Chapter 2 examines the volatility of tax revenues in OECD countries, with a specific focus on tax buoyancy. Using the unique Revenue Statistics dataset, it provides estimates of short- and long-run tax buoyancy in the OECD for total tax revenues and the main tax types between 1980 and 2021, and analyses how buoyancy has evolved over this period. It also examines short-run buoyancy over the business cycle and the impact of factors such as inflation and population ageing on tax buoyancy. Detailed country-level buoyancy estimates are included in an Annex.
Revenue Statistics 2023
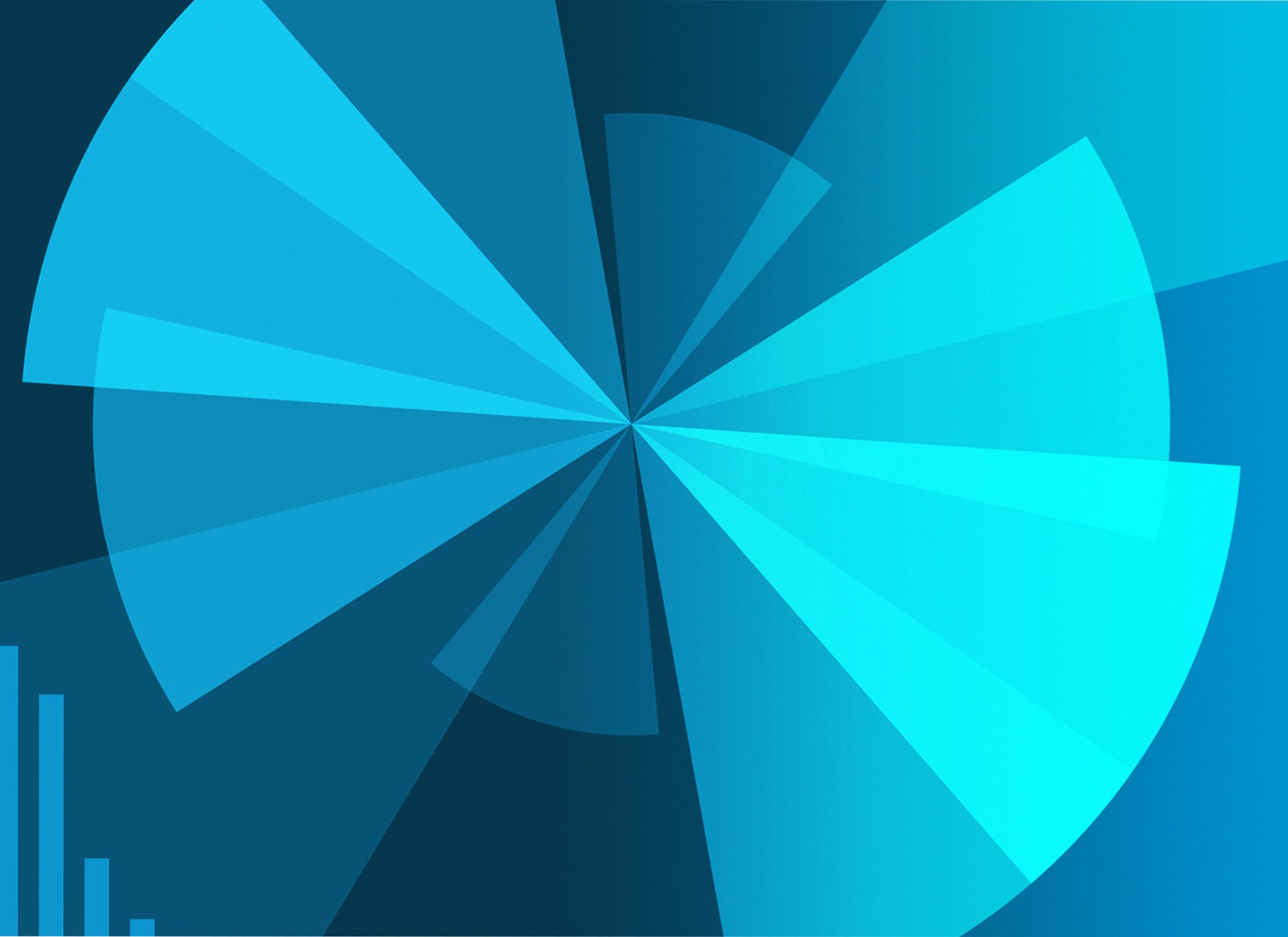
2. Tax revenue buoyancy in OECD countries
Abstract
Introduction
Since the OECD began publishing the Revenue Statistics report, tax-to-GDP ratios have gradually risen in most OECD countries. However, as discussed in Chapter 1, there have been numerous downturns in revenues across this period, some of which have been related to major external events including the oil shock, the Global Financial Crisis and, most recently, the COVID-19 pandemic. These downturns have affected different taxes in different ways.
This Special Feature uses Revenue Statistics data to examine the volatility of revenues over the past 40 years. By providing insights into the factors behind short- and long-run changes in revenues from different tax types, the chapter aims to inform strategies to optimise fiscal policy over the business cycle and to ensure fiscal sustainability over the longer term. It may also help governments to enhance the resilience of public finances in the event of future shocks.
Following a brief introduction to tax buoyancy, the chapter estimates the buoyancy of total tax revenues and of revenues from different tax types between 1980 and 2021 on average across the OECD and for individual countries. The chapter also investigates whether and how tax buoyancy in OECD countries has changed over this period. Finally, the Special Feature examines how short-run tax buoyancy varies over the business cycle in OECD countries and analyses the potential impact of high inflation or population ageing.
Introducing tax buoyancy
The buoyancy and elasticity of tax revenues are two of the most common indicators of tax revenue volatility (Box 2.1). In one of the earliest papers on the topic, (Musgrave and Miller, 1948[1]) measured tax elasticity as the ratio of the percentage change in tax yield to a given percentage change in income and defined the concept of “built-in flexibility”, the automatic compensatory movement of tax revenues in response to changes in national income. (Groves and Kahn, 1952[2]) developed a regression model to estimate tax elasticity using log tax revenues as the dependent variable and log income as the independent variable. This method is still widely used today to estimate both tax elasticity and tax buoyancy in the long run. (Prest, 1962[3]) introduced the Proportional Adjustment approach to remove the impact of discretionary tax policies on tax revenues and estimate tax elasticity. Later refined by (Mansfield, 1972[4]), this is one of the most common methods for estimating tax elasticity.1
Early papers rarely differentiated between short- and long-run tax buoyancy or elasticity, using the long-run estimate to study both the growth potential and cyclical variability of tax revenues until (Sobel and Holcombe, 1996[5]) proposed time series econometric models to produce unbiased estimates of short- and long-run buoyancy or elasticity. These econometric models have since been used in many studies of tax volatility, including (Belinga et al., 2014[6]), which estimates short- and long-run tax buoyancy in 34 OECD countries between 1965 and 2012; (Dudine and Jalles, 2017[7]), which produces tax buoyancy estimates for 107 countries between 1980 and 2014; and (Deli et al., 2018[8]), which estimates tax buoyancy in 25 OECD countries between 1965 and 2015. Other methods for estimating tax buoyancy have been used by the OECD Fiscal Network (Dougherty, de Biase and Lorenzoni, 2022[9]) and in a recent IMF working paper by (Cornevin, Corrales and Angel, 2023[10]).2
This Special Feature uses econometric models proposed by (Sobel and Holcombe, 1996[5]) and data from Revenue Statistics to estimate short- and long-run tax buoyancy in 38 OECD countries using two estimation approaches: the Mean Group (MG) estimator and the Pooled Mean Group (PMG) estimator.3 Like most research in this area, this Special Feature uses GDP as a proxy of the tax base.
Box 2.1. Indicators of revenue volatility
The volatility of tax revenues is commonly analysed with reference to three concepts: buoyancy, elasticity and stability. Each aims to quantify changes in tax revenues over time in relation to changes in economic conditions, tax bases or tax policies, and each can be measured over the short and long run.
Tax buoyancy measures changes in tax revenues with respect to changes in the tax base (Mansfield, 1972[4]).
Long-run tax buoyancy measures the impact of economic activity on tax revenues by comparing long-term changes in tax revenues with GDP. Long-run tax buoyancy larger than one (also known as ‘unity’) indicates that tax revenues grow faster than GDP over the long term. This indicator is thus useful for understanding the relationship between economic growth and long-term fiscal sustainability (Belinga et al., 2014[6]).
Short-run tax buoyancy measures the cyclical variability of tax revenues. It shows the percentage change in tax revenues that occurs as a result of a one percent change in GDP over a short period of time, and is an indicator of a tax system’s ability to stabilise the economy over the business cycle (Deli et al., 2018[8]). Short-run tax buoyancy larger than one indicates that tax revenues fluctuate more than GDP over the business cycle.
Tax elasticity is similar to tax buoyancy except that it measures the automatic response of tax revenues to changes in GDP by removing the impact of discretionary tax policy on tax revenues. Although tax elasticity is a better indicator than tax buoyancy for informing tax policies and forecasting tax revenues, the need to isolate the impact of discretionary tax policy on revenues means that estimating tax elasticity requires more data than tax buoyancy analysis and this data can be harder to acquire. It is thus a major challenge to analyse tax elasticity across multiple countries over a long period.
Tax stability is the capacity of a tax to produce a certain amount of revenues and to sustain this level (Groves and Kahn, 1952[2]). A tax can be considered to function as a stabiliser of total tax revenues if it decreases the overall variation in tax revenues over the business cycle. A simple measure of tax stability is the coefficient of variation, which is defined as the standard deviation of tax revenues as a share of GDP divided by its mean (Haughton, 1998[11]).
(Haughton, 1998[11]) proposes the revenue stabilising coefficient, which is the coefficient of variation of the total tax-to-GDP ratio subtracted by the coefficient of variation of the tax-to-GDP ratio of all taxes minus one selected tax of interest. This indicator can be used to analyse whether a certain tax functions as a stabiliser of total tax revenues.
In addition to these indicators, there exist simpler measures of tax volatility, such as using standard deviation to calculate the dispersion of tax revenues or tax-to-GDP ratios.
Tax revenue data in Revenue Statistics
This Special Feature considers revenues between 1980 and 20214 from six tax types: personal income tax (PIT), corporate income tax (CIT), social security contributions (SSCs), taxes on property, value added tax (VAT) and excises. Data exists for all years between 1980 and 2021 for 26 OECD countries, while the available data starts from a later period for 12 countries: from 1990 in Chile, Colombia and Costa Rica; 1991 in Hungary and Poland, 1993 in Czechia and 1995 for the remaining countries.5 Data exclude zero values and outliers (Box 2.2).
Figure 2.1 shows the evolution of total tax revenues as well as revenues from the six different tax types as a share of GDP on average across the OECD since 1980. Based on this graph, several observations can be made as context for the analysis of tax revenue buoyancy.
First, the total tax-to-GDP ratio has risen gradually for the OECD on average, from 30.1% of GDP in 1980 to 34.0% in 2022, without many major year-on-year changes. Second, revenues from four tax types (CIT, SSCs, taxes on property and VAT) as a share of GDP display an upward trend over the long term while revenues from PIT and excises have declined as a share of GDP. Third, individual tax types tend to be more volatile than total tax revenues as measured by the standard deviation. Fourth, revenues from CIT show greater volatility than most other tax types. The standard deviation6 of CIT revenues (indexed values), was 14.2 between 1980 and 2021, compared to only 2.9 for total tax revenues between 1980 and 2022.
Figure 2.1. Evolution of tax revenues in the OECD, 1980-2022
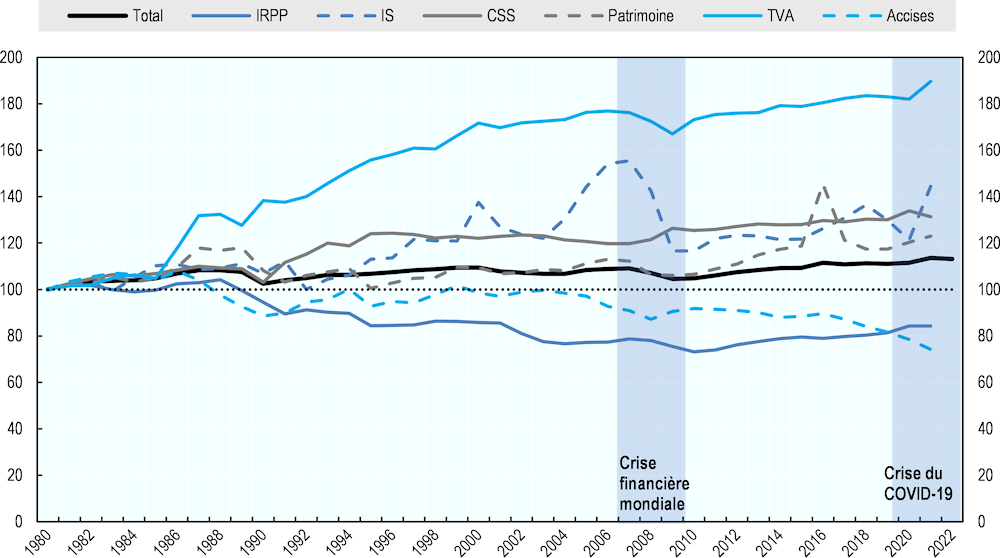
Note: The graph shows tax revenues as a share of GDP indexed by setting 1980 ratios to 100 across tax types so that they are comparable. The spike in property taxes revenues in 2016 is due to a one-off revenue inflow to Iceland. Data for 2022 is not available for individual tax types.
Source: Authors’ calculations.
Box 2.2. Buoyancy estimation methodologies
This box describes the two methodologies used to calculate the buoyancy of tax revenues in this Special Feature: the time series regression Mean Group (MG) estimator and the panel regression Pooled Mean Group (PMG) estimator.
The MG estimator calculates simple group averages after obtaining buoyancy estimates for individual countries (Pesaran and Smith, 1995[12]). The averages exclude outlier results, which for the purpose of this analysis are defined as statistically insignificant estimates that are either close to zero (<0.1), negative (<0) or particularly large (>8), consistent with approaches from other studies (Dudine and Jalles, 2017[7]; Deli et al., 2018[8]). The country-specific estimation applies the Engle-Granger two-step method (Engle and Granger, 1987[13]) to econometric models proposed by (Sobel and Holcombe, 1996[5]).
In the first step, long-run tax buoyancy is obtained from a time series regression model:
(1)
where denotes tax revenues in a country in year t and denotes the corresponding GDP level. is the constant term and measures the long-run effect of a change in GDP level on tax revenues (i.e. long-run tax buoyancy). is the error term of the regression. Equation (1) describes the long-run relationship between tax revenues and GDP.
In the second step, short-run tax buoyancy is estimated by an Error Correction Model (ECM) with the Ordinary Least Squares (OLS) technique using the estimated error from equation (1) as input:
(2)
where denotes the first difference of log tax revenues in a country in year and denotes the first difference of corresponding log GDP. is the error correction term and measures the deviation of two variables from their long-run relationship during the prior time period. It can be substituted with the estimated error (lagged once) from equation (1). is the constant term and measures the instantaneous response of tax revenues to a change in GDP (i.e. short-run tax buoyancy). is the speed of adjustment; it measures how quickly buoyancy converges to the long-term equilibrium value. is the error term of the ECM.
Short- and long-run tax buoyancy for each OECD country are estimated based on individual country time series using equations (1) and (2) respectively.
The PMG estimator, developed by (Pesaran, Shin and Smith, 1999[14]), combines pooling and averaging to allow the intercept (i.e. the constant term), short-run coefficients and error variances to differ across countries but restricts the long-run coefficients to being equal for all countries. It uses the maximum likelihood estimation method to compute a common long-run tax buoyancy and a country-specific short-run tax buoyancy based on the panel dataset of 38 OECD countries between 1980 and 2021. It estimates equation (2) across time and countries.
Both estimation approaches have their strengths and drawbacks. The PMG estimator takes advantage of the additional information and data variability from large panel datasets. It is a more efficient estimator that requires less data to produce consistent estimates. However, the PMG estimator constrains long-run coefficients to be equal across countries and cannot produce estimates for individual countries because it combines pooling and averaging. On the other hand, the MG estimator allows all estimated parameters to be different across countries but may produce inconsistent estimates if data is limited (Belinga et al., 2014[6]).
Data treatment
Among the tax types considered, a few OECD countries do not levy certain taxes; for example, Australia and New Zealand do not levy SSCs while the United States does not levy VAT. Revenues from these tax types are recorded as zero in the Revenue Statistics database for these countries. To prevent the zeros from entering the regression and confusing the relationship between tax revenues and GDP, all observations with a zero value are removed from the data analysed.
One outlier result has also been removed. All OECD countries report year-on-year changes of less than 5 percentage points (p.p.) in total tax revenues and for individual tax types between 1980 and 2021 except Iceland, where property tax revenues as a share of GDP increased by more than 15 p.p. in 2016.7 To address this outlier, the value for Iceland’s property tax revenues in 2016 is replaced by the average of property tax revenues in 2015 and 2017; Iceland’s total tax revenues in 2016 are re-calculated accordingly.
Note: Following the suggestion of (Sobel and Holcombe, 1996[5]), equation (1) is estimated using the Dynamic Ordinary Least Squares technique developed by (Stock and Watson, 1993[15]) to account for possible endogeneity within the model as well as serial correlation in the error term. The regression also applies Newey-West correction for standard errors to deal with autocorrelation and heteroskedasticity (Newey and West, 1987[16]).
Short- and long-run tax buoyancy in the OECD
This section presents the tax buoyancy results generated by the two estimation methods in turn, then analyses both sets of results together. It also identifies caveats that need to be taken into account when interpreting the estimates and examines these caveats in detail with respect to the buoyancy of CIT revenues and the possibility of a time lag in the impact of changes in GDP on revenues. Finally, it analyses whether the results differ when using real rather than nominal data.
MG estimator results
Short- and long-run tax buoyancy estimated using the MG estimator are presented in Table 2.1. The results show that buoyancies for total tax revenues were close to unity8 on average across OECD countries between 1980 and 2021 (individual country estimates are shown in Annex 2.A.).
Long-run tax buoyancies were larger than 1.1 for seven OECD countries and were smaller than 0.9 in two countries; for the remainder, they were between these thresholds. For short-run tax buoyancy, estimates were above 1.1 for eleven countries and below 0.9 for nine countries. The standard deviation of buoyancy for total tax revenues across the OECD was smaller than for the six tax types, indicating that the buoyancy of total tax revenues was more narrowly distributed across countries.
Most of the six individual tax types displayed short- and long-run tax buoyancy close to unity. However, there were four notable exceptions:
For CIT, tax buoyancy was significantly larger than unity, especially in the short run.9 (Please see Box 2.3 for a deeper exploration of the CIT buoyancy estimates.)
Both short- and long-run VAT buoyancy were larger than unity, albeit to a lesser extent than CIT.
The short-run buoyancy of SSCs and long-run buoyancy of excises were slightly below unity.
Table 2.1. Buoyancy of nominal tax revenues by tax type using the MG estimator, 1980-2021
Long-run buoyancy |
Short-run buoyancy |
|||||
---|---|---|---|---|---|---|
Mean |
Median |
Std. |
Mean |
Median |
Std. |
|
Total |
1.028 |
1.018 |
0.083 |
1.008 |
0.999 |
0.218 |
PIT |
1.059 |
0.946 |
0.501 |
1.024 |
0.935 |
0.613 |
CIT |
1.377 |
1.364 |
0.378 |
2.096 |
1.788 |
1.148 |
SSC |
1.083 |
1.007 |
0.427 |
0.892 |
0.796 |
0.699 |
Property |
1.071 |
1.048 |
0.299 |
0.986 |
0.907 |
0.503 |
VAT |
1.262 |
1.099 |
0.900 |
1.119 |
1.064 |
0.539 |
Excises |
0.865 |
0.894 |
0.278 |
0.963 |
0.967 |
0.379 |
Note: Under the Mean Group estimator, tax buoyancies of individual OECD countries are estimated first. Then simple averages are calculated after removing outliers (e.g. estimates that are statistically insignificant and close to zero or negative). Detailed information of nominal tax revenue buoyancies for each OECD country is provided in Annex 2.A. at the end of the Special Feature.
Source: Authors’ calculations based on (OECD, 2022[17]), Revenue Statistics 2022; and (OECD, 2022[18]), OECD National Accounts.
PMG estimator results
Table 2.2 shows the panel regression results using the PMG estimator for total tax revenues and the six tax types. The buoyancy estimates produced by the panel regression are largely in line with those of the time series regression above. Both short- and long-run buoyancy of total tax revenues and PIT were close to unity between 1980 and 2021, while CIT had the highest buoyancy, especially in the short run. VAT had the second-highest short- and long-run buoyancy, while the short-run buoyancy of SSCs was below unity.
However, there were two notable differences between the results produced by the different methodologies. First, the short-run buoyancy of taxes on property was smaller than unity under the PMG estimator while it was close to unity under the MG estimator. Second, both the short- and long-run buoyancy of excises under the PMG were smaller than unity while, under the MG estimator, the short-run buoyancy of excises was close to unity and long-run buoyancy was slightly below unity.
Table 2.2. Buoyancy of nominal tax revenues by tax type using the PMG estimator, 1980-2021
Long-run |
Short-run |
Speed of adjustment |
|
---|---|---|---|
Total |
1.056*** |
1.032*** |
-0.190*** |
PIT |
0.997*** |
0.957*** |
-0.207*** |
CIT |
1.424*** |
2.482*** |
-0.310*** |
SSC |
0.965*** |
0.881*** |
-0.205*** |
Property |
1.048*** |
0.756*** |
-0.199*** |
VAT |
1.128*** |
1.140*** |
-0.287*** |
Excises |
0.514*** |
0.619*** |
-0.149*** |
Note: *, ** and *** refer to statistical significance at the 10%, 5% and 1% levels respectively. Under the Pooled Mean Group estimator, tax buoyancies are estimated based on the panel data of 38 OECD countries between 1980 and 2021. It combines both pooling and averaging, so tax buoyancies of individual countries are not available.
Source: Authors’ calculations based on (OECD, 2022[17]), Revenue Statistics 2022; and (OECD, 2022[18]), OECD National Accounts.
The differences in results between the two methodologies may be caused by different estimation techniques, different ways of calculating averages, and the inherent differences between panel data and time series data. Panel data in general has advantages over time series data insofar as it contains more information and data variation, and it can deal with omitted variable bias (Torres-Reyna, 2007[19]). The advantages of panel data become more pronounced for tax types where data may be limited for some countries.
Analysing the results
Several conclusions may be drawn from the short- and long-run tax buoyancy estimates generated by the two methodologies presented above:
Over the long term, tax revenues grew at the same pace as GDP for the OECD as a whole between 1980 and 2021.
On average, OECD tax revenues were as volatile as GDP over the business cycle.
With the highest short- and long-run buoyancy, revenues from CIT grew faster than GDP in the long term and was the most effective economic stabiliser in the short term.
VAT revenues also grew faster than GDP in the long term and VAT is a relatively good stabiliser.
SSCs were a relatively poor economic stabiliser over the business cycle and revenues from excises are least sensitive to changes in GDP.
These conclusions require several caveats. First, tax buoyancy includes the revenue impact of discretionary policy measures. As such, the response of tax revenues to changes in GDP over a given period of time is affected by tax policies implemented during the same period. Tax buoyancy estimates may change once the impact of policies is separated. Second, since GDP is used as a proxy for different tax bases, the response of tax revenues to changes in GDP is determined by how tax revenues react to changes in tax bases and how tax bases react to changes in GDP. The implications for CIT are discussed in Box 2.3. Lastly, there may be a temporal mismatch between tax revenues and GDP due to specific tax collection mechanisms or statutory lags in declaring the taxable base (Mourre and Princen, 2019[20]). Box 2.4 investigates this issue in greater detail.
Box 2.3. Investigating the limitations of tax buoyancy analysis for CIT revenues
As identified in the estimates presented in this Special Feature and in the literature on tax buoyancy more broadly, revenues from CIT have a buoyancy significantly above unity, especially in the short run. However, these findings should be interpreted with caution as they might be affected by two major limitations of tax buoyancy analysis.
Tax buoyancy estimates conflate changes in tax revenues attributable to changes in GDP with the revenue impact of discretionary policy measures. As such, estimates of the response of tax revenues to GDP growth may change if the impact of changes in tax policy can be isolated from the calculation. (Cornevin, Corrales and Angel, 2023[10]) also notes that, if macroeconomic indicators such as GDP are used as proxy tax bases, buoyancy estimates may differ across tax types because specific tax bases differ in their responses to changes in macroeconomic conditions.
To investigate how these limitations could affect tax buoyancy results, this analysis has been extended to estimate changes in CIT revenues relative to changes in gross operating surplus (rather than GDP), and it includes the statutory CIT rate between 2000 and 2021 as an additional control variable. Gross operating surplus is the difference between the value added generated by production activities and the sum of compensation of employees and taxes on production and imports less subsidies. It is often used to approximate the CIT base (Mourre and Princen, 2019[20]).
Including additional control variables (e.g. tax policy parameters) allows tax buoyancy to be estimated while holding control variables unchanged. Having more explanatory variables also mitigates the potential for omitted variable bias, which could be caused by a correlation of tax policy with both GDP and tax revenues. Changes to statutory tax rates are one of the most important tax policy measures; many tax buoyancy studies use tax rates to control for discretionary policy impact (Belinga et al., 2014[6]; Dudine and Jalles, 2017[7]; Deli et al., 2018[8]; Lagravinese, Liberati and Sacchi, 2020[21]; Cornevin, Corrales and Angel, 2023[10]).
Table 2.3. Buoyancy of nominal CIT revenues under different model specifications, 2000-2021
GDP tax base (no control for rate) |
GDP tax base (with control for rate) |
GOS tax base (no control for rate) |
GOS tax base (with control for rate) |
|
---|---|---|---|---|
Long-run |
1.155*** |
1.607*** |
1.222*** |
1.341*** |
Short-run |
2.989*** |
3.135*** |
1.659*** |
1.652*** |
Note: *, ** and *** refer to statistical significance at the 10%, 5% and 1% levels, respectively. Figures are in nominal terms and estimated using Pooled Mean Group estimator. Under the Pooled Mean Group estimator, tax buoyancies are estimated based on the panel data of OECD countries between 2000 and 2021. Gross operating surplus (GOS) data is obtained from the OECD National Accounts Database and includes 35 OECD countries except Chile, Israel and New Zealand. Statutory CIT rate data comes from the OECD Corporate Tax Statistics database.
Source: Authors' calculations based on (OECD, 2022[17]), Revenue Statistics 2022; (OECD, 2022[18]), OECD National Accounts; and (OECD, 2023[22]), OECD Corporate Tax Statistics.
Table 2.3 presents the buoyancy estimates of CIT revenues under different model specifications. The results yield two main findings. First, using gross operating surplus rather than GDP as the base for CIT yields similar long-run buoyancy for CIT but reduces short-run buoyancy by almost half. This implies that the strong response of CIT revenues to changes in GDP over the business cycle is partly due to a high positive correlation between gross operating surplus and GDP. This is supported by the empirical evidence that the growth rates of companies’ aggregated earnings and of GDP tend to share similar trends, with earnings growth cycles regularly displaying much larger amplitudes than GDP growth cycles (ECB, 2007[23]). Despite this effect, the buoyancy of CIT remains significantly above unity in both the short- and long run.
Second, controlling for the statutory CIT rate leads to higher long-run tax buoyancy. The same holds when using gross operating surplus as the tax base although the increase is smaller. This suggests that the tax rate is negatively correlated with both tax bases and tax revenues in the long run, and that the long-run buoyancy of CIT is underestimated in the ‘no control’ model. Controlling for the tax rate has little effect on short-run buoyancy for CIT revenues.
These results are partly consistent with findings from (Belinga et al., 2014[6]) for OECD countries over the period 1980-2012 and with (Dudine and Jalles, 2017[7]) for advanced economies between 1980 and 2014. They find that controlling for the tax rate yields higher short- and long-run CIT buoyancy. The discrepancy concerning short-run buoyancy is likely due to different time periods used in the estimation: their results are based on data before 2012 and 2014 respectively, while the average statutory CIT rate in the OECD has shown little change since 2008, after falling from 30.4% to 24.0% between 2000 and 2008. Including more recent data thus leads to smaller correlation between the tax rate and other variables. Other papers using more recent data also find no significant change in short-run CIT buoyancy after controlling for the tax rate (Deli et al., 2018[8]; Cornevin, Corrales and Angel, 2023[10]).
Results using real rather than nominal data
The tax buoyancy coefficients shown above are estimated using nominal tax and GDP data, which include a price component and a real component. To understand how price movements affect tax buoyancy, Annex Table 2.A.8. shows the time series regression results when short- and long-run tax buoyancy are estimated using real data. These results suggest that both short- and long-run tax buoyancy estimated with real data are larger than those estimated with nominal data, albeit to different extents for total tax revenues and across most tax types. The panel regression results are mixed: while most buoyancy estimates using real data are close to the estimates based on nominal data, they decreased for PIT and excises in the long run, and SSCs in the short run. The real buoyancy of CIT revenues has increased in the short run (see Annex Table 2.A.9).
Other studies on real tax buoyancy show differing results: (Haughton, 1998[11]) argues that nominal buoyancy could understate the responsiveness of tax revenues to changes in GDP and tax buoyancy in nominal terms may bias towards one. (Belinga et al., 2014[6]) and (Dudine and Jalles, 2017[7]) find that total tax buoyancy in real terms is smaller than in nominal terms, particularly in the long run; (Deli et al., 2018[8]) does not find any significant differences between nominal and real total tax buoyancy; (Cornevin, Corrales and Angel, 2023[10]) investigates the real short-run buoyancies of PIT, CIT and VAT, finding no significant differences for PIT and VAT but higher real short-run CIT buoyancy. The different conclusions may be caused by differences in methodology, country sample or time coverage.
Box 2.4. Is there a temporal mismatch between tax revenues and GDP?
Tax buoyancy coefficients estimated in this special feature match annual tax revenue data from the Revenue Statistics database with fiscal year GDP data from the OECD National Accounts database. These results may be misleading if there are delays in the response of tax revenues to changes in GDP, for example due to loss carry-over (carry-forward/backward) in the case of CIT, specific tax collection mechanisms or statutory lags in declaring the taxable base (Mourre and Princen, 2019[20]). This box investigates whether such a temporal mismatch exists in OECD data by examining the evolution of tax revenues and GDP.
PIT and CIT are chosen for this analysis as their tax payments are usually based on taxable income from the previous year (Choudhry, 1975[24]; Mourre and Princen, 2019[20]). This box examines how revenues from PIT and CIT changed between 2007 and 2010, a period that covers the Global Financial Crisis (GFC), and between 2018 and 2021, which covers the COVID-19 crisis (2020-21). Most OECD countries experienced large declines in revenues and GDP during these two episodes (OECD, 2021[25]). If a temporal mismatch exists, revenues from PIT and CIT should fall one year after substantial declines in nominal GDP. Annex Figure 2.A.1 to Annex Figure 2.A.5 illustrate the evolution of tax revenues and GDP by country. Due to space constraints, only five OECD countries are shown.
The results suggest that PIT and CIT revenues largely moved in tandem with nominal GDP in the OECD during the GFC and the COVID-19 crisis, although individual country results differ:
For Belgium, income tax revenues grew in line with GDP except in 2019, when PIT revenues declined slightly despite strong nominal GDP growth.
For France, PIT revenues and GDP moved in unison during both crises, but CIT revenues were more stable than GDP during the COVID-19 crisis.
Japan’s income tax revenue trajectory was similar to that of GDP during the GFC. However, there was a notable mismatch between PIT revenues and GDP in 2019 and 2020.
In Switzerland, data shows no close correlation between PIT revenues and GDP during both crises as the former continued to increase despite GDP fluctuations. CIT revenues followed the trajectory of GDP, especially during the COVID-19 crisis.
In the United States, growth in income tax revenues was similar to that of GDP, especially during the COVID-19 crisis.
There are three possible explanations for the apparent absence of a delay in the response of tax revenues to GDP. First, OECD countries predominantly report their tax revenue data for Revenue Statistics on an accrual basis, meaning that revenues are recorded at the time when the tax liability was created, not when tax authorities received payment (OECD, 2021[25]).
Second, revenue data used in buoyancy estimations includes the impact of discretionary tax policies. During the COVID-19 crisis, for example, governments in OECD countries implemented support measures to mitigate the negative impact of the pandemic on businesses and households, some of which had immediate effects on income tax revenues even though the revenues themselves were based on the tax base prior to the pandemic.
Finally, differences in collection channels may be a factor. For example, PIT and CIT revenues collected through pay-as-you-earn (PAYE) or advance payments are mostly paid during the year that the income was earned, while revenues that are collected via formal assessments may be determined in a later year due to the time required for tax declarations and investigation procedures. A delay in the response of tax revenues to changes in GDP may therefore be less obvious if a majority of PIT and CIT revenues are collected via PAYE and advanced payments rather than formal assessments.
The evolution of tax buoyancy in OECD countries
This section investigates how tax buoyancy evolved in OECD countries between 1980 and 2021. The dataset is divided into three sub-periods: 1980-1999, 2000-2010 and 2011-2021. The first period is longer than the second and third periods because revenue data are not available for some OECD countries in the 1980s. Table 2.4 presents short- and long-run tax buoyancy estimates using the PMG estimator for these different periods using nominal data. The same results are also plotted in Figure 2.2.
Table 2.4. Buoyancy of nominal tax revenues by tax type over 1980-1999, 2000-2010 and 2011-2021
Long-run buoyancy |
Short-run buoyancy |
|||||
---|---|---|---|---|---|---|
1980-1999 |
2000-2010 |
2011-2021 |
1980-1999 |
2000-2010 |
2011-2021 |
|
Total |
0.846*** |
1.014*** |
1.069*** |
0.828*** |
1.211*** |
0.942*** |
PIT |
0.760*** |
1.239*** |
0.984*** |
0.641*** |
1.340*** |
0.784*** |
CIT |
1.967*** |
1.251*** |
1.476*** |
2.904*** |
3.768*** |
2.232*** |
SSC |
0.835*** |
1.030*** |
0.680*** |
0.872* |
0.688*** |
0.475*** |
Property |
-0.005 |
1.236*** |
1.004*** |
0.168 |
1.032*** |
0.596*** |
VAT |
1.091*** |
1.034*** |
1.125*** |
1.129*** |
1.282*** |
1.264*** |
Excises |
0.641*** |
0.705*** |
0.684*** |
0.496 |
0.329* |
0.843*** |
Note: *, ** and *** refer to statistical significance at the 10%, 5% and 1% levels respectively. Figures are estimated using Pooled Mean Group estimator. Under the Pooled Mean Group estimator, tax buoyancies are estimated based on the panel data of 38 OECD countries during different time periods. Slovenia is dropped for VAT and excises estimation over 1980-1999 due to insufficient data.
Source: Authors’ calculations based on (OECD, 2022[17]), Revenue Statistics 2022; and (OECD, 2022[18]), OECD National Accounts.
Figure 2.2. Buoyancy of nominal tax revenues by tax type, 1980-1999, 2000-2010 and 2011-2021
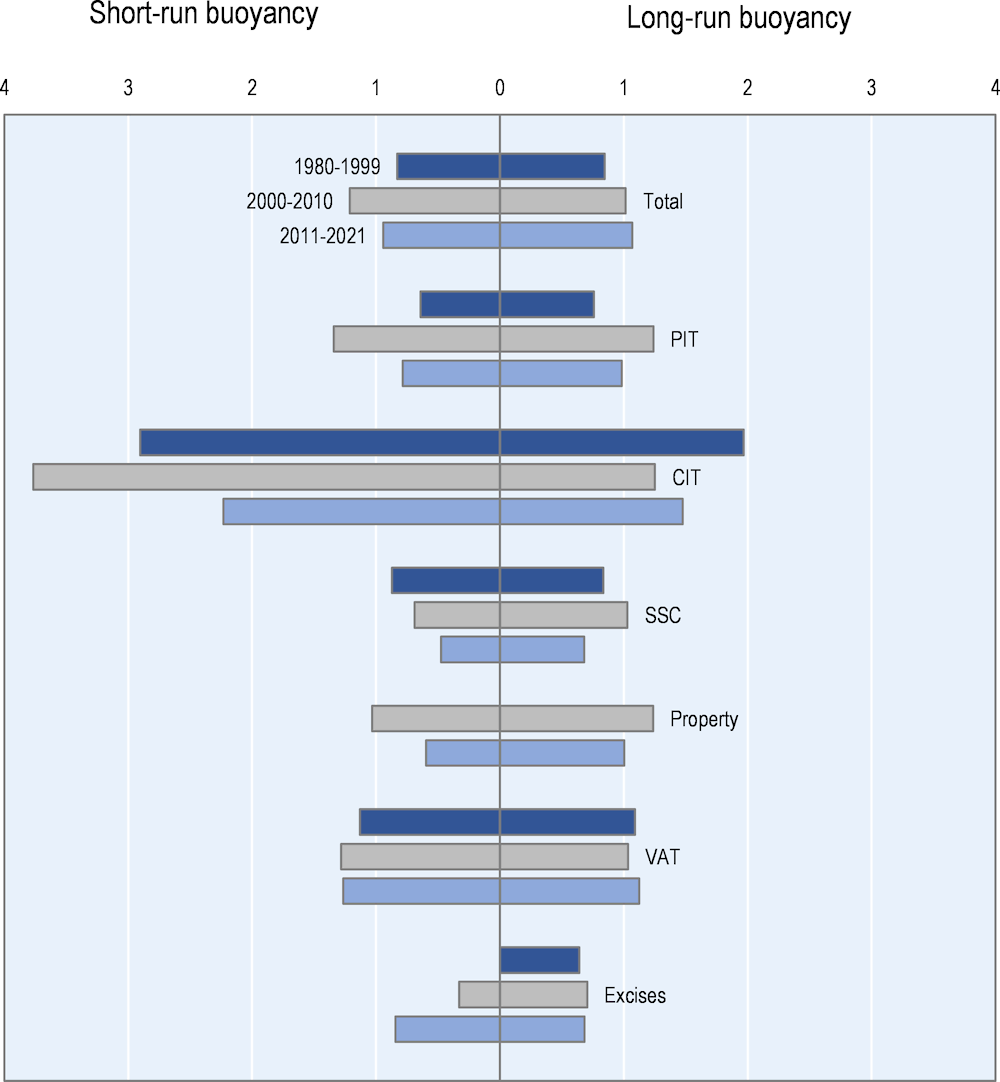
Note: Figures are estimated using Pooled Mean Group estimator. Under Pooled Mean Group estimator, tax buoyancies are estimated based on the panel data of 38 OECD countries during different time periods. Slovenia is dropped for VAT and excises estimation over 1980-1999 due to insufficient data.
Source: Authors’ calculations based on (OECD, 2022[17]), Revenue Statistics 2022; and (OECD, 2022[18]), OECD National Accounts.
For total tax revenues, long-run buoyancy gradually increased over the three periods. Improved tax capacity and changes in tax structures in the OECD are possible explanations for this increase. Short-run buoyancy, which measures tax revenue fluctuation over the business cycle, jumped substantially in 2000-2010 before falling back to unity. The greater volatility in this second period may be due to the Global Financial Crisis, which had a greater negative impact on OECD tax revenues than the recent COVID-19 crisis, in particular revenues from PIT and CIT (OECD, 2021[25]).
Considering the evolution of tax buoyancy for specific tax types, the main findings are as follows:
For PIT, long-run buoyancy increased significantly after 1980 but fell in the third period. One possible explanation for the higher buoyancy in the first two periods is an increase in the progressivity of PIT systems in the OECD (Belinga et al., 2014[6]). The short-run buoyancy of PIT displayed a similar pattern to total tax revenues, rising above unity before falling back, likely due to the impact of the Global Financial Crisis. (OECD, 2021[25]) found that revenues from PIT as a share of GDP dropped on average in 2009, implying a larger decrease of tax revenues than GDP, whereas the opposite occurred in 2020.
For CIT, long-run buoyancy exhibits the opposite trajectory to PIT: it decreased significantly over the three periods despite rising slightly in the third period. (Deli et al., 2018[8]) also find a substantial decline in long-run CIT buoyancy in the post-2000 period compared to the pre-2000 period. One possible explanation for this tendency is that many OECD countries have reduced CIT rates in recent decades, which may have suppressed CIT buoyancy (Box 2.3). The short-run buoyancy of CIT increased substantially in 2000-2010 but has declined in recent years, similar to the short-run buoyancy of total tax revenues and PIT.
The increase in long-run CIT buoyancy in the third period (as well as the decrease in PIT buoyancy) may be related to tax arbitrage. In many OECD countries, a tendency has recently been documented of taxpayers shifting part of their income taxable under PIT to CIT in order to reduce their tax liability, benefitting from lower CIT rates and other tax advantages (OECD, Forthcoming[26]).
Both the short- and long-run buoyancy of SSCs fell over the periods under analysis, a finding consistent with other studies. Changes in the age structure of the population in OECD countries may be a factor behind this change.
For taxes on property, both short- and long-run buoyancy declined after 2000. Results are not statistically significant for 1980-1999. The decline in short-run buoyancy in the third period is likely to be related to subdued property markets in OECD countries after the Global Financial Crisis.
Among taxes on goods and services, the short- and long-run buoyancy of VAT increased between 1980 and 2021. The long-run buoyancy of excises is the lowest among the six tax types in almost every time period, increasing only slightly since 1980, although short-run buoyancy has increased substantially, albeit from a low base.
For comparison, Annex Table 2.A.10 and Annex Figure 2.A.6 show tax buoyancy estimates for the three periods using real tax and GDP data. Most of the conclusions drawn from nominal data hold for real tax buoyancy, with a few exceptions concerning long-run results.
Interaction between tax buoyancy and other socio-economic factors
To broaden the analysis of the factors behind revenue trends in OECD countries, this section investigates the interaction between tax buoyancy and three other socio-economic factors of interest: the business cycle, inflation and the age structure of the population.
Tax buoyancy under different economic conditions
Numerous studies have found that short-run tax buoyancy varies at different stages of the business cycle, indicating asymmetric responses of tax revenues to changes in GDP during periods of economic expansion and contraction. To better understand the power of OECD tax systems to stabilise the economy across the business cycle, short-run tax buoyancy is estimated using equation (3), following the method used in (Belinga et al., 2014[6]) and (Deli et al., 2018[8]).
(3)
Equation (3) is a panel regression ECM similar to Equation (2) but includes the country dimension denoted by and country fixed effect as well as a dummy variable. The dummy variable in the equation takes a value of one for years of growth and a value of zero for years of contraction.
This Special Feature defines years of growth and contraction in two scenarios: a moderate boom-bust cycle where annual real GDP changes of larger than 0.5% (-0.5%) constitute economic growth (economic contraction); and a strong boom-bust cycle, where real GDP changes in excess of 1.0% (-1.0%) constitute economic growth (economic contraction).
Table 2.5 shows that, in a moderate business cycle, the short-run buoyancy of total tax revenues is largely the same for years of economic growth as for years of economic contraction. However, it is larger during economic contractions for most tax types except taxes on property and VAT. In a strong business cycle, short-run buoyancy is significantly larger during years of economic contraction than in years of growth for total tax revenues, CIT, SSCs, taxes on property and excises. This indicates that OECD taxes are on average more buoyant during recessions than during growth periods, consistent with findings in other studies (Belinga et al., 2014[6]; Dudine and Jalles, 2017[7]; Deli et al., 2018[8]). The results also indicate that, as economic recession deepens, tax revenues become more volatile relative to GDP.
Table 2.5. Asymmetric short-run tax buoyancy over the business cycle, 1980-2021
Moderate business cycle |
Strong business cycle |
|||
---|---|---|---|---|
> 0.5% |
< -0.5% |
> 1% |
< -1% |
|
Total |
1.019*** |
1.009*** |
1.007*** |
1.115*** |
PIT |
0.922*** |
1.089* |
1.002*** |
0.843 |
CIT |
2.361*** |
2.696*** |
2.075*** |
3.302*** |
SSC |
0.742*** |
0.954*** |
0.783*** |
0.935*** |
Property |
0.783*** |
0.740* |
0.817*** |
1.188* |
VAT |
1.160*** |
0.838*** |
1.132*** |
0.851*** |
Excises |
0.497*** |
1.237** |
0.429*** |
1.544*** |
Note: *, ** and *** refer to statistical significance at the 10%, 5% and 1% levels respectively. Figures are in nominal terms and estimated using Pooled Mean Group estimator. Under Pooled Mean Group estimator, tax buoyancies are estimated based on the panel data of 38 OECD countries between 1980 and 2021. In a moderate business cycle, real GDP growth of larger than 0.5% constitutes economic growth and smaller than -0.5% constitutes economic contraction. In a strong business cycle, real GDP growth larger than 1.0% constitutes economic growth and smaller than -1.0% constitutes economic contraction.
Source: Authors’ calculations based on (OECD, 2022[17]), Revenue Statistics 2022; and (OECD, 2022[18]), OECD National Accounts.
Impact of inflation on tax buoyancy
High inflation in OECD countries in 2022 revived interest in the impact of inflation on tax revenues. This sub-section investigates the impact of inflation on short-run tax buoyancy using the same approach as above but creates a dummy variable that takes a value of one for years of higher inflation and a value of zero for years of lower inflation. Analysis is conducted for two scenarios: in a baseline scenario, lower/higher inflation is defined as a rate of inflation smaller/larger than 2%, which is the inflation target of the European Central Bank; in an extreme scenario, lower inflation is defined as a rate of inflation below 1% and higher inflation as a rate of inflation above 3%.
Table 2.6 shows that, in the baseline scenario, OECD average short-run buoyancy remained stable regardless of the inflation level for total tax revenues, PIT, taxes on property and VAT. It was larger during years of higher inflation for SSCs and larger during years of lower inflation for CIT and excises. In the extreme scenario, short-run tax buoyancy was larger during years of higher inflation for total tax revenues and VAT and larger during years of lower inflation for CIT and excises.
Table 2.6. Impact of inflation on short-run tax buoyancy, 1980-2021
Baseline scenario |
Extreme scenario |
|||
---|---|---|---|---|
Lower inflation |
Higher inflation |
Lower inflation |
Higher inflation |
|
Total |
0.963*** |
0.979*** |
0.726*** |
0.926*** |
PIT |
0.878*** |
0.958*** |
0.929*** |
0.883*** |
CIT |
2.958*** |
2.235*** |
2.787*** |
2.031*** |
SSC |
0.566*** |
0.941*** |
1.195 |
1.142* |
Property |
0.832*** |
0.731*** |
0.587 |
0.365 |
VAT |
1.030*** |
1.105*** |
1.173** |
1.379*** |
Excises |
0.716*** |
0.543*** |
0.641*** |
0.424** |
Note: *, ** and *** refer to statistical significance at the 10%, 5% and 1% levels respectively. Figures are in nominal terms and estimated using Pooled Mean Group estimator. Under Pooled Mean Group estimator, tax buoyancies are estimated based on the panel data of 38 OECD countries between 1980 and 2021. In the baseline scenario, higher/lower inflation is defined as having an inflation rate larger/smaller than 2%. In the extreme scenario, higher/lower inflation is defined as having an inflation rate larger than 3% and smaller than 1% respectively.
Source: Authors' calculations based on (OECD, 2022[17]), Revenue Statistics 2022; (OECD, 2022[18]), OECD National Accounts; and (OECD, 2023[27]), OECD Prices and Purchasing Power Parities database.
Impact of population ageing on tax buoyancy
(OECD/WHO, 2020[28]) qualifies a country as having an “ageing society” if the share of people aged 65 years or more is between 7% and 14% of the total population, as an “aged society” if this share is between 15% and 20%, and as a “super-aged society” if this share is 21% or higher. By this measure, many OECD countries have ‘aged’ societies: in recent decades, the share of the population aged 65 years and over has nearly doubled on average across OECD countries, increasing from less than 9% in 1960 to more than 17% in 2019 (OECD, 2021[29]).
To analyse the impact of population ageing on tax buoyancy, this Special Feature considers a country as having a “younger society” if the share of its population aged 65 years or older is smaller than 15%, while a country has an “older society” if the share is above this threshold. Results shown in Table 2.7 indicate that short-run buoyancy was notably larger in a “younger society” for total tax revenues, CIT, SSCs and VAT, while it was slightly larger for PIT and taxes on property. The short-run buoyancy of excises was larger in an “older society”, although the difference was trivial. The results suggest that tax revenues were more stable over the business cycle in countries with an ageing population.
Table 2.7. Impact of population ageing on short-run tax buoyancy, 1980-2021
Younger society |
Older society |
|
---|---|---|
Total |
1.023*** |
0.787*** |
PIT |
0.918*** |
0.875*** |
CIT |
2.377*** |
2.049*** |
SSC |
0.962** |
0.444*** |
Property |
0.685*** |
0.630*** |
VAT |
1.028*** |
0.892*** |
Excises |
0.459* |
0.464*** |
Note: *, ** and *** refer to statistical significance at the 10%, 5% and 1% levels respectively. Figures are in nominal terms and estimated using Pooled Mean Group estimator. Under Pooled Mean Group estimator, tax buoyancies are estimated based on the panel data of 38 OECD countries between 1980 and 2021. Older/younger society is defined as having the share of people aged 65 years or more larger/smaller than 15%.
Source: Authors' calculations based on (OECD, 2022[17]), Revenue Statistics 2022; (OECD, 2022[18]), OECD National Accounts; and (OECD, 2023[30]), OECD Health database.
Box 2.5. Short- and long-run tax buoyancy in different geographical regions
In the future, estimates of tax buoyancy using OECD data could be expanded to a much broader range of countries by taking advantage of the OECD Global Revenue Statistics database. At present, this contains harmonised data on tax revenues over time for over 125 economies, including in Africa, Asia-Pacific and Latin America and the Caribbean (LAC).
Table 2.8 shows the short- and long-run buoyancy of total tax revenues in these three regions between 2000 and 2021. On average, both short- and long-run buoyancy of total tax revenues were above unity for Africa (33 countries), suggesting that tax revenues in African countries have grown rapidly in the last two decades. The figures are close to the buoyancy estimates of around 1.2 for low-income developing countries from (Dudine and Jalles, 2017[7]).
Table 2.8. Buoyancy of nominal CIT revenues under different model specifications, 2000-2021
Africa (2000-2021) |
Asia-Pacific (2000-2021) |
LAC (1990-2021) |
|
---|---|---|---|
Long-run |
1.161*** |
1.033*** |
1.068*** |
Short-run |
1.155*** |
1.367*** |
1.035*** |
Speed of adjustment |
-0.417*** |
-0.272*** |
-0.257*** |
Note: *, ** and *** refer to statistical significance at the 10%, 5% and 1% levels respectively. Figures are in nominal terms and estimated using Pooled Mean Group estimator. Under Pooled Mean Group estimator, tax buoyancies are estimated based on the panel data of 33 African countries and 29 Asian and Pacific economies between 2000 and 2021, as well as 26 LAC countries between 1990 and 2021. Nauru is excluded from Asia-Pacific sample due to limited data and high tax-to-GDP volatility. Venezuela is excluded from LAC sample due to missing data since 2014.
Source: Authors’ calculations based on (OECD, 2023[31]), Global Revenue Statistics database.
For the Asia-Pacific (29 economies), long-run buoyancy is close to one while short-run buoyancy is significantly above one and the highest across regions, implying high short-term volatility of tax revenues over the business cycle. The Asia-Pacific results are consistent with findings from (Dudine and Jalles, 2017[7]) with respect to emerging market economies.
Finally, both short- and long-run total tax buoyancy are close to unity in the LAC region, similar to the results for the OECD in this Special Feature.
The response of tax revenues to changes in GDP shows distinct patterns in different geographical regions. It would be interesting to further expand the analysis by including more tax types, controlling for tax policy parameters, examining buoyancy evolution over time and investigating the reasons behind regional differences.
Note: Tax revenue data comes from the Global Revenue Statistics database, which also indicates the source of GDP data used for each fiscal year. For Africa and Asia-Pacific, unbalanced panels between 2000 and 2021 are used, while the LAC region has an almost balanced panel over the period 1990-2021. The PMG estimator is used to estimate buoyancy in each region to account for the relatively short time series.
Conclusion
To analyse the volatility of tax revenues in the OECD, this Special Feature estimates the buoyancy of total tax revenues and revenues from six main tax types for all 38 OECD countries between 1980 and 2021. It finds that tax revenues in the OECD typically increased at the same pace as GDP growth over the long term and had similar volatility to the business cycle in the short run. Revenues from CIT and VAT were more buoyant than revenues from other tax types. SSCs and excises were more stable revenue sources during short-term economic fluctuations. The long-run buoyancy of total tax revenues and most tax types has increased since 1980, with the exception of CIT and SSCs.
The short-run buoyancy for all taxes except excises rose sharply in 2000-2010, likely due to the asymmetric impact of the Global Financial Crisis on revenues and GDP in 2008-09, before falling in 2011-2021. Short-run tax buoyancy tended to be larger during periods of economic contraction than during economic growth. It was also larger during periods of high inflation for most taxes except CIT and excises. Tax revenues appear to be more stable over the business cycle in countries with an aged population.
The results of the tax buoyancy estimates must be taken with caution as the estimates are affected by tax policies implemented over the sample period; estimates may change once the impact of tax policies is removed. This caveat warns against using historical estimates of tax buoyancy to make predictions for the evolution of tax revenues in the future. In addition, since GDP is used as a proxy tax base, the response of tax revenues to changes in GDP may be partly affected by how real tax bases react to changes in GDP.
Further analysis of tax volatility in OECD countries could include estimates of tax elasticity to disentangle the automatic response of tax revenues to changes in GDP and discretionary tax policy impacts. Tax buoyancy in non-OECD countries could also be estimated using the Global Revenue Statistics database (Box 2.5). In addition, measuring changes in tax revenues relative to changes in tax bases and changes in tax bases relative to changes in GDP can provide more information about the sources of tax buoyancy variation and why it differs across taxes, between countries and over time.
References
[6] Belinga, V. et al. (2014), “Tax buoyancy in OECD countries”, International Monetary Fund, Washington, D.C., https://www.imf.org/external/pubs/ft/wp/2014/wp14110.pdf.
[24] Choudhry, N. (1975), “A Study of the Elasticity of the West Malaysian Income Tax System, 1961-70”, IMF Staff Papers, Vol. 22/2, pp. 494-509, https://www.jstor.org/stable/3866486.
[10] Cornevin, A., J. Corrales and J. Angel (2023), “A Deep Dive into Tax Buoyancy: Comparing Estimation Techniques in a Large Heterogeneous Panel”, IMF Working Papers, International Monetary Fund, Washington, D.C., https://www.imf.org/-/media/Files/Publications/WP/2023/English/wpiea2023071-print-pdf.ashx.
[8] Deli, Y. et al. (2018), “Dynamic tax revenue buoyancy estimates for a panel of OECD countries”, ESRI Working Papers, https://www.esri.ie/pubs/WP592.pdf.
[9] Dougherty, S., P. de Biase and L. Lorenzoni (2022), “Funding the future: The impact of population ageing on revenues across levels of government”, OECD Working Papers, OECD Publishing, Paris, https://doi.org/10.1787/2b0f063e-en.
[7] Dudine, P. and J. Jalles (2017), “How buoyant is the tax system? New evidence from a large heterogeneous panel”, IMF Working Papers, International Monetary Fund, Washington, D.C., https://www.imf.org/-/media/Files/Publications/WP/wp1704.ashx.
[23] ECB (2007), “The relationship between listed companies’ earnings growth and output growth in the economy as a whole”, in Monthly Bulletin, September 2007, European Central Bank, Frankfurt, https://www.ecb.europa.eu/pub/pdf/other/mb200709_focus05.en.pdf.
[13] Engle, R. and C. Granger (1987), “Co-Integration and Error Correction: Representation, Estimation, and Testing”, Econometrica, Vol. 55/2, pp. 251-276, https://www.jstor.org/stable/1913236.
[2] Groves, H. and C. Kahn (1952), “The Stability of State and Local Tax Yields”, The American Economic Review, Vol. 42/1, pp. 87-102, https://www.jstor.org/stable/1914417.
[11] Haughton, J. (1998), “Estimating tax buoyancy, elasticity, and stability”, African Economic Policy Paper, https://pdf.usaid.gov/pdf_docs/Pnace024.pdf.
[21] Lagravinese, R., P. Liberati and A. Sacchi (2020), “Tax Buoyancy in OECD Countries: New Empirical Evidence”, Journal of Macroeconomics, Vol. 63/C, http://www.sciencedirect.com/science/article/pii/S0164070419302836.
[4] Mansfield, C. (1972), “Elasticity and Buoyancy of a Tax System: A Method Applied to Paraguay”, IMF Staff Papers, Vol. 19/2, pp. 425-446, https://www.jstor.org/stable/3866368.
[20] Mourre, G. and S. Princen (2019), “The Dynamics of Tax Elasticities in the Whole European Union”, CESifo Economic Studies, pp. 204-235, https://academic.oup.com/cesifo/article-pdf/65/2/204/28704406/ify028.pdf.
[1] Musgrave, R. and M. Miller (1948), “Built-in Flexibility”, The American Economic Review, Vol. 38/1, pp. 122-128, https://www.jstor.org/stable/1801943.
[16] Newey, W. and K. West (1987), “A Simple, Positive Semi-definite, Heteroskedasticity and Autocorrelation Consistent Covariance Matrix”, Econometrica, Vol. 55/3, pp. 703-708, https://www.jstor.org/stable/1913610.
[31] OECD (2023), “Global Revenue Statistics database”, in OECD.Stat (database), OECD Publishing, Paris, https://www.oecd.org/tax/tax-policy/global-revenue-statistics-database.htm (accessed on 20 September 2023).
[22] OECD (2023), “OECD Corporate Tax Statistics: Statutory Corporate Income Tax Rates”, in OECD.Stat (database), OECD Publishing, Paris, https://stats.oecd.org/Index.aspx?DataSetCode=CTS_CIT# (accessed on 20 September 2023).
[30] OECD (2023), “OECD Health: Demographic References”, in OECD.Stat (database), OECD Publishing, Paris, https://stats.oecd.org/Index.aspx?DataSetCode=HEALTH_DEMR (accessed on 20 September 2023).
[27] OECD (2023), “OECD Prices and Purchasing Power Parities: Consumer Price Indices”, in OECD.Stat (database), OECD Publishing, Paris, https://stats.oecd.org/Index.aspx?DataSetCode=PRICES_CPI (accessed on 20 September 2023).
[18] OECD (2022), “OECD National Accounts: Gross Domestic Product”, in OECD.Stat (database), OECD Publishing, Paris, https://stats.oecd.org/Index.aspx?DataSetCode=SNA_TABLE1 (accessed on 1 February 2023).
[17] OECD (2022), Revenue Statistics 2022: The Impact of COVID-19 on OECD Tax Revenues, OECD Publishing, Paris, https://doi.org/10.1787/8a691b03-en.
[33] OECD (2022), Tax Policy Reforms 2022: OECD and Selected Partner Economies, OECD Publishing, Paris, https://doi.org/10.1787/067c593d-en.
[34] OECD (2022), Tax Policy Reforms 2022: OECD and Selected Partner Economies, OECD Publishing, Paris, https://doi.org/10.1787/067c593d-en.
[32] OECD (2021), Health at a Glance 2021: OECD Indicators, OECD Publishing, Paris, https://doi.org/10.1787/ae3016b9-en.
[29] OECD (2021), Health at a Glance 2021: OECD Indicators, OECD Publishing, Paris, https://doi.org/10.1787/ae3016b9-en.
[25] OECD (2021), Revenue Statistics 2021: The Initial Impact of COVID-19 on OECD Tax Revenues, OECD Publishing, Paris, https://doi.org/10.1787/6e87f932-en.
[26] OECD (Forthcoming), Business income and tax arbitrage: implications for OECD tax systems, OECD Publishing, Paris.
[28] OECD/WHO (2020), Health at a Glance: Asia/Pacific 2020: Measuring Progress Towards Universal Health Coverage, OECD Publishing, Paris, https://doi.org/10.1787/26b007cd-en.
[14] Pesaran, M., Y. Shin and R. Smith (1999), “Pooled Mean Group Estimation of Dynamic Heterogeneous Panels”, Journal of the American Statistical Association, Vol. 94/446, pp. 621-634, https://www.jstor.org/stable/2670182.
[12] Pesaran, M. and R. Smith (1995), “Estimating long-run relationships from dynamic heterogeneous panels”, Journal of Econometrics, Vol. 68/1, pp. 79-113, https://www.sciencedirect.com/science/article/pii/030440769401644F.
[3] Prest, A. (1962), “The Sensitivity of the Yield of Personal Income Tax in the United Kingdom”, The Economic Journal, Vol. 72/287, pp. 576-596, https://www.jstor.org/stable/2228439.
[5] Sobel, R. and R. Holcombe (1996), “Measuring the growth and variability of tax bases over the business cycle”, National Tax Journal, Vol. 49/4, https://www.journals.uchicago.edu/doi/epdf/10.1086/NTJ41789225.
[15] Stock, J. and M. Watson (1993), “A Simple Estimator of Cointegrating Vectors in Higher Order Integrated Systems”, Econometrica, Vol. 61/4, pp. 783-820, https://www.princeton.edu/~mwatson/papers/Stock_Watson_Ecta_1993.pdf.
[19] Torres-Reyna, O. (2007), Panel Data Analysis: Fixed and Random Effects using Stata, https://www.princeton.edu/~otorres/Panel101.pdf.
Annex 2.A. Country-specific tax buoyancy estimates
Annex Table 2.A.1. Buoyancy of total tax revenue by country, 1980-2021
Nominal |
Real |
|||||
---|---|---|---|---|---|---|
Long-run buoyancy |
Short-run buoyancy |
Speed of adjustment |
Long-run buoyancy |
Short-run buoyancy |
Speed of adjustment |
|
Australia |
1.017*** |
1.004*** |
-0.222* |
1.018*** |
1.760*** |
-0.285* |
Austria |
1.005*** |
1.065*** |
-0.238 |
1.114*** |
0.964*** |
-0.193 |
Belgium |
1.000*** |
0.924*** |
-0.303* |
1.051*** |
0.831*** |
-0.300* |
Canada |
0.929*** |
0.709*** |
-0.133 |
0.934*** |
0.607*** |
-0.127 |
Switzerland |
1.118*** |
0.763*** |
-0.310** |
1.259*** |
0.669*** |
-0.177 |
Chile |
1.083*** |
1.709*** |
-0.702* |
1.177*** |
2.108*** |
-0.602* |
Colombia |
1.132*** |
1.244*** |
-0.506* |
1.279*** |
1.530*** |
-0.503** |
Costa Rica |
1.067*** |
1.115*** |
-0.544* |
1.184*** |
1.549*** |
-0.487* |
Czechia |
1.071*** |
0.993*** |
-0.571* |
1.059*** |
1.120*** |
-0.450 |
Germany |
1.148*** |
1.181*** |
-0.577** |
1.255*** |
1.114*** |
-0.372* |
Denmark |
1.010*** |
1.169*** |
-0.375** |
1.104*** |
1.189*** |
-0.457*** |
Spain |
1.187*** |
1.144*** |
-0.266* |
1.318*** |
1.030*** |
-0.273* |
Estonia |
1.015*** |
0.732*** |
-0.676** |
1.034*** |
0.609*** |
-0.638** |
Finland |
1.003*** |
1.103*** |
-0.232* |
1.084*** |
1.013*** |
-0.176* |
France |
1.068*** |
0.955*** |
-0.195 |
1.137*** |
0.882*** |
-0.190 |
United Kingdom |
0.939*** |
0.832*** |
-0.148 |
0.904*** |
1.088*** |
-0.130 |
Greece |
1.048*** |
0.946*** |
-0.269** |
1.722*** |
0.709*** |
-0.0799 |
Hungary |
0.950*** |
0.813*** |
-0.523** |
0.901*** |
0.855*** |
-0.495** |
Ireland |
0.866*** |
0.688*** |
-0.0168 |
0.805*** |
0.481** |
-0.0786 |
Iceland |
1.080*** |
0.956*** |
-0.327* |
1.236*** |
1.125*** |
-0.347* |
Israel |
0.885*** |
1.344*** |
-0.408 |
0.849*** |
1.636*** |
-0.290 |
Italy |
1.065*** |
1.065*** |
-0.396* |
1.538*** |
0.887*** |
-0.297* |
Japan |
1.260*** |
1.073*** |
0.0939 |
1.383*** |
1.187*** |
0.0940 |
Korea |
1.103*** |
0.993*** |
-0.197 |
1.118*** |
1.004*** |
-0.280 |
Lithuania |
0.967*** |
1.032*** |
-0.233 |
0.896*** |
1.006*** |
-0.157 |
Luxembourg |
0.975*** |
0.605*** |
-0.284* |
0.992*** |
0.514** |
-0.311* |
Latvia |
1.005*** |
0.979*** |
-0.186 |
1.019*** |
0.955*** |
-0.170 |
Mexico |
1.032*** |
0.926*** |
-0.0644 |
1.024*** |
1.126** |
-0.104 |
Netherlands |
0.904*** |
0.725*** |
-0.0994 |
0.837*** |
0.843*** |
-0.0787 |
Norway |
1.018*** |
1.017*** |
-0.285* |
0.948*** |
1.352*** |
-0.178 |
New Zealand |
0.925*** |
1.101*** |
-0.110 |
0.920*** |
0.849* |
-0.274* |
Poland |
1.048*** |
0.952*** |
-0.352 |
0.976*** |
1.078*** |
-0.202 |
Portugal |
1.109*** |
1.081*** |
-0.273 |
1.450*** |
1.171*** |
-0.192 |
Slovak Republic |
0.933*** |
0.650*** |
-0.0527 |
0.888*** |
0.769*** |
-0.107 |
Slovenia |
0.995*** |
0.975*** |
-0.354 |
0.961*** |
0.971*** |
-0.336 |
Sweden |
0.974*** |
1.210*** |
-0.159 |
0.895*** |
1.356*** |
-0.352** |
Türkiye |
1.065*** |
1.077*** |
-0.554** |
1.525*** |
0.957*** |
-0.0590 |
United States |
1.054*** |
1.441*** |
-0.273* |
1.048*** |
1.605*** |
-0.458** |
Source: Authors’ calculations based on (OECD, 2022[17]), Revenue Statistics 2022; and (OECD, 2022[18]), OECD National Accounts.
Annex Table 2.A.2. Buoyancy of personal income tax by country, 1980-2021
Nominal |
Real |
|||||
---|---|---|---|---|---|---|
Long-run buoyancy |
Short-run buoyancy |
Speed of adjustment |
Long-run buoyancy |
Short-run buoyancy |
Speed of adjustment |
|
Australia |
0.950*** |
0.865** |
-0.242* |
0.875*** |
2.176*** |
-0.221 |
Austria |
0.971*** |
1.089** |
-0.602*** |
1.092*** |
1.136** |
-0.497** |
Belgium |
0.772*** |
0.503* |
-0.277* |
0.683*** |
0.341 |
-0.225 |
Canada |
0.852*** |
0.777*** |
-0.156 |
0.860*** |
0.509 |
-0.180 |
Switzerland |
0.905*** |
0.0520 |
-0.233 |
0.886*** |
-0.515 |
-0.199 |
Chile |
1.090*** |
0.324 |
-0.554* |
1.073*** |
-0.408 |
-0.535* |
Colombia |
1.216*** |
1.145 |
-0.435* |
3.592*** |
0.398 |
-0.0794 |
Costa Rica |
3.683*** |
3.881** |
-0.758* |
6.469*** |
4.661 |
-0.353 |
Czechia |
1.009*** |
0.799* |
-0.0984 |
0.722*** |
0.749* |
0.0601 |
Germany |
1.110*** |
1.500*** |
-0.406** |
1.071*** |
1.561*** |
-0.244 |
Denmark |
0.991*** |
0.771*** |
-0.327* |
1.127*** |
0.560* |
-0.341** |
Spain |
1.079*** |
1.087*** |
-0.221* |
1.318*** |
0.870* |
-0.228* |
Estonia |
0.842*** |
0.916*** |
-0.258 |
0.596*** |
0.841*** |
-0.301 |
Finland |
0.845*** |
1.011*** |
-0.541*** |
0.758*** |
0.877*** |
-0.618*** |
France |
2.000*** |
0.808 |
-0.225 |
2.154*** |
0.999 |
-0.227* |
United Kingdom |
0.856*** |
0.685** |
-0.370* |
0.902*** |
0.774** |
-0.337* |
Greece |
0.941*** |
0.796*** |
-0.447** |
1.993*** |
0.404 |
-0.225* |
Hungary |
0.783*** |
1.098*** |
-0.206 |
0.400** |
0.875 |
-0.271 |
Ireland |
0.857*** |
0.565*** |
-0.312* |
0.798*** |
0.345 |
-0.312* |
Iceland |
1.218*** |
0.945*** |
-0.226* |
1.840*** |
0.907** |
-0.0684 |
Israel |
0.493*** |
1.558* |
0.0278 |
0.289 |
1.960** |
0.0874 |
Italy |
0.901*** |
1.047*** |
-0.916*** |
1.414*** |
0.674** |
-0.595*** |
Japan |
1.164*** |
1.407*** |
-0.305* |
0.829*** |
1.468*** |
-0.198 |
Korea |
1.317*** |
0.889** |
-0.210 |
1.249*** |
0.572 |
-0.149 |
Lithuania |
0.585** |
1.984*** |
-0.0981 |
0.296 |
2.491*** |
-0.126 |
Luxembourg |
0.844*** |
0.628* |
-0.0600 |
0.835*** |
0.0354 |
-0.104 |
Latvia |
1.080*** |
1.094*** |
-0.873* |
1.197*** |
1.145*** |
-0.776* |
Mexico |
1.483*** |
0.573 |
-0.253 |
2.575*** |
0.701 |
-0.293 |
Netherlands |
0.739*** |
0.120 |
-0.116 |
0.512** |
-0.204 |
-0.0963 |
Norway |
0.942*** |
0.201 |
-0.300* |
0.942*** |
0.0258 |
-0.403* |
New Zealand |
0.747*** |
1.108*** |
-0.0402 |
0.482*** |
1.237** |
-0.176 |
Poland |
1.260*** |
0.809** |
-0.821** |
0.824*** |
1.242 |
-0.235 |
Portugal |
1.043*** |
1.255*** |
-0.249 |
1.261*** |
0.592 |
-0.211 |
Slovak Republic |
0.794*** |
0.701 |
-0.106 |
0.724*** |
1.216* |
-0.0942 |
Slovenia |
0.930*** |
1.128*** |
-0.552* |
0.831*** |
1.149*** |
-0.483 |
Sweden |
0.805*** |
0.935*** |
-0.189 |
0.456*** |
1.485*** |
-0.386* |
Türkiye |
1.006*** |
1.045*** |
-0.504*** |
0.945*** |
0.873* |
-0.325* |
United States |
1.122*** |
1.822*** |
-0.325* |
1.099*** |
2.120*** |
-0.421** |
Source: Authors’ calculations based on (OECD, 2022[17]), Revenue Statistics 2022; and (OECD, 2022[18]), OECD National Accounts.
Annex Table 2.A.3. Buoyancy of corporate income tax by country, 1980-2021
Nominal |
Real |
|||||
---|---|---|---|---|---|---|
Long-run buoyancy |
Short-run buoyancy |
Speed of adjustment |
Long-run buoyancy |
Short-run buoyancy |
Speed of adjustment |
|
Australia |
1.327*** |
1.817** |
-0.233 |
1.653*** |
1.926 |
-0.284* |
Austria |
1.484*** |
3.807*** |
-0.558** |
2.038*** |
3.998*** |
-0.703*** |
Belgium |
1.623*** |
2.262** |
-0.281* |
1.939*** |
2.595** |
-0.325* |
Canada |
1.470*** |
1.456* |
-0.308* |
1.598*** |
2.386** |
-0.420** |
Switzerland |
1.842*** |
1.578*** |
-0.196 |
2.169*** |
2.444*** |
-0.426** |
Chile |
1.683*** |
3.587*** |
-0.376* |
2.717*** |
3.767** |
-0.332 |
Colombia |
1.521*** |
1.154 |
-0.508* |
2.949*** |
1.880 |
-0.266 |
Costa Rica |
1.549*** |
2.419** |
-0.909* |
1.749** |
3.538* |
-0.829** |
Czechia |
1.131*** |
1.785** |
-0.309 |
1.084*** |
1.747*** |
-0.710** |
Germany |
1.208*** |
1.491 |
-0.490** |
1.345*** |
2.802 |
-0.458** |
Denmark |
1.636*** |
4.430*** |
-0.488*** |
2.141*** |
5.821*** |
-0.710*** |
Spain |
1.791*** |
2.089*** |
-0.375* |
1.848*** |
2.756*** |
-0.370* |
Estonia |
1.393*** |
0.654 |
-0.651* |
1.492** |
0.284 |
-0.643** |
Finland |
2.334*** |
4.894** |
-0.435* |
2.536*** |
6.863*** |
-0.739*** |
France |
1.259*** |
2.440** |
-0.523** |
1.557*** |
3.737** |
-0.712*** |
United Kingdom |
0.883*** |
1.791** |
-0.190 |
0.621** |
2.140** |
-0.240* |
Greece |
1.738*** |
1.649*** |
-0.452** |
3.237*** |
2.906** |
-0.190 |
Hungary |
0.936*** |
1.240* |
-0.284 |
0.330 |
1.228 |
-0.393 |
Ireland |
1.353*** |
1.347*** |
-0.0999 |
1.436*** |
1.793*** |
-0.145 |
Iceland |
1.602*** |
0.727 |
-0.713*** |
2.311*** |
1.203 |
-0.600** |
Israel |
1.019*** |
3.269** |
-0.740** |
1.098*** |
5.523*** |
-0.441 |
Italy |
0.731* |
1.459*** |
-0.277* |
0.693* |
1.387 |
-0.317* |
Japan |
1.027*** |
2.331*** |
-0.290 |
0.919*** |
3.658*** |
-0.274 |
Korea |
1.213*** |
0.838 |
-0.399* |
1.407*** |
0.806 |
-0.358 |
Lithuania |
1.786*** |
2.746** |
-0.391 |
2.107*** |
3.987** |
-0.385 |
Luxembourg |
0.897*** |
0.409 |
-0.343* |
0.932*** |
0.356 |
-0.326* |
Latvia |
0.547 |
2.105 |
-0.605* |
-0.202 |
2.384 |
-0.536 |
Mexico |
1.905*** |
0.984 |
-0.595** |
3.802*** |
1.641 |
-0.620* |
Netherlands |
1.175*** |
2.644** |
-0.420* |
1.284*** |
4.153*** |
-0.448* |
Norway |
1.764*** |
4.759*** |
-0.201 |
1.701** |
6.802* |
-0.182 |
New Zealand |
1.323*** |
0.952 |
-0.505** |
1.608*** |
2.691* |
-0.578** |
Poland |
1.037*** |
1.076** |
-0.315 |
0.829*** |
2.887** |
-0.762** |
Portugal |
1.529*** |
2.949*** |
-0.616*** |
2.429*** |
3.931*** |
-0.747*** |
Slovak Republic |
1.107*** |
1.359** |
-0.285 |
1.178*** |
2.093*** |
-0.258 |
Slovenia |
2.040*** |
2.850*** |
-0.223 |
2.390** |
3.999*** |
-0.163 |
Sweden |
1.375*** |
1.543 |
-0.470** |
1.785*** |
2.818* |
-0.492** |
Türkiye |
1.008*** |
1.011*** |
-0.289 |
1.353*** |
-0.0853 |
-0.260* |
United States |
1.100*** |
3.761** |
-0.194 |
1.061*** |
4.965*** |
-0.271* |
Source: Authors’ calculations based on (OECD, 2022[17]), Revenue Statistics 2022; and (OECD, 2022[18]), OECD National Accounts.
Annex Table 2.A.4. Buoyancy of social security contributions by country, 1980-2021
Nominal |
Real |
|||||
---|---|---|---|---|---|---|
Long-run buoyancy |
Short-run buoyancy |
Speed of adjustment |
Long-run buoyancy |
Short-run buoyancy |
Speed of adjustment |
|
Australia |
n/a |
n/a |
n/a |
n/a |
n/a |
n/a |
Austria |
1.056*** |
0.713*** |
-0.0687 |
1.221*** |
0.520*** |
-0.0324 |
Belgium |
0.924*** |
0.801*** |
-0.292* |
1.036*** |
0.366* |
-0.225* |
Canada |
1.024*** |
0.401* |
-0.330* |
1.141*** |
0.0700 |
-0.259* |
Switzerland |
1.024*** |
0.414* |
-0.380* |
1.306*** |
-0.339 |
-0.151 |
Chile |
1.051*** |
0.345*** |
-0.316 |
1.056*** |
0.514* |
-0.613* |
Colombia |
0.713*** |
1.563*** |
-0.747** |
0.147 |
1.727* |
-0.721** |
Costa Rica |
1.045*** |
0.902*** |
-0.835* |
1.279*** |
0.699** |
-0.463* |
Czechia |
1.055*** |
0.991*** |
-0.359 |
1.048*** |
1.052*** |
-0.282 |
Germany |
1.158*** |
0.873*** |
-0.194 |
1.321*** |
0.622** |
-0.194 |
Denmark |
2.887** |
4.303 |
-0.405** |
0.617 |
8.724 |
-0.260* |
Spain |
1.032*** |
0.821*** |
-0.441* |
1.077*** |
0.578*** |
-0.538** |
Estonia |
0.968*** |
0.601*** |
-0.326 |
1.002*** |
0.398** |
-0.248 |
Finland |
1.008*** |
0.771*** |
-0.236** |
1.347*** |
0.342 |
-0.0925 |
France |
0.733*** |
0.902*** |
-0.119 |
0.732*** |
0.548* |
-0.188 |
United Kingdom |
0.986*** |
0.509*** |
-0.0664 |
0.980*** |
0.735*** |
-0.0685 |
Greece |
1.027*** |
0.909*** |
-0.175 |
1.733*** |
0.815*** |
-0.0915 |
Hungary |
0.871*** |
0.663*** |
-0.507** |
0.834*** |
1.189** |
-0.465** |
Ireland |
0.895*** |
0.332** |
-0.221 |
0.920*** |
0.0219 |
-0.268* |
Iceland |
1.256*** |
1.017** |
-0.274 |
2.554*** |
0.0931 |
-0.0937 |
Israel |
0.965*** |
0.792*** |
-0.623* |
1.002*** |
0.897*** |
-0.581* |
Italy |
0.879*** |
0.932*** |
-0.429* |
1.054*** |
0.672** |
-0.433** |
Japan |
1.293*** |
0.677*** |
-0.00183 |
1.760*** |
0.649*** |
-0.0111 |
Korea |
2.631*** |
2.347*** |
-0.351* |
2.934*** |
2.164* |
-0.190 |
Lithuania |
1.132*** |
0.334 |
-0.632* |
1.177*** |
-0.0671 |
-0.523* |
Luxembourg |
1.007*** |
0.313** |
-0.197 |
1.022*** |
0.394** |
-0.250 |
Latvia |
0.880*** |
0.751*** |
-0.0755 |
0.740*** |
0.496** |
-0.129 |
Mexico |
1.007*** |
1.000*** |
-0.216 |
1.211*** |
0.622** |
-0.193* |
Netherlands |
0.759*** |
0.467 |
-0.404** |
0.613*** |
0.512 |
-0.344* |
Norway |
0.934*** |
0.236* |
-0.195 |
0.955*** |
-0.104 |
-0.167 |
New Zealand |
n/a |
n/a |
n/a |
n/a |
n/a |
n/a |
Poland |
0.971*** |
1.048*** |
-0.207 |
1.034*** |
0.798 |
-0.266 |
Portugal |
1.068*** |
0.953*** |
-0.0193 |
1.433*** |
0.931*** |
-0.00410 |
Slovak Republic |
0.937*** |
0.375* |
-0.0253 |
0.928*** |
0.376 |
-0.156 |
Slovenia |
1.005*** |
0.714*** |
0.0190 |
1.070*** |
0.511*** |
-0.0457 |
Sweden |
0.734*** |
1.365*** |
-0.0710 |
0.654*** |
1.192** |
-0.187 |
Türkiye |
1.106*** |
1.000*** |
-0.458** |
2.014*** |
0.998 |
-0.269 |
United States |
0.965*** |
0.988*** |
-0.222* |
0.974*** |
0.983*** |
-0.363*** |
Source: Authors’ calculations based on (OECD, 2022[17]), Revenue Statistics 2022; and (OECD, 2022[18]), OECD National Accounts.
Annex Table 2.A.5. Buoyancy of taxes on property by country, 1980-2021
Nominal |
Real |
|||||
---|---|---|---|---|---|---|
Long-run buoyancy |
Short-run buoyancy |
Speed of adjustment |
Long-run buoyancy |
Short-run buoyancy |
Speed of adjustment |
|
Australia |
1.012*** |
0.926* |
-0.269 |
1.130*** |
1.685* |
-0.293* |
Austria |
0.811*** |
0.307 |
-0.224 |
-0.169 |
0.421 |
-0.208 |
Belgium |
1.978*** |
1.414** |
-0.253* |
2.650*** |
1.437** |
-0.303* |
Canada |
1.008*** |
0.278 |
-0.447** |
1.200*** |
-0.214 |
-0.195* |
Switzerland |
0.903*** |
0.330 |
-0.172 |
0.869*** |
0.604 |
-0.125 |
Chile |
0.766*** |
0.554 |
-0.185 |
0.306* |
1.267* |
-0.332* |
Colombia |
1.134*** |
1.341** |
-0.608** |
2.860*** |
-0.734 |
-0.0834 |
Costa Rica |
1.247*** |
0.783 |
-0.308 |
1.826*** |
-0.00333 |
-0.391* |
Czechia |
0.874*** |
2.401* |
-0.404 |
0.838*** |
3.624** |
-0.351 |
Germany |
1.051*** |
0.696 |
-0.0748 |
0.911*** |
0.427 |
0.00501 |
Denmark |
0.948*** |
0.852 |
-0.417** |
0.770*** |
0.800 |
-0.413** |
Spain |
1.472*** |
1.128*** |
-0.271 |
1.802*** |
1.358** |
-0.251 |
Estonia |
0.599*** |
0.0888 |
-0.526* |
0.297* |
-0.282 |
-0.373 |
Finland |
1.176*** |
1.268*** |
-0.197 |
1.289*** |
1.228** |
-0.209 |
France |
1.398*** |
1.369*** |
-0.418** |
1.889*** |
1.070** |
-0.367** |
United Kingdom |
1.133*** |
0.568 |
-0.183 |
1.138*** |
1.793** |
-0.195 |
Greece |
1.317*** |
0.868** |
-0.541*** |
3.543*** |
0.478 |
-0.154 |
Hungary |
1.277*** |
1.194*** |
-0.402* |
2.636*** |
0.296 |
-0.361* |
Ireland |
1.077*** |
0.937** |
-0.0188 |
1.136*** |
0.654 |
-0.0225 |
Iceland |
0.764*** |
0.997*** |
-0.234 |
0.694*** |
0.0815 |
-0.291** |
Israel |
1.074*** |
1.458*** |
-0.903* |
1.068*** |
1.833*** |
-0.930* |
Italy |
1.714*** |
0.382 |
-0.300* |
3.506*** |
-1.211 |
-0.316* |
Japan |
1.431*** |
0.866** |
-0.111 |
1.222*** |
0.716* |
-0.169 |
Korea |
1.336*** |
1.267*** |
-0.312 |
1.314*** |
1.573*** |
-0.182 |
Lithuania |
0.612*** |
-0.146 |
-0.209 |
0.414*** |
-0.483 |
-0.236 |
Luxembourg |
1.215*** |
1.546** |
-0.126 |
1.307*** |
1.804*** |
-0.171 |
Latvia |
0.904*** |
0.618** |
-0.471 |
0.877*** |
0.590 |
-0.503 |
Mexico |
0.916*** |
0.895*** |
-0.296 |
2.076*** |
0.475 |
-0.313* |
Netherlands |
1.152*** |
0.764 |
-0.434** |
1.248*** |
1.058 |
-0.333** |
Norway |
1.035*** |
0.732*** |
-0.301** |
1.254*** |
0.770 |
-0.184* |
New Zealand |
0.920*** |
0.907*** |
-0.195* |
0.832*** |
0.684* |
-0.190* |
Poland |
0.808*** |
1.097*** |
-0.730* |
0.932*** |
0.377 |
-0.291* |
Portugal |
1.437*** |
1.375*** |
-0.733*** |
2.798*** |
1.590** |
-0.779*** |
Slovak Republic |
0.606*** |
-0.279 |
0.0277 |
0.353*** |
-0.707** |
-0.193 |
Slovenia |
0.808*** |
0.449 |
-0.458* |
0.650** |
0.472 |
-0.434* |
Sweden |
0.672*** |
2.489* |
-0.351** |
0.692 |
1.201 |
-0.418*** |
Türkiye |
1.045*** |
1.044*** |
-0.731*** |
1.678*** |
-0.691 |
-0.597*** |
United States |
1.075*** |
0.393 |
-0.905*** |
1.156*** |
0.156 |
-0.906*** |
Source: Authors’ calculations based on (OECD, 2022[17]), Revenue Statistics 2022; and (OECD, 2022[18]), OECD National Accounts.
Annex Table 2.A.6. Buoyancy of value added tax by country, 1980-2021
Nominal |
Real |
|||||
---|---|---|---|---|---|---|
Long-run buoyancy |
Short-run buoyancy |
Speed of adjustment |
Long-run buoyancy |
Short-run buoyancy |
Speed of adjustment |
|
Australia |
0.990*** |
0.342 |
-0.468 |
0.976** |
2.263* |
-0.450* |
Austria |
0.912*** |
1.010*** |
-0.873*** |
0.879*** |
0.862*** |
-0.909*** |
Belgium |
1.025*** |
1.220*** |
-0.356* |
0.987*** |
1.506*** |
-0.275 |
Canada |
1.425*** |
0.698 |
-0.517** |
1.878*** |
0.559 |
-0.348 |
Switzerland |
1.385*** |
0.611 |
-0.191 |
1.359*** |
0.973 |
-0.181 |
Chile |
1.033*** |
0.901*** |
-0.723** |
1.025*** |
1.453*** |
-0.811*** |
Colombia |
1.128*** |
1.472*** |
-0.491* |
1.387*** |
1.757*** |
-0.492** |
Costa Rica |
1.105*** |
1.186*** |
-0.636** |
1.025*** |
1.170 |
-0.550** |
Czechia |
1.290*** |
0.809** |
-0.366 |
1.505*** |
0.895** |
-0.615** |
Germany |
1.271*** |
1.372*** |
-0.163 |
1.512*** |
1.261*** |
-0.113 |
Denmark |
1.099*** |
0.906*** |
-0.366** |
1.110*** |
1.147*** |
-0.320* |
Spain |
1.242*** |
1.319** |
-0.374 |
1.436*** |
1.684** |
-0.244 |
Estonia |
1.084*** |
0.885*** |
-1.048** |
1.165*** |
0.950*** |
-1.042** |
Finland |
1.130*** |
1.214*** |
-0.181 |
1.268*** |
1.137*** |
-0.220* |
France |
0.921*** |
0.889*** |
-0.225 |
0.730*** |
1.070*** |
-0.185 |
United Kingdom |
1.162*** |
1.175*** |
-0.320* |
1.348*** |
1.493*** |
-0.254 |
Greece |
1.030*** |
1.026*** |
-0.317* |
1.361*** |
1.074*** |
-0.177 |
Hungary |
1.192*** |
0.882*** |
-0.533* |
1.454*** |
0.487 |
-0.604** |
Ireland |
0.883*** |
0.859*** |
0.0717 |
0.828*** |
0.519* |
0.0404 |
Iceland |
0.913*** |
0.760** |
-0.452** |
0.715*** |
1.338*** |
-0.327 |
Israel |
1.011*** |
0.883*** |
-0.618** |
1.007*** |
0.651* |
-0.407 |
Italy |
1.175*** |
1.215*** |
-0.717** |
1.535*** |
1.588*** |
-0.577** |
Japan |
6.607* |
1.580* |
-0.0442 |
5.973*** |
0.424 |
-0.179 |
Korea |
1.036*** |
1.091*** |
-0.394* |
0.982*** |
1.398*** |
-0.403* |
Lithuania |
1.030*** |
1.326*** |
-0.260 |
0.983*** |
1.321*** |
-0.290 |
Luxembourg |
1.125*** |
0.666* |
-0.207 |
1.235*** |
0.632 |
-0.263* |
Latvia |
1.088*** |
1.091*** |
-0.170 |
1.213*** |
1.345*** |
-0.157 |
Mexico |
1.072*** |
1.030*** |
-0.125 |
1.625*** |
1.110** |
-0.279* |
Netherlands |
1.047*** |
1.064*** |
-0.167 |
1.078*** |
1.103*** |
-0.139 |
Norway |
1.010*** |
0.366* |
-0.206 |
1.103*** |
1.126* |
-0.502** |
New Zealand |
1.181*** |
3.798*** |
-0.884*** |
1.420*** |
-1.180 |
-0.726*** |
Poland |
1.099*** |
1.199*** |
-0.305 |
1.206*** |
1.879*** |
-0.332 |
Portugal |
1.395*** |
1.381*** |
-0.765*** |
2.167*** |
1.674*** |
-0.627** |
Slovak Republic |
1.105*** |
0.892** |
-0.381 |
0.944*** |
0.764* |
-0.226 |
Slovenia |
1.244*** |
1.740*** |
-1.013*** |
1.396** |
1.695** |
-1.003*** |
Sweden |
1.191*** |
1.425*** |
-0.840*** |
1.523*** |
0.961** |
-0.276* |
Türkiye |
1.063*** |
1.139*** |
-0.250 |
1.435*** |
1.275*** |
-0.127 |
United States |
n/a |
n/a |
n/a |
n/a |
n/a |
n/a |
Source: Authors’ calculations based on (OECD, 2022[17]), Revenue Statistics 2022; and (OECD, 2022[18]), OECD National Accounts.
Annex Table 2.A.7. Buoyancy of excises by country, 1980-2021
Nominal |
Real |
|||||
---|---|---|---|---|---|---|
Long-run buoyancy |
Short-run buoyancy |
Speed of adjustment |
Long-run buoyancy |
Short-run buoyancy |
Speed of adjustment |
|
Australia |
0.667*** |
0.784* |
-0.103 |
0.255** |
0.509 |
-0.137 |
Austria |
0.759*** |
1.046** |
-0.179 |
0.725*** |
1.187*** |
-0.115 |
Belgium |
0.952*** |
1.063*** |
-0.189 |
1.040*** |
1.113*** |
-0.161 |
Canada |
0.494*** |
-0.0435 |
-0.486*** |
0.0353 |
-0.333 |
-0.454*** |
Switzerland |
0.716*** |
0.406 |
-0.277* |
0.641*** |
0.0711 |
-0.274 |
Chile |
0.799*** |
0.731** |
-0.497** |
0.492*** |
0.964* |
-0.314* |
Colombia |
0.976*** |
0.993*** |
-0.311 |
0.860*** |
1.676** |
-0.348* |
Costa Rica |
1.142*** |
1.606*** |
-0.0722 |
1.177*** |
2.834*** |
-0.131 |
Czechia |
0.985*** |
1.009* |
-0.379 |
1.136*** |
1.415** |
-0.278 |
Germany |
0.881*** |
1.188*** |
0.0698 |
0.933*** |
1.051** |
0.0533 |
Denmark |
0.813*** |
1.658*** |
-0.130 |
0.567** |
2.404*** |
-0.107 |
Spain |
1.380*** |
1.371*** |
-0.0600 |
1.719*** |
1.074** |
-0.0725 |
Estonia |
1.157*** |
0.395 |
-1.109** |
1.404*** |
0.0780 |
-1.114** |
Finland |
0.772*** |
0.718*** |
-0.312* |
0.569*** |
0.567* |
-0.424* |
France |
0.838*** |
0.903*** |
-0.218* |
1.098*** |
0.672* |
-0.0503 |
United Kingdom |
0.685*** |
0.646*** |
0.0328 |
0.440*** |
0.761*** |
-0.0290 |
Greece |
0.862*** |
1.003*** |
-0.227* |
0.573** |
0.161 |
-0.355** |
Hungary |
0.738*** |
0.949*** |
-0.475 |
0.375*** |
0.802** |
-0.0290 |
Ireland |
0.554*** |
0.564*** |
0.0492 |
0.293*** |
0.418* |
-0.0604 |
Iceland |
1.295*** |
-0.179 |
-0.233 |
2.022*** |
0.848 |
-0.156 |
Israel |
1.161*** |
0.896 |
0.220 |
1.219*** |
0.836 |
0.245 |
Italy |
0.564 |
1.374*** |
-0.245* |
1.377** |
1.020* |
-0.168 |
Japan |
0.238 |
0.519 |
-0.372* |
0.266 |
0.700 |
-0.638** |
Korea |
1.048*** |
1.328*** |
-0.0926 |
1.022*** |
1.281*** |
-0.0933 |
Lithuania |
0.907*** |
0.596*** |
-0.471* |
0.798*** |
0.405 |
-0.430 |
Luxembourg |
0.935*** |
1.011** |
-0.0391 |
0.781*** |
1.045* |
-0.0347 |
Latvia |
0.995*** |
0.618*** |
-0.648** |
0.993*** |
0.271 |
-0.616** |
Mexico |
1.033*** |
0.832*** |
-0.154 |
0.186 |
-0.114 |
-0.149 |
Netherlands |
1.247*** |
1.138*** |
-0.167 |
1.431*** |
1.403*** |
-0.134 |
Norway |
0.532*** |
0.599** |
-0.498*** |
0.0410 |
1.495** |
-0.429** |
New Zealand |
0.109 |
1.980*** |
-0.232* |
-0.621*** |
0.217 |
-0.291* |
Poland |
0.984*** |
0.986*** |
-0.540* |
1.121*** |
1.011 |
-0.296 |
Portugal |
1.033*** |
1.269*** |
-0.214 |
0.697*** |
0.963* |
-0.237 |
Slovak Republic |
1.083*** |
0.943* |
-1.117** |
1.108*** |
1.039* |
-1.113*** |
Slovenia |
1.258*** |
1.457* |
-0.753** |
1.858*** |
0.758 |
-0.750** |
Sweden |
0.550*** |
0.800*** |
-0.305** |
0.188** |
0.398 |
-0.407** |
Türkiye |
1.076*** |
1.125** |
-0.278 |
3.366*** |
-0.681 |
-0.156* |
United States |
0.665*** |
0.176 |
-0.429** |
0.374*** |
0.0380 |
-0.510*** |
Source: Authors’ calculations based on (OECD, 2022[17]), Revenue Statistics 2022; and (OECD, 2022[18]), OECD National Accounts.
Annex Table 2.A.8. Buoyancy of real tax revenues by tax type using MG estimator, 1980-2021
Long-run buoyancy |
Short-run buoyancy |
|||||
---|---|---|---|---|---|---|
Mean |
Median |
Std. |
Mean |
Median |
Std. |
|
Total |
1.101 |
1.050 |
0.212 |
1.066 |
1.009 |
0.348 |
PIT |
1.210 |
0.894 |
1.074 |
1.149 |
0.877 |
0.821 |
CIT |
1.712 |
1.608 |
0.741 |
2.970 |
2.756 |
1.586 |
SSC |
1.165 |
1.051 |
0.511 |
0.769 |
0.661 |
1.482 |
Property |
1.383 |
1.156 |
0.837 |
0.991 |
0.785 |
0.757 |
VAT |
1.372 |
1.235 |
0.824 |
1.182 |
1.142 |
0.419 |
Excises |
0.902 |
0.829 |
0.667 |
0.978 |
0.964 |
0.565 |
Note: Real tax revenues are calculated by dividing nominal tax revenues from Revenue Statistics database with GDP deflator from OECD National Accounts database. Under Mean Group estimator, tax buoyancies of individual OECD countries are estimated first. Then simple averages are calculated after removing outliers (e.g. estimates that are statistically insignificant and close to zero or negative). Detailed information of real tax buoyancies for each OECD country is provided in the Annex at the end of this special feature.
Source: Authors’ calculations based on (OECD, 2022[17]), Revenue Statistics 2022; and (OECD, 2022[18]), OECD National Accounts.
Annex Table 2.A.9. Buoyancy of real tax revenues by tax type using PMG estimator, 1980-2021
Long-run |
Short-run |
Speed of adjustment |
|
---|---|---|---|
Total |
1.026*** |
1.052*** |
-0.159*** |
PIT |
0.846*** |
0.920*** |
-0.178*** |
CIT |
1.399*** |
3.059*** |
-0.287*** |
SSC |
1.005*** |
0.709*** |
-0.180*** |
Property |
1.073*** |
0.793*** |
-0.153*** |
VAT |
1.132*** |
1.238*** |
-0.275*** |
Excises |
0.201*** |
0.644*** |
-0.168*** |
Note: *, ** and *** refer to statistical significance at the 10%, 5% and 1% levels respectively. Real tax revenues are calculated by dividing nominal tax revenues from Revenue Statistics database with GDP deflator from OECD National Accounts database. Under Pooled Mean Group estimator, tax buoyancies are estimated based on the panel data of 38 OECD countries between 1980 and 2021. It combines both pooling and averaging so tax buoyancies of individual countries are not available.
Source: Authors’ calculations based on (OECD, 2022[17]), Revenue Statistics 2022; and (OECD, 2022[18]), OECD National Accounts.
Annex Table 2.A.10. Buoyancy of real tax revenues by tax type over 1980-1999, 2000-2010 and 2011-2021
Long-run buoyancy |
Short-run buoyancy |
|||||
---|---|---|---|---|---|---|
1980-1999 |
2000-2010 |
2011-2021 |
1980-1999 |
2000-2010 |
2011-2021 |
|
Total |
1.292*** |
0.989*** |
1.135*** |
0.893*** |
1.426*** |
1.045*** |
PIT |
0.771*** |
0.719*** |
1.620*** |
1.080*** |
1.339*** |
0.881*** |
CIT |
2.417*** |
1.431*** |
1.968*** |
2.967*** |
4.280*** |
3.166*** |
SSC |
1.117*** |
1.063*** |
1.300*** |
0.833* |
0.648** |
0.410** |
Property |
1.292*** |
0.668*** |
0.984*** |
0.788* |
1.177*** |
0.528* |
VAT |
0.611*** |
1.046*** |
1.240*** |
1.316*** |
1.714*** |
1.186*** |
Excises |
0.199** |
0.349*** |
0.558*** |
0.677* |
0.400 |
0.979*** |
Note: *, ** and *** refer to statistical significance at the 10%, 5% and 1% levels respectively. Figures are estimated using Pooled Mean Group estimator. Under Pooled Mean Group estimator, tax buoyancies are estimated based on the panel data of 38 OECD countries during different time periods. Real tax revenues are calculated by dividing nominal tax revenues from Revenue Statistics database with GDP deflator from OECD National Accounts database. Slovenia is dropped for VAT and excises estimation over 1980-1999 due to insufficient data.
Source: Authors’ calculations based on (OECD, 2022[17]), Revenue Statistics 2022; and (OECD, 2022[18]), OECD National Accounts.
Annex Figure 2.A.1. Belgium: income tax revenues and GDP during the Global Financial Crisis and the COVID-19 crisis
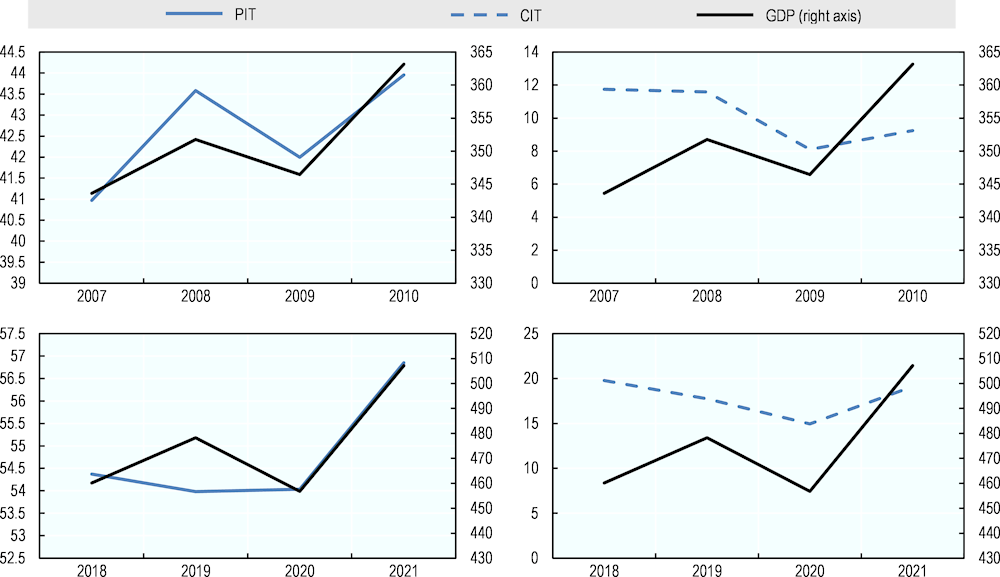
Source: Authors’ calculations based on (OECD, 2022[17]), Revenue Statistics 2022; and (OECD, 2022[18]), OECD National Accounts.
Annex Figure 2.A.2. France: income tax revenues and GDP during the Global Financial Crisis and the COVID-19 crisis
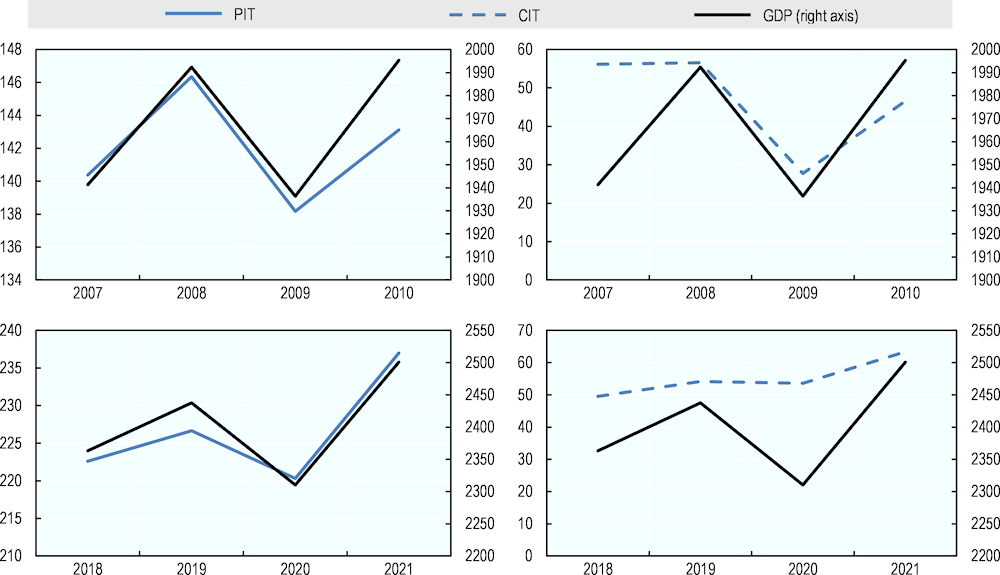
Source: Authors’ calculations based on (OECD, 2022[17]), Revenue Statistics 2022; and (OECD, 2022[18]), OECD National Accounts.
Annex Figure 2.A.3. Japan: income tax revenues and GDP during the Global Financial Crisis and the COVID-19 crisis
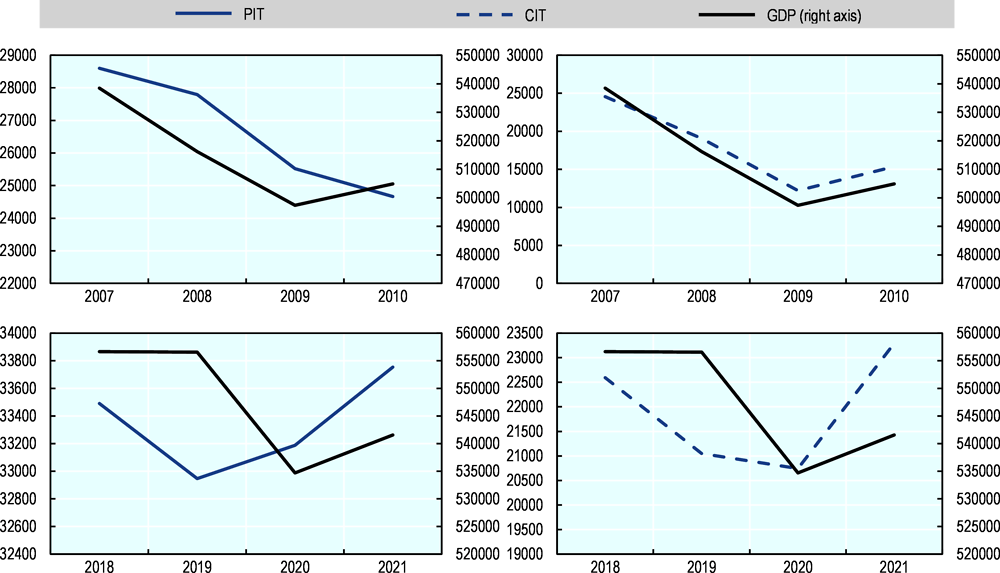
Source: Authors' calculations based on (OECD, 2022[17]), Revenue Statistics 2022; and (OECD, 2022[18]), OECD National Accounts.
Annex Figure 2.A.4. Switzerland: income tax revenues and GDP during the Global Financial Crisis and the COVID-19 crisis
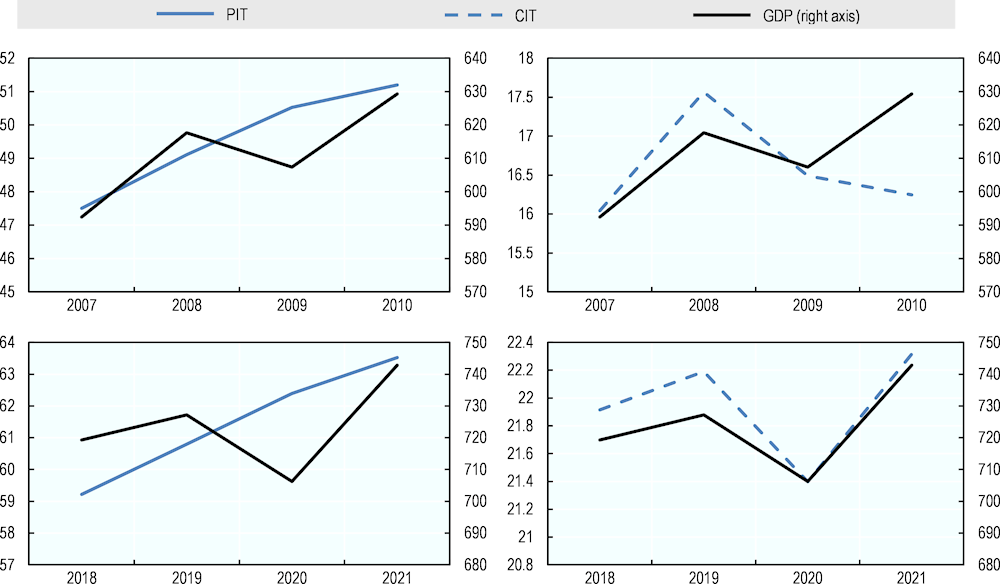
Source: Authors' calculations based on (OECD, 2022[17]), Revenue Statistics 2022; and (OECD, 2022[18]), OECD National Accounts.
Annex Figure 2.A.5. United States: income tax revenues and GDP during the Global Financial Crisis and the COVID-19 crisis
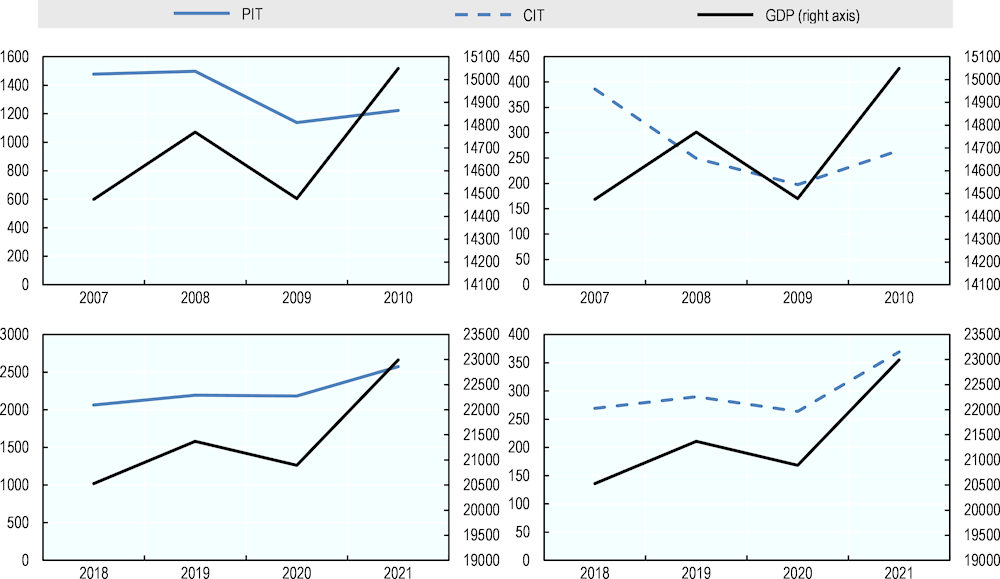
Source: Authors' calculations based on (OECD, 2022[17]), Revenue Statistics 2022; and (OECD, 2022[18]), OECD National Accounts.
Annex Figure 2.A.6. Buoyancy of real tax revenues by tax type, 1980-1999, 2000-2010 and 2011-2021
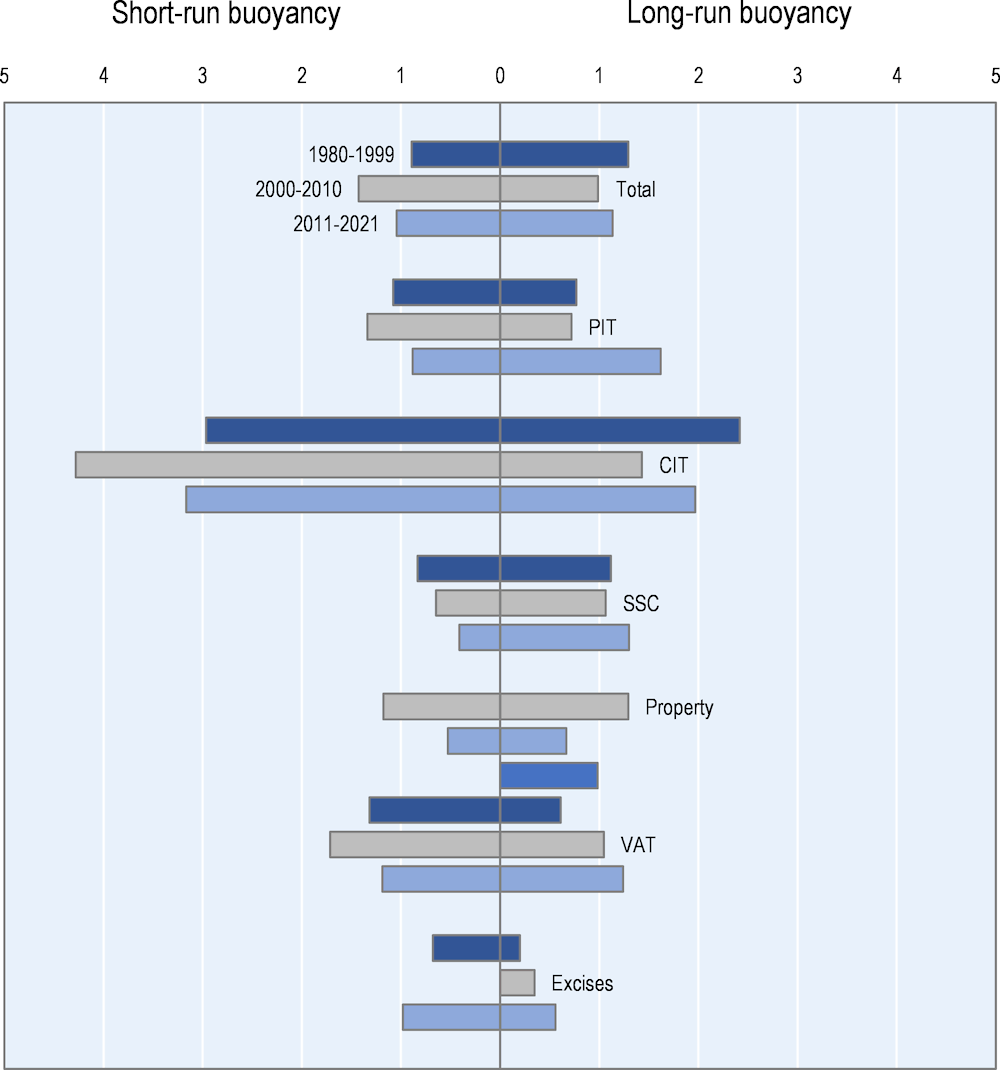
Note: Figures are estimated using Pooled Mean Group estimator. Under Pooled Mean Group estimator, tax buoyancies are estimated based on the panel data of 38 OECD countries during different time periods. Real tax revenues are calculated by dividing nominal tax revenues from Revenue Statistics database with GDP deflator from OECD National Accounts database. Slovenia is dropped for VAT and excises estimation over 1980-1999 due to insufficient data.
Source: Authors’ calculations based on (OECD, 2022[17]), Revenue Statistics 2022; and (OECD, 2022[18]), OECD National Accounts.
Notes
← 1. Other estimation methods for tax elasticity include introducing dummy variables to represent tax policies, keeping tax policy parameters (e.g. tax rate and tax base) unchanged and using the Divisia index. When reliable and sufficient information is available, the Proportional Adjustment method produces the best results as it can take into account large and small discretionary policy changes without bias.
← 2. (Dougherty, de Biase and Lorenzoni, 2022[9]) use an auto-regressive distributed lag model estimated with Ordinary Least Squares in a one-step approach to obtain long-run tax buoyancy in OECD countries across revenue type and level of government. (Cornevin, Corrales and Angel, 2023[10]) use a one-step ECM estimated with three “generation” estimators. They define the Mean Group estimator and Pooled Mean Group estimator as “first generation” estimators; the common correlated effects estimators, which control for cross-sectional dependence, are defined as “second generation” estimators, and the dynamic common correlated effects estimators are the “third generation” estimators.
← 3. These two estimators are also used in (Belinga et al., 2014[6]), (Dudine and Jalles, 2017[7]), (Deli et al., 2018[8]) and (Cornevin, Corrales and Angel, 2023[10]).
← 4. While tax data of some OECD countries starts from 1965, the starting year of the analysis is set to 1980 to reduce missing data while maintaining a sufficiently large sample.
← 5. These countries are Estonia, Israel, Lithuania, Latvia, Slovak Republic and Slovenia.
← 6. Standard deviation is used to measure dispersion of data around its mean.
← 7. In 2016, Iceland received revenues from one-off stability contributions from entities that previously operated as commercial or savings banks and were concluding operations. These one-off stability contributions amounted to about 15.7% of Iceland’s GDP in 2016, leading to unusually high tax revenues for that year.
← 8. In this Special Feature, “close to unity” is defined as tax buoyancy falling roughly between 0.9 and 1.1, "above unity" is defined as tax buoyancy larger than 1.1, and “below unity” is defined as tax buoyancy smaller than 0.9 for the sake of simplicity.
← 9. This is consistent with findings elsewhere in the literature, for example (Belinga et al., 2014[6]); (Dudine and Jalles, 2017[7]); (Deli et al., 2018[8]); (Dougherty, de Biase and Lorenzoni, 2022[9]); (OECD, 2022[34]); and (Cornevin, Corrales and Angel, 2023[10]).