This chapter defines concepts related to systemic threats and reviews the analytical and governance approaches and strategies to manage these threats and build resilience to contain them. This aims to help policymakers build safeguards, buffers and ultimately resilience to physical, economic, social and environmental shocks. Recovery and adaptation in the aftermath of disruptions is a requirement for interconnected 21st century economic, industrial, social, and health-based systems and resilience is an increasingly important theme and a crucial part of strategies to avoid systemic collapse.
A Systemic Recovery
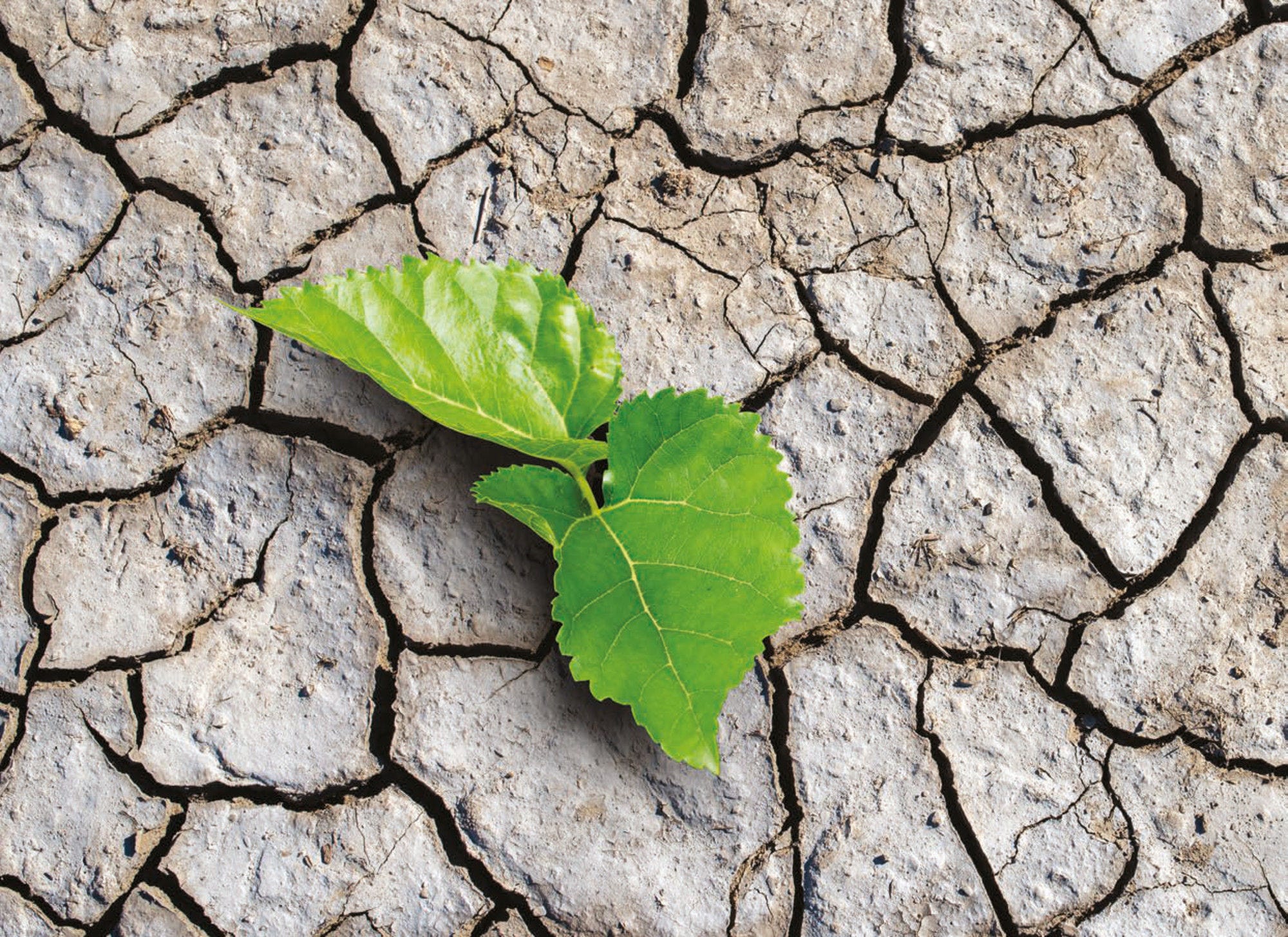
4. Resilience Strategies and Approaches to Contain Systemic Threats
Abstract
Introduction
Modern society proceeds on the assumption that a number of complex systems will work reliably, both individually and in their interactions with other human and natural systems. This bold assumption generally holds true. Yet this assumption is being challenged by the scientific community and, increasingly by policymakers too, on the basis and improved understanding of how systems work, and by drawing on the lessons of disruptive historical events.
The immense variety of complex systems gives rise to an equally vast array of systemic risks, identifying which is made more difficult by the characteristics of the systems in question. It is possible though to characterise the nature of these threats as involving a process of contagion that spreads individual failures to the system as a whole, and where disruption in one area can cascade through the system as a whole. The key insight here is that interconnectedness brings many benefits, but it also poses new problems and can intensify the danger from existing threats. Moreover, although the consequences of a systemic threat being realised may be dramatic, the probability of this event occurring may be small. This combination of high impact, low probability, and propagation that is hard to predict makes the task of the policymaker trying to address systemic risk arduous. It requires a different approach to dealing with risks and potential system failures.
This chapter argues that resilience can provide a philosophical and methodological basis to address systemic risk in a more useful way than traditional approaches based on risk management. Resilience approaches emphasise the characteristics and capabilities that allow a system to recover from and adapt to disruption. For a resilience approach to be useful to policymaking, the domains of resilience have to be identified, along with the potential sources of system collapse.
The risks associated with the trends most likely to influence our economies and societies in the future, notably AI and digitalisation, are not amenable to traditional approaches and will present challenges that we cannot anticipate or prepare to meet using traditional tools. The following sections look at how to define systemic threats and how to understand a growing global concern. The chapter will then look at the diverse nature of systemic threats and introduce the need for resilience as a philosophy and tool to understand and address systemic threats. The next section discusses how to use history as a lens to look at civilisational collapse or survival amidst systemic threats. The practical implications and methods for identification and management of systemic threats are then outlined, before concluding with a discussion of future systemic challenges facing society.
Systemic threats, a growing global concern
Defining systemic threats
Systemic threats have been defined in numerous ways such as Centeno et al. (2015) “the threat that individual failures, accidents, or disruptions present to a system through the process of contagion”. The International Risk Governance Center contends that systemic threats arise when “systems [...] are highly interconnected and intertwined with one another”, where a disruption to one area triggers cascading damages to other nested or dependent nodes (IRGC, 2018). Further, IRGC (2018) states that “external shocks to interconnected systems, or unsustainable stresses, may cause uncontrolled feedback and cascading effects, extreme events, and unwanted side effects”, implying that the potential for cascading disruption is a growing and critical concern for many facets of daily life.
Systems susceptible to systemic risks are intertwined with one another in a series of nested relationships. Renn (2016) describes how such interconnectivity facilitates stochastic, non-linear, and spatially interspersed causal structures that, if triggered, contribute to a ‘domino effect’ that can permanently alter a broader infrastructural, environmental, or social system. Due to this nature, “there is a high likelihood of catastrophic events once the risk arrives” (IRGC, 2018).
Certain triggers of systemic threats can be violent and forceful, jarring a relatively stable and sustainable system into an altogether different configuration. Such events are generally ‘low-probability yet high‑consequence’, and are difficult to predict via conventional modelling techniques. Once the acute disruption occurs, a chain reaction of systemic shift occurs until a new stasis is achieved. Other triggers are more chronic in nature, for example gradual climactic shift or slight overfishing within a given ocean or sea. These are initially limited in impact, yet can eventually be overwhelming and unstoppable in their effect. IRGC’s Guidelines for the Governance of Systemic Risk (2018) states that such threats are best addressed early through the detection and interpretation of weak signals, although further notes that slow‑moving chronic systemic threats can be nearly imperceptible in their earliest stages.
Key to slow-moving chronic threats is the notion of transitions. Lucas et al. (2018) and Pelling (2012) frame these transitions as ‘tipping points’ where a system edges towards a critical inflection point that may foster a transformation from one system permutation in favour of another. If breached, tipping points can cause feedback loops and nonlinear effects that cause a system to shift and change in an increasingly dramatic form.
Systemic threats are characterised by their capacity to percolate across complex interconnected systems - either through an abrupt shock, or gradual stress (IRGC, 2018). Systemic threats are particularly difficult to model and calculate via a risk-based approach due to a mixture of the weak signals of the potential risk event plus the nested interaction effects by which a systemic threat disrupts a system in an indirect manner. For example, the, 2008 financial crisis began as a collection of relatively contained failures of financial firms, which ended in a substantial financial collapse across much of the world.
The diverse nature of systemic threats - the need for resilience
Many systems would benefit from a resilience-based approach, particularly systems with inherent nested interdependencies with others, or those prone to low-probability, high-consequence events that are difficult to accurately predict or model. Resilience helps these systems prepare for disruptions, cope with and recover from them if they occur, and adapt to new context conditions (National Academy of Sciences (NAS), 2012; Linkov and Trump, 2019).
Resilience has been used as a metaphor to describe how systems absorb threats and maintain their inherent structure and behaviour. More specifically, resilience is used as a global state of preparedness, where targeted systems can absorb unexpected and potentially high consequence shocks and stresses (Larkin et al., 2015). Common usage of resilience causes scholars to infer several principles of what resilience actually means. The first principle includes the positivity of resilience, or the notion that resilience is an inherently beneficial goal to achieve. The second includes the measurement of resilience by characteristics believed to apply to a given system - effectively driving an inductive approach to resilience thinking (Bene et al., 2012). Third, resilience thinking is often viewed in a context-agnostic framework, where principles of resilience can be applied to various situations and cases interchangeably.
We define resilience as the capability of a system to recover in the midst of shocks or stresses over time. Recovery implicates multiple interactions between factors, and across scales and sub-systems, that are usually unexpected and complex in nature. Given such concerns, resilience differs from traditional methodological approaches of protecting against risk, where these uncertain and complex shocks and stresses that affect targeted systems are inherently outside of the design of the system’s intended purpose. Preparation for such events contains only limited guidance, and promoting traditional risk approaches such as bolstering system hardness is often excessively difficult and prohibitively expensive. Resilience allows us to address these concerns within a framework of resource constraints and the need to protect against low probability, high consequence events, sometimes described as ‘black swans.’ In other words, resilience is preferred to traditional risk management strategies where a systems-theory of protecting against risk is required, and where the potential risks in question are highly unlikely yet potentially catastrophic in nature.
Resilience affords greater clarity on systemic threats by focusing upon the inherent structure of the system, its core characteristics, and the relationship that various sub-systems have with one another to generate an ecosystem’s baseline state of health (IRGC, 2018). Walker et al. (2004) define ecosystem equilibria as a characteristic of “basins of attraction”, where the components and characteristics of a system drive it towards a baseline state of health and performance. For example, the Pacific Ocean ecosystem has a tremendous diversity of flora and fauna whose roles in complex food webs have been reinforced by millions of years of evolution and adaptivity; a localised oil spill may damage small points of ecosystem health but is unlikely to dramatically and permanently shift the species dynamics and food webs across most of the Ocean. However, constant exposure microplastic or other pollution can jolt system equilibria in a manner that favours a differing basin of attraction. Unfortunately, we are moving in that direction already, where huge regions of oxygen-depletion in the Pacific Ocean are contributing to ‘dead zones’ where virtually no marine life can survive.
Basins of attraction are comprised of complex interconnected and adaptive systems that are constantly under stress, yet only shift to a new equilibrium if a tipping point has been breached and the system is trending towards a new basin. Resilience-based approaches can help us understand when and how certain ecosystems might shift from one steady-state to another (Linkov et al., 2018), as well as define the biological and ecological drivers which cause an ecosystem to arrive at a steady equilibrium altogether.
Resilience as a philosophy and tool
Resilience for complex systems
As a term, resilience has centuries of use as a descriptor in diverse fields. The modern application has centred upon analysing how systems bounce back from disruption. This seems simple enough at first glance, yet the methodological application and analysis of how systems do, in fact, bounce back post-disruption can be quite challenging.
A, 2012 National Academy of Sciences (NAS) report on disaster resilience defines resilience as the ability of a system to perform four functions with respect to adverse events: planning and preparation, absorption, recovery, and adaptation. Nevertheless, quantitative approaches to resilience have neglected to combine those aspects of the NAS understanding that focus on management processes (planning/preparation and adaptation) with those that focus on performance under extreme loadings or shocks (absorption and recovery). Advancing the fundamental understanding and practical application of resilience requires greater attention to the development of resilience process metrics, as well as comparison of resilience approaches in multiple engineering contexts to extract generalisable principles.
A core problem is that risk and resilience are two fundamentally different concepts, yet are being conflated. The Oxford Dictionary defines risk as “a situation involving exposure to danger [threat]”, while resilience is defined as “the capacity to recover quickly from difficulties.” The risk framework considers all efforts to prevent or absorb threats before they occur, while resilience is focuses on recovery from losses after a shock has occurred. However, the National Academy (2012) and others define resilience as “the ability to anticipate, prepare for, and adapt to changing conditions and withstand, respond to, and recover rapidly from disruptions.” In this definition, adapt and recover are resilience concepts, while withstand and respond to are risk concepts, thus the risk component is clearly added to the definition of resilience. Further, approaches to risk and resilience quantification differ. Risk assessment quantifies the likelihood and consequences of an event to identify critical components of a system vulnerable to specific threat, and to harden them to avoid losses. In contrast, resilience-based methods adopt a ‘threat agnostic’ viewpoint.
We understand resilience as the property of a system and a network, where it is imperative for systems planners to understand the complex and interconnected nature within which most individuals, organisations, and activities operate. Risk-based approaches can be helpful to understand how specific threats have an impact upon a system, yet often lack the necessary characteristic of reviewing how linkages and nested relationships with other systems leave one vulnerable to cascading failure and systemic threat. Resilience-based approaches serve as an avenue to understand and even quantify a web of complex interconnected networks and their potential for disruption via cascading systemic threat.
Resilience is both a philosophy and a methodological practice that emphasises the role of recovery post-disruption as much as absorption of a threat and its consequences. This mindset is grounded upon ensuring system survival, as well as a general acceptance that it is virtually impossible to prevent or mitigate all categories of risk simultaneously, and before they occur. Methodologically, resilience practitioners seek use limited financial and labour resources to prepare their system for a wide variety of threats - all the while acknowledging that regardless of how well the system plans for such threats, disruption will happen.
Risk assessment and management is concerned with accounting for systemic threats, but this typically on a threat-by-threat basis to derive a precise quantitative understanding of how a given threat exploits a system’s vulnerabilities and generates harmful consequences. Such an exercise works well when the universe of relevant threats is thoroughly categorised and understood, but has limitations when reviewing systemic risk to complex interconnected systems. Resilience complements traditional risk-based approaches by reviewing how systems perform and function in a variety of scenarios, agnostic of any specific threat.
Some theoretical and empirical implications of the definition of resilience above have to be taken in consideration. They are seldom, or are not explicitly, included in assessment.
Time and experiential learning
Linkov et al. (2014) outlined resilience as a function of system performance over time, which we extend to argue that system resilience includes the past experiences that a given system has encountered that have stressed its capacities for service delivery or normal function. In other words, exposure to previous shocks and stresses can have a direct effect upon the system’s ability to recover from future shocks and stresses. Coupled with the ability of a system to absorb shocks and stresses while still maintaining important functions, recovery serves as an essential component to judge whether a system is resilient in the face of challenges. This phenomenon is driven by the adaptive capacity of a system. Systems that have been exposed to shocks and stresses are more likely to have the experience and memory to adapt in the face of new and emerging challenges, like the human body producing antibodies to infections.
The shifting capacity of a system
Stresses can occur throughout the system’s development. Individual strategies can both improve an individual system’s resilience to certain stresses while also increasing the system’s brittleness in the face of certain shocks. In other words, it is possible for a system to become increasingly adaptive, yet also become increasingly brittle and susceptible to disruptions from shocks and stresses. For example, while investment markets continually adapt and develop resilience to external shocks, they become increasingly brittle through growing system complexity and appetite for risky investments. These actions are individually rational (i.e. investors seek to grow profits by approving riskier trades that are generally sound but have a higher chance of failure), yet can increase the potential for the market as a whole to enter recession as a large enough aggregate of investments fail and companies enter default. In this way, the stock market slowly trends towards brittleness in a rational manner over time.
How resilience addresses systemic threats
The key question resilience practitioners seek to answer is “how can I make sure my system performs as well as possible during disruption, and recovers quickly” (Figure 4.1)? More specifically, “How can I make sure my system is not vulnerable to cascading disruption posed by systemic threats”?
These questions are particularly salient for the study of complex systems, where large organisations like hospitals rely upon the smooth operation of various connected systems and subsystems to function properly (the energy grid, information systems, patient administration, medical supply chains, etc.). Regardless of the situation to which it is applied, resilience requires one to think in terms of how to manage systemic, cascading threats, where a disruption to one subsystem can trigger dramatic changes to other connected systems. This is a complex task with few formalised answers, but a central requirement for analysts is to frame resilience as a function of both time and space due to the multitemporal and cross‑disciplinary view by which one must review systemic threats.
Figure 4.1. Role of Resilience in Systems, Emphasising Importance of Combating Disruptions
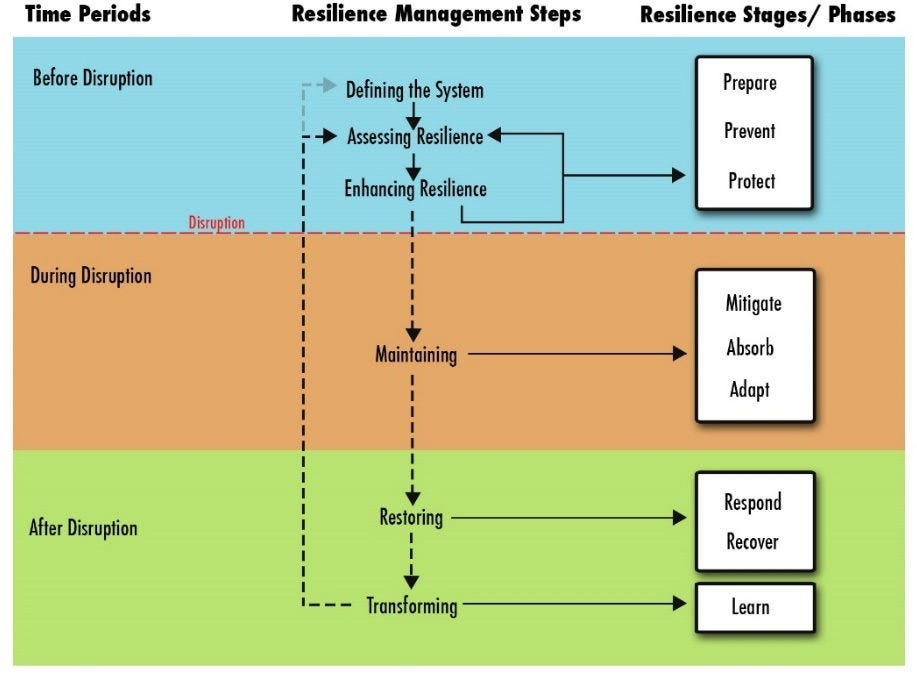
Stages of resilience
Resilience is less a singular moment when a disruption causes losses, but instead a process of how a system operates before, during, and after the threat arrives. System resilience is an ever-changing characteristic whereby a system’s core functions are constantly shifting to deal with threats.
Most conventional, risk-based approaches emphasise the plan/prepare and withstand/absorb phases to identify, assess, and prevent/mitigate threat (Linkov et al., 2018). Regardless of whether a specific threat is considered, these stages focus upon identifying and interpreting signals associated with threats to a system; exploring the structure and connections that a system has with others; and identifying strategies that preserve a system’s core capacity to function regardless of the disruption that occurs (Patriarca et al., 2018; Park et al., 2013).
While the plan/prepare and absorb/withstand stages are important to help a system address threats before they occur and as they arise, resilience approaches must also consider how a system performs after the threat has arrived. This includes recovery and adaptation. Recovery includes all efforts to regain lost system function as quickly, cheaply, and efficiently as possible. Adaptation centres on a system’s capacity to change and better deal with future threats of a similar nature. Dealing with recovery and adaptation constitute the main additions by resilience to risk analysis, assessment, and management, and force stakeholders to take account of percolation effects due to disruptions. The role of adaptation and recovery is the focus of resilience analyst. A system with a robust capacity for recovery can weather disruptions that would otherwise break even the most hardened of system components.
Domains of resilience
The spatial component of resilience requires one to consider how a disruption to one system can trigger consequences in others - including those that have indirect or unapparent linkages to the disrupted system.
Alberts and Hayes (2003) identify four different Network-Centric Operation (NCO) domains important to a system’s agility, or what they later define as “the ability to successfully effect, cope with, and/or exploit changes in circumstances” (Alberts and Hayes, 2006). Each domain is impacted in a different yet equally important manner when a critical event or disruption arises, and success in one domain may not guarantee the same outcome in the other areas.
The physical domain represents where the event and responses occur across the environment and is typically the most obviously compromised system in the midst and aftermath of an external shock or critical risk event. Elements here can include infrastructural characteristics ranging from transportation to energy or cyber networks (DiMase et al., 2015). The physical domain of resilience thinking generally includes those infrastructural factors that are most directly impacted by a hazardous event, where the other domains include outcomes and actions that are a response to damage to physical capabilities and assets. In this domain, the objective of resilience analysis is to bring the infrastructural or systems asset back to full efficiency and functionality.
The information domain is where knowledge and data exist, change, and are shared, including public or private databases, which are increasingly under potential attack from private hackers and other aggressive opponents (Osawa, 2011; Zhao and Zhao, 2010). Another growing target for information domain-type risks includes stored online communications (Murray et al., 2014; Berghel et al., 2015; Petrie and Roth, 2015). For this domain, the objectives of resilience management are to prepare information assets for a variety of potential attacks while also assuring that these systems will react quickly and securely to such threats in the immediate aftermath. In this way, risk preparedness, risk absorption, and risk adaptation make information and cybersecurity resilience a growing priority for a variety of governmental and business stakeholders (Linkov et al., 2013; Collier et al., 2014; Bjorck et al., 2015).
The cognitive domain includes perceptions, beliefs, values, and levels of awareness, which inform decision-making (Linkov et al., 2013; Eisenberg et al., 2014). Along with the social domain, the cognitive domain is the “locus of meaning, where people make sense of the data accessed from the information domain.” (Linkov et al., 2016). Such factors are easy to overlook or dismiss due to a reliance upon physical infrastructure and communication systems to organise the public in response to a disaster, yet perceptions, values, and level of awareness of the public concerning strategies to overcome shocks and stresses are essential to the successful implementation of resilience operations (Wood et al, 2012). In other words, without clear, transparent, and sensible policy recommendations that acknowledge established beliefs, values, and perceptions, even the best-laid plans of resilience will fail. A robust accounting for the cognitive domain is particularly important for instances where policymakers and risk managers may have a disconnect with the local population. For such cases, policy solutions which may seem simply common sense to the policymaker or risk manager and assumed to be robust, may be rejected by locals as contrary to established custom or practice.
The social domain is characterised by interactions between and within the entities involved. Social aspects have impacts on physical health (Ebi and Semenza, 2008). For example, individuals or communities can have better recovery in the face of epidemic when they also have strong social support and social cohesion. The social domain also ties into the information domain in regard to trust in information. When the community does not trust the source of information, they often do not trust the information itself or have to take the time to verify it (Longstaff, 2005).
While the physical and cognitive domains attract a lot of attention in both overall resilience and hazard- specific resilience, the information domain is of great importance for overall functioning, given its impact on citizen response. Not all individuals understand and interpret information the same way and attention has to be paid to getting information out effectively and in a timely fashion during a crisis. Adequate information is crucial in real time for authorities to make informed and appropriate decisions. As important as information is in itself, human interpretation of data is important, since raw numbers can be misleading if not considered in its context. The way in which authorities and citizens handle information should be evaluated with careful consideration for the communities being discussed.
For smaller communities, organisations, and businesses, discussions of resilience may centre on the ability of local governments and communities to address long-term concerns such as the impact of climate change (Berkes and Jolly, 2002; Karvetski et al, 2011), ecological disasters (Adger et al., 2005; Cross, 2001), earthquakes (Bruneau et al., 2003), and cybersecurity (Williams and Manheke, 2010), as well as other hazards. For larger communities and governments, such concerns are similar yet often more complex and varied in nature, involving hundreds to potentially thousands of stakeholders and the interactions of various systems.
These domains often overlap. For example, information has to be shared over physical infrastructures and within social groups. A focus on domains ensures that a policymaker or risk manager acquires a holistic understanding of their policy realm and are able to understand how a shock or stress could trigger cascading consequences that were previously difficult to comprehend.
History as a lens for civilisational collapse or survival
Though systemic threats and civilisational disruption are framed as 21st Century concerns, they also help frame reasons why previous civilisations collapsed or survived in the midst of extreme disruption. Learning from past events can allow us to identify why some societies, economies, or ecologies persisted despite disruption, while others collapsed in the face of an adverse event.
Defining and understanding collapse in history
Collapse is a particularly difficult term to succinctly define in a manner that various disciplines and scholars would appreciate. For example, collapse could refer to elimination, as happened to the Austro-Hungarian Empire after World War I, or it could refer to civilisations that were fundamentally changed by disruption, despite their persistence and ability to survive in a differing form, such as the Eastern Roman Empire surviving for nearly a thousand years after its Western counterpart.
For our purposes, collapse refers to the permanent breakdown of complexity in the socioeconomic network of a given state. Significant disruptions such as economic collapse or invasion represent situations where activities requiring significant resources or energy to operate are not able to continue. In these situations, societal complexity decreases in the face of disruption until it reaches a sustainable inflection point, thereby reforming and rebuilding into an entirely new configuration.
In the face of severe disruption, the order and stability provided by a complex society is lost and replaced by increased levels of disorder and anarchy. In a workshop sponsored by the Princeton Institute for International and Regional Studies Global Systemic Risk project, (PIIR GRS) loss of civilisational complexity and order was seen as not necessarily due to the extreme degradation of the nodes of civilisational systems (centres of commerce, religious houses, or centres of legal and judicial authority), but may also be triggered by a disruption of the linkages between those nodes (transportation networks, trade routes, communications systems, environmental conditions that prevent collaboration of civilisations due to changing climactic conditions). Historical civilisational collapse is viewed as a systemic exercise, where such disruptions are understood as a disruption of a basic nested system requirement (i.e., a link between nodes) that the society cannot survive without in its current form. Disruption to that nested system requirement cascades into other systemic losses and even collapse and contributes to a reformation of a society or civilisation in an entirely new manner.
To address systemic threats’ potential to trigger collapse, core questions discussed by Princeton Institute for International and Regional Studies (PIIRS) Global Systemic Risk (GSR) research included: How fragile are the connections between all the system nodes? How could these be disrupted? How could such disruptions lead to a catastrophic breakdown? What would the costs associated be and for whom? These questions require one to acknowledge that societal collapse is almost always due to a multivariate causal explanation - no single event or cause is responsible for the society’s disruption and fall. Instead, multiple interlocking disruptions trigger feedback loops that amplify the effect of the disruption upon societal systems.
Sources of collapse
Princeton’s PIIRS GSR notes that one of the most common sources of collapse is the failure of political authority. This can take two stages: its most obvious stage is the breakdown of the monopolisation of control over the means of violence. Authority and the administration of justice is increasingly localised to a regional or even household level, increasing the uncertainty that an encounter would lead to a legitimised violent outcome with few options for adjudication by a higher power. In its most Hobbesian stage, it is literally “all against all” where “mere anarchy is loosed upon the world.”
A much more important breakdown might precede or succeed this: the diminishing of the idea of communal legitimacy. All systems rely on some understanding of “rules that need not be spoken.” This can range from the epistemological authority to ethical authority, to bureaucratic authority. Other sources of collapse might be crises in economic production and consumption or the collapse of infrastructures. Another form of collapse might involve the cultural and physical segregation of individual. Today, technology makes it almost impossible not be aware of what is going on thousands of miles away and to have at least intermittent contact with people around the world. But while multiple connections are usually a sign of order, they can also lead to contagion.
The most common forms of collapse might be summarised as the Biblical four horsemen: war, conquest, famine, and plague.
What collapses when?
One of the most common critiques of the “collapse” literature is that it has a binary bias—civilisation and barbarism, that it occurs very quickly, and that once it does, it leaves little comprehensible residue. (Thus, the common Armageddon trope of post-apocalyptic savages not understanding what a car is or what buildings were for.) We can think of the following as critical variables of the “timing” of collapse.
What survives? Just because a large system with a clear hierarchy collapses does not mean that daily life at the household level is disrupted. Those at the social bottom may not even recognise that anything has happened for some time. The key task here is to measure the territorial/demographic scope of fragmentation and aggregation. This is particularly useful for comparing parts of a previous system that undergoes dramatic change, but with very different consequences, as in the example of the West/East Roman Empire(s).
How long does it survive? With the new interest in resilience, we can also imagine a series of cycles of fragmentation and aggregation as the system reorganises itself. Thus, a “civilisation” or a cultural system may persist in its individual branches following a systemic “collapse.”
When we think of a collapse, we often imagine it in a short time span—a day as in the case of nuclear aftermath, a generation in terms of an eroding empire. But the velocity of a collapse may vary across time and space and we need to recognise “stages” or “tipping points.” We can also think of cycles. Holling (2001) provides a theoretical model of the cycles of ecosystem organisation that attest to “adaptive capacity.”
Why does it collapse?
When collapse is due to exogenous causes, nothing about the society would lead one to expect a collapse, but the entry of some other factor or event such an invasion or natural cause such as environmental change destroys the basis of the system. On the other side of causality, the system collapses because of its own endogenous qualities. For example, it depends too much on a tightly knit and complex base, which cannot endure, or an elite becomes corrupt and no longer does the system maintenance required by its function in a society. Helbing (2018) focuses on the degree of interdependence and the possible limits of organisation. Downey et al. (2016) identified a “boom-bust” cycle in the European Neolithic that seems to indicate a loss of resilience due to the introduction of agriculture.
Systemic threats in history
While contemporary technology and the level of global integration may be new, many of the systems, mechanisms, dynamics, and foundations of civilisation (food, water, health, trade, transportation, peace, security, and dependence on technologies) are the same. Different historical failures may have systemic commonalities that have not yet been studied from an interdisciplinary point of view.
We may begin with Joseph Tainter’s definition of social complexity: “the size of a society, the number and distinctiveness of its parts, the variety of specialised roles that it incorporates, the number of distinct social personalities present, and the variety of mechanisms for organising these into a coherent, functioning whole” (Tainter, 1988). The maintenance of this complexity requires ever more amounts of physical and social energy to maintain, and this in and of itself becomes an increasing strain on society. Peter Turchin (2015) has a similar fascination with what he might call “organisation” as described in Ultrasociety. This may be best expressed by the exponential increase in both population and per capita energy use that has endangered our survival as a species. In short, we take for granted an unprecedented level of social organisation in the modern world, the fragility of which is a critical topic of study.
The second motivation follows the work by Kai Erikson (1977) and his belief that social life may sometimes be best understood through the prism of catastrophe. The argument is simple: if we wish to understand the most important social structures, we might best analyse what happens when these and their supporting institutions disappear. How much crime without police, how much illness without medicine, how much exchange without markets? When significant aspects of society come apart, we can better appreciate what they contributed to the status quo ante and how societies evolve to deal with their development. We have significant amounts of historical analysis of catastrophes, but have mined relatively little of this for sociological insights.
Identification and management of systemic threats
Methodological input requirements for risk and resilience of systemic threats
Risk quantification is an essential element of any risk or resilience management tool. Along with a consideration of the scope and severity of the hazards that may accrue from a given activity, classification efforts largely depend on the type and abundance of information available. A decision maker is unlikely to consecrate significant time and resources to promoting a costly and time-intensive classification effort for a project with a small and inconsequential universe of potential negative outcomes. Likewise, decision makers would be less hasty in their efforts to push early-stage risk classification forward without thorough analysis (although there are tragic and famous cases to the contrary). While early stage classification efforts are imperfect in focus due to their unavoidably subjective nature, they generally serve as a reflection of the realities facing decision makers and stakeholders.
For cases where more objective information is available, risk quantification allows for greater precision with the risk classification effort (assuming, of course, that the data and model used are both relevant and rigorous). This precision is derived from robust sources of lab or field data that, if produced in a transparent and scientifically defensible manner, indicate statistically significant trends or indications of risk and hazard. Over time, multiple trials and datasets with similar indications ultimately contribute to risk profiles that establish best practices. As new studies and information become available, these best practices may be improved or updated to sharpen existing perceptions of risk. Because of this, the process of improving risk classification for a given project or material is continuously evolving. Just because quantitative information provides for more objective judgment, however, does not indicate a total absence of subjectivity in the risk classification process.
There are many instances where objective data is not available, for example because acquiring information may be legally or morally irresponsible, the application in question may be too novel for rigorous experimentation to have taken place, or the available data may be outdated or irrelevant for the particular risk application at hand. Such concerns are common with respect to new or emerging technologies or future risks. Under these limitations, risk and resilience managers are required to turn to qualitative information, for example expert opinion - a process which can set risk priorities in order if done correctly.
Regardless of the approach chosen to classify risk, resilience analysis quantification requires several component parts for it to be conducted in a transparent and defensible manner. These characteristics include (Merad and Trump, 2020) the availability of an outlined and transparent dataset, derived qualitatively or quantitatively; a framework or approach to process the data in a scientifically defensible and easily replicable fashion; pre-established notions of resilience success and failure, or various gradients of both; and considerations of temporal shifts that may strengthen or weaken system resilience in the midst of a variety of factors, including those which are highly unlikely yet particularly consequential.
Dataset requirements do not strongly differ from more traditional risk assessment or other decision analytical methods. Risk and resilience analysis requires a dataset with a clear connection to the host infrastructure or system and the various adverse events that could threaten it, along with a consideration of how recent and relevant the data may be to decision making. Regardless of the type of data collected (qualitative, quantitative, or a mix of the two), the dataset must have a clear and indisputable connection with the resilience project. This should be a relatively simple exercise, however, as the dataset is either collected directly by the resilience analysts for their given project or is acquired from a similar project’s data collection activities. Where no quantitative data is available, qualitative information may be classified in such a way as to serve as a temporary placeholder to allow analysis to continue (Vugrin et al., 2011). In this way, the type or quality of data available can directly inform the method chosen to process available information for resilience analysis (Francis and Bekera, 2014; Ayyub, 2014).
After acquiring a dataset, the next requirement of resilience quantification is a framework to process that data. Method selection is driven by a variety of factors, notably the quality and robustness of available data as well as statutory requirements for output and transparency (Francis and Bekera, 2014; Linkov et al., 2014).
A crucial step is the imposition of pre-determined notions of system success or failure. This is consistent with virtually any other branch of scientific inquiry, where users must establish some notion of ‘goodness’ or ‘badness’ that they seek to identify prior to data manipulation and results classification. Such efforts to establish system resilience success and failure could be generalist in nature (as in, deploying categorical variables or quantitative cut-off points that signify a positive or negative performance under certain stressors) or specific (as with the use of extensive quantitative data to inform precise points of system failure, as with the use of levees in flood management). Opting either way is at the discretion of the resilience analyst and their stakeholders, in line with the degree of precision needed to assess system resilience. Some stakeholders may be satisfied with answers such as “there is a moderate probability that system x could fail under condition y”, while in other cases, stakeholders may need to know the exact conditions and points at which degradation and/or failure occur. Generally, with more information available and the more potential the system has of incurring damage to society if broken, the greater the precision needed to assess systemic resilience success or failure.
The great challenge of resilience analysis and decision making is to consider a wide range of time horizons over which hazards and challenges could arise to shock a system, project, or infrastructural asset, where such events may not be projected to be possible until several years, decades, or even centuries into the future. Given this, an analyst should seek to discuss shifting preferences, threats, and system capabilities over time with stakeholders and managers to gain a more accurate view of how a system may be challenged and behave in the midst of an external shock, with additional considerations with regards to how those systems could evolve and become more resilient over time.
One solution is to use a structured framework for selection metrics and organising the assessment. The individual performance factors are kept separate for more easy interpretation but can be aggregated to a single score, if relevant. The Resilience Matrix, described in the next section, provides a two-dimensional approach to selecting metrics, rather than a one-dimensional list of factors. More specifically, the Resilience Matrix explicitly incorporates the temporal phases of the event cycle, identified in the National Academy of Sciences definition of resilience: prepare, absorb, recover, and adapt.
A semi-quantitative approach: Resilience Matrix
A matrix assessment methodology affords users the capability to construct a framework that compares various decision metrics on a broad, ‘big picture’ level of resilience thinking and decision making. Resilience matrix approaches assist local level stakeholders and policymakers focused on resilience performance, as well as broader and regional emergency response teams who seek to institute resilience thinking to “adopt a more holistic view of resilience necessary to reduce the impact of an adverse event” (Linkov et al., 2013). Collectively, the development and execution of resilience matrices will provide robust and transparent policy guidance for national policy goals, while also offering improvements to large-scale system resilience for areas ranging from industry to energy to medicine (Kelic et al., 2013; Rosati et al., 2015; Roege et al., 2014).
Figure 4.2. Resilience Matrix (RM)
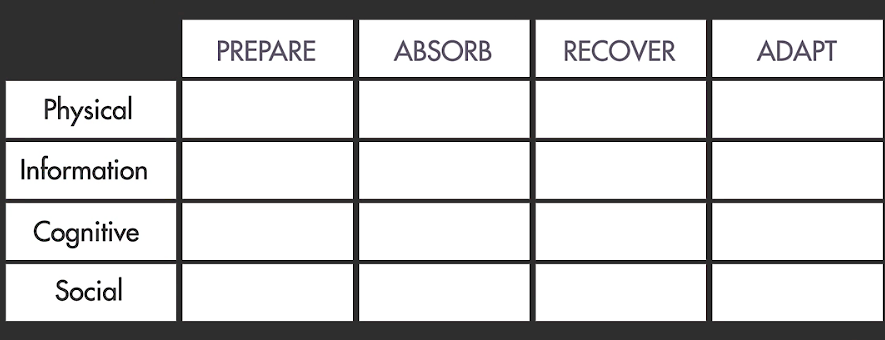
Note: The y-axis includes domains of resilience, the x-axis includes stages of resilience as established by the US National Academy of Sciences (NAS)
Source: Linkov et al. (2013)
Resilience corresponds to a system’s ability to perform critical functions in the midst of catastrophic and unexpected happenings. Described by Linkov et al. (2013), a resilience matrix collectively provides a unifying framework to assess system resilience which may be applied productively to societies and groups, when seen as systems (Figure 4.2). Linkov et al.’s (2013) formal Resilience Matrix (RM) classifies four general resilience domains of complex systems that include a mixture of physical infrastructure and more abstract capabilities and takes into account the performance of these domains throughout the event’s occurrence and disruption. The RM does not define specific metrics or attributes to use, but it gives guidelines to select the appropriate measurements to judge functionality from the perspective of a broader system. The RM guidelines diverge from the accomplishments of different community resilience progressions by taking advantage of a stakeholder-driven approach to characterise signs and ranges of system progression that are directly related to the community. Progression is characterised in relation to the necessities of the local environment rather than against the advancement of some generalised or national goal, which could or could not be acceptable in the local setting.
Cutter et al. (2014) reflect the difficulty to specify values of community resilience that are accepted nationally, and no clear formulation for the approval of an external source of values of community resilience is given at this time. As a consequence, the acceptability and usability of any resilience judgment can only be assessed by the community in which it is utilised. Stakeholders are prompted to incorporate values from those identified by other resilience assessment strategies, where accessible, as important signals which connect the RM with other formulations to balance the strength of both approaches. The RM’s simplified guidelines promote other strong attributes as well. Interdependences are ubiquitous in all systems, but the time and cost it takes usually prohibits the investigation and modelling of all of these dependencies.
The basic idea underlying the use of the RM is that to create resilience, achievement in all sectors of the system must be identified. This is different from the methodology of solutions which maximise singular factors of the system. A consequence of such a narrow focus is that failures in the system can lead to cascading effects; the collapses of communities in light of calamities are frequently an effect of overflowing collapses from critical components in the system that are not identified as such. To be resilient on any scale, singular time steps cannot be relied upon to restore functionality. Even though the real relationship between system factors may not be revealed, by improving the resilience of all aspects of the system, performance can be kept or quickly restored. The Resilience Matrix methodology includes a set of guidelines for the resilience judgment for systems that has already been produced for cyber, energy, engineering, and ecological systems.
The RM consists of a framework to conduct assessments regarding the performance of complex and of incorporated systems or projects across varying focal points. Generally, risk matrix frameworks consist of a 4x4 matrix, “where one axis contains the major subcomponents of any system and the other axis lists the stages of a disruptive event” (Fox-Lent et al., 2015). Next, matrix rows include the four primary domains to be considered within any systemic evaluation project, including physical, information, cognitive, and social (Alberts and Hayes, 2003). Additionally, matrix columns illustrate the four steps of disaster management, including the plan/prepare, absorb, recover, and adapt phases of resilience management as outlined by the National Academies of Science (Committee on Increasing National Resilience to Hazards and Disasters, 2012). Altogether, these sixteen cells give a basic description of the performance of the system throughout an adverse event.
In order to begin a resilience assessment utilising the matrix approach, Fox-Lent et al. (2015) recommend: (1) clearly outline the system or project’s boundaries along with an array of hazard and threat scenarios that could impact the system; (2) enumerate critical system functions and capabilities that must be maintained throughout a crisis or shock; (3) select indicators for each critical function and subsequently compute performance scores in each matrix cell; and (4) aggregate all cells of the matrix—if necessary— to provide an overall system resilience rating, which will provide information about the system’s ability to respond to and overcome the effects of an external shock.
The RM method can be scaled to any observable system (from local to national to international). The system can be portrayed as business, a neighbourhood community, a city, or even broader as an entire region. Each part of the matrix serves as a signal of the performance of the system’s given necessary function. Rather than figure a set of universally accepted values, the RM receives data based on local experience to find signals that have to do with the local problem. These indicators should take into account some of the necessary characteristics of resilient systems that have been proclaimed by others - modularity, dispersion, redundancy, flexibility, adaptability, resourcefulness, robustness, diversity, anticipation, and feedback response (Park et al., 2013; Frazier et al., 2010) - and take into account where each attribute is most reasonable with the system that is being observed. To act as a screening function, the RM allows for the utilisation of the most convenient and most significant data, whether it involves a numerical aspect or a qualitative aspect.
Ultimately, the resilience matrix approach offers a potential framework to compare and contrast various decision metrics from multiple disciplines that reside in the same matrix cells. In this way, such an approach will greatly assist those focused upon improving system and infrastructural resilience performance alongside those people required to prepare for and respond to emergencies. Resilience thinking allows its users to take on a holistic view of the process of bolstering systemic resilience properties by ensuring that the given system is adequately prepared for a host of potential challenges and that a variety of domain and temporal horizons are considered throughout the resilience evaluation process.
A quantitative approach: Network science
Network science approaches for resilience are rooted in the premise that resilience necessarily has a temporal dimension. Holling (2001) points out that there are two conceptual ways to characterise resilience. The first, more traditional paradigm, concentrates on stability near an equilibrium steady state, where resistance to disturbance and speed of return to the equilibrium are used to measure the property. The second paradigm emphasises conditions far from any equilibrium steady state, where instabilities can flip a system into another regime of behaviour. The former group of methods define resilience from the engineering perspective, while the latter are termed ecological resilience. What is common between both paradigms is that they look at the dynamics of a system taking place in time: engineering resilience specifically mentions “speed of return to the equilibrium”, while ecological resilience looks at a “steady state” of a system. Another common property of both paradigms is the assumption that the state of a system needs to be measured at some points in time so that it is possible to determine whether the system has returned to the original equilibrium. Finally, it is important to notice that resilience is defined with respect to a disturbance or instability. With those three prerequisites in mind, quantitative approaches to resilience characterisation investigate the evolution of a system in time both under normal conditions and under stress.
Most complex systems may be decomposed into simpler components with certain relationships between them. For example, transportation infrastructure may be represented as a set of intersections connected by roadways; global population may be mapped to a set of cities connected by airlines, railways, or roads; ecological systems can be decomposed to a set of species with food-chain relationships. In cases where such a decomposition is possible, it is often convenient to deploy methods developed in a branch of mathematics called graph theory, or network science. Network science represents a system under study as a set of points, called nodes, connected with relationships referred to as links. Dynamics of a system is then defined as a composition of individual node states, which in turn depend on their neighbouring nodes as well as on internal and external factors.
An important question is whether the approach should be threat agnostic or not. Threat agnostic approaches (Ganin et al., 2016; Linkov et al., 2018) maintain that resilience is defined regardless of a specific threat that hits the system. The rationale here is that it is often impossible to predict what hits the system, how much of a disruption will ensue, and what the likelihood of a threat scenario is. The opposite group of methods define resilience by modelling a specific threat. These methods often imply and require that a probability be assigned to each threat as well as that an algorithm be defined to model how a threat affects the network. While in the world of perfect knowledge, these approaches may offer a more realistic way to prioritise resilience-enhancing investments, they appear to conflate resilience analysis with risk analysis. Ganin et al. (2016) argue that risk and resilience analyses should be complementary, but separate, and claim that resilience analysis is, in part, motivated by the imperfect knowledge about the threat space.
Networks may be “directed” and “undirected”. In undirected networks, links do not define a node serving as an origin or a destination. Both nodes are equivalent with respect to the relationship defined by the link. An example of such a relationship is friendship in a social network. In a directed network, nodes are not equivalent with respect to the link. In a transportation network for example, a road may take traffic from point A to point B but not necessarily the other way round. Mixed networks may contain both directed and undirected links.
Another important class of networks are interconnected networks. In the case of two interconnected (coupled) networks, system nodes may be logically separated into two sets or layers corresponding to each network. Consider for example, a power distribution system and an information network controlling it. In this case, links among power nodes may represent transmission lines and links among computers may define cyber connections. Notably, computers need power to function while the power distribution is controlled by the cyber system. This interdependency may be defined with links going from nodes in one layer to nodes in the other and vice versa.
One of the most resilience-relevant problems studied in graph theory is connectivity. It is argued that the state of the system is defined by the size of the network’s largest connected component. The larger the set of connected nodes is, the better the system is able to function. Well-known classical results here are based on percolation theory, where nodes and/or links are removed at random and the connectivity of the remaining sub-network is analysed. Percolation theory establishes that the distribution of links among network nodes (degree distribution) is a key characteristic in determining network robustness (Kitsak et al., 2010; Linkov et al., 2013). Yet, percolation modelling typically results in a point estimation of connectivity, or robustness, after a random removal of a certain number of nodes or links and does not look at system dynamics. One step towards resilience quantification is to look at the stability of connectivity between nodes in multiple percolations. As nodes or links are removed at random, different disruptions disconnect different sets of nodes. Based on a graph’s degree distribution, Kitsak et al. (2010) answer the questions of what nodes will be connected in multiple percolations, what the likelihood is, and how the size of the persistently connected component changes with the number of percolations.
As we said earlier, resilience can be either defined as engineering or ecological. The next group of approaches we look at builds on the engineering definition. Specifically, those frameworks aim at modelling both a disruption and a system’s recovery process and define a performance function of the system. For example, Ganin et al. (2016) define a system’s critical functionality as “a metric of system performance set by the stakeholders, to derive an integrated measure of resilience”. Critical functionality serves as a function of time characterising the state of the system. Resilience is evaluated with respect to a class of adverse events (or potential attacks on targeted nodes or links) over a certain time interval. A control time (Kitsak et al., 2010) can be set a priori, for instance, by stakeholders or estimated as the mean time between adverse events.
Performance functions capture both the absorption and recovery resilience phases defined by the National Academy of Sciences but stop short of a giving a straightforward way to address planning and adaptation. Moreover, these approaches often need to be tailored to a specific system so that its disruption response is captured meaningfully. Finally, it is not always possible to enumerate, let alone, model, all possible disruptions in the class of adverse events considered.
Applications of performance function methods to realistic systems include studies of malware spreading in a computer network (Linkov et al., 2019), where the authors studied the trade-off between over regulation and under regulation of computer users, arguing that too many rules may result in some rules being neglected and, in fact, result in a lower resilience. Another example is epidemic modelling in a metapopulation network (Massaro et al., 2018), where it was found that travel restrictions may be harmful to a system’s resilience. Specifically, critical functionality was defined based on the number of people infected and the number of people restricted from travel. Insufficient travel restrictions were shown to only slow down the epidemics without significant changes to the final number of infections. As people movement was diminished for a longer period of time, resilience was lower than that without any restrictions.
An example of a mixed approach to resilience evaluation is given by Ganin et al. (2017). The authors quantified an urban transportation system’s efficiency through delays experienced by auto commuters under normal conditions, and resilience as additional delays ensuing from a roadways disruption. The approach borrowed from engineering resilience by allowing traffic redistribution, which may be viewed as recovery, and from ecological resilience by studying the resulting steady state equilibrium achieved by the system. The authors evaluated resilience and efficiency in 40 real urban areas in the United States. Networks were built by mapping intersections to nodes and roadways to links. The authors proposed graph theory inspired metrics to quantify traffic loads on links. Based on the loads they evaluated delays. The results demonstrated that many urban road systems that operate inefficiently under normal conditions are nevertheless resilient to disruption, whereas some more efficient cities are more fragile. The implication was that resilience, not just efficiency, should be considered explicitly in roadway project selection and justify investment opportunities related to disaster and other disruptions.
Conclusion: Making resilience useful for decision makers
Due to its relative infancy for determining risk and system robustness, no single method has been solidified as the ‘go-to’ approach for conducting resilience analysis. Generally speaking, this would be a significant limitation for the quantitatively and methodologically driven, who view standardisation as the ability of resilience to be generalised to a variety of fields and cases seamlessly. In this, proponents of standardisation are not entirely wrong, as the sheer diversity of cases in which resilience thinking is proposed requires some movement towards consistency and method objectivity. However, rather than developing and implementing a single standard for resiliency across disciplines and countries, a more effective approach would be to further a suite of methods and tools that can be utilised and modified based upon a country, company, or discipline’s institutional, political, economic, and cultural incentives and needs.
The main barrier to furthering this suite of methods is the lack of a formal definition or centralised governing body. Resilience thinking and resilience analysis possesses different meanings for different disciplines, which will only become more entrenched and divided as time passes. Should no consensus definition be reached, there still remains the possibility that some shared meaning may be accepted in different types of resilience methodologies, which require something of a shared language to convince their audience that their method’s findings are legitimate and acceptable.
For qualitative methods, tool development is a bit easier due to a reduced reliance upon strict mathematical tools and more upon the need to acquire information for an emerging topic of high uncertainty and risk. Despite the different and specified needs of various disciplines when utilising qualitative approaches to resilience thinking, the general approach expressed by most users is one of user-defined categorical metrics which are filled out by a pre-determined list of subject experts or lay stakeholders. In such an exercise, the opinions of experts serve as indicators of risk and system resilience and offer a context-rich view of resilience decision making for a particular case. In this way, qualitative methods share a common function of eliciting feedback from the world at large and processing results in a transparent and meaningful way, making qualitative methodologies inherently generalisable despite intellectual differences across disciplines.
We would argue that the main hurdle towards resilience-based method and tool development for qualitative methods centres on their overall acceptance by the quantitative community. Across various disciplines, criticisms have been levelled at qualitative methodologies’ lack of objectivity in pursuit of scientific understanding, with quantitative methods and mathematical approaches being easier to accept and verify (Mahoney and Goertz, 2006; King et al. 1994). However, we contend that as resilience is used to tackle cutting edge emerging systems and systemic threats, quantitative information may not always be available or useful to resolve a context poor situation (Ritchie et al., 2013; Trump et al., 2018). In this way, qualitative research in resilience thinking and analysis will help bridge initial gaps in risk understanding by offering an expert-driven view of a system’s resilience for a given array of external shocks and challenges.
Semi-quantitative methods may help assuage the concerns of the quantitatively driven due to the use of mixed qualitative and quantitative data in evaluating resilience decisions. Specifically, risk matrices categorise available objective data into a small set of classification factors that inform overall resilience decision making - allowing for a transparent and scientifically defensible method of conducting resilience analysis. While some information is lost in the transformation of quantitative data to qualitative categorical metrics, this method can simplify resilience-based decision making by breaking down systemic factors into a small number of easily understood subsets. Additionally, this method allows its users to integrate qualitative information and elicited expert opinion alongside available data, bringing additional context to the available dataset. This approach has a slightly steeper learning curve than traditional qualitative research methods; resilience matrices require some fundamental understanding of the math behind matrices as well as understanding of proper use (to avoid the garbage-in, garbage-out problem that haunts any decision analytical tool), which may prevent some from placing such matrices in their resilience tool kit.
Quantitative methods like network science enjoy perhaps the greatest level of trust amongst lay stakeholders due to the perception of objectivity and raw scientific explanatory power in a variety of applications. Under the assumption of correct math, lay stakeholders can physically witness the transformation of data into rigorous findings of risk and benefit and may ultimately help pave the way to notions of causality for a particular application of resilience management. Where valid data is plentiful, quantitative methods can go a long way towards advancing most fields in science, let alone resilience thinking. However, incompleteness or lack of clarity in existing data can put a damper on research, and even the mathematical method used to generate objective outcomes may in itself be inherently subjective. An additional concern includes the even steeper learning curve than qualitative or semi-quantitative methods; users are frequently tasked with mastering advanced formulas or computer programs even prior to looking at a dataset. Often, implementation of such methods will require a model to be custom-built by an external consultant or academic. This is not to discourage the use of these methods - their contribution to science is extensive and frequently proven across virtually all fields - yet we must note the drawbacks of quantitative-only approaches to resilience along with the complications that their users will face in the midst of high uncertainty and context-poor information limitations.
By its nature, resilience classification is difficult. If it were not so, there would be little need to discuss the pros and cons of differing ideologies of resilience practice. However, when used properly, these methods can do much good through their ability to inform complex and uncertain resilience analysis and decision making by providing some structure for any methodological venture (Jackson, 2018). More methods have and will undoubtedly continue to creep into the field as more disciplines come to embrace resilience thinking, making full method standardisation unlikely. Yet this may open the door for shared fundamental concepts of resilience analysis across ideological and theoretical divides. In other words, each individual discipline will transform resilience to fit its own needs, yet these methods will serve as the cornerstones of a structure that will allow resilience thinkers to have a shared philosophical discussion.
References
Adger, W. N., et al. (2005), "Social-ecological resilience to coastal disasters." Science, 309(5737), 1036-1039.
Ayyub, B. M. (2014), "Systems resilience for multihazard environments: definition, metrics, and valuation for decision making." Risk Analysis, 34(2), 340-355.
Berghel, H. (2015), "Cyber Chutzpah: The Sony Hack and the Celebration of Hyperbole." Computer 2, 77-80.
Berkes, F., and Jolly, D. (2002), “Adapting to climate change: social-ecological resilience in a Canadian western Arctic community”, Conservation Ecology, 5(2), 18.
Bjorck, F., et al. (2015), "Cyber Resilience-Fundamentals for a Definition." in New Contributions in Information Systems and Technologies, Springer International Publishing, 311-316.
Bruneau, M., et al. (2003), A framework to quantitatively assess and enhance the seismic resilience of communities. Earthquake spectra, 19(4), 733-752.
Centeno, M. A., et al. (2015), “The emergence of global systemic risk”, Annual Review of Sociology, 41,65-85.
Collier, Z., et al. (2014), "Cybersecurity standards: managing risk and creating resilience." Computer, 47(9), 70-76.
Cross, J. A. (2001), “Megacities and small towns: different perspectives on hazard vulnerability”, Glob Environ Change Part B: Environmental Hazards, 3(2), 63-80.
Cutter, S. L., Ash, K. D., and Emrich, C. T. (2014), “The geographies of community disaster resilience”, Glob Environ Change, 29, 65-77
Downey, S. et al. (2016) “European Neolithic societies showed early warning signals of population collapse”, Proceedings of the National Academy of Sciences, Aug 2016, 113 (35) 9751-9756; DOI: https://doi.org/10.1073/pnas.1602504113
Ebi, K. L., and Semenza, J. C. (2008), "Community-based adaptation to the health impacts of climate change." American Journal of Preventive Medicine, 35(5), 501-507.
Erikson, K.T. (1977), Everything in its Path, Simon and Schuster, New York https://www.simonandschuster.com/books/Everything-in-its-Path/Kai-T-Erikson/9780671240677
Eisenberg, D. A., et al. (2014), “Resilience metrics: lessons from military doctrines”, Solutions 5(5):76-87
Fox-Lent, C., Bates, M. E., and Linkov, I. (2015), “A matrix approach to community resilience assessment: an illustrative case at Rockaway Peninsula”, Environment Systems and Decisions, 35(2), 209-218.
Francis, R., and Bekera, B. (2014), "A metric and frameworks for resilience analysis of engineered and infrastructure systems." Reliability Engineering and System Safety, 121,90-103.
Ganin A. A., et al. (Dec. 2017), “Resilience and efficiency in transportation networks,” Science Advances, 3(12), e1701079.
Ganin, A. A., et al. (Jan. 2016), “Operational resilience: concepts, design and analysis,” Scientific Reports, 6, 19540.
Gao, J., et al. (2012), “Networks formed from interdependent networks”, Nature Physics, 8(1), 40.
Helbing, D. (2012), “Systemic risks in society and economics”, in Social Self-Organization (pp. 261-284), Springer, Berlin, Heidelberg.
Holling, C. S. (2001), “Understanding the complexity of economic, ecological, and social systems”, Ecosystems, 4(5), 390-405.
Jackson, P. (2018), “The New Systemic Risk: Adoption of New Technology and Reliance on Third-Party Service Providers”, Regulatory Intelligence, Thompson Reuters.
Karvetski, C. W., et al. (2011), “Climate change scenarios: Risk and impact analysis for Alaska coastal infrastructure”, International Journal of Risk Assessment and Management, 15(2-3), 258-274.
Kaur, S., S. Sharma, S., and A. Singh (2015), "Cyber Security: Attacks, Implications and Legitimations across the Globe", International Journal of Computer Applications, 114(6),
King, G., R. Keohane, and S. Verba (1994), Scientific inference in qualitative research, Princeton University Press, Princeton, NJ.
Kitsak, M., et al. (2010), “Identification of influential spreaders in complex networks”, Nature Physics, 6(11), 888.
Larkin, S., et al. (2015), “Benchmarking agency and organisational practices in resilience decision making”, Environment Systems and Decisions, 35(2), 185-195.
Linkov, I., and B.D. Trump (2019), The science and practice of resilience, Springer, Cham.
Linkov, I. and E. Moberg, E. (2011), Multi-criteria decision analysis: environmental applications and case studies, CRC Press, Boca Raton
Linkov, I. et al. (2019), “Rulemaking for Insider Threat Mitigation”, in Cyber Resilience of Systems and Networks (pp. 265-286), Springer, Cham.
Linkov, I., Trump, B. D., and Fox-Lent, K. (2016), Resilience: Approaches to risk analysis and governance. IRGC, Resource Guide on Resilience, EPFL International Risk Governance Center, Lausanne, v29-07- 2016, available at https://www.irgc.org/risk-governance/resilience/ (accessed at 10.10. 2016),
Linkov, I., B.D. Trump and J. Keisler, J. (2018), “Risk and resilience must be independently managed”, Nature, 555(7694), 30-30.
Linkov, I. et al. (2018), “Resilience at OECD: Current State and Future Directions”, IEEE Engineering Management Review, 46(4), 128-135.
Lino, C. (2014), "Cybersecurity in the federal government: Failing to maintain a secure cyber infrastructure." Bulletin of the American Society for Information Science and Technology, 41(1), 24-28.
Longstaff, P. H. (2005), "Security, resilience, and communication in unpredictable environments such as terrorism, natural disasters, and complex technology”, Center for Information Policy Research, Harvard University.
Lucas, K., O. Renn, and C. Jaeger, C. (2018), “Systemic Risks: Theory and Mathematical Modeling”, Advanced Theory and Simulations, 1(11), 1800051.
Mahoney, J., and G. Goertz (2006), "A tale of two cultures: Contrasting quantitative and qualitative research", Political Analysis, 14(3), 227-249.
Massaro, E. et al. (2018), “Resilience management during large-scale epidemic outbreaks”, Scientific reports, 8(1), 1859.
Merad, M., and B.D. Trump, (2020), Expertise Under Scrutiny: 21st Century Decision Making for Environmental Health and Safety, Springer International Publications, Cham
Murray, P., and K. Michael, K. (2014), "What are the downsides of the government storing metadata for up to 2 years?", 6PR 882 NewsTalk: Drive Aug. 2014.
National Academy of Sciences (NAS) Committee on Science, Engineering, and Public Policy. (2012), “Disaster Resilience: A National Imperative,” The National Academies Press, Washington DC.
Osawa, J. (2011), "As Sony counts hacking costs, analysts see billion-dollar repair bill", The Wall Street Journal, 9 May 2011.
Pelling, M. (2012), “Resilience and transformation. Climate Change and the Crisis of Capitalism: A Chance to Reclaim Self”, Society and Nature, 51-65.
Petrie, C., and V. Roth, V. (2015), "How Badly Do You Want Privacy?" IEEE Internet Computing, 2, 92‑94.
Renn, O. (2016), Inclusive resilience: A new approach to risk governance”, International Risk Governance Center, IRGC.
Ritchie, J. et al. (eds.) (2013), Qualitative research practice: A guide for social science students and researchers, Sage Publications, London.
Trump, B.D., M.V. Florin, and I. Linkov (2018), IRGC Resource Guide on Resilience Volume 2, International Risk Governance Center (IRGC),
Turchin, P. (2015), Ultrasociety, Beresta Books, Chaplin CT.
Vugrin, E.D., D.E. Warren and M.A. Ehlen (2011), “A resilience assessment framework for infrastructure and economic systems: Quantitative and qualitative resilience analysis of petrochemical supply chains to a hurricane”, Process Safety Progress, 30(3), 280-290.
Williams, P.A., and R.J. Manheke (2010), “Small Business: A Cyber Resilience Vulnerability”, Proceedings of the 1st International Cyber Resilience Conference, Edith Cowan University, Perth Western Australia, 23 August 2010.
Wood, M., et al. (2012), “Flood risk management: US Army Corps of Engineers and layperson perceptions”, Risk Analysis, 32(8), 1349-1368.
Yatsalo, B. et al. (2016), “Multi-criteria risk management with the use of DecernsMCDA: methods and case studies”, Environment Systems and Decisions, 36(3), 266276.
Zhao, J. J., and Zhao, S. Y. (2010), "Opportunities and threats: A security assessment of state e-government websites" Government Information Quarterly, 27(1), 49-56.
Zigrand, J-P. (2015), How Can We Control Systemic Risk? World Economic Forum, 10 August 2015 https://www.weforum.org/agenda/2015/08/how-can-we-control-systemic-risk/