the HHI and the C4, which are both defined here in value terms. Alternative specifications with volume-based measures gave practically identical results and are not shown. In the first two specifications, neither of the two concentration measures is statistically significant, and the sign is inconsistent (positive in column 1, negative in column 2). The crop fixed effects, however, do pick up important differences between crops. For instance, these results show that seed prices for cotton are on average USD 9.9 per kg higher than for wheat and barley seed (the reference category used here). Within the country fixed effects (not shown here), most results were small and not statistically significant.
Columns 3 and 4 try to account for potential non-linear effects of market concentration by including a squared term of respectively the HHI and C4 measure. In both cases, both the linear (first-order) and squared (second-order) effect are statistically significant. For the HHI measure, the coefficients imply that moving from a market with five equally large firms (corresponding to a HHI of 0.2) to a market with three equally large firms (corresponding to a HHI of 0.33) would increase the average price by only USD 0.5/kg. However, in more concentrated markets the price effect accelerates: moving from a HHI of 0.33 to a HHI of 0.5 would increase the average price by USD 5.1/kg, all else equal, and the effects accelerate for higher levels. For the C4 measure, the coefficients imply that an increase in the market share held by the four largest firms would decrease the average price as long as the C4 ratio remains below approximately 80%; above that threshold, higher ratios increase prices, although the estimated coefficients imply that the price increases would be modest. Moving from a C4 ratio of 80% to 100% would only increase the average price by around USD 3.5/kg according to these estimates. In other words, these estimates provide contradictory results.
Columns 5 and 6 add an indicator showing whether GM technology is used in the market and the volume shares of public plant breeders and of farm-saved seed, respectively. By themselves, these variables do not appear to exert a major impact on prices; and other coefficients are not affected by the inclusion of these additional controls.
A shortcoming of the analysis in Table 6.1 is that, by construction, the linear specification expects variables to exert the same effect on all crop seed markets, regardless of the initial price level. For instance, the linear specification expects an increase in the volume share of public breeders to have the same price effect in USD/kg regardless of the crop. For several explanatory variables it seems more plausible that the impact would be a proportional increase or decrease of the price, rather than such an absolute change. To analyse this, Table 6.2 resents results of a logarithmic specification.
As the dependent variable is in logarithmic form, the interpretation of the coefficients presented in Table 6.2 requires some care. For explanatory variables which are in logarithmic form (such as market size), the estimated coefficients are elasticities. For instance, a coefficient of 0.12 would imply that an increase by 1% in the explanatory variable leads to an increase of prices by 0.12%. For variables which are expressed as a share (such as the volume share of public breeders), a similar interpretation holds: for an estimated coefficient of -0.76, a one percentage point increase in the volume share of public breeders would decrease prices by around 0.76%. The interpretation is somewhat more complicated for indicator variables which can only take a value of zero or one, as is the case for the GM indicator and the crop and country fixed effects; these are discussed in more detail below.
Table 6.2. Determinants of seed prices (logarithmic specification)
Dependent variable: log (average seed price)
Explanatory variables: |
(1) |
(2) |
(3) |
(4) |
(5) |
(6) |
---|---|---|---|---|---|---|
Log(Market size in mln USD) |
0.122** |
0.129** |
0.140*** |
0.136*** |
||
|
(0.058) |
(0.054) |
(0.049) |
(0.05) |
||
Log(Market volume in tons) |
-0.096* |
-0.088* |
||||
|
(0.052) |
(0.053) |
||||
Log(HHI (Value)) |
0.017 |
-0.062 |
||||
|
(0.137) |
(0.131) |
||||
Log(C4 (Value)) |
0.252 |
0.062 |
0.052 |
-0.49 |
||
|
(0.34) |
(0.358) |
(0.252) |
(0.515) |
||
Log² (C4 (Value)) |
-0.589 |
|||||
|
(0.432) |
|||||
GM used? (Yes = 1, No = 0) |
0.121 |
0.164 |
||||
|
(0.193) |
(0.186) |
||||
Volume share of public breeders |
-0.756*** |
-0.711** |
||||
|
(0.289) |
(0.291) |
||||
Volume share of farm-saved seed |
-1.191*** |
-1.167*** |
||||
|
(0.332) |
(0.352) |
||||
Crop fixed effects |
(Reference category: wheat and barley) |
|||||
Cotton |
4.796*** |
4.756*** |
4.054*** |
4.042*** |
4.336*** |
4.370*** |
|
(0.193) |
(0.178) |
(0.349) |
(0.365) |
(0.196) |
(0.2) |
Maize |
3.654*** |
3.598*** |
3.438*** |
3.419*** |
3.485*** |
3.492*** |
|
(0.107) |
(0.12) |
(0.165) |
(0.173) |
(0.107) |
(0.108) |
Potato |
-0.966*** |
-0.974*** |
-1.332*** |
-1.330*** |
-1.072*** |
-1.122*** |
|
(0.176) |
(0.172) |
(0.093) |
(0.099) |
(0.14) |
(0.151) |
Rapeseed |
4.905*** |
4.864*** |
4.214*** |
4.219*** |
4.750*** |
4.740*** |
|
(0.096) |
(0.097) |
(0.292) |
(0.299) |
(0.116) |
(0.118) |
Soybean |
1.929*** |
1.912*** |
1.743*** |
1.747*** |
1.788*** |
1.784*** |
|
(0.229) |
(0.216) |
(0.258) |
(0.253) |
(0.19) |
(0.182) |
Sugar beet |
6.416*** |
6.316*** |
5.593*** |
5.569*** |
6.188*** |
6.218*** |
|
(0.165) |
(0.188) |
(0.38) |
(0.392) |
(0.169) |
(0.176) |
Sunflower |
4.581*** |
4.502*** |
4.090*** |
4.060*** |
4.346*** |
4.387*** |
|
(0.135) |
(0.164) |
(0.296) |
(0.306) |
(0.178) |
(0.179) |
Country fixed effects |
Yes |
Yes |
Yes |
Yes |
Yes |
Yes |
Constant |
-3.046*** |
-3.026*** |
-0.904 |
-0.904 |
-3.136*** |
-3.238*** |
|
(0.434) |
(0.425) |
(0.746) |
(0.792) |
(0.415) |
(0.415) |
Observations |
87 |
87 |
87 |
87 |
87 |
87 |
Adjusted R2 |
0.97 |
0.97 |
0.97 |
0.97 |
0.98 |
0.98 |
F Statistic |
69.705*** (df = 45; 41) |
70.655*** (df = 45; 41) |
67.673*** (df = 45; 41) |
67.431*** (df = 45; 41) |
78.065*** (df = 48; 38) |
75.617*** (df = 49; 37) |
Note: Log(.) denotes the natural logarithm. Output of OLS regressions with crop and country fixed effects. Numbers in brackets denote heteroscedasticity-robust standard errors. Significance levels: *p<0.1; **p<0.05; ***p<0.01. HHI (Value), C4 Value, Volume share of public breeders, and Volume share of farm-saved seed all range from 0 to 1.
Source: OECD analysis using the Kleffmann amis®AgriGlobe® database.
The first two columns in Table 6.2 show that market concentration measures are not statistically significant. In columns 3 and 4, market volume is used as an alternative measure of market size, to capture the potential effect of economies of scale; however, this change in variable does not seem to affect the estimates of other variables. Column 5 adds variables for GM, public breeding and farm-saved seed. The coefficient on the GM indicator is discussed in more detail below. The estimates imply that a one percentage point increase in the volume share of public plant breeding results in a decrease in prices by around 0.76%, all else equal, while a one percentage point increase in the share of farm-saved seed would reduce prices by 1.2%.
Finally, column 6 adds the square of the log of the C4 indicator, analogously to the inclusion of the squared term in Table 6.1. Neither the linear nor the squared C4 indicator are statistically significant, indicating no clear effect of market concentration on prices. Moreover, the sign and magnitude of the effects is puzzling, as these coefficients would imply that higher market concentration leads to increases in prices as long as the four largest firms have a combined market share below 60%, but that prices would decrease if market concentration rises above this level. Other coefficients remain largely unchanged.
Given a logarithmic formulation, the correct interpretation of coefficients on indicator variables (variables which can only take a value of zero or one) is not trivial. The calculation of the implied percentage change in average seed prices for such variables is detailed in a footnote.2
For the GM indicator, the estimated coefficient is small and has a large standard error, which means the data provides no clear evidence that GM seed is more expensive than non-GM seed. The estimated percentage change in the final specification is +16%, but with a range from -20% to +67%, indicating considerable uncertainty around the estimate.
Table 6.3 shows how prices of seed differ across crops (compared to the reference category of wheat and barley seed), after controlling for all other factors included in the analysis. Estimates are based on the estimated coefficients in the final column of Table 6.2 and reveal large differences in average seed prices between crops, with sugar beet seed almost five hundred times as expensive (per kg) as seed for wheat and barley.
Table 6.3. Crop effects on average seed prices (relative to wheat and barley seed prices)
Multiple at estimated value |
Multiple at lower bound |
Multiple at upper bound |
Significance level of estimated coefficient |
|
---|---|---|---|---|
Cotton |
77.5 |
52.4 |
114.7 |
*** |
Maize |
32.7 |
26.4 |
40.4 |
*** |
Potato |
0.3 |
0.2 |
0.4 |
*** |
Rapeseed |
113.6 |
90.2 |
143.2 |
*** |
Soybean |
5.9 |
4.1 |
8.4 |
*** |
Sugar beet |
494.0 |
349.9 |
697.5 |
*** |
Sunflower |
79.1 |
55.7 |
112.4 |
*** |
Note: This table shows by what multiple the average seed price of crops differ from prices for wheat and barley seed. Estimates derived from the coefficient estimates in the last column of Table 6.2 using the Kennedy transform as detailed in footnote 2. Given their magnitude, effects are shown as multiples, i.e. the price of sunflower seed is on average 75 times higher than that of wheat and barley. Final column shows significance levels of corresponding coefficients in the last column of Table 6.2: *p<0.1; **p<0.05; ***p<0.01.
Source: OECD analysis using the Kleffmann amis®AgriGlobe® database.
Likewise, Figure 6.2 and Table 6.3 show how seed prices differ across countries (compared to Argentina, the reference category), after controlling for all other factors included in the analysis. These estimates are derived from the country effects of the specification in the final column of Table 6.2. As the confidence intervals in Figure 6.2 show, there tends to be considerable uncertainty around the estimated country effects, which means that country rankings are probably not very robust. However, comparing the extremes of the rankings, it does appear that Greece, Slovenia and Portugal, among others, have higher seed prices on average than Australia, the Russian Federation or Belarus. One important limitation in interpreting these country effects is that the analysis does not control for the quality of seed; the country-specific differences in price found here may reflect such quality differences.
Figure 6.2. Country differences in average seed prices
Note: Estimates for Canada not available due to small number of observations. Shaded area shows estimated percentage impact between the boundaries of the 95% confidence interval around the estimated country effect (see main text for methodological notes).
Source: OECD analysis using the Kleffmann amis®AgriGlobe® database.
Table 6.4. Country differences in average seed prices (relative to Argentina)
Percentage change at estimated value |
Percentage change at lower bound |
Percentage change at upper bound |
Significance level of estimated coefficient |
|
---|---|---|---|---|
Australia |
6% |
-34% |
70% |
|
Austria |
160% |
40% |
384% |
*** |
Belarus |
-23% |
-63% |
59% |
|
Belgium |
124% |
23% |
310% |
*** |
Brazil |
30% |
-16% |
101% |
|
Bulgaria |
104% |
17% |
254% |
*** |
Croatia |
150% |
39% |
350% |
*** |
Czech Republic |
102% |
2% |
300% |
** |
Denmark |
173% |
49% |
401% |
*** |
France |
146% |
43% |
326% |
*** |
Germany |
110% |
22% |
262% |
*** |
Greece |
275% |
104% |
588% |
*** |
Hungary |
62% |
-19% |
225% |
|
Indonesia |
48% |
-13% |
151% |
|
Italy |
221% |
88% |
447% |
*** |
Latvia |
191% |
59% |
435% |
*** |
Mexico |
101% |
14% |
253% |
*** |
Netherlands |
177% |
55% |
397% |
*** |
Paraguay |
36% |
-16% |
121% |
|
Philippines |
181% |
62% |
388% |
*** |
Poland |
100% |
9% |
268% |
** |
Portugal |
239% |
82% |
529% |
*** |
Romania |
140% |
39% |
315% |
*** |
Russian Federation |
-10% |
-49% |
60% |
|
Serbia |
133% |
32% |
310% |
*** |
Slovakia |
176% |
45% |
427% |
*** |
Slovenia |
246% |
81% |
559% |
*** |
South Africa |
116% |
29% |
263% |
*** |
Spain |
120% |
35% |
260% |
*** |
Sweden |
149% |
34% |
363% |
*** |
Thailand |
62% |
-6% |
181% |
* |
Turkey |
103% |
11% |
270% |
** |
Ukraine |
21% |
-28% |
105% |
|
United Kingdom |
139% |
36% |
317% |
*** |
United States |
141% |
23% |
373% |
*** |
Uruguay |
26% |
-24% |
108% |
Note: Table shows estimated price differences for each country compared to Argentina. Estimates derived from the country fixed effects corresponding to the last column of Table 6.2, using the Kennedy transform as detailed in footnote 2. Final column shows significance levels of corresponding coefficients in the last column of Table 6.2: *p<0.1; **p<0.05; ***p<0.01.
Source: OECD analysis using the Kleffmann amis®AgriGlobe® database.
A greater presence of public plant breeding seems to reduce average prices according to the estimates in Table 6.2. This effect could reflect competitive pressure from public plant breeders, but it could also reflect a “composition effect” whereby public plant breeders themselves sell seed at lower prices (thus lowering the average seed price in the market) without affecting the prices of private firms.
Summarising the various specifications, the data do not provide clear evidence of a connection between market concentration and seed prices. Table 6.1 found some evidence that above a certain threshold more concentrated markets have higher prices, although the effects appear modest in magnitude; the effect is not found in the alternative specifications of Table 6.2 however, and is even reversed in some specifications.
Overall, the findings of the regression analysis provide no strong evidence of a link between market concentration and average seed prices in the markets studied here.3
6.2. Market concentration and innovation in the European Union
The Kleffmann database by itself does not allow for an analysis of how market concentration affects innovation. In this section, a measure of the rate of innovation in European crop seed markets is constructed to allow for such an analysis.
Data on innovation
Innovation is defined here as the annual number of new varieties introduced in a market, where a market is a combination of a country and a crop (e.g. the French maize seed market), consistent with the definition used earlier in studying market concentration.
There is more than one way to measure the number of new varieties. One possibility would be to use the number of new plant breeders’ rights or patents issued. However, differences between jurisdictions make it hard to compare these numbers. To obtain a comparable measure, the analysis here focuses on the European Union. In the European Union, marketing of seed requires that varieties are registered in the National List of one EU Member State. Hence, the number of new entries on the National List can be used as a measure of innovation. While this approach is not without its shortcomings, it provides a reasonable proxy for innovation, as discussed in more detail in Annex 6.A.4
Table 6.5 shows the median number of new varieties for which registration in the National List was granted between 2013 and 2017.5 Using a median instead of an average avoids distortions in the data caused by years with exceptionally high or low numbers of approved entries to the National List. The table does not include data for cotton, as plant breeding on cotton is relatively rare in the European Union.6 The final two rows of the table compare the EU total for National List entries with EU-wide plant breeders’ rights; the two figures have a strong correlation.
Figure 6.3 and Figure 6.4 visualise these data by crop and by country, respectively. By far the largest number of new varieties is introduced for maize, with more than 700 new varieties per year. Wheat, at slightly above 300 new varieties per year, is a distant second, closely followed by rapeseed and sugar beet. Among the crops included here, the lowest number of new varieties is introduced for soybean, at around 40 per year.7
Italy and France have the highest number of new variety introductions per year (Figure 6.4), driven in particular by high numbers of new maize and wheat varieties. Another crop where specific countries dominate is sugar beet, where Germany, France and Spain together account for about half of new varieties.
Table 6.5. Median number of new varieties in the European Union, 2013-2017
Country |
Measure |
Maize |
Soybean |
Wheat |
Barley |
Rapeseed |
Sunflower |
Potato |
Sugar beet |
Total |
Austria |
NLI |
20.5 |
6.0 |
11.0 |
9.5 |
5.0 |
3.0 |
2.0 |
- |
60.5 |
Belgium |
NLI |
11.0 |
- |
4.0 |
1.5 |
- |
- |
1.0 |
15.0 |
32.5 |
Bulgaria |
NLI |
30.5 |
1.0 |
10.5 |
3.5 |
8.5 |
27.0 |
1.0 |
- |
85.5 |
Croatia |
NLI |
19.0 |
1.0 |
10.0 |
2.0 |
4.0 |
3.0 |
2.0 |
9.0 |
54.0 |
Czech Rep. |
NLI |
36.0 |
1.0 |
15.0 |
11.0 |
13.0 |
3.0 |
4.0 |
14.0 |
101.0 |
Denmark |
NLI |
5.0 |
- |
11.0 |
14.0 |
26.0 |
- |
2.0 |
16.0 |
74.0 |
Estonia |
NLI |
2.5 |
1.0 |
7.0 |
9.0 |
10.0 |
- |
1.0 |
- |
31.5 |
Finland |
NLI |
- |
- |
3.5 |
6.0 |
3.0 |
- |
1.0 |
- |
13.5 |
France |
NLI |
122.0 |
6.0 |
50.0 |
22.0 |
27.0 |
21.0 |
12.0 |
42.0 |
339.0 |
Germany |
NLI |
36.0 |
1.5 |
21.0 |
17.0 |
23.0 |
- |
9.0 |
51.0 |
163.5 |
Hungary |
NLI |
16.0 |
4.0 |
15.0 |
4.0 |
32.0 |
5.0 |
1.5 |
12.0 |
124.0 |
Ireland |
NLI |
2.0 |
- |
2.5 |
3.0 |
- |
- |
4.0 |
- |
11.5 |
Italy |
NLI |
188.0 |
6.0 |
36.0 |
3.5 |
14.0 |
63.0 |
1.0 |
6.5 |
342.0 |
Latvia |
NLI |
1.0 |
- |
3.0 |
3.0 |
5.0 |
- |
4.0 |
- |
16.0 |
Lithuania |
NLI |
12.0 |
1.0 |
12.0 |
9.0 |
14.0 |
- |
- |
13.0 |
61.0 |
Netherlands |
NLI |
34.0 |
- |
5.0 |
2.0 |
- |
- |
35.0 |
- |
173.0 |
Poland |
NLI |
21.0 |
1.5 |
12.0 |
12.0 |
18.0 |
- |
5.0 |
15.0 |
89.5 |
Portugal |
NLI |
22.0 |
- |
- |
- |
- |
10.0 |
1.0 |
- |
41.0 |
Romania |
NLI |
42.0 |
5.0 |
8.0 |
2.0 |
6.0 |
22.0 |
2.0 |
2.0 |
93.0 |
Slovakia |
NLI |
65.0 |
1.5 |
16.0 |
7.0 |
21.0 |
18.0 |
1.0 |
- |
131.5 |
Slovenia |
NLI |
11.0 |
1.0 |
5.5 |
- |
3.0 |
- |
1.0 |
- |
24.0 |
Spain |
NLI |
25.0 |
- |
17.0 |
6.0 |
1.0 |
2.0 |
1.5 |
30.0 |
110.5 |
Sweden |
NLI |
1.5 |
- |
7.0 |
3.0 |
3.0 |
- |
1.0 |
10.0 |
25.5 |
European Union |
Total NLI |
737.0 |
39.5 |
305.0 |
170.0 |
272.5 |
178.0 |
99.0 |
243.5 |
2 311.0 |
European Union |
PBR |
213.0 |
12.0 |
149.0 |
75.0 |
91.0 |
66.0 |
69.0 |
13.0 |
766.0 |
Note: NLI is National List; PBR is Plant breeders’ right. Country data is the median number of annual approved applications to the National List, 2013-2017 (based on grant start date). EU PBR data shows the median number of plant breeders’ rights granted at EU-level over the same period.
Source: OECD analysis using the UPOV PLUTO database (version 16 Feb 2018).
Figure 6.3. Annual number of new varieties in the European Union by crop, 2013-2017
Note: Data shows the sum across EU member states of the median number of annual approved applications to the National List, 2013-2017 (based on grant start date).
Source: OECD analysis using the UPOV PLUTO database (version 16 Feb 2018).
Figure 6.4. Median number of new varieties in the European Union, by country, 2013-2017
Note: Median number of annual approved applications to the National List, 2013-2017 (based on grant start date).
Source: OECD analysis using the UPOV PLUTO database (version 16 Feb 2018).
Market concentration and innovation
Combining the data in Table 6.5 with market data from Kleffmann makes it possible to explore the determinants of the rate of innovation in the EU plant breeding sector, including the potential impact of market concentration. Table 6.6 presents results of a regression analysis where the dependent variable is the logarithm of the median number of new entries on the national list (a logarithmic specification was chosen as it provided the best fit for the data).
Table 6.6. Determinants of innovation
Dependent variable: Log (median number of new entries on the National List, 2013-2017)
Explanatory variables: |
(1) |
(2) |
(3) |
(4) |
(5) |
(6) |
---|---|---|---|---|---|---|
Log(Market size in mln USD) |
0.484*** |
0.473*** |
0.481*** |
0.470*** |
0.446*** |
0.432** |
(0.122) |
(0.128) |
(0.119) |
(0.131) |
(0.172) |
(0.184) |
|
Log(HHI (Value)) |
-0.476 |
0.822 |
0.334 |
|||
(0.35) |
(1.485) |
(1.543) |
||||
Log(C4 (Value)) |
-0.409 |
-0.035 |
-0.556 |
|||
(0.662) |
(1.449) |
(1.663) |
||||
Log²(HHI (Value)) |
0.425 |
0.199 |
||||
(0.438) |
(0.478) |
|||||
Log² (C4 (Value)) |
0.452 |
-0.657 |
||||
(1.518) |
(1.71) |
|||||
Volume share of public breeders |
-1.585 |
-2.083** |
||||
(1.013) |
(0.839) |
|||||
Volume share of farm-saved seed |
0.391 |
0.669 |
||||
(1.118) |
(1.123) |
|||||
Crop fixed effects |
(Reference category: wheat and barley) |
|||||
Maize |
0.253 |
0.125 |
0.335 |
0.126 |
0.065 |
-0.132 |
(0.208) |
(0.27) |
(0.246) |
(0.274) |
(0.355) |
(0.308) |
|
Potato |
-0.185 |
-0.171 |
-0.134 |
-0.149 |
-0.443 |
-0.582 |
(0.196) |
(0.211) |
(0.2) |
(0.235) |
(0.357) |
(0.413) |
|
Rapeseed |
0.388 |
0.283 |
0.464* |
0.288 |
0.16 |
-0.029 |
(0.244) |
(0.236) |
(0.244) |
(0.238) |
(0.315) |
(0.277) |
|
Sugar beet |
1.195*** |
1.001** |
1.243*** |
0.985** |
0.924* |
0.594 |
(0.425) |
(0.4) |
(0.392) |
(0.401) |
(0.501) |
(0.518) |
|
Sunflower |
-0.063 |
-0.24 |
0.03 |
-0.258 |
-0.378 |
-0.62 |
(0.393) |
(0.399) |
(0.377) |
(0.395) |
(0.481) |
(0.445) |
|
Country fixed effects |
Yes |
Yes |
Yes |
Yes |
Yes |
Yes |
Constant |
0.461 |
1.275** |
1.35 |
1.353** |
1.478 |
1.592** |
(0.817) |
(0.528) |
(1.399) |
(0.609) |
(1.368) |
(0.741) |
|
Observations |
52 |
51 |
52 |
51 |
50 |
50 |
Adjusted R2 |
0.42 |
0.38 |
0.41 |
0.35 |
0.34 |
0.33 |
F Statistic |
2.428** (df = 26; 25) |
2.172** (df = 26; 24) |
2.312** (df = 27; 24) |
2.008** (df = 27; 23) |
1.859* (df = 29; 20) |
1.824* (df = 29; 20) |
Note: Log(.) denotes the natural logarithm. Output of OLS regressions with crop and country fixed effects. Numbers in brackets denote heteroscedasticity-robust standard errors. Significance levels: *p<0.1; **p<0.05; ***p<0.01. HHI (Value), C4 Value, Volume share of public breeders, and Volume share of farm-saved seed all range from 0 to 1.
Source: OECD analysis using the Kleffmann amis®AgriGlobe® database.
A first insight regards the role of market size. Across all specifications, the estimated elasticity of innovation with respect to market size is around 0.4-0.5, indicating that a 1% increase in market size increases the innovation rate by around 0.4-0.5%.
The role of market concentration is less clear. In the first two specifications, a negative effect is found, albeit not statistically significant in the case of the second specification. In the remaining specifications a term is added to capture “inverted-U” patterns between concentration and innovation (Aghion et al., 2005[104]). The estimated coefficients are not statistically significant and contradictory. For instance, the pattern implied by the third specification is a U-shaped curve, with increasing market concentration first reducing, then increasing innovation. By contrast, the estimates in the last column would imply an inverse U-shaped pattern. As these coefficients are all imprecisely estimated and varying across specifications, the data hence does not show any strong evidence of a relationship between market concentration and innovation in plant breeding in the dataset used here.
The final two specifications show a negative impact of the volume share of public plant breeding on innovation. The estimates indicate that a one percentage point increase in the share of public breeders reduces the annual number of new varieties by 1.6% to 2%. By contrast, the share of farm-saved seed does not appear to play a major role.
All of the specifications correct for crop and country effects. The coefficients for crop effects in Table 6.6 can be transformed into an estimated percentage change with respect to the reference category (wheat and barley). Figure 6.5 shows these estimates for the second-to-last specification of Table 6.6.8 The data indicates that innovation rates are not meaningfully different between wheat and barley (the reference category) on the one hand and maize or rapeseed on the other. By contrast, sugar beet in most specifications appears to have a higher innovation rate, while sunflower and potato appear to have lower innovation rates. However, all of these effects are estimated with considerable uncertainty.
Figure 6.5. Crop differences in innovation rate
Note: Shaded area shows estimated percentage impact between the boundaries of the 95% confidence interval around the estimated crop effect (see main text for methodological notes).
Source: OECD analysis using the Kleffmann amis®AgriGlobe® database.
Similarly, Figure 6.6 shows estimated country effects, expressed as a percentage change compared to the reference category (Austria). Italy appears as a clear outlier here; the estimated effect implies that Italy’s innovation rate is six times that of Austria, after controlling for all other effects in the analysis. This estimate is based on a single data point (for maize) only and should be interpreted with caution. Slovakia and the Netherlands also appear to have a higher innovation rate compared with Austria. At the other end of the spectrum, innovation rates appear lower in Belgium, Poland, Latvia, Sweden, and Spain, although in the latter case the effect is measured imprecisely.
Figure 6.6. Country differences in innovation rate
Note: Shaded area shows estimated percentage impact between the boundaries of the 95% confidence interval around the estimated country effect (see main text for methodological notes). Estimate for Italy truncated here at 500%; interval maximum is at 804%.
Source: OECD analysis using the Kleffmann amis®AgriGlobe® database.
The analysis has several limitations which need to be kept in mind. First, the overall number of observations (52) is relatively limited. Second, the measure of innovation used here is an imperfect indicator of actual innovative activity in these markets. For instance, if crops are suitable for many agro-ecological zones of the European Union but are only registered in a single country, the measure would simultaneously overstate innovation in some markets and understate it in others. Despite those potential shortcomings, the analysis presented here shows that there is at least no obvious relationship between market concentration and innovation in EU crop seed markets.
6.3. Conclusion
This chapter used disaggregated data on market concentration in seed to study effects on prices and innovation. The statistical analysis did not find clear evidence of an effect of market concentration on seed prices, after controlling for crop-specific and country-specific effects on prices. There appear to be some structural differences in price levels between countries which are not explained by market concentration levels; these could be due to quality differences and the presence of public plant breeders in some markets.
Regarding innovation, a statistical analysis for the European Union similarly did not find any clear evidence of a negative impact of market concentration on innovation, or of an “inverted-U” shaped relationship. The analysis did uncover a strong positive effect of market size, with a 1% increase in total market size leading to a 0.4-0.5% increase in the number of new varieties introduced per year, on average. Moreover, the rate of innovation appears lower when public breeders account for a larger share of the market. The analysis of innovation effects needs to be interpreted with caution, as the measure used here is only an imperfect approximation of the “true” degree of innovation in plant breeding.
The statistical analyses hence do not show a clear-cut relationship between market concentration on the one hand and prices and innovation on the other. However, the analysis here is necessarily limited and could not take into account other factors such as multimarket contact, the possibility for outsider firms to enter a market, quality differences affecting price levels, or the R&D pipelines of different firms. On the other hand, in some cases the statistical analyses did suggest systematic differences between countries in terms of price levels or innovation rates, apart from potential effects of market concentration. This raises the possibility that other policies besides competition policy could affect the performance of seed markets. The potential for such complementary policy options is discussed in the next chapter, after a review of how competition authorities have evaluated the recent mergers.
Annex 6.A. Data on innovation in plant breeding in the European Union
Measuring innovation in plant breeding
For the empirical analysis, the rate of innovation in plant breeding is defined as the annual number of new varieties introduced in a market, where a market is defined as a combination of a country and a crop (e.g. the French maize seed market), and where the number of new varieties is measured by entries on the National List of EU Member States. This section explains the choice of methodology and the underlying dataset.
One approach to count the number of new varieties would be to count the number of plant breeders’ rights or patents on plant varieties issued each year. However, there are several problems with this approach. First, jurisdictions differ in how intellectual property rights protection for new varieties is organised. In some countries (e.g. the United States, Australia), plant varieties can also be protected under the regular system of utility patents. While plant breeders’ rights are monitored by UPOV, the International Union for the Protection of New Varieties of Plants, there is no similar harmonised database for utility patents on plant varieties, making it difficult to measure the number of new plant varieties across countries. For some varieties, breeders may also choose not to obtain a plant breeders’ right.9
Moreover, within the European Union, plant varieties in the European Union can be protected by a plant breeders’ right either at the national level or at the European level, but not both at the same time. This creates a difficulty in accurately measuring the number of new varieties at the national level. Using only country-level plant breeders’ rights would grossly understate the rate of innovation, given the importance of EU-level plant breeders’ rights. On the other hand, EU-wide plant breeders’ rights cannot easily be allocated to specific countries. Performing an analysis only at the aggregate level for the European Union is a possibility, but this assumes that the EU seed market is effectively a single market, which seems implausible given variations in agro-ecological conditions across the European Union; moreover, it greatly reduces the number of observations.
An alternative measure of new varieties can be based on entries to a National List. Several UPOV member states operate such a list, which includes all varieties authorised to be traded commercially. This is in particular the case for EU Member states. All varieties to be sold in the European Union need to be registered in the National List of one Member State. The registration process requires testing whether the new variety is distinct, uniform and stable (DUS-testing) and, for agricultural varieties, whether the new variety has a sufficient value for cultivation or use (VCU-testing).
The number of new varieties on the National List has its shortcomings as a measure of innovation. First, the release of a new variety is not only a measure of innovation but also reflects different company strategies; some firms may focus on providing a wide portfolio of “niche” varieties while others prefer to focus on providing a smaller number of varieties bred for broad adaptation. Second, not all varieties will be commercially successful, even after passing the VCU tests. However, VCU tests do provide some guarantee that the new variety is a valuable addition.10
Another concern is that, as registration in a single country is sufficient for marketing throughout the European Union, the number of new entries likely overstates innovation in some markets and understates it in others. However, because of the need for VCU testing, it seems plausible that plant breeders will apply for registration in the National List of the country for which the variety is best adapted. This makes the number of new entries in the National List a reasonable, though imperfect, proxy for the rate of innovation in a country.
In the empirical analysis, the innovation measure uses the number of approved applications to the National List, instead of just the number of applications. A drawback of this choice is that for countries and crops with relatively low numbers of applications, this measure may indicate zero innovations – which means it is not possible to distinguish between crops without breeder interest and crops where breeders have tried in vain to innovate. In addition, the process of DUS and VCU testing typically takes two years, which adds an extra time lag to the already long R&D cycle in plant breeding. However, using the number of applications could be misleading, as there is anecdotal evidence that plant breeders often see the National List application process as the de facto last stage of plant breeding research, where public tests substitute for testing by the plant breeder. Suggestive evidence for this effect is found in the relatively large number of National List applications withdrawn by plant breeders, presumably as early test results disappoint. An alternative explanation is that testing for the National List provides an opportunity for plant breeders to see the performance of their varieties against the competition; for internal trials, plant breeders may not always have the latest competitor varieties available. Regardless of the precise mechanism, the large number of withdrawn applications suggests that approved applications are a better measure of useful innovation in plant varieties.
Data for the analysis was obtained from the PLUTO plant variety database maintained by UPOV, the International Union for the Protection of New Varieties of Plants. The PLUTO database contains information on plant breeders’ rights, plant patents and national listings of varieties for 4 420 different species (including ornamental species) in 59 UPOV members, including the European Union, as well as the varieties certified by the OECD Seed Schemes. As of January 2018, the database contained over 800 000 entries. The species with the most entries are maize (15% of the total), wheat (5%), barley (4%), roses (4%), sunflower (3%) and tomatoes (3%). In addition to the European Union (17% of all entries) and the certified varieties of the OECD Seed Schemes (9%), the countries with the greatest number of entries are the Netherlands (8%), France (7%), the United States (6%), the United Kingdom (4%) and Germany (4%).11 While the UPOV Convention only regulates intellectual property rights in plant varieties independent of whether they are approved for commercial use in a country, the PLUTO database nevertheless contains information provided by the UPOV members on varieties included in their National List.
The list of crop seed markets included in the analysis is similar to that studied in the previous chapter (maize, soybean, wheat and barley, rapeseed, sunflower, potato, sugar beet, cotton). For cereals, our analysis here distinguishes wheat and barley. Annex Table 6.A.1 provides the definitions of these crops in terms of the UPOV codes in the PLUTO database (which correspond to Latin names of the crops).
Annex Table 6.A.1. Crop definitions used
Crop |
UPOV code |
---|---|
Maize |
ZEAAA_MAY |
Soybean |
GLYCI_MAX |
Wheat |
TRITI_AES, TRITI_AES_AES, TRITI_TUR_DUR |
Barley |
HORDE_VUL |
Rapeseed |
BRASS_NAP, BRASS_NAP_NUS |
Sunflower |
HLNTS_ANN |
Potato |
SOLAN_TUB |
Sugar beet |
BETAA_VUL_GVS, BETAA_VUL_GV |
Cotton |
GOSSY_HIR |
Trends in innovation per crop, 1996-2017
Using the number of new approved varieties in the National List per crop allows for an analysis of trends in innovation in the European Union over the past two decades (1996-2017). As shown in the following figures, there is no clear overall trend of increasing or decreasing innovation, apart from the increase generated by accession of new EU Members.
Figure 6.A.1 displays data for maize. Over the period, France and Italy have remained the largest sources of new varieties. The rate of new introductions appears stable over time in most countries.
Annex Figure 6.A.1. New varieties of maize, 1996-2017
Note: Annual number of approved applications to the National List (based on grant start date). For newer EU Member States, data is included starting from the year of accession to the European Union.
Source: OECD analysis using the UPOV PLUTO database (version 16 Feb 2018).
For soybean, shown in Figure 6.A.2, there is some evidence of a decline over time in new varieties registered in Italy. At the same time, the number of new varieties appears to increase in France and Austria.
Annex Figure 6.A.2. New varieties of soybean, 1996-2017
Note: Annual number of approved applications to the National List (based on grant start date). For newer EU Member States, data is included starting from the year of accession to the European Union.
Source: OECD analysis using the UPOV PLUTO database (version 16 Feb 2018).
For wheat (Figure 6.A.3) and barley (Figure 6.A.4), new varieties are introduced in several countries, and the rate of innovation tends to fluctuate over time. In both cases, France is an important source of new varieties. Leaving aside the strong increase over time as new countries join the European Union, the data shows no clear pattern of an increase or decrease in innovation.
Figure 6.A.5 shows the introduction of new varieties of rapeseed. There is evidence of an increase in innovation, especially in the United Kingdom.
For sunflower seed, Figure 6.A.6 shows a shifting geographical pattern over time. While France and Spain were important sources of new varieties in the 1990s, their contribution has since decreased, while Italy has increased its rate of new variety introductions. New Member states (in particular Bulgaria and Romania) have been active as well. Data for 2017 seems to show a decline, although this may be due to delays in data submission to UPOV.
Annex Figure 6.A.3. New varieties of wheat, 1996-2017
Note: Annual number of approved applications to the National List (based on grant start date). For newer EU Member States, data is included starting from the year of accession to the European Union.
Source: OECD analysis using the UPOV PLUTO database (version 16 Feb 2018).
Annex Figure 6.A.4. New varieties of barley, 1996-2017
Note: Annual number of approved applications to the National List (based on grant start date). For newer EU Member States, data is included starting from the year of accession to the European Union.
Source: OECD analysis using the UPOV PLUTO database (version 16 Feb 2018).
Annex Figure 6.A.5. New varieties of rapeseed, 1996-2017
Note: Annual number of approved applications to the National List (based on grant start date). For newer EU Member States, data is included starting from the year of accession to the European Union.
Source: OECD analysis using the UPOV PLUTO database (version 16 Feb 2018).
Annex Figure 6.A.6. New varieties of sunflower, 1996-2017
Note: Annual number of approved applications to the National List (based on grant start date). For newer EU Member States, data is included starting from the year of accession to the European Union.
Source: OECD analysis using the UPOV PLUTO database (version 16 Feb 2018).
New variety introductions for potato are shown in Annex Figure 6.A.7, showing a strong increase over time in new varieties introduced in the Netherlands, while the rate of innovation remains broadly stable in Germany and France. Despite an initial increase in variety registrations in new member states around 2004, the contribution of these countries (especially the Czech Republic and Poland) appears to have waned over time.
Finally, France, Germany and Spain account for most of the new varieties of sugar beet (Annex Figure 6.A.8), with evidence of an increase in new introductions over time.
Annex Figure 6.A.7. New varieties of potato, 1996-2017
Note: Annual number of approved applications to the National List (based on grant start date). For newer EU Member States, data is included starting from the year of accession to the European Union.
Source: OECD analysis using the UPOV PLUTO database (version 16 Feb 2018).
Annex Figure 6.A.8. New varieties of sugar beet, 1996-2017
Note: Annual number of approved applications to the National List (based on grant start date). For newer EU Member States, data is included starting from the year of accession to the European Union.
Source: OECD analysis using the UPOV PLUTO database (version 16 Feb 2018).
Notes
← 1. The countries listed are those where at least one public sector research institute was included among the ten largest firms in the market. The Kleffmann database is based on the ownership of varieties; it is possible that multiplication and distribution are outsourced to the private sector in these markets.
← 2. For an indicator variable in a logarithmic regression, the impact of moving from zero to one is given by where is the true (population) value of the coefficient. However, as this expression is nonlinear, Jensen’s inequality implies that substituting the estimated value in this formula would give a biased estimate of the percentage change, even if itself is an unbiased estimator of . Assuming errors are lognormally distributed, Kennedy (1981[232]) proposed using where is the estimated variance of . Although this formula is not exact, it provides a good approximation (Van Garderen and Shah, 2002[233]) and is therefore the approach followed here. Constructing exact confidence intervals is difficult given the small sample size (Giles, 2011[234]). Instead, the bounds shown in the table are obtained by using Kennedy’s transformation to the bounds of the 95% confidence interval for . While this is not an exact confidence interval for the percentage change, it gives some indication of the uncertainty around the estimated percentage change.
← 3. As mentioned in Chapter 5, there is a considerable degree of multimarket contact between seed firms, which in other industries has been found to lead to higher prices. Unfortunately, the current dataset makes it difficult to evaluate the impact on seed prices, as the dataset does not have full coverage across crops and countries. Hence, any empirical measure of whether firms have contact across different markets would tend to understate the true level of multimarket contact.
← 4. Data used in this analysis comes from the PLUTO plant variety database maintained by UPOV, the International Union for the Protection of New Varieties of Plants (see the annex to this chapter for details).
← 5. For most observations, the measure used is the year of the grant start date from the UPOV database; missing values for this variable were replaced by the year of the grant publication date where available.
← 6. The median number of approved entries across the European Union was three per year (one in Bulgaria, two in Spain).
← 7. A more detailed overview over time, by crop and by country, is provided Annex 6.A.
← 8. The final two specifications are to be preferred, as they both find evidence for an effect of the volume share of public plant breeders on innovation. Between these two specifications, the second-to-last specification has a higher adjusted R² and is therefore chosen here.
← 9. This could be the case, for instance, if the plant variety has a rapid turnover, which reduces the usefulness of obtaining intellectual property rights protection; or in the case of a hybrid where biology provides a degree of intellectual property protection.
← 10. For a discussion of breeding progress as measured in official trials in Germany, see, for example, Laidig et al. (2014[239]) and Laidig et al. (2017[252]).
← 11. One limitation is that contributing data to the UPOV database is voluntary; UPOV members are not required to provide data or, when they do provide data, they are not required to provide information on all items. As a result, not all countries are equally represented in the database.