This chapter examines education-related inequalities in health status and its determinants across OECD and European countries. The chapter starts with the brief discussion on the links between health and employment to show that being in good health is instrumental in achieving good labour market outcomes. The chapter then analyses the extent to which exposure to behavioural risk factors (overweight, smoking and heavy drinking) differs across European and OECD countries and, within countries, across the social spectrum. Lastly, education-related health inequalities are investigated considering three health variables (self-assessed health status, limitations in daily activities and multiple chronic conditions), and patterns of health inequalities across European and OECD countries are analysed.
Health for Everyone?
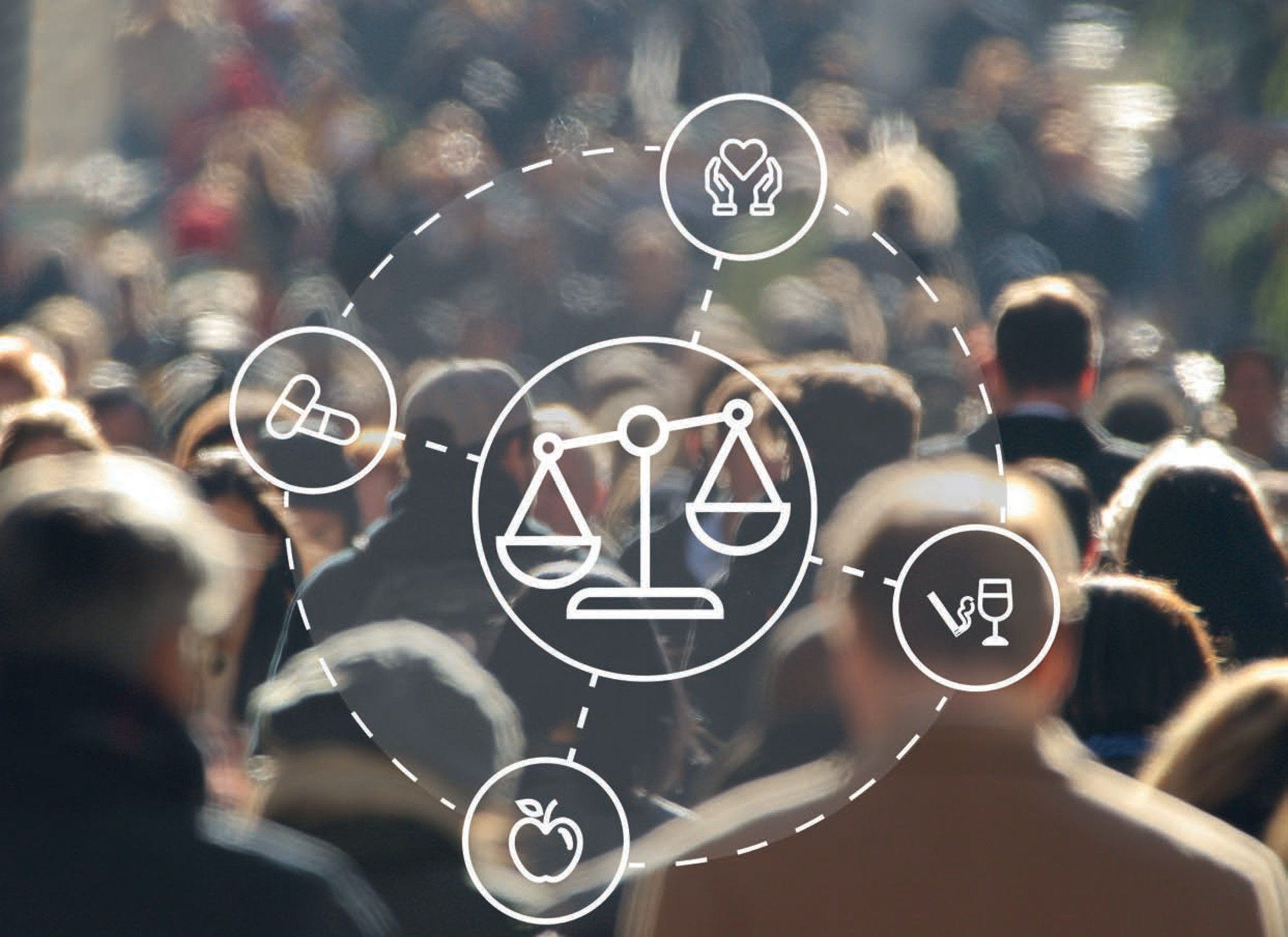
2. Inequalities in health and its determinants
Abstract
Note by Turkey: The information in this document with reference to “Cyprus” relates to the southern part of the Island. There is no single authority representing both Turkish and Greek Cypriot people on the Island. Turkey recognises the Turkish Republic of Northern Cyprus (TRNC). Until a lasting and equitable solution is found within the context of the United Nations, Turkey shall preserve its position concerning the “Cyprus issue”.
Note by all the European Union Member States of the OECD and the European Union: The Republic of Cyprus is recognised by all members of the United Nations with the exception of Turkey. The information in this document relates to the area under the effective control of the Government of the Republic of Cyprus.
2.1. Introduction
Health is one of the main ingredients of a good life. Not only does a good health status improve economic standing through its impact on labour market outcomes, but it also affect individuals’ well-being. However, not everyone has the same opportunity to lead a healthy life. Large differences in health exist between and within countries. Indeed, there is a large social gradient in health outcomes for which the odds are consistently stacked in favour of those better off (Marmot and Brunner, 2005[1]; Marmot et al., 1991[2]; Marmot and Wilkinson, 2006[3]). The least well-off and the least educated are, for example, less likely to be in good health and have a higher risk of dying prematurely than those who face more favourable socio-economic circumstances. A greater prevalence of risky health behaviour among people with a lower level of education contribute to poorer health outcomes and higher mortality rates. Less educated people are, for example, more likely to smoke and be overweight than their counterparts with higher levels of education (Cutler and Lleras-Muney, 2006[4]; OECD, 2016[5]).
This chapter examines education-related inequalities in health status and its determinants across OECD and European countries. It starts with a discussion of the links between health and employment, assessing the extent of the detrimental labour market impacts of ill health (2.2). Based on data from national health surveys, it then turns to an analysis of inequalities in behavioural risk factors including overweight, smoking and heavy drinking (2.3), and investigates education-related health inequalities considering three health variables (self-assessed health status, limitations in daily activities and multiple chronic conditions) (2.4). The chapter concludes by assessing whether some countries systematically display larger inequalities in health and its determinants (2.5).
2.2. Poor health outcomes are detrimental to labour market participation
Being in good health is instrumental in achieving good labour market outcomes. By reducing the individual’s capacity to work long hours, a deteriorated health status decreases the chance of getting into employment and of being productive at work. As shown by a large body of evidence, illness and disability also increase the risk of job loss and early retirement and reduce the earning capacity (Orchard, 2015[6]; James, Devaux and Sassi, 2017[7]). Across Europe, for example, people who have at least two chronic diseases have a 30% reduced probability of being in employment than those without any chronic conditions (OECD, 2016[5]).
The lower labour force participation for people in poor health and their reduced productivity reinforce health inequalities within and between countries. Indeed, and as demonstrated by previous studies on social determinants of health (Marmot and Brunner, 2005[1]), the least well-off and the least educated are less likely to be in good health, and are more likely to be exposed to risk factors detrimental to their health (see Sections 2.3 and 2.4 respectively). This subsequently lowers their employment prospects, while at the same time unemployment worsens mental and physical health. This two-way causal relationship is well documented (see Box 2.1). Assessing the extent of the detrimental labour market impacts of ill health can encourage policy makers to take more decisive action seeking to break the vicious circle between ill health, risk factors and poor labour market outcomes.
Box 2.1. The two-way causal relationship between health and employment
The relationship between health and employment runs not only from work to health, but also from health to work.
On one hand, the literature provides evidence that work impacts health. Employment (e.g. employment status, working hours) and working conditions (e.g. job decision latitude, job demand, and job strains) influence people’s physical and mental health (James, Devaux and Sassi, 2017[7]).
On the other hand, several recent studies, based on longitudinal data, provide evidence of the impact of the health status on employment prospects (OECD, 2017[8]; Devaux and Sassi, 2013[9]; OECD, 2016[5]). Chronic diseases (such as cardiovascular diseases, respiratory problems, diabetes, and serious mental health conditions) as well as risk factors (smoking, obesity) have important labour market impacts in terms of reduced employment, earlier retirement, higher number of sick leave and lower income (as summarised in the table below).
Using longitudinal data, (OECD, 2016[5]) shows for example that the employment rate of people aged 50‑59 who have one or more chronic diseases is lower than that of people who do not suffer from any disease. The same is true for people who are obese or smokers. In addition, people aged 50‑59 suffering from severe depression are more than twice as likely to leave the labour market (OECD, 2016[5]). The table below provides a brief summary of some of the health effects on labour market outcomes.
Summary of the impact of health on labour market outcomes
|
|
Employment probability |
Wages |
Sick leave |
Early retirement |
---|---|---|---|---|---|
Risk factors |
Obesity |
Negative effect |
Negative effect |
Positive effect |
Mixed findings |
Smoking |
Negative effect |
Negative effect |
Positive effect |
Positive effect |
|
Harmful alcohol use |
Mixed findings |
Mixed findings |
Mixed findings |
Mixed findings |
|
Chronic diseases |
Cardiovascular diseases |
Negative effect |
Negative effect |
Mixed findings |
Mixed findings |
Diabetes |
Negative effect |
Negative effect |
Positive effect |
Mixed findings |
Source: Adapted from Devaux and Sassi (2015[10]).
More recently, the Preventing Ageing Unequally (OECD, 2017[8]) report, using longudinal data from European and other OECD countries, showed that inequalities in education, health, employment and earnings reinforce each other and evolve over the life course. Findings confirm that, at all ages, men and women in bad health work less and earn less when they work. Over the whole career, bad health reduces lifetime earnings by 33% and 17% for men with low and high levels of education, respectively (with smaller effects for women).
This section analyses the relationship between health and labour market outcomes and compares the magnitude of the association across European and OECD countries. It uses micro-level data from European and national surveys (Box 2.2 provides the main characteristics of the surveys used in the report). These surveys ask respondents to report their employment status and absenteeism linked to health problems, which provides a unique opportunity to explore the links between health and labour market outcomes across countries. This section explores the association between poor health and the probability of being in employment or absent using logistic estimations (Box 2.3). Table 2.1 describes the variables used in this chapter, in particular one which summarises the information on a person’s health status by counting the number of deteriorated health indicators.
Box 2.2. Surveys used in the report
The European Health Interview Survey (EHIS-2)
The European Health Interview Survey is coordinated by Statistical Office of the European Union (Eurostat). The second wave was conducted between 2013 and 2015 in all 28 EU Member States, Iceland and Norway. It is a general population survey that provides information on health status, health determinants, and health care activities in the European Union.
The Canadian Community Health Survey (CCHS)
The Canadian Community Health Survey is coordinated by Statistics Canada. This cross-sectional survey was conducted in 2015‑16. It collects information related to health status, health care utilisation and health determinants for the Canadian population. The CCHS covers the population living in the ten provinces and the three territories.
The Chilean National Socio-Economic Characterisation Survey (CASEN)
The Chilean National Socioeconomic Characterisation Survey is managed by the Ministry of Social Development. The survey was conducted in 2017 to collect a range of information on education, employment, income and health status. The sample of CASEN is representative for the national population.
The Medical Expenditure Panel Survey 2016 (MEPS)
The Medical Expenditure Panel Survey is managed by the Agency for Healthcare Research and Quality. It is a set of large-scale surveys of families and individuals, their medical providers, and employers across the United States. MEPS is the most complete source of data on the cost and use of health care and health insurance coverage in the United States.
2.2.1. Everywhere, ill health is associated with low employment rates
Employment rates measure the extent to which available labour resources (people available to work) are being used. Employment rates are sensitive to the economic cycle in a country, and are also affected by governments’ higher education and income support policies. Cross-country differences in employment rates thus reflect structural differences in national labour markets. By way of context, Figure 2.1 presents the employment rates estimated in the different surveys used in the report. Greece and Croatia display the lowest employment rates (49% and 54% respectively), followed by some Southern and Central European Countries (Spain, Italy, Bulgaria, Slovenia, Portugal, Poland and the Slovak Republic) and Chile with employment rates between 59% and 64% of the working age population. Germany, the United Sates, Estonia, and some Nordic countries (Iceland, Norway and Sweden) are at the other end of the scale with rates above 75%. On average across European and OECD countries, 67% of the working age population is in employment.
Table 2.1. Description of variables used in this section and coverage of the population
Dependant variables |
Self-declared labour status: dummy variable identifying individuals in employment. |
|
Self-declared absence from work due to health problems: dummy variable identifying, among the employed, those who have had at least one absence from work due to illness in the past 12 months. |
Explanatory variables |
Number of deteriorated health indicators: an ordinal variable based on four dummy variables identifying (a) people reporting a poor self-assessed health status (very poor, poor and fair health), (b) those reporting being “severely limited” and “limited” in daily activities, (c) those who declare they have suffered from depression in the past 12 months (d) people declaring two or more chronic conditions. For EHIS, (c) and (d) are based on a list of 14 chronic variables people can declare they suffer from (see Eurostat (2013[11]). The number of the deteriorated health indicators variable counts the number of dimensions according to which a person is deemed in poor health per these four dummy variables. If a person is deemed in poor health on any of the four dimensions the value will be 1, 2 if they are in poor health according to any combination of 2 poor health dummies. The maximum value is 4 and zero reflects the best possible health. The higher the number is, the worse the person’s health is expected to be. In this section, unless otherwise indicated, people deemed “in poor health” have one or more deteriorated health indicators. |
Control variables |
Age, sex, household income, education, marital status, degree of urbanisation, and citizenship. |
Country coverage and exclusions |
United States, Canada, Chile, 28 EHIS countries covered. Ireland is excluded in both the employment and absenteeism models because of the poor quality of the employment status variable. Romania is excluded from the absenteeism model due to the poor quality of data on absence from work. |
Population covered |
The age was restricted to the working age population (people who are 18 and older and younger than 65). |
Source: Authors. The description of variables in this table refers to EHIS used for 28 of the 31 countries studied. Relevant descriptive statistics and additional explanations about variables used for other countries surveys are available in the annex of this chapter (Annex Table 2.A.1). In particular, for Chile the number of deteriorated health indicators is slightly underestimated due to the absence of questions on chronic diseases.
Figure 2.1. Employment rates across European and OECD countries, 2014

Note: The working age population in European and National Survey refers to people aged 18‑64. EHIS-based estimates of labour force participation of the working age population are very aligned with those of the European Labour Force Survey (ELFS).
Source: National health survey data.
Box 2.3. Method – Logistic estimation
The predicted probability to be in employment (or to be absent from work among employed people) is estimated using a logit model.
The binary variable , representing the probability to be employed over the past twelve months of the survey (or to be absent from work), is explained by the unobserved latent variable with :
Where [1]
The latent variable is made dependent on the respondent’s health status () and all other individuals’ characteristics () which refer to control variables such as age, sex, household income, education, marital status, degree of urbanisation, and citizenship. , β and μ are parameter vectors, and ε an error term.
The multivariate analyses help isolate the impact of health, once other structural differences among population groups have been adjusted for. For poor and good health status, Equation [1] is used to generate predicted values of . Estimations are produced for each country, while estimations for the average is a simple average of country specific estimations.
People in poor health have a lower probability of being employed
Across countries on average, and everything else being equal, the labour market participation rates of people who have no deteriorated health indicators and those with at least one differ by 8 percentage points (Figure 2.2). In this section, unless otherwise mentioned, the term “in poor health” will be used to describe those with at least one deteriorated health indicator. Box 2.4 highlights relevant results from analyses carried out using other specifications of the health variable.
In all countries, and as in previous studies (Orchard, 2015[6]; Knebelmann and Prinz, 2016[12]; OECD, 2016[5]), ill health translates into lower employment, but the scale of the problem varies considerably across countries (Figure 2.2). Romania, Norway and Bulgaria stand out as countries where the difference in labour market participation between those is good and poor health is much larger than on average (19, 16, and 14 percentage points, respectively). However, in these countries, the proportion of the population in poor health is relatively small (20%, 33% and 29%, respectively).
Portugal, Finland and Latvia present an opposite pattern, where the share of the population in poor health is comparatively high (53%, 59% and 56%, respectively) but the gap in employment rates between those in good and poor health relatively small (4, 6 and 2 percentage points).
Sweden and Germany stand out as well-performing countries where despite fairly high proportions of the population in poor health (42% in Sweden, and 52% in Germany), the gap in employment rates between those in poor and good health is small and the overall employment rate of the population is high.
Differences in labour market participation rates between people in good and bad health relate to country-specific labour market and health policies. General and specific activation labour market policies can for example facilitate access to paid work for people in poor health. In Sweden, active labour policies and employment protection are found to increase the opportunities for people with chronic illness to remain in work (Burström et al., 2005[13]). Policies encouraging employers to remove physical barriers to work, providing equal training opportunities for people in poor health, providing flexible work arrangements, or reinforcing employment protection regulations might also reduce differences in labour market participation rates between people in good and poor health. At the same time, public health policies designed to better prevent and manage chronic conditions at work can also make the workforce healthier and more productive, reducing differences in labour market participation. In Belgium, for example, prevention advisers give guidance to workplaces on psychological well-being and support the preparation of risk assessment plans to reduce stress and improve working conditions (OECD/EU, 2018[14]). Such actions support workers experiencing mental ill health to stay in work and maximise opportunities and fulfilment for employees.
Figure 2.2. Probability of being in employment among the working-age population, by health status, European and OECD countries

Note: Data from 29 European countries, Canada, Chile and the United States. Results correspond to predicted probabilities estimated with logit models (all else being equal) (see Box 2.3). The dots refer to the probability of being in employment by health status. The size of the lower dot reflects the proportion of people in poor health in the working-age population (slightly underestimated for Chile). The difference in the probability of being employed between people in good and poor health is significant at the 95% confidence level in all countries except Croatia, Latvia, Luxembourg and Sweden.
Source: OECD estimates based on national health survey data.
In the absence of ill health, labour market participation could increase by 3 percentage points
Across European and OECD countries, the labour market participation rates could increase by 3 percentage points in the absence of ill health. This estimate, which does not factor in labour market dynamics, is simply computed as the difference between the estimated employment rate if everyone was in good health and the actual employment rate. Figure 2.3 shows that the impact of poor health on labour market participation is smallest in Sweden, Latvia, Italy and Croatia (with an impact of around 1 percentage point) and greatest in Chile and Norway. In these countries, labour market participation rates could increase by around 5 percentage points if everyone was in good health.
Box 2.4. Using and combining different health variables
European and national health surveys used in the report contain several self-reported health status indicators (self-assessed health status, limitations in daily activities, multi-morbidity, and depression).
All the analyses were conducted separately on each health indicator, leading to similar results:
The labour market participation rates of people in good and bad self-assessed-health differ by 12 percentage points. While people aged between 18‑64 in good health have a 70% chance of being employed, the predicted probability for those in poor health is only 58%.
People suffering from depression also have a considerable lower chance of being employed: the labour market participation rate of people with depression is 15 percentage points lower than for those who do not suffer from this condition.
The four health variables reflect different dimensions of health which may be cumulated by individuals or not. To reduce the impact of cultural bias of self-reported data (notably for the self-assessed health status) and to capture the diversity of health issues individuals might face, a variable reflecting the number of deteriorated health indicators was created (Table 2.1). A person’s probability of being employed decreases as the number of deteriorated health indicators they face increases. Across countries, 70% of those who face no health problem are predicted to be employed, versus 68% of people with one deteriorated indicator of health. People with two deteriorated indicators of health are 8 percentage points less likely to work than people who face no health problem. Only 54% of the people with three deteriorated indicators of health are employed versus 43% of the people with the worse health (having four deteriorated health indicators).
Figure 2.3. Impact of poor health on labour market participation, European and OECD countries

Note: Data from 29 European countries, Canada, Chile and the United States. The impact of poor health on labour market participation is the difference between the estimated employment rate if everyone was in good health (people have none of the four health issues) and the actual weighted employment rate.
Source: OECD estimates based on national health survey data.
2.2.2. Absenteeism is significantly higher among those with higher numbers of deteriorated health indicators
When they have a job, people in poor health are less productive at work. A simple and intuitive way to assess the loss of productivity related to illness is to contrast the rates of absenteeism among people in good and bad health everything else being equal using logistic regressions1.
Across countries, 34% of workers declare having missed work in the past year due to illness. The proportion of the working population declaring at least one absence from work due to illness varies across countries: from nearly one in two in Nordic countries (Finland, Denmark, Norway and Iceland) and Germany to less than one in five in Cyprus, Bulgaria, Greece and Croatia.
The probability to be absent from work differs considerably with a person’s health status. Nearly half of those in poor health are likely to be absent from work at least once in the year (47%) versus 26% of those who face no health problem (Figure 2.4). The gap in leave-taking behaviour between those in good and poor health also varies across countries. The gap is relatively small in Germany, the United States, Finland, Iceland and Norway (where taking time from work because of illness is very common) as well as Malta, Canada, Hungary, Lithuania and Estonia (where it is not). Countries where this gap is most marked include Spain, Poland, the Slovak Republic, the United Kingdom, Luxembourg, Denmark, and Belgium. Here, the differences in the probability of being absent from work between people with at least one health issue and those with no health issue is 23 percentage points or more.
Figure 2.4. Probability to be absent from work among employed people by health status, European and OECD countries

Note: Data from 28 European countries, Canada and the United States. Results correspond to predicted probabilities estimated with logit models (all else being equal) (see Box 2.3). The dots refer to the probability of being absent from work by health status, while the size of the upper dot refers to the proportion of the working population in poor health (slightly underestimated for Chile). Difference between those in good health and bad health is significant at the 95% confidence level for all countries.
Source: OECD estimates based on national health survey data.
2.2.3. The chance of being fully productive is higher among people in good health than among those in poor health
A somewhat simplistic but intuitive postulate is to deem that people fulfil their productive potential if they are employed and, in that case, if they do not take time off work due to illness during the year. Based on the previous analysis, the probability of being fully productive among people in good health and in poor health can be estimated by combining the likelihood of being employed with that of being absent from work.
By this metric, results show that people in good health have a greater chance of being fully productive than people in poor health. On average, around half of the people in good health reach their full productive potential across European and OECD countries (51%). By contrast, only 33% of people with at least one deteriorated health indicator in poor health are fully productive (Figure 2.5).
Figure 2.5. Labour market outcomes of the working-age population in good and poor health, European and OECD countries

Note: Data from 28 European countries, Canada and the United States. Working but absent includes employed people who said they were absent from work at least once in the previous year for a health reason.
Source: OECD estimates based on national health survey data.
This information can also be used to assess and compare the magnitude of the impact of ill health on the labour market across countries. For each country, following the same line of argument, the burden of ill health corresponds to the gap between the proportion of the population which would be fully productive if everyone was in good health (or if people in poor health were as engaged in the labour market as those in good health) and the actual proportion of the population fully productive. Figure 2.6 compares the potential increase in fully productive population under this assumption across countries2.
On average across European and OECD countries, an additional 7.4 percentage points of the working age population could be fully productive. Overall, countries with the lowest burden of ill health are Malta, Greece, Italy and Cyprus. In these countries, the fully productive active population could increase by 5 percentage points (or less) in the absence of ill health. At the other end of the scale, in Luxembourg, Denmark, Latvia and the United Kingdom the fully productive active population could potentially grow by 9 percentage points or more. In most of these countries, the share of the working age population in poor health is above average.
Country-specific labour market policies (such as activation labour market policies, or the scope of compensated sick leave and the generosity of the benefit schemes) and health policies (such as public health actions to support workers experiencing chronic ill health stay in work) contribute to explaining these cross-country differences. The present analysis could serve as a starting point to identify which sets of policies overall help sustaining a more healthy and productive active population.
Figure 2.6. Increase in fully productive active population, European and OECD countries

Note: Data from 28 European countries, Canada and the United States. The ranking of countries is based on the increase (in percentage points) in the proportion of fully productive people if everyone was in good health. This estimate is simply computed as the difference between the estimated proportion of people working and not being absent from work if everyone was in good health and the actual proportion of people working and not being absent from work (from the survey).
Source: OECD estimates based on national health survey data.
Caution should, however, be exerted in the interpretation of these results as a high labour market participation of people in poor health is not an end objective per se. Presenteeism, which describes the situation when workers go to work while sick and unable to work effectively due to their illness, is also an important challenge across European and OECD countries. According to the European Working Condition Survey (EWCS), 42.5% of employees in the EU reported that they worked while being sick in 2015. Presenteeism is undesirable for employers and employees alike: it is associated with lower productivity, and has long-term effects on future health. Skagen and Collins (2016[16]) found that presenteeism increased the risk for future sickness absence and decreased self-rated health (Skagen and Collins, 2016[15]). Over the long term, presenteeism can result in increased welfare payments for disability and sick leave. According to the European Agency for Safety and Health at Work, presenteeism costs organisations more than sickness absence.
2.3. Behavioural risk factors are unequally distributed across socio-economic groups
Environmental factors (for example exposure to pollution, poor working and living conditions, violence), behavioural and lifestyle factors (exercise, diet, smoking, and alcohol consumption) and a broad range of socio-economic factors (income, education, social status and networks, gender, etc.) each can have a direct impact on a person’s health status. For instance, smoking and lack of physical exercise directly affect the risk of illness or death, as do poor working and living conditions (Marmot and Wilkinson, 2006[3]). Outdoor air pollution, for example, can cause respiratory diseases, lung cancer, and cardio-vascular diseases (OECD, 2017[16]).
Importantly, the degree of exposure of an individual to many of these determinants is strongly correlated with − and shaped by − social circumstances. Difficult material circumstances often results in poor living conditions and limits the ability to pay for healthy food and other health-enhancing goods and services. Less educated people are more likely to have a poor working environment, with high exposure to physical pain and mental health risk factors (Siegrist, Montano and Hoven, 2014[17]). They are also less informed about the health impacts of their behavioural factors or able to act upon this information. Related to this, they are more likely to smoke and be overweight than their counterparts with higher levels of education (Cutler and Lleras-Muney, 2006[4]; OECD, 2016[5]). In sum, many determinants are unequally distributed along the social spectrum, reinforce each other, and increase the disadvantages and vulnerability of the less well-off (CSDH, 2008[18]).
Among health determinants, lifestyle plays an important role in explaining population health. For example, while at population-level around half of the decline in mortality by cardiovascular diseases in the United Kingdom between 2000 and 2007 can be attributed to improvement in treatment, the reduction in risk factors accounts for an additional third of the mortality decline and it is much more important in explaining improvements among the lower income groups (Bajekal et al., 2012[19]).
Table 2.2. Data used to analyse behavioural risk factors
|
Description of variables and coverage on the basis of EHIS-2 |
---|---|
Dependent variables |
Smoking status: dummy variable identifying daily and occasional smokers versus non-smoker. |
Obese and overweight: dummy variable identifying respondents with BMI>= 25 versus those with BMI < 25. BMI is calculated based on self-declared height and weight. |
|
Alcohol consumption: dummy variable identifying people with heavy drinking behaviour versus those having light and moderate drinking behaviour. Women are heavy drinkers if they consume on one day more than 20 grams of alcohol. Men are heavy drinkers if they drink on one day more 40 grams. |
|
Explanatory variables |
Education is a categorical variable reflecting three different levels of education: 1. less than primary, primary and lower secondary level (ISCED 0‑2); 2. upper secondary and post-secondary non-tertiary level (ISCED 3‑4); 3. tertiary level (ISCED 5‑8). |
Standardisation for |
Age |
Country coverage and exclusions |
United States, Canada and 30 EHIS countries. For France, the Netherlands, Italy and the United States data on alcohol consumption is not available. No data on risk factors in the Chile survey. |
Covered population |
The population aged 18 years and above. |
Note: The description of variables in this table refers to EHIS used for 30 of the 32 countries studied. Relevant descriptive statistics and additional explanations about variables used for other countries surveys are available in the annex of this chapter (Annex Table 2.A.3).
Using an approach generally privileged by public health specialists, this section presents new evidence on the distribution of behavioural risk factors along the socio-economic spectrum. To summarise the methodological approach:
Table 2.2 presents the variables used in this section.
Education level is used to identify social groups with increased risk of smoking, overweight, and heavy drinking, and it will also be the marker used for health inequalities (Section 2.4). Education has the advantage of being stable beyond early adulthood and it is less likely to be influenced by disease or other shocks than income. In addition, the level of education is a good predictor of the behavioural risk factors under consideration as well as health outcomes. Finally it is well correlated with other social determinants of behavioural risk factor and health including better jobs and working conditions – and income (Shavers, 2007[20]; Devaux and Sassi, 2013[9]).
As the prevalence, distribution and relationship with socio-economic status of various behavioural risk factors differ considerably across gender, analyses are thus undertaken separately for men and women.
Inequalities are described by contrasting the indirectly age-standardised probabilities of the high and low education groups (for an explanation see Box 1.2 in the first chapter of the report)3. The summary measures used to capture and compare inequalities at population level are the slope index of inequalities (SII) and relative index of inequalities RII (Box 2.5). Given the general emphasis of this report on absolute inequalities, SIIs are presented and discussed in the body of the chapter and RIIs can be found in the Annex tables at the end of the chapter.
Box 2.5. The Slope Index of Inequality and the Relative Index of Inequality
To assess the magnitude of health-related inequalities in countries, slope index of inequalities (SII) and relative indexes of inequality (RII) are calculated. SIIs and RIIs are summary measures of inequalities often favoured by public health specialists and epidemiologists for a number of reasons.
First, compared with simple ratios or differences between the advantaged and disadvantaged socio-economic groups in the variable of interest (e.g. smoking, being in poor health), RIIs and SIIs take into account both the relative size of the groups obtaining a given level of education in a country and the association between the variable of interest and education for each education group. SIIs and RIIs are based on logistic regression estimates of the variable of interest for every education group adjusted for age and sex. In essence, SIIs measure the difference between the estimated values of the variable of interest of the person with the lowest and highest education level in the population (for a more detailed description see WHO (2017[21]). The relative index of inequalities is then the ratio between the rate for the least educated person and the rate for the most educated person.
Second, the interpretation of RIIs and SIIs is quite straightforward and somewhat easier than for other measures of socio-economic inequalities (including for example the concentration index). If there is no inequality, SII takes the value zero. Greater absolute values indicate higher levels of inequality (positive values indicate a higher concentration of the indicator among the disadvantaged and negative values indicate a higher concentration of the indicator among the advantaged). The interpretation of RIIs is similar to the relative risk. A large score on the RII implies large health differences between the highest and lowest education groups. For example, an RII of 1.5 implies that the least educated people are 50% more likely to assess their health as poor than the most educated people.
Source and more details: Schneider (2005[22]) and WHO (2017[21]).
2.3.1. Poor health behaviour is more prevalent among the disadvantaged
This section reviews in turn inequalities in overweight and obesity, in smoking and heavy alcohol consumption.
Everywhere, the least educated women are more likely to be overweight
Overweight and obesity, which are known risk factors for numerous health problems (including for example hypertension, diabetes, cardiovascular diseases and some forms of cancer) has increased in many European and OECD countries over the past decades (OECD, 2017[23]; OECD/EU, 2018[14]). The prevalence of overweight and obesity is generally higher among men than women. On average across countries, 60% of men were overweight compared to 47% of women. Among men, the prevalence varies from 53% in France, Italy and the Netherlands to more than 67% in Croatia and the United States (see Annex Table 2.A.3). Among women, the prevalence ranges between less than 40% in Italy, Cyprus, and Luxembourg and 55% or more in the United States and Malta.
In all countries, the most educated women are less likely to be overweight than the least educated (Figure 2.7). On average across all countries, 52% of women with less than a high school degree are overweight or obese compared to 36% of those with tertiary education. Slovenia, Luxembourg, Spain, France, Croatia, Portugal, and Austria display the largest gap, with differences of more than 20 percentage points. In Latvia, Malta, Finland, Canada and Norway these gaps are relatively small (10 percentage points or less).
Taking into account the entire distribution of education levels of the female population, the overall gradient of inequality is significant in all countries. Absolute education-related inequalities to the detriment of the least educated women are highest in Luxembourg, Spain and Slovenia. The lowest inequalities can be observed in Latvia, Malta and Canada (Table 2.3).
Figure 2.7. Age-standardised probability to be overweight by education level, women

Note: Data from 30 European countries, Canada and the United States. Results correspond to the age-standardised probabilities estimated with logit models. Difference between education groups is significant at the 95% confidence level for all countries, except Malta.
Source: OECD estimates based on national health survey data.
The differences in the probability of being overweight between education groups are smaller for men than for women (Figure 2.8). On average across European and OECD countries, the probabilities of being overweight display inequalities to the detriment of the least educated men: 58% of men with the lowest level of education are overweight or obese compared to 54% of those with the highest level. The largest gaps between education levels are found in Luxembourg, Sweden, Spain and Denmark with differences of more than 10 percentage points. In the United States, Canada, Lithuania, Romania, Iceland and Bulgaria, this gap is smaller (less than 2 percentage points). In Latvia, Poland, Slovenia, Cyprus, Hungary, Estonia and Croatia men with the highest level of education have a higher risk of being overweight than those with the lowest level of education.
Figure 2.8. Age-standardised probability to be overweight by education level, men

Note: Data from 30 European countries, Canada and the United States. Results correspond to the age-standardised probabilities estimated with logit models. Difference between education groups is significant at the 95% confidence level for Denmark, France, Germany, Greece, Italy, Luxembourg, the Netherlands, Norway, Portugal, Spain, Sweden, the United Kingdom and Canada.
Source: OECD estimates based on national health survey data.
Computed over the whole population, inequalities to the disadvantage of the least educated men are smaller than those for women, but not significant in 13 out of 32 countries. Absolute education related-inequalities are highest in Luxembourg, Denmark and Sweden (Table 2.3). Canada and the United States, display the smallest absolute education-related inequalities, followed by Portugal and Finland. In Poland, the gradient is reverse: the likelihood of being overweight and obese rises with educational attainment.
Interestingly, Luxembourg, France, Spain, Italy and Denmark are high inequality countries to the detriment of the least educated people among both men and women.
Table 2.3. Absolute education-related inequality in overweight, women and men
|
…decreases when education decreases |
…does not differ across education groups |
…increases when education decreases |
---|---|---|---|
Women – The probability of being overweight… |
- |
- |
LVA, MLT, CAN, NOR, FIN, POL, SVK, ROU, BGR, HUN, LTU, GBR, EST, CZE, CYP, ISL, NLD, SWE, DEU, IRL, HRV, USA, DNK, ITA, GRC, AUT, BEL, PRT, FRA, SVN, ESP, LUX |
Men – The probability of being overweight… |
POL |
BGR, CYP, CZE, EST, HRV, HUN, IRL, ISL, LTU, LVA, MLT, ROU, SVN |
CAN, USA, PRT, FIN, BEL, SVK, GBR, NOR, AUT, GRC, DEU, FRA, ITA, NLD, ESP, SWE, DNK, LUX |
Note: Within each cell, countries are ranked from lowest to highest degree of inequality using the SII.
Source: OECD estimates based on national health survey data.
Overall, the results found here are generally aligned with (Devaux and Sassi, 2013[9]), which, across 11 OECD countries, found larger education-related inequalities in overweight in women than in men. Within Europe, their study showed that the largest inequalities existed in France, Sweden and Austria among men and in Spain, Italy and France among women. These countries are ranked similarly in Table 2.3.
Gender differences in education-related inequalities in overweight might relate to the fact that highly educated women are more concerned about weight control than men with the same level of education, notably because obesity has more adverse impact on labour market outcomes among women than men. Other possible explanations relate to the pattern of physical activity, where low-paid job for women are less physically demanding than those taken up by men (Devaux and Sassi, 2013[9]).
In most EU and OECD countries, the people with the lowest level of education are more likely to smoke
Tobacco consumption, which is the largest avoidable health risk in OECD countries, has been decreasing over the past decade from 23% in 2006 to 18% in 2017 (OECD Health Statistics 2019). Sill, tobacco consumption is a major public health issue worldwide. In European and OECD countries, men smoke more than women. In total, nearly one in three men were daily or occasional smokers compared to one in five women (see Annex Table 2.A.4). Among men, smoking rates vary from more than 40% in Romania, Greece, Lithuania, Latvia, Cyprus, and Bulgaria to 23% (or less) in Northern European countries (Sweden, Iceland, Norway, Finland, Denmark, and the United Kingdom) as well as Canada and the United States. Among women, the prevalence is highest in Croatia, Austria, Greece, and Bulgaria (above 25%). Smoking rates are comparably low in the United States, Portugal, Lithuania, and Romania (below 15%).
Practically everywhere across European and OECD countries, men and women of low education are more likely to smoke than the ones with higher education (Figure 2.9 and Figure 2.10). On average, 24% of the least educated women smoke compared to 12% of the most educated women (Figure 2.9). The gaps in smoking rates are the largest in the Czech Republic, Austria, Norway, the Slovak Republic, Hungary, Belgium and Ireland with differences of more than 17 percentage points. By contrast, these gaps are smaller than 5 percentage points in Bulgaria, Portugal, Greece, and Italy. Only Romania and Cyprus display a reverse pattern: Here, the most educated women are more likely to smoke than the least educated.
Figure 2.9. Age-standardised probability of smoking by education level, women

Note: Data from 30 European countries, Canada and the United States. Results correspond to age-standardised probabilities estimated with logit models. Difference between education groups is significant at the 95% confidence level for all countries except Bulgaria, Cyprus, Greece and Portugal.
Source: OECD estimates based on national health survey data.
When the entire population is taken into account, inequalities in smoking among women are to the detriment of the least educated in a majority of countries. The overall gradient of education-related inequality in smoking, measured with the slope index of inequality that takes into account the full distribution across education groups, is significant, to the detriment of the least educated women, in 26 out of 32 countries. Inequalities are comparably large in Hungary and Ireland (Table 2.4). They are relatively small in Italy, Spain, Lithuania, France and the United States. In three countries (Romania, Portugal, and Cyprus), the gradient is reverse and significant. In other words, as education increases overall, so does the likelihood to smoke.
Social disparities in smoking are larger among men and more clearly play in favour of the most educated (Figure 2.10). On average across all countries, 39% of the least educated men smoke compared to 20% of the most educated men. The largest gaps are found in Hungary, Lithuania, Latvia, Belgium, Estonia, Poland and the Slovak Republic, with differences of more than 23 percentage points between the least and the most educated men. The smallest gaps can be observed in Romania, Greece and Italy with differences of less than 12 percentage points.
The overall gradient of absolute education-related inequality in smoking is significant in all countries. It shows a higher degree of inequalities in Lithuania, Hungary and Latvia, whereas inequalities are smaller in Romania, Greece, Italy, Sweden, France, Germany, and the United States (Table 2.4).
Figure 2.10. Age-standardised probability of smoking by education level, men

Note: Data from 30 European countries, Canada and the United States. Results correspond to the age-standardised probabilities estimated with logit models. Difference between education groups is significant at the 95% confidence level for all countries and the average.
Source: OECD estimates based on national health survey data.
Table 2.4. Absolute education-related inequality in smoking
|
…decreases when education decreases |
…does not differ across education groups |
…increases when education decreases |
---|---|---|---|
Women – The probability of smoking… |
PRT, ROU, CYP |
BGR, GRC, HRV |
ITA, ESP, LTU, FRA, USA, SVN, DNK, POL, MLT, EST, LVA, DEU, CZE, FIN, GBR, NLD, CAN, SWE, AUT, LUX, SVK, ISL, BEL, NOR, IRL, HUN |
Men – The probability of smoking… |
- |
- |
ROU, GRC, ITA, SWE, FRA, DEU, USA, CYP, HRV, PRT, ESP, CAN, NLD, SVN, FIN, GBR, DNK, ISL, BGR, AUT, LUX, MLT, NOR, CZE, IRL, POL, SVK, EST, BEL, LVA, HUN, LTU |
Note: Within each cell, countries are ranked from lowest to highest degree of inequality using the SII.
Source: OECD estimates based on national health survey data.
Cross-country variation in the risk of smoking by education level has been explored in previous research. Huijts et al., 2017[25] show among a pool sample of men and women that the risk of being a daily smoker is higher when respondents’ level of education is lower in each country. Large education-related inequalities in smoking in favour of the most educated people have been found in Northern European countries, while a reverse gradient could be observed for Southern European countries (Huisman, Kunst and Mackenbach, 2005[24]). Less recently, Schaap (2010[25]) showed that socio-economic inequalities in smoking were larger in Northern and Eastern European countries (Schaap, 2010[25]).
The results described in this chapter are generally in line with these studies showing that some Northern European countries (Ireland, and Norway) and Central and Eastern European countries (Hungary, the Slovak Republic, and Estonia) are high inequality countries in smoking to the detriment of the least educated people. Results also support a reverse gradient among women in some Southern European countries (including Portugal, and Cyprus).
While tobacco consumption is an addictive behaviour, differences in health literacy may also partly explain why people of lower education smoke more often than others. The least educated are less informed about the risks of smoking and are less able to act upon this information. People with low education have significantly higher initiation rates and are less likely to stop smoking than people with higher levels of education (OECD, 2017[16]). In addition, education may provide the necessary resources (both cultural and psychosocial) to cope with adverse personal events in a more healthy way than through smoking (Huisman, Kunst and Mackenbach, 2005[24]).
There is no clear social gradient in heavy drinking in the majority of countries
Heavy alcohol consumption (i.e. having a daily amount of pure alcohol of 20 grams or more for women, and 40 grams or more for men) increases the risk of harmful consequences in terms of both morbidity and mortality4 (Sassi, 2015[26]). Rates of heavy drinking display a large degree of variation across countries, and are generally more common among men than women. On average across European and OECD countries, 5% of men report heavy drinking compared to 3% among women (Annex Table 2.A.5). Among men, the United Kingdom, Romania, and Portugal have the highest proportion of heavy drinkers (above 10%), while these shares stand only at 1% in Cyprus and Iceland. For women, the share of heavy alcohol consumers is highest in the United Kingdom (17%), Ireland and Belgium (7‑8%). By comparison, in Croatia and Estonia no heavy female drinkers were included in the EHIS-2 sample.
Contrary to the risk of smoking and of being overweight, the social gradient in heavy alcohol consumption is not clear (Figure 2.11). For women, the differences in heavy drinking between the least and most educated population groups are only significant in nine countries: in Lithuania, Portugal, Romania and Canada the least educated women drink more; in Austria, Belgium, Germany, Luxembourg and the United Kingdom the most educated women have a higher risk of heavy drinking (Annex Table 2.A.5).
At population level, heavy drinking is a greater problem as education decreases for women in three countries (Lithuania, Romania and Canada). In seven countries, better education is significantly associated with increased heavy drinking overall (Austria, Germany, Norway, Denmark, Belgium, the United Kingdom and Luxembourg) (Table 2.5). In the remaining 17 countries, there is no social gradient in heavy drinking.
Figure 2.11. Age-standardised probability to be a heavy drinker by education level, women

Note: Data from 27 European countries and Canada. Results correspond to the age-standardised probabilities estimated with logit models. Difference between education groups is significant at the 95% confidence level for Austria, Belgium, Germany, Lithuania, Luxembourg, Portugal, Romania, the United Kingdom and Canada.
Source: OECD calculations based on national health surveys.
For men, the gradient is more consistent across countries and in the expected direction. In 25 countries, the highest educated men are less at risk of heavy drinking compared to those with the lowest level of education (although the relationship between education and heavy drinking is significant in only eight countries – see Annex Table 2.A.5). Lithuania, Romania, Portugal, the Slovak Republic, Belgium and Slovenia present the largest gap in heavy drinking between education levels – more than 6 percentage points (Figure 2.12).
For men, at population level, heavy drinking becomes less frequent as education rises in 13 countries. Inequalities are higher in Portugal, Lithuania, and Romania and smaller in Canada and Spain (Table 2.5). The social gradient is not significant in 15 countries.
Figure 2.12. Age-standardised probability to be heavy drinker by education level, men

Note: Data from 27 European countries and Canada. Results correspond to the age-standardised probabilities estimated with logit models. Difference between education groups is significant at the 95% confidence level for Belgium, Bulgaria, Estonia, Hungary, Lithuania, Luxembourg, Poland, Portugal, Romania, Slovak Republic, Slovenia, Spain and Canada.
Source: OECD calculations based on national health surveys.
Table 2.5. Absolute education-related inequality in heavy drinking
|
…decreases when education decreases |
…does not differ across education groups |
…increases when education decreases |
---|---|---|---|
Women – The probability of heavy drinking… |
AUT, DEU, NOR, DNK, BEL, GBR, LUX |
CYP, SVN, HUN, FIN, EST, BGR, PRT, CZE, ISL, MLT, SVK, GRC, POL, ESP, LVA, IRL, SWE |
CAN, ROU, LTU |
Men – The probability of heavy drinking… |
- |
IRL, CZE, GBR, ISL, GRC, AUT, NOR, DNK, SWE, FIN, MLT, HRV, CYP, POL, DEU |
CAN, ESP, BGR, LUX, LVA, EST, SVN, HUN, SVK, BEL, ROU, LTU, PRT |
Note: Within each cell, countries are ranked from lowest to highest degree of inequality using the SII.
Source: OECD estimates based on national health survey data.
The difference of patterns in the social gradient in drinking behaviour between genders has already been demonstrated elsewhere. OECD (2015) showed that higher educated women are more likely to be heavy drinkers than their counterparts in lower education groups, while the reverse pattern is found for men in seven countries (least educated men are more at risk of heavy drinking) (Sassi, 2015[26]). The reverse social gradient among women is also confirmed by a meta-analysis covering 33 countries (Grittner et al., 2013[27]). Most plausibly, for women with higher education, drinking behaviour is associated with a wider range of social activities. They might have more opportunities of socialising and going out with colleagues compared to women with a lower level of education. It might also be possible that women with higher education drink more heavily because they take better paid jobs which involves higher degrees of responsibility and level of stress (Sassi, 2015[26]).
2.3.2. Some countries concentrate inequalities in behavioural risk factors
The final question is whether patterns emerge for some countries when considering jointly the degrees of inequalities in the various behavioural risk factors described above. The grouping presented in Figure 2.13 results from ranking each country’s level of inequality for each risk factors for men and women separately based on the rank of the SII. For each risk factor, it allows for an identification of countries with higher, lower and intermediate levels of inequality.
Across European and OECD countries, the highest inequalities in risk factors to the detriment of the least educated people can be observed in Belgium, Luxembourg, Hungary, the Netherlands, Lithuania, the Slovak Republic, Austria, and Slovenia. By contrast, inequalities are comparably low in Cyprus, Poland, Romania, Malta, Croatia, Bulgaria and Greece.
Figure 2.13 also permits to distinguish three groups of countries:
In the first group (e.g., Norway, Iceland, the Czech Republic, Hungary and Ireland) considerable inequalities in smoking to the detriment of the least educated exist but inequalities in overweight and heavy drinking are less strong.
The second group of countries (e.g., Denmark, Spain, Italy, France, and Greece) shows the opposite pattern: inequalities in overweight strongly disadvantage the least educated people but inequalities in smoking and heavy drinking are less strong.
The last group of countries (Romania and Lithuania) combines high inequalities in heavy drinking to the detriment of the least educated people (women and men) and less strong inequalities in smoking and overweight.
Figure 2.13. Summary of inequalities in risk factors

Note: The countries have been ranked from most unequal to least unequal. The grouping results from ranking each countries’ level of inequality for each risk factors for men and women separately based on the rank of the SII. The sum of the six ranks is used to identify countries with higher, lower and intermediate levels of inequality in behavioural risk factor. For each indicator, countries were clustered into three groups of roughly equivalent size. Light/medium/dark blue indicate low/intermediate/high levels of inequality to the detriment of the poor/less educated for a given indicator. A white cell indicates no significant inequality. Countries with inequalities to the detriment of the rich/higher educated were ranked in the category of lower levels of inequality (cells are marked with #).
Source: OECD estimates based on national health survey data.
2.4. Socio-economic inequalities in health outcomes
Health is one of the main ingredients of a good life. In their meta-analysis of 29 studies, Ngamaba et al (2017), for example, show that better health is consistently associated with greater well-being and life satisfaction (Ngamaba, Panagioti and Armitage, 2017[28]). A good health status also improves economic standing through its impact on the labour market outcomes (see Section 2.2).
However, not everyone has the same opportunity to lead a healthy life. Large differences in health exist between and within countries. Indeed, there is a large social gradient in health outcomes for which the odds are consistently stacked in favour of those better off (Marmot and Brunner, 2005[1]; Marmot et al., 1991[2]; Marmot and Wilkinson, 2006[3]). The least well-off and the least educated are, for example, less likely to be in good health and have a higher risk of dying prematurely than those who face more favourable socio-economic circumstances. The socio-economic gradient in health status exist in practically all countries (Murtin et al., 2017[29]; Balaj et al., 2017[30]; Leão et al., 2018[31]).
Assessing the magnitude of health inequalities within and between OECD countries is of particular importance given that reducing health inequalities is often a stated objective of countries health policies. As highlighted in Chapter 1, reducing health inequalities is also germane to the overall agenda to make societies more inclusive. As an illustration, investing in people’s health is a central recommendation in OECD’s Framework for Policy Action on Inclusive Growth which aims to help governments to make economic growth more inclusive, in a way that creates opportunities for all groups of the population and distributes the dividends of increased prosperity fairly across society (OECD, 2017[8])
Using micro-level data from national health surveys, this section provides new evidence on education-related inequalities in health status across European and OECD countries.
2.4.1. Inequalities in longevity by socio-economic status are substantial
By way of context, it is important to highlight that life expectancy has steadily increased over the past decade. On average across OECD countries, life expectancy at birth has increased by over ten years since 1970 (OECD Health Statistics, 2019). These trends mostly relate to a reduction of mortality rates from cardiovascular diseases and cancer.
However, the gains in life expectancy have not been equally shared. Large inequalities in life expectancy exist by socio-economic status including education level, income or occupational group, as illustrated, for instance, by Figure 2.14. On average across countries for which data are available, people with no high-school diploma can expect to live about six years less than those with a tertiary education. Inequalities in life expectancy are particularly large in Central and Eastern Europe. In the Slovak Republic, Hungary, Latvia, Poland, alongside Chile, a person with a low level of education can expect to live more than eight years less than those with a high level of education. These gaps in life expectancy are smaller in Canada, Croatia, Sweden and Italy, where they stand between three and four years.
The education gap in life expectancy is due to higher mortality rates among the least educated at different ages. The least educated people have, for example, higher death rates from circulatory diseases and cancer (Mackenbach et al., 2016[32]; Mackenbach et al., 2017[33]). A greater prevalence of smoking and of excessive alcohol consumption, particularly among low-educated men, contribute to these higher mortality rates.
To complement these data showing evidence of social gradients in longevity by educational attainment, the next section provides inequality estimates for morbidity indicators including self-assessed health status, limitations in daily activities and multiple chronic conditions.
Figure 2.14. Gap in life expectancy at birth, 2016 (or nearest year)

Source: Eurostat Database and OECD Health Statistics 2019.
2.4.2. Socio-economic differences in morbidity are also marked
This section investigates socio-economic differences in morbidity by looking at three variables: i) self-assessed health status, ii) limitations in daily activities and iii) multiple chronic conditions (see Table 2.6 for a list of variables and countries covered in this section). The method used is identical to the one presented in the beginning of Section 2.3, with the difference that the indirect standardisation factors gender in addition to age.
Table 2.6. Data used and coverage of the analysis for health status
|
Description of variables and coverage on the basis of EHIS-2 |
---|---|
Dependant variables |
Self-assessed health status: dummy variable identifying people reporting very poor, poor, fair health status versus good or very good health status. |
Limitations in daily activities: dummy variable identifying people reporting being severely limited and limited versus not limited. |
|
Multiple chronic conditions: dummy variable identifying people with two or more chronic conditions versus zero or one chronic condition. |
|
Explanatory variables |
Education is categorised into three different levels of education: 1. less than primary, primary and lower secondary level; 2. upper secondary and post-secondary non-tertiary level; 3. tertiary level |
Variables standardised for |
Age, sex |
Country coverage and exclusions |
30 EHIS countries, Canada, Chile and United States. The number of chronic diseases is not available in Chile. |
Covered population |
The population aged 18 and above. |
Note: The description of variables in this table refers to EHIS used for 30 of the 33 countries studied. Additional explanations about variables used for other countries surveys are available in Annex Table 2.A.1.
In all countries, the least educated have a higher risk of assessing their own health as poor
Self-assessed-health5 and other reported measures of health are good summary measures which capture different dimensions of health including medical and physical functioning. They are recognised as good predictors of people’s future health care use and mortality (Palladino et al., 2016[34]). However, as any other survey-based indicator, these measures of health are subject to reporting bias of self-reported data such as under-diagnosis and reporting errors. In addition, there are cross-country comparisons issues due to cultural differences.
Across OECD and European countries, Lithuania, Latvia and Portugal report the highest percentages of poor self-assessed health. Here, one in two persons consider their health status as not good. By comparison, these shares are below 20% in Ireland and Malta (Annex Table 2.A.6).
As expected, there is a large gap in self-assessed health across education groups (Figure 2.15): 44% of the least educated people assess their health as poor, compared to 23% of the people in the highest education group. Differences of 25 percentage points or more between education groups can be observed in the United States, Iceland, the Slovak Republic, Hungary, Luxembourg, and Portugal. In Romania, Malta and Italy these gaps are less than 16 percentage points.
Figure 2.15. Age-sex standardised probability of reporting a poor-self assessed health status by education level

Note: Data from 30 European countries, Canada, Chile and the United States. Results correspond to age-sex standardised probabilities. Differences between education groups are significant at the 95% confidence level for all countries.
Source: OECD estimates based on national health survey data.
Although smaller than for self-assessed health, differences between the least and most educated population groups in the probabilities of reporting limitations in daily activities and multi-morbidities go in the same direction. People in lower education groups are 16 percentage points more likely to report limitations in daily activities, and 11 percentage points more likely to report multi-morbidity compared to their counterparts in higher education groups (Annex Table 2.A.6 and 7). For the risk of reporting multi-morbidities, the highest differences between education groups are found in the Slovak Republic, Luxembourg, Cyprus and Iceland, while the lowest differences are found in Romania, Poland, Latvia, Estonia, and Germany. For the risk of reporting limitation in daily activities, the highest differences between education groups are found in the Slovak Republic, Luxembourg, Hungary and Lithuania, and the lowest in Malta, Canada, Italy, Chile, and Romania.
Absolute inequalities in self-assessed health are the highest in Portugal, Luxembourg Hungary, the Slovak Republic and the United States
All 33 countries included in this analysis display inequalities in health to the detriment of the poor for all measures available. To measure and compare the magnitude of health inequalities across countries taking into account the distribution over the whole population, Slope index of inequalities were calculated for all three health variables (Box 2.5 presents the methodology). Table 2.7 shows that inequalities to the detriment of the poor exist for all variables in all countries, and ranks them in each case from the lowest to highest degree of inequality. For self-assessed health, Portugal and Luxembourg present by far the largest absolute inequalities to the detriment of the least educated people. Hungary, the Slovak Republic and the United States also present very large absolute inequalities in self-assessed health. By contrast, Romania, Chile, Sweden, the Netherlands, Austria, Italy, Denmark, Estonia, and Norway are low inequality countries.
There is some consistency in country rankings when also considering inequalities in limitations in daily activities and in multi-morbidity. Luxembourg, Portugal, Hungary and the Slovak Republic display high level of absolute inequalities for all three analysed health variables. To some extent, this is also true for Greece and Iceland. On other hand, Romania and Sweden are faring relatively well in having low education-related inequalities across the different health outcomes. Absolute inequalities also appear more limited in Chile, the Netherlands and Canada (see all numerical values in Annex Table 2.A.6-7).
Table 2.7. Education-related inequalities in health
|
…does not differ across education groups |
…increases when education decreases |
---|---|---|
The probability of reporting a poor self-assessed health… |
- |
ROU, SWE, NLD, CHL, AUT, ITA, DEU, EST, NOR, BGR, CAN, POL, MLT, GBR, HRV, BEL, FRA, ESP, CZE, DNK, IRL, LTU, CYP, SVN, FIN, GRC, LVA, ISL, USA, SVK, HUN, LUX, PRT |
The probability of reporting limitation in daily activities … |
- |
ROU, CHL, DNK, SWE, CAN, MLT, NLD, ITA, SVN, POL, DEU, USA, ESP, CZE, AUT, GBR, EST, FIN, NOR, FRA, LVA, CYP, ISL, BGR, BEL ,IRL, PRT ,HRV, SVK, GRC, HUN, LUX, LTU |
The probability of reporting multiple chronic conditions … |
- |
ROU, POL, EST, SWE, LVA, BGR, CAN, MLT, DEU, SVN, FIN, FRA, CZE, GBR, ITA, LTU, USA, DNK, NLD, GRC, HRV, BEL, AUT, HUN, IRL, NOR, ESP, CYP, SVK, ISL, PRT, LUX |
Note: Data from 30 European countries, Canada, Chile and the United States. The data for multiple chronic conditions was not available for Chile. Countries are ranked from lowest to highest degree of inequality using the SII (numerical values in Annex Table 2.A.6-7).
Source: OECD estimates based on national health survey data.
These results support recent findings exploring inequalities in poor self-reported health in 26 EU countries (Leão et al., 2018[31]). Using cross-sectional data from the EU-SILC survey, high level of inequalities were found in some Southern European countries (including Cyprus, Greece, and Portugal) and in some Central and Eastern European countries (including Hungary, Slovenia, and the Slovak Republic). The use of the European Social Survey also confirmed that Greece and Portugal have large inequalities in self-reported general health (Eikemo et al., 2008[35]). EHIS data used in this chapter, however, does not point to Spain and even less Italy as being among the highest inequality countries with regards to self-assessed health – or other health indicators for that matter. This result is, however, consistent with findings from Mackenbach et al (2008[36]) which showed that inequalities in mortality and in self-assessed health were smaller in those two countries.
Outside of Europe, a recent analysis showed that gaps between the self-assessed health of groups with high and low education in various states of the United States were in general greater than in most European countries (Präg and Subramanian, 2017[37]). The population-level summary measure used here generally confirms this although some European countries (Portugal, Luxembourg, Hungary and the Slovak Republic) exhibit larger absolute educational inequalities.
Institutional determinants might partly explain these cross-country variations in socio-economic health inequalities. High level of income inequality, and fragmented social provision have indeed been associated with larger health inequalities (Eikemo et al., 2008[35]; Präg and Subramanian, 2017[37]; Ásgeirsdóttir and Ragnarsdóttir, 2013[38]; Leão et al., 2018[31]).
2.4.3. Summary of inequalities in health status
Some countries concentrate education-related inequalities in health status
Considering jointly the information on inequalities across different health status variables suggests inequalities are consistently higher (or lower) in some countries. Additional analyses combining the SIIs for self-assessed health, activity limitations and multiple chronic conditions was used to distinguish three groups of countries with low, intermediate and high levels of inequalities (see Box 2.6)6. Based on this, Figure 2.16 ranks countries by increasing life expectancy at birth while the colour of the bar indicates the level of inequality based on this grouping (darker shades correspond to more widespread inequalities to the detriment of the least educated). This analysis shows:
The highest education-related inequalities in health status to the detriment of the least educated are found in Lithuania, Hungary, the Slovak Republic, Croatia, Portugal, Luxembourg, Iceland, Greece, Ireland, Belgium and Cyprus. In seven of these countries, the life expectancy at birth is above the average of all countries considered (80.2 years). The Slovak Republic, Hungary, Lithuania, and Croatia combine a relatively low life expectancy at birth with very large health inequalities to the detriment of the least educated.
Northern European countries (Denmark, Sweden, the Netherlands, Germany), Poland, Romania, Estonia, Malta, Italy and Canada, Chile present the lowest level of education-related inequalities in health status to the detriment of the least educated. In four of these countries (Romania, Estonia, Poland, and Chile), the life expectancy at birth is below the average of all countries considered (80.2 years). Italy, Malta and Sweden combine a high life expectancy at birth but very low health inequalities to the detriment of the least educated.
Bulgaria, Latvia, the United States, the Czech Republic, the United Kingdom, Finland, Austria, Slovenia, Norway, France, and Spain have more intermediate levels of inequalities in health.
Overall, the correlation between the overall health status in a country (as measured by life expectancy at birth) and the level of education-related health inequalities is very weak.
Box 2.6. The principal component analysis
The principal component analysis (PCA) is carried out on the SII for the three health outcome measures (self-assessed health status, limitations in daily activities and multiple chronic conditions). Using an orthogonal transformation, the PCA convert a set of observations of possibly correlated variables into a set of values of linearly uncorrelated variables. It helps summarising the information contained on each country and identifying “regularities” between the values they take.
To summarise the results of the PCA, countries are divided in three groups of roughly equivalent sizes in which inequalities are relatively low, medium and large. These “convenient thirtiles” are based on the position of the countries on the first axis of the PCA with minor adjustment to ensure countries which are “very close” on that axis belong to the same groups.
Figure 2.16. Life expectancy at birth and summary level of education-related health inequalities across European and OECD countries

Note: *The data for multiple chronic conditions was not available for Chile, however various analyses based on comparisons of data for self-assessed health and activity limitations unambiguously demonstrate that the country belongs to the low inequalities group.
Source: OECD estimates based on national health survey data.
The ranking of countries is broadly consistent when using an alternative measure of inequalities
An alternative grouping using a similar methodology but comparing relative inequalities led to broadly consistent results with a few exception. Alongside SIIs, RIIs were computed which capture relative inequalities by education level (results are presented in Annex Table 2.A.6-7). The grouping obtained in the PCAs combining RIIs for self-assessed health, activity limitations and multiple chronic diseases is compared with the one obtained with SIIs in Figure 2.7. With some exceptions (Malta, Croatia and Lithuania), the pattern observed for absolute education-related inequalities in health status also generally applies to relative education-related inequalities in health status.
Malta would be clustered into the high inequality group if RIIs were used instead of SIIs to determine inequalities in health outcomes. This is due to the fact that the general prevalence in poor health outcomes in Malta–and hence the absolute differences between the different education groups- is comparably low. Looking at relative differences instead would signal higher overall inequalities. The reverse is true for Croatia and Lithuania: They would be grouped into the category of low inequality countries if RIIs were used instead of SIIs.
Figure 2.17. Absolute and relative education-related inequality in health, European and OECD countries

Note: The groupings by level of absolute and relative inequalities in health status are derived from PCA analysis of the SIIs and RIIs (available in Annex Table 2.A.6-7). Data for multiple chronic conditions was not available for Chile so the country is not included.
Source: OECD estimates based on national health survey data.
Using income as a proxy of socio-economic status provides a different grouping of countries
While the focus of this section is on differences in health by education level, income is an alternative proxy of socio-economic status to measure health inequalities. Absolute and relative income-related inequalities for all three health indictors were estimated on the basis of SIIs and RIIs (results in Annex Table 2.A.8) and groupings by level of inequality were elaborated as above. As with education, results first show the existence income-related inequalities to the detriment of the poor in all countries considered. Figure 2.18 compares the grouping of countries by level of (absolute) education-related inequalities with that based on income. Income and education tend to be differently associated to health status across countries. In particular, results show that:
There is a relatively weak correlation between absolute education- and income-related inequalities (correlation coefficient of 0.23).
14 out of 32 countries belong to the same groups of inequality whether inequalities are captured through education or income level (Romania, Poland, Malta, Germany, Italy, France, Latvia, Finland, Spain, Belgium, Croatia, Iceland, Hungary, Luxembourg).
Among the other countries, Sweden, Estonia and Denmark have low levels of education-related inequalities in health status but have high levels of income-related inequalities. The same is true for Slovenia, the United Kingdom and the United States, which have all high levels of income-related health inequalities and intermediate levels of education-related health inequalities. In these countries, social health inequalities are thus more pronounced when income is used as a measure of the differences in the social structure of the countries. In line with these results, the United States, the United Kingdom, Estonia and Slovenia have previously been found as high income-related health inequalities countries (van Doorslaer et al., 1997[39]; Ásgeirsdóttir and Ragnarsdóttir, 2013[38]). More recently, Hero, Zaslavsky and Blendon (2017) have shown that the United States, Chile and Portugal have among the largest income-related differences in self-rated health (Hero, Zaslavsky and Blendon, 2017[40]). In that regards, results presented in this chapter for Chile differ from previous findings: SII measured by education and income point to low levels of inequalities in Chile compared to other OECD countries (results in Annex Table 2.A.8). Although health inequalities have been reduced recently in Chile (Cabieses et al., 2015[41]), further research would need to confirm this result.
Greece, Lithuania, Ireland, Norway, Austria, Czech Republic, and Bulgaria show a reverse pattern. They have low levels of income-related health inequalities combined with high (Ireland, Lithuania and Greece) or medium (Bulgaria, the Czech Republic, Austria and Norway) levels of education-related health inequality.
Figure 2.18. Absolute inequalities in health status comparing education and income

Note: The rank of absolute inequalities in health status are derived from PCAs analyses presented above (box 2.6). Numerical results are available upon request. The data for multiple chronic conditions was not available for Chile so the country is not included.
Source: OECD estimates based on national health survey data.
The fact that countries have different levels of inequalities depending on whether the summary marker of people’s social position is income or education is not a surprise:
Each country is characterised by a distribution of the population across education levels which can be more or less unequal;
The wealth gap between people in the first and last income quintile is also likely to vary across countries;
Finally, the marginal impacts of income and education on health are unlikely to be identical.
A similar set of arguments could be made to explain why patters between relative and absolute inequalities differ.
Systematically linking the differences in the above pattern with countries characteristics is clearly beyond the scope of this report. Their presentation, however, highlights a number of points:
Pretty much no matter how they are measured, inequalities in health status are a problem in all countries considered;
Some countries clearly have high levels of inequality, no matter how they are measured (e.g., Belgium, Croatia, Iceland, Hungary and Luxembourg). Others are doing better with low levels of inequalities (Romania, Poland, Malta, Germany, and Italy) but some of them need to figure out how to do better on average health outcomes while trying not to leave people behind (Romania and Poland);
At the end of the day though, deciding on priorities and policies to tackle health inequalities requires looking at various measures simultaneously –it is never enough to look at one type of measure of inequality for one indicator on one measure of welfare.
2.5. Synthesis and conclusion
2.5.1. Poor health undermine people’s ability to work
The first section of the chapter reminds the importance of tackling poor health, not only because it undermines people’s welfare but also because it limits their ability to work. Poor health, measured by the number of deteriorated health indicators, translates into lower employment rates and higher absenteeism rates. On average across European and OECD countries, 70% of those who face no health problem are employed, versus 62% of people with at least one deteriorated health indicator. The labour market participation rates could increase by 3 percentage points in the absence of ill health on average across countries, ranging from less than 1 percentage point in Sweden to more than five in Chile.
When they have a job, people in poor health are less productive at work. Nearly half of those who consider themselves in poor health are likely to be absent from work at least once a year compared to only around a quarter of the people in good health. In total, a third of all people in poor health are employed and do not take sick leave during any year, compared to half of those in good health. In the absence of ill health, an additional 7.4 percentage points of the working age population could be fully productive across European and OECD countries. The burden of ill health on the labour market is the highest in Luxembourg and Denmark (around 10 percentage points) and comparably low in Malta (less than 4 percentage points).
Country-specific labour market policies (such as activation labour market policies, or the scope of compensated sick leave and the generosity of the benefit schemes) and health policies (such as public health actions to support workers experiencing chronic ill health to stay in work) contribute to explaining these cross-country differences. The present analysis could serve as a starting point to identify which sets of policies overall contribute to sustaining a more healthy and productive active population.
2.5.2. Exposure to behavioural risk factors becomes more prevalent as education decreases
The second section of the chapter confirms that behavioural risk factors are unequally distributed along the social spectrum: less educated people tend to have higher exposure rates to overweight, smoking and heavy drinking (although considerable difference exist across genders). In particular, results show that:
In all countries included in the analysis, the distribution of obesity and overweight is significantly unequal and to the detriment of the least educated for women. The same is true for men in 18 countries but the gradient is not significant in 13 countries. In Poland, inequalities in obesity are to the detriment of the most educated men. In Luxembourg, France, Spain, Italy and Denmark inequalities are relatively high, for both men and women.
For both women and men, smoking rates are twice as high for people in the lowest education group compared to those in the highest one. The summary measures indicates significant inequalities to the detriment of women with lower education in 26 countries and to the detriment of higher educated women in Romania, Cyprus and Portugal. There was no significant gradient in the remaining three countries: Bulgaria, Greece and Croatia. For men, inequalities to the detriment of the least educated are significant everywhere and highest in Baltic countries, Belgium and Hungary.
Heavy drinking among men becomes less frequent as education rises in 13 countries. Inequalities to the detriment of the least educated men are higher in Portugal, Lithuania, Romania, and comparably small in Canada, Spain and Bulgaria. The social gradient in heavy drinking is less clear or consistent among women. In seven countries, more education is associated with increased heavy alcohol consumption (Austria, Germany, Norway, Denmark, Belgium, the United Kingdom and Luxembourg), while this harmful drinking habit becomes more prevalent as education decreases in Lithuania, Romania and Canada. There is no social gradient in 15 countries for men and 17 for women. Inequalities to the detriment of the least educated are largest for both men and women in Romania and Lithuania.
2.5.3. All countries display inequalities in health to the detriment of the least educated people
The last section provides new evidence on education-related health inequalities across European and OECD countries. All countries display a significant gradient of inequality to the disadvantage of the least educated people. The results consistently show that lower-educated people have a higher likelihood to report a poor health status, with variations across countries and across health status variables. The findings show:
People in the lowest education group are 21 percentage points more likely to assess their health as poor compared to their counterparts in the highest education group.
People with less than a high school degree are 16 percentage points more likely to report limitations in daily activities compared to those with tertiary education.
People in the group with the lowest level of education groups are 11 percentage points more likely to suffer from multi-morbidities compared to those in the group with the highest level of education.
For these three variables which capture health status, population-level summary measures of inequalities show a gradient detrimental to the poor in all countries.
Considering jointly inequalities across the three health status variables suggests inequalities are consistently higher in some countries:
The highest education-related inequalities in health status to the detriment of the least educated are found in Greece, Portugal, Cyprus, Croatia, Lithuania, the Slovak Republic, Hungary as well as Luxembourg, Belgium, Ireland and Iceland.
Northern European countries (Denmark, Sweden, the Netherlands, Germany), Slovenia, Poland, Romania, Estonia, Malta, Italy, Canada and Chile present the lowest level of education-related inequalities in health status to the detriment of the least educated. Results suggest that there is a weak correlation between life expectancy at birth and levels of absolute inequalities.
2.5.4. Policy responses need to be comprehensive, and to better target those most affected
A series of policy options exist to redress inequalities in health and its determinants. On one hand, given the variety of health determinants, improving health outcomes and reducing health inequalities require a comprehensive policy approach characterised by coordinated actions from national institutions and the private sector. Outside of the health sector, the regulation of the labour market, redistributive fiscal policy measures, opportunities for decent housing, or reducing access barrier to schooling are key policy areas of interest to reduce health inequalities (Forster, Kentikelenis and Bambra, 2018[42]).
On the other hand, more tailored solutions seem to be required to reduce inequalities in risky health behaviour. Public health policies not only should target men and women differently, but may also focus more on particular population groups that are vulnerable to risky health behaviour -notably those with lower levels of education. A number of OECD and European countries have introduced a package of regulation on tobacco, beverages, and food products to tackle risky health behaviour, including taxation instruments, labelling norms, and age restrictions (Forster, Kentikelenis and Bambra, 2018[42]; Sassi, 2015[26]), with significant impact on lower socio-economic groups (Sassi F, 2009[43]). Empirical evidence for example show that tobacco tax increases are an effective measure for reducing smoking among persons of low socio-economic status (Bader, Boisclair and Ferrence, 2011[44]; Thomas et al., 2008[45]), and that they reduce socio-economic inequality in tobacco consumption over the long run (Tabuchi, Iso and Brunner, 2018[46]).
To raise awareness of unhealthy lifestyles and drive change, efforts to improve health literacy should be strengthened. This may be particularly beneficial to people with lower levels of education by enhancing their understanding of the long-term consequences of risky health behaviour. It can also have wider effects by developing individual’s knowledge and empower them to act on health information, and by helping people in need for health care navigate the health system more effectively.
Finally, the health systems can contribute to improving health and overcoming inequalities by ensuring access to quality services irrespective of people’s socio-economic circumstances and ethnic origin. Improving access to culturally safe and responsive services for minorities or indigenous populations is for example critical to tackle health inequalities related to ethnicity and immigration
To what extent the health systems provide care to all segments of the population based on need will be further explored in the following chapters.
References
[38] Ásgeirsdóttir, T. and D. Ragnarsdóttir (2013), “Determinants of relative and absolute concentration indices: Evidence from 26 European countries”, International Journal for Equity in Health, http://dx.doi.org/10.1186/1475-9276-12-53.
[44] Bader, P., D. Boisclair and R. Ferrence (2011), Effects of tobacco taxation and pricing on smoking behavior in high risk populations: A knowledge synthesis, http://dx.doi.org/10.3390/ijerph8114118.
[19] Bajekal, M. et al. (2012), “Analysing Recent Socioeconomic Trends in Coronary Heart Disease Mortality in England, 2000–2007: A Population Modelling Study”, PLoS Medicine, Vol. 9/6, p. e1001237, http://dx.doi.org/10.1371/journal.pmed.1001237.
[30] Balaj, M. et al. (2017), The social determinants of inequalities in self-reported health in Europe: Findings from the European social survey (2014) special module on the social determinants of health, http://dx.doi.org/10.1093/eurpub/ckw217.
[13] Burström, B. et al. (2005), “Inequality in the Social Consequences of Illness: How Well Do People with Long-Term Illness Fare in the British and Swedish Labor Markets?”, International Journal of Health Services, http://dx.doi.org/10.2190/6pp1-tdeq-h44d-4ljq.
[41] Cabieses, B. et al. (2015), “Did socioeconomic inequality in self-reported health in chile fall after the equity-based healthcare reform of 2005? A concentration index decomposition analysis”, PLoS ONE, http://dx.doi.org/10.1371/journal.pone.0138227.
[18] CSDH (2008), Closing the gap in a generation: health equity through action on the social determinants of health. Final Report of the Commission on Social Determinants of Health., World Health Organisation, Geneva.
[4] Cutler, D. and A. Lleras-Muney (2006), Education and Health: Evaluating Theories and Evidence, http://dx.doi.org/10.3386/w12352.
[10] Devaux, M. and F. Sassi (2015), “The Labour Market Impacts of Obesity, Smoking, Alcohol Use and Related Chronic Diseases”, OECD Health Working Papers, No. 86, OECD Publishing, Paris, https://dx.doi.org/10.1787/5jrqcn5fpv0v-en.
[9] Devaux, M. and F. Sassi (2013), “Social inequalities in obesity and overweight in 11 OECD countries”, European Journal of Public Health, http://dx.doi.org/10.1093/eurpub/ckr058.
[35] Eikemo, T. et al. (2008), “Health inequalities according to educational level in different welfare regimes: A comparison of 23 European countries”, Sociology of Health and Illness, http://dx.doi.org/10.1111/j.1467-9566.2007.01073.x.
[11] Eurostat (2013), European Health Interview Survey (EHIS wave 2), Methodological Manual, https://ec.europa.eu/eurostat/documents/3859598/5926729/KS-RA-13-018-EN.PDF/26c7ea80-01d8-420e-bdc6-e9d5f6578e7c.
[42] Forster, T., A. Kentikelenis and C. Bambra (2018), Health Inequalities in Europe: Setting the Stage for Progressive Policy Action, TASC, Dublin, https://www.tasc.ie/download/pdf/18456_health_inequalities_innerweb.pdf (accessed on 30 November 2018).
[48] Griswold, M. et al. (2018), “Alcohol use and burden for 195 countries and territories, 1990-2016: A systematic analysis for the Global Burden of Disease Study 2016”, The Lancet, http://dx.doi.org/10.1016/S0140-6736(18)31310-2.
[27] Grittner, U. et al. (2013), “Alcohol consumption and social inequality at the individual and country levels - Results from an international study”, European Journal of Public Health, http://dx.doi.org/10.1093/eurpub/cks044.
[40] Hero, J., A. Zaslavsky and R. Blendon (2017), “The United States leads other nations in differences by income in perceptions of health and health care”, Health Affairs, Vol. 36/6, pp. 1032-1040, http://dx.doi.org/10.1377/hlthaff.2017.0006.
[24] Huisman, M., A. Kunst and J. Mackenbach (2005), “Inequalities in the prevalence of smoking in the European Union: comparing education and income”, Preventive Medicine, Vol. 40/6, pp. 756-764, http://dx.doi.org/10.1016/J.YPMED.2004.09.022.
[7] James, C., M. Devaux and F. Sassi (2017), “Inclusive growth and health”, OECD Health Working Papers, No. 103, OECD Publishing, Paris, https://dx.doi.org/10.1787/93d52bcd-en.
[12] Knebelmann, J. and C. Prinz (2016), “The Impact of Depression on Employment of Older Workers in Europe”, OECD Social, Employment and Migration Working Papers, No. 170, OECD Publishing, Paris, http://dx.doi.org/10.1787/106dbdae-en.
[31] Leão, T. et al. (2018), “Welfare states, the Great Recession and health: Trends in educational inequalities in self-reported health in 26 European countries”, PLoS ONE, http://dx.doi.org/10.1371/journal.pone.0193165.
[33] Mackenbach, J. et al. (2017), “Trends in inequalities in mortality amenable to health care in 17 european countries”, Health Affairs, Vol. 36/6, pp. 1110-1118, http://dx.doi.org/10.1377/hlthaff.2016.1674.
[32] Mackenbach, J. et al. (2016), “Changes in mortality inequalities over two decades: Register based study of European countries”, BMJ (Online), http://dx.doi.org/10.1136/bmj.i1732.
[36] Mackenbach, J. et al. (2008), “Socioeconomic Inequalities in Health in 22 European Countries”, New England Journal of Medicine, Vol. 358, pp. 2468-81, http://www.nejm.org (accessed on 21 February 2019).
[1] Marmot, M. and E. Brunner (2005), “Cohort profile: The Whitehall II study”, International Journal of Epidemiology, http://dx.doi.org/10.1093/ije/dyh372.
[2] Marmot, M. et al. (1991), “Health inequalities among British civil servants: the Whitehall II study.”, Lancet (London, England), Vol. 337/8754, pp. 1387-93, http://dx.doi.org/10.1016/0140-6736(91)93068-K.
[3] Marmot, M. and R. Wilkinson (eds.) (2006), Social determinants of health, Oxford University Press.
[29] Murtin, F. et al. (2017), “Inequalities in longevity by education in OECD countries: Insights from new OECD estimates”, OECD Statistics Working Papers, No. 2017/2, OECD Publishing, Paris, https://dx.doi.org/10.1787/6b64d9cf-en.
[28] Ngamaba, K., M. Panagioti and C. Armitage (2017), “How strongly related are health status and subjective well-being? Systematic review and meta-analysis”, European Journal of Public Health, http://dx.doi.org/10.1093/eurpub/ckx081.
[47] O’Donnell, O. et al. (2007), Analyzing Health Equity Using Household Survey Data, The World Bank, http://dx.doi.org/10.1596/978-0-8213-6933-3.
[16] OECD (2017), Health at a Glance 2017: OECD Indicators, OECD Publishing, Paris, https://dx.doi.org/10.1787/health_glance-2017-en.
[23] OECD (2017), Obesity Update, http://www.oecd.org/health/obesity-update.htm.
[8] OECD (2017), Preventing Ageing Unequally, OECD Publishing, Paris, https://dx.doi.org/10.1787/9789264279087-en.
[5] OECD (2016), “The labour market impacts of ill-health”, in Health at a Glance: Europe 2016: State of Health in the EU Cycle, OECD Publishing, Paris, https://dx.doi.org/10.1787/health_glance_eur-2016-4-en.
[14] OECD/EU (2018), Health at a Glance: Europe 2018: State of Health in the EU Cycle, OECD Publishing, Paris/European Union, Brussels, https://dx.doi.org/10.1787/health_glance_eur-2018-en.
[6] Orchard, C. (2015), “Adult Health in Great Britain, 2013”, Office for National Statistics, United Kingdom, https://www.ons.gov.uk/peoplepopulationandcommunity/healthandsocialcare/healthandlifeexpectancies/compendium/opinionsandlifestylesurvey/2015-03-19/adulthealthingreatbritain2013.
[34] Palladino, R. et al. (2016), “Associations between multimorbidity, healthcare utilisation and health status: evidence from 16 European countries”, Age and Ageing, Vol. 45/3, pp. 431-435, http://dx.doi.org/10.1093/ageing/afw044.
[37] Präg, P. and S. Subramanian (2017), “Educational inequalities in self-rated health across US states and European countries”, International Journal of Public Health, Vol. 62/6, pp. 709-716, http://dx.doi.org/10.1007/s00038-017-0981-6.
[43] Sassi F, C. (2009), IMPROVING LIFESTYLES, TACKLING OBESITY: THE HEALTH AND ECONOMIC IMPACT OF PREVENTION STRATEGIES, https://doi.org/10.1787/220087432153.
[26] Sassi, F. (ed.) (2015), Tackling Harmful Alcohol Use: Economics and Public Health Policy, OECD Publishing, Paris, https://dx.doi.org/10.1787/9789264181069-en.
[25] Schaap, M. (2010), Socio-economic inequality in smoking.
[22] Schneider, M. et al. (2005), “Methods for measuring health inequalities (Part II)”, Epidemiological Bulletin / PAHO, Vol. 26/1.
[20] Shavers, V. (2007), “Measurement of Socioeconomic Status in Health Disparities Research”, Journal of the National Medical Association, Vol. Vol.99/9, pp. Pp 1013-1023.
[17] Siegrist, J., D. Montano and H. Hoven (2014), Working conditions and health inequalities, evidence and policy implications, http://www.health-gradient.eu.
[15] Skagen, K. and A. Collins (2016), “The consequences of sickness presenteeism on health and wellbeing over time: A systematic review”, Social Science & Medicine, Vol. 161, pp. 169-177, http://dx.doi.org/10.1016/J.SOCSCIMED.2016.06.005.
[46] Tabuchi, T., H. Iso and E. Brunner (2018), Tobacco control measures to reduce socioeconomic inequality in smoking: The necessity, time-course perspective, and future implications, http://dx.doi.org/10.2188/jea.JE20160206.
[45] Thomas, S. et al. (2008), “Population tobacco control interventions and their effects on social inequalities in smoking: Systematic review”, Tobacco Control, http://dx.doi.org/10.1136/tc.2007.023911.
[39] van Doorslaer, E. et al. (1997), “Income-related inequalities in health: some international comparisons”, Journal of Health Economics, Vol. 16/1, pp. 93-112, http://dx.doi.org/10.1016/s0167-6296(96)00532-2.
[21] WHO (2017), Health Equity Assessment Toolkit Built-in Database Edition.
Annex 2.A. Detailed results on Chapter 2
Annex Table 2.A.1. Description of variables for surveys other than EHIS
MEPS 2016 (USA) |
Poor self-assessed health: dummy variable with people who reported a good, fair or poor health status, versus those who reported either a very good or an excellent one. |
2+ chronic diseases: dummy variable with people who reported having suffered from 2 or more chronic conditions versus 0 or 1 chronic disease in the past 12 months. The following chronic conditions are considered: diabetes, hypertension, emphysema or chronic bronchitis, asthma, heart disease, ever had a stroke and/or ever had a heart attack. |
|
Depression: dummy variable with people who reported a score strictly above 3 when asked to rate their feelings overall in the past 2 weeks on a scale from 1 to 6, versus those who reported a score inferior or equal to 3. A score above 3 reflects a person’s tendency towards depression. |
|
Smoking: dummy variable with people who reported smoking currently, versus those who reported not smoking currently. |
|
Education: categorical variable reflecting three different levels of education: 1. No degree; 2. General education diploma and high school diploma; 3. Bachelor’ degree, master’s degree and doctor. |
|
CCHS 2017 (Canada) |
Absence from work: dummy variable with people who reported having missed work, versus those who report not having done so in the past 3 months because of any of the following conditions: a chronic condition, an injury, an acute infectious disease, any other acute physical condition or an acute mental health condition. |
Poor self-assessed health: dummy variable with people who reported a good, fair or poor health status, versus those who reported either a very good or an excellent one. |
|
2+ chronic diseases: dummy variable with people reporting having suffered from 2 or more chronic conditions versus 0 or 1 chronic disease in the past 12 months. The following chronic conditions are considered: diabetes, hypertension, COPD, asthma, heart disease and/or effects of a stroke. |
|
Depression: dummy variable with people reporting having a mood disorder (depression, bipolar, mania, dysthymia) versus those who do not report one. |
|
Education: categorical variable reflecting three different levels of education: 1.Less than secondary school graduation; 2.Secondary school graduation, no post-secondary graduation; 3.Post-secondary certificate diploma or university degree. |
|
CASEN 2017 (Chile) |
Poor self-assessed health: dummy variable with people who reported a score below or equal to 5 when asked to grade their health status on a scale from 1 (very bad) to 7 (very good), versus those who reported a score strictly superior to 5. |
Chronic diseases: not available in the survey. |
|
Education categorical variable reflecting three different levels of education: 1. Less than primary and primary (no education, kinder and pre-kinder garden, special education, primary and basic education), 2. Secondary education (medium, technical and professional education level), and 3. Post-graduate level or more. |
|
Risk factors: not available in the survey. |
Note: In Canada and the United States, the dummy variable of poor health has been re-scaled to be comparable to the one used in other national health survey data. In EHIS, the following chronic conditions are considered: asthma, chronic bronchitis (or chronic obstructive pulmonary disease, emphysema), myocardial infarction (heart attack, or chronic consequences of myocardial infarction), coronary heart disease or angina pectoris, high blood pressure (hypertension), stroke (cerebral haemorrhage, cerebral thrombosis) or chronic consequences of stroke, arthrosis, low back disorder, neck disorder, diabetes, allergy, cirrhosis of the liver, urinary incontinence, kidney problems and depressions.
Detailed results on labour market variables
Annex Table 2.A.2. Descriptive statistics and predicted probabilities: employment and absence from work due to illness
Working-age population |
Working population |
|||||||||
---|---|---|---|---|---|---|---|---|---|---|
% employed |
% in poor health# |
PP to be employed if no health problem |
PP to be employed if poor health |
GS |
% absent |
% in poor health |
PP to be absent if no health problem |
PP to be absent if poor health |
GS |
|
EU27/26 |
66% |
41% |
69% |
61% |
32% |
37% |
25% |
46% |
||
OECD |
68% |
43% |
71% |
64% |
36% |
39% |
28% |
49% |
||
Total |
67% |
41% |
70% |
62% |
34% |
37% |
26% |
47% |
||
Austria |
68% |
41% |
74% |
66% |
* |
49% |
36% |
40% |
61% |
* |
Belgium |
66% |
32% |
67% |
59% |
* |
39% |
28% |
33% |
59% |
* |
Bulgaria |
60% |
29% |
63% |
49% |
* |
15% |
22% |
10% |
32% |
* |
Canada |
67% |
43% |
71% |
63% |
* |
33% |
38% |
27% |
42% |
* |
Chile |
64% |
37% |
66% |
62% |
* |
- |
35% |
- |
- |
|
Croatia |
54% |
45% |
54% |
51% |
19% |
39% |
11% |
31% |
* |
|
Cyprus |
66% |
24% |
68% |
57% |
* |
14% |
18% |
11% |
30% |
* |
Czech Republic |
69% |
40% |
70% |
61% |
* |
22% |
37% |
15% |
34% |
* |
Denmark |
72% |
46% |
79% |
73% |
* |
52% |
42% |
42% |
67% |
* |
Estonia |
78% |
45% |
81% |
74% |
* |
29% |
41% |
21% |
40% |
* |
Finland |
66% |
59% |
70% |
64% |
* |
58% |
56% |
47% |
65% |
* |
France |
66% |
41% |
66% |
61% |
* |
30% |
38% |
23% |
44% |
* |
Germany |
81% |
52% |
83% |
79% |
* |
62% |
50% |
53% |
71% |
* |
Greece |
49% |
30% |
51% |
42% |
* |
17% |
22% |
12% |
30% |
* |
Hungary |
66% |
44% |
69% |
60% |
* |
21% |
38% |
14% |
32% |
* |
Iceland |
77% |
47% |
82% |
74% |
* |
50% |
43% |
41% |
60% |
* |
Italy |
59% |
33% |
61% |
57% |
* |
30% |
30% |
24% |
43% |
* |
Latvia |
71% |
56% |
72% |
70% |
30% |
53% |
19% |
40% |
* |
|
Lithuania |
69% |
45% |
71% |
65% |
* |
22% |
43% |
15% |
32% |
* |
Luxembourg |
68% |
51% |
75% |
71% |
46% |
48% |
35% |
60% |
* |
|
Malta |
65% |
28% |
65% |
58% |
* |
41% |
22% |
37% |
52% |
* |
Netherlands |
68% |
37% |
73% |
62% |
* |
44% |
31% |
37% |
59% |
* |
Norway |
76% |
33% |
82% |
66% |
* |
50% |
27% |
44% |
64% |
* |
Poland |
64% |
39% |
64% |
56% |
* |
23% |
33% |
14% |
38% |
* |
Portugal |
63% |
53% |
65% |
61% |
* |
26% |
49% |
15% |
37% |
* |
Romania |
66% |
20% |
70% |
51% |
* |
- |
33% |
- |
- |
|
Slovak Republic |
64% |
42% |
62% |
53% |
* |
20% |
35% |
12% |
36% |
* |
Slovenia |
61% |
51% |
63% |
58% |
* |
34% |
45% |
25% |
46% |
* |
Spain |
59% |
37% |
65% |
58% |
* |
21% |
32% |
16% |
39% |
* |
Sweden |
76% |
42% |
76% |
74% |
43% |
39% |
35% |
56% |
* |
|
United Kingdom |
74% |
38% |
76% |
65% |
* |
34% |
33% |
26% |
50% |
* |
United States |
79% |
41% |
79% |
72% |
* |
48% |
38% |
40% |
56% |
* |
Note: PP stands for predicted probabilities estimated with logit models, GS for global significance, and * means significant at 5%. # “Poor health” means the person has at least one of four deteriorated health indicators (see Table 2.1 for definition of variable). In Chile, the variable is based on three health indicators only. The proportion of people reporting absence from work due to illness in Canada (in the past three months) is not directly comparable with other countries (in the past 12 months). When the definition of a variable is different from EHIS, the country is not included in the average for that variable.
Source: OECD calculations based on national health survey data.
Detailed results on risk factors
Annex Table 2.A.3. Descriptive statistics, standardised probabilities and inequality index: overweight among women and men
Women |
Men |
|||||||||||
---|---|---|---|---|---|---|---|---|---|---|---|---|
Prevalence |
SP – Low education |
SP – High education |
GS |
SII* |
RII* |
Prevalence |
SP – Low education |
SP – High education |
GS |
SII* |
RII* |
|
EU28 |
46% |
52% |
35% |
59% |
57% |
53% |
||||||
OECD |
47% |
52% |
36% |
59% |
58% |
53% |
||||||
Total |
47% |
52% |
36% |
60% |
58% |
54% |
||||||
Austria |
40% |
49% |
28% |
* |
0.28* |
2.1* |
56% |
55% |
50% |
* |
0.12* |
1.2* |
Belgium |
42% |
49% |
31% |
* |
0.33* |
2.4* |
54% |
54% |
51% |
* |
0.09* |
1.2* |
Bulgaria |
47% |
51% |
36% |
* |
0.17* |
1.4* |
62% |
57% |
57% |
* |
-0.02 |
1.0 |
Canada |
52% |
59% |
49% |
* |
0.14* |
1.3* |
65% |
65% |
64% |
* |
0.04* |
1.1* |
Croatia |
48% |
58% |
36% |
* |
0.23* |
1.6* |
67% |
59% |
61% |
0.00 |
1.0 |
|
Cyprus |
38% |
46% |
31% |
* |
0.20* |
1.6* |
59% |
56% |
59% |
-0.05 |
0.9 |
|
Czech Republic |
49% |
53% |
37% |
* |
0.20* |
1.4* |
64% |
60% |
54% |
0.06 |
1.1 |
|
Denmark |
40% |
49% |
31% |
* |
0.24* |
1.8* |
54% |
55% |
44% |
* |
0.21* |
1.5* |
Estonia |
51% |
57% |
42% |
* |
0.19* |
1.5* |
56% |
50% |
52% |
-0.04 |
0.9 |
|
Finland |
47% |
51% |
41% |
* |
0.16* |
1.4* |
61% |
61% |
56% |
0.07* |
1.1* |
|
France |
41% |
48% |
26% |
* |
0.35* |
2.4* |
53% |
53% |
45% |
* |
0.13* |
1.3* |
Germany |
44% |
51% |
33% |
* |
0.21* |
1.6* |
59% |
61% |
53% |
* |
0.13* |
1.2* |
Greece |
48% |
52% |
38% |
* |
0.26* |
1.7* |
66% |
66% |
58% |
* |
0.13* |
1.2* |
Hungary |
49% |
52% |
39% |
* |
0.19* |
1.5* |
61% |
55% |
58% |
-0.01 |
1.0 |
|
Iceland |
48% |
52% |
37% |
* |
0.20* |
1.5* |
65% |
60% |
59% |
0.02 |
1.0 |
|
Ireland |
48% |
54% |
38% |
* |
0.22* |
1.6* |
62% |
62% |
59% |
0.01 |
1.0 |
|
Italy |
36% |
42% |
25% |
* |
0.25* |
2.0* |
53% |
55% |
45% |
* |
0.13* |
1.3* |
Latvia |
54% |
55% |
48% |
* |
0.10* |
1.2* |
58% |
53% |
58% |
-0.06 |
0.9 |
|
Lithuania |
53% |
59% |
44% |
* |
0.19* |
1.4* |
58% |
54% |
53% |
0.05 |
1.1 |
|
Luxembourg |
39% |
49% |
24% |
* |
0.39* |
2.9* |
55% |
63% |
46% |
* |
0.25* |
1.6* |
Malta |
55% |
57% |
50% |
0.13* |
1.3* |
66% |
66% |
61% |
0.06 |
1.1 |
||
Netherlands |
45% |
50% |
36% |
* |
0.21* |
1.6* |
53% |
53% |
44% |
* |
0.13* |
1.3* |
Norway |
40% |
43% |
33% |
* |
0.15* |
1.5* |
57% |
58% |
48% |
* |
0.12* |
1.2* |
Poland |
47% |
49% |
38% |
* |
0.16* |
1.4* |
64% |
54% |
59% |
* |
-0.07* |
0.9* |
Portugal |
50% |
54% |
32% |
* |
0.35* |
2.0* |
57% |
57% |
49% |
* |
0.07* |
1.1* |
Romania |
49% |
52% |
35% |
* |
0.16* |
1.4* |
63% |
58% |
57% |
* |
-0.03 |
1.0 |
Slovak Republic |
46% |
50% |
37% |
* |
0.16* |
1.4* |
62% |
57% |
53% |
* |
0.09* |
1.2* |
Slovenia |
48% |
55% |
30% |
* |
0.36* |
2.2* |
65% |
54% |
58% |
* |
0.02 |
1.0 |
Spain |
45% |
53% |
29% |
* |
0.38* |
2.3* |
61% |
62% |
51% |
* |
0.16* |
1.3* |
Sweden |
44% |
46% |
32% |
* |
0.21* |
1.6* |
55% |
57% |
42% |
* |
0.21* |
1.5* |
United Kingdom |
51% |
58% |
43% |
* |
0.19* |
1.4* |
59% |
60% |
53% |
* |
0.10* |
1.2* |
United States |
60% |
63% |
50% |
* |
0.24* |
1.5* |
71% |
71% |
70% |
* |
0.06* |
1.1* |
Note: SP stands for standardised probabilities (age-standardised) estimated with logit models, GS for global significance, SII for slope index of inequalities, RII for relative index of inequalities and * means significant at 5%. There is no available data for overweight in Chile.
Source: OECD calculations based on national health survey data.
Annex Table 2.A.4. Descriptive statistics, standardised probabilities and inequality index: smoking among women and men
Women |
Men |
|||||||||||
---|---|---|---|---|---|---|---|---|---|---|---|---|
Prevalence |
SP – Low education |
SP– High education |
GS |
SII* |
RII* |
Prevalence |
SP – Low education |
SP – High education |
GS |
SII* |
RII* |
|
EU28 |
20% |
24% |
13% |
32% |
40% |
20% |
||||||
OECD |
20% |
25% |
11% |
29% |
38% |
18% |
||||||
Total |
20% |
24% |
12% |
31% |
39% |
20% |
||||||
Austria |
28% |
36% |
15% |
* |
0.23* |
2.4* |
33% |
42% |
23% |
* |
0.24* |
2.3* |
Belgium |
20% |
28% |
9% |
* |
0.25* |
3.5* |
27% |
39% |
11% |
* |
0.35* |
3.9* |
Bulgaria |
28% |
25% |
24% |
* |
-0.02 |
0.9 |
45% |
52% |
32% |
* |
0.24* |
1.7* |
Canada |
18% |
29% |
13% |
* |
0.21* |
3.4* |
22% |
35% |
17% |
* |
0.21* |
2.7* |
Croatia |
26% |
26% |
19% |
* |
0.02 |
1.1 |
33% |
40% |
22% |
* |
0.19* |
1.8* |
Cyprus |
19% |
17% |
20% |
-0.09* |
0.6* |
44% |
47% |
35% |
0.18* |
1.6* |
||
Czech Republic |
23% |
31% |
7% |
* |
0.21* |
2.7* |
36% |
44% |
22% |
* |
0.27* |
2.3* |
Denmark |
20% |
27% |
13% |
* |
0.16* |
2.3* |
23% |
38% |
15% |
* |
0.23* |
3.0* |
Estonia |
20% |
26% |
13% |
* |
0.19* |
2.7* |
39% |
54% |
28% |
* |
0.33* |
2.5* |
Finland |
17% |
21% |
9% |
* |
0.21* |
3.7* |
23% |
33% |
14% |
* |
0.23* |
2.9* |
France |
25% |
27% |
17% |
* |
0.14* |
1.8* |
33% |
38% |
25% |
* |
0.18* |
1.8* |
Germany |
19% |
23% |
10% |
* |
0.20* |
2.6* |
25% |
32% |
18% |
* |
0.18* |
2.1* |
Greece |
28% |
27% |
23% |
* |
-0.02 |
0.9 |
41% |
44% |
34% |
* |
0.14* |
1.4* |
Hungary |
22% |
29% |
10% |
* |
0.29* |
3.5* |
34% |
50% |
17% |
* |
0.41* |
3.6* |
Iceland |
18% |
26% |
10% |
* |
0.24* |
3.9* |
21% |
31% |
13% |
* |
0.24* |
3.4* |
Ireland |
20% |
28% |
10% |
* |
0.27* |
4.6* |
24% |
34% |
14% |
* |
0.27* |
3.7* |
Italy |
18% |
18% |
14% |
* |
0.04* |
1.3* |
29% |
32% |
21% |
* |
0.17* |
1.8* |
Latvia |
20% |
26% |
11% |
* |
0.20* |
3.0* |
44% |
55% |
26% |
* |
0.36* |
2.4* |
Lithuania |
13% |
14% |
6% |
* |
0.13* |
2.8* |
43% |
51% |
19% |
* |
0.43* |
3.0* |
Luxembourg |
18% |
22% |
10% |
* |
0.23* |
3.5* |
24% |
33% |
14% |
* |
0.25* |
3.1* |
Malta |
22% |
26% |
12% |
* |
0.19* |
2.6* |
28% |
34% |
15% |
* |
0.26* |
2.6* |
Netherlands |
22% |
28% |
13% |
* |
0.21* |
2.8* |
30% |
38% |
20% |
* |
0.22* |
2.2* |
Norway |
20% |
30% |
10% |
* |
0.26* |
3.8* |
22% |
32% |
12% |
* |
0.26* |
3.7* |
Poland |
21% |
25% |
11% |
* |
0.16* |
2.2* |
34% |
43% |
17% |
* |
0.29* |
2.4* |
Portugal |
14% |
13% |
11% |
* |
-0.04* |
0.8* |
28% |
30% |
16% |
* |
0.20* |
2.0* |
Romania |
13% |
12% |
17% |
-0.07* |
0.5* |
41% |
41% |
32% |
0.07* |
1.2* |
||
Slovak Republic |
22% |
31% |
11% |
* |
0.23* |
3.0* |
39% |
47% |
24% |
* |
0.32* |
2.4* |
Slovenia |
22% |
24% |
11% |
* |
0.16* |
2.0* |
29% |
35% |
16% |
* |
0.23* |
2.4* |
Spain |
21% |
22% |
16% |
* |
0.06* |
1.3* |
31% |
36% |
21% |
* |
0.21* |
2.0* |
Sweden |
16% |
23% |
9% |
* |
0.22* |
3.9* |
18% |
24% |
11% |
* |
0.17* |
2.6* |
United Kingdom |
16% |
24% |
8% |
* |
0.21* |
4.0* |
19% |
30% |
11% |
* |
0.23* |
4.3* |
United States |
12% |
19% |
7% |
* |
0.14* |
3.3* |
17% |
25% |
10% |
* |
0.18* |
3.0* |
Note: SP stands for standardised probabilities (age-standardised) estimated with logit models, GS for global significance, SII for slope index of inequalities, RII for relative index of inequalities and * means significant at 5%. There is no available data for smoking in Chile.
Source: OECD calculations based on national health survey data.
Annex Table 2.A.5. Descriptive statistics, standardised probabilities and inequality index: heavy drinking among women and men
Women |
Men |
||||||||||||
---|---|---|---|---|---|---|---|---|---|---|---|---|---|
Prevalence |
SP – Low education |
SP – High education |
GS |
SII* |
RII* |
Prevalence |
SP – Low education |
SP – High education |
GS |
SII* |
RII* |
||
EU19/20 |
3% |
3% |
4% |
6% |
8% |
4% |
|||||||
OECD |
3% |
3% |
4% |
5% |
8% |
4% |
|||||||
Total |
3% |
3% |
3% |
5% |
8% |
4% |
|||||||
Austria |
1% |
1% |
2% |
-0.01* |
0.2* |
2% |
2% |
1% |
0.01 |
2.0 |
|||
Belgium |
7% |
3% |
8% |
* |
-0.08* |
0.4* |
7% |
13% |
5% |
* |
0.09* |
3.4* |
|
Bulgaria |
1% |
1% |
1% |
0.00 |
1.3 |
2% |
4% |
1% |
0.05* |
13.4* |
|||
Canada |
2% |
2% |
2% |
* |
0.01* |
1.5* |
4% |
6% |
4% |
* |
0.02* |
1.7* |
|
Croatia |
- |
- |
- |
3% |
5% |
1% |
0.04 |
1.1 |
|||||
Cyprus |
1% |
2% |
0% |
0.03 |
18.5 |
1% |
2% |
0% |
0.03 |
4.8 |
|||
Czech Republic |
2% |
2% |
2% |
-0.00 |
0.9 |
4% |
2% |
2% |
0.02 |
1.7 |
|||
Denmark |
6% |
7% |
8% |
-0.05* |
0.5* |
6% |
10% |
7% |
0.01 |
1.1 |
|||
Estonia |
0% |
1% |
0% |
0.00 |
3.4 |
2% |
4% |
1% |
* |
0.07* |
17.6* |
||
Finland |
4% |
6% |
4% |
0.01 |
1.1 |
6% |
8% |
5% |
0.00 |
1.0 |
|||
France |
- |
- |
- |
- |
- |
- |
|||||||
Germany |
3% |
2% |
4% |
-0.03* |
0.4* |
4% |
6% |
4% |
0.02 |
1.5 |
|||
Greece |
1% |
2% |
1% |
-0.01 |
0.7 |
3% |
4% |
2% |
0.01 |
1.6 |
|||
Hungary |
1% |
2% |
1% |
0.01 |
2.0 |
4% |
9% |
3% |
* |
0.08* |
7.1* |
||
Iceland |
1% |
1% |
1% |
-0.00 |
0.8 |
1% |
3% |
1% |
0.02 |
6.5 |
|||
Ireland |
8% |
5% |
8% |
* |
-0.02 |
0.8 |
9% |
11% |
8% |
0.03 |
1.4 |
||
Italy |
- |
- |
- |
- |
- |
- |
|||||||
Latvia |
2% |
3% |
3% |
* |
-0.01 |
0.4 |
8% |
12% |
6% |
0.06* |
2.2* |
||
Lithuania |
1% |
9% |
0% |
* |
0.06* |
14.7* |
7% |
17% |
3% |
* |
0.17* |
9.9* |
|
Luxembourg |
4% |
1% |
7% |
* |
-0.11* |
0.1* |
6% |
8% |
4% |
0.06* |
2.5 |
||
Malta |
2% |
3% |
2% |
-0.00 |
0.9 |
7% |
8% |
6% |
-0.02 |
0.7 |
|||
Netherlands |
- |
- |
- |
- |
- |
- |
|||||||
Norway |
2% |
1% |
2% |
-0.03* |
0.3* |
2% |
2% |
2% |
0.01 |
1.6 |
|||
Poland |
2% |
2% |
3% |
-0.01 |
0.4 |
5% |
8% |
5% |
0.02 |
1.6 |
|||
Portugal |
2% |
3% |
1% |
0.00 |
1.0 |
11% |
16% |
8% |
* |
0.22* |
6.2* |
||
Romania |
2% |
4% |
1% |
* |
0.05* |
18.7* |
12% |
16% |
7% |
0.12* |
2.7* |
||
Slovak Republic |
1% |
1% |
1% |
-0.00 |
0.5 |
2% |
8% |
1% |
* |
0.08* |
16.3* |
||
Slovenia |
1% |
1% |
1% |
0.01 |
2.5 |
4% |
8% |
1% |
0.07* |
6.8* |
|||
Spain |
2% |
2% |
3% |
-0.01 |
0.6 |
5% |
7% |
4% |
0.04* |
2.0* |
|||
Sweden |
3% |
1% |
3% |
-0.03 |
0.3 |
2% |
3% |
3% |
0.01 |
1.3 |
|||
United Kingdom |
17% |
14% |
21% |
-0.09* |
0.6* |
18% |
19% |
17% |
* |
0.02 |
1.1 |
Note: SP stands for standardised probabilities (age-standardised) estimated with logit models, GS for global significance, SII for slope index of inequalities, RII for relative index of inequalities and * means significant at 5%. There is no available data for heavy drinking in Chile, the United States, France, the Netherlands, and Italy. In Croatia, Cyprus, Estonia, Iceland, Luxembourg, it was not possible to control for covariates in the indirect standardisation.
Source: OECD calculations based on national health survey data.
Detailed results on health status
Annex Table 2.A.6. Descriptive statistics, standardised probabilities and inequality index: poor self-assessed health and limitations in daily activities
Poor self-assessed health |
Limitations in daily activities |
|||||||||||
---|---|---|---|---|---|---|---|---|---|---|---|---|
Prevalence |
SP – Low education |
SP – High education |
GS |
SII* |
RII* |
Prevalence |
SP – Low education |
SP – High education |
GS |
SII* |
RII* |
|
32% |
43% |
22% |
30% |
39% |
24% |
|||||||
EU28 |
34% |
46% |
24% |
29% |
40% |
24% |
||||||
OECD |
33% |
44% |
23% |
29% |
39% |
23% |
||||||
Total |
32% |
44% |
23% |
28% |
39% |
23% |
||||||
Austria |
22% |
37% |
16% |
* |
0.21* |
3.1* |
32% |
41% |
26% |
* |
0.17* |
1.7* |
Belgium |
23% |
34% |
15% |
* |
0.27* |
3.5* |
23% |
32% |
16% |
* |
0.22* |
2.8* |
Bulgaria |
34% |
46% |
26% |
* |
0.24* |
1.9* |
25% |
38% |
21% |
* |
0.21* |
2.2* |
Canada |
43% |
57% |
38% |
* |
0.25* |
1.8* |
19% |
29% |
19% |
* |
0.13* |
2.0* |
Chile |
42% |
49% |
26% |
* |
0.21* |
1.7* |
8% |
16% |
6% |
* |
0.08* |
3.2* |
Croatia |
41% |
52% |
30% |
* |
0.26* |
1.8* |
34% |
44% |
25% |
* |
0.24* |
1.9* |
Cyprus |
20% |
36% |
14% |
* |
0.29* |
4.0* |
22% |
33% |
17% |
* |
0.21* |
2.3* |
Czech Republic |
32% |
46% |
23% |
* |
0.27* |
1.9* |
36% |
44% |
28% |
* |
0.16* |
1.5* |
Denmark |
24% |
41% |
18% |
* |
0.27* |
2.9* |
37% |
45% |
33% |
0.11* |
1.3* |
|
Estonia |
42% |
52% |
34% |
* |
0.23* |
1.7* |
38% |
48% |
33% |
0.18* |
1.6* |
|
Finland |
37% |
49% |
27% |
* |
0.30* |
2.1* |
38% |
48% |
30% |
* |
0.18* |
1.6* |
France |
32% |
40% |
22% |
* |
0.27* |
2.4* |
26% |
35% |
20% |
* |
0.19* |
2.1* |
Germany |
29% |
41% |
22% |
* |
0.22* |
2.2* |
23% |
32% |
18% |
* |
0.16* |
2.0* |
Greece |
26% |
40% |
19% |
* |
0.31* |
2.9* |
30% |
41% |
23% |
* |
0.25* |
2.1* |
Hungary |
39% |
53% |
25% |
* |
0.38* |
2.7* |
30% |
43% |
22% |
* |
0.26* |
2.4* |
Iceland |
26% |
40% |
15% |
* |
0.34* |
3.9* |
26% |
35% |
20% |
* |
0.21* |
2.2* |
Ireland |
17% |
29% |
12% |
* |
0.28* |
4.3* |
28% |
39% |
22% |
* |
0.23* |
2.1* |
Italy |
31% |
37% |
22% |
* |
0.22* |
2.1* |
27% |
32% |
22% |
* |
0.14* |
1.7* |
Latvia |
51% |
61% |
37% |
* |
0.31* |
1.8* |
43% |
51% |
35% |
* |
0.19* |
1.5* |
Lithuania |
52% |
56% |
36% |
* |
0.28* |
1.6* |
36% |
49% |
27% |
* |
0.28* |
2.0* |
Luxembourg |
30% |
46% |
17% |
* |
0.41* |
4.5* |
36% |
45% |
25% |
* |
0.28* |
2.3* |
Malta |
17% |
25% |
12% |
* |
0.26* |
4.3* |
17% |
22% |
15% |
0.13* |
2.0* |
|
Netherlands |
23% |
33% |
17% |
* |
0.20* |
2.5* |
29% |
36% |
23% |
* |
0.14* |
1.6* |
Norway |
21% |
33% |
15% |
* |
0.23* |
3.2* |
17% |
26% |
11% |
* |
0.19* |
3.1* |
Poland |
38% |
46% |
28% |
* |
0.25* |
1.8* |
23% |
33% |
19% |
* |
0.15* |
1.8* |
Portugal |
50% |
57% |
28% |
* |
0.48* |
2.5* |
32% |
37% |
25% |
* |
0.23* |
1.9* |
Romania |
28% |
35% |
23% |
* |
0.12* |
1.4* |
16% |
23% |
12% |
* |
0.07* |
1.5* |
Slovak Republic |
35% |
49% |
23% |
* |
0.37* |
2.6* |
40% |
50% |
30% |
* |
0.25* |
1.8* |
Slovenia |
35% |
45% |
23% |
* |
0.29* |
2.4* |
38% |
45% |
31% |
* |
0.15* |
1.5* |
Spain |
29% |
37% |
20% |
* |
0.27* |
2.4* |
25% |
32% |
20% |
* |
0.16* |
1.8* |
Sweden |
25% |
35% |
19% |
* |
0.16* |
2.0* |
30% |
38% |
25% |
0.12* |
1.5* |
|
United Kingdom |
24% |
41% |
18% |
* |
0.26* |
2.6* |
30% |
43% |
26% |
* |
0.17* |
1.7* |
United States |
43% |
60% |
30% |
* |
0.35* |
2.2* |
24% |
36% |
22% |
* |
0.16* |
1.9* |
Note: SP stands for standardised probabilities (age-sex standardised) estimated with logit models, GS for global significance, SII for slope index of inequalities, RII for relative index of inequalities and * means significant at 5%.
Source: OECD calculations based on national health survey data.
Annex Table 2.A.7. Descriptive statistics, standardised probabilities and inequality index: multiple chronic conditions
Multiple chronic conditions |
||||||
---|---|---|---|---|---|---|
Prevalence |
SP – Low education |
SP – High education |
GS |
SII* |
RII* |
|
EU28 |
32% |
38% |
27% |
|||
OECD |
32% |
38% |
27% |
|||
Total |
31% |
37% |
26% |
|||
Austria |
34% |
43% |
29% |
* |
0.16* |
1.7* |
Belgium |
28% |
36% |
22% |
* |
0.16* |
1.8* |
Bulgaria |
26% |
34% |
25% |
* |
0.09* |
1.4* |
Canada |
15% |
23% |
15% |
* |
0.09* |
1.9* |
Croatia |
37% |
42% |
29% |
* |
0.16* |
1.5* |
Cyprus |
20% |
32% |
16% |
* |
0.20* |
2.5* |
Czech Republic |
30% |
36% |
27% |
* |
0.12* |
1.4* |
Denmark |
28% |
36% |
23% |
* |
0.14* |
1.6* |
Estonia |
26% |
31% |
25% |
0.06* |
1.2* |
|
Finland |
49% |
51% |
42% |
* |
0.12* |
1.2* |
France |
36% |
39% |
30% |
* |
0.12* |
1.4* |
Germany |
50% |
51% |
45% |
* |
0.10* |
1.2* |
Greece |
26% |
32% |
21% |
* |
0.16* |
1.7* |
Hungary |
37% |
45% |
31% |
* |
0.17* |
1.6* |
Iceland |
37% |
44% |
29% |
* |
0.22* |
1.8* |
Ireland |
24% |
32% |
20% |
* |
0.17* |
1.8* |
Italy |
32% |
35% |
26% |
* |
0.13* |
1.5* |
Latvia |
41% |
43% |
37% |
0.08* |
1.2* |
|
Lithuania |
31% |
38% |
27% |
* |
0.13* |
1.4* |
Luxembourg |
39% |
44% |
28% |
* |
0.24* |
1.9* |
Malta |
23% |
26% |
20% |
0.09* |
1.4* |
|
Netherlands |
28% |
34% |
22% |
* |
0.15* |
1.7* |
Norway |
24% |
31% |
17% |
* |
0.18* |
2.1* |
Poland |
35% |
37% |
32% |
* |
0.05* |
1.1* |
Portugal |
43% |
48% |
34% |
* |
0.23* |
1.7* |
Romania |
18% |
22% |
18% |
0.00 |
1.0 |
|
Slovak Republic |
30% |
42% |
25% |
* |
0.21* |
1.9* |
Slovenia |
41% |
45% |
36% |
0.10* |
1.3* |
|
Spain |
30% |
34% |
23% |
* |
0.19* |
1.7* |
Sweden |
31% |
36% |
27% |
0.07* |
1.3* |
|
United Kingdom |
25% |
34% |
23% |
* |
0.12* |
1.5* |
United States |
18% |
25% |
13% |
* |
0.13* |
2.1* |
Note: SP stands for standardised probabilities (age-sex standardised) estimated with logit models, GS for global significance, SII for slope index of inequalities, RII for relative index of inequalities and * means significant at 5%. There is no available data for multiple chronic conditions in Chile.
Source: OECD calculations based on national health survey data.
Annex Table 2.A.8. Inequality index: income-related health inequalities
Poor self-assessed health |
Activity limitation |
Multiple chronic conditions |
||||
---|---|---|---|---|---|---|
SII* |
RII* |
SII* |
RII* |
SII* |
RII* |
|
Austria |
0.20* |
2.9* |
0.15* |
1.6* |
0.11* |
1.4* |
Belgium |
0.29* |
3.8* |
0.25* |
3.2* |
0.17* |
1.8* |
Bulgaria |
0.19* |
1.7* |
0.21* |
2.1* |
0.07* |
1.3* |
Canada |
0.33* |
2.2* |
0.22* |
3.3* |
0.15* |
2.8* |
Chile |
0.13* |
2.2* |
0.06* |
2.1* |
- |
- |
Croatia |
0.35* |
2.5* |
0.32* |
2.7* |
0.27* |
2.1* |
Cyprus |
0.22* |
2.6* |
0.20* |
2.2* |
0.16* |
2.0* |
Czech Republic |
0.24* |
1.8* |
0.16* |
1.4* |
0.10* |
1.3* |
Denmark |
0.36* |
5.8* |
0.27* |
2.2* |
0.26* |
2.5* |
Estonia |
0.44* |
4.2* |
0.35* |
3.4* |
0.19* |
2.4* |
Finland |
0.30* |
2.1* |
0.19* |
1.6* |
0.16* |
1.3* |
France |
0.31* |
2.9* |
0.22* |
2.4* |
0.14* |
1.5* |
Germany |
0.25* |
2.4* |
0.17* |
2.2* |
0.11* |
1.3* |
Greece |
0.20* |
1.9* |
0.14* |
1.5* |
0.10* |
1.4* |
Hungary |
0.35* |
2.5* |
0.27* |
2.5* |
0.17* |
1.6* |
Iceland |
0.24* |
2.6* |
0.24* |
2.6* |
0.21* |
1.8* |
Ireland |
0.02 |
1.1 |
0.05* |
1.1* |
0.05* |
1.2* |
Italy |
0.19* |
1.9* |
0.14* |
1.7* |
0.11* |
1.4* |
Latvia |
0.28* |
1.7* |
0.18* |
1.5* |
0.14* |
1.4* |
Lithuania |
0.16* |
1.3* |
0.23* |
1.8* |
0.14* |
1.5* |
Luxembourg |
0.43* |
5.8* |
0.29* |
2.4* |
0.25* |
2.0* |
Malta |
0.21* |
3.4* |
0.14* |
2.2* |
0.12* |
1.6* |
Netherlands |
0.24* |
2.9* |
0.19* |
1.9* |
0.17* |
1.9* |
Norway |
0.21* |
2.9* |
0.17* |
2.8* |
0.14* |
1.8* |
Poland |
0.21* |
1.7* |
0.18* |
2.1* |
0.06* |
1.2* |
Portugal |
0.33* |
1.9* |
0.20* |
1.8* |
0.15* |
1.4* |
Romania |
0.09* |
1.3* |
0.03* |
1.2* |
-0.01 |
0.9 |
Slovak Republic |
0.28* |
2.0* |
0.18* |
1.5* |
0.21* |
1.8* |
Slovenia |
0.34* |
3.3* |
0.23* |
1.9* |
0.16* |
1.5* |
Spain |
0.29* |
2.7* |
0.19* |
2.0* |
0.17* |
1.6* |
Sweden |
0.31* |
3.9* |
0.26* |
2.6* |
0.16* |
1.7* |
United Kingdom |
0.33* |
3.4* |
0.27* |
2.2* |
0.20* |
2.0* |
United States |
0.37* |
2.3* |
0.25* |
2.8* |
0.18* |
2.8* |
Note: SII for slope index of inequalities, RII for relative index of inequalities, and * means significant at 5%. There is no available data for multiple chronic conditions in Chile.
Source: OECD calculations based on national health survey data.
Notes
← 1. EHIS enquires about the number and duration of absences but the question’s formulation is such that results cannot be used to produce a more refined estimate of the loss of productivity due to ill health. Furthermore, they are not comparable with other national health surveys.
← 2. Essentially this measure is an increase in labour supply – not an increase in actual employment which would require a structural model.
← 3. For a more technical presentation of the indirect standardisation method, see Box 3.1 in Chapter 3. Control variable in the indirect standardisation process include income, marital status, education, labour status, size of household, degree of urbanisation.
← 4. The risk of all-cause mortality, and of cancers generally rises with increasing levels of alcohol consumption, and it is important to note that the level of alcohol consumption that minimises health loss is zero (Griswold et al., 2018[48]).
← 5. While there are sophisticated methods to reflect the information contained in the categorical self-assessed health status variables (such as generating predictions of an underlying latent variable and rescale these predictions to a 0-1 interval) (O’Donnell et al., 2007[47]), the decision was taken to dichotomise the variable as this was only one of the variables describing health status.
← 6. A robustness analysis, which consisted of attributing a rank to each country for its level of inequality (measured by the SII) for each health variables and summing of the three ranks, confirmed the grouping of countries.