When individuals have highly unstable incomes and do not have the financial means to cope, they are said to be economically insecure. Economic insecurity is thus marked by an exposure, and a vulnerability to income instability. While low-income individuals are the most vulnerable to income shocks – in terms of having insufficient liquid assets to draw on – almost 50% of people on middle incomes in working-age households and 20% of higher income earners are at risk too. Given the frequency with which people experience income shocks, and the inadequacy of people’s financial buffers, economic insecurity affects one in six people in working‑age households in European OECD countries. The burden of economic insecurity falls predominantly on the unemployed and insecure workers, who are likely to face a heightened risk of economic insecurity going forward, given the digital transformations occurring in labour markets, which appear to affect people disproportionately and negatively in occupations with already high rates of economic insecurity
On Shaky Ground? Income Instability and Economic Insecurity in Europe
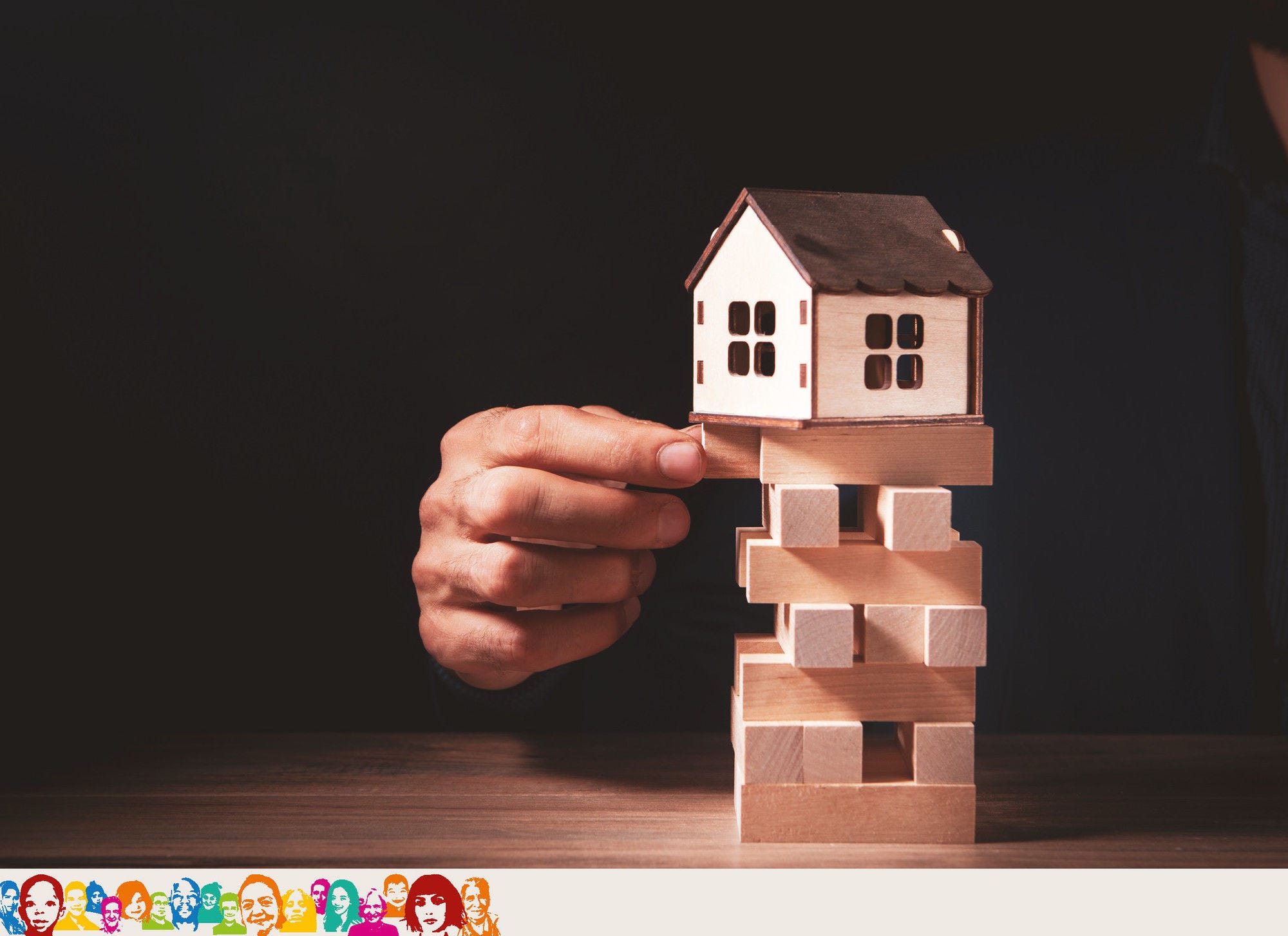
2. Economic insecurity
Abstract
2.1. Why should we focus on economic insecurity?
Economic insecurity refers to a person’s exposure, and vulnerability, to an economic loss (Hacker, 2018[1]). The previous chapter highlighted that many people in European OECD countries are exposed to economic losses, in the form of income instability. However, people may have the capacity to withstand fluctuations in their income by virtue of drawing on their assets, taking on loans, reducing their consumption or relying on friends and family for support – which makes them less vulnerable to economic loss.
Managing income shocks is becoming increasingly difficult in the context of COVID-19 and the cost‑of‑living crisis, which are eroding the purchasing power of people’s real incomes1 and leading some to draw down their savings and take on more debt (Box 2.1). More than 90% of European respondents to the recent OECD Risks that Matter survey reported that they are concerned about inflation and the cost of living in their country, and almost half are worried about paying for all of life’s essentials, such as food, energy and housing, and servicing their debt.2 The COVID-19 and cost-of-living crises have come at a time when many, particularly those at the bottom of the income and wealth distributions, already have low financial buffers and high levels of indebtedness and are thus in a more vulnerable position to manage future shocks. Even before these crises, almost one in ten households in the bottom 40% of the income distribution were over-indebted (that is, they had debt levels over three times their disposable income) (OECD, 2021[2]).
Further, these challenges to financial self-management are occurring at a time when governments have started to withdraw the extraordinary fiscal support that they introduced to deal with the twin COVID-19 and cost‑of‑living crises and now have less room for future large-scale fiscal stimulus. While governments acted swiftly to cushion the impacts of the cost-of-living crisis, policy measures were relatively expensive, as they were overwhelmingly broad-based (OECD, 2023[3]). Unlike the cost-of-living measures, government responses to COVID‑19 tended to be targeted at those most vulnerable to employment and income loss. However, most of the OECD countries that expanded unemployment benefits in response to COVID-19 have now wound them back, and many countries have ended their job retention schemes or tightened their eligibility or generosity (OECD, 2022[4]). Nevertheless, governments still play an important role (considered in Chapter 3) in providing financial support to people with unstable incomes and in delivering programmes to boost private means of managing income shocks.
Economic insecurity is therefore a pressing concern and risk for many. Like income instability, it is associated with serious negative effects in terms of individual and societal well‑being. It dampens living standards, fertility rates and general economic activity; and it is associated with food insecurity, poor adult health, mortgage delinquency, anxiety, fractured family formation, geographic immobility, political discontent and worse childhood outcomes in education, health and behaviour (Hacker and Jacobs, 2008[5]; Guiso et al., 2020[6]; Avram et al., 2019[7]; Rohde et al., 2017[8]; Smith, Stoddard and Barnes, 2007[9]; Reeves, McKee and Stuckler, 2014[10]; Stiglitz, Sen and Fitoussi, 2009[11]; Bhargava and Lown, 2006[12]; Mansour, 2018[13]; Stoetzer, Giesecke and Klüver, 2021[14]). The effects on childhood outcomes, health and geographic immobility have important implications for social mobility and human capital accumulation, which in turn highlights the influence of economic insecurity on current and future well-being (Boarini and Osberg, 2014[15]).
This chapter begins by analysing how vulnerable individuals in OECD countries are to potential economic losses (Section 2.2). It then combines the estimates of income instability in Chapter 1 with this analysis of vulnerability to measure economic insecurity (Section 2.3). Finally, Section 2.4 discusses the extent of economic insecurity in European OECD countries and describes the characteristics of people who are currently experiencing economic insecurity or are likely to be at risk of it in the future.
2.2. How vulnerable are individuals to economic loss?
To determine whether individuals are vulnerable to economic loss, this chapter uses the measure of asset inadequacy, which is common in many studies (Demertzis, Domínguez-Jiménez and Lusardi, 2020[16]; McKnight and Rucci, 2020[17]; Hacker, 2018[1]). Vulnerable (or financially fragile)3 individuals are those who do not have sufficient liquid assets4 to stay above the poverty line – with the threshold set at 50% of national median income – for three months (Balestra and Tonkin, 2018[18]; OECD, 2020[19]). In contrast, financially resilient individuals are those who have sufficient assets to stay out of poverty for at least three months.
On average across European OECD countries, 45% of people living in working-age5 households are financially fragile, ranging from less than 30% in Austria and the Netherlands up to more than 60% in Greece and Latvia6 (Figure 2.1). Financial fragility is more severe in countries where it is more prevalent. While, in Austria, the median financially fragile person holds almost enough liquid assets to keep themselves out of poverty for roughly a month, their Greek counterpart only has about a week’s worth. Indeed, Greece is one of the few countries where the rate of financial fragility increased markedly in the past decade (along with Germany and the Slovak Republic) – as holdings of liquid assets fell significantly during the Global Financial Crisis.
Figure 2.1. Almost one-in-two people living in working-age households are financially fragile
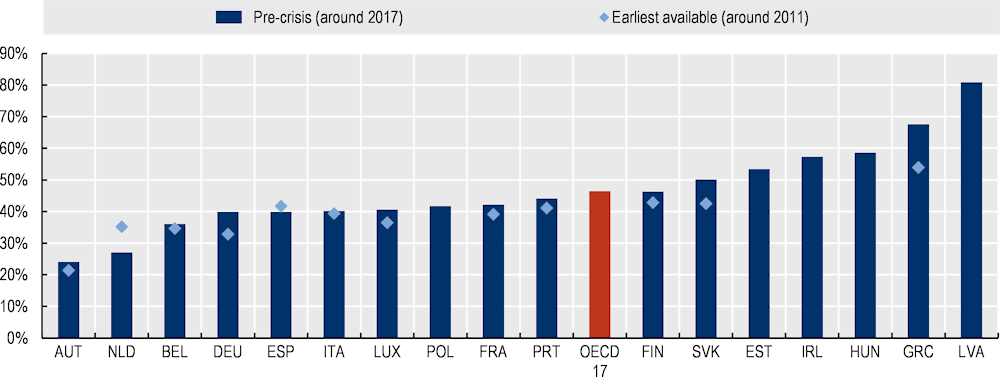
Note: Liquid assets are defined as the sum of currency and deposits; bonds and other debt securities; mutual funds and other investment funds; and other non-pension financial assets. The pre-crisis data refer to: 2016 for Finland, Italy, Lithuania, and Poland; 2017 for Austria, Belgium, Estonia, France, Germany, Hungary, Latvia, the Netherlands, Portugal, and the Slovak Republic; 2018 for Greece, Ireland, Luxembourg, and Spain. The earliest available year refers to: 2009 for France, Finland, and Greece; 2010 for Belgium, Italy, the Netherlands, and the Slovak Republic; 2011 for Austria, Germany, Luxembourg, and Portugal; and 2012 for Spain. The poverty line is based on disposable income, which is estimated by using a Machine Learning algorithm to impute disposable income from the gross income variable (the sum of wages and salaries, self-employment income, and property income and social transfers received all recorded before the payment of income taxes) available in the HFCS dataset. The analysis focuses only on those countries for which the results of the statistical matching procedure are highly satisfactory for 17 out of the 19 OECD-EU countries covered in the third wave of the HFCS. See Annex 2.A for more information on the method for estimating disposable income.
Source: OECD computations based on the Eurosystem Household Finance and Consumption Survey (HFCS), https://www.ecb.europa.eu/stats/ecb_surveys/hfcs/html/index.en.html.
Box 2.1. Financial fragility in times of economic crises
Recent crises have affected households’ finances in different ways and left them with varying abilities to respond to future challenges. Even before the onset of COVID-19, almost half of low-income individuals lacked emergency savings, and households in the bottom half of the wealth distribution had little to no net wealth (OECD, 2021[2]). Moreover, the scant liquid assets held by low-wealth households were primarily in bank accounts or bonds, which earned low rates of return (OECD, 2021[2]). In contrast, the top 10% experienced the fastest growth in their wealth in the decade leading up to the pandemic and by 2018 held 50% of total household wealth in OECD countries (OECD, 2021[2]).
Households’ financial prospects during COVID-19 depended largely on where they sat on the wealth distribution. In Europe, households in the top wealth quintile increased their savings more than other households, as asset prices quickly recovered after the initial shock to the point where prices in 2021 were markedly higher than in 2017, and they had limited opportunities for discretionary spending in the form of travel and going to restaurants (Household Finance and Consumption Network, 2023[20]). In contrast, households with little wealth to begin with ran down their savings or incurred debt (OECD, 2021[2]). Indebtedness rates declined for European households in the top three income quintiles, but there were increases in the shares of households with at least one unemployed adult and of young households (aged under 35) in debt (Household Finance and Consumption Network, 2023[20]).
While governments’ fiscal measures and the European Central Bank’s monetary policy settings helped to maintain household incomes, some European households saw their incomes fall during the pandemic. In Spain and Portugal, for example, 40% of households had lower income levels than in 2019. Households that experienced income losses during COVID-19 most frequently cut back on spending on food, clothing, travel and other non-durable consumer goods and services, although drawing down savings, selling assets and deferring debt were also common, particularly in France, Greece, Italy and Portugal (Household Finance and Consumption Network, 2023[20]). These households tended to have limited financial buffers to manage COVID-19, which put them at a disadvantage in dealing with the subsequent cost-of-living crisis.
Low‑income households were at a disadvantage for a few reasons when the cost-of-living crisis hit. First, they bore the brunt of the initial price spikes, as a higher proportion of their spending is on energy and food (OECD, 2022[4]). Recent OECD analysis (OECD, n.d.[21]) reveals that, all other things being equal, low-income households spend 4.2 percentage points more on food and 1.8 percentage points more on domestic energy than households in the middle of the income distribution (and 9.4 and 3.5 percentage points more on food and energy, respectively, than higher-income households). Second, low‑income households have less capacity to absorb increases in their cost of living even when facing similar rates of inflation as other households (OECD, 2022[4]). They have less scope to substitute for lower-price alternatives when they are often already buying cheaper versions of a given item (Argente and Lee, 2020[22]) and typically have lower savings and borrowing capacity to buffer the rise in the cost of living (OECD, 2022[4]; Balestra and Oehler, 2023[23]; OECD, 2021[2]). Further, low-income and/or low‑wealth households tend to earn relatively lower rates of return, since most of their meagre liquid assets are held in bank accounts with rates that do not keep pace with inflation.
In effect, successive recent shocks have had cumulative, negative effects on households with low incomes and/or low wealth – leaving them vulnerable to future shocks in the absence of opportunities to build their financial buffers.
Who are the financially fragile?
Financial fragility is not confined to the bottom of the income distribution. While more than two in three individuals living in working‑age lower-income households7 are financially fragile, almost one in two individuals living in working-age middle-class households are too, on average (Figure 2.2). In Ireland, Estonia, Greece, Hungary and Latvia, financial fragility affects the majority of middle-class individuals. Moreover, on average, financial fragility also affects one in six higher-income individuals, with this share being above 30% in Greece and Hungary and as high as 60% in Latvia.
Figure 2.2. More than 40% of individuals in middle-class households are financially fragile
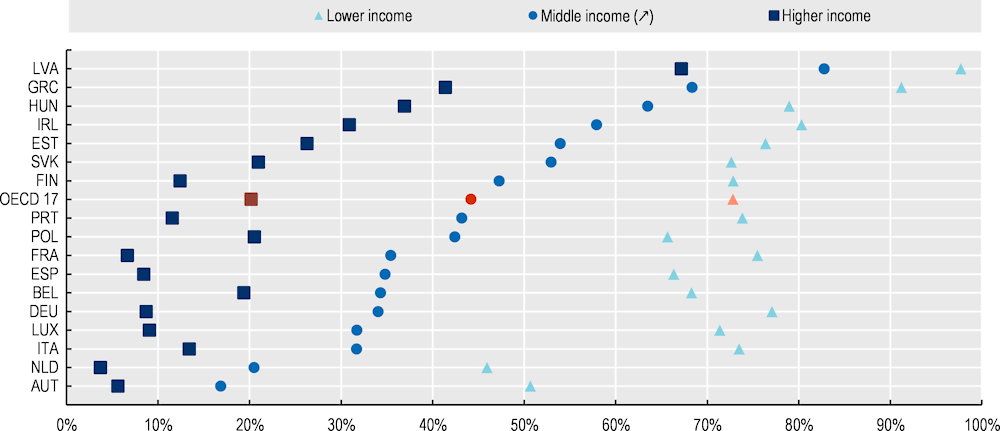
Note: The third wave of the Household Finance and Consumption Survey (HFCS) is used because it contains micro-level structural information on European households' assets and liabilities, e.g. real assets and their financing, liabilities/credit constraints, private businesses, financial assets, intergenerational transfers and gifts, and consumption and saving. However, the HFCS does not include information on household disposable income, and so a Machine Learning algorithm has been used to impute disposable income from the gross income variable (the sum of wages and salaries, self-employment income, and property income and social transfers received, all recorded before the payment of income taxes) available in the HFCS dataset (see Annex 2.A). The analysis focuses only on those countries for which the results of the statistical matching procedure are highly satisfactory: 17 out of the 19 OECD-EU countries covered in the 3rd wave of the HFCS. Lower income: <75% of the median equivalised disposable income. Middle income: >=75% and <200% of the median equivalised disposable income. Higher-income: >=200% of the median equivalised disposable income. Data are from 2016 for Finland, Italy and Poland; 2017 for Austria, Belgium, Estonia, France, Germany, Hungary, Latvia, the Netherlands, Portugal and the Slovak Republic; 2018 for Greece, Ireland, Luxembourg and Spain.
Source: OECD estimates based on the Eurosystem Household Finance and Consumption Survey (HFCS), https://www.ecb.europa.eu/stats/ecb_surveys/hfcs/html/index.en.html.
Since financial fragility is widespread across the income distribution, income is unlikely to be the sole determinant of an individual’s vulnerability to a potential economic loss. Education, housing tenure and number of children are equally, if not more, important than income (Figure 2.3). For instance, households headed by someone with a tertiary degree have a 33 percentage point lower likelihood of being financially fragile, while higher-income individuals have a 23 percentage point lower chance of financial fragility. These individuals have more opportunities to save and invest in assets, which reduces the risk of financial fragility. On average, among households with higher incomes, 60% of those who are not financially fragile manage to save, compared to 37% of the financially fragile. (For individuals in lower-income households, the rates are as low as 28% and 16%, respectively.) Similarly, housing is another important source of financial resilience, providing a large, even if illiquid, financial buffer (for example, re‑mortgaging or downsizing can release housing equity into liquid financial assets, if needed). As a result, renters are 24 percentage points more likely to be financially fragile than homeowners.
Figure 2.3. Low education, low income, renting and having multiple children are strong predictors of financial fragility
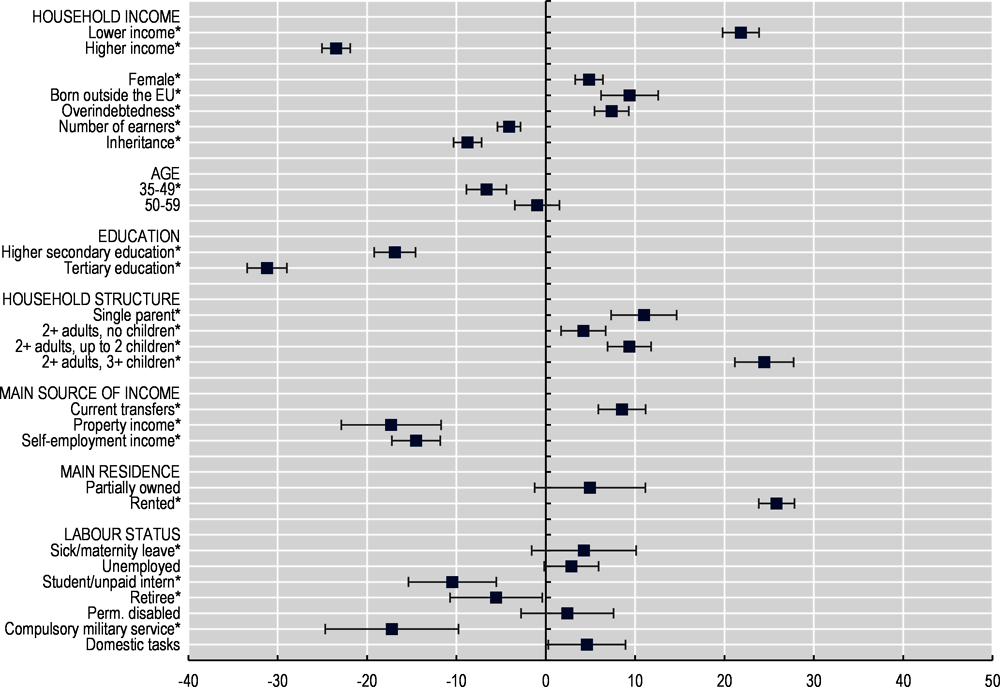
Note: * significant at the 5% level. Marginal effects from a logit model. The sample includes individuals in households with working-aged (18-59) employed heads. For the entire regression table with odds ratios, see Annex 2.B. The sample includes Austria, Belgium, Estonia, Finland, France, Germany, Greece, Hungary, Ireland, Italy, Latvia, Luxembourg, the Netherlands, Poland, Portugal, Spain and the Slovak Republic. Data are from 2016 for Finland, Italy and Poland; 2017 for Austria, Belgium, Estonia, France, Germany, Hungary, Latvia, the Netherlands, Portugal and the Slovak Republic; 2018 for Greece, Ireland, Luxembourg and Spain.
Source: OECD estimates based on the Eurosystem Household Finance and Consumption Survey (HFCS), https://www.ecb.europa.eu/stats/ecb_surveys/hfcs/html/index.en.html.
2.3. Estimating economic insecurity as the interplay between income instability and financial fragility
The results presented in the previous section revealed that people across the income distribution are vulnerable to potential economic losses, but they did not reveal who is most likely to experience an economic loss. In the remainder of this chapter, these two strands are combined to estimate economic insecurity.
Economic insecurity can be challenging to measure, as, conceptually, it is people’s anxiety or fear of the prospect of facing adverse events and having difficulty recovering (Osberg, 1998[24]; Hacker, 2008[25]; Stiglitz, Sen and Fitoussi, 2009[11]; Bossert and D’Ambrosio, 2013[26]; Boarini and Osberg, 2014[15]). In operational terms, economic insecurity is estimated as people’s exposure and vulnerability to the risk of unexpected, negative income shocks (Osberg, 1998[24]; Boarini and Osberg, 2014[15]). Most research measures observed (current or recent) economic insecurity based on prior experiences of income instability and predicts future risks (Hacker, Rehm and Schlesinger, 2013[27]; Hendren, 2017[28]; Rhem, 2016[29]). Such an approach uses widely available data on individual or household income and wealth, but requires the assumption that recent past experiences are a good approximation of the future risks of economic insecurity (Hacker, Rehm and Schlesinger, 2013[27]; Hendren, 2017[28]; Rhem, 2016[29]).
In contrast, perceived economic insecurity measures exposure to negative income shocks by using individuals’ view of their risk of income instability in the future – measured in terms of individuals’ worry or anxiety about the likelihood of an economic shock, such as the risk of job loss (Hacker, Rehm and Schlesinger, 2013[27]; Hendren, 2017[28]; Dominitz and Manski, 1996[30]; Green, Felstead and Burchell, 2000[31]; Mau, Mewes and Schöneck, 2012[32]; Nau and Soener, 2019[33]; Burgoon and Dekker, 2010[34]). This forward-looking approach has the benefit of capturing the uncertainty that is inherent in the concept of economic insecurity and encapsulates individuals’ own estimates of the risks they face, which cannot be done using measures of past experiences of income instability. However, information on people’s anxiety about their future incomes is rarely captured in international household income and wealth surveys. One exception is the Household Finance and Consumption Survey (HFCS), which includes a survey question asking respondents to estimate the precise numerical probability that they will lose their jobs in the near future.8
Given the availability of European surveys with information on income, wealth and perceptions, this chapter estimates the likelihood of currently or recently experiencing economic insecurity and the perception of experiencing economic insecurity in the near future in European OECD countries. To supplement the forward-looking approach taken in the chapter, the link between economic insecurity and the digitalisation of the labour market is also examined, which gives an indication of economic insecurity over the longer term (Box 2.2).
Box 2.2. Estimating current or recent economic insecurity using the HFCS and EU-SILC
This chapter cannot directly measure observed economic insecurity, because there is no single European data source that includes all requisite information – namely, monthly income/employment status and liquid asset holdings. Instead, this chapter predicts the chance of an individual experiencing economic insecurity using the Household Finance and Consumption Survey (HFCS) and the European Union Statistics on Income and Living Conditions (EU‑SILC).
The HFCS is used to estimate economic insecurity, because it contains rich information on individuals’ asset holdings and their perceptions of future income loss. However, the HFCS does not contain information on infra- and inter-annual income instability, which is why the EU‑SILC was used in Chapter 1. As a result, this chapter predicts who is likely to experience income instability in the HFCS based on their shared characteristics with people who experience income instability according to the EU‑SILC. Fortunately, the HFCS includes the same set of economic and socio-demographic variables used in the EU-SILC to predict income instability. Further, most of the predictors are coded equivalently and distributed similarly in both surveys.
In order to predict income instability in the HFCS, a statistical model is first estimated in the EU-SILC. The model – based on a Random Forests algorithm estimated separately for each country – predicts the probability of an individual’s total income instability being above a threshold (the top quartile for income instability, averaged across all countries). The model is then used to predict the probability of having an unstable income in the HFCS using the socio-economic characteristics that are common to both surveys, including household disposable income, which is predicted in the HFCS based on a machine learning approach (see Annex 2.A for further details).
Finally, individuals who have (predicted) highly unstable incomes are considered to be economically insecure if they do not have liquid assets to support themselves at the level of the OECD income poverty line – 50% of the national median – for at least three months. More information on the prediction model is in Annex 2.C.
2.4. Who has experienced economic insecurity and who is at risk in the future?
On average, in the European OECD countries studied, 17% of people in working-age households are estimated to be economically insecure, since their incomes are predicted to be highly unstable (in the top quartile for income instability, averaged across all countries) and they do not have sufficient liquid financial assets to support themselves at the level of the OECD income poverty line – 50% of the national median – for at least three months (Figure 2.4). Another 13% of people in working-age households have highly unstable incomes, but enough financial assets to cushion themselves (Figure 2.4). Despite their assets, however, having an unstable income can make it difficult for these people to save, which in turn can increase their risk of economic insecurity in the future.9
The extent of economic insecurity spans from 9% in the Slovak Republic and Austria to 25% in Latvia (Figure 2.4). In general, countries with high rates of economic insecurity tend to have both high levels of financial fragility (Figure 2.1) and higher-than-average levels of income instability (Figure 1.2) – except for Latvia, which has a medium level of income instability, but a very high rate of financial fragility, and Spain, which has a low level of financial fragility, but high income instability. Luxembourg and Germany, on the other hand, have low levels of economic insecurity, income instability and financial fragility.10 However, levels of income instability, financial fragility and economic insecurity do not always go hand-in-hand. For example, Italy has a medium level of income instability and below-average financial fragility. Despite this, Italy has a rate of economic insecurity that is higher than countries with more income instability and financial fragility, such as Estonia and Ireland – indicating that income instability and financial fragility are more likely to co-occur in Italy than in these other countries.
Figure 2.4. Almost one in six people in working-age households are economically insecure
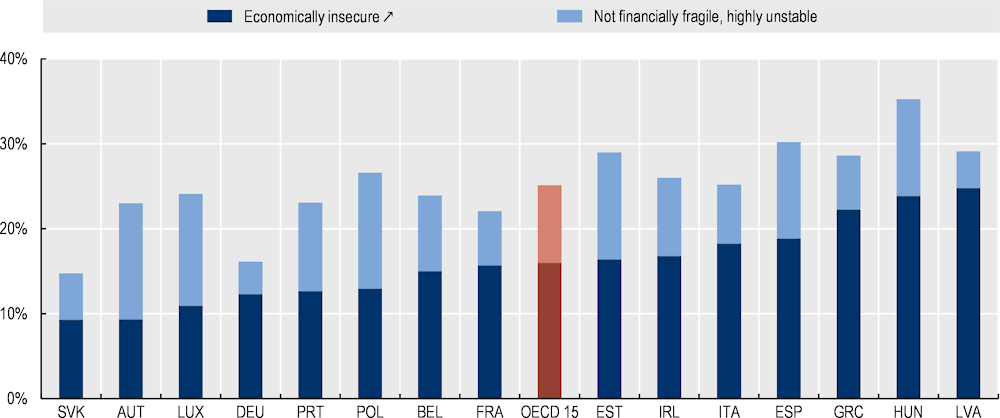
Note: The economically insecure are people living in working-age households who are likely to have highly unstable incomes and insufficient liquid assets to support them at the level of the OECD income poverty line for at least three months. Liquid assets represent those that can more easily be liquidated if needed urgently, including currency and deposits; bonds and debt securities; mutual funds and other investment funds; and other non-pension financial assets. The chart only shows countries for which information on both instability and fragility is available.
Source: OECD estimates based on the European Union Statistics on Income and Living Conditions (EU‑SILC), https://ec.europa.eu/eurostat/web/income-and-living-conditions; and the Eurosystem Household Finance and Consumption Survey (HFCS), https://www.ecb.europa.eu/stats/ecb_surveys/hfcs/html/index.en.html.
People who face the highest probability of experiencing economic insecurity are those who are in households with lower employment intensity, on average, across European OECD countries (Figure 2.5). This includes households with a single income earner, or where both adults lack job security (on temporary contracts or no contract at all). The probability of experiencing economic insecurity is also high for young couples and single, female‑headed households – reflecting gender imbalances in employment intensity. Women have lower participation rates and higher unemployment rates and are more likely than men to be in single‑income-earning households. Indeed, while 78% of working-age men in the sample are employed, the same is true for only 68% of women.
Figure 2.5. Economic insecurity is greatest in households with children and an intermittent attachment to the workforce
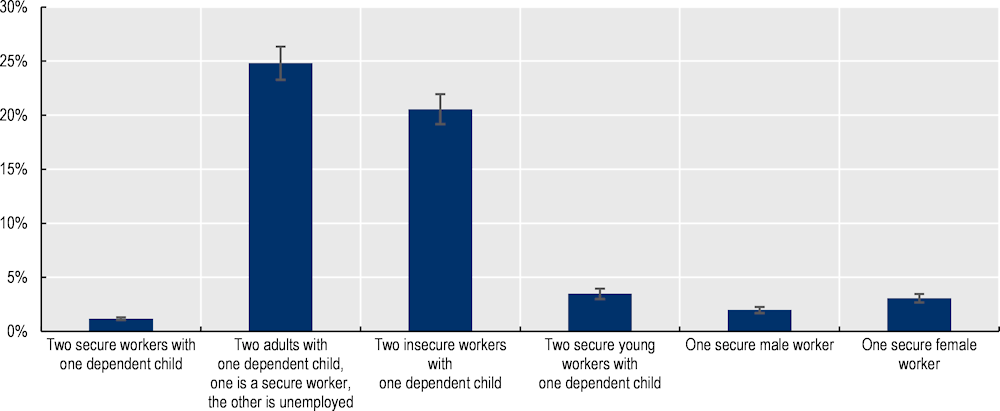
Note: The predictions are obtained using a logit model, which accounts for differences in educational level and household head age, family size, employment levels and job security. The sample includes Austria, Belgium, Estonia, France, Germany, Greece, Hungary, Ireland, Italy, Latvia, Luxembourg, Poland, Portugal, Spain and the Slovak Republic. Data are from 2016 for Italy and Poland; 2017 for Austria, Belgium, Estonia, France, Germany, Hungary, Latvia, Portugal and the Slovak Republic; 2018 for Greece, Ireland, Luxembourg and Spain. The term “secure worker” is used to refer to employees who have permanent contracts. “Insecure workers” have temporary or no contracts.
Source: OECD estimates based on the European Union Statistics on Income and Living Conditions (EU-SILC), https://ec.europa.eu/eurostat/web/income-and-living-conditions; and the Eurosystem Household Finance and Consumption Survey (HFCS), https://www.ecb.europa.eu/stats/ecb_surveys/hfcs/html/index.en.html.
While households with weaker attachments to the labour market along with young households are most likely to experience economic insecurity in Europe, the risks they face differ across countries (Figure 2.6). Individuals who have an unemployed person in their household face the highest chance of being economically insecure in Latvia, Hungary, Estonia, Belgium and Ireland, while workers who lack job security in Spain, Luxembourg, Poland, France, the Slovak Republic and Poland face relatively high risks (along with Latvia, Hungary, Belgium and Estonia). Belonging to a young household generally has a small effect across countries, except in Latvia.
Figure 2.6. The economic insecurity penalty associated with employment varies across countries
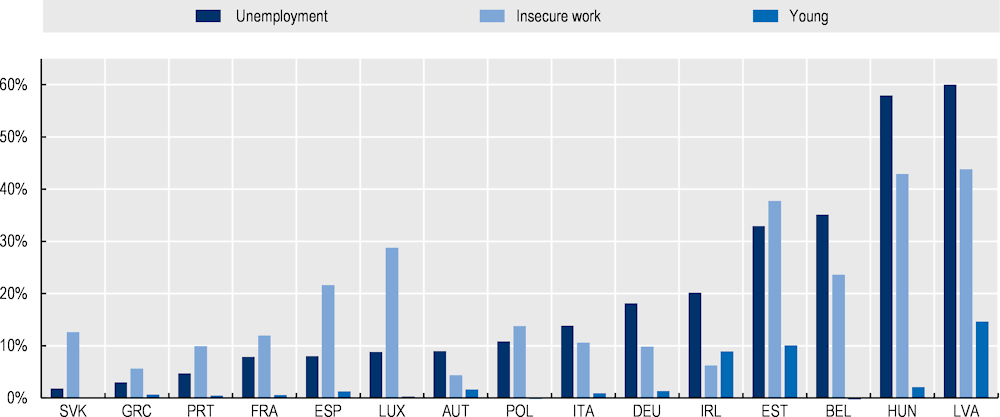
Note: The predictions are obtained using a logit model, which accounts for differences in educational level and household head age, family size, employment levels and job security. The sample includes Austria, Belgium, Estonia, France, Germany, Greece, Hungary, Ireland, Italy, Latvia, Luxembourg, Poland, Portugal, Spain and the Slovak Republic. Data are from 2016 for Italy and Poland; 2017 for Austria, Belgium, Estonia, France, Germany, Hungary, Latvia, Portugal and the Slovak Republic; 2018 for Greece, Ireland, Luxembourg and Spain.
Source: OECD estimates based on the European Union Statistics on Income and Living Conditions (EU-SILC), https://ec.europa.eu/eurostat/web/income-and-living-conditions; and the Eurosystem Household Finance and Consumption Survey (HFCS), https://www.ecb.europa.eu/stats/ecb_surveys/hfcs/html/index.en.html.
Who is at risk of economic insecurity in the future?
One in five people in European OECD countries believe they are at risk of economic insecurity in the near future – as they are financially fragile and perceive a high risk (50% or more chance) of losing their job in the next year.11 About 15% of people perceive that they are highly likely to experience income instability in the next year and currently have enough assets to stay out of poverty for at least three months (they are not financially fragile). Meanwhile, one-quarter of people are financially fragile but do not believe that they have a high chance of losing their job in the next twelve months – indicating that a substantial share of people who are financially fragile do not expect to experience income instability in the near future.
People who have experienced economic insecurity perceive themselves as facing higher risks of negative shocks in the near future compared to the economically secure – 68% of those who are economically insecure believe they have a high chance of losing their job in the next year compared to a quarter of economically secure people. Further, 30% of men perceive a high risk of losing their job in the near future compared to 34% of women, which reflects the latter’s weaker attachment to the labour market.
The relationship between lived experience of economic insecurity and anxiety about future economic insecurity plays out at the country level. There is a positive correlation between rates of economic insecurity and near‑term future risk in European OECD countries (Figure 2.7). Countries like Latvia and Greece, with high levels of economic insecurity, also have high levels of perceived insecurity, while Austria and Luxembourg have low levels of both. These results – coupled with the frequent changes in income that people experience (Chapter 1) – suggest that people in European OECD countries face repeated spells of economic insecurity, or at least they believe that economic insecurity is unlikely to be a one-off experience.
Figure 2.7. Economic insecurity is strongly correlated with perceived risk of unemployment over the next 12 months
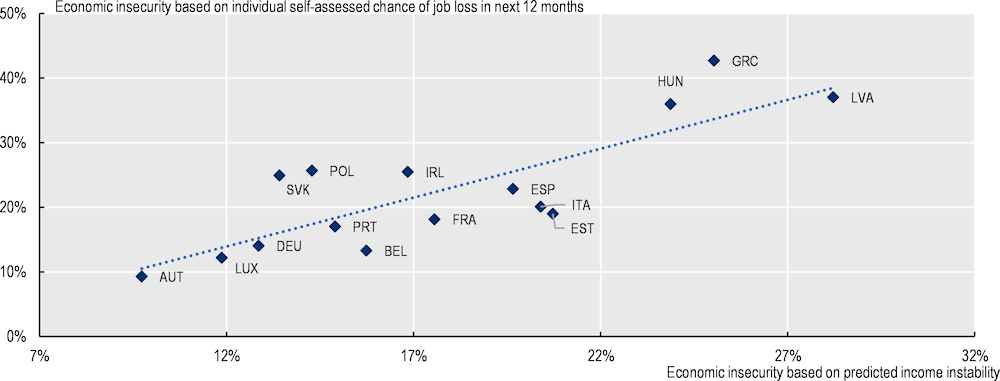
Note: Data are from 2016 for Italy and Poland; 2017 for Austria, Belgium, Estonia, France, Germany, Hungary, Latvia, Portugal and the Slovak Republic; 2018 for Greece, Ireland, Luxembourg and Spain.
Source: OECD estimates based on the European Union Statistics on Income and Living Conditions (EU-SILC), https://ec.europa.eu/eurostat/web/income-and-living-conditions; and the Eurosystem Household Finance and Consumption Survey (HFCS), https://www.ecb.europa.eu/stats/ecb_surveys/hfcs/html/index.en.html.
The risks of economic insecurity may persist beyond the next twelve months, as a consequence of the incipient technological changes in the labour market, such as the rise of artificial intelligence (AI) and other forms of automation (for example robotics). While firms’ AI adoption is still relatively low, the technologies are progressing rapidly, costs are falling and workers are increasingly developing AI skills – which indicates that OECD countries could be on the brink of an AI revolution (OECD, 2023[35]).
AI is making its greatest gains in non-routine and cognitive tasks – demarcating it from previous automation technologies. As such, occupations with lower rates of economic insecurity, such as professionals and managers, are more exposed to AI than workers who face higher risks of economic insecurity, including cleaners, food preparation assistants, labourers, rubbish collectors and other elementary workers (Figure 2.8, Panel A). A high exposure to AI does not necessarily translate to employment precarity or increase the risk of economic insecurity. AI has not (yet) led to a reduction in employment: some high‑skilled workers have had better employment prospects after the introduction of AI, and there is some evidence that those exposed to AI have seen their wages increase, as they spend more time on complex work tasks, while AI undertakes simpler tasks (OECD, 2023[35]).
In contrast, occupations with higher rates of economic insecurity face relatively high risks from automation more broadly (OECD, 2023[35]). At-risk occupations tend to have fewer skills that are difficult to automate (so-called bottleneck skills), which places them at greater risk of job loss – although, even for occupations at high risk of automation, only a moderate amount (15-25%) of important skills and abilities are automatable (Lassébie and Quintini, 2022[36]).
Some workers with a high risk of automation, such as those in elementary occupations, face the extra burden of having few viable and desirable job transitions12 they can make in the event they lose their jobs (as demonstrated by the dark red bubble in Panel B of Figure 2.8). While craft workers, plant and machine operators, and assemblers face high automation risks and have a medium rate of economic insecurity, they have the greatest number of viable and desirable jobs they can transition to (represented by the dark blue bubbles), which indicates they have the best opportunities to move into new roles if their current occupations are automated. In effect, these workers may not see their rates of economic insecurity rise markedly, even though they are in highly automatable roles.
Figure 2.8. Workers in occupations that are exposed to economic insecurity are less likely to reap the benefits of AI and are more at risk of automation than workers in more secure occupations
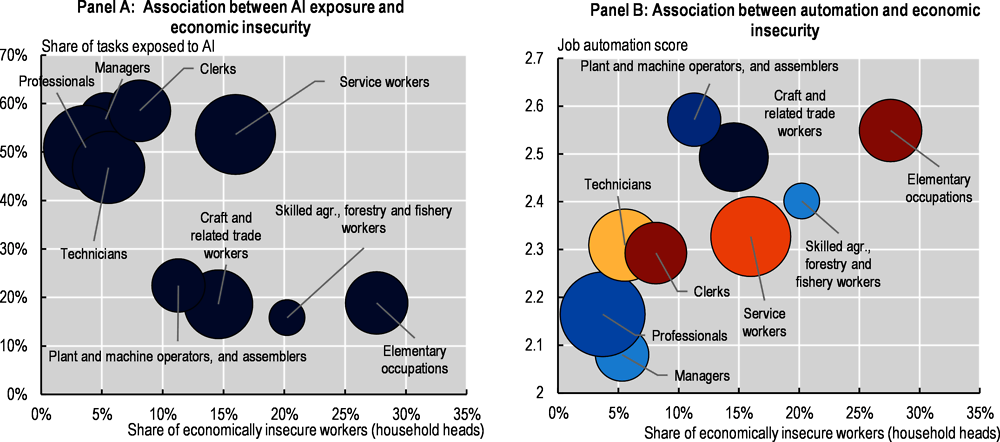
Note: Exposure to artificial intelligence (AI) is based on Kanders et al (2020[37]), who map the suitability for machine learning scores developed by Brynjolfsson, Mitchell and Rock (2018[38]) to thousands of tasks in European countries. Exposure is measured as the share of tasks that are not bottlenecks to AI. Automation risk is based on Lassébie and Quintini (2022[36]), who surveyed experts on the degree of automatability for 98 skills and abilities. The risk of automation is then calculated for each occupation as the average rating for each skill or ability used in the occupation across all expert responses weighted by the skills or abilities’ importance in the occupation as rated by O*NET. Finally, the scores are applied to European Skills, Competencies, and Occupations in the HFCS using the Kanders et al. (2020[37]) crosswalk. Scale is 0-5 for all occupations. Each bubble’s size indicates the share of workers in a given occupation. In Panel B, bubbles are coloured based on the number of highly viable and desirable job transitions that are likely in each occupation group – ranging from dark red (5 transitions), to orange (7), yellow (8), light blue (10), medium blue (11), and finally dark blue (19-22). Highly viable transitions are those with a good fit for an individual’s current occupation (based on their education, skills, experience and types of work activities), while desirable transitions are those where the job mover can make at least 75% of their current occupation’s median earnings (Kanders et al., 2020[37]). Unweighted average of Austria, Belgium, Estonia, France, Germany, Greece, Ireland, Italy, Latvia, Luxembourg, Poland, Portugal, the Slovak Republic and Spain.
Source: Kanders et al (2020[37]), Lassébie and Quintini (2022[36]) and OECD estimates based on the European Union Statistics on Income and Living Conditions (EU-SILC), https://ec.europa.eu/eurostat/web/income-and-living-conditions; and the Eurosystem Household Finance and Consumption Survey (HFCS), https://www.ecb.europa.eu/stats/ecb_surveys/hfcs/html/index.en.html.
Taken together, these results indicate that people who currently face high risks of economic insecurity tend to be in occupations that are more likely to be negatively affected by the digital transition. They may be less likely to reap the gains of AI, while facing higher risks of automation, and in some cases, with fewer options for job transitions. If such risks were to precipitate, people who are currently economically insecure may have difficulty escaping in the future. This underscores the need for policies to help people reduce their risks of economic insecurity by smoothing their incomes and imbuing them with the financial literacy necessary to keep their finances under control, manage their expenses and plan to weather potential future shocks.
This chapter also shows that financial fragility and economic insecurity are distinct, although intertwined, phenomena. While financial fragility affects nearly half of the middle-income population in European OECD countries, and a large share of higher-income earners, these groups are much less likely to experience (or be at risk of) economic insecurity than lower-income earners. This is because lower-income earners, such as the unemployed and workers who lack job security, are more often subjected to high income instability (Chapter 1). Conversely, more than one in ten people with highly unstable incomes have sufficient assets to avoid financial fragility – which indicates that they can smooth their incomes, at least in the short term. Thus, by examining each element of economic insecurity in isolation and as an ensemble, this chapter has been able to identify different at-risk groups.
While the novel approach used in this report has shed new light on the extent and risks of income instability and economic insecurity in European OECD countries, it is possible to extend the analysis to examine other forms of instability, such as family breakdowns, and the effects of economic insecurity. For example, the effects of experiencing economic insecurity on a range of well-being outcomes, such as health, housing insecurity, social exclusion and investments in education, could be analysed using the data sources and methods in this report. Focusing on the effects of economic insecurity in European OECD countries would close a gap in the research literature, as the majority of studies examine the effects of economic insecurity only in the United States. Further, such analysis could lead to new insights for health, education and labour market policies. Nevertheless, this report’s analysis highlights the pressing need for a range of policies that support people at risk of income instability and/or financial fragility. The next chapter considers a selection of such policy options.
References
[22] Argente, D. and M. Lee (2020), “Cost of Living Inequality During the Great Recession”, Journal of European Economic Association, Vol. 19/2, pp. 913-952, https://doi.org/10.1093/jeea/jvaa018.
[7] Avram, S. et al. (2019), “Household income volatility in the UK, 2009-2017”, Understanding Society Working Paper Series, No. 2019-07, https://www.understandingsociety.ac.uk/sites/default/files/downloads/working-papers/2019-07.pdf.
[23] Balestra, C. and F. Oehler (2023), “Measuring the joint distribution of household income, consumption and wealth at the micro level”, OECD Papers on Well-being and Inequalities, No. 11, OECD Publishing, Paris, https://doi.org/10.1787/f9d85db6-en.
[18] Balestra, C. and R. Tonkin (2018), “Inequalities in household wealth across OECD countries: Evidence from the OECD Wealth Distribution Database”, OECD Statistics Working Papers, No. 2018/01, OECD Publishing, Paris, https://doi.org/10.1787/7e1bf673-en.
[12] Bhargava, V. and J. Lown (2006), “Preparedness for Financial Emergencies: Evidence from the Survey of Consumer Finances”, Association for Financial Counseling and Planning Education, pp. 17- 26, https://ssrn.com/abstract=2232126.
[39] Blanchet, T., L. Chancel and A. Gethin (2019), “How Unequal Is Europe? Evidence from Distributional National Accounts, 1980-2017”, WID.world Working Paper Series, No. 2019/06, https://wid.world/document/bcg2019-full-paper/.
[15] Boarini, R. and L. Osberg (2014), “Economic Insecurity: Editors’ Introduction”, Review of Income and Wealth, Vol. 60/S1, pp. S1-S4, https://doi.org/10.1111/ROIW.12118.
[26] Bossert, W. and C. D’Ambrosio (2013), “Measuring economic insecurity”, International Economic Review, Vol. 54/3, pp. 1017-1030, https://doi.org/10.1111/IERE.12026.
[38] Brynjolfsson, E., T. Mitchell and D. Rock (2018), “What can machines learn, and what does it mean for occupations and the economy?”, AEA Papers and Proceedings, Vol. 108, pp. 43-47, https://www.aeaweb.org/articles?id=10.1257/pandp.20181019.
[34] Burgoon, B. and F. Dekker (2010), “Flexible employment, economic insecurity and social policy preferences in Europe:”, Journal of European Social Policy, Vol. 20/2, pp. 126-141, https://doi.org/10.1177/0958928709358789.
[16] Demertzis, M., M. Domínguez-Jiménez and A. Lusardi (2020), The Financial Fragility of European Households in the Time of COVID-19, Bruegel, https://www.bruegel.org/2020/07/the-financial-fragility-of-european-households-in-the-time-of-covid-19/ (accessed on 15 June 2022).
[30] Dominitz, J. and C. Manski (1996), “Perceptions of economic insecurity: Evidence from the Survey of Economic Expectations”, NBER Working Papers, No. 5690, National Bureau of Economic Research, https://ideas.repec.org/p/nbr/nberwo/5690.html (accessed on 15 June 2022).
[31] Green, F., A. Felstead and B. Burchell (2000), “Job insecurity and the difficulty of regaining employment: An empirical study of unemployment expectations”, Oxford Bulletin of Economics and Statistics, Vol. 62/s1, pp. 855-883, https://doi.org/10.1111/1468-0084.0620s1855.
[6] Guiso, L. et al. (2020), “Economic insecurity and the demand of populism in europe”, Einaudi Institute for Economics and Finance, http://www.heliosherrera.com/populism.pdf.
[1] Hacker, J. (2018), “Economic security”, in For Good Measure: Advancing Research on Well-being Metrics Beyond GDP, OECD Publishing, Paris, https://doi.org/10.1787/9789264307278-10-en.
[25] Hacker, J. (2008), The Great Risk Shift: The New Economic Insecurity and the Decline of the American Dream, New York: Oxford University Press.
[5] Hacker, J. and E. Jacobs (2008), The Rising Instability of American Family Incomes, 1969-2004: Evidence from the Panel Study of Income Dynamics, Economic Policy Institute.
[27] Hacker, J., P. Rehm and M. Schlesinger (2013), “The insecure American: Economic experiences, financial worries, and policy attitudes”, Perspectives on Politics, Vol. 11/1, pp. 23-49, https://doi.org/10.1017/S1537592712003647.
[28] Hendren, N. (2017), “Knowledge of future job loss and implications for unemployment insurance”, American Economic Review, Vol. 107/7, pp. 1778-1823, https://doi.org/10.1257/aer.20151655.
[20] Household Finance and Consumption Network (2023), Household Finance and Consumption Survey: Results from the 2021 wave, https://doi.org/10.2866/31818.
[37] Kanders, K. et al. (2020), Mapping Career Causeways: Supporting Workers At Risk, https://media.nesta.org.uk/documents/Mapping_Career_Causeways_01_G2XA7Sl.pdf.
[36] Lassébie, J. and G. Quintini (2022), “What skills and abilities can automation technologies replicate and what does it mean for workers?: New evidence”, OECD Social, Employment and Migration Working Papers, No. 282, OECD Publishing, Paris, https://doi.org/10.1787/646aad77-en.
[13] Mansour, F. (2018), “Economic Insecurity and Fertility: Does Income Volatility Impact the Decision to Remain a One-Child Family?”, Journal of Family and Economic Issues, Vol. 39/2, pp. 243-257, https://doi.org/10.1007/S10834-017-9559-Y/TABLES/8.
[32] Mau, S., J. Mewes and N. Schöneck (2012), “What determines subjective socio-economic insecurity? Context and class in comparative perspective”, Socio-Economic Review, Vol. 10/4, pp. 655-682, https://doi.org/10.1093/SER/MWS001.
[17] McKnight, A. and M. Rucci (2020), “The financial resilience of households: 22 country study with new estimates, breakdowns by household characteristics and a review of policy options”, CASE Papers, No. 219, Centre for Analysis of Social Exclusion, LSE, https://ideas.repec.org/p/cep/sticas/-219.html.
[33] Nau, M. and M. Soener (2019), “Income precarity and the financial crisis”, Socio-Economic Review, Vol. 17/3, pp. 523-544, https://doi.org/10.1093/SER/MWX020.
[35] OECD (2023), OECD Employment Outlook 2023: Artificial Intelligence and the Labour Market, OECD Publishing, Paris, https://doi.org/10.1787/08785bba-en.
[3] OECD (2023), Worries About Affording Essentials in a High-inflation Environment, https://www.oecd.org/els/soc/OECD2023-RTM2022-PolicyBrief-Inflation.pdf.
[4] OECD (2022), OECD Employment Outlook 2022: Building Back More Inclusive Labour Markets, OECD Publishing, https://doi.org/10.1787/1bb305a6-en.
[2] OECD (2021), “Inequalities in household wealth and financial insecurity of households”, OECD Policy Insights on Well-being, Inclusion and Equal Opportunity, No. 2, OECD Publishing, Paris, https://doi.org/10.1787/b60226a0-en.
[19] OECD (2020), How’s Life? 2020: Measuring Well-being, OECD Publishing, Paris, https://doi.org/10.1787/9870c393-en.
[40] OECD (2019), Under Pressure: The Squeezed Middle Class, OECD Publishing, Paris, https://doi.org/10.1787/689afed1-en.
[21] OECD (n.d.), OECD Papers on Well-being and Inequalities, OECD Publishing, Paris, https://doi.org/10.1787/4ca48f7c-en.
[24] Osberg, L. (1998), Economic Insecurity, Discussion Papers 0088, University of New South Wales, Social Policy Research Centre, https://ideas.repec.org/p/wop/sprcdp/0088.html.
[10] Reeves, A., M. McKee and D. Stuckler (2014), “Economic suicides in the Great Recession in Europe and North America”, The British Journal of Psychiatry: The Journal of Mental Science, Vol. 205/3, pp. 246-247, https://doi.org/10.1192/BJP.BP.114.144766.
[29] Rhem, P. (2016), Risk Inequality and Welfare States: Social Policy Preferences, Development, and Dynamics, Cambridge University Press, Cambridge, MA.
[8] Rohde, N. et al. (2017), “Is it vulnerability or economic insecurity that matters for health?”, Journal of Economic Behavior & Organization, Vol. 134, pp. 307-319, https://doi.org/10.1016/J.JEBO.2016.12.010.
[9] Smith, T., C. Stoddard and M. Barnes (2007), “Why the Poor Get Fat: Weight Gain and Economic Insecurity”, SSRN Electronic Journal, https://doi.org/10.2139/SSRN.979189.
[11] Stiglitz, J., A. Sen and J. Fitoussi (2009), Report by the Commission on the Measurement of Economic Performance and Social Progress, Commission on the Measurement of Economic Performance and Social Progress, http://ec.europa.eu/eurostat/documents/118025/118123/Fitoussi+Commission+report (accessed on 3 July 2018).
[14] Stoetzer, L., J. Giesecke and H. Klüver (2021), “How does income inequality affect the support for populist parties?”, The Journal of European Public Policy, Vol. 30/1, pp. 1-20, https://doi.org/10.1080/13501763.2021.1981981.
Annex 2.A. Methodology used for estimating disposable incomes in the HFCS
The Household Finance and Consumption Survey (HFCS), the primary source of microdata on households’ wealth holdings in European countries, provides information on market incomes (i.e. incomes before taxes and transfers) and social benefit receipt, but not on taxes and social insurance contributions paid (except for Finland and Italy). Jointly analysing wealth holdings and disposable incomes of European countries is therefore not directly possible. It is also not possible to study the wealth holdings of (income) poor households, as poverty is traditionally defined with reference to the median equivalised disposable household income.
To address this limitation, this chapter makes use of novel methods in statistical matching to impute disposable income from the gross income variable available in the HFCS dataset. To do so, a predicted distribution of disposable income in each country and year was derived, using ensemble methods of machine learning. The model was trained on the EU Statistics on Income and Living Conditions (EU-SILC) micro-dataset data from the corresponding income years 2010 to 2018, which contains reliable information on both disposable and gross income and for which a large set of socio-demographic variables were harmonised to match those in the HFCS dataset. The distribution of gross income is very similar between the HFCS and EU-SILC, although incomes tend to be higher at the top in the HFCS, which is likely due to oversampling.
The main challenge of the statistical matching exercise was to find a well-functioning approach that could allow the predicted disposable income to be a function of the entire gross income distribution and an array of socio-demographic variables. A machine learning approach was preferred over other viable options (e.g. hot-deck imputations) not only because it retained better predictive power, but also because it did not require assumptions about the nature of the relationship between disposable income, gross income and socio-demographic characteristics.
The machine learning method chosen, called “Xgboost”, relies on boosted regression trees. It has become widely acknowledged for its very good performance and has recently been used in Blanchet, Chancel and Gethin (2019[39]) for a very similar matching exercise in which the authors match different income concepts together in multiple datasets over a relatively long time period.
Similarly to Blanchet, Chancel and Gethin (2019[39]), both the donor (EU-SILC) and recipient (HFCS) samples were harmonised, and the distribution of both gross and disposable income was segmented into percentiles. As the models are in a Gaussian (or continuous) form, the predicted disposable income distribution was defined in a continuous space despite the fact that the main predictor, gross income, was restricted to percentile levels. Other predictors included: household type, marital status, weekly number of hours worked for the household head and the second household head (when applicable), labour status for the household head and the second household head (when applicable), occupation type for the household head and the second household head (when applicable), and variables decomposing individual gross income for both household heads. Following good practice, first all input variables were standardised, then the model output was de-standardised by applying the same scaling factor used for standardisation. Cross-validation was also used to reduce the risk of overfitting. Finally, we decided to top-code gross and disposable income variables when training the model, as it significantly increased performance between the 1st and the 99th percentile. All observations above the 99th percentile were given the value of the 99th percentile. The model was also tested by gathering all available waves for each country separately; however, the results obtained by restricting the predictions to each country and wave were more satisfactory. As the HFCS dataset contains five different imputations, after training the model, predicted income was matched on all five imputations separately.
For each country and year, EU-SILC observations were split into a training sample (75%) and a testing sample (25%). The model was then run separately for each country and year, and the results were assessed on the testing sample. For those countries where information on disposable income is available in the HFCS, i.e. Italy and Finland, the model’s performance was evaluated by comparing the predicted and observed distributions. The model can be summarised with the following specification:
In each wave and country, the predicted disposable income percentiles are therefore an arbitrary function of all gross income percentiles until the 99th, and of the socio-demographic and income covariates listed above.
The results from the machine learning model are highly satisfactory. The mean cross-validation prediction errors across years and countries remain below 7% at the very most, and the mean squared error on the test sample does not go past 4% of a standard deviation in most countries. For those countries where information on disposable income is available in the HFCS, i.e. Italy and Finland, the model’s performance was evaluated by comparing the predicted and the observed distributions. Figure 2.A.1 compares the distribution of predicted and actual household disposable income (at the individual level) for both countries. The model performs rather well along the entire distribution bar at the very top end (top 1%). The discrepancy at the top is caused by the top-winsorisation that was implemented to improve the general performance of the matching algorithm. This, however, does not represent a major source of concern for the analysis in this chapter, since the prevalence of financial fragility at the very top of the income distribution is almost negligible.
Figure 2.A.1. Kernel density plots of predicted and actual equivalised household disposable income for Finland and Italy
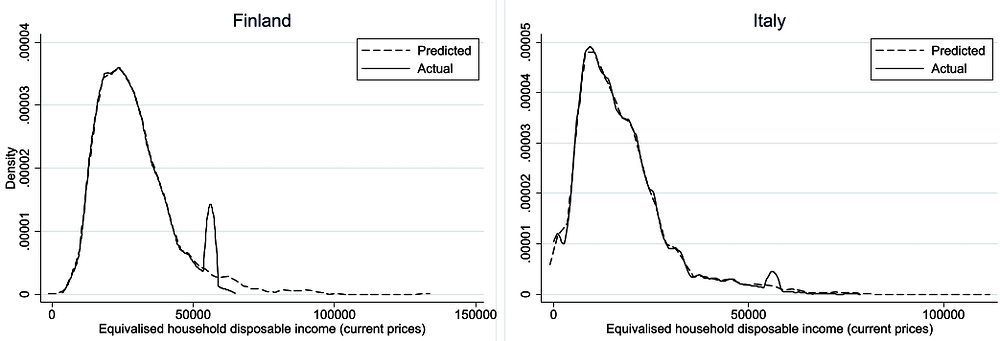
Source: OECD calculations based on the European Union Statistics on Income and Living Conditions (EU-SILC), https://ec.europa.eu/eurostat/web/income-and-living-conditions; and the Eurosystem Household Finance and Consumption Survey (HFCS), https://www.ecb.europa.eu/stats/ecb_surveys/hfcs/html/index.en.html.
Annex 2.B. Odds ratios of the logit model
Annex Table 2.B.1. Full logit results
Dependent variable: Financial fragility |
Odds ratios |
---|---|
Age: 35-49 |
0.83*** |
(0.03) |
|
Age: 50-59 |
0.96 |
(0.04) |
|
Lower income |
2.27*** |
(0.08) |
|
Higher income |
0.27*** |
(0.02) |
|
Born outside Europe |
1.64*** |
(0.10) |
|
Any substantial gift or inheritance received |
0.60*** |
(0.02) |
|
Over-indebtedness |
1.61*** |
(0.06) |
|
Number of household members in employment |
0.89*** |
(0.02) |
|
Upper secondary education |
0.52*** |
(0.02) |
|
Tertiary education |
0.24*** |
(0.01) |
|
One adult, at least one child |
1.69*** |
(0.12) |
|
Two or more adults, no children |
1.22*** |
(0.05) |
|
Two or more adults, one or two children |
1.62*** |
(0.08) |
|
Two or more adults, at least three children |
3.05*** |
(0.19) |
|
Female |
1.16*** |
(0.03) |
|
Main income Source: Current transfers |
1.64*** |
(0.07) |
|
Main income Source: Property income |
0.36*** |
(0.05) |
|
Main income Source: Self-employment income |
0.68*** |
(0.05) |
|
Main residence: Partially owned |
1.32** |
(0.16) |
|
Main residence: Rented |
2.82*** |
(0.10) |
|
Labour status: Sick/maternity leave |
1.16 |
(0.15) |
|
Labour status: Unemployed |
1.45*** |
(0.08) |
|
Labour status: Student/pupil/unpaid intern |
0.49*** |
(0.05) |
|
Labour status: Retiree or early retiree |
0.79*** |
(0.07) |
|
Labour status: Permanently disabled |
1.11 |
(0.10) |
|
Labour status: Compulsory military service or equiv. social service |
1.61 |
(0.48) |
|
Labour status: Domestic tasks |
1.29*** |
(0.11) |
|
Constant |
0.20*** |
(0.02) |
|
Observations |
171,629 |
Note: Robust s.e. in parentheses. *** p<0.01, ** p<0.05, * p<0.1
Source: OECD computations from the Eurosystem Household Finance and Consumption Survey (HFCS), https://www.ecb.europa.eu/stats/ecb_surveys/hfcs/html/index.en.html.
Annex 2.C. Predicting income instability in the HFCS
The analysis on economic insecurity in this chapter combines information about financial fragility from the Household Finance and Consumption Survey (HFCS) with information on income instability from EU Statistics on Income and Living Conditions (EU-SILC). The HFCS dataset is augmented with a variable indicating whether an individual is predicted to be in the top quartile in terms of income instability, based on the squared coefficient of variation of monthly income described in Chapter 1. The prediction is carried out with a country-specific algorithm estimated (or “trained”) on the EU-SILC dataset, where income instability is observed. This Annex describes the methodological details of the prediction procedure.
The algorithm only keeps individual variables for the household head along with household variables, and only considers households whose household head is aged below 60 both in the HFCS and in the first year of the four-year panel in the EU-SILC. The household head is defined as the person with the highest individual employment income in the (first) year of the survey (panel). Only households with stable composition – with nobody leaving or entering the household (including no new-born children) – over four years are kept from the panels in the EU-SILC.
The predictions are based on the set of variables that are expected to be relevant in predicting the probability of having income instability above the selected threshold (the average 75th percentile of the distribution across the included countries) and are consistently reported in both the EU-SILC and the HFCS. Most of the predictors go through a rigorous process of transformation and harmonisation aimed at making them equivalently coded and similarly distributed in the two surveys. Annex Table 2.C.1 describes each predictor and, if applicable, reports whether any transformation has been applied.
Annex Table 2.C.1. Variables used as predictors for the algorithm
Type of predictors |
Name of variable |
Transformations |
---|---|---|
Household economic situation |
Total household gross income |
Applying square root equivalence scale; convert to constant euros, if needed |
Current rent related to occupied dwelling |
Applying square root equivalence scale; convert to constant euros, if needed |
|
Household head individual variables |
Age of the household head |
|
Sex of the household head |
||
Household socio-demographic predictors |
Household size |
Censored at 11, meaning that household with more than 10 members are recoded to 11 |
Share of workers |
Number of workers divided by uncensored household size. Variable defining occupational status is recoded for comparability |
|
Share of unemployed |
Number of unemployed divided by uncensored household size. Variable defining occupational status is recoded for comparability |
|
Share of students |
Number of students divided by uncensored household size. Variable defining occupational status is recoded for comparability |
|
Share of retired |
Number of retired divided by uncensored household size. Variable defining occupational status is recoded for comparability |
|
Share of female workers |
Number of female workers divided by uncensored household size. Variable defining occupational status is recoded for comparability |
|
Share of secured workers |
Number of workers with long-term contract divided by uncensored household size. Variable defining job contracts is recoded for comparability |
|
Share of young workers |
Number of young workers divided by uncensored household size. Variable defining occupational status is recoded for comparability |
|
Share with primary education or below |
Number of individuals with primary education or below divided by uncensored household size. Variable defining education is recoded for comparability |
|
Share with secondary education |
Number of individuals with secondary education divided by uncensored household size. Variable defining education is recoded for comparability |
|
Share with tertiary education or above |
Number of individuals with tertiary education or above divided by uncensored household size. Variable defining education is recoded for comparability |
|
Share of children younger than 16 |
Number of children younger than 16 divided by uncensored household size |
|
Share of children younger than 3 |
Number of children younger than 3 divided by uncensored household size |
|
Presence of married person in the household |
Dummy equal to 1 if there is at least one married person in the household. Variable defining marital status is recoded for comparability |
When missing values prevent the count of members with certain characteristics from being reliable (for example, an adult having missing employment status leads to an unreliable count for the share of workers), then the related variable (such as the share of workers) is set to missing, regardless of what is reported for the other household members.
As for the classification algorithm, an appropriate choice for the classification problem is the Random Forest algorithm. Three parameters require optimisation to run the algorithm. They are (partially) optimised on a three-dimensional (discrete) grid minimising the out-of-bag misclassification rate. The number of trees is the best in a grid from 100 to 1 500, with a pace of 50. The number of variables to use at each split is the best between the integers from 1 to 15 (the number of predictors). The action to be taken on missing values is the best between omitting the household with missing values or imputing with the median.
Random forest is trained on two pooled waves of the EU-SILC (2018 and 2019, so that the model is trained on a period of time similar to the HFCS) and tested for overfitting on the two previous waves (2016 and 2017). In the EU-SILC, monthly income instability is measured by the squared coefficient of variation of monthly household market income and defines the dummy reporting which households belong to the high instability group, as previously defined.
The evaluation of the prediction quality is performed on the test sample. A crisp assignment to the highest quartile is also done using a cut-off that ensures the share of 1 in the test set is to be approximately equal to the true share of 1 in the training set. Annex Table 2.C.2 summarises the main indicators of classification performance for each country, i.e. the misclassification rate in the test set as compared to the expected error rate of the random classifier, and the area under the receiving operator curve (AUC). These indicators demonstrate the good performance of the algorithm in most countries: the misclassification rates range from 15% to 25%, which is always far below the random classifier expected error rates, and the AUC ranges between 0.77 and 0.89.
The final prediction on the HFCS can be performed only on individuals belonging to households without missing information. This leads to a dataset with merged information consisting of almost 102 000 individuals, with each country counting from more than 2 000 (Latvia) to more than 24 000 (France) individuals. Individuals with high income instability on the HFCS are selected as those with the highest predicted probability, in a number such that, for each country, the share of predicted highly unstable in the HFCS is equal to the share of observed highly unstable in the training sample in the EU‑SILC.
Annex Table 2.C.2. Performance of the classification algorithm
Error rate in the test set, expected error rate of the random classifier and Area Under receiving operator Curve (where an area score closer to 1 implies a better prediction)
Country |
AUT |
BEL |
DEU |
ESP |
EST |
FRA |
GRC |
HUN |
IRL |
ITA |
LUX |
LVA |
POL |
PRT |
SVK |
---|---|---|---|---|---|---|---|---|---|---|---|---|---|---|---|
Error rate in test set |
0.22 |
0.17 |
- |
0.21 |
0.24 |
0.16 |
0.28 |
0.24 |
0.23 |
0.21 |
0.19 |
0.26 |
0.25 |
0.22 |
0.18 |
Expected error rate of the random classifier |
0.35 |
0.35 |
0.27 |
0.42 |
0.41 |
0.34 |
0.41 |
0.46 |
0.38 |
0.38 |
0.36 |
0.41 |
0.38 |
0.35 |
0.26 |
AUC |
0.79 |
0.86 |
0.85 |
0.84 |
0.79 |
0.85 |
0.73 |
0.8 |
0.77 |
0.79 |
0.79 |
0.77 |
0.75 |
0.78 |
0.69 |
Note: The error rate in the test set is the share of misclassified individuals in the test set. Expected error rate of the random classifier is the share of misclassified individuals that are expected if the algorithm randomly classifies the individuals in the two classes (0 and 1) with the constraint of reproducing their true known proportions. AUC stands for Area Under receiving operator Curve, it usually takes values between 0.5 (random classification) and 1 (perfect classification); compared to the error rate, it evaluates classification performance at different values of the probability cut-off.
Notes
← 1. In this report, analysis is based on changes in nominal, rather than real, income that are the result of employment shocks in the years leading up to the COVID-19 pandemic. Before the pandemic and the cost-of-living crisis, differences between real and nominal incomes were negligible and so are unlikely to influence the results presented.
← 2. The OECD Risks that Matter survey (2023[3]) covered the following European Union countries: Austria, Belgium, Denmark, Estonia, Finland, France, Germany, Greece, Ireland, Italy, Latvia, Lithuania, the Netherlands, Poland, Portugal, Slovenia and Spain.
← 3. In previous OECD work, financial fragility has also been termed financial vulnerability (Balestra and Tonkin, 2018[18]) or financial insecurity (OECD, 2021[2]).
← 4. Liquid assets can be more easily converted into cash if needed urgently, and include currency and deposits, bonds and other debt securities, mutual funds and other investment funds, and other non-pension financial assets. This chapter uses the terms liquid assets and financial buffers interchangeably.
← 5. Similar to Chapter 1, this chapter examines prime-age households where the household head is aged 18-59 at the beginning of the period. To aid readability, working-age household is used instead of prime working-age household and working-age household.
← 6. For the remainder of this chapter, the third wave of the Household Finance and Consumption Survey (HFCS) from 2017 is used to assess financial fragility and economic insecurity, even though the fourth wave from 2022 is available. The third wave of the HFCS maps more closely to the EU‑SILC data from 2013-2019, which was used to estimate income instability. Using data that were collected during, or immediately following, COVID‑19 is unlikely to give a realistic indication of the long-run, structural levels of income instability that countries could return to as economies recover.
← 7. In line with OECD (2019[40]), this chapter groups people by whether they are in working-age households with disposable income less than 75% of the median national income (lower income), or in working-age households with disposable income between 75% and 200% of the median national income (middle income), or in working-age households with disposable income above 200% of the median national income (higher income). Working-age households are those headed by someone aged 18 to 59.
← 8. The OECD Risks That Matter Survey (2023[3]) includes questions about people’s concerns about various economic risks in the next year, including their risk of losing their job. However, the Risks That Matter Survey does not ask people about their household’s assets, which means the survey cannot be used to measure economic insecurity as the combination of income instability and financial fragility.
← 9. This section uses the terms “estimated economic insecurity” and “economic insecurity” interchangeably to refer to estimates of economic insecurity based on the predictions described in Box 2.2. These terms are used in contrast to “near future risk of economic insecurity”, which is based on individuals’ perceptions of their chance of losing their job in the next year.
← 10. A complete analysis of countries with low levels of income instability is not possible because some of the European countries covered in Chapter 1 are not included in the Household Finance and Consumption Survey. This includes Czech Republic, Switzerland, Norway, Slovenia and the United Kingdom.
← 11. The near future risk of economic insecurity is estimated in terms of individuals’ perceptions of their risk of losing their job in the next twelve months. People who stated in the Household Finance and Consumption Survey (HFCS) that they believed they face a 50% or greater risk of losing their job in the next year were identified as having perceived highly unstable incomes. Those who are perceived to be economically insecure are financially fragile and expect that they are highly likely to lose their job (and hence experience a fall in their income) in the near future.
← 12. Viable transitions are those that are a good fit for workers in terms of their skills, education and experience; the types of work activities; and the interpersonal, physical and structural aspects of work. Desirable transitions have similar expected earnings to a person’s current occupation (Kanders et al., 2020[37]).