Modernising Access to Social Protection
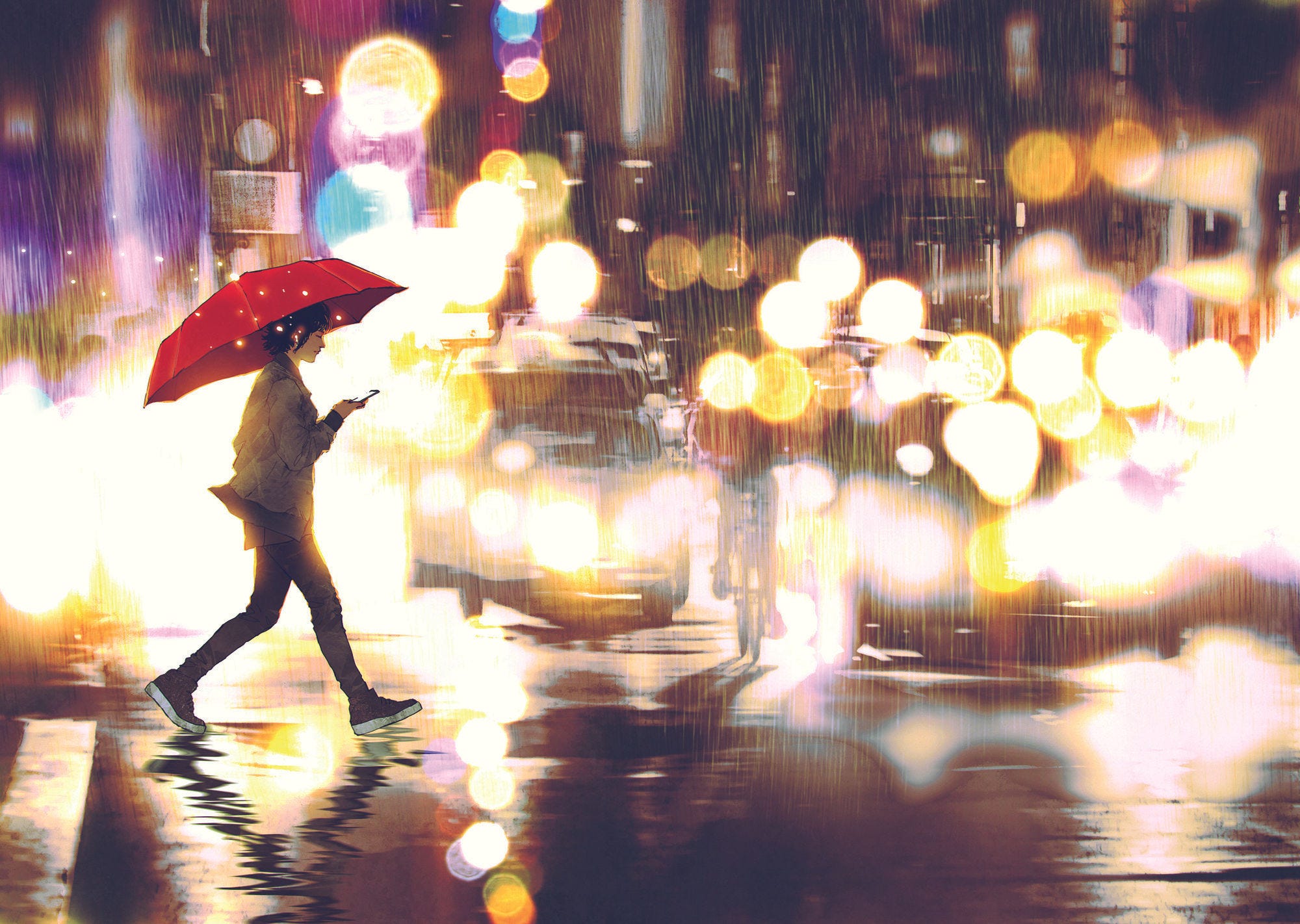
5. Managing the challenges of leveraging technology and data advances to improve social protection
Abstract
There are significant risks and challenges associated with deploying advanced digital technologies and data in social protection. Governments have put considerable effort into measures to mitigate the risks, including legal, regulatory and accountability frameworks to protect people’s privacy and to govern use of automated systems. Some countries are now going beyond these measures, implementing initiatives that also improve their overall interactions with citizens and modernise the way they do business, such as offering services through multiple channels, involving service users in solution design, and achieving incremental improvements through agile working methods. This chapter also discusses some of the broad range of capacities required to successfully meet the challenges of deploying digital solutions such as effective governance, a leadership culture that promotes innovation, an appropriately skilled workforce, and investments in modern technology infrastructure.
Key findings
The previous chapter in this report explores the different approaches countries are taking to leverage advances in technology and data to improve the design, delivery and coverage of social protection benefits and services. However, this does not come without considerable challenges and risks. Furthermore, while the potential benefits may be significant, they are uncertain and often only materialise in the longer-term. This chapter discusses the challenges governments face as they increasingly digitalise social protection systems, together with the measures they are adopting to manage those challenges. The literature and countries’ responses to the OECD’s questionnaire Harnessing Technology and Data to Improve Social Protection Coverage and Social Service Delivery (OECD, 2023[1]) highlight the following key issues and measures:
Technology projects can fail if the foundations that underpin and enable technological improvements are not in place. A wide range of foundations are necessary for building digital capacity, including supportive policy, legal and operational environments, the availability of a range of specialised skills, and modern technology infrastructure.
Technology improvements and innovations – particularly those aimed at better integration – can touch on and significantly alter the operational processes of a range of government agencies and other providers. This requires a high degree of cross-governmental collaboration which takes considerable organisational (and sometimes political) commitment, time and resources.
Data sit at the heart of much government innovation and as countries increasingly collect, link and share more data, countries are considering how to manage the risks involved to make the most of the vast amount of data being generated in social systems.
Commonly, data used for social protection purposes are people’s personal information and governments have a duty to protect people’s privacy when using their data. While legislation and rules exist to regulate the use of rapidly evolving technology and data, they are often complex and difficult to navigate making it challenging for agencies to act safely and effectively.
Discriminatory biases can be built into automated processes and decision-making. Public confidence in governments’ use of advanced technology and data solutions takes time to build and can be quickly lost. The possibility of errors and/or biases, particularly in relation to already disadvantaged populations, and the potential implications of those errors requires there to be transparent procedures in place that explain how people’s information is being used together with protections and controls for addressing any issues if they occur.
Greater use of data-driven and/or digitalised processes in social protection creates the risk of reinforcing or creating new sources of exclusion and disadvantage for some groups. Increased digitalisation can exclude those individuals who have limited access and/or ability to engage with digital services. This is a particular challenge when people with limited digital access are also key priority groups for social protection measures.
Governments are seeking to optimise the benefits of rapid developments in technology and data while mitigating the risks involved with instruments such as legal and regulatory frameworks for example to protect people’s privacy and to govern data management and use.
Governments are also going beyond these measures, implementing initiatives that improve their overall interactions with individuals and communities, that enhance public trust and confidence and modernise the way they do business, including offering services through multiple channels, involving service users in solution design, achieving incremental improvements through agile working methods and encouraging innovative technology and data cultures through leadership and champions.
5.1. Introduction
Significant benefits can be realised from harnessing technology and data advances to enhance national social protection systems, from improving the effectiveness and timeliness of social programmes, for example the speed at which benefits can be scaled up and down to expanding benefit provision to a larger share of eligible beneficiaries, examples of which are outlined in Chapter 4 in this report and (Verhagen, forthcoming[2]).
Many social benefits – even in the world’s wealthiest countries – do not reach all intended recipients (Chapter 1). Many individuals across OECD countries feel they cannot access benefits easily in times of need (Chapter 1, Figure 1.4), and people do not always enrol in benefit programmes for which they are eligible. They may have little or no information about a benefit or its eligibility criteria, and/or entitlement rules may be perceived as too complex or cumbersome. The application process can also make a programme less accessible: it may be bureaucratically cumbersome, requiring time, education, and other resources that potential claimants may not have, for different reasons (Chapter 2 and (OECD, 2023[3])).
Incomplete take‑up of benefits leads to suboptimal outcomes. When groups or individuals miss out on the social benefits for which they are eligible, benefits become less effective for poverty reduction, income smoothing and risk management. Importantly, poor benefits coverage may also prevent eligible individuals from accessing services that are tied to benefit receipt, such as job-search support and other active labour market policies. Ineffective social services can also contribute to poorer outcomes and inefficient use of resources.
While technology and data advances can play an important role in improving the design, delivery and coverage of social protection benefits and services, they also create complex challenges for governments, and the risks involved in adopting new digital and data technologies can be significant (Verhagen, forthcoming[2]). Governments are attempting to strike a balance between undertaking necessary transformations and mitigating the risks involved in doing this (ISSA, 2023[4]). For example, the Department of Work and Pensions (DWP) in the United Kingdom has created an Artificial Intelligence (AI) Lighthouse Programme to safely explore their use of emerging Generative AI technology; one project is looking explicitly at supporting the interaction between a Work Coach and a citizen when face‑to-face in a Job Centre. DWP see significant opportunities in using AI but are also very aware of the potential risks of such technology and have established a framework and process to explore such technology in a safe, ethical and transparent way.
This chapter first explores the challenges governments are facing as they increasingly utilise advanced technologies and data. Key challenges emerging from the literature and case studies provided in response to the OECD Questionnaire are discussed. These include mobilising the necessary capacities and enablers, getting the data right and using it appropriately, and mitigating the risks of further entrenching bias, discrimination and exclusion through accountability mechanisms and processes. Secondly, the chapter outlines some of the measures countries are adopting to manage those challenges, discusses lessons learned and potential ways forward for governments as a result.
5.2. The challenges of leveraging technology and data advances
5.2.1. Mobilising the necessary capacities and enablers
Digitalisation cannot be an objective of its own, as digital solutions only improve the provision of services if they are fulfilling their objectives well and are adopted by users. Thus, added value and user-friendliness are critical factors for digital platforms supporting service provision (OECD, 2022[5]). To ensure technology and data-driven improvements and innovations are successful, governments must first ensure a broad range of capacities and enablers are in place. Those capacities include the policy landscape, governance and leadership, operating environments, human resources, co‑operation across different levels of government, and investments in modern technology infrastructure to support advanced digitalisation projects.
Policy landscape
The policy landscape required to support increased and advanced uses of technology and data to improve public services including social protection coverage is multi-faceted. It includes legal, regulatory and governance frameworks, risk management models, strategies e.g., for promoting digital inclusion, clarifying data sovereignty and ownership issues and policy settings e.g., to avoid or manage the misuse of data. Embracing the results from greater use of technology and data can present significant challenges to the status quo and demands redirection of government resources, improved agency collaboration, changes to service delivery models, improved individual-level data, and better monitoring of policy and service outcomes.
Advanced uses of technology and/or data often requires an enabling legislative ecosystem which can include enabling general legislation such as privacy laws as well as changes to content-specific legislation (refer Chapter 4 for discussion on Article 22 of the EU’s GDPR). For example, in the Slovak Republic a legislative amendment was a prerequisite to the creation of a new proactive service for the citizen in the provision of a childbirth allowance upon the birth of a child. The childbirth allowance is a state social benefit, which the state provides proactively without the participation of a beneficiary (see Box 5.1).
Ideally the policy landscape will align with a government’s overall vision for digital government. International organisations are supporting governments’ efforts to realise digital transformation with legal instruments like the OECD’s 2014 Recommendation of the Council on Digital Government Strategies. The Recommendation offers a whole‑of-government approach that addresses the cross-cutting role of technology in the design and implementation of public policies, and in the delivery of outcomes. It emphasises the crucial contribution of technology as a strategic driver to create open, innovative, participatory and trustworthy public sectors, to improve social inclusiveness and government accountability, and to bring together government and non-government actors to contribute to national development and long-term sustainable growth (OECD, 2014[6]).
Digital government strategies need to become firmly embedded in mainstream modernisation policies and service design so that relevant stakeholders outside of government are included and feel ownership for the final outcomes of major policy reforms. The OECD recommends that strategies for effective digital government need to reflect public expectations in terms of economic and social value, openness, innovation, personalised service delivery and dialogue with people and businesses. In the Communiqué of the Meeting of the Public Governance Committee at Ministerial Level held in Venice in November 2010, Ministers acknowledged the importance of technology as key ally to foster innovation in governance, public management and public service delivery, and to build openness, integrity and transparency to maintain trust, acknowledging that trust in government is one of the most precious national assets (OECD, 2010[7]).
Box 5.1. Legal amendments as a prerequisite for automatic enrolment (Slovak Republic)
Several legal amendments were required for the Slovak Republic to automatically provide a childbirth allowance upon the birth of a child (a state‑provided social benefit) without any involvement from the beneficiary.
Changes and amendments to certain measures were required in several Acts, for instance to reduce the administrative burden by using public administration information systems. The government also had to amend and supplement certain Acts on the childbirth allowance and the allowance for multiple children born at the same time.
Source: (OECD, 2023[1]).
Governance and leadership
A critical enabler to support the more systemic use of technology and data (and possibly one of the most challenging enablers to affect) is the leadership required to execute the necessary change(s) to fully realise the value of technology advances. This includes leadership at the political and senior management level as well as at the functional and technical levels. Greater use of data in decision-making requires shifts in mind-sets, priorities and ways of working where there may be resistance due to other “business-as-usual” pressures and hard, inconvenient questions that can emerge with deeper data analysis. By way of example, quantitative evaluations may show programmes that have strong stakeholder and/or political support to be ineffective or of low impact.
Public trust, sometimes referred to as social licence, is important when scaling digital and data-driven innovations and automated decision-making. The OECD’s Good Practice Principles for Public Service Design and Delivery in the Digital Age promotes three principles that will help to achieve accountability and transparency in the design and delivery of public services to reinforce and strengthen public trust: be open and transparent in the design and delivery of public services, ensure the trustworthy and ethical use of digital tools and data, and establish an enabling environment for a culture and practice of public service design and delivery (OECD, 2022[8]).
These principles can be hard to observe. Initiatives are not always well publicised, the roll-out of new web-based applications is not always smooth, there may be general resistance to changing a system that “works”, and the public may not be able to easily access information about whether developments are pilots or fully operational. Governments and social security institutions may also not want to openly publicise the results from pilots or trials in cases where they did not achieve the desired results.
Public trust takes time to build and can be quickly lost. Prior poor experiences with government agencies, negative media stories and general distrust in governments can exacerbate doubts about governments’ ability to manage digital and data-driven innovations. (Wagner and Ferro, 2020[9]). Indeed, 81% of respondents to a cross-national survey covering 36 countries reported that a negative experience would decrease their level of trust in the government (Mailes, Carrasco and Arcuri, 2021[10]). More pointedly, in a US survey on attitudes to AI development and governance, just 27% of respondents said they have “a great deal of confidence” or “a fair amount of confidence” that the US federal government could develop AI. By contrast, 32% had “no confidence” that the US federal government could develop AI (Zhang and Dafoe, 2019[11]). Data from the OECD’s Trust Survey indicates that only about one‑third of respondents across 22 countries believe a public agency would even adopt innovative ideas to improve public service provision (OECD, 2022, p. 80[12]).
The Data Innovation Program in Canada illustrates one way in which trust was built with citizens over time. Because the project requires individuals’ consent for data sharing and use to be sought upfront, the project is both time and resource intensive but the benefits as a result are considered worthwhile (Box 5.2). It is important to note however that obtaining consent when using very large, national data sets is often difficult if not impossible. Some countries are working with their relevant national privacy body to develop better approaches that help build public confidence.
Box 5.2. Building trust through voluntary data sharing arrangements (British Colombia, Canada)
The Data Innovation Program consistently links, de‑identifies and provides access to administrative datasets in one secure environment and is available for use by government analysts and academic researchers to conduct population-level research. The Program aims to address a previous lack of a whole‑of-government approach to data sharing and usage, which made data-driven decision-making incomplete, time‑consuming, and resource intensive.
A key challenge the Program faces is data sharing and acquisition and in response to this challenge a critical success factor is that data sharing is voluntary. However, this means there is significant up-front time required to build trust with data providers.
The challenge has been approached through the following steps:
taking the time to educate potential data providers on the Program governance model,
developing a framework that allows data providers (government agencies) to maintain control over access to the data they provide, with the opportunity to pre‑review publications developed using that data; and
starting small and using completed research projects to demonstrate that the Program is a responsible data custodian.
Source: (OECD, 2023[1]).
Operating environments
Digitalisation represents a major opportunity to enhance service effectiveness and efficiency, via interfaces for people using the services, as well as the back-office infrastructure for service providers to deliver knowledge‑based services and automate administrative processes. The extent to which the benefits of digitalisation are realised in practice depends crucially on how the digital infrastructure is implemented and successful implementation relies in large part on operating environments that are ready or mature enough for greater digitalisation.
Since digitalisation efforts can fundamentally change the way organisations work, they may involve considerable structural change and/or standardisation in the way government departments, agencies, and providers are organised and operate. For example, Belgium’s Crossroads Bank for Social Security (discussed in more detail in Chapter 4) required the back-office functions of all 3 000 organisations involved to be restructured and the organisational processes to be re‑engineered and automated. Similarly, albeit on a smaller scale, New Zealand’s efforts to provide digitised services for new parents and caregivers through SmartStart (Box 5.3) required several agencies to adapt and co‑ordinate their processes. A key feature of these re‑organisation efforts is a focus on providing more customer-centric services and ensuring this remains the key goal requires engagement with external stakeholders, advocacy groups and service users themselves. The risk of not adapting organisational structures and processes is that technology enhancements are fragmented, projects are unsuccessful or worse still, lead to poorer outcomes. Simply automating processes may replicate existing errors and inefficiencies.
Box 5.3. SmartStart in New Zealand
SmartStart is an online tool aimed at parents and caregivers who are planning to or about to have a baby. It gives people online access to integrated government information, services and support related to each phase of pregnancy and early childhood development up to six years of age.
Using SmartStart, an expectant parent can create a profile and add their due date to personalise the timeline with key dates that align with the important tasks they need to complete, such as choosing a lead maternity carer. Parents and caregivers can get tips on keeping themselves and their baby or child healthy and safe, as well as contact details for organisations that can offer help and support.
Users can also complete specific tasks online such as registering the birth of a new baby. As part of the same process, users can consent to sharing their baby’s registration information with Inland Revenue to apply for an Inland Revenue number for their baby and Best Start payments, and with the Ministry for Social Development to update their benefit entitlement details. They can also complete a Childcare Subsidy application and submit the form online. Users are invited to apply for a new post-natal tax credit “Best Start” through SmartStart. As part of this process, families give consent for Inland Revenue to use the information they provide to determine their eligibility for other Working for Families tax credits. This appears to have resulted in high take‑up of Best Start. Take up of other Working for Families tax credits has also increased with the increase particularly pronounced for Asian mothers, a group is estimated to have had particularly low take‑up in preceding years.
A key challenge with this integrated service is that government agencies need to think broader than their own ministerial deliverables, strategically, operationally and technically. To ensure a modern, more joined-up and citizen-focused public service, the focus must be on the customer, and their needs, and not on the agency.
Building government digital services means more than offering new digital services. It means changing existing processes and practices, changing the functions of existing teams, and often integrating more than one different agency’s processes and practices into a single customer experience. Progressing such change is far more challenging than the development of a new online service.
Source: (OECD, 2023[1]).
Attracting, developing and retaining talented staff
To support a shift towards digital government, investment is needed in developing the skills of civil servants (Burtscher, S. Piano and B. Welby, 2024[13]). Social security organisations need to attract, develop and retain staff who are equipped for ongoing digital transformation, people with the necessary skills and mindsets. A continuum of skills is required, from frontline staff and senior decision-makers (who are confident using data to make decisions) at one end of the continuum who may need to be data aware and/or data capable to technical experts at the other. A recent OECD working paper that reviews good practices across OECD countries to foster skills for digital government presents different approaches in public administration to providing both training activities and informal learning opportunities. It also provides insights into how relevant skills can be identified through competence frameworks, how they can be assessed, and how learning opportunities can be evaluated (Burtscher, S. Piano and B. Welby, 2024[13]).
A broad range of technical expertise is necessary, for example, to collect, organise, and analyse data across different institutions; to exploit new data sources to better inform policy making; to improve the technical infrastructure; and to evaluate programme effectiveness. Specialised staff are also needed to interrogate, evaluate and keep systems and models up to date. The ability to evaluate systems is crucial not only for their basic functioning but also to ensure that they are not discriminatory or regenerating pre‑existing bias.
The skills required are even more specialised the more advanced and complex the emerging systems of data and analytics become (Redden, Brand and Terzieva, 2020[14]). Many relevant skills are already in short supply, in both social security institutions and in the broader labour market. For example, the Canada Revenue Agency experienced inefficiencies in their Chat Services Project relating to a lack of specialist staff to undertake a complex project that was treading new ground and being innovative. Given rapidly developing technologies, skill requirements will likely increase and change over time, which risks exacerbating the human resource challenges organisations face (ISSA, 2022[15]; Ranerup and Henriksen, 2020[16]). In addition, the public sector can struggle to compete with private sector salaries for highly sought after technical roles such as data scientists.
Given that certain skill in addition to specialised technical skills are necessary to support digitalisation efforts, for example content experts and behaviouralists, organisations can benefit from having multidisciplinary teams (ISSA, 2022[15]; OECD, 2022[17]). While some expertise can be developed within social security institutions, it is not always straightforward or desirable for welfare officials to transition from claims processing and benefit design to managing data innovation and advanced analytics projects. As such, the effectiveness of digital and data-driven improvements and innovations may depend on the way welfare officers interact with the system (Lokshin and Umapathi, 2022[18]).
Welfare experts are still required to interact with service users and for their knowledge about the needs of those service users, application processes and available service providers. When Sweden introduced automatic social assistance decision-making, welfare officers’ roles changed, but they were still needed to offer help and support to applicants as they underwent the process of applications and appeals in the automatic system (Ranerup and Henriksen, 2020[16]).
Multidisciplinary teams can be particularly valuable when deploying AI and predictive models, to help to ensure that decisions generated by these advanced analytical methods are accurate, explainable, and fair. AI is increasingly focused on how to act in unknown and complex situations. It will therefore be important to evaluate its performance against a range of metrics, informed by different fields, including statistics, philosophy and social science (ISSA, 2020[19]).
Cross-government co‑operation
Social protection systems sit within broader system and policy settings such as education, health, employment and tax policy, family and children policy, housing, legal aid and financial services (McClanahan et al., 2021[20]). Successful implementation of digital solutions aimed at improving social protection may require co‑operation across government agencies which can be costly in terms of time and financial resources making technology solutions, particularly those requiring significant co‑ordination, difficult to achieve in practice (OECD, 2022[8]; McClanahan et al., 2021[20]).
The “Chile Grows with You” (Chile Crece Contigo) policy for example which was implemented in 2006 as a holistic approach to early childhood development benefits and services had to scale back ambitions for a high degree of cross-sectoral co‑ordination. While in principle, the policy envisaged a high degree of cross-sectoral co‑ordination and even full integration, including shared policy making, one study found that co‑ordination was in fact limited to inter-sectoral financial transfers from the lead ministry (Ministry of Social Development) to other ministries involved. Multi‑agency plans and budgets were not prepared, followed, or assessed. Rather, co‑ordination in practice was limited to identifying performance indicators and sectoral contractual agreements. The education sector was not included in key decisions at all, despite the implications of the policy for it (McClanahan et al., 2021[20]).
Effective co‑operation and co‑ordination are particularly important when a project requires government agencies to share data. This requires not only a mutual willingness to co‑operate, but also practical agreements for shared resources, regulations and infrastructure (OECD, 2023[21]). Australia experienced this when developing the National Disability Data Asset using the new Data Availability and Transparency Act to undertake a multi‑agency data sharing project. Through the initiative Australia has found that to successfully establish multi‑agency arrangements requires commitment, time, co‑operation, and mutual respect – both vertical (different levels of government) and horizontal (different levels within government, from officer to Ministerial level) co‑operation.
Challenges involved in reaching practicable information-sharing agreements are also highlighted in a Canadian example where issues around ownership and control of data, particularly for Indigenous populations, has required active collaboration within and between federal, provincial and territorial governments (Box 5.4).
Box 5.4. Cross-government information sharing as a key challenge for service digitalisation in Canada
While advancements in service digitalisation have accelerated in Canada over the past five years, information sharing across governmental entities and between levels of government remains a gap in the current Canadian context. Privacy and enabling programme legislation, data security requirements, in addition to Ownership, Control, Access, and Possession (OCAP) considerations for First Nations, Indigenous, and Métis populations are all elements that require review and analysis. Adjustments will be necessary to ensure that when data are shared, all laws and regulations are respected.
These elements are under active exploration and collaboration within and between Federal/Provincial/Territorial government officials. The establishment of a Digital ID is a key file being advanced at the most senior levels across Federal/Provincial/Territorial governments, with a view to also enabling OCAP for all Digital ID users and removing barriers to data sharing within and across governments. Shared credentialing use is also expanding.
Source: (OECD, 2023[1]).
Investing in the necessary infrastructure
Modern IT infrastructure and processes are essential foundations for the provision of effective digitalised public services. In many cases it will be necessary to modernise existing infrastructure prior to or in conjunction with digital and data transformation(s). In 2021‑22 the OECD supported Lithuania to develop a new approach to personalised services for people in vulnerable situations which included reviewing Lithuania’s IT infrastructure. The OECD recommended that Lithuania modernise the IT infrastructure for both social and employment services to better support service provision including digital service offerings, involving end-users throughout each phase of the modernisation process (OECD, 2023[22]).
Investments in IT transformations require well-scoped and costed business cases to convince governments to make what are often significant investments in digital systems, particularly given potential benefits can be uncertain and often materialise in the longer-term. The complexity of designing and iteratively implementing an integrated digital system that fully responds to the changing needs of users at all levels of administration, while also placing people at the centre, is often under-estimated. The time and cost, not only for set-up, but also for take‑up, maintenance and continuous adaptation, needs to be considered. Ultimately, the cost for people to access and use a system needs to be minimal, and the benefits tangible to all. If the benefits are not visible, the risk is failure i.e., the new system is not used, or worse, creates significant setbacks (Barca and Chirchir, 2019[23]).
Investment cases should also consider the needs of marginalised groups who may lack access to the infrastructure and skills necessary to benefit from technology advancements. For example, Internet connections may be sparse or unreliable in rural or geographically isolated areas, some groups may not have access to devices. In addition, there may be skill gaps for current and potential applicants that need to be addressed.
Depending on the extent of infrastructure development or modernisation required governments may not have all the necessary capabilities and capacities and while development and maintenance tasks can be contracted out, governments should take care to ensure they retain system ownership. Private development partners may play a helpful role in building and maintaining technical solutions. For instance, the pension insurance DRV-Bund in Germany was able to use technology from a major cloud provider to cut costs involved with integrating a chatbot into its website (ISSA, 2022[24]). Likewise, British Colombia partnered with an academic institution to support the development of its Data Innovation Programme. However, governments may expose themselves to both short- and long-term risks if they do not retain ownership of systems and data when managing their public-private partnerships. This is particularly important where social protection organisations are nascent and evolving, such as in low- and middle‑income countries (Barca and Chirchir, 2019[23]).
5.2.2. Getting the data right
Countries are increasingly collecting, sharing and using more data. Some countries are creating new data, for example through increased linking of administrative datasets across government agencies and making that data more widely available in useable formats. A small number of countries are also testing the value of using new or non-traditional data sources such as cellular phone or banking data for policy and research and to improve service design and delivery. Governments are carefully considering how they manage the challenges of optimising the value of their expanding data holdings.
Greater use of data can help to drive efficiency, effectiveness and innovation. However, if something goes wrong, for example sensitive information is made available when it should not have been, it may harm not only the individual(s) involved but also damage public trust and confidence. This is particularly acute in social services where much of the information used is people’s personal, and often highly sensitive, information. For instance, if abusers of victims/survivors of domestic violence access classified information through privacy leaks, they may expose their victims to further violence (OECD, 2023[21]). Another example is the potential misuse of a person’s health data by an employer to discriminate against them in the workplace.
The answer however is not to avoid the use of data because of potential harms. There are both individual and public benefits to providing social services for example and evaluating their effectiveness. While Article 12 of the International Bill of Rights states that no one shall be subjected to arbitrary interference with their privacy, family, home or correspondence, Article 27 specifies that everyone has the right to freely participate in the cultural life of the community, to enjoy the arts and to share in scientific advancement and its benefits; arguably this includes the right to benefit from data and technology advancements. All OECD countries have legal safeguards in place to mitigate the risks associated with the collection, use and disclosure of personal information to ensure information is used in a responsible, transparent, and trustworthy way. There is also an increasing number of ways in which countries are protecting people’s data that go beyond laws and regulations, some of which are described below.
Data governance
Good data governance plays a fundamental role in helping governments and agencies become more data driven as part of their digital strategy and is critical to governments maximising the benefits of data access and sharing, while addressing related risks and challenges. The OECD describes data governance as a diverse set of arrangements, including technical, policy, regulatory or institutional provisions, that affect data and their cycle (creation, collection, storage, use, protection, access, sharing and deletion) across policy domains and organisational and national borders (OECD, 2024[25]). The characteristics of a mature data organisation might include data informing a continuous evolution of business strategy, the organisation constantly looking for ways to leverage new datasets, the right data protection measures being in place and data governance integrated into business processes.
Enabling the right cultural, policy, institutional, organisational, and technical environment is necessary to realising the value from data. Yet, organisations often face legacy challenges inherited from analogue business models, ranging from outdated data infrastructures and data silos to skill gaps, regulatory barriers, the lack of leadership and accountability, and an organisational culture which is not prone to digital innovation and change. New challenges have also arisen resulting from the misuse and abuse of peoples’ data. Furthermore, governments struggle to keep up with technological change and to fully understand the policy implications of data in terms of trust and basic rights (OECD, 2019[26]).
To achieve a data driven public sector the OECD proposed a holistic data governance model comprising three core layers (strategic, tactical and delivery) (OECD, 2019[26]). The strategic layer includes leadership, vision and national data strategies e.g. a data sovereignty strategy in countries with an indigenous and/or ethnic minority population whose conception of data is not the same as the democratic regime. The tactical layer enables the coherent implementation and steering of data-driven policies, strategies and/or initiatives. It includes data-related legislation and regulations as instruments that help countries define, drive and ensure compliance with the rules and policies guiding data management, including data openness, protection and sharing. The delivery layer allows for the day-to-day implementation (or deployment) of organisational, sectoral, national or cross-border data strategies.
The social sector can learn from the considerable advances that have been made in the health sector, including to data governance, to promote access to personal health data that can serve health-related public interests and bring significant benefits to individuals and society. In December 2016, the OECD Recommendation on Health Data Governance was adopted which identified core elements to strengthen health data governance, improve the interoperability of health data, thereby unlocking its potential while protecting individuals’ privacy (OECD, 2017[27]). The Recommendation provides policy guidance to promote the use of personal health data for health-related public policy objectives, while maintaining public trust and confidence that any risks to privacy and security are minimised and appropriately managed. It is designed to be technology neutral and robust to the evolution of health data and health data technologies.
In 2022, the OECD’s Health Committee in co‑operation with the Committee on Digital Economy Policy provided a report on how the Recommendation was being implemented (OECD, 2022[28]). The results of a survey that informed the report showed that many countries were still working toward implementation of the Recommendation. Among those countries who had lower scores for data governance, there were gaps in addressing data privacy and security protections for key health datasets such as having a data protection officer and providing staff training, access controls, managing re‑identification risks, and protecting data when they are linked and accessed. The OECD agreed it would continue to support the implementation and dissemination of the Recommendation and that a new series of country reviews of health information systems would be used to support countries in their efforts to develop health data governance.
The 2023 Health at a Glance contains a thematic chapter – Digital Health at a Glance which examines the readiness of countries to advance integrated approaches to digital health. The focus is on a non-exhaustive list of indicators of readiness to realise benefits from digital health while minimising its harms. The chapter also provides the groundwork for a more comprehensive approach to a robust suite of digital health indicators for readiness over time. While data are not currently available across all dimensions of digital health readiness (analytic, data, technology and human factor readiness) the chapter details the dimensions of a framework and signals where more regular data collection are needed (OECD, 2023[29]).
Data accuracy
Data quality is central to realising the potential of greater data use and is particularly important to initiatives aimed at improving social protection coverage, including through the use of predictive models and automated decision-making based on AI (Osoba and Welser, 2017[30]). Ideally, data need to be inclusive, timely and complete. No one data source is comprehensive. Administrative data for example suffers shortcomings in that records only cover those who are registered in government systems which may exclude, misrepresent or even overrepresent some groups. Administrative data are also often criticised for being deficit based because they are focused on the negative rather than positive aspects of a person’s life such as benefit receipt or being known to the justice system. Furthermore, the conditions and/or incentives for people to provide accurate data do not always exist.
Survey data also has limitations. While countries have developed surveys that attempt to cover traditionally marginalised and excluded groups and to collect more sensitive information, achieving better representation remains a challenge. Surveying hard to reach groups is both complex and expensive.
Access to timely data is important, particularly for operational purposes. As Employment and Social Development Canada (ESDC) found when developing the Canadian E‑vulnerability index, a key challenge is to find ways to ensure that data are both high-quality and timely (Box 5.5). Poor-quality or incomplete data may result in shortcomings in model predictions and automated decisions. For instance, research undertaken in Canada suggests that using poor-quality data (in the form of duplicate values) for predictive decision support in child protection services can create errors leading to sub-optimal foster care placement (Vogl, 2020[31]). Errors may compound when models rely on integrated data from various data sources and agencies.
Issues associated with incomplete data may have specific implications for disadvantaged and marginalised groups. For instance, certain populations might be over- or under-represented in datasets due to different experiences, statistical definitions and measurement. For example, Chile reports that undocumented migrants are underrepresented in its social registry as they typically do not have a national identification number, as are residents of very remote communities (such as islands) due to limited outreach or mobile connectivity. Similarly, OECD research shows that homelessness amongst women is typically underreported because homeless women tend to be less visible and are harder to capture in standard data collection approaches. Furthermore, those temporally sleeping in domestic violence shelters (a leading cause for women homelessness) are not statistically defined as homeless in around half of OECD countries (OECD, forthcoming[32]).
Box 5.5. The importance of data availability and timeliness – the case of Canada
The E‑vulnerability Index (EVI) uses existing survey data from Statistics Canada, including data from the Census of Population, the Canadian Internet Use Survey, and the Programme for International Assessment of Adult Competencies.
The EVI is a key data input for analysis and decision-making at ESDC, informing service design and targeted outreach activities to populations most disadvantaged by the move to digital services. Internal users show continuous demand for EVI, requesting additional data points and disaggregation. However, ongoing challenges related to data availability and timeliness make it harder to improve and update the EVI index over time.
While caveats on EVI source data staleness and on any other data limitations are added to publications to inform users of the index limitations, ESDC has also collaborated with key data partners to improve the timeliness of data source accessibility. Additionally, ESDC is exploring alternative methodologies for the EVI compilation, to leverage source data which is available on a timelier and/or disaggregated basis. The EVI will be updated during 2024.
Source: (OECD, 2023[1]; CONADI, 2023[33]).
Data privacy
The data collected and used for social protection can be highly sensitive and managing privacy is a constant challenge. Privacy risks are heightened when sensitive information is used for operational purposes such as generating automated decisions for individuals or contacting people directly (OECD, 2019[34]; OECD, 2013[35]).1 Creation and use of large datasets and data lakes also carry complex challenges that test the ability of governments and agencies to apply all relevant legal frameworks and regulations to protect individuals.
All OECD member countries, and 71% of countries around the world, have laws in place to protect (sensitive) data and privacy (OECD, 2023[36]). Perhaps the best-known instrument is the 2018 EU General Data Protection Regulation (GDPR) which has advanced data protection principles in Europe. The European Union continues to develop its regulatory framework with the European Parliament and the Council of the European Union reaching a political agreement in June 2023 to amend the European Data Act to harmonise rules on fair access to, and use of data (European Commission, 2023[37]). The United States is also strengthening its data regulatory regime with several new federal and state bills aimed at changing the way technology firms and privacy regulation works (Fazlioglu, 2023[38]).
Some countries have enshrined the right to privacy in national constitutions or bills-of-rights. In Chile and Mexico for example, privacy protection rules have been adopted from constitutions into social assistance operational manuals. The Ministry of Planning and Co‑operation in Chile must legally guarantee Solidario’s beneficiaries’ privacy and data protection. Despite these measures however, both countries experience significant enforcement gaps which weaken the effect of these regulations (Carmona, 2018[39]). There are also international human rights instruments and conventions that protect privacy such as the Universal Declaration of Human Rights while other international organisations such as the UN and OCED have created guidelines (Carmona, 2018[39]).
While critically important, multiple regulatory frameworks can cause confusion, they can be complex and difficult to navigate making it challenging for agencies to act safely and effectively. In addition to having to adhere to overarching data privacy frameworks and laws there may be other agreements, rules and responsibilities that must be observed, for example in specific legislation or government policies. Recognising this challenge, in 2021 the New Zealand Government introduced the Data Protection and Use Policy. A key aim was to help government agencies and social service providers navigate the various laws, regulations, rules, conventions and guidelines and to ensure the respectful, trusted and transparent use of people’s personal information.
Data breaches are becoming more common with governments finding themselves managing data breaches on an increasingly regular basis. In most cases, government data breaches involve personally identifying data, such as names, Social Security numbers, and birthdates, the loss of which can result in substantial consequences for victims as well as erode public trust in government’s use of data. The risk of a data breach may increase when aspects of a social protection programme are outsourced to a third party. For instance, if elements like payment delivery are managed by private firms, information flows become more complex, requiring additional data security rules related to both data sharing and processing (Carmona, 2018[39]). In the example of outsourcing data management for the Transport Agency in Sweden, there was a departure from the legislation that was supposed to govern data handling that occurred without any malicious intent (Box 5.6).
Countries are adopting a range of measures to both prevent data breaches occurring and for managing them when they do, measures such as protective security frameworks, staff training, data loss prevention tools, access controls and guidance for handling personal information security breaches. These efforts are often supported by Privacy or Information Commissioners.
Box 5.6. Risk of data regulation breach in public-private partnerships in Sweden
In 2015, the Swedish Transport Agency experienced a considerable data breach in association with outsourcing of data handling. Confidential data about military personnel, along with defence plans and witness protection details, were exposed. Fortunately, there is no evidence that information was leaked to third parties because of the security breach.
The Swedish Transport Agency had contracted private firm IBM to run its IT systems. The contract included outsourcing maintenance and functioning of hardware, networks and programmes. However, in the process of outsourcing data handling, the director general of the Transport Agency was able to abstain from closely following standard regulations under the National Security Act, the Personal Data Act and the Publicity and Privacy Act.
Investigations by the Swedish Security Service and the Transport agency found that IBM staff without the necessary security clearances had been able to access confidential information. While the data were found to have been exposed to non-cleared staff, there was no evidence that IBM had mishandled the information.
Overreach and lack of legal basis
Linking data across agencies and providers, which is becoming increasingly common, raises complex issues regarding informed consent. It can be difficult to predict when someone’s information is collected for a particular administrative purpose whether it will be linked with data from other sources and used for other purposes such as research, data analytics, or even enrolment in other programmes. This makes informed consent difficult (Lokshin and Umapathi, 2022[18]).
Data integration can introduce the potential for overreach, i.e. a deviation from the intention under which the data were originally collected (Levy, Chasalow and Riley, 2021[42]) and there are examples of integrated datasets created for one purpose being used for another. For example, the Florida Department of Child and Family collected multidimensional data on students’ education, health, and home environment. However, these data were subsequently interfaced with Sheriff’s Office records to identify and maintain a database of juveniles who were at risk of becoming prolific offenders.
Historically some social protection agencies have failed to fully consider the legal and ethical implications of automating a process or system. The United Nations Special Rapporteur for Extreme Poverty and Human Rights notes several cases where automated systems were implemented without paying sufficient attention to the underlying legal basis. For instance, in February 2020 the District Court of the Hague ordered an immediate halt to the Netherland’s System Risk Indication system because it violated human rights norms. In June 2020, the Court of Appeal ordered the United Kingdom’s DWP to fix a design flaw in the Universal Credit which was causing irrational fluctuations and reductions in how much benefit some people received (Special Rapporteur on extreme poverty and human rights, 2019[43]).
5.2.3. Mitigating the risks of further entrenching discrimination, bias and exclusion
Discrimination, stigmatisation and exclusion can result from use of models and automated systems
There is a risk that discrimination, stigmatisation and exclusion can result from the use of predictive models, automated decision-support tools, and other targeting mechanisms. Several factors can cause discriminatory outcomes including algorithmic bias (i.e. systematic and replicable errors in computer systems for example where algorithms have been trained on datasets reflecting existing prejudices), unevidenced variable selection or poorly constructed criteria, an algorithm being used in a situation for which it was not intended, and/or the use of poor including biased data.
Evaluations of algorithmic decisions have found they can be discriminatory even when variables by which discrimination can be measured, such as gender, ethnicity or age, are themselves not included. As Osoba and Walker (2017, p. 17[30]) state, “applying a procedurally-correct algorithm to biased data is a good way to teach artificial agents to imitate whatever bias the data contains”. Data may be biased for different reasons. First, certain population groups could be over- or under-represented. Second, an algorithm may mirror decisions taken by biased individuals and third, algorithmic decision support can create self-reinforcing feedback loops. When attention is focused on a certain population group(s) more data are gathered about them that may then provide evidence that even more attention should be focused on that same group (O’Neil, 2016[44]).
Examples of discriminatory outcomes resulting from the use of algorithms suggest that disadvantaged groups are more likely to be exposed to these outcomes than others. For example, in Austria an algorithm was used to allocate job applicants into two groups: one receiving a higher degree of job search support and one receiving less. However, it discounted the chances of employment among groups with certain characteristics who already tended to face disadvantages in the labour market in a way that disproportionately allocated them to the group receiving less support (Box 5.7).
The algorithm used to predict the risk of fraud among recipients of France’s Family Allowances Fund (CNAF) has been criticised for several reasons. One criticism is that the algorithm targets people in precarious positions because their status is associated with risk factors that are correlated with precariousness. For example, higher risk scores are allocated to individuals who must file complex income declarations (for APL, activity bonus, disabled adult allowance, etc.) which has allegedly meant that those on minimum-income benefits are disproportionately likely to be controlled for (Benoît Collombat, 2022[45]).
Similarly, the automated means-testing algorithm that underpins the Universal Credit programme in the United Kingdom has been criticised for miscalculating some individuals’ entitlements, causing benefit entitlements to fluctuate significantly. Monthly earnings are a key input variable and for those whose earnings are irregular, such as contractors and other workers in insecure jobs the algorithm can perform poorly (Human Rights Watch, 2020[46]). This is particularly problematic because it disproportionately affects those who are already in precarious situations who earn income from several and/or insecure jobs.
New research in the United States shows that Black American taxpayers are three to five times more likely to be audited on their tax returns, compared to other taxpayers (Hadi Elzayn et al., 2023[47]). Although the tax collection agency does not collect information on race, the algorithms used to select tax units for auditing have created a racial bias. People filing for the Earned Income Tax Credit are more likely to be selected for audits. The IRS has identified this problem in the algorithm and is making changes to how people are selected for audit.
Box 5.7. Risk of predictive models leading to misleading results in Austria
The Austrian Government used an algorithm to predict jobseekers’ employment prospects with the aim of tailoring employment support interventions for individuals. Services that actively help jobseekers into jobs, such as job search assistance and job placements, are prioritised for those who are predicted to have moderate employment prospects. Those who are predicted to have low employment prospects are allocated to crisis support measures.
However, studies show that the algorithm discounts the employment prospects of women over 30, women with care responsibilities, migrants, and persons with disability. Systematically misclassifying these groups of people risks limiting them to crisis support rather than active employment support thereby reducing their chances of entering employment. This is not only discriminatory, but also weakens the chances of groups who tend to already face disadvantages in the labour market.
Errors and biases in models and automated systems can be hard to detect. Explaining algorithmic models is complex and, in some cases, impossible because they are both inscrutable and nonintuitive (Selbst and Barocas, 2018[50]). This can result in errors going undetected until many people are affected (Redden, Brand and Terzieva, 2020[14]). Australia’s Robodebt Scheme, introduced in 2015 to assess entitlements to payments highlights the challenges of detecting a systemic issue in an automated system. While individual members of the Administrative Appeals Tribunal (the body responsible for conducting merit reviews of administrative decisions under Australian federal government laws) noted problems with overpayment calculations, the systemic nature of the problem was not identified immediately, and the scheme continued to operate until 2019 (see Box 5.8).
Box 5.8. Robodebt in Australia
From 2015 to 2019 the Department of Human Services implemented a debt recovery scheme – Robodebt – to recover overpayments to welfare recipients dating back to 2010‑11. To calculate the overpayments, social security payment data was matched with annual income data from the Australian Taxation Office and a process known as “income averaging” was used to assess income and benefit entitlement. Debt notices would then be issued to affected welfare recipients who would have to prove they did not owe a debt, which was often many years old.
The process both produced inaccurate results and did not comply with the income calculation provisions of the Social Security Act 1991. Despite adverse findings by the Administrative Appeals Tribunal to some cases, the systemic nature of the problem was not immediately identified, and the scheme continued to operate. By the end of 2016, the scheme was the subject of heavy public criticism, but it continued until November 2019, when it was announced that debts would no longer be raised solely based on averaged income. That was followed in 2020 by the settlement of a class action and an apology, in June 2020, from the then prime minister, the Hon Scott Morrison. A 2022 Royal Commission into the Robodebt Scheme made 57 recommendations as the result of its inquiry; the recommendation relating to automated decision-making is discussed below.
Errors and biases can make someone appear ineligible for a benefit they are legally entitled to – a false negative (OECD, 2019[34]). A false negative is an outcome where the model incorrectly predicts the negative class resulting in some individuals receiving less “treatment” or services than they need which may result in poorer outcomes for some priority groups. A false positive is an outcome where the model incorrectly predicts the positive class, and some individuals may receive more “treatment” or services than they need which can result in an inefficient allocation of resources. Both have implications although research suggests people are more concerned with avoiding false negatives.
Exclusion
Increased reliance on digitalised services risks excluding people without digital access. Further, these people are likely to be the same people who already suffer poorer access to social services. Globally, more than 84% of national governments now offer at least one online service (ISSA, 2022[52]). Despite the increased opportunities digital services present, the access to, and use of digital infrastructure and tools, is uneven. An estimated 2.9 billion people do not use the Internet. The digital divide is even starker when viewed from the lens of age, gender, poverty and location (ISSA, 2022[52]). Across the OECD, 22% of 55‑74 year‑olds state that they do not use the internet, in Türkiye and Mexico it is more than 50% (Figure 5.1).
The risk of exclusion extends to linked datasets that governments increasingly use to determine eligibility for services and benefits. For example, the Canadian benefit system faces problems regarding its ability to include Indigenous populations in their linked data bases that provide the foundation for benefit eligibility (Box 5.9).
Figure 5.1. One‑fifth of 55‑74 year‑olds across the OECD do not use the Internet
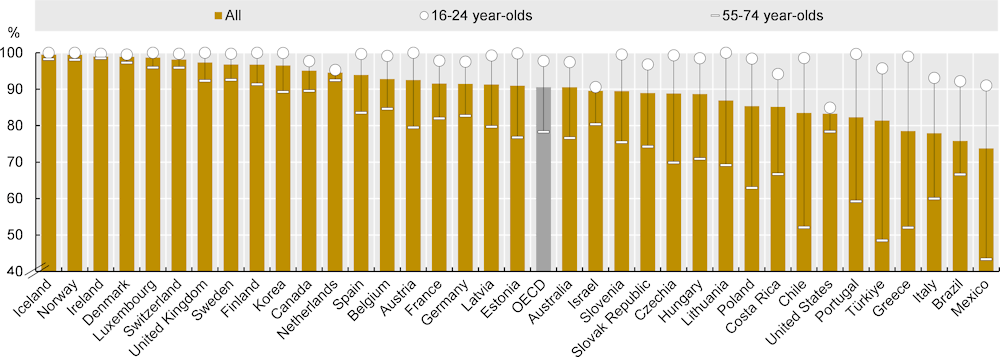
Source: OECD (2022), ICT Access and Usage by Households and Individuals Database, http://oe.cd/hhind (accessed in January 2022).
The literature is very clear, while the potential positive impacts of the digital transformation are substantial, without deliberate efforts to correct digital inequities, it may compound existing vulnerabilities. Access to the internet and relevant devices, such as a mobile phone, will be critically important to how people benefit from new services (OECD, 2022[17]) and people will also need the necessary skills and capacities to use relevant technologies and devices to make use of services (ISSA, 2022[52]).
Many countries are already actively working to address this challenge with governments including in their digital strategies explicit provisions to promote digital inclusion for those more likely to miss out. Other approaches include engaging directly with people and providing training, intermediation or subsidies for devices. In the United States for example, eligible households can access the Affordable Connectivity Program which helps ensure households can afford the broadband they need for work, school and healthcare. The benefit provides a discount of up to USD 30 per month toward internet service for eligible households and up to USD 75 per month for households on qualifying Tribal lands. There is also a one‑time discount of up to USD 100 to purchase a device. However, due to funding constraints, this programme will no longer be accepting new applications after February 2024.
Governments are also taking indirect approaches, working on language and communication, improvements to the user experience and creating intuitive user interfaces (ISSA, 2022[52]). For instance, the German Social Insurance for Agriculture, Forestry, and Horticulture used a website as a forum to disseminate information about the rights of marginalised migrant seasonal workers in the languages most frequently spoken and understood (Box 5.9).
Box 5.9. Including everyone in digitalised solutions
Canada: Indigenous populations face difficulties accessing key social benefits
In Canada, several important social benefits at the federal level (e.g., Canada Child Benefit, Canada Workers Benefit) and at the provincial level are delivered through or linked to the tax system. To be eligible, individuals must therefore complete a tax return. In 2021, Statistics Canada reported just over 28.1 million tax filers, or roughly 87% of the population aged 15 years and over. This suggests about 13% of Canadians are not filing a tax return and are potentially not receiving benefits from key social programmes.
Rates of non-tax filing are particularly elevated among Indigenous populations. There are several reasons for this, including that Indigenous populations in Canada may be concerned that the disclosure of personal and financial information to the government might ultimately cause them harm. For instance, a report by Prosper Canada found that Indigenous peoples fear that applying for and receiving benefits may lead to “scrutiny by social services and potential removal of children” or that “additional one‑time income may jeopardise needed housing or childcare subsidies.” Heightened distrust in government may likely stem from historical experiences of discrimination.
Indigenous people are also more likely to lack personal identification, such as a birth certificate, which is required to obtain a social insurance number, itself a requirement for filing taxes and accessing many social benefits.
Geographical remoteness is another key barrier to completing tax returns, especially for Indigenous populations. There are many reasons why remoteness presents a barrier, including the lack of access to Internet for online tax filing software and virtual support. Indeed, only 43% of First Nations reserve areas and 49% of the North had 50/10 unlimited broadband coverage in 2021. This compares to about 91% of all Canadian households.
Germany: Communicating information about workers’ rights to non-native speakers
The German Social Insurance for Agriculture, Forestry, and Horticulture aimed to promote safety at work and protect the health of workers. However, they realised that there might be knowledge gaps about the issues of occupational health and safety among seasonal workers. Addressing this, they developed a web platform in 2021.
To ensure that seasonal workers, many of whom are from Central and Eastern Europe, can access information, the platform was made available in ten languages. It prioritises clarity of information and contains a mix of text, images, and videos to ensure that information is easily digestible.
The platform also contains a section with an updated list of real-world questions asked by workers, and points users to the telephone and email for other queries.
Mitigating the risks of generating discrimination, stigmatisation and exclusion
The increasing digitalisation of public services means issues associated with implementing automated systems including the use of algorithms will continue to arise and governments need to have in place appropriate accountability frameworks and procedures. Without them, technology and data-driven innovations risk disempowering and disengaging people and eroding public trust and confidence as discussed earlier. Principle 1.5 of the OECD’s AI principles (discussed in Chapter 4), which arguably can be usefully applied beyond AI technologies specifies that AI actors should be accountable for the proper functioning of AI systems, based on their roles, the context, and consistent with the state of art (OECD, 2019[61]).
In the Netherlands nearly 26 000 families were falsely accused of fraud by the Dutch tax authorities between 2005 and 2019 due to discriminative algorithms. Risk profiles were created for individuals applying for childcare benefits in which “foreign sounding names” and “dual nationality” were used as indicators of potential fraud. As a result, thousands of (racialised) low- and middle‑income families were subjected to scrutiny, falsely accused of fraud, and asked to pay back benefits they had obtained legally, which in many cases amounted to tens of thousands of euros. The consequences were devastating. Families went into debt, many ended up in poverty with some losing their homes and/or jobs. More than 1 000 children were placed in state custody as a result (The European Parliament: parliamentary question, 2022[62]). The Dutch Government’s lack of action and accountability even after it was clear something was wrong led to the eventual resignation of the government in 2021.
Incidents such as the Dutch childcare benefit scandal as well as the Robodebt Scheme in Australia offer important lessons for how the potentially negative impacts of automated systems and algorithms can be mitigated. According to Assistant Professor Błażej Kuźniacki, lack of transparency was one of the causes of the Dutch scandal. Dutch legislation did not allow AI automated decision-making to be checked and there was not enough human interaction; further, procedures were too automatised and secretive. AI was allegedly able to use information that had no legal importance in decision making, such as sex, religion, ethnicity, and address which can lead to discriminatory treatment. If tax authorities are not able to explain their decisions, they cannot justify them effectively. The higher the risks, the higher the explainability requirements should be (Błażej Kuźniacki, 2023[63]).
Two of the Australian Royal Commission into the Robodebt Scheme’s 57 recommendations specifically addressed automated decision-making:
Recommendation 17.1: Reform of legislation and implementation of regulation
The Commonwealth should consider legislative reform to introduce a consistent legal framework in which automation in government services can operate.
Where automated decision-making is implemented:
there should be a clear path for those affected by decisions to seek review,
departmental websites should contain information advising that automated decision-making is used and explaining in plain language how the process works,
business rules and algorithms should be made available, to enable independent expert scrutiny.
Recommendation 17.2: Establishment of a body to monitor and audit automated decision-making
The Commonwealth should consider establishing a body, or expanding an existing body, with the power to monitor and audit automate decision-making processes regarding their technical aspects and their impact in respect of fairness, the avoiding of bias, and client usability.
The Australian Government accepted, or accepted in principle, all recommendations made by the Royal Commission into the Robodebt Scheme, including recommendations 17.1 and 17.2.
The Australian Government accepted recommendation 17.1, and committed to consider opportunities for legislative reform to introduce a consistent legal framework in which automation in government services can operate ethically, without bias and with appropriate safeguards, which will include consideration of:
review pathways for those affected by decisions, and
transparency about the use of automated decision-making, and how such decision-making processes operate, for persons affected by decisions and to enable independent scrutiny.
The Australian Government accepted recommendation 17.2 and agreed to consider establishing a body, or expanding the functions of an existing body, with the power to monitor and audit ADM processes.
Both cases highlight the critical importance of transparency and explainability and the need for meaningful human involvement, particularly when automated decisions can, potentially and significantly impact people’s lives. Transparency involves disclosing when automated systems are being used e.g., to make a prediction, recommendation or decision, with disclosure being proportionate to the importance of the interaction. Transparency also includes being able to provide information about how an automated system was developed and deployed, what information was used and how, how an output was arrived at, who is responsible for that output and how it can be appealed. An additional aspect of transparency is facilitating, as necessary, public, multi-stakeholder engagement to foster general awareness and understanding of automated systems and to increase acceptance and trust (OECD, 2019[64]).
Explainability is the idea that an automated system or algorithm and its output can be explained in a way that “makes sense” to people at an acceptable level enabling those who have been adversely affected by an output to understand and challenge it. This includes providing – in clear and simple terms, and as appropriate in the context – the main factors included in a decision, the determinant factors, and the data, logic or algorithm used to reach a decision (OECD, 2019[64]). Some algorithms are more readily explainable but potentially less accurate (and vice versa) and so while requiring explainability may negatively affect the performance of an algorithm, it may in some cases be an outweighing factor.
There should always be a degree of human involvement in automated decision-making, proportionate to the potential impact of the outputs generated. Principle 1.2(b) of the OECD’s AI principles specifies that AI actors should implement mechanisms and safeguards, such as capacity for human determination, that are appropriate to the context and consistent with the state of art (OECD, 2019[61]).
Article 22 of the GDPR stipulates that organisations deploying automated decision-making under permissible uses must “implement suitable measures to safeguard the data subject’s rights and freedoms and legitimate interests.” The latter shall include, at least, the rights “to obtain human intervention on the part of the controller, to express his or her point of view and to contest the decision” (Sebastião Barros Vale and Gabriela Zanfir-Fortuna, 2022[65]). While inserting humans into the loop of automated systems is a crucial way of helping to achieve accountability and oversight, this doesn’t come without challenges. For example, what level of oversight, accountability and liability are attached to human-made decisions? What qualifications and/or expertise is required to question an automated decision?
European Data Protection Board guidelines on automated individual decision-making and profiling state that a controller cannot avoid Article 22 provisions by fabricating human involvement. For example, if someone routinely applies automatically generated profiles to individuals without any actual influence on the result, this would still be a decision based solely on automated processing. To qualify as human involvement, the controller must ensure that any oversight of the decision is meaningful, rather than just a token gesture. It should be carried out by someone who has the authority and competence to change the decision. The controller should identify and record the degree of any human involvement in the decision-making process and at what stage this takes place (European Data Protection Board, 2017[66]).
The right to review an automated decision or output is an important feature of an accountability framework. Those negatively impacted by automated decision-making should be able to appeal a decision and know how to do that. As the OECD’s 2019 Recommendation on AI specifies, those that are adversely affected by an AI system should be able to challenge the outcome(s) of the system based on easy-to‑understand information about the factors that served as the basis for the prediction, recommendation or decision (OECD, 2019[61]). Grievances and investigations should be taken seriously and made publicly available together with the outcome(s) so that lessons can be learned and shared with others undertaking similar work.
Some groups may not know they have been overlooked or have the resources to address any issues (Lokshin and Umapathi, 2022[18]; Barca and Chirchir, 2019[23]). Complaint processes should account for this with public agencies ensuring that marginalised and excluded populations are supported in making any application for a review of a decision. Staff who engage with social security applicants need to be able to explain how a decision was reached and provide information about how that decision can be reviewed. This requires staff to be adequately trained and for there to be sufficient complaint processes to be place. Furthermore, public agencies should consider developing algorithms in-house using internal experts and/or understand and be able to explain algorithms developed by external partners (OECD, 2019[34]).
It may also be necessary for lawyers, judges or other arbitrators to receive training on the functioning and fallibility of algorithms (Citron, 2007[67]; Gilman, 2020[68]). This will help those individuals who have been exposed to negative outcomes from issues such as data breaches, unjustified automated decisions, or other negative outcomes to question decision-makers’ actions and take legal action if necessary.
5.3. Embracing the challenges – a way forward
Governments have put considerable effort into measures such as legal, regulatory and accountability frameworks, data governance and management, and strategies and policies to promote the respectful use of people’s personal information and to protect their privacy. International organisations are supporting government efforts, for example by developing legal instruments such as the forementioned OECD Recommendation on Digital Government Strategies and Recommendation on Health Data Governance and international sharing of good practices such as the ISSA’s Webinar Series on AI. The European Law Institute has designed a set of Model Rules on Impact Assessment of Algorithmic Decision-Making Systems to supplement European legislation on AI in the specific context of public administration (European Law Institute, 2022[69]).
These measures are well covered in the literature, and they are continually being improved. This section explores approaches governments are taking to balance optimising the benefits of rapid developments in technology and data to improve public services with the challenges of doing so that go further than specific legal and technical solutions. Approaches or strategies that improve governments’ overall interactions with people and communities, enhance public trust and confidence and modernise government operations that countries can learn from as they undertake their own digital transformations.
5.3.1. Service offerings through multiple channels.
A key solution to addressing the challenge of reinforcing or creating new sources of exclusion and disadvantage through increased use of digitalised solutions is to provide alternative service delivery channels, combining digital offers with call centre and in-person options. Multiple service channels are particularly important for people with high and complex needs for whom online services are often not appropriate, people living in remote locations and/or people who are unable to or choose not to access digital solutions (Box 5.10). Furthermore, those outside regular customer groupings are far less likely to access online services (ISSA and United Nations University, 2022[70]).
Many governments offer human touchpoints alongside digital options. For instance, when a city in Sweden implemented automated decision-making in unemployment assistance, caseworkers remained in close contact with benefit applicants. While decision-making was automated, based on certain rules, caseworkers helped applicants with their applications, including explaining the process and helping to file appeals if needed (Ranerup and Henriksen, 2020[16]).
A 2022 ISSA and UNU-EGOV survey of social security organisations showed that most who participated in the survey utilise a mixed set of service delivery channels. Of the responding institutions, 91% have websites and offer online services, while 86% utilise paper forms and physical service centres. This is also reflected in 64% of institutions still accepting letters and application forms via post. Surprisingly, only 58% of institutions have call centres, the use of which has proven highly effective and efficient as a service channel both before and during the pandemic. Almost half of the institutions, or 48%, use various forms of SMS/text messaging in their communication with customers. A small number of institutions (46%) have solutions based on mobile applications (or “apps”) which often incorporate notifications.
Least common, at 17%, are stand-alone kiosks, but this is likely to be due to other technology-based solutions being less costly and more flexible. In short, online service offers exist but the customers’ utilisation of these electronic services (e‑services) is still mixed, and for various reasons are still limited amongst institutions that deliver and manage social security services globally (ISSA and United Nations University, 2022[70]).
Importantly, the survey results showed that in-person services can play an important role in helping people who wish to, to transition to digital services. For instance, floor walkers at physical service centres have long been applied by both the public and private sector. Through observations, floor walkers help identify individuals who are using digital devices and thus have the potential digital skills, advise them on online service offers or assist customers on standalone kiosks or computers.
One of the recommendations resulting from the ISSA and UNU-EGOV survey was to promote digital inclusion, gender inclusion and digital empowerment through dedicated initiatives including training both service providers and call centre staff to act as floor walkers and promoters of digital service offers and digital skills development initiatives. Other initiatives include actively monitoring customers and proactively informing them of self-service terminals; make digital skills training available; develop short instruction videos and clickable demos of key services with targeted messages to marginalised custom groups; and, provide material directly or through partnerships with libraries and community centres or stakeholders representing the customers group in questions (ISSA and United Nations University, 2022[70]).
Box 5.10. Ensuring access for everyone through multi-channel service offerings
Canada: Using human support staff to ease possible concerns among benefit applicants
When Service Nova Scotia took over the administration of the Property Tax Rebate for Seniors programme (PTRS) in 2018 they realised that all those who receive PTRS also met the requirements for the Heating Assistance Rebate Program (HARP).
Due to information and privacy protocols, the department was unable to automatically provide the HARP rebate to PTRS recipients. Instead, an opt-in feature was developed to confirm applicant consent.
To abate possible concerns about checking the opt-in feature for HARP, the programme developed a holistic plan for engagement with easily digestible information and human assistants. The programme worked with communications staff to ensure that the application form had concise and easy-to-read messages about opting in for HARP. The customer support staff at the department were also provided with messaging about how the opt-in feature works.
Indiana, the United States: Misguided automation can inadvertently lead to declining enrolment rates
The state of Indiana wanted to lower the administrative costs and increase convenience for clients and operators. Therefore, they contracted IBM in 2006 to automate caseworker assistance for the state’s welfare services. The roll-out of the new system started in 2007. However, it was terminated two years later due to performance issues.
An evaluation found that the automated system created additional burdens related to the application and recertification, leading to sharp declines in key benefit enrolment rates. Key reasons behind enrolment declines were found to be a lack of personalised human assistance from caseworkers, overburdened call centres resulting in significant delays and technical issues, as well as a lower tolerance for application and recertifications errors.
United Kingdom: Offering in-person support to those who cannot claim online
Most recipients of the United Kingdom’s Universal Credit access the application process online, but there are cases where the online process is not sufficient. The Department for Work and Pensions (DWP) has found that 98% of households who make a claim for Universal Credit do so online. However, there is a small number of people with complex needs or without access to the internet who are not able to use the online process. In response the DWP provides a range of support to make the service more accessible.
Help to Claim support is delivered independently by Citizens Advice, in partnership with Citizens Advice Scotland, with support provided through telephone and digital channels. Those individuals who are unable to access support via these channels can go to their local jobcentre, local libraries, or local advice centres where they can use computers with internet access free of charge. Jobcentres remain open to provide access to services for claimants who need face‑to-face support. There is also a telephone number displayed outside each Jobcentre with details of how to contact DWP. In addition, DWP has contracted Interpreter and Translation Services which can be arranged for claimants where English is not their first language, or who are deaf, hard of hearing or speech impaired.
Source: (OECD, 2023[1]; Wu and Meyer, 2023[71]).
5.3.2. Involving service users in solution design
Taking a user-centric approach to communication, channel and service design can help to address the barriers to accessing and using digital services. An increasingly popular way of ensuring positive user experiences is to adopt user-centred design methods when developing and implementing digital solutions (OECD, 2009[72]; OECD, 2022[8]). Service user involvement can range from providing feedback on existing initiatives to co-design and co-creation of new ones. It can also involve piloting, testing, and scaling services with continuous feedback and improvement mechanisms (ISSA, 2023[73]). It has been shown that user-centred approaches can help to ensure services meet the needs of a wider range of users (World Bank, 2022[74]). Indeed, government agencies and social security institutions globally have started making this transition. It is estimated that 60% of government agencies worldwide have integrated user-centred design methodologies by 2023 (Gartner, 2022[75]).
By way of example, Ireland is moving to systematic involvement of service users in the creation of digital and other solutions to help combat non-take‑up of benefits and services. They start with customer research questions such as: What is the customer understanding and experience of a current service? Is use of terminology challenging? How does the flow and functionality of the service work for them? They also ask business research questions: What are the common issues customers contact us about? What would improve the process? Are there untapped opportunities? Prototype solutions are tested with customers. This approach is leading to balancing customer and business requirements, meeting accessibility objectives, easier to use services, greater take up of online services, and enabling space to support customers that are unable to access online services (ISSA, 2023[76]).
Employment and Social Development Canada has developed and implemented an innovative Service Transformation Plan (STP) designed to employ a client-centric outside‑in approach, with clients at the centre of everything. Included in the STP is the Client Centric Policy Playbook which recognises that clients deserve programmes and services that provide the best experience for them, when and where they need it. The Playbook strengthens the ability to engage clients in the design of programme and service policies. Through extensive engagement with policy experts and employees on-the‑ground, the Playbook has brought together innovative best practices, tools and resources for engaging clients. This solution enhances client experience by giving clients an opportunity to be part of the policy generation process and by ensuring that programmes and services are reflective of their needs (OPSI, 2019[77]).
New technologies can also help facilitate feedback on existing services and customer experience. A review by the World Bank found that new technology can facilitate service user feedback as a technical contribution to the design of policy and service provision. In a best-case scenario, such technological solutions to feedback mechanisms would also support the inclusion of communities who have traditionally been excluded or marginalised in the social dialogue (World Bank, 2016[78]). Many countries have adopted client experience measurement surveys to gather and analyse client feedback to improve service delivery. Such surveys can provide accurate and reliable data on drivers of customer satisfaction, where service improvements can be made, and information on client groups facing barriers that can lead to more in-depth investigation.
5.3.3. Achieving incremental improvements through agile working methods
New working methods, which will also require new capacities and skill mixes, are necessary if government organisations are going to meet people’s changing needs. The risk of technology solutions failing can be mitigated through more agile ways of working, for example, re‑use of existing assets, later re‑use of products developed, developing solutions within existing enterprise architecture, and testing and prototyping solutions throughout the development process. While it may not be the way governments have worked historically, agile methodologies that employ a people and results-focused approach to technology development can be flexible and fast and deliver continuous improvements at potentially lower costs and avoid larger and more expensive problems later.
It is important to start with a comprehensive assessment of the current state and future needs, before focusing on developing simple and well-designed systems that address those needs, testing as you go. More advanced features can be added later, supported by continuous evaluation and ongoing improvements (Box 5.11) (Barca and Chirchir, 2019[23]). Sufficient funding is critical; the resources required to pilot and scale and for ongoing maintenance need to be identified and realistically costed.
Continuous testing can contribute to the initiatives with the most potential being selected, increasing the chances of successful implementation. In France for instance, the National Family Allowances Fund’s digital inclusion programme invests in two special test sites that experiment with and evaluate digital inclusion programmes, based on a structured evaluation protocol. Only the most promising initiatives are presented to a steering committee for approval and finally integrated into a core curriculum (ISSA, 2022[52]).
Furthermore, as data volumes grow, machine models need to be continuously trained and evaluated for robust performance. This implies having in place a strong performance framework to evaluate model performance using a consistent set of metrics. Metrics typically include accuracy (the proportion of the total number of predictions that were correct), precision (the proportion out of all positive predictions was correct) and recall (the proportion of correct positive predictions out of all positives a model could have made).
Services Australia, the agency responsible for delivering social services and means-tested social security payments in Australia has leveraged data analytics to reliably assess claims through Straight Through Processing (STP). With the onset of COVID‑19 Services Australia extended its automated decision-making capabilities to expedite payments to the unprecedented volume of claimants for the Jobseeker programme. The aim was to provide payments to people in need as fast as possible and to assure the government that automated payments, while socially responsible and administratively efficient.
While Services Australia had implemented STP for other categories of payments in the past, the crucial difference was the scale and speed at which it was implemented for Jobseeker claims. It was therefore important to measure and demonstrate the administrative efficiency of automating the claims process while providing assurance on the integrity of the payment outcome. While there was an existing business framework to guide the development of automation products, the data-driven assurance process which conducts checks on a statistically valid sample of automated payment decisions was key to demonstrating reliability and accuracy. A payment accuracy benchmark of >95% for automated payment decisions and data-driven analysis were used to measure achievements against this target. An accuracy target of 99% has been achieved (ISSA, 2022[15]).
Box 5.11. Iterative development of an integrated one‑stop shop in Spain
The General Treasury of Social Security (TGSS) in Spain used agile working methods with iterative development cycles to implement a new portal called Importass. The portal offers a digital one‑stop shop for administrative and tax-related tasks such as managing employment, freelancing, or the hiring of labour for domestic tasks. It is accessible via mobile devices, on the web, the Electronic Office and the Social Security app.
The development of Importass was inspired by agile work methods, using self-organised and cross-functional teams that worked with iterative versions. Evaluations were conducted throughout the development process, using quantitative analysis, service design, user experience research, and process analysis. The teams adopted a user-centred approach, analysing user profiles, conducting focus group discussions, and employee and citizen interviews. Based on this, processes were re‑engineered continuously.
The new portal has become widely used since its introduction, with:
2.6 million users, of which 73% are new users
On average taking six minutes to register
Two million working life report applications received
54.7% of users accessing the portal from their mobile phone.
The rapid transition to fully digital services during the COVID‑19 pandemic highlighted the challenges of scaling at speed solutions that have not necessarily been sufficiently tested. In a 2020 international survey, following the outbreak of the pandemic,2 while around half of respondents reported that online services met all or most of their needs, 7 in 10 reported experiencing problems during their most recent digital interaction with government (Mailes, Carrasco and Arcuri, 2021[10]). Respondents cited length of time required, the inability to use fully online services, and difficulties switching between channels as common sources of frustration (Mailes, Carrasco and Arcuri, 2021[10]).
COVID‑19 has accelerated the pace of technological change as well as cement existing divides. Measures are being put into place to ensure there is equal access to, and use of digital infrastructure and tools. By way of example, social distancing has transformed the way we connect and innovate at work. To help employers, recruiters and educators ensure that Europeans are equipped with digital skills in the post-pandemic context, the European Commission launched (European Commission, 2020[79]) new digital competence guidelines (Centeno, 2020[80]) that include practical steps, key actions, tips and online resources for digital users. These help people make best use of their digital competences from the perspective of the “employability path” – from education to sustainable employment, and entrepreneurship (European Commission, 2020[81]).
5.3.4. Encouraging an innovative technology and data culture through leadership and champions
Informed and supportive leaders and champions can help to drive reform agendas and to promote an innovation culture (OECD, 2014[6]). Given the many challenges and costs involved in undertaking digital and/or data-driven projects, it is necessary that both leaders and staff understand the challenges as well as the potential benefits. Employment and Social Development Canada for example trained a network of change ambassadors and deployed them throughout the country. The ambassadors are employees who can explain the process of change, agile ways of working and service transformation objectives to their colleagues. They are also responsible for presenting ideas to working groups and for providing employee feedback (ISSA, 2023[73]).
Individual people and positions at various levels can play important leadership roles. First 5 South Carolina, discussed in more detail in Chapter 5 is an initiative that connects families with young children in South Carolina with public services. Having a dedicated project manager, as well as a variety of champions, has enabled challenges such as ensuring adequate time is allocated from all partners to address project needs has enabled the initiative to move forward at several points to avoid delays and to ensure the correct information is being collected. The Estonian Proactive Service Provision for Disabled People project (Box 5.12) exhibits the importance of the use of novel service design methods, openness towards change and having a strong project manager.
Some countries are strengthening data and technology leadership capability through enabling innovative and cross-cutting data governance and policy approaches often led by data champions and/or data officers, positioned at senior levels. Estonia’s data governance journey for example has gone through three distinct stages. In the early 2000s, the focus was on developing systems and digitizing paper-based documents. Up to the mid‑2010s, data were managed primarily for service delivery, however in the late 2010s a paradigm shift occurred as the understanding of data’s inherent value grew, leading to managing data as a valuable asset.
According to the Estonian Government’s Chief Data Officer, Ott Velsberg, the next phase of the journey involves leveraging AI-powered data within both the government and private sector to transform various domains such as education, research and development, and the legal system. Effective data governance is possible if approached strategically. It requires several pieces that make up the whole – management involvement and support, a clear understanding of the benefits and goals, competent people with the right tools and guidelines, and continuous monitoring and reiteration for improvement (Ott Velsberg et al., 2023[83]).
Box 5.12. Leadership in government as a success factor in Estonia
The “Proactive service provision for disabled people” project was implemented by the Social Insurance Board in 2020. The aim of the project is to automate the disability application process to simplify the current system for users and reduce personnel resource costs for the Social Insurance Board (e.g. the application review process is very time‑consuming for the physicians involved).
Certain key factors have contributed to the success of the project. Firstly, the role of the government Office, who has been leading the innovation programme enables different government actors to experiment with a novel service design framework. This has enabled actors a framework within which they can approach the collaboration process, which was very smooth considering the various challenges (e.g. limited resources and time, incompatibilities with the legal framework, etc.) participants faced. Individual actors were motivated to contribute additional resources to ensure a positive result from the collaborative arrangement. Another key success factor was the role of the project manager. She was not a member of the Social Insurance Board which meant she was uninhibited by established organisational legacies. As a result, she brought a collaborative mindset to the project, which proved crucial in bringing about change, as she held an alternative perspective, which allowed for the rethinking of established work routines.
References
[48] Allhutter, D. et al. (2020), “Algorithmic Profiling of Job Seekers in Austria: How Austerity Politics Are Made Effective”, Frontiers in Big Data, https://doi.org/10.3389/fdata.2020.00005.
[23] Barca, V. and R. Chirchir (2019), Building an integrated and digital social protection information system, Deutsche Gesellschaft für Internationale Zusammenarbeit (GIZ), https://www.giz.de/en/downloads/giz2019-en-integrated-digital-social-protection-information-system.pdf.
[40] BBC (2017), Sweden data leak ’a disaster’, says PM, BBC, https://www.bbc.com/news/technology-40705473.
[45] Benoît Collombat (2022), Investigation into the algorithm that scores CAF beneficiaries, France Bleu, https://www.francebleu.fr/infos/economie-social/enquete-sur-l-algorithme-qui-note-les-allocataires-de-la-caf-5560273 (accessed on 3 January 2024).
[63] Błażej Kuźniacki (2023), The Dutch childcare benefit scandal shows that we need explainable AI rules, University of Amsterdam, https://www.uva.nl/en/shared-content/faculteiten/en/faculteit-der-rechtsgeleerdheid/news/2023/02/childcare-benefit-scandal-transparency.html?cb (accessed on November 2023).
[13] Burtscher, M., S. Piano and B. Welby (2024), “Developing skills for digital government: A review of good practices across OECD governments”, OECD Social, Employment and Migration Working Papers, No. 303, OECD Publishing, Paris, https://doi.org/10.1787/f4dab2e9-en.
[60] Canada, S. (2023), Summary characteristics of Canadian tax filers (preliminary T1 Family File), https://doi.org/10.25318/1110004701-eng.
[59] Canada, S. (2022), Population estimates on July 1st, by age and sex, https://doi.org/10.25318/1710000501-eng.
[39] Carmona, M. (2018), Is biometric technology in social protection programmes illegal or arbitrary? An analysis of privacy and data protection, ILO, https://www.ilo.org/wcmsp5/groups/public/---ed_protect/---soc_sec/documents/publication/wcms_631504.pdf.
[67] Citron, D. (2007), “Technological due process”, Washington University Law Review, Vol. 85, pp. 1249-1313, https://ssrn.com/abstract=1012360.
[33] CONADI (2023), Corporación Nacional de Desarrollo Indígena, CONADI, https://www.conadi.gob.cl.
[37] European Commission (2023), Data Act, European Commission, https://digital-strategy.ec.europa.eu/en/policies/data-act.
[81] European Commission (2020), Digital Solutions During the Pandemic.
[79] European Commission (2020), Upskilling for life after the pandemic: Commission launches new digital competence guidelines, European Commission, https://ec.europa.eu/commission/presscorner/detail/en/mex_20_1338.
[66] European Data Protection Board (2017), Guidelines on Automated individual decision-making and Profiling for the purposes of Regulation 2016/679, European Data Protection Board, Brussels.
[69] European Law Institute (2022), Model Rules on Impact Assessment of Algorithmic Decision-Making Systems Used by Public Administration.
[38] Fazlioglu, M. (2023), U.S. privacy legislation in 2023: Something old, something new?, The International Association of Privacy Professionals, https://iapp.org/news/a/u-s-federal-privacy-legislation-in-2023-something-old-something-new/.
[75] Gartner (2022), How Government CIOs Can Adopt Human-Centered Design Into Their Operating Model, Gartner, https://www.gartner.com/en/articles/how-government-cios-can-adopt-human-centered-design-into-their-operating-model.
[68] Gilman, M. (2020), Poverty Algorithms: A poverty lawyer’s guide to fighting automated decision-making harms on low-income communities, Data & Society, https://datasociety.net/library/poverty-lawgorithms/.
[53] Government of Canada (2023), Current trends - High-speed broadband, https://crtc.gc.ca/eng/publications/reports/PolicyMonitoring/ban.htm.
[47] Hadi Elzayn et al. (2023), “Measuring and Mitigating Racial Disparities in Tax Audits”, Stanford Institute for Economic Policy Research (SIEPR), Stanford.
[49] Human Rights Watch (2022), IMF/World Bank: Targeted Safety Net Programs Fall Short on Rights Protection, Human Rights Watch, https://www.hrw.org/news/2022/04/14/imf/world-bank-targeted-safety-net-programs-fall-short-rights-protection.
[46] Human Rights Watch (2020), Automated Hardship: How the Tech-Driven Overhaul of the UK’s Social Security System Worsens Poverty, Human Rights Watch, https://www.hrw.org/sites/default/files/media_2020/09/uk0920_web_0.pdf.
[4] ISSA (2023), Setting an innovation ambition in social security organizations, ISSA, https://ww1.issa.int/analysis/setting-innovation-ambition-social-security-organizations.
[76] ISSA (2023), Technical Seminar: Social security and human rights – Ensuring access and combatting the non-take-up of social benefits, ISSA, Belval, Luxembourg.
[73] ISSA (2023), Towards customer-centric design and agile methodologies in social security institutions, ISSA, https://ww1.issa.int/analysis/towards-customer-centric-design-and-agile-methodologies-social-security-institutions.
[24] ISSA (2022), Artificial intelligence in social security institutions: The case of intelligent chatbots, ISSA, https://ww1.issa.int/analysis/artificial-intelligence-social-security-institutions-case-intelligent-chatbots.
[15] ISSA (2022), Data-driven innovation in social security: Good practices from Asia and the Pacific, ISSA, https://ww1.issa.int/analysis/data-driven-innovation-social-security-good-practices-asia-and-pacific (accessed on 5 September 2023).
[52] ISSA (2022), Leaving no one behind: Experiences in digital inclusion from Europe, ISSA, Geneva, https://ww1.issa.int/analysis/leaving-no-one-behind-experiences-digital-inclusion-europe.
[19] ISSA (2020), Artificial Intelligence in Social Security: Background and Experiences, ISSA, https://ww1.issa.int/analysis/artificial-intelligence-social-security-background-and-experiences.
[70] ISSA and United Nations University (2022), Digital inclusion Improving social security service delivery, ISSA.
[42] Levy, K., K. Chasalow and S. Riley (2021), “Algorithms and Decision-Making in the Public Sector”, Annual Review of Law and Social Science, Vol. 17/1, pp. 309-334, https://doi.org/10.1146/annurev-lawsocsci-041221-023808.
[18] Lokshin, M. and N. Umapathi (2022), AI for social protection: Mind the people, Brookings, https://www.brookings.edu/articles/ai-for-social-protection-mind-the-people/.
[10] Mailes, G., M. Carrasco and A. Arcuri (2021), The Global Trust Imperative, BCG, https://www.bcg.com/the-global-trust-imperative.
[20] McClanahan, S. et al. (2021), Global research on governance and social protection: Global Overview, UN DESA, ILO, https://www.un.org/development/desa/dspd/wp-content/uploads/sites/22/2021/08/Global-overview_SP-Governance_June-2021.pdf.
[82] Ministry of Social Security (2023), Importass. El nuevo Portal de la Tesorería, Ministry of Inclusion, Social Security, and Migration Spain, https://sede.seg-social.gob.es/wps/portal/sede/sede/Inicio/informacionUtil/Importass/.
[84] Nõmmik, S. and V. Lember (2021), Proactive service provision for disabled people, Estonia, TROPICO, https://tropico-project.eu/cases/administration-costs-for-bureaucracy/proactive-service-provision-for-disabled-people/.
[25] OECD (2024), Why data governance matters, https://www.oecd.org/digital/data-governance/#:~:text=Data%20governance%20refers%20to%20diverse,and%20organisational%20and%20national%20borders. (accessed on 3 January 2024).
[36] OECD (2023), “Executive summary”, in OECD Employment Outlook 2023: Artificial Intelligence and the Labour Market, OECD Publishing, Paris, https://doi.org/10.1787/34f4cc8d-en.
[29] OECD (2023), Health at a Glance 2023: OECD Indicators, OECD Publishing, Paris, https://doi.org/10.1787/7a7afb35-en.
[3] OECD (2023), Main Findings from the 2022 OECD Risks that Matter Survey, OECD Publishing, Paris, https://doi.org/10.1787/70aea928-en.
[22] OECD (2023), Personalised Services for People in Vulnerable Situations in Lithuania: Towards a More Integrated Approach, OECD Publishing, Paris, https://doi.org/10.1787/e028d183-en.
[21] OECD (2023), Supporting Lives Free from Intimate Partner Violence: Towards Better Integration of Services for Victims/Survivors, OECD Publishing, Paris, https://doi.org/10.1787/d61633e7-en.
[1] OECD (2023), The OECD questionnaire: Harnessing Technology and Data to Improve Social Protection Coverage and Social Service Delivery, OECD, Paris.
[12] OECD (2022), Building Trust to Reinforce Democracy: Main Findings from the 2021 OECD Survey on Drivers of Trust in Public Institutions, OECD Publishing, Paris, https://doi.org/10.1787/b407f99c-en.
[17] OECD (2022), Declaration on a Trusted, Sustainable and Inclusive Digital Future, OECD, Paris, https://legalinstruments.oecd.org/en/instruments/OECD-LEGAL-0488.
[5] OECD (2022), Impact evaluation of the digital tool for employment counsellors in Spain: SEND@, OECD, Paris, https://www.oecd.org/els/emp/FinalReport-EvaluationOfSEND.pdf.
[8] OECD (2022), “OECD Good Practice Principles for Public Service Design and Delivery in the Digital Age”, OECD Public Governance Policy Papers, No. 23, OECD Publishing, Paris, https://doi.org/10.1787/2ade500b-en.
[28] OECD (2022), Report on the implementation of the OECD Recommendation on health data governance, OECD, Paris, https://one.oecd.org/document/C(2022)25/en/pdf (accessed on 3 January 2024).
[34] OECD (2019), Harnessing new social data for effective social policy and service delivery, OECD, Paris, https://www.oecd.org/els/soc/Workshop-NewSocialData-16Oct2019-BackgroundNote.pdf.
[64] OECD (2019), “OECD Framework for the Classification of AI systems”, OECD Digital Economy Papers, No. 323, OECD Publishing, Paris, https://doi.org/10.1787/cb6d9eca-en.
[61] OECD (2019), Recommendation of the Council on Artificial Intelligence, OECD, Paris, https://legalinstruments.oecd.org/en/instruments/oecd-legal-0449.
[26] OECD (2019), The Path to Becoming a Data-Driven Public Sector, OECD Digital Government Studies, OECD Publishing, Paris, https://doi.org/10.1787/059814a7-en.
[27] OECD (2017), Ministerial statement: The Next Generation of Health Reforms, OECD, Paris, https://www.oecd.org/health/ministerial-statement-2017.pdf.
[6] OECD (2014), OECD Recommendation on Digital Government Strategies, OECD, Paris, https://www.oecd.org/gov/digital-government/recommendation-on-digital-government-strategies.htm.
[35] OECD (2013), The OECD Privacy Framework, OECD, https://www.oecd.org/sti/ieconomy/oecd_privacy_framework.pdf.
[7] OECD (2010), Meeting of the Public Governance Committee at Ministerial Level: Communique, OECD Public Governance Committee, Venice.
[72] OECD (2009), Rethinking e-Government Services: User-Centred Approaches, OECD Publishing, Paris, https://doi.org/10.1787/9789264059412-en.
[32] OECD (forthcoming), Better Capturing the Experiences of Homelessness Among Women, Joint Research Centre.
[54] Office of the Auditor General of Canada (2022), Access to Benefits for Hard-to-Reach Populations, Office of the Auditor General of Canada, https://www.oag-bvg.gc.ca/internet/English/parl_oag_202205_01_e_44033.html.
[80] Okeefe, W. (ed.) (2020), DigComp at Work Implementation Guide, Publications Office of the European Union, Luxembourg, https://doi.org/10.2760/936769.
[44] O’Neil, C. (2016), Weapons of Math Destruction: How Big Data Increases Inequality and Threatens Democracy, Crown Publishing Group, New York, NY, USA.
[77] OPSI (2019), The Client Centric Policy Playbook, https://oecd-opsi.org/innovations/the-client-centric-policy-playbook/.
[30] Osoba, O. and W. Welser (2017), An Intelligence in Our Image: The Risks of Bias and Errors in Artificial Intelligence, RAND Corporation, Santa Monika, http://www.rand.org/t/RR1744.
[83] Ott Velsberg et al. (2023), Data Deluge: Do We Control Data, or Does Data Control Us?.
[55] Prosper Canada (2018), Increasing Indigenous benefit take-up in Canada, Prosper Canada, https://prospercanada.org/getattachment/f4add5df-0edb-4883-b804-60661f500c56/Increasing-Indigenous-benefit-take-up-in-Canada.aspx.
[16] Ranerup, A. and H. Henriksen (2020), Digital Discretion: Unpacking Human and Technological Agency in Automated Decision Making in Sweden’s Social Services, Social Science Computer Review, https://doi.org/10.1177/0894439320980434.
[14] Redden, J., J. Brand and V. Terzieva (2020), Data Harm Record, Data Justice Lab, https://datajusticelab.org/data-harm-record/.
[56] Robson, J. and S. Schwartz (2020), “Who Doesn’t File a Tax Return? A Portrait of Non-Filers”, Canadian Public Policy, https://doi.org/10.3138/cpp.2019-063.
[57] Sanders, C. and K. Burnett (2019), “A Case Study in Personal Identification and Social Determinants of Health: Unregistered Births among Indigenous People in Northern Ontario”, International Journal of Environmental Research and Public Health, https://doi.org/10.3390/ijerph16040567.
[65] Sebastião Barros Vale and Gabriela Zanfir-Fortuna (2022), aking Under the GDPR: Practical Cases from Courts and Data Protection Authorities, Future of Privacy Forum.
[50] Selbst, A. and S. Barocas (2018), “The Intuitive Appeal of Explainable Machines”, Fordham Law Review, https://ir.lawnet.fordham.edu/flr/vol87/iss3/11.
[43] Special Rapporteur on extreme poverty and human rights (2019), Digital technology, social protection and human rights: Report, OHCHR, https://www.ohchr.org/en/calls-for-input/digital-technology-social-protection-and-human-rights-report.
[58] SVLFG (2023), Seasonal Labour, SVLFG, https://www.agriwork-germany.de/webapp-saisonarbeit/.
[41] Swedish Transport Agency (2023), Frågor och svar kring uppgifter i media om vår it-upphandling, Swedish Transport Agency, https://www.transportstyrelsen.se/sv/Om-transportstyrelsen/fragor-och-svar/#153857.
[62] The European Parliament: parliamentary question (2022), The Dutch childcare benefit scandal, institutional racism and algorithms, https://www.europarl.europa.eu/doceo/document/O-9-2022-000028_EN.html (accessed on November 2023).
[51] The Royal Commission into the Robodebt Scheme (2023), Report: The Royal Commission into the Robodebt Scheme, The Royal Commission into the Robodebt Scheme, https://robodebt.royalcommission.gov.au/publications/report.
[2] Verhagen, A. (forthcoming), Using AI to Manage Guaranteed Minimum Income Benefits: Opportunities, Risk and Possible Policy Directions, OECD Publishing, Paris.
[31] Vogl, T. (2020), “Artificial Intelligence and Organizational Memory in Government: The Experience of Record Duplication in the Child Welfare Sector in Canada”, The 21st Annual International Conference on Digital Government Research, https://doi.org/10.1145/3396956.3396971.
[9] Wagner, B. and C. Ferro (2020), Data protection for social protection: key issues for low- and middle-income countries, Deutsche Gesellschaft für Internationale Zusammenarbeit (GIZ), https://socialprotection.org/sites/default/files/publications_files/GIZ_Data_Protection_For_Social_Protection.pdf.
[74] World Bank (2022), Service Upgrade: The GovTech Approach to Citizen Centered Services, World Bank, https://thedocs.worldbank.org/en/doc/c7837e4efad1f6d6a1d97d20f2e1fb15-0350062022/original/Service-Upgrade-The-GovTech-Approach-to-Citizen-Centered-Services.pdf.
[78] World Bank (2016), https://www.worldbank.org/en/publication/wdr2016, World Bank, https://www.worldbank.org/en/publication/wdr2016.
[71] Wu, D. and B. Meyer (2023), “Certification and Recertification in Welfare Programs: What Happens When Automation Goes Wrong?”, NBER Working Papers, https://www.nber.org/system/files/working_papers/w31437/w31437.pdf.
[11] Zhang, B. and A. Dafoe (2019), Artificial Intelligence: American Attitudes and Trends, University of Oxford, https://governanceai.github.io/US-Public-Opinion-Report-Jan-2019/index.html.
Notes
← 1. The OECD Council Recommendation concerning Guidelines covering the Protection of Privacy and Transborder Flows of Personal Data recognises this tension, noting that “more extensive and innovative uses of personal data bring greater economic and social benefits, but also increase privacy risks” (OECD, 2013[35]).
← 2. The survey cited refers to BCG 2020 Digital Government Citizen Survey, a survey of 24 500 citizens across 36 countries: Argentina, Australia, Austria, Bangladesh, Canada, Chile, China, Denmark, Estonia, France, Germany, Hong Kong, India, Indonesia, Japan, Kazakhstan, Kenya, Malaysia, Morocco, the Netherlands, New Zealand, Nigeria, Norway, Poland, Qatar, Russian Federation, Saudi Arabia, Singapore, South Africa, South Korea, Sweden, Switzerland, United Arab Emirates, United Kingdom, Ukraine, and United States (Mailes, Carrasco and Arcuri, 2021[10]).