Dorothy Adams
Modernising Access to Social Protection
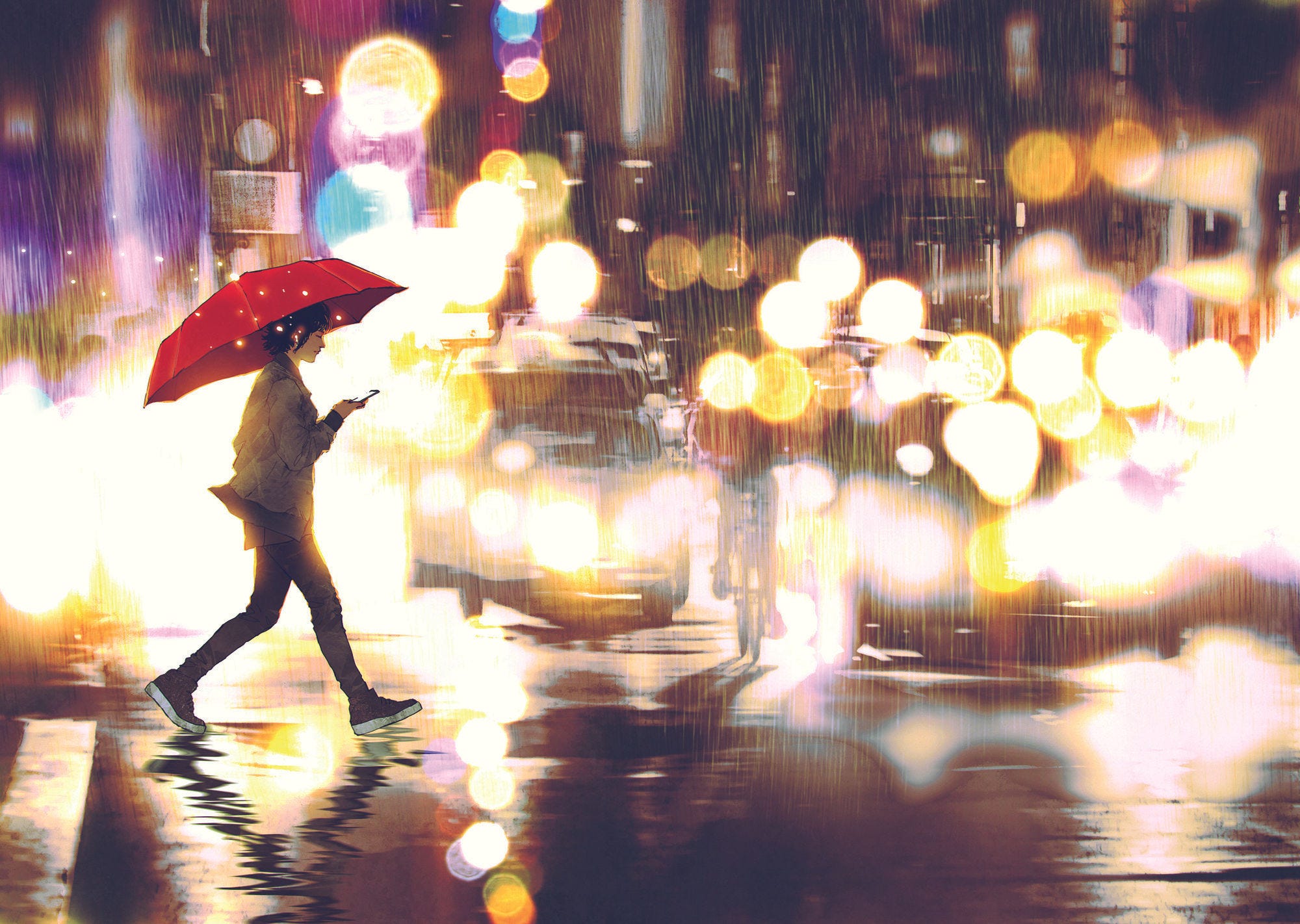
4. Leveraging technology and data advances to improve social programme coverage and service delivery
Abstract
This chapter explores the different approaches OECD countries are taking with advanced digital technologies and data to help improve the take up of social protection programmes and to make service provision more efficient. Technologies like websites, portals and apps are now commonly used to make it easier for people to learn about, apply for, and interact with government services. Advances in data collection and use sit at the heart of governments’ increased reliance on technology. Government agencies are progressively linking their administrative databases to assess benefit eligibility automatically, adjust benefits and, in some cases, automatically enrol service users into social programmes. So far, deployment of more advanced technologies like AI and ground-breaking uses of data tend to be small-scale, ad hoc projects to determine feasibility and scope of deployment. This is related to the significant risks and challenges associated with the use of advanced technologies and data in social protection, which are discussed in Chapter 5 of this report.
Key Findings
OECD countries are leveraging rapid advances in technology and data to improve the design, delivery and coverage of social protection programmes. This chapter explores the different approaches countries are taking, and to what effect. The literature and countries’ responses to the OECD’s questionnaire: Harnessing Technology and Data to Improve Social Protection Coverage and Social Service Delivery (OECD, 2023[1]), hereafter known as the OECD Questionnaire, offer clear and consistent themes:
Many OECD countries are moving in a similar and progressive direction, relying increasingly on technology and data to enhance the customer experience of public services, improve efficiency (for example, pre‑filling application forms) and to ensure groups in vulnerable situations are aware of, and accessing, the benefits and services for which they are eligible.
Digitalised welfare systems are starting to change the nature of the bureaucratic encounter between the state and individuals. More services are now available online, enabling welfare agencies to focus resources on people with needs that are not suited to automated systems, such as service users with complex needs who require multiple and intensive social protection services.
Better and smarter use of data sits at the heart of governments’ increased reliance on digital technologies to improve social protection. For example, countries are increasingly linking administrative data to measure non-take‑up, make information more readily available, and lower the administrative burden on claimants, for example, by automatically assessing eligibility for benefits and services and/or to adjusting benefits where there has been a change in circumstances. In their most advanced form, data linkages are used to enable automatic enrolment of service users into benefit programmes. At least three countries now grant child benefits automatically upon the birth of a baby (Estonia, Norway and the Slovak Republic), and Canada grants some minimum pension benefits automatically.
While greater use is being made of government data, government agencies are yet to exploit, in a systematic way, the value of data from non-governmental sources to understand and shape social policy and services.
Despite progress in the government sector, advanced uses of technology and data are less common in the public sector than in the private sector, and less common again in the social sector. Reasons for this include ethical and legal concerns – not least of all related to privacy – and scepticism about whether computer-driven systems are appropriate in the sphere of public policy and administration, and social policy in particular.
There is a gap between the examples of leading-edge uses of advanced technology and data in the literature and those provided by countries via the OECD questionnaire. This suggests that many advanced uses of technology, and AI particularly, continue to be small, ad hoc test cases to determine feasibility, functionality and scope of deployment. Countries are thinking carefully about how to take advantage of new technologies and proceeding with caution, implementing and monitoring small scale projects before determining whether to take them to scale. Several countries however provided examples of comprehensive change programmes that involve modernising their technology platforms, changing operating models and ensuring the necessary cultural shifts to revolutionise how public services are provided.
The use of AI in social protection remains limited, and – thus far – other statistical methods remain more common in automated decision-making and data analytics. One exception is the use of AI-powered chatbots, which many countries are now implementing.
4.1. Introduction
The ability of a country to care for its people and respond to their needs over the life course depends on its ability to identify those who are in need, enrol them in tailored benefits and services that work, and follow up to cater to evolving circumstances. This requires having in place a modern and well-functioning national social protection system.
Understanding the demand for social protection and better co‑ordination and monitoring of the supply of social protection programmes and operational decision-making requires the collection, processing, storing, flow (within the social protection sector and between the social protection and other sectors) and use of data. Governments also need to be able to monitor programme impacts and track and adequately plan expenditure. These actions require dynamic and real-time data and information exchange if the goal of universal social protection coverage is to be achieved (Chirchir and Barca, 2020[2]).
Across different levels of government in OECD countries, advances in data and technology are being leveraged to improve the design, delivery and coverage of social protection programmes. This chapter first explores what advanced technologies countries are adopting to increase the uptake of social protection such as automatic assessment, enrolment, and adjustment of benefits, and what they have learned from their experiences to date.
Second, the chapter outlines how countries are improving social protection design and delivery through the better use of data including use of new data sources. Advances in technology go hand in hand with advances in data collection and use. Finally, the chapter describes how some countries are taking advantage of massive strides in AI technologies while managing the challenges of doing so. While adoption of AI techniques in the public sector remains limited, advocates argue that AI could radically improve the efficiency and quality of public service delivery (see Chapter 1 of this report and (Verhagen, forthcoming[3])), in areas such as education, healthcare and social protection.
While technology and data advances have delivered and continue to promise significant strides in productivity, services for the previously excluded, and entirely new offerings, they also raise complex, contemporary ethical issues. The challenges associated with increased use of technology and data in social protection and the steps governments are taking to ensure their safe, ethical and equitable use are discussed in Chapter 6. Concerns include digital advances posing risks to numerous professions, threatening privacy, and making people increasingly dependent on automated processes that they find opaque and unaccountable (OECD, 2023[4]; Griffiths, 2021[5]). There are implications for human rights and human agency. Furthermore, digital technologies potentially widen socio-economic inequalities by disproportionally benefiting some people over others, typically those who are already disadvantaged.
4.2. Advanced technology use
Definitions of technology vary, from very narrow to broad and encompassing. Simply put, technology is the application of scientific knowledge for practical purposes, to solve problems and make people’s lives easier and more productive. Technology also refers to the resulting tangible (hardware) and intangible (software) products, systems, services and infrastructure.
The focus of this report is the use of digital technology in the field of social protection. Digital technology is the creation and practical use of digital or computerised devices, methods and systems that help to create, store, analyse and share information; it can also refer to the use of algorithms or applications to analyse and, ideally, solve a problem. Digital technologies are dynamic and constantly evolving and have had a profound impact on how people live their lives, with most people using some form of technology every day, to communicate, to learn and to work.
Most businesses use digital technology to manage operations and processes and to enhance the customer experience. Governments worldwide are also taking advantage of the digital wave to improve public services for individuals. Recognising that today’s technology is not only a strategic driver for improving public sector efficiency but that it can also support policy effectiveness and create more open, transparent, innovative, participatory and trustworthy governments, the OECD’s Recommendation in Digital Government Strategies aims to support digital government strategies that bring governments closer to citizens and businesses (OECD, 2014[6]).
Expectations of public services have changed, and people increasingly want (or expect) from government the same level of service that they receive in the private sector, such as streamlined transactions, increased transparency, new ways of approaching problems, and more personalised interactions. At the same time, demand for social protection is increasing. The Global Financial Crisis and COVID‑19 resulted in more (and new) people demanding social support. These shocks – in combination with longer-term megatrends like population ageing and shifts away from dependent employment – have squeezed government resources. Governments are therefore understandably looking for innovative and cost-effective digital solutions to improve the efficiency and effectiveness of public services as well as service user satisfaction.
4.2.1. Websites, portals and apps to help individuals learn about and apply for benefits
Perhaps the most common technology employed by governments in social protection is the use of websites, portals and applications to make it easier for people to learn about, apply for, and interact with government services. In Canada for example, where advancements in service digitalisation have accelerated in the past five years, secure on-line service portals which allow Canadians to access a variety of social protection programmes have been created, including tools such as My Service Canada, Digital Government and Service NL, Service ON, the City of Toronto’s Service and Benefit Finder Tool and CRA My Account.
Japan has implemented Mynaportal which provides individuals with information about services they can receive by using their My Number Card (an identification card). Individuals can, via Mynaportal view records of medical treatments, medications, and medical expenses paid at clinics. The portal is also linked to an online service where individuals can check their public pension records and estimates of future pension amounts. It is also possible to register a bank account for the receipt of public allowances, simplifying emergency allowance application processes.
Türkiye’s Ministry of Family and Social Services provides a simple information sheet on its website, that is regularly updated and includes information on available benefits, including who can apply, how much you would receive, what documents are required to apply and the benefit payment schedule. When someone visits a social assistance office, a government officer can quickly check what benefits they are entitled to using the centralised Integrated Social Assistance Service Information System. Most applications can be made via e-Devlet, which makes all information stored visible to residents.
The United States reports using a variety of digital tools and different data sources to improve the coverage and delivery of social protection programmes at various levels of government – federal, state, and local. The website “Benefits.gov” is the official benefits website of the United States federal government (Box 4.1). It serves as a one‑stop shop for citizens seeking benefits and resources. Prior to Benefits.gov, citizens looking for government benefit information had to search through a complicated maze of web pages hosted by different agencies; there was no easy-to‑use, single source of benefit information to help citizens understand what benefit programmes they may be eligible for, or how to apply. Today, millions of citizens as well as businesses and Federal and state government entities have easy, online access to information from across multiple Federal agencies.
At the state level, First 5 South Carolina (First Five SC) responds to a the United States Government priority for early childhood (parent knowledge building) and a common eligibility system connects families with South Carolina’s public services for young children (Box 4.2). A centralised website checks eligibility for over 40 services and allows families to apply online. Service categories include early childcare and early education; health and safety; special needs and early intervention; food and nutrition; and parenting and family support.
Box 4.1. Web-based technology to improve access to social protection and services in the United States
Benefits.gov
Benefits.gov (formerly GovBenefits.gov) was launched in April 2002 as part of the Office of Management and Budget’s E-Government Strategy plan. The site was one of 24 initiatives designed to make government services more streamlined and accessible to the public. Benefits.gov was charged with creating a website to simplify the benefits search process and provide greater transparency to citizen users, while reducing redundancy across government.
Each of the Benefits.gov partners entered a memorandum of understanding with the Department of Labor (DOL) agreeing to provide governance, benefit information, and funding to support the programme. Benefits.gov shares monthly data on key performance indicators for each of the Federal partner agencies and their portfolio of benefits on the site. This data includes top benefit views, citizen feedback comments and trends, top referrals, usage by state and top views by agency. Benefits.gov implemented an annual content review cycle consisting of monthly data calls to programme owners, which ensures the quality and accuracy of content through timely reviews of state and federal information.
The Benefits.gov content strategy focuses on a combination of translating legal-speak to plain language and informing the end user of what to expect and what they will need when making an application, to ensure a better-informed applicant before referring them to the agency website to complete the forms. Benefits.gov manages the complexity of content reviews by following an established data call process to review and update benefit programme content to maintain accuracy. Benefits.gov can publish content updates to the site within hours of a request when immediate changes are needed and manages ad hoc content updates throughout the year.
Benefits.gov leverages metrics and customer satisfaction data to better understand customer needs and where to continue to evolve and directly meet those needs. The overall Benefits.gov score in the last year was 63 out of 100, which is aligned with government’s overall score, according to American Customer Satisfaction Index (ACSI).
Four key goals of the programme guide the strategic plan and are evaluated annually. One key goal is to Inform Citizens, which includes raising awareness of the site and activities like content curation, publishing, and outreach services via Benefits.gov, its sister sites (GovLoans and SSABest), newsletter campaigns and social media channels to help disseminate information to potential beneficiaries and drive traffic to agency resources. As a result of outreach efforts and campaigns in FY2022, the programme saw 64% growth in social media and 14% growth in email subscriptions to benefit programme content.
First Five SC
The US state of South Carolina has a mixed service delivery system for early childhood, with over 60 programmes and services at 10 public agencies. It was difficult for families to learn about programmes available for parents and young children, as well as to enrol and participate. A statewide needs assessment and gap analysis showed that a technology solution would help meet the needs of families to find information in a centralised manner, learn about potential eligibility for programmes, and apply.
Ten agencies participate in First Five SC. Partner agencies can offer their services using contact information provided by families via the eligibility screener. Data that are provided by families may be retrieved by partner agencies either through a secure link or through data integration with a web service. Families must provide consent for both levels of information sharing. While individual agencies can retrieve data from First Five SC, data cannot be shared between partner agencies.
The data submitted via First Five SC and shared with agencies are subject to relevant data governance structures and kept in accordance with state and federal requirements for data security. During testing, potential users and the SC Family Voice Council were asked about perceptions and family beliefs about security and their views were reflected in the final two‑step consent process (i.e., for the eligibility screener and for the application process).
Source: (OECD, 2023[1]).
4.2.2. Automated assessment, enrolment and adjustment of benefits
Automated systems in social protection in OECD countries include assessing eligibility for benefits and services, determining benefit levels, and adjusting benefits where there has been a change in an individual’s circumstances. This can lessen the frequency of over- and underpayments, and link income support more closely to labour supply. Low-income households are typically liquidity constrained and may not respond to work incentives if benefit pay-outs are too far in the future, especially if taking up work/increasing working hours is associated with costs (such as transport or childcare (Hyee and Immervoll, 2022[7])).
Automated systems can also enable governments to adjust support quickly in response to changing macroeconomic conditions; for example, providing work incentives and activation support is more important and effective, in tight labour markets. The experience of the COVID‑19 pandemic shows that broadly accessible social protection programmes can be insufficiently responsive to needs on the ground, and responsive programmes can be inaccessible.
The New Zealand Government uses automated decision-making to adjust financial assistance for a client due to a change in personal circumstances and to grant benefits in some, limited cases such as the granting of the Winter Energy Payment which is universally available to people receiving certain benefits and pensions and does not require an application form.
Automatic enrolment of service users into benefit programmes for which they are entitled, without the need for an application, is less common. Automatic enrolment is however increasingly used in granting of child benefits to new parents – perhaps related to the ease of identifying new children at birth in hospitals. In the Slovak Republic a new childbirth allowance is provided proactively on the birth of a child without any participation by the family in the process. In Norway too, a child support benefit is automatically paid to all those eligible giving birth in a Norwegian hospital. Estonia is using technology and linked information to make e‑offers for family benefits, avoiding the need for families to have to apply. Once the birth of a child is registered in the Population Register a benefits offer can be found in the self-service portal of the Social Insurance Board within a week (see Proactive Family Benefits (Observatory of Public Service Innovation, 2020[8])).
Box 4.2. What is an automated system?
Automated systems can automate part or all of an administrative decision-making process e.g., they can execute a decision, or recommend a decision, or provide preliminary assessments and/or automate aspects of a fact-finding process which may influence subsequent decisions. They range from traditional rules-based systems (e.g., a system which calculates a rate of benefit payment in accordance with a formula set out in legislation) through to more specialised systems which use automated tools to predict and decide. The key feature of such systems is the use of pre‑set logical parameters to perform actions, or make decisions, without the direct involvement by a human being at the time of decision (Commonwealth Ombudsman, 2019[9]).
The European Law Institute (ELI), when developing Model Rules to supplement European legislation on AI, defined an “Algorithmic Decision-Making System” as a computational process, including one derived from machine learning, statistics, or other data processing or artificial intelligence techniques, that makes a decision, or supports human decision-making used by a public authority (European Law Institute, 2022[10]).
While use of automated decision-making by public authorities is rapidly increasing, fully automated decision-making resulting in a decision that may have a significant impact on a person’s life is not permitted in a number of countries or at least requires additional safeguards to be enshrined in legislation. For example, Article 22 of the EU’s GDPR enshrines a right not to subject an individual to a decision “based solely on automated processing” which has a legal or similarly significant effect on that individual (Sebastião Barros Vale and Gabriela Zanfir-Fortuna, 2022[11]). Arguably, Article 22 would require meaningful human involvement in many social protection processes and decisions in EU countries.
Canada’s Old Age Security Act was amended in 2012 to include an automatic enrolment regime that eliminates the need for many seniors to apply for Old Age Security (OAS) benefits, which reduces the burden for seniors to complete applications (Box 4.3). Prior to the change, all seniors had to submit an initial application for OAS benefits and provide all the necessary income and marital status information to determine eligibility for the means-tested Guaranteed Income Supplement (GIS). Now, seniors are automatically enrolled for the OAS pension where there is sufficient data (e.g. age and income tax data) to determine their eligibility. The latest available data show that close to half of all new OAS pensioners receive their pension without the need to fill in an application form. Furthermore, the initiative helped to generate efficiencies in the processing of OAS and GIS benefits.
Box 4.3. Auto‑enrolment for the Old Age Security pension and the Guaranteed Income Supplement in Canada
The Old Age Security (OAS) programme is the first pillar of the Canada’s retirement income system and is funded out of general tax revenues. It is a non-contributory, residence‑based programme that ensures a minimum income for seniors. These benefits serve as a foundation upon which seniors can add income from other sources such as employer-sponsored pension plans and personal savings and investments.
The benefits under the OAS programme include the basic OAS pension, which is paid to all persons aged 65 or over who meet the legal status and residence requirements, the Guaranteed Income Supplement (GIS) for low-income seniors who are recipients of the OAS pension, and Allowances for low-income Canadians aged 60 to 64 who are the spouses or common-law partners of GIS recipients, or who are widows or widowers.
Ensuring take‑up of OAS benefits is a top priority for the Government of Canada. The current take‑up rate for the OAS pension is estimated to be 96.8%, while take‑up for the GIS is estimated to be 92.2% (figure available only for tax‑filers). Clearly there remains some seniors who are eligible for the GIS but do not receive it.
In 2012, the Old Age Security Act was amended to include an automatic enrolment regime that eliminates the need for many seniors to apply for OAS benefits. This initiative, which started in 2013, reduces the burden for seniors of completing paper applications. The department automatically enrols seniors for the OAS pension where there is sufficient data to determine their eligibility. The latest available data show that close to half of all new OAS pensioners receive their pension without the need to fill in an application.
Over the years, automatic enrolment has been expanded to include the GIS. This measure ensures that all new pensioners who are automatically enrolled for the OAS pension will be assessed every year to determine their entitlement to the GIS.
Individuals who turn 64 may be selected for automatic enrolment for the OAS pension (and the GIS) without having to complete an initial application. These clients receive a letter notifying them that they will be enrolled for both the OAS pension and the GIS without the need to apply and will receive benefits automatically, if eligible, the month after they turn 65. Once automatically enrolled, clients will be automatically considered for the GIS each year, in July, provided they file their income tax return on time.
For the remaining half of new OAS pensioners, the government does not have sufficient information for automatic enrolment. For these individuals, there remains the need for an application. However, to help reduce their administrative burden, seniors now only need to file one application for both the OAS pension and the GIS.
Source: (OECD, 2023[1]).
Automatic enrolment can also make income support benefits more responsive to evolving needs. Recent crises, such as the COVID‑19 pandemic, have shown that income support needs can emerge suddenly, and overwhelm benefit infrastructures based on careful assessments of current incomes or prior contribution histories. High frequency data on income that is linked to the agencies administering benefits can also enable close‑to-real-time benefit adjustments according to claimants’ fluctuating income. By way of example, during COVID‑19 those who were registered in Türkiye’s Integrated Social Assistance Service Information System received a cash benefit automatically, without needing to apply for it.
In response to the energy crisis the City of Vienna created three energy support measures. One measure – the energy cost allowance did not require an application. Data were retrieved from internal data sources and linked with data from co‑operation partners such as the labour market service and housing assistance. These linked data were then entered into the City of Vienna’s system and benefits were paid automatically. Once someone’s data were in the system, further payments such as the energy bonus could also be paid automatically if their circumstances (income, number of people in the household, etc.) did not change. If circumstances did change, letters including passwords were sent to households who were asked to re‑enter their data.
France has a comprehensive, staged reform programme underway to improve the delivery of benefits administered by Caisse nationale des allocations familiales (CNAF, National Fund for Family Allocations) by 2027. The CNAF administers a total of 19 family, housing, and social inclusion benefits (CNAF, 2023[12]). The reform seeks to, amongst other things automate the retrieval of income information from administrative data for continuing claims; automate means testing; pre‑fill claims forms with income information to simplify the claims process for claimants; and create a single application form for all benefits. The first step, in 2023 was to improve the reliability of income data and the automatic retrieval of income information for means-testing for the housing benefit. Once this has occurred means-testing for the Prime d’activité (an in-work benefit for low-income workers) should occur automatically for all recipients of housing benefits (to check their entitlement to the Prime d’activité). Automatic implementation of the means-test for the Prime d’activité as well as the Social Assistance benefit Revenue de solidarité active for recipients of all benefits administered by CNAF to determine their entitlement will follow. The aim is that by 2027 there will be a single application form for all benefits administered by CNAF, including for claimants who are not already in CNAF’s database.
In Korea, National Tax Service and social insurance assessments data are linked to facilitate the periodic and consistent determination of income/earnings, including through a shortened income declaration cycle for applicable income tax filings. Furthermore, to ensure a more accurate assessment of the incomes of dependent contractors and platform workers, reporting obligations have been strengthened for businesses who contracted with them, as well as for labour matching platforms (OECD, 2023[13]).
4.2.3. Raising awareness of benefits and services
Digital tools and modern communication channels (e.g., social media and text messaging) – often coupled with new insights from behavioural science – are being increasingly used to improve public awareness of the availability of a service or benefit, as well as to improve the customer experience. Examples include advising job seekers of vacancies via text messaging, public awareness campaigns carried out online, and electronic access to benefit information and receipt through user-friendly mobile applications.
To improve the awareness of and access to OAS-related benefits (see Box 4.3). Canada has created an interactive OAS Benefits Estimator which makes it easier for citizens to get personalised information about benefits they are entitled to. The Benefits Estimator, which can be used to determine eligibility and entitlement for the OAS pension, the GIS and the Allowance for the Survivor is an easy-to‑use, anonymous, self-service online tool. It does not collect or store any personal data. In fewer than ten minutes, users can obtain a customised estimate of the benefit amount to which they are entitled. Since its launch in November 2022, version 1 has provided an average of 48 000 estimates a month on Canada.ca. User research validated the value of the tool, finding an 85% success rate, in contrast to the 35% success rate for the existing OAS payment tables on Canada.ca (OECD, 2023[1]).
4.2.4. Technology can enable faster and more flexible responses including during crises
While not without challenges, digital technology has gone a long way towards making OECD governments’ responses to crises faster, more agile and more flexible. Many countries turned to digital solutions for the “new” problems the COVID‑19 pandemic created. This included using existing technology in different ways, adapting it quickly and rolling it out at scale in very short timeframes. For example, as part of their national vaccination, lockdowns and contact tracing programmes Australia like many countries integrated COVID‑19 digital vaccination certificates with check-in apps (Box 4.4). An app, which had the advantage of being technology people were already familiar with, provided an easy, voluntary way for individuals to show their vaccination status and enabled businesses and organisations to verify vaccination status quickly and securely, creating a seamless experience for Australians.
At a supranational level, the EU Digital COVID‑19 Certificate (EUDCC) was created to facilitate travel within the EU during COVID‑19 and was viewed as a crucial element in Europe’s response to the pandemic. The EUDCC was available to all EU citizens as well as to travellers from outside of the EU. It was launched in July 2021, and by December 2022, member states had issued more than 2 billion EUDCCs. With 51 non-EU countries and territories connected to the system in addition to the 27 member states, the EUDCC was viewed as a global standard for COVID‑19 certification (European Commission, 2021[14]).
Box 4.4. Integration of COVID‑19 digital vaccination certificates with check-in apps in Australia
From August 2021, Australian Governments were tackling COVID‑19 through a national vaccination program, lockdowns and contact tracing. Australians needed a simple and easy way to demonstrate they had been vaccinated when entering a public venue. Australia Data and Digital Ministers drove intergovernmental action to successfully roll out a national solution integrating Commonwealth COVID‑19 digital vaccination certificates with state‑based check-in apps. This included establishing data sharing agreements between the Commonwealth and each jurisdiction to protect the privacy and data security of citizens.
The solution provided people with an easy, voluntary way to show their COVID‑19 vaccination status using familiar technology. When using a mobile device, individuals were able to share their COVID‑19 digital certificate with a state or territory check-in app from their Medicare Online Account (MOA) through myGov or the Medicare Express Plus mobile app or by using an Individual Healthcare Identifier (IHI) member service (via myGov). Citizens had to consent to adding their digital certificate to a check-in app.
The technology implementation enabled integration of the COVID‑19 digital certificate with eight different state and territory check-in apps, each with a slightly different user experience in line with jurisdictional requirements. The solution implemented was able to be scaled to handle the surges in traffic which were experienced when each jurisdiction went live.
Over 13 million individuals shared their COVID‑19 digital certificate with a check-in app over 39 million times. Re‑sharing occurred as individuals received COVID‑19 booster vaccinations and refreshed their proof of vaccination. As there are no longer public health orders in place, the transfer of encrypted vaccination data between Services Australia and each jurisdiction has been disabled as their Agreement expired. By the end of May 2023, all states and territories had turned off their check-in apps.
The solution, which reused secure Commonwealth infrastructure was based on five key principles:
1. Will not impact critical business systems.
2. Minimises the impact of and opportunity for fraud.
3. Performs at scale.
4. Is inclusive in design.
5. Creates a simple, helpful, respectful and transparent experience.
Source: (OECD, 2023[1]).
While governments were already making much greater use of web-based technology before COVID‑19, the pandemic drove a dramatic and rapid transformation in web-based services when in-person services shut down and the need for online pathways to social benefits skyrocketed. By way of example, in the US, GetCalFresh.org, a website that helps Californians apply for food assistance, initially started in 2013 as a project in San Francisco County (one of 58 counties in the state) and by 2019 became available state‑wide. COVID‑19 drove up applications for assistance via GetCalFresh.org significantly; since launching state‑wide GetCalFresh.org has assisted with ~50% of all applications for food assistance benefits in California, and ~75% of all online applications (Box 4.5).
Box 4.5. GetCalFresh.org in California, USA
GetCalFresh.org began as a partnership between Code for America (a civic tech non-profit organisation), the San Francisco-Marin Food Bank and the San Francisco municipal government, to help people submit applications to the federally funded Supplemental Nutrition Assistance Program (SNAP). At the time, California residents in need of food assistance were forced to navigate complex, hard to access, and ineffective online systems. These systems yielded relatively few and low-quality applications, contributing significantly to the need for California to increase its SNAP participation rate. For a single unemployed individual with zero income to apply for food assistance benefits, they would need to answer over 100 questions across 50 different screens, taking on average 30‑45 minutes to complete. This reality of cognitive burden and bureaucratic obstacles for applicants drove Code for America to design and build an online application experience that now takes about 10 minutes to complete and is available state‑wide.
For GetCalFresh.org to serve clients across California in applying for and maintaining food assistance benefits, it consistently collects and shares data from numerous different partners in various levels of government and in civil society. County governments receive applicant data from GetCalFresh.org for the purpose of determining benefit eligibility. They also share aggregate data with on-the‑ground organisations so that they can track and report their outreach efforts.
To ensure the safety of client information GetCalFresh.org limits the information they ask for to that which is strictly necessary. For the information that is collected, access is limited, and data are retained for a fixed period, after which all personally identifying information is removed. This data are stored securely using best practices of modern cloud architecture.
Today, GetCalFresh.org is maintained and operated by Code for America in partnership with the California Department of Social Services (CDSS), and since 2020 has helped at least 4 million people access over USD 6.5 billion in benefits.
Note: The findings reported here were performed with the permission of the California Department of Social Services. The opinions and conclusions expressed herein are solely those of the authors and should not be considered as representing the policy of the collaborating agency or of any agency of the California Government.
Source: (OECD, 2023[1]).
4.2.5. “Digital by design”: A digital overhaul of social protection in the United Kingdom
While digital technologies are an increasingly critical aspect of the delivery of social protection in many countries, the United Kingdom is the first country to have designed its single working-age benefit – Universal Credit – to be fundamentally “digital by design”. Intended to simplify the benefit system and to incentivise paid employment and higher earnings among working-age people both in and out of work, the Universal Credit is the flagship welfare policy of the Conservative Government, and standard bearer of its digital transformation strategy. It replaces six means-tested “legacy” benefits and tax credits, integrating elements for adults, housing costs and children, together with any supplements for disability and childcare costs into a single award paid monthly in arrears into one bank account per individual or couple (Griffiths, 2021[5]).
The Universal Credit has attracted both proponents and critics. Proponents highlight the greater efficiency and effectiveness of digitalisation evidenced by its performance in the wake of the huge surge in claims during COVID‑19. Following the lockdown in the United Kingdom in March 2020, an unprecedented 3.7 million people applied for the Universal Credit, around seven times the usual volume (Department of Work & Pensions (DWP), 2020[15]). More than nine out of ten eligible claims, together with around 1 million Universal Credit advance payments, were paid in full and on time (Department of Work & Pensions (DWP), 2020[15]). By December 2020, 5.9 million people were in receipt of the Universal Credit (Department of Work & Pensions, 2021[16]) compared with 1.9 million in March 2020. That the system was able “to work at great volume through [an] unprecedented claims spike” while achieving operational performance levels apparently in excess of pre‑COVID rates was attributed by some to the benefit’s automated features (Griffiths, 2021[5]).
Critics of the Universal Credit point to the “digital divide” between people with the skills and resources to access digital technologies, and those without, the erosion of citizen’s social rights, greater administrative burden and compliance costs for claimants, and frequently gendered effects. For example, because the UC is paid into one bank account it can make it easier for one person in the household to control the finances potentially increasing the risk of financial and other forms of domestic abuse.
Some commentators believe the potential for greater administrative burden on claimants is not well enough understood and that research and policy interest about digitalisation in the UC, and in welfare systems more generally, would benefit from a broadening out to include questions of administrative burdens, together with exploration of their wider effects and impacts on claimants (Griffiths, 2021[5]). Concerns about the increasing digital divide as more interactions with government agencies go online is not unique to the United Kingdom’s UC and is discussed in more detail in Chapter 5.
4.3. Making better use of new and existing data
Underpinning many advanced technology uses is new data or at least data that have not been traditionally used for social policy purposes. The ever-growing digital footprint of people globally, such as digital recordings of people’s online activities, has created many new sources of data, of previously unimaginable volume, in a variety of formats. Some of these data are structured, e.g. records from credit card companies, credit agencies and hospitals. Other data are semi- or unstructured and can come from the ever-increasing number of sensors that record individuals’ locations, workout activities and sleep patterns, social media posts and internet searches, pictures and videos, satellite data, and others. These data are often referred to as big data.
While a government programme database, such as a social registry, is not typically considered big data, governments are increasingly generating large volumes of data that have the characteristics of big data. They are using this data together with data from non-traditional sources for activities such as fraud detection, tax evasion, national security and law enforcement. Examples of big data use in social services is not yet common. Italian researchers have suggested that more geographically disaggregated poverty measures could be created based on combining official statistics with mobile phone data (Marchetti et al., 2015[17]). In Costa Rica, satellite‑based poverty estimates are being used to target social worker outreach campaigns. This section explores how OECD countries are using and enhancing their data holdings to improve services for individuals.
As mentioned above, advances in technology go hand in hand with advances in data collection and use. Improving the uptake of social protection fundamentally depends on being able to measure coverage and understand where the gaps are. This requires good-quality data from multiple sources. New data and smarter uses of data are steadily emerging that complement and extend the use of more traditional forms of microdata, such as survey data (e.g. from labour force surveys (LFSs) and administrative data (e.g., tax records and social security numbers). Novel data types, such as satellite imagery, mobile phone data and web and social media data, are being used, for example, in some countries to target outreach and determine eligibility for benefits and services.
Governments are progressively making more use of integrated digitised administrative databases, as well as non-government data, to better integrate services, reduce administrative burden, and to create tailored service packages for individuals. Both individual-level, identifiable data (for operational decisions) and de‑identified data (for better evidence‑based policy making for example) offer enormous potential for improved social protection coverage and more effective i.e., integrated, and targeted service delivery. This section first describes innovations in data collection, creation and linking before outlining novel ways in which these data are being used.
4.3.1. Traditional and evolving forms of survey data
Population surveys have long been a powerful tool for identifying the needs of different population groups, exploring issues such as people’s experiences of government services, and for measuring the impact of government investment in public services. Government agencies use the results of these types of surveys to identify how and where they should be using public resources and to assess how different groups in the community are experiencing existing policies, to assess programme outcomes relative to targets.
An example of a population survey conducted in many OECD countries is the labour force survey (LFS), a national household survey. LFSs are the main source of headline indicators of the labour market for short-term monitoring as well as more structural information on the number and characteristics of the employed, their jobs and working conditions, the job search activities of those without work, etc. A further example are general social surveys (GSS). Whereas LFSs are generally conducted regularly (typically quarterly), GSSs may only be conducted once every few years. A GSS is a personal interview survey that collects information on a wide range of demographic characteristics of respondents and members of their household; behavioural items such as civic participation and voting; personal psychological evaluations, including measures of happiness, well-being and life satisfaction; and can include attitudinal questions on public issues such as abortion, crime and punishment, race relations, gender roles, and spending priorities. These tend to have repeated questions over time and often ad hoc modules.
Survey questionnaires administered by national statistical offices (NSOs) are generally still completed face‑to-face (using computer-assisted questionnaires) or over the telephone. Globally, survey response rates using these traditional methods have been declining and statistical offices have been exploring other options for collecting information. Online surveys have become a popular data collection method as they are inexpensive (relatively) and are easy to create, disseminate, and gather responses to. However, not having access to the internet can be a barrier for some respondents, representativeness is harder to ensure, and some topics may not always lend themselves to online survey questions.
In recent years statistical agencies have been modifying surveys to cover a broader range of social risks and needs. In France, the Survey on the Living Environment and Safety combined face‑to-face interviews with a self-administered module on serious violence to elicit particularly sensitive information. Some surveys such as the OECD’s Risks that Matter (RTM) Survey, the Eurobarometer and the European Social Survey investigate respondents’ worries about personal and national risks. The RTM collects representative data for 27 countries on people’s perceptions of the main social and economic risks they face and how well they think public social protection addresses those risks. The Eurobarometer also conducts special rounds to investigate current attitudes, such as the recent survey of EU challenges and priorities in 2023. Other like polls have a more international focus, including the Lloyd’s Register Foundation World Risk Poll, the Pew Research Center Global Attitudes & Trends survey, and World Values Survey.
Some official national surveys also collect data on individuals’ perceptions of government and society. For instance, in Mexico, the Encuesta Nacional de Calidad e Impacto Gubernamental asks respondents about their confidence in a range of actors, including public services, government officials, and private individuals such as neighbours. Similarly, the Encuesta de Percepción Ciudadana in Colombia asks respondents if they think that public bodies treat individuals equally and without preferences.
New survey data can help to build a more comprehensive understanding of the outcomes of particular population groups and of specific risks about which little was previously known. In Mexico, for example, efforts to prevent, address, punish and eradicate violence against women make considerable reference to statistics on intimate partner violence based on a specialised, ongoing survey on violence (National Institute of Statistics and Geography (INEGI), 2022[18]).
A survey of the homeless population in France revealed that almost a quarter of individuals making use of services for the homeless had formerly been in out-of-home care (Frechon and Marpsat, 2016[19]). This finding influenced measures in a new child protection law introduced in 2022, which amongst other things guarantees extended care support to all care leavers up to the age of 21. Extended care was previously reserved to a third of the care leaver population and rarely up to the age of 21; the objective is to prevent so-called “dry exits” from child welfare services at age 18 and reduce homeless numbers. The law also provides for a “right to return” to care up to the age of 21 if initially refused at age 18 (OECD, 2022[20]).
More is also being done to cover hard-to-reach populations in surveys. For example, annual homeless counts are now being conducted in many cities by interviewers (volunteer or paid) who may walk city streets at night to talk with people without housing. Even these intensive efforts however may underestimate the homeless population in a systematically biased way – women, for example, are much more likely to experience homelessness without “sleeping rough” and may therefore be undercounted (OECD, forthcoming[21]). Better information about particularly hard-to-reach populations who may not exist in other data sources can help to improve the reach and retention of social protection programmes and services for those individuals in the most vulnerable situations.
Due to the inherent challenges of surveys, statistical agencies are increasingly augmenting or even replacing surveys with administrative data. For example, several European countries gather income and some demographic information for the EU Statistics on Income and Living Conditions through registers rather than survey questions. Portugal’s National Statistical Office studied the feasibility of replacing questions in the 2021 population and housing census with administrative data-based measurements. They identified 12 out of the 27 mandatory variables for which administrative data could be an acceptable replacement in the medium to long term. The Norwegian Statistical Office has access to one hundred registries for statistical purposes and is developing indicators relying on geographic information system data to gauge distances to emergency services from homes and workplaces.
4.3.2. Improving and expanding the use of linked data
Government agencies are increasing linking their administrative databases across ministries, agencies and levels of government. This is done for a range of operational, research and policy purposes, typically with strict rules and procedures in place to safeguard the security and privacy of people’s information. Linked data can contribute to breaking down topic silos, enhancing co‑operation across departments and improving access to benefits and services. Identifiable information about a person can help with operational decisions such as ensuring someone is receiving their full and correct benefit entitlements or to make the service user experience better. The “Transforming the Collection of Student Information” project in Australia for example enables prefilling of student information into some welfare claims, using a data linkage with the Department of Education. Prefilled data supports the agency to accurately assess customer circumstances, supporting efficiency and accuracy of payment delivery.
In a new ISSA report outlining major developments and trends for social security in Europe, leveraging data exchange for data-driven social security (together with digitalisation to improve service quality) are identified as key developments. Social security institutions throughout the region have recognized the value of exchanging data as part of their efforts to provide better and more holistic services, better identify target populations, and expand coverage. Examples provided in the report include France’s URSSAF National Fund which exchanged data to identify and support self-employed workers during COVID-19. The new harmonized data exchange model enabled better identification of people’s needs and served as a coordination mechanism between different institutions to provide social security coverage to self-employed workers and difficult-to-cover groups. In the Netherlands, the Social Insurance Bank (Sociale Verzekeringsbank – SVB) has developed a multi-party data exchange to address the non-take up of an income support supplement by identifying the target population while still complying with data protection regulations (ISSA, 2024[22]).
The increased use of social information systems and registries for operational purposes
More countries are using social information systems and registries to support identification (particularly of people previously unknown to the system), outreach and determination of potential eligibility for social programmes. While social information systems are typically based on linked administrative data provided by government agencies, social registries enable individuals themselves to register and be considered for inclusion in social programmes based on an assessment of their needs and eligibility.
While there are risks associated with both social information systems and registries, for example relating to information security or the possibility of some population groups being excluded, they are becoming crucial to the design, implementation, monitoring and evaluation of social policies and services in many countries.
The data that social information systems and registers contain can also be used to create tools and methods to assess eligibility for programmes and benefits, identify who is missing out and to make offers. In addition, and as was seen during the COVID‑19 pandemic, information systems and registries can play a key role in responding to crisis and emergency situations, when people in vulnerable situations need to be contacted quickly and/or to identify potential recipients of social benefits. This is a critical component of a responsive social protection system.
In Europe, the Belgian Crossroads Bank for Social Security (CBSS) which has existed for over 30 years is a noteworthy initiative (also see Chapter 3). The CBSS co‑ordinates information exchanges between the country’s 3 000 social security actors, allowing the automatic granting of several benefits (Box 4.6) (CBSS, 2023[23]).
Box 4.6. Crossroads Bank for Social Security (CBSS) (Belgium)
Belgium sought to address the problems arising from the lack of co‑ordination and integration of information flows across different social security actors. For example, an information burden is imposed on citizens and companies if they are required to provide the same information several times. It started as a co‑ordinated information management programme and led to the creation of a permanent and interoperable social security network, which includes all social security institutions operating in Belgium. It therefore acts as a public services integrator in the social security sector. This has allowed the reengineering and full automation of social security organisational processes for the benefit of relevant institutions, citizens and companies.
CBSS has fully integrated the workflows of around 3 000 social security national institutions, making the whole process available online. This provided single and fast access to all social services and benefits for customers as well as infrastructure and systems to the involved organisations, which increased agility and data transparency. CBSS illustrates how the introduction of a one‑stop shop to implement electronic service delivery can lead to a structural reform process. In this case, ICTs transformed the delivery of social security services, by initiating a business reengineering process within and across all 3 000 organisations involved in the Belgian social security system. At the same time, back-office functions were automated significantly, reducing a large amount of duplication of information because of the large number of social security actors.
The new ICT-based system significantly increased the reuse of information and made it possible to send responses to beneficiaries and civil servants automatically. This led to a considerable simplification of procedures and introduced a new, more integrated, personalised way of communicating with citizens and companies, which is better aligned with the needs of the final users.
Source: (CBSS, 2023[23])
Social registries are more common in the Latin American OECD countries and are being used to help capture new potential beneficiaries (Economic Commission for Latin America and the Caribbean (ECLAC), 2023[24]). In Costa Rica, social protection coverage is being addressed in part through SINIRUBE, a common database that draws together all registries from social programmes. This is helping to eliminate overlaps and increase coverage by enabling identification of potentially eligible beneficiaries not yet covered by those programmes. SINIRUBE has been used to assess the targeting of some social programmes (such as scholarships and non-contributory pensions) and there are plans to further increase the coverage of SINIRUBE and to incorporate individuals in remote locations or without access who are not yet included. SINIRUBE shows promise as a central tool for selecting beneficiaries for all social programmes to help improve targeting and evaluation of social policies. This is critical as some estimates suggest that in some social programmes, more than 40% of beneficiaries are middle and high-income households while according to the law the programmes are targeted to those in poverty (OECD, 2023[25]).
Chile’s Integrated Social Information System (SIIS) is the digital platform that supports its social protection system and co‑ordinates all data from municipalities and public entities in the Social Information Registry (RIS). The RIS contains data on individuals and families who are or could be recipients of public benefits and programmes, the benefits and amounts they obtain from these, the characteristics that make them eligible for social benefits and their socio-economic circumstances. The information in the RIS is provided by municipalities and by public and private entities administering statutory social benefits. RIS data are available so that these same stakeholders can use them and, where appropriate, better administer the programmes they are responsible for, always with due regard for individuals’ right to privacy (Economic Commission for Latin America and the Caribbean (ECLAC), 2023[24]). The SIIS also includes the Social Registry of Households (RSH) which is discussed in more depth in Chapter 3.
Colombia’s social registry, Sistema de Identificación de Potenciales Beneficiarios de Programas Sociales (Sisbén), was first introduced in 1995 and is the main targeting instrument for social programmes in Colombia. As of March 2024, around 34 million people are registered in Sisbén, equivalent to approximately 70% of Colombia’s population. Despite the challenges faced in maintaining updated and accurate information, Sisbén remains a broadly used instrument and 21 social programmes at the national level currently use it for targeting, including flagship cash transfer programmes in the Department of Social Prosperity (Departamento de Prosperidad Social, DPS).
Unsurprisingly, COVID‑19 accelerated the shift towards greater use of digital data sources for operational purposes when traditional data collection methods such as face to face meetings were no longer viable during multiple and sustained lockdowns. Integrated, cross-agency administrative data were used during the pandemic in many countries, for example in Brazil, Colombia and Türkiye, to facilitate outreach, assess eligibility for benefits and update beneficiaries’ files.
With the onset of the pandemic, Türkiye very quickly linked social protection services to their e‑Devlet platform. While the general use of e‑government services for downloading and submitting completed forms – although not necessarily for social protection – was already increasing in Türkiye during COVID‑19 there were around 4 million electronic applications for the Pandemic Support Programme (PSP) via e‑Devlet in two weeks. Furthermore, those already registered in the Integrated Social Assistance Service Information System received the cash benefit automatically, without needing to apply for it. Being able to apply for social protection programmes through e‑Devlet has enhanced access to social protection with the digital processes that were initially only possible for the PSP now in place for all social protection measures (Burattini et al., 2022[26]).
Following the pandemic, the United States’ Department of Labor is testing an automated income verification service through a federal data sharing partnership with the Internal Revenue Service (IRS) which aims to build a more responsive income support system in the event of a future recession or national emergency. With such income data linking in place, programmes like Disaster Unemployment Assistance (DUA) and Pandemic Unemployment Assistance (PUA) – which currently rely on manual income verification processes and fragmented data – can be administered more quickly and efficiently.
Linked data for research and policy purposes
Several OECD countries are taking a systematic, cross-government approach to linking administrative data for research and policy purposes such as creating estimates of service coverage and gaps, measuring the impact of social services on peoples’ well-being, and modelling the impacts of potential policy changes on different population groups. Individually identifiable data are linked before the resulting dataset(s) are stripped of information that could be used to identify individual people governed by strict protocols to ensure that there can be no spontaneous recognition of people in any analytical outputs.
One example of good practice comes from New Zealand, which has established the Integrated Data Infrastructure (IDI) to enable longitudinal research into the causes and correlates of social outcomes for New Zealanders. The data are de‑identified, which means it cannot be used to take actions for individuals. Rather, the IDI has been used to better understand early benefit entrants’ vulnerability to long-term welfare dependency and target services accordingly, and to identify relationships between individual measures of disadvantage and a measure of educational success resulting in a new school-based equity funding model (see Box 4.7).
Box 4.7. Integrated Data Infrastructure (New Zealand)
The IDI is a large research database developed and managed by Stats NZ. The IDI takes data from databases from various government agencies, non-government organisations, and Stats NZ surveys (including the Census), and the data are linked together, or integrated, to form the IDI. Data about people and households is de‑identified (names, dates of birth, and addresses are removed, and numbers that can be used to identify people are encrypted). The data are about life events, like education, income, benefits, migration, justice, and health.
The IDI has been used in a range of ways to better understand the coverage, impact, and effectiveness of social policies and services:
Study of estimates of Working for Families eligibility and take‑up rates
New Zealand’s Ministry for Social Development (MSD) undertook a study using the IDI to estimate families’ eligibility for, and take up of, the main Working for Families tax credit payments, Family Tax Credit, and the In-work Tax Credit. Working for Families tax credits are paid to families with dependent children to help with the cost of raising a family. The use of linked data has enabled MSD to produce estimates of eligibility and take‑up for nationally representative samples of families. This includes families who do not receive Working for Families but could be eligible. Results revealed a decline in the eligibility rate from 72% of families in the 2010 tax year to 49% in the 2020 tax year. Growth in incomes over a period when abatement and payment rates increasingly targeted payments to lower income families contributed to the decline. The results also highlighted differences in take‑up rates by ethnic group, and opportunities for addressing these.
One of the recommendations of a review of New Zealand’s welfare system in 2019 was annual reporting on take‑up rates for income support payments. The study is part of MSD’s programme to build evidence on eligibility for and take‑up of different payments in the New Zealand income support system. One of the methodological findings of the study was that estimation of Working for Families eligibility and take‑up requires several assumptions and simplifications and has a range of limitations and sources of potential error. Given this, there is considerable uncertainty in the estimates for any particular year taken in isolation. In addition, there is around an 18‑month lag between the end of a tax year and comprehensive data becoming available in the IDI. This further reduces the usefulness of producing estimates on an annual basis. Nevertheless, patterns of receipt across longer time periods are likely to be indicative of real changes, as are sustained and large differences between different population groups. This means that there is likely to be value in updating the study every three or so years to identify emerging patterns or issues.
Social outcomes model
The IDI has been used to develop a Social Outcomes Model, which projects a range of future outcomes and service use for each adult in New Zealand, based on analysis of past trends using historical data in the IDI as well as economic forecasts. The Social Outcomes Model is itself made up of multiple, interconnected models that use past trends to help project future outcomes, including benefit receipt, emergency and public housing use, income and employment, mental health service use, police proceedings, corrections sentences, and education.
The model can be used for a range of analytical purposes, including describing the characteristics of different groups of people; estimating what people’s future outcomes are likely to be, understanding how likely different outcomes are; comparing outcomes for different groups and over time, and creating “what-if” scenarios. The model has been used to estimate how many people are likely to need support in the future, and for how long; for example, it is used to estimate the future time that different groups of people will be supported by benefits, will be employed, or will be in public housing.
Measuring the effectiveness of employment assistance
MSD has developed a methodology for systematically assessing the effectiveness of its employment assistance (i.e., Active Labour Market policies), drawing on a range of linked administrative data, including data held in the IDI. This work helps the ministry understand what employment interventions are working for whom, and whether effectiveness is changing over time. These insights inform MSD’s investment strategies and regular adjustments to how it delivers interventions.
The main method used to estimate the impact of employment assistance (EA) interventions is Propensity Score Matching, which constructs a matched group of non-participants who have the same (or similar) characteristics as participants. Effectiveness is assessed against five main outcomes that EA interventions are expected to have a positive impact on: employment, income, justice, educational qualifications, and welfare. Once there is an effectiveness rating for each outcome domain, the ratings are combined to arrive at an overall rating of a programme: effective, promising, mixed, makes no difference, likely negative, and negative. The effectiveness of around half the expenditure could be assessed in 2019/20, the balance could not be evaluated using this method, largely because it was not technically feasible.
Monitoring the effectiveness of ALMPs is an obligation in the Public Finance Act to report on the efficiency and effectiveness of MSD’s expenditure. Using standardised evaluative monitoring based on linked data enables MSD to provide high quality, consistent and up to date information about performance. The effectiveness monitoring of ALMPs is applied at scale and covers all ALMP participants from the year 2000 onwards; results are updated each time the data in the IDI is updated (currently three times a year).
In 2015 the Multi-Agency Data Integration Project (MADIP), was initiated by three Australian social protection and services agencies (the Departments of Social Services and Health and Aged Care, and Services Australia) in partnership with the National Statistical Office, the Australian Bureau of Statistics. The project brought together different data sources and used a then emerging technology known as statistical data integration to improve the coverage, effectiveness and delivery of social protection and social services. At all times, close adherence to privacy and ethical governance arrangements was maintained to support the integration of data across government. MADIP is being effectively leveraged for new improvements in the delivery of services, two examples of which are provided in Box 4.8.
Box 4.8. MADIP applications in Australia
AIR-MADIP
The AIR-MADIP project was initiated in 2021 to inform the Australian Government’s COVID‑19 Vaccine Strategy. The Australian Immunisation Register (AIR) was linked to MADIP enabling more granular breakdowns and analysis of selected socio-demographic cohorts, which allowed COVID‑19 policy interventions to be targeted accordingly.
The project brought together a range of Commonwealth health system datasets incorporating data from all levels of government – national, jurisdictional and non-government organisations. The rich socio-demographic information on people living in Australia alongside critical health outcomes such as vaccination records and utilisation of government-subsidised or funded services (where eligible) informed COVID‑19 health policy for more vulnerable populations.
AIR-MADIP continues to inform the Australian Government’s COVID‑19 response and is a pilot for integration of Commonwealth data with information from privately held collections and states and territories. The success of the project and learnings are being applied in other health areas such as the broader National Immunisation Program as well as to the design of other whole‑of-government health data initiatives including the Australian Centre for Disease Control.
A collaborative partnership of data analysts and health experts across government, research and academia also continue to use the project to deliver regular policy-relevant insights.
The National Disability Data Asset
Nine Australian state and territory governments are working with the disability community to establish the National Disability Data Asset. Once established, the disability data asset will bring together de‑identified information from many different government agencies about Australians with and without disabilities, including information about health, education, employment, social security, justice and disability-specific services. The disability data asset will help to better understand the experiences of people with disability and how to improve the way policies, programmes and services support them. It will also provide more information to the disability community to advocate for change and to help inform decisions about supports and services.
The initiative is the first test of using data sharing arrangements in the Data Availability and Transparency Act 2022, which was enacted to promote better availability of public sector data, enable sharing of that data, enhance integrity and transparency, and to build confidence. The National Disability Data Asset went through an 18‑month testing period where the best ways to share, link and access information were assessed.
Source: (OECD, 2023[1]).
Lithuania’s State Data Agency has started to join administrative data from various registers and informational systems to create a data lake, a centralised repository that enables storage of structured and unstructured data at any scale. This joined-up data enables Lithuanian ministries to analyse data in more depth. In accordance with the Law on Official Statistics and State Data Management of the Republic of Lithuania, a ministry has the right to request administrative data based on the State Data Management Program to carry out their activities and to undertake detailed analyses.
In Canada, British Colombia’s Data Innovation programme available for use by government analysts and academic researchers to conduct population-level research. The programme links, de‑identifies and provides access to administrative datasets in one secure environment. The programme creates a streamlined and consistent approach to obtaining, linking and storing data safely for researchers to use, unlocking the value and shared benefits of public sector data by collecting and integrating it under one governance regulation, in one place. In France too, where the issue of estimating non-take‑up has been prioritised, government researchers have published rates of non-take‑up of minimum pensions based on linked tax information (Chapter 3).
Making linked data available to external as well as government-employed researchers can broaden the knowledge base about what works to improve the effectiveness of social policy interventions and social protection coverage. To make it easier for researchers to gain approval for using linked data, several OECD countries have created framework institutions that facilitate data merging. Among these are Canada’s Social Data Linkage Environment, France’s Centre for Secure Data Access, Finland’s upcoming FINDATA, the United Kingdom’s Administrative Data Research (ADR UK) Strategic Hub, NZ’s IDI and the United States’ Census Bureau’s Data Linkage Infrastructure. While details differ, these programmes typically combine several functions such as creating an inventory of data sources, reviewing research proposals, helping researchers gain approval from concerned agencies, linking the data and providing secure access.
4.3.3. Novel and new ways in which data are being used
Increased access to novel data sources is driving innovations in automated decision-making, risk prediction, evaluation methods and other analytical applications. There is a broad range of analytical (and statistical) methods that can be applied to novel data to tackle social and other issues. Increasingly both private and public sector organisations are employing advanced analytical techniques to be more responsive, enhance the service user experience and significantly improve their decision-making. Advanced analytics is an umbrella term referring to a range of data analysis techniques used primarily for predictive purposes, such as machine learning, predictive modelling, neural networks; techniques included in some definitions of AI.
While the priority of maximising the potential of digital technologies and data to deliver public value has been growing for years COVID‑19 accelerated this trend, as there was no choice but to deliver public services digitally. In a context of increased demand for digital public services, governments needed to ensure the quality of public services, capitalising on the opportunity offered by digital tools and data to transform service design and delivery while preventing the emergence of new forms of digital divides and exclusion (OECD, 2022[28]).
There is no shortage of new public-sector data analytics use cases. Government entities have created real-time pandemic dashboards, conducted geospatial mapping for drawing new public transportation routes, and analysed public sentiment to inform economic recovery investment. While several of these examples were born out of necessity, public-sector agencies were already beginning, pre‑pandemic, to embrace the role data-driven decision-making can play in optimising government resources by targeting them more effectively and enabling civil servants to focus their efforts on activities that deliver the greatest results (McKinsey & Company, 2021[29]).
A relatively common application of analytics now is to identify high-risk individuals among existing clients of a social service agency or in the population at large. For instance, the Chilean Ministry for Social Development is exploring how to identify vulnerable families beyond the needs-adjusted income score in their social registry (Chapter 3). New Zealand’s Youth Service has a risk-scoring algorithm that aims to predict which school leavers are at high risk of becoming long-term benefit recipients. The algorithm uses data to analyse factors such as how well the former student did at school, whether their parents received welfare benefits and if they were in contact with child protective services. Service providers then approach those deemed most at risk to offer a service (Box 4.9).
Box 4.9. Youth Service referral system and predictive tool in New Zealand
New Zealand’s Youth Service provides coaching for 15 to 19‑year‑olds who could achieve better outcomes with the right help. It is designed to make sure that young people who access the service are not limited to those who are motivated to proactively ask for help. The automated referral system uses a statistical predictive modelling tool and eligibility rules to help predict need for young people aged 15 – 17 years old who have left school early.
The predictive modelling tool considers factors such as: demographic information, whether a young person’s parents received income support, the school history of a young person (including educational achievement, reason for leaving school, and truancy history), and the level of contact a young person has had with Oranga Tamariki, New Zealand’s child protection agency. These factors have been linked to the likelihood of a young person needing support.
The automated referral system also tells MSD what level of support a young person may need. The model which uses data from the Ministry of Education and Oranga Tamariki produces service level intensity indicators for school leavers: High, Medium, Low or Very Low. Contact details of those predicted to have the highest need, and who are eligible, are passed onto the Youth Service. Youth support specialists then contact the young person to ask if they want help, and together, through a needs assessment, they work out what might help them.
Young people can choose whether or not to participate in the service.
Source: (OECD, 2023[1]).
Increased access to different administrative databases (including at the subnational level), often combined with new data visualisation techniques, has deepened the understanding of policy makers and the public alike on how much life chances can vary between adjacent neighbourhoods (Chetty et al., 2018[30]). A study from Ontario analysed call records to a human services helpline to reveal regional variations in service gaps (Dillon Consulting, 2018[31]). A New Zealand study looking at the uptake of B4 School Checks, which is a free health and development screen for children at age four, used data from regional District Health Boards and found that national averages were hiding large regional differences in uptake (Nichola Shackleton, 2021[32]).
Lithuania monitors the effectiveness of social support measures across municipalities using linked survey and administrative data. Social support indicators such as poverty reduction, assistance and prevention are combined into a common index and then converted into ranks on a 10‑point scale, where 1 represents the worst result and 10 represents the best. All indicators have the same weight in the index. The ranks show the situation of the municipality in that year in comparison with other municipalities.
Access to administrative datasets has led to inventive and more systematic (and sometimes cheaper) use of quantitative methods to evaluate social programmes, including longer-term and more ex-post evaluations. In the domain of active labour market policies (ALMPs), the OECD’s Directorate for Employment, Labour and Social Affairs (ELS) has been working together with the European Commission’s Directorate General for Employment, Social Affairs and Inclusion on a project that covers six EU countries and Canada (OECD, 2020[33]). The project aims to improve the effectiveness of ALMPs and strengthen countries’ capacity for evidence-informed policy making.
Routine access to administrative data can allow researchers to design evaluations in a detailed manner prior to a policy change or programme implementation and to start carrying them out and have first results shortly thereafter (Langedijk, Vollbracht and Paruolo, 2019[34]). Short computer- and mobile‑phone-based surveys can provide complementary data at more frequent intervals. Furthermore, big data from non-traditional sources can deliver additional information that is available more quickly or that is complementary, for instance allowing insights on public attitudes towards a policy (Global Pulse, 2016[35]).
Administrative data can also provide more accurate measures of key programme outcomes. For instance, a recent evaluation of anti-poverty programmes based on both survey and administrative data found that survey data often under-stated the incomes of low-income respondents. As a result, evaluations based on survey data alone found a lower poverty-reducing impact of the studied programmes (Meyer and Mittag, 2019[36]). Moreover, potentially eligible beneficiaries for a social benefit can be identified. A list of these individuals can then serve as a sampling frame for studies on why they do not apply and on policy interventions that might incentivise them to do so. Examples include an evaluation of policies to increase applications for food stamps among likely eligible individuals (Finkelstein and Notowidigdo, 2019[37]) and Belgium’s TAKE project (Chapter 3) that applies different research methodologies including microsimulation models, a field experiment and micro econometric analyses based on survey and administrative data to study non-take up of various benefits in Belgium (TAKE-Project, n.d.[38]).
In 2018, New Zealand trialled an initiative to address low take up of a hardship payment within the income support system (see Box 4.10). A key feature of the initiative was the use of a micro simulation modelling approach which uses data on people’s characteristics to determine if they meet the policy criteria. A randomised control trial would have been the usual method for evaluating the intervention, but this approach was considered inappropriate by stakeholders – as some individuals predicted to be experiencing severe financial hardship would have been allocated to the control group. Instead, a difference‑in-difference model was developed to assess the effectiveness of the intervention. The campaign involved modelling who was eligible but not receiving the payments and proactively contacting individuals to tell them about the payment using either phone calls, emails, or letters. The campaign successfully increased take‑up of the payment by ten percentage points and was subsequently turned into a business-as-usual process.
Box 4.10. Temporary Additional Support (TAS) campaign in New Zealand
New Zealand’s TAS is a supplementary benefit for people who experience financial hardship. For many years advocates on behalf of benefit recipients expressed concerned that the number of people receiving the TAS payment was considerably less than the total who appeared eligible. It was argued that this low rate of take‑up meant the welfare system was not adequately protecting families from financial hardship and poverty. Barriers to accessing TAS payments were thought to be highest among vulnerable populations including those with compromised physical and mental health. Analysis suggested several potential reasons for incomplete take‑up of TAS including it was complicated and difficult for potential claimants to understand, it required substantial effort to apply, it had burdensome compliance requirements related to reporting changes in circumstances, and it automatically expired after 13 weeks and required a reapplication if the claimant was still eligible.
The purpose of the campaign was to trial an initiative to address the low take up of TAS. The campaign, using a microsimulation model to identify people who were not receiving the payment but appeared eligible suggested that only 68% of eligible people were receiving the payment. However, there was uncertainty about the estimate because the administrative data used in the eligibility calculation was not always up-to-date or comprehensive.
The campaign showed that proactive contact using the microsimulation model was able to increase take‑up of TAS amongst the high need group. The trial also demonstrated the relative effectiveness of contact by phone, letters, and emails – with the former having the largest impact on take‑up.
Source: (OECD, 2023[1]; Rea and Hyslop, 2023[39]).
The value demonstrated by advanced uses of data is contributing to calls to strengthen data collection. To understand domestic violence underreporting, the University of Chicago partnered with several city agencies in Chicago, merging medical record data from emergency room visits to the University of Chicago Medical Center from 2008 through 2018 with information on crime victimisation from the Chicago Police Department. Worldwide, around 26% of ever married/partnered women aged 15 and older report having experienced some form of physical and/or sexual intimate partner violence (OECD, 2023[40]). In the United States, the figure may be as high as 41% and even that is likely to be an underestimate due to barriers to reporting (Graber et al., 2023[41]). Linked data can help to improve these estimates.
The University of Chicago focused their analysis on adult ER patients who had had an X-ray. The data revealed that 60 out of every 10 000 adults receiving an X-ray go on to report a domestic violence victimisation to local law enforcement authorities within a few days of the ER visit. But only 5.5 out of 10 000 disclosed domestic violence to medical professionals in the ER. One implication of this findings is that many survivors are reporting to agencies –law enforcement –that are not well-equipped to handle complex medical and social needs of domestic violence survivors. Meanwhile, in most cases, institutions that might be better equipped to do this work –such as medical providers and their social service agency partners –do not know about these opportunities to help (Graber et al., 2023[41]).
Further, while the rate of reporting to law enforcement is higher than to medical professionals in the ER, almost half of survivors of domestic violence do not report the abuse to law enforcement either. That means most domestic violence survivors are unknown to the social service agencies who might be able to help (Graber et al., 2023[41]). An outcome of the findings is that the city of Chicago, in its new strategic plan to address gender-based violence has joined institutions like the World Health Organization in pushing to strengthen data collection across sectors and expand where survivors can be connected to services, including in the healthcare system. In addition, interdisciplinary teams around the United States, for example the Lutheran Settlement House Bilingual Domestic Violence Program in Philadelphia are exploring ways to support healthcare providers so that they can be more helpful to survivors.
4.4. AI: The transformative technology of our time?
AI is reshaping societies and economies. It promises to generate productivity gains, promote innovation and growth, improve well-being and help to address global challenges such as climate change, resource scarcity and health crises. In the government sector, advocates argue that AI could radically improve the efficiency and quality of public service delivery, in areas such as education, healthcare and social protection. AI could be used to improve access to social protection for example through more precise targeting of eligible beneficiaries, faster and more accurate eligibility decisions, and/or AI-assisted job matching assess eligibility and needs, make enrolment decisions, provide information about available programmes and benefits, to adjust benefits, and monitor and manage benefit delivery.
At the same time however, significant challenges exist. For example, a key risk when using AI to manage social benefits, is that assessments of benefit eligibility are incorrect or systematically biased against certain demographic groups (Verhagen, forthcoming[3]). The OECD’s 2023 Employment Outlook which reviews the emerging evidence about the impact of AI on the labour market found that while workers and employers both reported AI can lead to greater worker engagement and physical safety, the downside is that with the removal of simple tasks workers can be left with a more intense, higher-paced work environment (OECD, 2023[4]). The risks and challenges associated with digitalisation of social protection including through greater use of AI are discussed in more detail in Chapter 5.
Definitions of AI are often broad and encompassing, and it can be tempting to subsume within it most examples of analytics; however, not all analytical methods deploy AI techniques. The OECD describe an AI system as a machine‑based system that can, for a given set of human-defined objectives, make predictions, recommendations, or decisions influencing real or virtual environments. It uses machine and/or human-based inputs to perceive real and/or virtual environments; abstract such perceptions into models (in an automated manner e.g., with machine learning1 or manually); and use model inference to formulate options for information or action. AI systems are designed to operate with varying levels of autonomy (OECD, 2019[42]). The OECD was aiming for a description that was understandable, technically accurate, technology-neutral and applicable to short- and long-term time horizons. The description, which is adopted for the purposes of this report, is also broad enough to encompass many of the definitions of AI commonly used by scientific, business and policy communities.2
Adoption of AI techniques in the public sector remains limited, relative to other sectors. It is used by some countries for activities such as fraud detection; the United Kingdom Government for example is using AI to help detect fraud in social benefits claims (Marr, 2018[43]). The Republic of Korea has made considerable advancements in using big data for error and fraud detection among health insurance claims. Korea’s National Health Insurance Service (NHIS) houses big data on a range of socio‑economic, health behaviour, healthcare utilisation and long-term care variables to which smart audit algorithms are applied to predict healthcare facilities with high probability of fraudulent claims, thereby pre‑emptively supporting investigators (ISSA, 2022[44]).
According to (Ohlenburg, 2020[45]) reasons for the relatively slow uptake in the government sector include ethical and legal concerns, as well as scepticism about whether computer-driven systems are appropriate in the sphere of public policy and administration. Leveraging AI requires complementary investments in data, skills and digitalised workflows, as well as changes to organisational processes. As a result, adoption varies across sectors, industries and companies. The challenges including issues such as data accessibility and shortages of AI talent are discussed in Chapter 5.
Despite the challenges of applying AI in the government sector, countries recognise its enormous promise and are thinking carefully about how best to take advantage of it. As of June 2021, 20 EU Member States and Norway had published their national AI strategies, while another seven Member States are in the final drafting phase (Joint Research Centre, 2021[46]). Estonia’s AI strategy for example covers a wide range of areas, including environmental applications, emergency assistance, cybersecurity and social services. Estonia’s approach to AI-enabled digital public services is implemented as part of #KrattAI, an interoperable network of AI applications that allow citizens to use public services through voice interactions with virtual assistants. An intelligent conversational assistant for instance is being used for the treatment of people at risk of long-term unemployment in the context of unemployment insurance (Ott Velsberg, 2020[47]).
4.4.1. Chatbots and digital assistance
Examples of AI deployment provided by countries that responded to the OECD questionnaire were either of automated back-office processes (e.g., processing large amounts of data from traditional databases and unstructured text and images from scanned paper media) and/or automated support (i.e., chat bots and digital assistance). Several social security institutions have implemented smart chatbots to improve online customer services, with 24/7 availability in different industries and for different types of services. These intelligent assistants can mimic human behaviour and are able to autonomously respond to user requests.
In an ISSA 2021 survey of 166 government agencies across the world about AI adoption, chatbots emerged as the frontrunners with 26% of respondents already implementing them and another 59% planning to implement them within three years. In a review of 230 AI-enabled public services across the EU, chatbots emerged as the first choice, accounting for over one‑fifth of use cases. In 2017 Deloitte predicted that the global conversational AI market, including chatbots and intelligent virtual assistants would have a Compound Annual Growth Rate of 22% between 2020 and 2025 reaching almost 14 billion US dollars (ISSA, 2022[48]).
The Social Insurance Institution (Kela) in Finland set up two chatbots, Kela-Kelpo and FPA-Folke, to help clients find information about benefits on Kela’s self-service web portal. Based on natural language processing, the chatbots speak two languages – Finnish and Swedish – and they also understand English. These conversational chatbots make it easier to discover and interpret information and to complete benefit applications. Furthermore, they provide customised tips based on contextual variables as clients fill out applications for benefits such as parental benefits and social assistance. A dedicated chatbot was temporarily deployed to address queries on COVID‑19‑related social assistance (ISSA, 2022[48]).
Services Australia uses a Digital Assistant to provide real-time assistance to customers with a range of questions related to social security payments.
The Austrian Social Insurance (Dachverband der österreichischen Sozialversicherungsträger) deployed an intelligent conversational assistant – OSC Caro – that provides digital assistance to members in several areas, such as childcare benefits, health benefits, and reimbursements (ISSA, 2020[49]).
Similarly, Brazil’s National Social Security Institute (Instituto Nacional do Seguro Social) has implemented an intelligent conversational assistant – named “Helô” – to provide automated 24/7 responses to member requests as part of myINSS’s personalised online services. “Helô” was implemented in phases. The first phase involved setting up a rules-based virtual assistant, using keywords. The second phase included the creation of a knowledge base to provide a more targeted service to citizens based on their profiles, and to enable integration with other social media and messaging platforms. In the first month of operation, a million calls were received, serving an average of 32 000 citizens a day; 57% of citizens using Helô said that it responded correctly (ISSA, 2022[48]).
Chatbots proved particularly valuable helping to meet the unprecedented demand for information during COVID‑19. Between March and May 2020, the Norwegian Labour and Welfare (NAV) administration’s intelligent conversation assistant responded to more than 8 000 daily requests, compared to 2000 before COVID‑19. A review of the conversation assistant found the main success factors were training the assistant based on a knowledge base updated daily, with priority given to a specific type of information and having a permanent link between the assistant and a human expert. New topics were added to the remit of the conversational assistant, especially to help employers and freelancers (ISSA, 2020[49]).
The National Employment Office (Office national de l’emploi – ONEM) in Belgium set up a chatbot to ease contact centre pressures during COVID‑19. The first chatbot was rolled out in May 2020 and gave citizens rapid access to copies of the tax certificates they needed to submit alongside their tax returns. In May 2021, the chatbot’s capabilities were expanded, and based on an analysis of the questions posed to it, an upgraded version was rolled out in December 2021. The chatbot can now answer a range of questions relating to unemployment and career breaks. It also serves as a promotional tool to encourage use of e‑box, Belgium’s virtual, secure mailbox that enables authorities to communicate safely with citizens. The chatbot can remember the context in which a customer is situated when they ask questions, ensuring that the chatbot can continue supporting the customer regardless of where and how the customer navigates the website. Furthermore, the themes covered by the chatbot are regularly updated based on the analysis of customers’ questions (ISSA, 2022[48]).
In Korea, an AI driven personalised conversation service (which remembers past conservations and uses them for the next call) is used to check on people’s well-being once or twice a week, chatting with people for about two minutes.
Not all chatbots, however, are developed using AI technology. Some chatbots only replicate human actions and tasks in digital systems, typically tasks that are repetitive and rule‑based i.e., they don’t think or learn. The Canada Revenue Agency (CRA) for example is developing Chatbot and Online Chat solutions (Box 4.11) as part of its ongoing development and enhancement of comprehensive products and strategies related to digital services which are based on varying maturity levels of AI. The existing Chatbot is rules-based using a Question-and-Answer model. It can only respond from its knowledge base answers that match a specific set of topics. The online chat solutions leverage user-centric chat technologies to help clients navigate complex non-account specific material without having to leave the digital channel. The CRA is taking A People First approach to informing the design and delivery of their digital services to ensure they meet clients’ evolving needs and expectations.
Box 4.11. End-to-End Digital Service Channel – Chat Services Project (CSP) in Canada
The CRA has deployed Chatbot and Online Chat solutions on a limited basis for public use. To date, Chatbot has answered 11.2 million questions, with client traffic increasingly significantly during the recent tax filing season. With the first iteration of Online chat, agents responded to over 56 000 non-account-specific chats between 1 March and 1 December 2022. The second iteration was launched in February 2023 and to 31 March 2023, agents responded to over 45 000 non-account-specific chats.
Efforts have begun on building an enterprise Chat Services solution for deployment across the CRA (the CSP) because despite the CRA’s existing digital presence, clients continue to experience challenges with accessing information they need from the CRA’s website. As a result, they abandon the digital portals and either complete their task incorrectly or resort to telephone services to get assistance. Chat technology presents an opportunity for the CRA to innovate and transform how it communicates online with clients and provides them with more options for a positive, seamless client experience.
The CSP initiative aligns with a number of the CRA’s objectives to create a cohesive “whole‑of-Agency” approach to delivering a tailored seamless client service experience. The approach includes a focus on digital and IT modernisation, content optimisation, and client experience initiatives across the organisation, building a more seamless and integrated service experience for clients and moving their service interactions to the first viable resolution point and the lowest cost channel. Further, the CRA is seeking to optimise its public web presence so that people can more easily find answers to their tax and benefit-related questions, adding chatbot and live chat agent services to help people navigate complex information and to get assistance through automated and live channels without having to leave the digital space.
The CSP will involve a wide‑scale application of cloud technology. Once the digital solutions are deployed, they will involve the two‑way transmission of data between host (the CRA) and client, the storage of data received from the client, and data-driven reviews of received client data to better address client pain points and call drivers. As a result, the CSP aims to ensure the safe and ethical use of the technology to:
maintain clients’ trust in the CRA as a credible and dependable source of information
build clients’ confidence towards the digital solutions as efficient and reliable methods to access services and benefits securely, and
instil confidence in clients that the technological infrastructure behind these digital solutions will safeguard their privacy and protect their confidential information from security breaches.
Source: (OECD, 2023[1]).
4.4.2. Automated back-office processes
Governments are using AI techniques to improve customer experience and enhance the stewardship of their resources, for example, to automate fraud detection and to reduce time spent by civil servants on customer support and administrative tasks and. Employment and Social Development Canada (ESDC) for example is leveraging robotic process automation (RPA) to improve staff and client experience, by automating repetitive tasks, and improving response times for clients. RPA has been implemented for benefits processing (social protection, social insurance), as well as for elements of call centre management. The ESDC is also using natural language processing (NLP) to automate the review of free‑text comments received on records of employment. The system follows specific business rules and takes simple actions to help reduce the manual workload of Service Canada officers and to ensure timely payment of benefits to Canadians.
Sweden is also deploying RPA to automate decision-making in social services. A review found that digitalisation in social services has a positive effect on civil servants’ discretionary practices mainly in terms of their ethical, democratic, and professional values. In addition, a human – technology hybrid actor redefines social assistance practices (Ranerup and Henriksen, 2022[50]). Finland’s Social Insurance Institution (Kansaneläkelaitos) uses AI image recognition to automate administrative processes through document recognition. Brazil’s National Social Security Institute uses AI to speed up the identification of deceased beneficiaries, to avoid undue payments which in many countries can be a manual and thereby slow process (ISSA, 2020[49]).
The Austrian Social Insurance agency (Dachverband der österreichischen Sozialversicherungsträger) uses a voice recognition system to support call centre services by automatically forwarding requests to the appropriate offices. The system’s linguistic model, which is based on AI, has been trained to recognise specific terms. In addition, AI is used to automatically distribute emails to relevant departments, with an accuracy rate of around 93%. In addition, there is an ongoing project to implement a semi‑automated AI-based medical reimbursement process where AI is used to automate several tasks such as recognising submitted documents, classifying diagnostics according to ICD‑10 codes, and extracting the data required for reimbursement (e.g., invoice amount and IBAN). Semi‑automatic processing speeds up the reimbursement process and supports the staff involved (ISSA, 2020[49]).
As discussed already, COVID‑19 triggered an unprecedented volume of requests for assistance and benefits. In Canada, the focus during the pandemic on implementing the Emergency Response Benefit (ERB) and simplified Employment Insurance (EI) claims meant the subsequent return to regular processing of EI resulted in a backlog of claim reviews. Implementation of a Pre‑ERB EI Recalculation Outcome Prediction Machine Learning model sought to minimise the number of older claims (pre‑March 2020) requiring review by an officer. The model was used to predict the most probable outcome of each recalculation and triage the associated work items accordingly, with recalculations that were unlikely to impact claimants. This project was conducted with oversight from the Artificial Intelligence Centre of Excellence in accordance with Treasury Board of Canada Secretariat guidelines and has subsequently undergone a peer review process.
4.4.3. Proactive identification to support outreach
A small number of examples exist where AI techniques are being used to identify individuals at risk of poor outcomes to then target specific services. To enhance its support to injured workers, Korea Workers’ Compensation and Welfare Service (COMWEL) has developed the Intelligent Rehabilitation Recommendation System (IRRS). While COMWEL has been implementing customised rehabilitation plans for injured workers since 2011, the process has relied on limited information and the experience of managers in charge, resulting in variable service quality and timeliness. IRRS, an AI-based system was developed to select the injured workers with the potential to be active, and design scientifically tailored rehabilitation services for them. The IRRS calculates a vulnerability index based on administrative data on 98 million workers accumulated since 2011, comprising details about workers’ compensation, unemployment insurance, the rehabilitation case management, using rule‑based filtering and case‑based reasoning methodology.
IRRS also suggests a rehabilitation plan based on the AI model. The workers selected for rehabilitation and return to work undergo consultation with the rehabilitation experts of COMWEL before AI-generated plans are finalised. The system was first implemented in early 2020 and although it was difficult to provide rehabilitation services then due to COVID‑19, 32 627 services were provided to industrial accident workers through IRRS as a customised integrated service in the year 2020. The IRRS has helped COMWEL achieve consistent service quality nationally while ensuring timely and appropriate interventions to ultimately improve the return-to-work ratio (ISSA, 2022[44]).
The Danish Agency for Labour Market and Recruitment (STAR) has developed a profiling model using machine learning techniques that predicts the likelihood of people becoming long-term (>26 weeks) unemployed. The model combines data from administrative records and an online survey that gathers behavioural information. In collaboration with the University of Copenhagen, a new survey instrument is currently being developed that aims to capture structural personality traits such as time and risk preferences. The system is voluntary for jobseekers to use but if they do, they get full access to the model’s results. The system does not automatically refer jobseekers to active labour market programmes (ALMPs), rather it supports caseworkers who keep full discretionary responsibility (Desiere, Langenbucher and Struyven, 2019[51]).
A recent OECD working paper exploring the use of AI in Public Employment Services in OECD countries finds that almost half of PES are utilising AI to enhance their services, most commonly to match jobseekers with vacancies and to identify jobseekers’ needs for support using profiling tools (Ailbhe Brioscú et al., Forthcoming[52]).
4.4.4. Future AI deployment
It is clear from both the literature and responses provided to the OECD questionnaire that countries are planning to increase their use of AI techniques over time. Czechia’s Ministry of Labour and Social Affairs for example is implementing a gradual programme of individual AI use‑cases or proofs of concept to increase efficiency, streamline the Ministry’s operations and enable faster and better communication with clients. Use cases are evaluated looking at expected benefits; technical requirements for the preparation and operation of the solution; financial requirements for running the solution; and meeting the entry conditions for implementing the solution. Use‑cases found to be cost-effective and technically feasible will be fully implemented.
Current use cases in Czechia cover a variety of activities including:
OCR (Optical Character Recognition) for manually filled forms and their subsequent processing (OCR is a technology that recognises text within a digital image)
A Chatbot/Voicebot for communicating with clients regarding general queries on benefits and allowances – primarily State Social Support
An internal chatbot for access to methodology, guidelines and workflows
Job matching – a search for suitable jobs based on a candidate’s CV
Monitoring of topics addressed in the call centre and/or other communication channels
Fraud detection and finding anomalies in benefit claims
Designing individual action plans for employment – training, retraining, etc.
Preparation of minutes of a client meeting in a defined structure
Predicting the budgetary impact of changes in legislation.
4.5. Transformation programmes
Several countries provided examples of comprehensive change programmes utilising technology and data to revolutionise how public services are provided that over the medium to longer-term involve modernising technology platforms, changing operating models and ensuring the necessary cultural shifts. In September 2021, Japan established a Digital Agency to promote digitalisation across society. Moving forward, the government is enhancing digitalisation for administrative services while learning from other countries’ advanced initiatives.
New Zealand has begun a multi-year transformation programme known as Te Pae Tawhiti, to improve the delivery of social protection and services that is supported by a strong technology platform. Tiered services will provide the range of supports clients need – from channels providing full self-service, which will work for large numbers of clients, through to intensive case management. Norway’s NAV is also undertaking a major multi-year modernisation programme (see Box 4.12). NAV’s strategy for 2030 describes a change towards more “push-based” services. NAV wants to be able to detect changes in a service user’s circumstances and use this information to automatically adjust existing benefits and/or automatically enrol service users into benefit programmes for which they are entitled. NAV already has some experience of this with the child support benefit which is administered automatically.
Box 4.12. Protection Coverage and Social Service Delivery in Norway
NAV’s focus over the last five to ten years has been to modernise its legacy systems by automating manual processes to improve efficiency and technical quality. NAV’s systems for social protection and social services are more than 20 years old and are built on outdated technical platforms such as mainframes, and in large part with people evaluating cases and making decisions.
Modernising systems and improving efficiency will enable NAV to focus more resources on the cases that are not suited to automated systems, such as service users with complex backgrounds that require many different and intensive social protection services.
The new systems are based on modern software architecture, using open-source software and public cloud services, and are being developed using agile processes. The focus areas are:
Automating the evaluation of requirements needed to receive benefits which requires the rules to be modernised. This is done using rule‑based systems. Using an AI approach would reduce the transparency of the system and traceability of decisions, so this is not something that is currently being explored.
Increasing the compliance of the system. By centralising and automating the evaluation of relevant laws supporting social services, users receive more equal results, compared to older systems where geographically distributed groups of people with responsible for doing the same job resulted in inequitable results depending on which region the user belonged to.
The older systems have several weaknesses with regards to privacy. The modern systems are built to encompass modern privacy requirements. This includes traceability of each case, also increasing the transparency for the user.
Sustainable technology and organisation. The new systems are built by stable teams, with ownership of the systems for the complete lifecycle, reducing the transfers between teams, with a more project-based mindset.
To achieve the goals of the modernisation plans, NAV is dependent on the quality of the data used as the base for the new automated services. There is a national programme for collecting the work and income data from employers, in a unified and systematic manner. This programme is a co‑operation between different national agencies and integrates with commercial systems that are used for managing employment and salaries. The transformation from manual to automated systems changes the requirements NAV have of these data. Data streams will need to be event based and have the possibility to listen to streams of events being captured across the Norwegian Government. Manual systems are better at handling ambiguity and low-quality data, whilst automated systems need data to be structured, consistent with high resolution.
Relevant laws will also need to be modernised, as many of them require a service user to apply for a benefit.
Source: (OECD, 2023[1]).
References
[52] Ailbhe Brioscú et al. (Forthcoming), “A New Dawn for Public Employment Services: Service Delivery in the Age of Artificial Intelligence”, OECD, Paris, http://oe.cd/ai-pes-2024.
[26] Burattini, B. et al. (2022), Digital innovations in delivering social protection in rural areas: Lessons for public provisioning during the post-pandemic recovery and beyond, Food and Agricultural Organization of the United Nations and International Policy Centre for Inclusive Growth., Rome and Brasília.
[23] CBSS (2023), Crossroads Bank for Social Security, http://www.ksz-bcss.fgov.be/nl/information-in-english (accessed on May 2024).
[30] Chetty, R. et al. (2018), “The Opportunity Atlas: Mapping the Childhood Roots of Social Mobility”, National Bureau of Economic Research Working Paper Series, Vol. No. 25147, https://doi.org/10.3386/w25147.
[2] Chirchir, R. and V. Barca (2020), “Building an integrated and digital social protection information system”, Deutsche Gesellschaft fur Internationale Zusammenarbeit (GIZ) GmbH, Bonn.
[12] CNAF (2023), The current reform of “at-source solidarity”: towards an automation of social benefits delivery in France.
[9] Commonwealth Ombudsman (2019), Automated Decision-making - Better Practice Guide, Commonwealth Ombudsman, the Office of the Australian Information Commissioner and the Attorney-General’s Department.
[16] Department of Work & Pensions (2021), Universal Credit Statistics: 29 April 2013 to 10 December 2020, https://www.gov.uk/government/statistics/universal-credit-statistics-29-april-2013-to-10-december-2020 (accessed on 26 October 2023).
[15] Department of Work & Pensions (DWP) (2020), Annual Report and Accounts 2019–2020, HC401.
[51] Desiere, S., K. Langenbucher and L. Struyven (2019), Statistical profiling in public employment services: An international comparison, OECD Publishing, Paris.
[31] Dillon Consulting (2018), Analyzing 211 Rural Unmet Service Needs, Rural Ontario Institute, http://211ontario.ca/wp-content/uploads/2018/11/ROI-211-Final-Report-Nov-23-2018.pdf.
[24] Economic Commission for Latin America and the Caribbean (ECLAC) (2023), Institutional Frameworks for Social Policy in Latin America and the Caribbean: a Central Element in Advancing towards Inclusive Social Development (LC/CDS.5/3).
[14] European Commission (2021), The EU Digital COVID Certificate: a global standard with more than 591 million certificates, https://digital-strategy.ec.europa.eu/en/news/eu-digital-covid-certificate-global-standard-more-591-million-certificates (accessed on 3 January 2024).
[10] European Law Institute (2022), Model Rules on Impact Assessment of Algorithmic Decision-Making Systems Used by Public Administration.
[37] Finkelstein, A. and M. Notowidigdo (2019), “Take-Up and Targeting: Experimental Evidence from SNAP”, The Quarterly Journal of Economics, Vol. 134/3, pp. 1505-1556, https://doi.org/10.1093/qje/qjz013.
[19] Frechon, I. and M. Marpsat (2016), “Placement dans l’enfance et précarité de la situation de logement”, Économie et Statistique 488-489, https://www.insee.fr/fr/statistiques/2123144?sommaire=2123156.
[35] Global Pulse (2016), Integrating Big Data into the Monitoring and Evaluation of Development Programmes, https://thecompassforsbc.org/wp-content/uploads/Integrating-Big-Data-into-the-Monitoring-and-Evaluation-of-Development-Programmes.pdf.
[41] Graber, R. et al. (2023), Making the invisible epidemic visible New approaches can help connect domestic violence survivors to services, Brookings.
[5] Griffiths, R. (2021), “Universal Credit and Automated Decision Making: A Case of the Digital Tail Wagging the Policy Dog?”, Social Policy and Society, pp. 1-18, https://doi.org/10.1017/S1474746421000749.
[7] Hyee, R. and H. Immervoll (2022), The new work incentive for Spain’s national Minimum Income Benefit. Policy issues and incentives in the international comparison, https://www.oecd.org/social/benefits-and-wages/Note-on-the-new-work-incentive-Spain.pdf.
[22] ISSA (2024), Social security developments and trends – Europe 2024, ISSA, Geneva.
[48] ISSA (2022), Artificial intelligence in social security institutions: The case of intelligent chatbots.
[44] ISSA (2022), Data-driven innovation in social security: Good practices from Asia and the Pacific.
[49] ISSA (2020), Artificial Intelligence in Social Security: Background and Experiences.
[47] ISSA (ed.) (2020), Transforming Estonian Government with AI, ISSA.
[46] Joint Research Centre, E. (2021), AI Watch - National strategies on Artificial Intelligence: A European perspective, 2021 edition.
[34] Langedijk, S., I. Vollbracht and P. Paruolo (2019), “The potential of administrative microdata for better policy-making in Europe”, in Crato, N. and P. Paruolo (eds.), Data-Driven Policy Impact Evaluation - How Access to Microdata is Transforming Policy Design, Springer Open, Cham, https://doi.org/10.1007/978-3-319-78461-8.
[17] Marchetti, S. et al. (2015), “Small Area Model-Based Estimators Using Big Data Sources”, Journal of Official Statistics, Vol. 31/2, pp. 263-281, https://doi.org/10.1515/jos-2015-0017.
[43] Marr, B. (2018), How The UK Government Uses Artificial Intelligence To Identify Welfare And State Benefits Fraud, Forbes.
[29] McKinsey & Company (2021), Accelerating data and analytics maturity in the US public sector, https://www.mckinsey.com/industries/public-sector/our-insights/accelerating-data-and-analytics-maturity-in-the-us-public-sector (accessed on 3 January 2024).
[36] Meyer, B. and N. Mittag (2019), “Using Linked Survey and Administrative Data to Better Measure Income: Implications for Poverty, Program Effectiveness, and Holes in the Safety Net”, American Economic Journal: Applied Economics, Vol. 11/2, pp. 176-204, https://doi.org/10.1257/app.20170478.
[18] National Institute of Statistics and Geography (INEGI) (2022), National Survey on the Dynamics of Relationships in Households (ENDIREH) 2021, https://www.inegi.org.mx/programas/endireh/2021/ (accessed on 3 January 2024).
[32] Nichola Shackleton (2021), Understanding service use at a local level Area-level attendance at B4 School Checks, Social Wellbeing Agency, Wellington, New Zealand.
[8] Observatory of Public Service Innovation (2020), Proactive Family Benefits, OECD, Observatory of Public Service Innovation, https://oecd-opsi.org/innovations/proactive-family-benefits/ (accessed on May 2024).
[13] OECD (2023), Benefit Reforms for Inclusive Societies in Korea: Income Security During Joblessness, OECD Publishing, Paris, https://doi.org/10.1787/96b7fd64-en.
[4] OECD (2023), “Executive summary”, in OECD Employment Outlook 2023: Artificial Intelligence and the Labour Market, OECD Publishing, Paris, https://doi.org/10.1787/34f4cc8d-en.
[25] OECD (2023), OECD Economic Surveys: Costa Rica 2023, OECD Publishing, Paris, https://doi.org/10.1787/8e8171b0-en.
[40] OECD (2023), Supporting Lives Free from Intimate Partner Violence: Towards Better Integration of Services for Victims/Survivors, OECD Publishing, Paris, https://doi.org/10.1787/d61633e7-en.
[1] OECD (2023), The OECD questionnaire: Harnessing Technology and Data to Improve Social Protection Coverage and Social Service Delivery, OECD, Paris.
[20] OECD (2022), Assisting Care Leavers: Time for Action, OECD Publishing, Paris, https://doi.org/10.1787/1939a9ec-en.
[28] OECD (2022), The OECD Good Practice Principles for Public Service Design and Delivery in the Digital Age, OECD, Paris.
[33] OECD (2020), OECD-EC project on policy impact evaluation through the use of linked administrative and survey data, OECD, https://www.oecd.org/els/emp/impact-evaluation-linked-data.htm (accessed on May 2024).
[42] OECD (2019), OECD Framework for the Classification of AI systems.
[6] OECD (2014), OECD Recommendation on Digital Government Strategies, OECD, Paris.
[21] OECD (forthcoming), Better capturing the experiences of homelessness among women, Joint Research Centre.
[45] Ohlenburg, T. (2020), AI in Social Protection – Exploring Opportunities and Mitigating Risks, Deutsche Gesellschaft für Internationale Zusammenarbeit (GIZ) GmbH, Bonn.
[50] Ranerup, A. and H. Henriksen (2022), “Digital Discretion: Unpacking Human and Technological Agency in Automated Decision Making in Sweden’s Social Services”, Social Science Computer Review, Vol. 40/2, pp. 445-461, https://doi.org/10.1177/0894439320980434.
[39] Rea, D. and D. Hyslop (2023), “Using a difference-in-difference control trial to test an intervention aimed at increasing the take-up of a welfare payment in New Zealand”, Observational Studies, Vol. 9/4, pp. 49-72, https://doi.org/10.1353/obs.2023.a906626.
[11] Sebastião Barros Vale and Gabriela Zanfir-Fortuna (2022), aking Under the GDPR: Practical Cases from Courts and Data Protection Authorities, Future of Privacy Forum.
[38] TAKE-Project (n.d.), Methodology, https://takeproject.wordpress.com/methodology/ (accessed on 6 August 2019).
[3] Verhagen, A. (forthcoming), Using AI to Manage Guaranteed Minimum Income Benefits: Opportunities, Risk and Possible Policy Directions, OECD Publishing, Paris.
[27] Wilson, M. and K. McLeod (2023), “How the Families Package increased income and created new opportunities for lifecourse research”, Policy Quarterly, Vol. 19/4.
Notes
← 1. Machine learning (ML) is a set of techniques to allow machines to learn in an automated manner through patterns and inferences rather than through explicit instructions from a human. ML approaches often teach machines to reach an outcome by showing them many examples of correct outcomes. ML contains numerous techniques that have been used by economists, researchers and technologists for decades. These range from linear and logistic regressions, decision trees and principal component analysis to deep neural networks (OECD, 2019[42]).
← 2. The OECD has recently clarified the definition of an AI system contained in the 2019 OECD Recommendation on AI (the “AI Principles”) to support their continued relevance. The following updated definition was adopted by the OECD Council on 8 November 2023:
An AI system is a machine‑based system that can, for a given set of human-defined explicit or implicit objectives, infers, from the input it receives, how to generate outputs such as makes predictions, content, recommendations, or decisions that can influence physical real or virtual environments. Different AI systems are designed to operate with varying vary in their levels of autonomy and adaptiveness after deployment.