Building on the OECD Regional and Metropolitan Databases, the OECD indicator framework on localising the United Nations Sustainable Development Goals (SDGs) and the OECD International Programme for Action on Climate (IPAC), this chapter presents a territorial climate indicator framework designed to monitor climate action and resilience efforts in cities and regions in a globally comparable manner. The framework also incorporates a method to measure the distance of cities and regions to net zero objectives. The chapter provides technical guidance on how to use the framework as a tool to better understand the diversity of local greenhouse gas emissions and emission reduction potential, as well as locally specific impacts and risks of climate change, all of which can help inform place‑specific climate strategies.
A Territorial Approach to Climate Action and Resilience
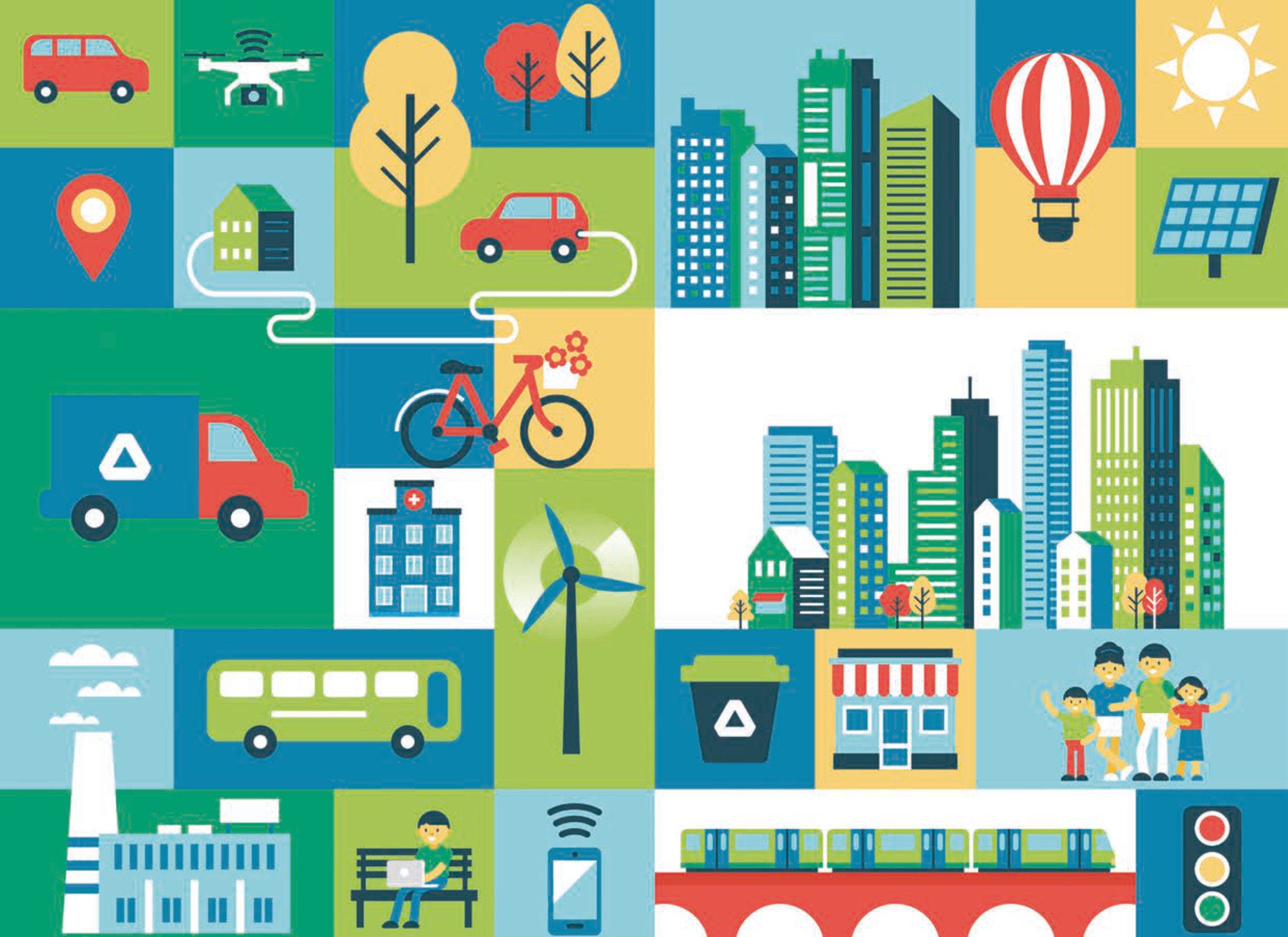
2. Introducing the OECD territorial climate indicator framework
Abstract
Introduction: Why measure local climate action?
A comprehensive understanding of climate challenges and opportunities at different territorial scales is a prerequisite for a territorial approach to climate action and resilience. As discussed in Chapter 1, a more granular understanding of how much greenhouse gas (GHG) is emitted and which climate impacts and risks exist in cities and regions is crucial for national governments to tailor their policies and strategies across places and redirect budgets towards the right locations. For local and regional governments, a comparative assessment of where they stand with regard to global and national climate targets, as well as against their peers, allows them to set priorities and allocate resources accordingly.
Typically, national governments use sector-based climate data at the national level in developing and implementing their climate policies, both for mitigation and adaptation. However, national averages tend to mask territorial disparities, which may hamper the effective implementation of climate policy interventions. Emerging evidence demonstrates that there are non-negligible territorial disparities in terms of climate impact in different places (Chapter 3). For example, during the past 5 years, daytime temperatures in half of OECD cities were 3 degrees Celsius (°C) higher than in the surrounding areas during the summer (OECD, 2022[1]). Some cities were even 7°C warmer. The diversity of local climate impacts implies that some of the climate systems tipping points (as discussed in Chapter 1) can be reached much faster in some geographical locations, creating potentially abrupt and irreversible changes to the environment. Moreover, place-specific climate impacts and risks are often people-specific, as most vulnerable groups in society tend to be more exposed to the impacts of climate change.
Only limited information about how cities and regions fare with regard to global and national climate targets is available. For example, the OECD’s ongoing research on localising the United Nations Sustainable Development Goals (SDGs) has demonstrated that none of the OECD regions has achieved the suggested outcomes for SDG 13 on climate action but they stand, on average, one-third of the way from accomplishing the suggested outcomes. Likewise, a majority (98%) of OECD cities have not achieved the target values of less than 111 tonnes of carbon dioxide equivalent per gigawatt-hour (tCO2-eq/GWh) and no increase in the demand of energy to cool buildings, measured as cooling degree days (OECD, 2020[2]). Developing more data in this area in a globally comparable manner will be instrumental for both national and subnational governments to understand where they stand and take necessary policy action.
Against this backdrop, this chapter presents an OECD territorial climate indicator framework designed to better understand locally specific climate trends and challenges as well as to monitor climate action and resilience efforts of cities and regions. This framework allows the unveiling of territorial disparities in terms of GHG emissions and trends, as well as climate impacts and risks. Furthermore, the framework incorporates a method to measure the “distance” of cities and regions from achieving net zero objectives, with emphasis on facilitating international comparisons. The objective of the chapter is to provide national and subnational governments with technical guidance on how to use the OECD territorial climate framework as a tool to advance place-based climate action and resilience policies.
Taking stock of existing climate-related indicator initiatives
Existing initiatives measuring local climate action
A handful of initiatives have developed indicator frameworks to measure GHG emissions, climate impacts and risks at the subnational scale and provided technical support and guidance for cities and regions (Table 2.1). A stocktaking exercise on such initiatives has led to the following key findings:
Initiatives such as the Carbon Disclosure Project (CDP) Cities, States and Regions Open Data Portal specifically emphasise climate action, while others, such as the European Bank for Reconstruction and Development (EBRD) Green City Methodology, extend their scope to wider sustainability.
Regarding the scope, many initiatives provide a self-assessment and reporting methodology for cities and regions. Such examples include the CDP-ICLEI Open Data Portal, the Global Covenant of Mayors (GCoM) Sustainable Energy and Climate Action Plan (SECAP), C40’s “Measuring progress in urban climate change adaptation” and the World Bank Urban Sustainability Framework. They assist cities in gathering data and reporting their climate action planning and implementation. Most of the initiatives focus on climate mitigation although there is an emerging trend to measure adaptation (e.g. the International Organization for Standardization [ISO] Sustainable Cities and Communities).
Most initiatives rely on the voluntary participation of cities in terms of data collection. For example, the GCoM SECAP compiles and discloses data reported by participating cities and regions. Very few initiatives (e.g. Net Zero Tracker) collect and disclose data by themselves.
Many initiatives allow flexibility in terms of reporting data, so cities and regions can customise indicator frameworks to their specific circumstances. For instance, the ERDB Green City Methodology and C40 Climate Change Adaptation Monitoring, Evaluation and Reporting (CCA MER) allow cities to select the most suitable indicators for their needs.
Some initiatives assist cities in keeping track of the outcomes of their climate action plans, such as the C40 CCA MER, so cities can determine the success or failure of their climate adaptation efforts. Others aim to visualise the distance of cities and regions to achieving climate targets. The Green City Methodology encourages cities to benchmark their mitigation performance in different sectors against national targets to identify areas of priority for mitigation investment through a traffic light system. None of the initiatives provide a tool to compare cities and regions with global climate targets.
Very few initiatives target metropolitan areas or functional urban areas (FUAs) beyond municipal administrative borders. SECAP offers the possibility of a joint action plan developed collectively by a group of neighbouring local authorities, aiming to achieve more effective results together than isolated. This joint initiative is targeted at small municipalities, inter-municipal networks and urban agglomerations such as a metropolis and its suburbs, among other cases.
The application of these indicator frameworks is primarily local and they are not designed for aggregation or disaggregation of climate data across different territorial scales. This means the indicators essentially lack standardisation or compatibility across places. For example, although a common measurement framework is provided to estimate GHG emissions, cities can choose different methodologies, thereby limiting data comparability as well as the possibility of aggregation and disaggregation of data.
While these key findings confirm the need for and usefulness of such indicator initiatives, they also demonstrate a lack of comparability and compatibility across cities, regions and countries.
Table 2.1. Mapping of international initiatives supporting the development of subnational climate data
Name |
Organisation and year |
Mandate/objective |
Scope (adaptation/ mitigation) |
Type of data (official statistics, geospatial data, self-reported) |
Geographical coverage |
Data collected |
Source |
---|---|---|---|---|---|---|---|
Cities, States and Regions Open Data Portal |
Carbon Disclosure Project (CDP) in collaboration with the International Council for Local Environmental Initiatives (ICLEI), referred to as CDP-ICLEI, 2019 |
Enable cities to report their climate commitments, provide guidance and support in enhancing their climate targets and benchmark the progress over time in climate risks, hazards, emissions and sector-specific data (i.e. transport, water and waste), while facilitating the assessment of financial implications and co-benefits of climate actions such as improved health, biodiversity and social inclusion. |
Mitigation, adaptation |
Self-reported data by cities and regions |
Global |
Self-reported environmental data from over 20 000 entities, including governance (targets, adaptation and mitigation plans) and primary environmental data (emissions, resource consumption). |
|
Sustainable Cities and Communities |
The International Organization for Standardization (ISO), 2016 |
Streamline city comparisons and enable alignment with other global action initiatives by establishing a standardised framework for evaluating urban sustainability based on city preparedness and resilience, using indicators that are tailored to foster resilient and smart cities. |
Mitigation, adaption |
Does not collect data, provides a methodology to carry out data collection and defines indicators |
Global |
Indicators for environmental, social and governance critical infrastructure, disaster, hazard mapping, water, resilience, resilient city stress, vulnerability and risk. |
|
Net Zero Tracker |
Energy and Climate Intelligence Unit, Data‑Driven EnviroLab, NewClimate Institute, Oxford Net Zero, 2021 |
Increase transparency and accountability of targets for net zero emissions pledged by national and subnational governments (including cities and regions). |
Mitigation |
Data collected from publicly available sources |
Global |
Information for each target, including the year of adoption, gas coverage, if there is any publicly available plan, reporting mechanism, if the net zero target is adopted by law, in a policy document, declaration/pledge or is proposed/in discussion. |
|
Geospatial Indicators Dashboard |
UrbanShift; Cities4Forest, 2022 |
Enable urban decision makers, researchers and stakeholders to utilise a data-driven approach to urban issues and opportunities and ensure alignment with global climate change initiatives, such as the SDGs. |
Mitigation, adaptation |
Open-source data, geospatial data |
Participating cities |
Indicators across seven key sustainability themes, including air quality, flooding, extreme heat, biodiversity, greenspace access, land protection and restoration and climate mitigation. |
|
Green City Methodology |
European Bank for Reconstruction and Development (ERDB), 2018 |
Assess and prioritise environmental challenges to develop an action plan to tackle the challenges through policy intervention and sustainable infrastructure investments, facilitate and stimulate public or private green investments in water and wastewater, urban transport, district energy, energy efficiency in buildings, renewable energy, solid waste and climate resilience, and provide technical support to city administrators to ensure that infrastructure investments are implemented effectively. |
Mitigation, adaptation |
Does not collect data |
Global |
Environmental, social and technical indicators across sectors (transport, buildings, industry and energy), including emissions, energy consumption, efficiency indicators, resilience and waste. Other data also include health quality indicators (air, water and soil quality). |
|
Urban Climate Change Adaptation (CCA) Monitoring, Evaluation, and Reporting (MER) Framework |
C40, 2019 |
Assess and monitor cities’ climate change adaptation plans and evaluate their results to make a case for adaptation, secure funding and implement plans as effectively as possible. |
Adaptation |
Does not collect data, provides a methodology to carry out data collection and defines indicators |
Global, but mainly C40 cities |
Output, outcome and impact. |
|
Sustainable Energy and Climate Action Plan (SECAP) |
Global Covenant of Mayors for Climate and Energy (GCoM), 2015 |
Provide a comprehensive strategy for both climate mitigation and adaptation plans, streamlining the process of formulating these plans through a harmonised data compilation and reporting framework available for all GCoM signatories. |
Mitigation, adaptation |
Does not collect data, provides a methodology to carry out data collection and defines indicators |
GcoM signatories, cities and regions (joint SECAP) |
Environmental, social, and corporate governance (ESG) indicators. |
Source: Based on sources listed in the respective column.
Existing climate-related indicator frameworks developed by the OECD
The OECD has a well-established expertise in generating data across a wide range of social, economic and environmental domains, including climate change. To ensure a more granular understanding of territorial disparities and allow international comparison, the OECD has developed several indicator frameworks with national and subnational climate data.
At the national level, the OECD International Programme for Action on Climate (IPAC) has developed a dashboard to support countries’ efforts in monitoring progress towards net zero emissions and a more resilient economy by 2050. The dashboard builds on the extensive availability of climate statistics from the OECD, the International Energy Agency (IEA) and the International Transport Forum (ITF). At the subnational level, the OECD Regional Database and the OECD Metropolitan Database provide a unique set of comparable statistics and indicators on 2 000 regions and 650 metropolitan areas across OECD countries. In addition, while not exclusively centred on climate, the OECD localised indicator framework for the SDGs incorporates relevant indicators at the subnational level within the broader SDG framework (Table 2.2).
Table 2.2. Existing OECD climate-related indicator frameworks
Indicator framework |
Description |
Geographical coverage |
Data sources |
---|---|---|---|
IPAC Dashboard |
The selection of indicators for IPAC builds on the conceptual frameworks and guidance elaborated for the development, measurement and use of environmental and green growth indicators adapted to climate change issues. The IPAC framework uses an adapted “pressure-state-response” model to structure the indicators. This adapted model integrates the topics covered in the assessments of the IPCC and is used in the United Nations Statistics Division (UNSD) and United Nations Economic Commission for Europe (UNECE) indicator frameworks. The criteria used for selecting the indicators and validating their choice are those developed with OECD member countries and already used for the OECD’s environmental and green growth indicators: policy relevance and utility for users, analytical soundness and measurability. |
National level |
Statistics from the OECD, IEA, ITF, Nuclear Energy Agency (NEA) and other international sources, including the United Nations Framework Convention on Climate Change (UNFCCC) |
OECD localised indicator framework for the SDGs |
The OECD localised framework for the SDGs is a unique, internationally comparable SDG indicator framework for cities and regions. It aims to support cities and regions in OECD and partner countries in measuring their distances towards achieving the SDGs. The indicator framework allows for documenting the share of OECD cities and regions that are on track with respect to the 2030 goals and quantifies the average distance that these cities and regions must travel in order to reach the desired outcomes. To do this, it normalises the SDG indicators from 0 to 100 – where 100 is the suggested end value of an indicator to be achieved by 2030 – and aggregates headline indicators that belong to the same goal to provide an index score towards each of the 17 SDGs. The distance to each SDG is defined as the number of units the index needs to travel to reach the maximum score of 100. The indicator framework includes more than 130 indicators and covers more than 600 regions and 600 cities in 65 out of the 105 SDG targets where regional and local governments are considered instrumental (although the regional and city coverage can vary widely from one indicator to another). It includes six indicators under SDG 13 on climate and several other climate-related indicators (e.g. percentage of total electricity production that comes from renewable sources on SDG 7; percentage of population with access to at least 1 hectare of green urban areas on SDG 11; and municipal waste rate [kilos per capita] on SDG 12). |
Subnational level – TL2, TL3 and FUAs |
Statistics of the OECD Regional and Metropolitan Databases and data from large international databases (e.g. Gallup World Poll, Global Power Plant Database) |
OECD Regional Database |
The OECD Regional Database provides a unique set of comparable statistics and indicators on about 2 000 regions in 36 OECD countries, plus Brazil, the People’s Republic of China, Colombia, India, Peru, the Russian Federation, South Africa and Tunisia. It currently encompasses yearly time series for more than 100 indicators of demography, economic accounts, labour market, social and innovation themes in OECD member countries and other economies. The OECD classifies its regions on two territorial levels, reflecting the administrative organisation of countries. The 398 OECD large (TL2) regions represent the first administrative tier of subnational government, for example, the Ontario Province in Canada. The 2 251 OECD small (TL3) regions correspond to administrative regions, with the exception of Australia, Canada and the United States. These TL3 regions are contained in a TL2 region, with the exception of the United States, for which the economic areas cross the states’ borders. For New Zealand, TL2 and TL3 levels are equivalent and defined by regional councils. All regions are defined within national borders. This classification – which, for European countries, is largely consistent with the Eurostat NUTS 2013 classification – facilitates greater comparability of geographic units at the same territorial level. Indeed, these two levels, which are officially established and relatively stable in all member countries, are used as a framework for implementing regional policies in most countries. |
Subnational level – TL2, TL3 |
Input from member states and data from large international databases |
OECD Metropolitan Database |
The OECD Metropolitan Database provides a set of economic, environmental, social, labour market and demographically estimated indicators on the 649 OECD metropolitan areas (FUAs with 250 000 or more inhabitants). The database relies on a consistent definition of FUAs applied across countries, which was developed in collaboration with the European Union. Using population density and travel-to-work flows as key information, an FUA consists of a densely inhabited city and a surrounding area (commuting zone) whose labour market is highly integrated with the city. The ultimate aim of the OECD-EU approach to FUAs is to create a harmonised definition of cities and their areas of influence for international comparisons as well as for policy analysis on topics related to urban development. Using FUAs allows designing policies at the right scale, for example, for mobility and accessibility to services. At the same time, FUAs provide a harmonised methodology to compare similar urban units in size and function. This is particularly relevant in the context of the SDGs, a universal global agenda that requires comparability across the globe in order to track progress towards sustainable development. |
Subnational level – FUAs |
Input from member states and data from large international databases |
OECD database on subnational government structure and finance |
The OECD database on subnational government structure and finance provides data on territorial organisation at subnational levels and public finance. Financial data cover the general government and subnational government (state and local government levels) sectors in OECD member countries and in the European Union. Four main dimensions are presented: expenditure (including investment), revenue, budget balance and debt. The database is updated annually. |
Subnational level – TL2, TL3 |
Data from OECD, International Monetary Fund (IMF) and Eurostat databases |
OECD-United Cities and Local Governments (UCLG) World Observatory on Subnational Government Finance and Investment |
The World Observatory on Subnational Government Finance and Investment (SNG-WOFI) is a joint endeavour led by the OECD and UCLG. It provides subnational government finance data at the global level, covering 135 countries worldwide in the latest 2022 edition. The data are updated every three years. For federal countries, the database differentiates between local and state/provincial government data. For unitary countries, the data concern the consolidated subnational government sector as a whole. |
Subnational government sector |
Input from member states and statistics from national databases |
OECD database on subnational government climate finance |
The OECD database on subnational government climate finance provides data on subnational government climate-significant expenditure and investment for 33 OECD and European Union (EU) countries, from 2001 to 2019. Data were collected using a standardised pioneer methodology based on national accounts data. For federal countries, the database differentiates between local and state/provincial government data. |
Subnational government sector, TL2 |
Data from OECD, IMF and Eurostat databases |
Compendium of Financial Instruments that Support Subnational Climate Action in OECD and EU Countries |
The Compendium of Financial Instruments that Support Subnational Climate Action in OECD and EU Countries provides an overview of some climate-related public revenue sources from higher levels of governments (the European Union, central governments and federal governments in federal countries) available to subnational governments (as of 2022). The compendium is complemented by an analysis of the diversity of climate-related revenue sources available to subnational governments and the gaps that exist. |
National |
Input from member states |
Source: Based on OECD (2022[10]), International Programme for Action on Climate, https://www.oecd.org/climate-action/ipac/; OECD (2020[2]), A Territorial Approach to the Sustainable Development Goals: Synthesis Report, https://doi.org/10.1787/e86fa715-en.
The OECD localised indicator framework for the SDGs is particularly inspiring for designing a global territorial climate indicator framework in two aspects. First, it allows international comparability across countries, cities and regions. As it uses the indicators for cities and regions in OECD member and partner countries that are consolidated in the OECD Metropolitan and Regional Databases, the indicator framework provides greater comparability of geographic units at the same territorial level across the OECD countries and beyond. Second, the OECD localised indicator framework for the SDGs allows cities and regions to measure their distances to global and national targets. It defines “end values” for 2030 based on the global targets of the SDGs to create a composite index by SDG. The composite index helps to visualise and communicate the distance each region must travel to reach the end value. The index by goal is estimated as the aggregation of normalised indicators that take values from 0 to 100, where 0 is the worst possible outcome and 100 is the end value of the goal. As featured in the example for the region of Flanders (Belgium), the index shows how far the region and the country are from reaching the suggested end value for each SDG (Figure 2.1).
The IPAC Dashboard also measures the annual difference between GHG emissions and nationally determined contribution (NDC) 2030 target and GHG emissions levels in comparison with the NDC target to provide an overview of the emission trajectory towards GHG neutrality.
Figure 2.1. Measuring the distance to achieving global and national targets of the SDGs: Example of the region of Flanders, Belgium
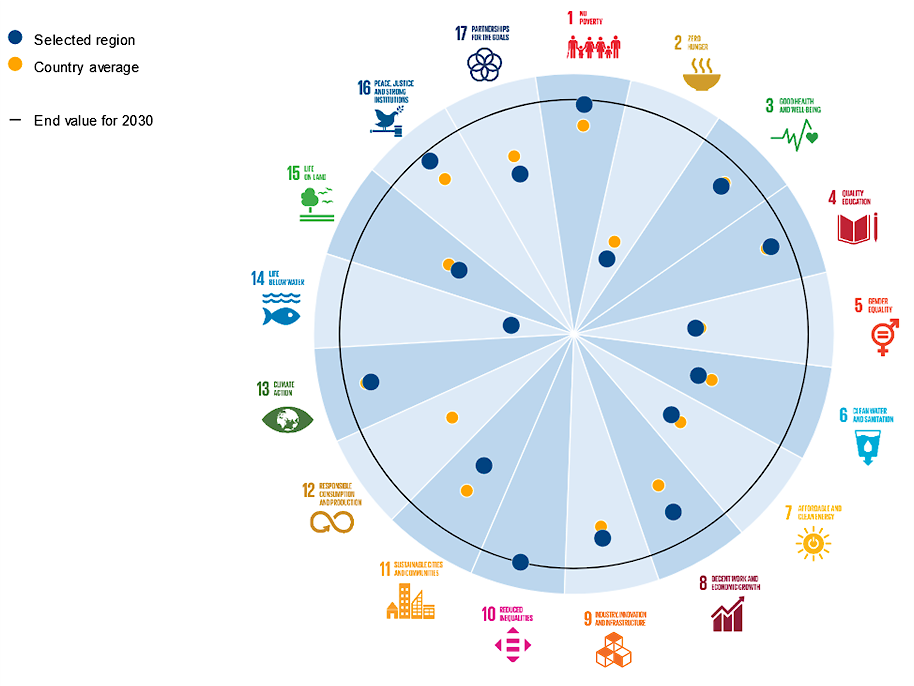
Source: OECD (2022[11]), Measuring the Distance to the SDGs in Regions and Cities, https://www.oecd-local-sdgs.org/index.html.
Overview of the OECD territorial climate indicator framework
This section offers a comprehensive overview of the OECD territorial climate indicator framework, highlighting its essential characteristics and distinctive attributes, including international comparability, the ability to aggregate and disaggregate climate data across various geographical scales and the possibility of measuring progress towards achieving global and national climate targets. The framework draws on the insights from existing OECD climate-related indicators presented in the previous section.
A “pressure-state-response” approach
The OECD territorial climate indicator framework uses the “pressure-state-response” approach to structure the indicators, aligning with the OECD IPAC Dashboard (Figure 2.2) as well as with the assessment reports of the IPCC. “Pressure indicators” primarily cover aspects related to climate mitigation, encompassing trends and drivers of GHG emissions. Conversely, “state indicators” predominantly feature indicators associated with climate adaptation and resilience, including the exposure to climate-related hazards and socio-economic factors driving vulnerability. “Response indicators” focus on actions and opportunities to address climate challenges. To enhance clarity, the framework is hereinafter presented under three categories: i) climate mitigation; ii) climate adaptation and resilience; and iii) actions and opportunities.
Figure 2.2. The pressure-state-response approach used in the IPAC indicator framework
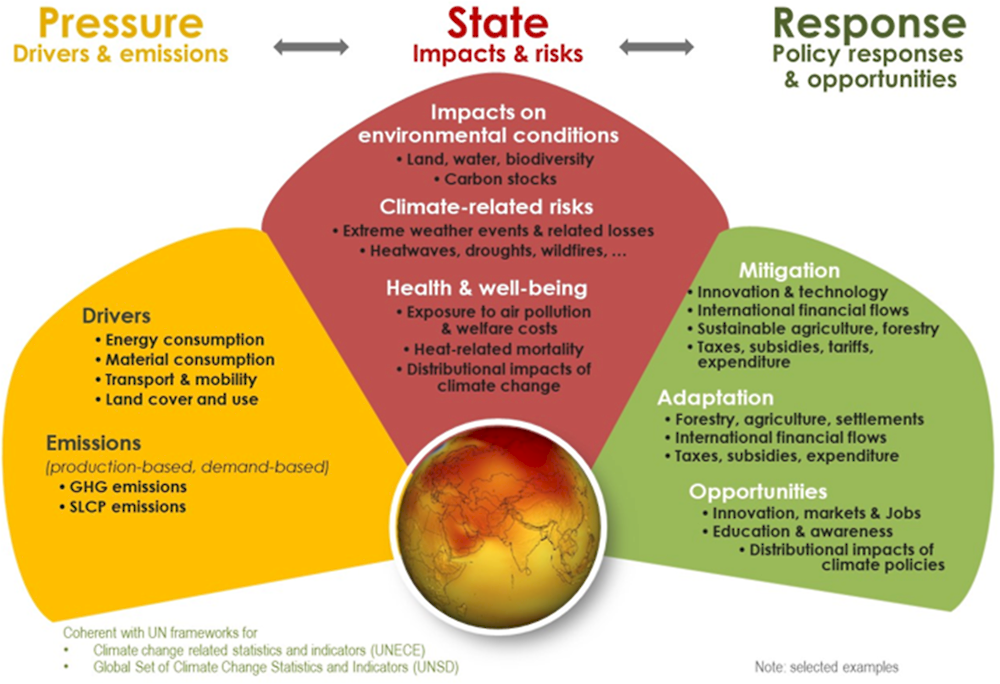
Source: OECD (2021[12]), “International Programme for Action on Climate (IPAC) - Proposal for a first edition of the indicator dashboard”, OECD, Paris.
The selection of indicators under each category is determined by the availability of data sources, their measurability and their policy relevance at the subnational level. The indicator framework consists of 45 indicators: 19 climate mitigation indicators, 20 climate adaptation and resilience indicators, and 6 indicators for actions and opportunities (Table 2.3). The rationale for each indicator is provided in the next section of this chapter and a complete list of territorial climate indicators and references are found in Annex 2.A. For 41 of the 45 indicators, data were available and collected via publicly available data sources. Although data are still to be estimated (i.e. either to be identified or computed) for the remaining six indicators, they are included in the framework due to their importance. They include indicators of consumption-based GHG emissions (Indicator 5), energy mix in total energy supply (Indicator 8), total final energy consumption by source (Indicator 9), and existence of subnational mitigation and adaptation targets and compliance with national targets (Indicator 40).
Table 2.3. OECD territorial climate indicator framework by category
Climate mitigation (19) |
Climate adaptation and resilience (20) |
Actions and opportunities (6) |
---|---|---|
1. Total GHG emissions, level and % change 2. GHG emissions per capita, level and % change 3. GHG emission intensities per unit of gross domestic product (GDP), level and % change 4. GHG emissions by sector: share of total emissions, level, per capita and % change 5. Consumption-based GHG emissions (to be estimated) 6. Electricity generation by source 7. Carbon intensity of electricity generation 8. Energy mix in total energy supply (to be estimated) 9. Total final energy consumption by source (to be estimated) 10. Emission intensity of the manufacturing industry 11. Access to public transport 12. Private vehicle ownership 13. Electric and hybrid vehicle adoption 14. Cooling and heating degree days, levels and change, residential built-up volume 15. Built-up area growth 16. Built-up area per capita 17. Difference between built-up area growth and population growth 18. Waste generation 19. Waste recovery |
Exposure to climate hazards 20. Air temperature and change 21. Number of hot days and change 22. Number of tropical nights and change 23. Number of icing days and change 24. Heat stress exposure 25. Urban heat island intensity (daytime and night-time) 26. Population exposure to fires 27. Burnt area by land cover type 28. Forest exposure to wildfire danger 29. Population exposure to river flooding 30. Population exposure to coastal flooding 31. Agricultural drought 32. Cumulated precipitation and change 33. Extreme precipitation days and change 34. Built-up exposure to violent storms |
40. Existence of subnational mitigation and adaptation targets and compliance with national targets (to be estimated) 41. Citizens’ satisfaction with efforts to preserve the environment 42. Green areas in cities 43. Climate-significant expenditure and investment 44. Existence of climate-related funds, grants and subsidies for subnational action 45. Patent applications in climate mitigation and adaptation technologies as % of total technologies |
Socio-economic factors driving vulnerability 35. Population density 36. Population aged < 5 37. Population aged > 70 38. Unemployment rate 39. Poverty rate |
International comparability across territorial scales
The OECD territorial climate indicator framework offers a unique approach that enables international comparability of cities and regions. Based on the OECD territorial classification and FUA methodology,1 the indicator framework allows countries, cities and regions to monitor and evaluate GHG emissions, climate impacts and risks using a standardised set of territorial climate indicators.
Among the 45 indicators included in the framework, 36 are available at TL2 level, 35 at TL3 level and 25 at the FUA level (Table 2.4). This harmonised and standardised set of indicators establishes a common language for national and subnational governments. It equips both with an integrated overview across territorial scales and a more in-depth analysis of specific regions or cities. This helps national governments to customise their analysis to suit specific contexts and needs, whether for national-level assessments, regional comparisons or targeted interventions within smaller geographic areas. For some indicators such as urban heat island intensity and green areas, this framework can also be extended to a more granular geographical level within FUAs corresponding to municipalities, counties or districts depending on countries, and referred to as small area units (SAUs).
Table 2.4. Geographical resolution and coverage of the OECD territorial climate indicator framework
Indicator |
TL2 (36) |
TL3 (35) |
FUA (25) |
---|---|---|---|
Climate mitigation |
|||
1. Total GHG emissions, level and % change |
(*) |
||
2. GHG emissions per capita, level and % change |
(*) |
||
3. GHG emission intensities per unit of GDP, level and % change |
|||
4. GHG emissions by sector: share of total emissions, level, per capita and % change |
(*) |
||
5. Consumption-based GHG emissions (to be estimated) |
To be defined |
||
6. Electricity generation by source carbon intensity of electricity generation |
|||
7. Carbon intensity of electricity generation |
|||
8. Energy mix in total energy supply (to be estimated) |
To be defined |
||
9. Total final energy consumption by source (to be estimated) |
To be defined |
||
10. Emission intensity of the manufacturing industry |
|||
11. Access to public transport |
(**) |
||
12. Private vehicle ownership |
|||
13. Electric and hybrid vehicle adoption |
|||
14. Cooling and heating degree days, levels and change, residential built-up volume |
|||
15. Built-up area growth; built-up area per capita |
|||
16. Built-up area per capita |
|||
17. Difference between built-up area growth and population growth |
|||
18. Waste generation |
|||
19. Waste recovery |
|||
Climate adaptation and resilience |
|||
20. Air temperature and change |
|||
21. Number of hot days and change |
|||
22. Number of tropical nights and change |
|||
23. Number of icing days and change |
|||
24. Heat stress exposure |
|||
25. Urban heat island intensity (daytime and night-time) |
|||
26. Population exposure to fires |
|||
27. Burnt area by land cover type |
|||
28. Forest exposure to wildfire danger |
|||
29. Population exposure to river flooding |
|||
30. Population exposure to coastal flooding |
|||
31. Agricultural drought |
|||
32. Cumulated precipitation and change |
|||
33. Extreme precipitation days and change |
|||
34. Built-up exposure to violent storms |
|||
35. Population density |
|||
36. Population aged < 5 |
|||
37. Population aged > 70 |
|||
38. Unemployment rate |
|||
39. Poverty rate |
|||
Actions and opportunities |
|||
40. Existence of subnational climate mitigation and adaptation targets and compliance with national targets (to be estimated) |
National scale |
||
41. Citizens’ satisfaction with efforts to preserve the environment |
|||
42. Green areas in cities |
(***) |
||
43. Climate-significant expenditure and investment |
National scale |
||
44. Existence of climate-related funds, grants and subsidies for subnational action |
National scale |
||
45. Patent applications in climate mitigation technologies as % of total technologies |
Note: (*) FUAs of more than 500 000 inhabitants; (**) metropolitan areas but only the largest metropolitan area in each OECD country; (***) FUA’s urban centre.
Compatibility with existing OECD climate-related indicator frameworks
The OECD territorial climate indicator framework is fully aligned with existing OECD climate-related indicator frameworks. Most importantly, the framework aims to complement the IPAC Dashboard on the subnational dimension. To this end, the indicator framework carefully selects indicators from the IPAC Dashboard that hold relevance at the local and regional scales, thereby offering a subnational perspective within the broader national context. Notably, out of the 29 indicators currently featured on the IPAC Dashboard, the OECD territorial climate indicator framework incorporates 18, with 13 of them already computed at the territorial level. Furthermore, the OECD territorial climate indicator framework integrates 11 climate-related indicators already defined in the OECD localised framework for the SDGs. This encompasses not only indicators under SDG 13 on climate action but also those under other goals such as SDG 1 on no poverty, SDG 7 on affordable and clean energy, SDG 8 on decent work and economic growth, SDG 11 on sustainable cities and communities, and SDG 12 on responsible consumption and production. Table 2.5 provides a breakdown of which indicators are part of the IPAC Dashboard, the OECD localised framework for the SDGs, or are included in both frameworks.
Table 2.5. Alignment of OECD territorial climate indicator framework with other OECD climate‑related indicator frameworks
Indicator |
OECD localised indicator framework for the SDGs (11) |
IPAC Dashboard (18) |
---|---|---|
Climate mitigation |
||
1. Total GHG emissions, level and % change |
||
2. GHG emissions per capita, level and % change |
||
3. GHG emission intensities per unit of GDP, level and % change |
||
4. GHG emissions by sector: share of total emissions, level, per capita and % change |
||
5. Consumption-based GHG emissions (to be estimated) |
||
6. Electricity generation by source |
SDG 7 |
|
7. Carbon intensity of electricity generation |
SDG 13 |
|
8. Energy mix in total energy supply (to be estimated) |
||
9. Total final energy consumption by source (to be estimated) |
||
10. Emission intensity of the manufacturing industry |
||
11. Access to public transport |
||
12. Private vehicle ownership |
||
13. Electric and hybrid vehicle adoption |
||
14. Cooling and heating degree days, levels and change, residential built-up volume |
SDG 13 |
|
15. Built-up area growth |
SDG 11 |
|
16. Built-up area per capita |
SDG 11 |
|
17. Difference between built-up area growth and population growth |
SDG 11 |
|
18. Waste generation |
SDG 12 |
|
19. Waste recovery |
SDG 12 |
|
Climate adaptation and resilience |
||
20. Air temperature change |
||
21. Number of hot days and change |
||
22. Number of tropical nights and change |
||
23. Number of icing days and change |
||
24. Heat stress exposure |
||
25. Urban heat island intensity (daytime and night-time) |
||
26. Population exposure to fires |
||
27. Burnt area by land cover type |
||
28. Forest exposure to wildfire danger |
||
29. Population exposure to river flooding |
||
30. Population exposure to coastal flooding |
||
31. Agricultural drought |
||
32. Cumulated precipitation and precipitation change respective to the 1981-2010 climatology |
||
33. Extreme precipitation days and change respective to the 1981-2010 climatology |
||
34. Built-up exposure to violent storms |
||
35. Population density |
||
36. Population aged < 5 |
||
37. Population aged > 70 |
||
38. Unemployment rate |
SDG 8 |
|
39. Poverty rate |
SDG 1 |
|
Actions and opportunities |
||
40. Existence of subnational climate mitigation and adaptation targets and compliance with national targets (to be estimated) |
||
41. Citizens’ satisfaction with efforts to preserve the environment |
SDG 13 |
|
42. Green areas in cities |
||
43. Subnational government climate finance database: climate-significant expenditure and investments |
||
44. Existence of climate-related funds, grants and subsidies for subnational action |
||
45. Patent applications in climate mitigation technologies as % of total technologies |
Source: Based on OECD (2022[10]), International Programme for Action on Climate, https://www.oecd.org/climate-action/ipac/; OECD (2020[2]), A Territorial Approach to the Sustainable Development Goals: Synthesis Report, https://doi.org/10.1787/e86fa715-en.
Measuring the distance of cities and regions to achieving global and national targets
The indicator framework also allows measuring the distance of cities and regions to reaching specific reference points for each indicator. It thus mirrors the structure of the SDG localised indicator framework, facilitating an understanding of how much progress a region or city needs to make to reach the desired outcome. The OECD territorial climate indicator framework attributes specific reference points 28 out of its 45 indicators (Table 2.6). For climate mitigation indicators as well as actions and opportunities indicators, the reference points correspond to policy targets, shedding light on the progress of cities and regions towards achieving climate neutrality. They are based either on sectoral knowledge or on “best performers”, which correspond to the simple average of the top performer region or city of each OECD country. In the case of climate adaptation and resilience indicators, the reference points refer to normality and provide insights into how distant cities and regions are from “climate normal”, which are based on the 1981-2010 baseline climatology.
Table 2.6. Reference points of the OECD territorial climate indicator framework
Indicator |
Reference point |
---|---|
Climate mitigation |
|
1. Total GHG emissions, level and % change |
The 2030 emissions per capita target (4.7 tCO2‑eq) defined based on computations derived from the IEA NZE Scenario for advanced economies (IEA, 2021[13]) multiplied by 2030 population projection |
2. GHG emissions per capita, level and % change |
The 2030 emissions per capita target (4.7 tCO2‑eq) defined based on computations derived from the IEA NZE Scenario for advanced economies (IEA, 2021[13]) |
4. GHG emissions by sector: share of total emissions, level, per capita and % change |
The 2030 emissions per capita target by sector, defined based on computations derived from the IEA NZE Scenario for advanced economies (IEA, 2021[13]): agriculture 0.1 tCO2-eq/capita; buildings 0.4 tCO2-eq/capita; industry 1.7 tCO2-eq/capita; power 1.3 tCO2-eq/capita; transport 1.3 tCO2-eq/capita |
5. Consumption-based GHG emissions |
The 2030 emissions per capita target (4.7 tCO2-eq) defined based on computations derived from the IEA NZE Scenario for advanced economies (IEA, 2021[13]) |
6. Electricity generation by source |
100% of electricity generated from low-carbon sources |
7. Carbon intensity of electricity generation |
Carbon intensity of electricity generated by the lowest emission-intensive source, i.e. 11 grams of carbon dioxide equivalent per kilowatt-hour (gCO2-eq/kWh) (wind) |
11. Access to public transport |
100% of the population with access to public transport |
12. Private vehicle ownership |
Best performers, i.e. 385 vehicles for 1 000 inhabitants |
13. Electric and hybrid vehicle adoption |
100% of electric or hybrid vehicle adoption |
16. Built-up area per capita |
Best performers, i.e. 65 square metres per capita for metropolitan areas |
18. Waste generation |
Best performers, i.e. 350 kg per capita per year |
19. Waste recovery |
100% of recycled municipal waste |
Climate adaptation and resilience |
|
20. Air temperature change |
Mean air temperature over 1981-2010 |
21. Number of hot days and change |
Mean number of hot days over 1981-2010 |
22. Number of tropical nights |
Mean number of tropical nights over 1981-2010 |
23. Number of icing days |
Mean number of icing days over 1981-2010 |
24. Heat stress exposure |
Mean number of days of different heat stress levels over 1981-2010 |
26. Population exposure to fires |
Reference point defined as 0% |
27. Burnt area by land cover type |
Reference point defined as 0% |
28. Forest exposure to wildfire danger |
Reference point defined as 0% |
31. Agricultural drought |
Mean cropland soil moisture over 1981-2010 |
32. Cumulated precipitation respective to the 1981-2010 climatology |
Mean cumulated precipitation over 1981-2010 |
33. Extreme precipitation days respective to the 1981-2010 climatology |
Mean extreme precipitation days over 1981-2010 |
34. Built-up exposure to violent storms |
Mean exposure over 1981-2010 |
Actions and opportunities |
|
40. Existence of subnational climate mitigation and adaptation targets and compliance with national targets |
100% of subnational governments (e.g. TL2, TL3 levels) with climate mitigation and adaptation targets complying with national targets |
41. Citizens’ satisfaction with efforts to preserve the environment |
Best performers |
42. Green areas in cities |
Best performers |
43. Climate-significant expenditure and investment |
Best performers |
45. Patent applications in climate mitigation technologies as % of total technologies |
Best performers |
Note: Reference points are yet to be defined for the following indicators: GHG emission intensities per unit of GDP, level and % change (Indicator 3), energy mix in total energy supply (Indicator 8), total final energy consumption by source (Indicator 9), emission intensity of the manufacturing industry (Indicator 10), built-up area growth (Indicator 15), difference between built-up area growth and population growth (Indicator 17), urban heat island intensity (daytime and night-time) (Indicator 25), population exposure to river flooding (Indicator 29), population exposure to coastal flooding (Indicator 30), existence of subnational mitigation and adaptation targets and compliance with national targets (Indicator 40) and existence of climate-related funds, grants and subsidies for subnational action (Indicator 44). Reference points are not applicable for the following indicators: cooling and heating degree days, levels and change, residential built-up volume (Indicator 14), population density (Indicator 35), population aged < 5 (Indicator 36), population aged > 70 (Indicator 37), unemployment rate (Indicator 38) and poverty rate (Indicator 39).
Indicators in detail: Relevance, data sources and reference points
This section provides a detailed discussion of the indicators under the OECD territorial climate indicator framework. Each subsequent sub-section further explains the relevance of measuring a particular indicator at the subnational level. It also explains the data sources used to estimate the indicator and the suggested reference points. The details of each indicator can also be found in Annex Table 2.A.1.
Climate mitigation indicators
GHG emissions (Indicators 1 to 5)
Global GHG emissions continue to rise and contribute to climate change. Average annual GHG emissions during the 2010-19 period were higher than in any previous decade, although the growth rate between 2010 and 2019 was lower than between 2000 and 2009 (Shukla et al., 2022[14]). Even though global CO2 emissions dropped by 5.8% in 2020 with the coronavirus disease 2019 (COVID-19) pandemic, they rebounded by 5% in 2021, approaching the peak in 2018-19 (IEA, 2022[15]). Reducing emissions implies co-ordinating mitigation policies at different territorial levels and this calls for a better understanding of the breakdown of emissions by sector at the local level.
In the IEA NZE Scenario (IEA, 2021[13]), CO2 emissions from energy-related and industrial processes in advanced economies, i.e. in OECD and EU 27 countries, drop from 12.3 gigatonne (Gt) of CO2-eq to around 5.5 GtCO2‑eq in 2030, which corresponds to a drop from 8.8 tCO2-eq per person in 2019 to 3.8 tCO2-eq per person in 2030. In this scenario, advanced economies reduce their emissions faster than emerging markets and developing economies. The share of CO2 emitted by global energy-related and industrial processes from advanced economies falls from 34% to 26%. Given that CO2 from fossil fuel and industry, methane (CH4) and nitrous oxide (N2O) account for respectively 12.8 GtCO2-eq, 2.2 GtCO2-eq and 0.8 GtCO2-eq of net anthropogenic GHG emissions in advanced economies (Crippa et al., 2021[16]), if we assume the same distribution of GHG in 2030, GHG emissions per capita, as defined in the framework, would have to drop to 4.7 tCO2-eq per person in 2030. Consequently, the indicator framework sets a reference point of 4.7 tCO2-eq per person for per capita production-based GHG emissions.
The IEA NZE Scenario also provides global CO2 emission profiles by sector until 2050, allowing the definition of a GHG emissions target by sector for 2030. Figure 2.3 shows total fossil fuel and industry CO2 emission reduction targets for 2030 in advanced economies. In the NZE Scenario, the power sector witnesses the largest reduction in emissions, with a 68% decrease in emissions from 2019 to 2030 for advanced economies, mainly due to significant reductions from coal-fired power plants. Emissions from buildings are cut by 54% from 2019 to 2030 by retrofitting and phasing out fossil fuel boilers. Emissions from transport, industry and agriculture fell by 48%, 45% and 49% respectively over this period. In terms of CO2 emissions per capita, this corresponds to 1.3 tCO2-eq for the power sector, 0.4 tCO2-eq for buildings, 1.7 tCO2-eq for industry, 1.3 tCO2-eq for transport and 0.1 tCO2-eq for agriculture. To estimate the progress of OECD cities and regions towards achieving net zero, these per capita targets are applied to sectoral GHG emissions at the different territorial levels. It is worth noting that waste is excluded, as the IEA NZE Scenario does not include emission scenarios for this sector. It is also worth noting that, in the current indicator framework, these targets are used as a reference point and do not correspond to actual policy targets for cities and regions, as they do not account for regional variations in population density, economic sectors and technologies or regional typology. For instance, in a country achieving the 2030 IEA NZE emission targets in terms of absolute GHG emissions, a remote region may still exhibit higher per capita emissions in agriculture and transport than a metropolitan region. For simplicity, the framework assumes a uniform convergence and applies the same sector per capita targets to all territorial scales.
Figure 2.3. Sectoral emission reduction targets for 2030
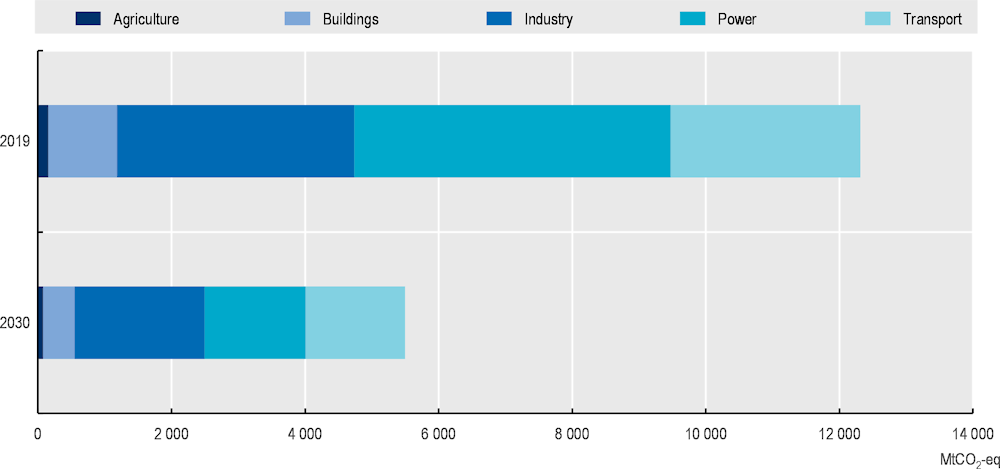
Note: OECD computations based on the IEA NZE Scenario (IEA, 2021[13]) combining total fossil fuel and industry CO2 emissions projections in advanced economies and global CO2 emissions projections by sector.
Although all OECD countries report annual production-based GHG emissions by sector at the national level, subnational inventories are either lacking or not consistent across and within countries. The Emissions Database for Global Atmospheric Research (EDGAR) (Crippa et al., 2021[16]) developed by the European Commission Joint Research Centre (JRC) attempts to fill this data gap. It is an independent, global database of anthropogenic GHG and air pollutant emissions and provides 0.1-degree resolution (approximately 11 kilometres, km) gridded maps at the global level, with yearly and monthly data from 1970 to 2018. These grids are obtained by disaggregating national inventories at the local level, using spatial proxies such as road networks, population density, built-up areas, power plants, cement plants, etc. Such gridded maps can then be aggregated at different territorial levels. However, the low resolution of the grids can lead to a misallocation of emissions when aggregating values for granular territorial units compared to the size of grid cells. Estimates for larger regions (TL2) are thus more accurate than for smaller units such as small regions (TL3) or FUAs. This data source covers the three most potent gases (CO2, CH4 and N2O). Fluorinated gases will be included in the future. The different sectors and subsectors covered are:
Agriculture: Enteric fermentation, manure management, agricultural waste burning, agricultural soils, indirect N2O emissions from agriculture.
Power industry: Electricity generation.
Industry: Combustion for manufacturing, chemical processes, iron and steel production, non‑ferrous metals production, non-energy use of fuels, solvent and product use, non-metallic minerals production, oil refineries and transformation industry, fuel exploitation (oil, coal, natural gas).
Buildings: Energy for buildings.
Waste: Wastewater handling, solid waste landfills, solid waste incineration.
Transport: Road transport, aviation, shipping, railways, pipelines, off-road transport.
Other: Fossil fuel fires, indirect emissions from nitrogen oxides (NOx) and ammonia (NH3).
Emissions from land use and land cover change (LULCC) are not included for the moment.
The proposed framework of indicators uses EDGAR for the following indicators:
Total GHG emissions, level and percentage change.
GHG emissions per capita, level and percentage change.
GHG emissions per unit of GDP: level and percentage change.
GHG emissions by sector.
Emission intensity of the manufacturing industry.
The proposed framework focuses on production-based territorial emissions, as subnational consumption-based inventories are unavailable for most countries. Consumption-based emissions account for emissions embedded in trade and ensure that cities and regions are not simply outsourcing their emissions outside their territorial boundaries to meet climate neutrality targets.
Energy (Indicators 6 to 9)
In 2019, approximately 34% (20 GtCO2-eq) of total net anthropogenic GHG emissions came from the energy supply sector (Shukla et al., 2022[14]). It is consequently a key sector to focus on to reduce GHG emissions.
Although national energy statistics are very detailed, subnational energy data are often lacking. Tracking progress towards achieving net zero targets at the local level would require looking at both energy consumption and supply. Final energy consumption by sector (residential, transport, agriculture, industry, commercial and public services) and fuel type (electricity, natural gas, coal, oil and petroleum products, biofuels and waste) would enable tracking changes in consumption, electrification and phasing out from fossil fuels in each sector to better accompany local areas and sectors not meeting the net zero targets. However, international databases on subnational final energy consumption data are lacking. Only a few countries, such as France and the United Kingdom, provide such data.
On the energy supply side, the indicator framework proposed in this paper includes power generation by source and the carbon intensity of electricity generation at the TL2 and TL3 levels. This enables tracking the shift towards low-carbon electricity. Subnational-level data on electricity production are also scarce but these indicators can be estimated from national statistics and the geolocation of power plants together with their technical characteristics. The proposed indicator framework uses the Global Power Plant Database (GPPD) (GEO, 2021[17]), the IEA electricity and heat database, and the harmonised global dataset of wind and solar farms (GWS) (Dunnett, Sorichetta and Taylor, 2020[18]) locations and power capacity.
The GPPD (GEO, 2021[17]) provides information on power plants located in 167 countries all over the world, including the 38 OECD countries. For each power plant, the GPPD provides the geographic co‑ordinates, the energy source (e.g. nuclear, gas, wind, etc.) and the capacity. As the coverage of wind and solar power plants in the GPPD is not exhaustive, the proposed indicator framework used the harmonised GWS (Dunnett, Sorichetta and Taylor, 2020[18]) farm locations and power instead to get the locations of wind and solar power sources. Electricity production at the power plant level was disaggregated from national-level electricity generation data by energy sources (IEA, 2022[19]), based on the capacity of the power plant relative to the total installed capacity for the same energy source. Regional indicators correspond to the aggregation of plant-level electricity generation data.
To assess the progress of OECD cities and regions towards achieving clean electricity generation, the proposed indicator framework sets a target of 100% of low-carbon electricity and carbon intensity of electricity generation corresponding to the lowest emission-intensive source, i.e. wind with a carbon intensity of 11 gCO2/kWh (IPCC, 2014[20]).
Industry (Indicators 4 and 10)
In 2019, approximately 24% (14 GtCO2-eq) of global net anthropogenic GHG emissions came from industry. Since 2000, industry emissions have been growing faster than emissions in any other sector, driven by increased basic materials extraction and production (Shukla et al., 2022[14]).
In OECD countries, the manufacturing sector is the third largest contributor to territorial emissions after the energy and transport sectors, accounting for 20% of total emissions (including oil refineries and the transformation industry). Within the manufacturing industry, emissions are particularly concentrated in four subsectors: the manufacture of coke and petroleum products, chemicals and chemical products, non‑metallic mineral products (i.e. cement) and of basic metals (i.e. steel). In EU27 countries, these 4 sectors account for 80% of total manufacturing GHG emissions. In the IEA NZE Scenario (IEA, 2021[13]), CO2 emissions in the industry sector in advanced economies are cut by 45% from 2019 to 2030.
The proposed indicator framework includes emissions per unit of gross value added (GVA) in TL2 regions to assess how emission-intensive the manufacturing sector is. To assess the distance of cities and regions to achieving 2030 targets, the indicator framework sets a target of a 45% reduction in industry emissions, which corresponds to 1.7 tCO2-eq per capita.
Buildings (Indicators 4 and 14)
In 2021, buildings accounted for 33% of global energy-related and process-related CO2 emissions (8% from the use of fossil fuels in buildings, 19% from the generation of electricity and heat used in buildings and 6% from the manufacture of materials used in buildings construction) (IEA, 2022[21]). In the IEA NZE Scenario (IEA, 2021[13]), CO2 emissions from buildings are cut by 54% from 2019 to 2030 in advanced economies.
The indicator framework proposed in this publication includes direct building emissions per capita based on EDGAR (Crippa et al., 2021[16]) at the TL2 and TL3 levels. This dataset only covers direct building emissions related to onsite combustion. Consequently, the carbon intensity of purchased electricity and heat used in buildings and the manufacturing of construction materials are not included. To assess the distance of cities and regions to 2030 targets, the indicator framework sets a target of a 54% reduction in building emissions, which corresponds to 0.4 tCO2-eq per capita.
Space heating is the main source of residential energy consumption. Across OECD countries, it accounts for 58% of total energy use (IEA, 2022[22]). Energy consumption for space cooling accounted for 16% of buildings’ final electricity consumption in 2021 and has more than trebled since 1990 (IEA, 2022[23]). To reflect building energy consumption drivers, the framework of indicators includes both cooling and heating degree days (level and change compared to the reference period 1981-2010) as well as residential built-up volume per capita.
Transport (Indicators 11 to 13)
In 2019, approximately 15% (8.7 GtCO2-eq) of global net anthropogenic GHG emissions came from transport (Shukla et al., 2022[14]). Unlike the energy supply and industry sectors, where average annual GHG emissions growth between 2010 and 2019 slowed down compared to the previous decade, those in the transport sector continued to rise by about 2% per year (Shukla et al., 2022[14]). In 2018, 45% of the transport CO2 emissions came from passenger road vehicles, 29% from road freight vehicles, 12% from aviation, 11% from shipping and 1% from rail (IEA, 2022[24]). Since 2010, sport utility vehicles (SUVs) have been the second-largest contributor to the increase in global CO2 emissions after the power sector but ahead of heavy industry, trucks and aviation (IEA, 2019[25]). In the IEA NZE Scenario (IEA, 2021[13]), CO2 emissions in the transport sector in advanced economies are cut by 48% from 2019 to 2030, which corresponds to 1.3 tCO2-eq/capita. Reaching climate neutrality and cutting oil use in transport requires making public transport more accessible, reducing private car use in large cities and accelerating the adoption of electric and more efficient vehicles (IEA, 2022[26]). Electric vehicles powered by low-emissions electricity offer the largest decarbonisation potential for land-based transport on a life cycle basis (Shukla et al., 2022[14]).
The proposed indicator framework consequently looks at the number of private vehicles per capita and the share of electric and hybrid vehicles at the TL2 level provided in the OECD Regional Database. It also includes public transport accessibility in the largest OECD cities by looking at the share of the population in cities within a ten-minute walk of public transport (bus, metro and tram). Public transport stop locations are extracted from open geographic database OpenStreetMap and walking times from each transport stop were calculated using Mapbox API. To assess the progress of OECD cities and regions towards achieving clean transport systems, the proposed indicator framework sets a target of 100% for the share of electric or hybrid vehicles and for public transport accessibility. As for the number of private motor vehicles per capita, the target corresponds to the “best performers”, i.e. 385 vehicles for 1 000 inhabitants.
Other relevant indicators that are not covered by the proposed indicator framework but that would also enable tracking progress in the transport sector include the average distances travelled per person per year, the modal share associated with each transport type (public transport, private car, walking, biking, etc.) and access to charging stations.
Agriculture, forestry and other land use (AFOLU) (Indicators 15 to 17)
In 2019, approximately 22% (13 GtCO2-eq) of global net anthropogenic GHG emissions came from AFOLU. AFOLU emissions originate mostly from deforestation and agricultural emissions from livestock, soil and nutrient management (Shukla et al., 2022[14]). The mitigation potential in the AFOLU sector is derived both from a reduction in emissions through better management of land and livestock, as well as from enhanced removal of GHG through carbon sinks. Consequently, the AFOLU sector offers significant near-term mitigation potential at a relatively low cost and can provide 20-30% of the 2050 emissions reduction described in scenarios that likely limit warming to 2°C or lower. The largest share of mitigation potential in the sector corresponds to measures in forests and natural ecosystems, followed by agriculture and demand-side measures (Shukla et al., 2022[14]).
Several indicators enable tracking climate action and resilience in the AFOLU sector. Natural carbon sinks, i.e. forests, peatlands, coastal wetlands, savannas and grasslands, can be monitored and their areas can be quantified at different territorial levels using land use and land cover (LULC) maps. These maps include the NASA MCD12Q1 MODIS yearly global land cover data at 500-metre (m) resolution (2001-20) (Friedl and Sulla-Menashe, 2019[27]) or the ESA Climate Change Initiative (CCI) yearly dataset at 300-m resolution (1992‑2020) (ESA, 2019[28]). More recent initiatives exploit Sentinel satellites, which provide multispectral (Sentinel-2) and RADAR (Sentinel-1) images at a much higher resolution (10 m) and a frequent time revisit (every 5 to 6 days), as well as automated image processing pipelines based on deep learning models to produce near real-time LULC estimates. The ESA WorldCover map (Zanaga et al., 2021[29]) provides yearly land cover mapping for 2020 at a 10-m resolution. Similarly, the Dynamic World dataset (Brown et al., 2022[30]) developed jointly by Google and the World Resources Institute provides near real-time LULC data at a 10-m resolution with a 5-day revisit (June 2015 to present). Using the ESA CCI land cover dataset, the proposed indicator framework includes tree cover changes in TL2 regions.
Urban sprawl and soil artificialisation contribute to the loss of natural carbon sinks. Comparing the growth and levels in built-up areas and in population enables to assess the anthropogenic pressure on lands, as highlighted in SDG target 11.3.1. The proposed indicator framework uses the Global Human Settlement population count (GHS-POP) (Schiavina, Freire and MacManus, 2022[31]) and built-up surface (GHS‑BUILT-S) layers (Pesaresi and Politis, 2022[32]). The framework sets a target for built-up area per capita based on the “best performers”, which corresponds to 65 square metres per capita for metropolitan areas.
Agriculture is also an important driver of GHG emissions, accounting for 9% of total production-based emissions in 2018 in OECD countries. Emissions in this sector are mainly due to the CH4 (65%) emitted by animals and rice cultivation and N2O (33%) released from pastures and crops using nitrogen fertilisers. These 2 gases have a much higher global warming potential than CO2 (28 for CH4 and 265 for N2O over 100 years). The proposed indicator framework includes agriculture emissions based on EDGAR (Crippa et al., 2021[16]) at the TL2 and TL3 levels.
Waste (Indicators 18 to 19)
Lowering material consumption, reducing waste and recycling more also play an important role in the net zero transition. In this field, the proposed indicator framework includes two indicators at the TL2 level: the mass of municipal waste per inhabitant and the share of municipal waste treated with recovery operations (recycling, composting and incineration with energy recovery). The proposed indicator framework sets a target of 100% for the share of recovered municipal waste. As for the mass of municipal waste per capita, the framework sets a target corresponding to the “best performers”, i.e. 350 kilograms (kg) per capita per year.
Climate adaptation and resilience indicators
This section provides a summary of the state indicators and data sources used in the localised framework of indicators. Due to data availability, this framework of indicators mostly focuses on climate risks and does not include indicators of economic impact, casualties and displaced populations resulting from climate change. Following the IPCC risk assessment framework (Figure 2.4), climate-related risks are based on three pillars:
Hazard is the potential occurrence of climate-related physical events or trends that may cause loss and damage.
Exposure is the presence of people, settlements, infrastructure or ecosystems in areas where climate hazard events could occur.
Vulnerability is the propensity to suffer adverse effects when impacted by climate hazard events. It includes a wide range of concepts such as weaknesses, sensitivities and incapacity to adapt.
Figure 2.4. The IPCC risk assessment framework
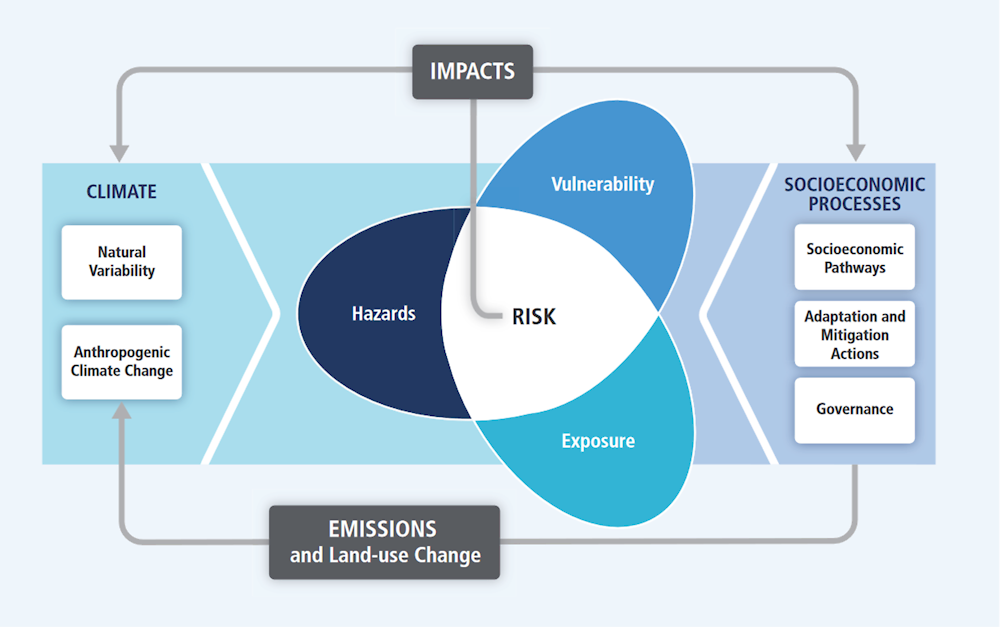
Source: IPCC (2014[33]), Climate Change 2014: Impacts, Adaptation, and Vulnerability. Part A: Global and Sectoral Aspects, https://www.ipcc.ch/site/assets/uploads/2018/02/WGIIAR5-PartA_FINAL.pdf.
Different temporal perspectives can also be considered to assess risks by looking at either historical data or projections by shared socio-economic pathways or representative concentration pathways.
The proposed indicator framework mostly looks at climate risk exposure and hazard dimensions. These indicators are consistent with the IPAC framework of indicators and follow the methodology described in (Maes et al., 2022[34]). This framework covers a wide range of indicators enabling the assessment of the cities and regions most exposed to certain climate hazards, which can be used as a basis to define policy priorities to adapt such places to the consequences of climate change.
Temperature trends, heat and cold (Indicators 20 to 25)
Climate change has led to an overall increase in temperatures but also to longer, more frequent and intense heat-related events worldwide. These periods of exceptional heat combined with other atmospheric conditions such as humidity and wind can have strong impacts on human health. It is estimated that 365 000 people died around the world in 2019 because of extreme heat (The Lancet, 2021[35]). The indicator framework includes six indicators related to temperature trends, heat and cold:
air temperature
number of hot days
number of tropical nights
number of icing days
population exposure to heat stress
urban heat island intensity.
Temperature trends are measured based on the annual mean of 2 m air temperature from ERA5-Land Monthly Averaged Data (Muñoz Sabater, 2019[36]). Hot days correspond to days during which the daily maximum temperature exceeds 35°C, tropical nights correspond to days during which the daily minimum temperature exceeds 20°C and icing days correspond to days during which the daily maximum temperature does not exceed 0°C. These indicators are also based on ERA5-Land (Muñoz Sabater, 2019[36]).
Population exposure to heat stress is measured by using the universal thermal climate index (UTCI), which considers air temperature, wind, solar radiation and humidity. Different levels of heat stress are defined: strong heat stress corresponds to UTCI values between 32°C and 38°C, very strong heat stress ranges between 38°C and 46°C, and extreme heat stress corresponds to UTCI values above 46°C. Population exposure to heat stress is measured by looking at the population-weighted average number of days per year with UTCI values corresponding to strong, very strong or extreme heat stress. To assess the distance of cities and regions to their respective climate normals, the indicator framework sets 1981-2010 averages as reference points.
In cities, the urban heat island effect can amplify the intensity of heat waves. Densely populated urban areas tend to be warmer than their lower-density surrounding areas due to reduced ventilation, the proximity of tall buildings, heat generated from human activities, the properties of urban building materials and the limited amount of vegetation (Masson-Delmotte et al., 2021[37]). The centres of Paris, France, and London, United Kingdom, for example, regularly record temperatures of around 4°C higher than rural surroundings at night. In Athens, Greece, this difference can reach 10°C during the summer. In Sydney, Australia, surface temperatures of the built environment can grow up to 16°C warmer in the sun than in shaded areas (Lombardi, Rodas and Ledesma, 2022[38]). The proposed indicator framework quantifies the intensity of the urban heat island effect in OECD FUAs following the methodology described in Chakraborty and Lee (2019[39]), which is based on MODIS Aqua and Terra (Wan, Hook and Hulley, 2021[40]) land surface temperature data as well as MODIS Land Cover (Friedl and Sulla-Menashe, 2019[27]) data. The urban heat island intensity corresponds to the average difference in the land surface temperature between urban lands and non-urban lands2 within FUAs. Averages are computed for summer days, from 1 June to 31 August for the Northern Hemisphere and from 1 December to 28 February for the Southern Hemisphere, at daytime, for 10.30 am and 1.30 pm.
Wildfires (Indicators 26 to 28)
Increasing heat stress combined with droughts has made vegetated land more vulnerable to wildfires. Wildfires can lead to large losses of forests and agricultural lands but also represent a security risk for settlements and populations. The proposed indicator framework defines exposure to fires in OECD regions in terms of forest and cropland area burnt and in terms of population at risk. Burnt area perimeters are extracted from the JRC’s global wildfire dataset (Artes Vivancos et al., 2019[41]). Burnt forest and cropland areas are derived by overlapping burnt areas with the Copernicus CCI land cover3 dataset (ESA, 2019[28]). The exposed population is defined as those living within 5 km of a burnt area and is derived from the Global Human Settlement (GHS) population grid for 2015 (Schiavina, Freire and MacManus, 2019[42]).
To also assess the likelihood of a wildfire starting, the indicator framework includes the share of forest exposed to at least three consecutive days of very high or extreme fire danger. Fire danger is defined using the Fire Weather Index (FWI) (EC, 2019[43]), which is a meteorologically based index developed in Canada and used globally to estimate fire danger. This index accounts for fuel moisture and the influence of wind on fire behaviour and spread. An FWI greater than five corresponds to a very high fire danger and one greater than six to an extreme fire danger.
River and coastal flooding (Indicators 29 to 30)
Changes in water cycles, combined with land artificialisation, can lead to more frequent and intense river flooding. River floodings are usually characterised by their return period, which corresponds to the average time between two flooding events of the same magnitude. The proposed indicator framework uses the River Flood Hazard Maps dataset to assess river flooding exposure in OECD regions and FUAs (Dottori et al., 2021[44]). The dataset provides the extent of flooded areas for events of different return periods, ranging from 10 to 500 years. River flooding risk was assessed in OECD cities and regions using the 100‑year return period. The extent of such floods is estimated from past events but 100-year return floods are likely to happen more than once per century in the future. Flood risk is increasing the most rapidly in Asia, Europe and North America, but without higher flood protection standards, flood events are projected to rise in all continents (Alfieri et al., 2017[45]). Flooded area extent maps were combined with the GHS population grid for 2015 (Schiavina, Freire and MacManus, 2019[42]) and Copernicus CCI land cover data (ESA, 2019[28]) to assess population and built-up area exposure.
Coastal regions are particularly exposed to climate change as they face rising sea levels, more frequent coastal storm surges and soil erosion. By 2050, more than 500 coastal cities will face a sea level rise of at least 0.5 m, putting over 800 million people at risk (C40 Cities, 2018[46]). The proposed indicator framework assesses coastal flood exposure using the World Bank Global Coastal Flood Hazard maps (Muis et al., 2016[47]). The maps present a global reanalysis of storm surges and extreme sea level events based on hydrodynamic modelling for different frequencies of occurrence. Population exposure to coastal flooding is measured by using the GHS population grid for 2015 (Schiavina, Freire and MacManus, 2019[42]) and the built-up area exposure using Copernicus CCI land cover data (ESA, 2019[28]).
Droughts (Indicator 31)
Changes in precipitations and other atmospheric conditions can lead to longer, more frequent and severe droughts, increasing pressure on agriculture, infrastructures and ecosystems. The agricultural sector can be particularly affected by droughts, as water scarcity has serious impacts on crop yields. To measure agricultural droughts in OECD regions, the proposed indicator framework includes the change over time in the water content of the superficial layer of croplands’ soil, which is important for water supply and vegetation health (Copernicus Global Land Service, n.d.[48]). The Copernicus CDS ERA5-Land monthly average data product (EC, 2022[49]) provides volumetric surface soil moisture, which corresponds to the volume of water in the surface soil layer of 0 to 7 centimetres deep, expressed as cubic metres (m3) of water per m3 soil. The Copernicus CCI land cover data (ESA, 2019[28]) is used to select only cropland4 areas. For each OECD region, changes in drought conditions are measured by comparing the surface soil moisture in cropland areas to the reference period 1981-2010.
Precipitation (Indicators 32 to 33)
Climate change is impacting precipitation patterns and distributions, disturbing the water cycle and influencing ecosystems, communities and economic activities. While some regions experience more frequent or extreme rainfall, others are facing prolonged droughts and reduced water availability, which can impact food security and heighten the risks of flooding, landslides and erosion. In cities, extreme precipitation can also lead to a higher risk of flash floods that overwhelm drainage systems and streets, posing significant challenges to infrastructures and people’s safety. The proposed indicator framework includes several precipitation indicators based on ERA5-Land data (Muñoz Sabater, 2019[36]). First, the total yearly precipitation (in millimetres, mm) and change with respect to the 1981-2010 climatology allow for the assessment of the impact of climate change on precipitation patterns. Then, the number of days with more than 20 mm of total precipitation and the change compared to 1981-2010 allows for measuring extreme precipitation days and the impact of climate change on their frequency.
Socio-economic indicators driving vulnerability (Indicators 35 to 39)
Certain population groups are particularly vulnerable to the impacts of climate change, as they may face increased health risks from heat stress, have less mobility during climate-related events or have fewer resources to adapt. The indicator framework consequently includes different socio-economic and demographic indicators on population density, the share of population under 5 years old and above 70 years old, as well as poverty and unemployment rates.
Actions and opportunities indicators
Existence of subnational climate mitigation and adaptation targets and compliance with national targets (Indicator 40)
Subnational climate mitigation and adaptation targets are among the key instruments used to implement a territorial approach. For subnational governments, such targets can clearly demonstrate their climate commitments and priorities to their constituencies, guide the allocation of resources accordingly and help monitor the progress of their climate action. Subnational climate targets can also inform national governments and help them define their own targets. The existence of GHG emission inventories, reduction targets and targets related to climate adaptation at the TL2 and TL3 levels would enable to assess if these targets are compliant with NDCs, long-term low emissions development strategies (LT‑LEDS), national adaptation plans (NAPs) and national adaptation strategies (NAS).
The framework sets a target of 100% for this indicator, i.e. all the subnational governments (e.g. TL2 and TL3 levels) have climate mitigation and adaptation targets complying with national targets. However, it has not been estimated yet and will be investigated in future work, as estimating the indicator will require full understanding of the currently available national and subnational mitigation and adaptation targets. For instance, careful consideration is required to define how to measure the compliance between national targets (e.g. NDCs, LT-LEDS, NAPs, NAS) and subnational targets, as national targets vary from one country to another and can take different forms. This is particularly the case for climate adaptation, as defining adaptation targets is a complex task in itself.
Citizens’ satisfaction with efforts to preserve the environment (Indicator 41)
Environmental conditions can influence how people feel and how they evaluate their lives and even affect their happiness. Notably, 74% of respondents of the 2019 Gallup World Poll perceive global warming as a very serious or somewhat serious threat to them and their families, and 65% believe that climate change will make their lives harder (Krekel and George, 2020[50]). The proposed indicator estimates the percentage of the population satisfied with efforts to preserve the environment by using data from the Gallup World Poll (2019[51]). The framework sets a target corresponding to the “best performers” for this indicator.
Green areas in cities (Indicator 42)
Adaptation policies to mitigate the impact of heat waves in cities include expanding vegetated areas to increase the number and extent of cooler areas. The proposed indicator framework includes two indicators on green areas in cities, one on the presence of green space and the other on accessibility.
The first indicator corresponds to the share of green areas in urban centres. It estimates the number of green areas (both private and public) in FUAs’ urban centres by using the high-resolution ESA WorldCover dataset (Zanaga et al., 2021[29]). The 10-m resolution dataset captures different kinds of green areas (trees, shrublands and grasslands) of different sizes, going from large parks to trees along main roads. To ensure comparability across OECD cities, only FUAs’ urban centres, as defined in the GHS degree of urbanisation methodology (EU et al., 2021[52]), were considered.
However, a large share of green areas in a city does not imply equal access to public green spaces across the city. The second indicator consequently corresponds to access to green areas in cities using a methodology adapted from Poelman (2018[53]). It is defined for FUAs’ urban centres as the share of the population with access to public green areas of at least 1 hectare (ha) within 400 m distance. Public green areas are delineated using OpenStreetMap (OpenStreetMap, 2023[54]). Urban green areas correspond to the following tags in OpenStreetMap: amenity: grave_yard; land use: cemetery, forest, recreation_ground, village_green; leisure: nature reserve, park, playground, recreation_ground, garden; tourism: zoo; natural: wood, scrub, heath, grassland, wetland. The reference population grid used to get the share of the population within a 400 m distance is GHS-POP 2020 (Schiavina et al., 2023[55]). The framework sets a target corresponding to the “best performers” for both the share of green areas and the access to public green spaces.
Climate-significant expenditure and investment (Indicator 43)
In many countries, especially federal countries, subnational governments can have extensive responsibilities in environmental protection, climate mitigation and adaptation. However, despite the importance of subnational expenditure and investment to drive the net zero transition, their magnitude remains relatively unknown and difficult to assess due to a lack of granular data at the national and subnational levels related to important methodological challenges. For several years now, the OECD has been leading initiatives to better assess subnational government finance in general and, more particularly, subnational climate-related financial indicators.
The OECD provides data on subnational government finance at several scales. First, the OECD database on subnational government structure and finance provides data for OECD and EU countries (updated annually). Then, the OECD-UCLG World Observatory on Subnational Government Finance and Investment (SNG-WOFI) provides subnational government finance data at a larger scale, covering 135 countries worldwide in the latest 2022 edition (the data are updated every three years) (OECD/UCLG, n.d.[56]). Finally, the forthcoming OECD Regional Government Finance and Investment Database (REGOFI) and OECD Municipal Finance Database (MUNIFI), to be launched in 2024, will provide data on the finance of regional governments and municipal governments in an aggregated and disaggregated manner (for individual regions and municipalities) for OECD and EU countries with available data.
These three databases use the same methodology to collect finance data based on the international classification of national accounts. Among other financial indicators, they provide data on the classification of expenditure and investment by functions (Classification of the Functions of Government, COFOG Level 1). COFOG classifies expenditure and investment into ten categories, including “environmental protection”, which covers waste management, wastewater management, pollution abatement and protection of biodiversity and landscape, and scientific research and development on environmental issues, which both relate to climate mitigation and adaptation (OECD, 2022[57]). However, this category does not record the entire set of environmental expenditures undertaken by governments, as climate and the environment have been increasingly acknowledged as a cross-cutting theme, and many other government expenditures have environmental protection as a secondary purpose (e.g. in transport, agriculture, housing, etc.).
In order to overcome this gap and develop a common terminology and understanding of subnational climate finance, the OECD developed a pilot methodology in 2022 to track subnational government climate expenditure and investment in the framework of a project in collaboration with the European Commission (EC) Directorate-General for Regional and Urban Policy (DG REGIO) called Financing Climate Action in Regions and Cities (OECD, n.d.[58]). This work resulted in the OECD Subnational Government Climate Finance Database, which provides comparable data on subnational public climate-significant expenditure and investment, which are defined as follows (OECD, 2022[57]; 2022[59]):
Climate-significant expenditure covers both current and capital expenditure. Current expenditure consists of staff expenditures, intermediate consumption, non-capital subsidies and tax expenditure. Interest expenditures are not included. Capital expenditure refers to indirect investment (capital transfers and capital subsidies) and direct investment (gross fixed capital formation [GFCF] minus disposals of non-financial, non-produced assets).
Climate-significant investment refers to a subset of capital expenditure, specifically direct investment (GFCF) minus disposals of non-financial, non-produced assets. Measuring investment provides a way to focus on the amounts invested in climate-related infrastructure specifically. Using this subset also provides a more accurate estimate of climate-related infrastructure investment spending than the overall spending category could provide.
The methodology relies on a more refined approach to the COFOG classification. Using the EU taxonomy for sustainability activities,5 3 first-level COFOG functions (economic affairs, environmental protection, and housing and community amenities) and 13 second-level COFOG functions (e.g. transport and energy, environmental protection, waste, water management, housing development) were identified as being “climate-significant”, meaning that expenditure in these areas contributes, to some extent, to climate adaptation or mitigation objectives. Then, in order to identify the share of expenditure and investment in each second-level COFOG function that could be considered climate-significant, proxy coefficients derived from internationally comparable datasets were then applied to each function, for all the OECD and EU countries included in the database. The framework sets a target corresponding to the “best performers” for this indicator. Data are presented in Chapter 4.
The existence of climate-related funds, grants and subsidies for subnational action (Indicator 44)
Supporting subnational government climate action requires a better understanding of existing financial programmes and instruments available to them. The OECD Compendium of Financial Instruments that Support Subnational Climate Action in OECD and EU Countries was developed in 2022 in the framework of a project in collaboration with the EC DG REGIO on Financing Climate Action in Regions and Cities (OECD, n.d.[58]). It provides qualitative information on public sources of revenue available to subnational governments in OECD and EU countries to fund and finance their climate action.
The compendium lists a total of 311 public sources of funding that subnational governments can mobilise to fund climate-related activities. It includes instruments provided by higher-level governments: the European Union, central governments, federal and state governments (in federal countries), regional governments (in some specific cases for unitary countries) and government-owned banks. Instruments range from grants to loans, loan guarantees, contractual agreements, funds and more.
Climate-related innovation (Indicator 45)
To assess climate-related innovation, the proposed localised framework includes patent applications in climate change mitigation and adaptation technologies as a percentage of total technologies. These are essential in the deployment of low-carbon technologies and the transition to net zero. The European Patent Office Worldwide Patent Statistical Database (PATSTAT) database (OECD, 2023[60]), which contains worldwide bibliographical and legal event patent data, is used to estimate this indicator. To assess the distance of regions to climate-related innovation, the indicator framework sets a target corresponding to the “best performers”.
Conclusions
Comparable and robust indicator frameworks can help both national and subnational governments unveil territorial disparities and distances to global and national targets on climate and help policy makers make informed decisions that effectively address the specific challenges and opportunities present in different cities and regions. This chapter has presented the OECD territorial climate indicator framework, which offers national, regional and local policy makers a tool to better understand where cities and regions stand with regard to global and national climate targets, as well as against their peers. It has also demonstrated that the proposed indicator framework can help policy makers structure and adjust their policies to the complexity and multi-faceted local climate-specific challenges and opportunities.
The indicator framework represents a key tool to advance multi-level governance and improve policy co‑ordination for climate action and resilience, as it offers a common language for multi-level action by using comparable and standardised indicators. The framework can serve as a powerful tool to promote both vertical (e.g. across levels of government) and horizontal collaboration (e.g. across sectors or policy areas) in the design and implementation of climate-related policy reforms. It offers the possibility for more comprehensive assessment and policy responses since it combines data at different scales, ranging from those related to administrative boundaries (the unit for political and administrative action) to those related to functional approaches (the economic geography of where people live and work), which can provide the evidence base to enhance inter-municipal and rural-urban co‑operation. The use of the indicator framework can benefit both national and subnational governments:
National governments can use the indicator framework to align policy priorities, incentives and objectives across national, regional and local governments, as well as rethink their climate action and resilience agenda through a bottom-up approach. It can also help national governments identify local aspects of climate mitigation and resilience that are not yet targeted by public policies. The use of the indicator framework can also help identify areas in need of support to target and allocate the resources for the implementation of national policies more fairly and efficiently, which is particularly important in times when public finances are constrained due to concomitant crises. Such resources are needed for infrastructure investments and for capacity development. For example, a city may need more support in terms of flood prevention and protection, while in another, heat stress and its resulting urban heat island effect could be a more pressing challenge. National governments can also use the indicator framework to compare GHG emissions trajectories and climate vulnerabilities between different cities and regions and identify trends that might not be visible otherwise. Consequently, the indicator framework can help standardise how climate data are collected and reported, minimising the potential bias of information and increasing data comparability.
Building on the results of the localised indicator framework, national governments can also adapt national climate policy frameworks such as the NDCs and NAPs to the specific needs and characteristics of different cities and regions. An important option is to disaggregate net zero targets and adaptation goals in NDCs and NAPs by regions/cities, recognising and valuing disparities of climate change impacts and exposure to climate risks. Similarly, the indicator framework can help policy makers consider and reflect local contexts in NAPs (e.g. more granular risk vulnerability assessment), although NAPs are relatively more advanced in recognising the importance of subnational actions. Most importantly, national governments can raise the ambition of climate targets/plans by leveraging the potential of subnational climate action. They can also stimulate stronger ownership of national climate policies from subnational actors by jointly developing climate targets so that they are widely accepted.
Subnational governments can improve their understanding of climate change impacts on their territories and adjust their policies and strategies accordingly. They can also identify whether their climate-related investments are addressing the most relevant climate challenges. Subnational governments can identify common areas of concern with other neighbouring cities and/or cities facing similar challenges and problems. This would allow them to exchange information on potential solutions to climate challenges and enhance partnerships. Furthermore, subnational governments can better understand how they contribute to climate action and analyse whether their targets align with those of national governments.
While the proposed indicator framework can already facilitate multi-level dialogue, localise national policy frameworks and promote efficient public expenditure, the indicator framework can be further extended to provide a more comprehensive assessment of the local climate challenges and opportunities. Major potential areas for future work can be summarised as follows:
Estimating more indicators. The indicator framework provides a comprehensive set of 45 comparable indicators. Out of these 45, 41 have already been estimated at different geographical levels based on publicly available data sources. Further work is required to make the remaining indicators available for analysis, including consumption-based GHG emissions.
Defining/refining reference points. The indicator framework attributes specific reference points 28 out of its 45 indicators to assess the progress of OECD cities and regions. Future research should explore the possibility of defining reference points for the remaining indicators. In addition, the proposed reference points can be further refined to adjust to the evolving global and national targets and to reflect local specificities.
Making indicators available at more granular territorial scales (e.g. FUA level). The proposed indicator framework aims to provide national and subnational governments with a tool to go beyond national averages. However, the geographical resolution/coverage varies depending on the geographical unit to which each indicator is associated. While 36 and 35 indicators are available for regions at TL2 and TL3 levels, respectively, only 25 indicators in the framework are currently available for cities (FUAs). Indicators with more granular territorial scales would allow a better understanding of territorial disparities, which can lead to more locally tailored and more effective policies.
Providing a visualisation tool. The indicator framework can draw inspiration from the SDG localised indicators. This includes a web tool to visualise the performance of cities and regions in comparison to national averages. It also indicates the remaining distance to the suggested end values required to reach the 2030 goals. This tool not only provides valuable insights into crucial policy areas but also identifies cities and regions encountering similar opportunities and challenges.
Developing more indicators under the “actions and opportunities” category. The indicator framework can be improved to provide a more comprehensive understanding of actions and opportunities at the subnational scale. An option is to develop indicators to examine institutional and governance dimensions (e.g. horizontal co-ordination mechanisms across departments in subnational governments). Another option is to develop indicators to measure policy impacts and effectiveness (e.g. cost efficiency of different policy instruments).
Annex 2.A. Complete list of territorial climate indicators and reference points
Annex Table 2.A.1. OECD territorial climate indicator framework
Indicator |
Data source |
Description |
Geographical resolution, coverage |
Temporal resolution, coverage |
Reference point |
---|---|---|---|---|---|
Climate mitigation: Pressure indicators (19) |
|||||
1. Total GHG emissions, level and % change |
Emissions Database for Global Atmospheric Research (EDGAR) (Crippa et al., 2021[16]) |
Total GHG emissions, expressed in tonnes of CO2 equivalents by considering the 3 main GHGs, namely CO2, CH4 and N2O and a 100-year global warming potential (GWP). CO2 emissions from land use, land use change, forestry and fluorinated gases are excluded. |
TL2 and TL3 regions, FUAs of more than 500 000 inhabitants |
1970-2018, yearly |
The 2030 emissions per capita target (4.7 tCO2‑eq) defined based on computations derived from the IEA NZE Scenario for advanced economies (IEA, 2021[13]) multiplied by 2030 population projection |
2. GHG emissions per capita, level and % change |
EDGAR (Crippa et al., 2021[16]) and Global Human Settlement Population (Schiavina, Freire and MacManus, 2019[42]) |
GHG emissions per capita, expressed in tonnes of CO2 equivalent per capita by considering the 3 main GHGs, namely CO2, CH4 and N2O and a 100-year GWP. CO2 emissions from land use, land use change, forestry and fluorinated gases are excluded. |
TL2 and TL3 regions, FUAs of more than 500 000 inhabitants |
1970-2018, yearly |
The 2030 emissions per capita target (4.7 tCO2‑eq) defined based on computations derived from the IEA NZE Scenario for advanced economies (IEA, 2021[13]) |
3. GHG emission intensities per unit of GDP, level and % change |
EDGAR (Crippa et al., 2021[16]) and OECD Regional Database |
GHG emission intensities per unit of GDP, expressed in tonnes of CO2 equivalent per unit of GDP in USD thousands in 2015 prices and purchasing price parity. |
TL2 and TL3 regions |
2000-18, yearly |
To be defined |
4. GHG emissions by sector: share of total emissions, level, per capita and % change |
GHG emissions by sectors: agriculture, buildings, industry, power and transport. |
TL2 and TL3 regions, FUAs of more than 500 000 inhabitants |
1970-2018, yearly |
The 2030 emissions per capita target by sector, defined based on computations derived from the IEA NZE Scenario for advanced economies (IEA, 2021[13]): agriculture 0.1 tCO2-eq/capita; buildings 0.4 tCO2-eq/capita; industry 1.7 tCO2-eq/capita; power 1.3 tCO2-eq/capita; transport 1.3 tCO2-eq/capita |
|
5. Consumption-based GHG emissions (to be estimated) |
To be defined |
GHG emissions embodied in goods and services consumed in a particular territory. |
To be defined |
To be defined |
The 2030 emissions per capita target (4.7 tCO2‑eq) defined based on computations derived from the IEA NZE Scenario for advanced economies (IEA, 2021[13]) |
6. Electricity generation by source |
Global Power Plant Database (GPPD) (GEO, 2021[17]) Harmonised global dataset of wind and solar farms (Dunnett, Sorichetta and Taylor, 2020[18]) |
Renewable energy sources include hydropower, wind, waste, biomass, wave and tidal, geothermal and solar. Fossil fuels include coal, oil, petroleum coke and natural gas. |
TL2 and TL3 regions |
2019 |
100% of electricity generated from low‑carbon sources |
7. Carbon intensity of electricity generation |
GPPD (GEO, 2021[17]) Harmonised global dataset of wind and solar farms (Dunnett, Sorichetta and Taylor, 2020[18]) |
Carbon Intensity of electricity generation corresponds to the amount of GHG emitted per unit of electricity produced (in gCO2-eq/kWh). |
TL2 and TL3 regions |
2019 |
Carbon intensity of electricity generated by the lowest emission-intensive source, i.e. 11 gCO2-eq/kWh (wind) |
8. Energy mix in total energy supply (to be estimated) |
To be defined |
This energy mix refers to all the primary energies consumed by an area to meet its needs. It is important to distinguish the energy mix from the electricity mix, which only covers the energy consumed to generate electricity. |
To be defined |
To be defined |
To be defined |
9. Total final energy consumption by source (to be estimated) |
To be defined |
The final energy consumption is the total energy consumed by end users, such as agriculture, industry, services or the residential sector. |
To be defined |
To be defined |
To be defined |
10. Emission intensity of the manufacturing industry |
EDGAR (Crippa et al., 2021[16]) and OECD Regional Database (OECD, 2022[61]) |
The emission intensity of the manufacturing industry corresponds to the GHG emissions per unit of GVA (tCO2-eq/USD thousands) in the sector. Manufacturing industry emissions include the combustion for manufacturing, oil refineries and transformation industry, chemical processes, non-metallic minerals production, iron and steel production, non‑ferrous metals production, non-energy use of fuels and solvents and products use. |
TL2 and TL3 regions |
2017 |
To be defined |
11. Access to public transport |
Open Street Map (Haklay and Weber, 2008[62]) and Mapbox Isochrone API |
Access to public transport is defined as the share of people having a public transport stop (metro, tram or bus) within a ten-minute walk. |
Metropolitan areas (only largest metropolitan area in each OECD country) |
2022 |
100% of the population with access to public transport |
12. Private vehicle ownership |
OECD Regional Database (OECD, 2022[63]) |
Private vehicle ownership is measured as the number of motor vehicles per 1 000 inhabitants. Motor vehicles include road vehicles, other than motorcycles, intended for passengers and designed for fewer than nine persons, including the driver. |
TL2 and TL3 regions |
2021 |
Best performers, e.g.. 385 vehicles for 1 000 inhabitants for TL2 regions |
13. Electric and hybrid vehicle adoption |
OECD Regional Database (OECD, 2022[63]) |
Adoption of electric and hybrid vehicles is measured as the share of private electric or hybrid vehicles in total private vehicles. |
TL2 and TL3 regions |
2021 |
100% of electric or hybrid vehicle adoption |
14. Cooling and heating degree days, levels and change, residential built-up volume |
High-resolution Historical Global Gridded Dataset Of Annual Cooling And Heating Degree Days (Mistry, 2019[64]), Global Human Settlement Built-up Volume (Pesaresi and Politis, 2022[32]). |
Heating degree days (respectively cooling) measure the heating needs of buildings. It is the sum of the differences between the mean outdoor temperature and a standard temperature (15°C). As space heating is the main source of residential energy consumption in OECD countries, heating degree days can be used as a proxy of residential pressure on energy consumption. The volume of residential buildings that need to be heated or cooled is also a measure of pressure on building energy consumption. |
TL2 and TL3 regions, FUAs |
1970-2019 |
Not applicable |
15. Built-up area growth |
Global Human Settlement Population (Schiavina et al., 2023[55]) and Built‑up Surface Grid (Pesaresi and Politis, 2023[65]) |
Land use pressure is measured by looking at the built-up area % change, the level of built-up area per capita and at the difference between built-up area growth and population growth. |
TL2, TL3 regions, FUAs |
1975-2030 (5-year interval) |
To be defined |
16. Built-up area per capita |
Global Human Settlement Population (Schiavina et al., 2023[55]) and Built‑up Surface Grid (Pesaresi and Politis, 2023[65]) |
Land use pressure is measured by looking at the built-up area % change, the level of built-up area per capita and at the difference between built-up area growth and population growth. |
TL2, TL3 regions, FUAs |
1975-2030 (5-year interval) |
Best performers, e.g.. 65 square metres per capita for metropolitan areas |
17. Difference between built-up area growth and population growth |
Global Human Settlement Population (Schiavina et al., 2023[55]) and Built‑up Surface Grid (Pesaresi and Politis, 2023[65]) |
Land use pressure is measured by looking at the built-up area % change, the level of built-up area per capita and at the difference between built-up area growth and population growth. |
TL2, TL3 regions, FUAs |
1975-2030 (5-year interval) |
To be defined |
18. Waste generation |
OECD Regional Database (OECD, 2022[63]) |
Waste generation corresponds to the amount of municipal waste per capita (in kg/capita). |
TL2 and TL3 regions |
2020 |
Best performers, e.g. 350 kg per capita per year for TL2 regions |
19. Waste recovery |
OECD Regional Database (OECD, 2022[63]) |
Waste recovery corresponds to the share of municipal waste that is treated with recovery operations. This includes waste that undergoes material recycling, composting, incineration with energy recovery and other forms of recovery. Landfilling is excluded. |
TL2 and TL3 regions |
2020 |
100% of recycled municipal waste |
Climate adaptation: State indicators (10) |
|||||
Exposure to climate-related hazards |
|||||
20. Air temperature and change |
ERA5-Land (Muñoz Sabater, 2019[36]) |
The air temperature corresponds to the annual average temperature at 2 m above the surface of the Earth. The change is defined with respect to the baseline 1981-2010 climatology. |
TL2 and TL3 regions, FUAs |
1981-2022 |
Mean air temperature over 1981-2010 |
21. Number of hot days and change |
ERA5-Land (Muñoz Sabater, 2019[36]) |
Hot days correspond to days during which the maximum daily temperature exceeds 35°C. The change is defined with respect to the baseline 1981-2010 climatology. |
TL2 and TL3 regions, FUAs |
1981-2022 |
Mean number of hot days over 1981‑2010 |
22. Number of tropical nights and change |
ERA5-Land (Muñoz Sabater, 2019[36]) |
Tropical nights correspond to days during which the minimum daily temperature is higher than 20°C. The change is defined with respect to the baseline 1981-2010 climatology. |
TL2 and TL3 regions, FUAs |
1981-2022 |
Mean number of tropical nights over 1981-2010 |
23. Number of icing days and change |
ERA5-Land (Muñoz Sabater, 2019[36]) |
Icing days correspond to days during which the maximum daily temperature does not exceed 0°C. The change is defined with respect to the baseline 1981-2010 climatology. |
TL2 and TL3 regions, FUAs |
1981-2022 |
Mean number of icing days over 1981‑2010 |
24. Heat stress exposure |
Copernicus thermal comfort indices derived from ERA5 reanalysis (ERA5-HEAT), Universal Thermal Climate Index (UTCI) |
Population exposure to heat stress is defined using the UTCI, which measures the impact of atmospheric conditions on the human body. UTCI values between 32°C and 38°C correspond to strong heat stress, 38°C to 46°C to very strong heat stress and above 46°C to extreme heat stress. |
TL2 and TL3 regions, FUAs |
1981-2021, yearly |
Mean number of days of different heat stress levels over 1981-2010 |
25. Urban heat island intensity (daytime and night-time) |
MODIS Aqua and Terra Land Surface Temperature and MODIS Land Cover Data |
The urban heat island intensity measures the difference in temperatures between the city and its surrounding area. The urban heat island intensity is here defined for a FUA as the difference in land surface temperature between the built-up area and its surroundings. |
FUAs, SAUs |
2012-21, yearly |
To be defined |
26. Population exposure to fires |
JRC’s Global Wildfire Dataset and Global Human Settlement Population (Schiavina, Freire and MacManus, 2019[42]) |
Wildfire population exposure is calculated as the population located within a 5 km buffer around fires. |
TL2 and TL3 regions |
2000-21 |
Reference point defined as 0% |
27. Burnt area by land cover type |
JRC’s Global Wildfire Dataset and ESA CCI Land Cover Data (ESA, 2019[28]) |
Burnt area by land cover type (forests and croplands) are measured as the share of forest area burnt as a share of the total forest area. |
TL2 and TL3 regions |
2000-21 |
Reference point defined as 0% |
28. Forest exposure to wildfire danger |
Copernicus Climate Data Store Fire Danger Indices Historical Data (EC, 2019[43]) |
Forest exposure to wildfire danger is measured using the Fire Weather Index (FWI) System and enables the identification of forests in areas at risk of burning and which have not necessarily burnt. |
TL2 and TL3 regions |
2000-21 |
Reference point defined as 0% |
29. Population exposure to river flooding |
JRC River Flood Hazard Maps (Dottori et al., 2021[44]), and Global Human Settlement Population (GHS-POP) Layer (Schiavina, Freire and MacManus, 2022[31]) |
Population exposure to river flooding is calculated for 100-year return period for river and coastal flooding. A return period refers to the recurrence of floods with a similar intensity. |
TL2 and TL3 regions, FUAs |
2015 |
To be defined |
30. Population exposure to coastal flooding |
World Bank Global Coastal Flood Hazard (Muis et al., 2016[47]) and Global Human Settlement Population (GHS-POP) Layer (Schiavina, Freire and MacManus, 2022[31]) |
Population exposure to coastal flooding is calculated for 100-year return period for river and coastal flooding. A return period refers to the estimated time interval between floods of similar intensity. |
TL2 and TL3 regions, FUAs |
2015 |
To be defined |
31. Agricultural drought |
ERA5-Land (Muñoz Sabater, 2019[36]) and ESA CCI Land Cover Data (ESA, 2019[28]) |
Droughts are defined in terms of cropland soil moisture anomaly, i.e. the percentage change in soil moisture compared to the reference period 1981-2010. |
TL2 and TL3 regions |
1981-2021, yearly |
Mean cropland soil moisture over 1981-2010 |
32. Cumulated precipitation and change |
ERA5-Land (Muñoz Sabater, 2019[36]) |
Cumulated precipitation refers to the accumulated liquid and frozen water, including rain and snow, that falls to the Earth’s surface. The change is defined with respect to the baseline 1981-2010 climatology. |
TL2, TL3 regions, and FUAs |
1981-2021, yearly |
Mean cumulated precipitation over 1981-2010 |
33. Extreme precipitation days and change |
ERA5-Land (Muñoz Sabater, 2019[36]) |
Extreme precipitation days correspond to days when the total amount of precipitation exceeds 20 mm. The change is defined with respect to the baseline 1981-2010 climatology. |
TL2, TL3 regions, and FUAs |
1981-2021, yearly |
Mean extreme precipitation days over 1981-2010 |
34. Built-up exposure to violent storms |
Copernicus Climate Data Store (CDS) ERA5 Hourly Data on Single Levels |
An area is considered exposed to violent storms or worse if, for at least 1 hour, the wind gust speed > 28.6 metres per second (threshold based on the Beaufort scale). |
TL2 and TL3 regions |
2000-21 |
Mean exposure over 1981-2010 |
Socio-economic factors driving vulnerability |
|||||
35. Population density |
OECD regional and metropolitan databases |
Areas with high population density are, in general, more vulnerable to climate-related impacts, as they experience increased resource and infrastructure stress, higher health risks and challenges related to migration and adaptation. |
TL2, TL3 and FUAs |
2000-22 |
Not applicable |
36. Population aged < 5 |
OECD regional and metropolitan databases |
Young children are particularly vulnerable to heat stress, diseases and malnutrition exacerbated by climate change. |
TL2, TL3 and FUAs |
2000-22 |
Not applicable |
37. Population aged > 70 |
OECD regional and metropolitan databases |
Elderly population often face increased health risks from extreme heat and limited mobility, which makes them particularly vulnerable to the impacts of climate change. |
TL2, TL3 and FUAs |
2000-22 |
Not applicable |
38. Unemployment rate |
OECD regional and metropolitan databases |
High unemployment rates lead to limited access to resources, increased social vulnerability and limited adaptative capacities to climate change impacts. |
TL2, TL3 and FUAs |
2000-22 |
Not applicable |
39. Poverty rate |
OECD regional and metropolitan databases |
Poverty rate is the ratio of the number of people whose income falls below the poverty line, defined as 60% of the median household income after taxes and transfers, over the total population. |
TL2, TL3 and FUAs |
2007-22 |
Not applicable |
Actions and opportunities: Response indicators (6) |
|||||
40. Existence of subnational climate mitigation and adaptation targets and compliance with national targets (to be estimated) |
To be defined |
Local emission reduction targets by 2030 would enable the assessment of their compliance with NDCs. |
National scale |
2030, 2050 targets |
100% of subnational governments (e.g. TL2, TL3 levels) with climate mitigation and adaptation targets complying with national targets |
41. Citizens’ satisfaction with efforts to preserve the environment |
OECD based on the Gallup World Poll (Gallup World Poll, 2019[51]) |
The percentage of the population satisfied with efforts to preserve the environment by using data from the Gallup World Poll (2019[51]). |
TL2 regions |
2008-18 |
Best performers |
42. Green areas in cities |
ESA World Cover 2020 (Zanaga et al., 2021[29]) and Global Human Settlement Urban Centre Database (Florczyk et al., 2019[66]), Access to Green Urban Areas in Europe (Poelman, 2018[53]) |
Green areas in cities, measured in two ways: - Share of green areas in urban centres using land cover data. Green areas correspond to trees, shrublands and grasslands. - The share of the population with access to public green areas of more than 1 ha within a 400 m walk. |
FUA’s urban centres, SAUs |
2021 |
Best performers |
43. Climate-significant expenditure and investments |
Subnational Government Climate Finance database, OECD Regional Statistics (database) (OECD, 2022[59]) |
Subnational government investment and expenditure in environmental protection and climate are important instruments for both climate mitigation and adaptation (OECD, 2022[57]). |
National scale |
2009-19 |
Best performers |
44. Existence of climate-related funds, grants and subsidies for subnational action |
OECD Compendium of Fiscal Tools for Subnational Climate Action (OECD, 2022[67]) |
Qualitative information on public sources of revenue available to subnational governments in OECD and EU countries to fund and finance their climate action. |
National scale |
2022 |
To be defined |
45. Patent applications in climate mitigation and adaptation technologies as % of total technologies |
PATSTAT database (OECD, 2023[60]), European Patent Organisation |
Patent applications in climate mitigation and adaptation technologies are essential in the deployment of low-carbon technologies and in the transition to net zero. |
TL2 and TL3 regions, FUAs |
To be defined |
Best performers |
References
[45] Alfieri, L. et al. (2017), “Global projections of river flood risk in a warmer world”, Earth’s Future, Vol. 5/2, pp. 171-182, https://doi.org/10.1002/2016ef000485.
[41] Artes Vivancos, T. et al. (2019), “A global wildfire dataset for the analysis of fire regimes and fire behaviour”, Scientific DAta, Vol. 6, p. 296, https://doi.org/10.6084/m9.figshare.10284101.
[68] Arup (2023), “Global CRAFT allows cities to report climate hazards and associated adaptation planning”, Climate Risk and Adaption Framework and Taxonomy (CRAFT), https://www.arup.com/projects/climate-risk-and-adaption-framework-and-taxonomy-project (accessed on 27 September 2023).
[30] Brown, C. et al. (2022), “Dynamic World, Near real-time global 10 m land use land cover mapping”, Scientific Data, Vol. 9/1, p. 251, https://doi.org/10.1038/s41597-022-01307-4.
[46] C40 Cities (2018), The Future We Don’t Want: How Climate Change Could Impact the World’s Greatest Cities, https://www.c40.org/what-we-do/scaling-up-climate-action/adaptation-water/the-future-we-dont-want/.
[8] C40 Cities/Ramboll/Ramboll Fonden (2019), Measuring Progress in Urban Climate Change Adaption Monitoring - Evaluating - Reporting Framework, https://www.c40.org/wp-content/static/other_uploads/images/2154_20190228_MER_Framework_Final.original.pdf?1553033351.
[70] Carter, J. et al. (2018), European Climate Risk Typology – Final Report, http://european-crt.org/methodology.html.
[3] CDP (2023), CDP Cities, States and Regions Open Data Portal, Carbon Disclosure Project, https://data.cdp.net/ (accessed on 27 September 2023).
[39] Chakraborty, T. and X. Lee (2019), “A simplified urban-extent algorithm to characterize surface urban heat islands on a global scale and examine vegetation control on their spatiotemporal variability”, International Journal of Applied Earth Observation and Geoinformation, Vol. 74, pp. 269-280.
[48] Copernicus Global Land Service (n.d.), Surface Soil Moisture, https://land.copernicus.eu/global/products/ssm (accessed on 2023).
[16] Crippa, M. et al. (2021), EDGAR v6.0 Greenhouse Gas Emissions, Joint Research Centre (JRC), European Commission.
[44] Dottori, F. et al. (2021), River Flood Hazard Maps for Europe and the Mediterranean Basin Region, Joint Research Centre (JRC), European Commission.
[18] Dunnett, S., A. Sorichetta and G. Taylor (2020), “Harmonised global datasets of wind and solar farm locations and power”, Scientific Data, Vol. 7/1, p. 130.
[7] EBRD (2022), Green City Action Plan Methology, European Bank for Reconstruction and Development, https://www.ebrdgreencities.com/assets/Uploads/PDF/GCAP_2-1_Methodology_January2022.pdf (accessed on 15 September 2023).
[49] EC (2022), ERA5-Land Monthly Averaged Data from 1950 to Present, European Centre for Medium-range Weather Forecast, European Commission, https://cds.climate.copernicus.eu/cdsapp#!/dataset/reanalysis-era5-land-monthly-means?tab=overview.
[43] EC (2019), Fire Danger Indices Historical Data from the Copernicus Emergency Management Service, Copernicus Climate Change Service, Climate Data Store, European Commission, https://doi.org/10.24381/cds.0e89c522.
[9] EC (2018), How to Develop a Sustainable Energy and Climate Action Plan (SECAP), Joint Research Centre, European Commission, https://doi.org/10.2760/223399.
[28] ESA (2019), Land Cover Classification Gridded Maps from 1992 to Present Derived from Satellite Observations, Climate Change Initiative, European Space Agency.
[52] EU et al. (2021), Applying the Degree of Urbanisation - A Methodological Manual to Define Cities, Towns and Rural Areas for International Comparisons - 2021 Edition, https://ec.europa.eu/eurostat/web/products-manuals-and-guidelines/-/ks-02-20-499.
[66] Florczyk et al. (2019), GHS Urban Centre Database 2015, Multitemporal and Multidimensional Attributes, R2019A, https://data.jrc.ec.europa.eu/dataset/53473144-b88c-44bc-b4a3-4583ed1f547e.
[27] Friedl, M. and D. Sulla-Menashe (2019), MCD12Q1 MODIS/Terra+Aqua Land Cover Type Yearly L3 Global 500m SIN Grid V006, NASA EOSDIS Land Processes DAAC.
[51] Gallup World Poll (2019), Gallup World Poll (database), http://www.gallup.com/services/170945/worldpoll.aspx.
[17] GEO (2021), Global Power Plant Database, Global Energy Observatory, Google, KTH Royal Institute of Technology in Stockholm, Enipedia, World Resources Institute.
[62] Haklay and Weber (2008), “OpenStreetMap: User-generated street maps”, IEEE Pervasive Computing, Vol. 7, pp. 12-18.
[26] IEA (2022), A 10-Point Plan to Cut Oil Use, International Energy Agency, Paris, https://www.iea.org/reports/a-10-point-plan-to-cut-oil-use.
[21] IEA (2022), Buildings, International Energy Agency, Paris, https://www.iea.org/reports/buildings.
[15] IEA (2022), Global Energy Review: CO2 Emissions in 2021, International Energy Agency, Paris, https://www.iea.org/reports/global-energy-review-co2-emissions-in-2021-2.
[19] IEA (2022), “OECD - Electricity and heat generation”, IEA Electricity Information Statistics (database), https://doi.org/10.1787/data-00457-en (accessed on 18 October 2022).
[22] IEA (2022), “Residential sector detailed data and indicators (Edition 2022)”, IEA Energy Efficiency Indicators (database), https://doi.org/10.1787/1973536b-en (accessed on 29 November 2023).
[23] IEA (2022), Space Cooling, International Energy Agency, Paris, https://www.iea.org/reports/space-cooling.
[24] IEA (2022), Transport Sector CO2 Emissions by Mode in the Sustainable Development Scenario, 2000-2030, International Energy Agency, Paris, https://www.iea.org/data-and-statistics/charts/transport-sector-co2-emissions-by-mode-in-the-sustainable-development-scenario-2000-2030.
[13] IEA (2021), Net Zero by 2050, International Energy Agency, Paris, https://www.iea.org/reports/net-zero-by-2050.
[25] IEA (2019), “Growing preference for SUVs challenges emissions reductions in passenger car market”, International Energy Agency, Paris.
[20] IPCC (2014), AR5 Climate Change 2014: Mitigation of Climate Change, Intergovernmental Panel on Climate Change.
[33] IPCC (2014), Climate Change 2014: Impacts, Adaptation, and Vulnerability. Part A: Global and Sectoral Aspects, Intergovernmental Panel on Climate Change, https://www.ipcc.ch/site/assets/uploads/2018/02/WGIIAR5-PartA_FINAL.pdf.
[4] ISO (2020), ISO 37123:2019 Sustainable Cities and Communities - Indicators for Resilient Cities, International Organization for Standardization, https://www.iso.org/standard/70428.html.
[50] Krekel, C. and M. George (2020), How Environmental Quality Affects Our Happiness, https://worldhappiness.report/ed/2020/how-environmental-quality-affects-our-happiness/.
[38] Lombardi, A., M. Rodas and M. Ledesma (2022), “Extreme heat is a silent killer of climate change: How can cities bit?”, https://oecdcogito.blog/2022/08/03/extreme-heat-is-the-silent-killer-of-climate-change-how-can-cities-beat-it/.
[6] Mackres, E., S. Shabou and T. Wong (2023), “Calculating indicators from global geospatial data sets for benchmarking and tracking change in the urban environment”, World Resources Institute, https://doi.org/10.46830/writn.22.00123.
[34] Maes, M. et al. (2022), “Monitoring exposure to climate-related hazards: Indicator methodology and key results”, OECD Environment Working Papers, No. 201, OECD Publishing, Paris, https://doi.org/10.1787/da074cb6-en.
[37] Masson-Delmotte, V. et al. (2021), IPCC, 2021: Climate Change 2021: The Physical Science Basis, Contribution of Working Group I to the Sixth Assessment Report of the Intergovernmental Panel on Climate Change, Cambridge University Press.
[64] Mistry, M. (2019), A High-resolution (0.25 degree) Historical Global Gridded Dataset of Monthly and Annual Cooling and Heating Degree Days (1970-2018) Based on GLDAS Data, PANGAEA, https://doi.org/10.1594/PANGAEA.903123.
[47] Muis, S. et al. (2016), “A global reanalysis of storm surges and extreme sea levels”, Nature Communications, Vol. 7/1, pp. 1-12, https://doi.org/10.1038/ncomms11969.
[36] Muñoz Sabater, J. (2019), ERA5-Land Monthly Averaged Data from 1981 to Present, https://doi.org/10.24381/cds.68d2bb30.
[5] Net Zero Tracker (2023), Methodology, https://zerotracker.net/methodology (accessed on 27 September 2023).
[60] OECD (2023), STI Micro-data Lab: Intellectual Property Database, OECD, Paris, http://oe.cd/ipstats.
[67] OECD (2022), Compendium of Financial Instruments that Support Subnational Climate Action in OECD and EU Countries, OECD, Paris, https://www.oecd.org/regional/compendiumsubnationalrevenue.htm.
[10] OECD (2022), International Programme for Action on Climate, OECD, Paris, https://www.oecd.org/climate-action/ipac/ (accessed on 18 October 2022).
[11] OECD (2022), Measuring the Distance to the SDGs in Regions and Cities, OECD, Paris, https://www.oecd-local-sdgs.org/index.html (accessed on 7 November 2022).
[1] OECD (2022), OECD Regions and Cities at a Glance 2022, OECD Publishing, Paris, https://doi.org/10.1787/14108660-en.
[61] OECD (2022), “Regional economy”, OECD Regional Statistics (database), https://doi.org/10.1787/6b288ab8-en (accessed on 18 October 2022).
[63] OECD (2022), “Regional social and environmental indicators”, OECD Regional Statistics (database), https://doi.org/10.1787/e6adb759-en (accessed on 18 October 2022).
[57] OECD (2022), “Subnational government climate expenditure and revenue tracking in OECD and EU Countries”, OECD Regional Development Papers, No. 32, OECD Publishing, Paris, https://doi.org/10.1787/1e8016d4-en.
[59] OECD (2022), “Subnational government climate finance database”, OECD Regional Statistics, https://stats.oecd.org/Index.aspx?datasetcode=SGCFD (accessed on 28 October 2022).
[12] OECD (2021), “International Programme for Action on Climate (IPAC) - Proposal for a first edition of the indicator dashboard”, OECD, Paris.
[2] OECD (2020), A Territorial Approach to the Sustainable Development Goals: Synthesis report, OECD Urban Policy Reviews, OECD Publishing, Paris, https://doi.org/10.1787/e86fa715-en.
[58] OECD (n.d.), Subnational Government Climate Finance Hub, http://www.oecd.org/regional/sngclimatefinancehub.htm (accessed on 28 November 2023).
[56] OECD/UCLG (n.d.), World Observatory on Subnational Government Finance and Investment, https://www.sng-wofi.org/ (accessed on 28 November 2023).
[54] OpenStreetMap (2023), “Planet dump”, https://planet.osm.org.
[65] Pesaresi, M. and P. Politis (2023), GHS-BUILT-S R2023A - GHS Built-up Surface Grid, Derived from Sentinel2 Composite and Landsat, Multitemporal (1975-2030), Joint Research Centre (JRC), European Commission, https://doi.org/10.2905/9F06F36F-4B11-47EC-ABB0-4F8B7B1D72EA.
[32] Pesaresi, M. and P. Politis (2022), GHS Built-up Surface Grid, Derived from Sentinel2 Composite and Landsat, Multitemporal (1975-2030), Joint Research Centre (JRC), European Commission, http://data.europa.eu/89h/d07d81b4-7680-4d28-b896-583745c27085.
[53] Poelman, H. (2018), “A walk to the park? Assessing access to green areas in Europe’s cities”, DG Regional ad Urban Policy Working Paper.
[55] Schiavina, M. et al. (2023), GHS-POP R2023A - GHS Population Grid Multitemporal (1975-2030), Joint Research Centre (JRC), European Commission, https://doi.org/10.2905/2FF68A52-5B5B-4A22-8F40-C41DA8332CFE.
[31] Schiavina, M., S. Freire and K. MacManus (2022), GHS-POP R2022A - GHS Population Grid Multitemporal (1975-2030), Joint Research Centre (JRC), European Commission, http://data.europa.eu/89h/d6d86a90-4351-4508-99c1-cb074b022c4a.
[42] Schiavina, M., S. Freire and K. MacManus (2019), GHS Population Grid Multitemporal (1975, 1990, 2000, 2015), Joint Research Centre (JRC), European Commission.
[14] Shukla, P. et al. (2022), IPCC, 2022: Climate Change 2022: Mitigation of Climate Change, Contribution of Working Group III to the Sixth Assessment Report of the Intergovernmental Panel on Climate Change, Cambridge University Press.
[35] The Lancet (2021), “Health in a world of extreme heat”, The Lancet, Vol. 398, https://www.thelancet.com/pdfs/journals/lancet/PIIS0140-6736(21)01860-2.pdf.
[40] Wan, Z., S. Hook and G. Hulley (2021), MODIS/MYD11A1 Aqua & MOD11A1 Terra Land Surface Temperature/Emissivity Daily L3 Global 1km SIN Grid V061.
[69] World Bank (2018), Urban Sustainability Framework (USF), World Bank, Washington, DC, https://documents1.worldbank.org/curated/en/339851517836894370/pdf/123149-Urban-Sustainability-Framework.pdf.
[29] Zanaga, D. et al. (2021), ESA WorldCover 10 m 2020 v100.
Notes
← 1. The OECD large regions (TL2) represent the first administrative tier of subnational government, such as provinces in Canada and states in the United States. The OECD small regions (TL3) correspond to administrative regions, except for Australia, Canada and the United States. An FUA is composed of a city and its commuting zone, encompassing the economic and functional extent of cities based on people’s daily movements.
← 2. Urban lands refer to built-up areas and non-urban lands refer to all other land cover classes, except water bodies.
← 3. Forests include the following Copernicus annual 300 m land cover (CCI-LC) classes: 50, 60, 61, 62, 70, 71, 72, 80, 81, 82, 90, 100 and 160.
← 4. Croplands include the following Copernicus annual 300 m land cover (CCI-LC) classes: cropland, rainfed, irrigated or post-flooding; mosaic cropland (>50%)/natural vegetation (tree, shrub, herbaceous cover) (<50%); and mosaic natural vegetation (tree, shrub, herbaceous cover) (>50%)/cropland (<50%).
← 5. Here we refer to expenditure and investment directed towards the economic activities the EU Technical Expert Group on Sustainable Finance (TEG) identified as significantly contributing to climate change adaptation and mitigation in their March 2020 report Taxonomy: Final Report of the Technical Expert Group on Sustainable Finance.