This chapter shows that productivity levels in Core Cities are low compared to the UK average and to other second-tier cities in OECD countries. Based on an analysis of more than 3.5 million records of workers, it identifies the factors responsible for lagging productivity. It finds that productivity would increase by 7.1 percentage points if the profile of the workforce and the sector composition were to adjust to the current UK average. If Core Cities were to generate agglomeration economies according to their potential, productivity would increase by another 4.1 percentage points.
Enhancing Productivity in UK Core Cities
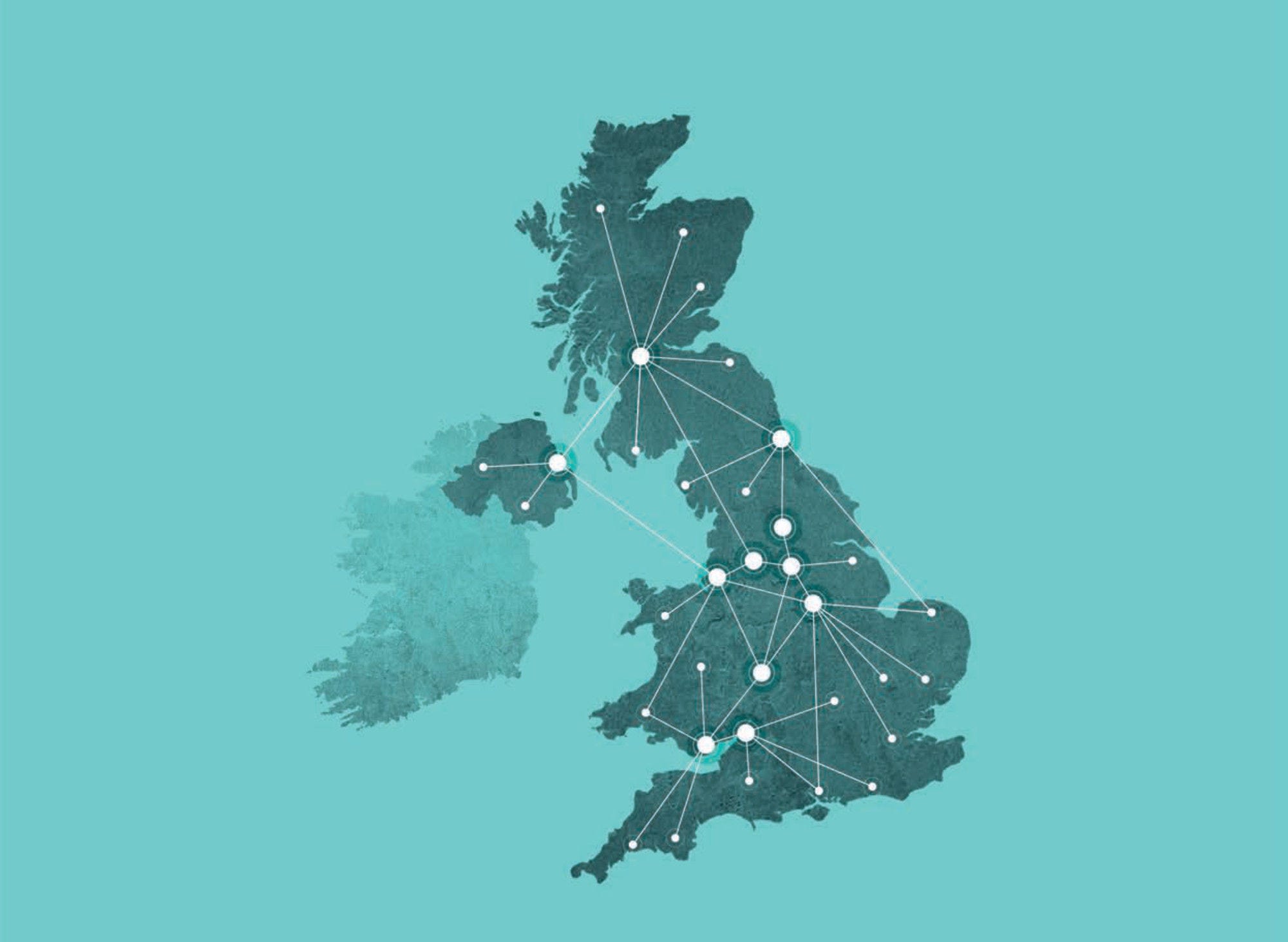
2. Productivity in Core Cities
Abstract
The UK productivity puzzle
The level of labour productivity is one of the most important determinants of long-term economic growth as it measures the output that an economy is capable of producing with its existing resources. It reflects an economy’s ability to produce more output by better combining inputs, developing new ideas and improving business models. Because there is only a finite number of workers in an economy, increasing labour productivity is the only possibility to increase output and living standards in the long term. Likewise, the large differences in per capita income across the world are largely the consequence of differences in labour productivity.
Thus, it is of little surprise that the recent productivity slowdown in the United Kingdom (UK) has sparked widespread interest. Since the onset of the 2007-08 financial crisis, labour productivity growth in the United Kingdom has been exceptionally weak. In 2018, the productivity level was just 2.5% higher than in 2008 and remained well below the level implied by a simple continuation of its pre-crisis trend. The weak performance has been called the “productivity puzzle” and has caught the interest of both academics and policymakers.
Figure 2.1. Productivity growth in the UK has slowed down after the financial crisis

Source: OECD calculations based on OECD (2019[1]), OECD Productivity Statistics (database) (accessed August 2019).
While productivity growth has slowed down across OECD countries, the decline in growth was particularly severe in the UK. As a consequence, differences in productivity levels between the UK and comparable economies has widened in recent years. Whereas productivity levels in France and Germany were approximately 9% higher than in the UK in 2008, this gap has increased to 15%-16% in 2018. Compared to the United States, the difference has been growing from 14% to 23% (Figure 2.2).
No consensus exists regarding the causes of the productivity slowdown. Explanations range from a decline in productivity-enhancing investment to an increasing dispersion in productivity levels between high-productivity firms and low-productivity firms. Some researchers even argue that the measurement error is to blame for indicators that show declining productivity (Box 2.1). According to this view, the benefits of new technologies, such as the Internet, cannot fully be measured and are therefore not adequately reflected in productivity statistics. However, only a small minority of researchers attributes the UK productivity puzzle entirely to measurement error.
Figure 2.2. The productivity gap between the UK and other countries has been widening
Productivity levels

Note: Labour productivity refers to real gross domestic product (GDP) in USD constant prices and purchasing power parities (PPP) per total hours worked.
Source: OECD calculations based on OECD (2019[1]), OECD Productivity Statistics (database) (accessed August 2019).
Box 2.1. Explaining the productivity puzzle
The weak productivity performance in the UK since the crisis has been extensively debated and analysed over recent years. Although many explanations have been put forth in the literature, the three main arguments can be summarised as follows:
1. Drop in investments: An important explanation is related to a decrease in investment. During the crisis, overall demand decreased, which reduced the real wages paid to the workers. The decrease in the labour costs and the increase in the costs of capital incited firms to reduce their capital investments (i.e. machinery, real estate, software) and increase the number of employed workers. The substitution of capital with labour in the production reduced the capital-labour ratio, thus generating a decrease in the productivity per worker (Pessoa and Van Reenen, 2014[2]). According to Bartnett et al. (2014[3]), the reduced investment in physical and intangible capital can explain one-third of the productivity gap in the UK.
2. Dispersion in firm-level productivity: There are substantial differences in the productivity levels across the firms in the UK. While a small group of firms are performing better than most other firms in Europe (i.e. the productivity frontier), most firms have low levels of productivity and growth (i.e. the laggards) (Haldane, 2018[4]). During the crisis, the distribution of productivity across firms widened as the lagging firms dropped further behind (Andrews, Criscuolo and Gal, 2016[5]). One of the explanations put forth is the breakdown of the so-called innovation “diffusion machine” (OECD, 2015[6]). According to this argument, top firms (or those at the productivity “frontier”) have continued to innovate and increase their productivity. The productive knowledge generated in these firms, however, has stopped diffusing to other less productive firms generating productivity differences within countries and industries.
3. Mismeasurement: One important explanation put forth is the difficulty in the measurement of productivity in the current economic structure. Proponents of this explanation argue that official statistics seem to underestimate economic activity (Feldstein, 2016[7]; Baily and Montalbano, 2016[8]). In the UK, estimates suggest that productivity growth could be up to 0.5 percentage points per year higher than indicated by official statistics, because of a failure to capture productivity growth in the digital economy (Haldane, 2018[4]). However, the bulk of the evidence suggests that even if there is some mismeasurement, it could only explain a small part of the productivity puzzle (Barnett et al., 2014[3]).
Low levels of productivity in the UK are sometimes attributed to its high employment rate. According to this line of reasoning, a higher rate of employment implies that more workers with low levels of productivity are employed instead of being unemployment. This drags down the average productivity levels. While such an effect can occur in principle, its quantitative magnitude is too small to play an important role in explaining productivity differences between the UK and other countries. Moreover, the productivity gap exists also relative to countries that have higher employment rates, such as Germany.
Source: Pessoa, J. and J. Van Reenen (2014[2]), “The UK Productivity and Jobs Puzzle: Does the Answer Lie in Wage Flexibility?”, http://dx.doi.org/10.1111/ecoj.12146; Barnett, A. et al. (2014), “The UK Productivity Puzzle”, Bank of England Quarterly Bulletin 2014; Andrews, D., C. Criscuolo and P. Gal (2016[5]), “The Best versus the Rest: The Global Productivity Slowdown, Divergence across Firms and the Role of Public Policy”, https://doi.org/10.1787/63629cc9-en; OECD (2015[6]), The Future of Productivity, https://doi.org/10.1787/9789264248533-en; Feldstein, M. (2016[7]), “Remarks at the Brookings Institution conference on productivity”, https://www.brookings.edu/wp-content/uploads/2016/08/feldstein-remarks.pdf; Baily, M. and N. Montalbano (2016[8]), Why Is US Productivity Growth So Slow? Possible Explanations and Policy Responses, https://www.brookings.edu/research/why-is-us-productivity-growth-so-slow-possible-explanations-and-policy-responses/ (accessed on 14 September 2019); Haldane, A. (2018[4]), “The UK’s productivity problem: Hub no spokes”, https://www.bankofengland.co.uk/-/media/boe/files/speech/2018/the-uks-productivity-problem-hub-no-spokes-speech-by-andy-haldane.
Weak average productivity outside of London holds back the aggregate productivity in the UK
Large productivity differences across the regions in the UK are another unusual element in the productivity puzzle. Although regional productivity gaps exist across the OECD countries, the levels of disparity between the most and the least productive regions are one of the highest among the OECD countries (OECD, 2017[9]; UK2070 Commission, 2019[10]). However, these regional differences in productivity are essentially driven by London, with the second-best performing region being above but close to the UK average. Most UK regions have below-average productivity performance. These regional disparities have been increasing since the early 2000s and have further steepened since the global financial crisis (Figure 2.3) (OECD, 2015[11]; 2017[9]). This widening of the productivity gap was also observed in one-third of OECD countries where growth in the aftermath of the financial crisis has been concentrated in a single, already highly productive regions (OECD, 2019[12]). Still, considering the UK context, continued divergence is surprising as the financial crisis could have been expected to affect in particular London-based firms in the financial sector.
Core Cities play a major role within the UK economy and they are important economic hubs for their surrounding regions. However, their productivity performance shows that they do not play this role to the extent that they could. Productivity levels in Core Cities are at or below the UK average, which is unsatisfactory for two reasons.1 First, their performance at or below the national average is worse than those of large cities in other countries, which tend to outperform their national averages. While second-tier cities in most other large OECD countries have productivity levels that are as high as or higher than the national average, GVA per worker in Core Cities is just 87% the UK average. This is the worst performance of second-tier cities among all OECD countries with at least 10 second-tier cities above 250 inhabitants (Figure 2.4).
Figure 2.3. The regional productivity gap is increasing
The gap in labour productivity between the top and bottom 20% of TL2 regions, real gross value added (GVA) per worker, in constant USD PPP

Note: Gross value added (GVA) per worker is expressed at constant prices (the year 2010) in USD purchasing power parities (PPPs). The labour productivity gap between the top and the bottom 20% of TL2 regions is calculated based on the unweighted average of the respective regions.
Source: OECD calculations based on OECD (2019[13]), OECD Regional Statistics (database) (accessed August 2019).
Second, in an international context, the performance around the national average is made worse by the fact that the UK is performing well below comparable countries in terms of productivity growth and productivity levels. Therefore, the productivity gap between Core Cities and comparable second-tier cities in other countries is even larger than their productivity gap relative to the UK average. Average GVA per worker in Core Cities is USD 69 370 (in PPP). In contrast, the average GVA per worker is 30.4% higher in Australia (USD 90 447), 30.3% higher in Germany (USD 90 418), 26.1% higher in the Netherlands (USD 87 454), 22.8% higher in France (USD 85 172) and 17.9% higher in Italy (USD 81 802). Taken together, this implies that Core Cities are not contributing to the UK economy as much as they could.
Figure 2.4. GVA per worker relative to the country average is low in Core Cities
GVA per worker in second-tier cities relative to the national average, 2016

Note: Average GVA per worker in the 10 largest functional urban areas (FUAs) outside of the largest FUA of a country relative to the national average. Only countries with 11 or more FUAs with more than 250 000 inhabitants are shown.
Source: OECD calculations based on OECD (2019[13]), OECD Regional Statistics (database) (accessed August 2019).
Figure 2.5. Productivity levels in Core Cities are low by international standards
GVA per worker of second-tier cities, 2016

Note: Figure plots the average labour productivity (measured by GVA per worker in USD PPP) in second-tier cities relative to average productivity across Core Cities. Largest cities outside of the capital, for which data is available, are considered as second-tier cities. Data refers to functional urban areas (FUAs).
Source: OECD calculations based on OECD (2019[13]), OECD Regional Statistics (database) (accessed August 2019).
Box 2.2. Measuring productivity
How to measure productivity?
Productivity is the efficiency with which firms convert inputs (labour, capital and raw materials) into outputs. When productivity increases, it allows increasing the output faster than the inputs. Although there are a number of ways to measure productivity, the two most commonly used productivity measures are labour productivity and total factor productivity (TFP) (Australian Government Productivity Commission, 2015[14]):
1. Labour productivity: Measures the growth in output per unit of labour used or wages.
a. Output per worker: One way of measuring productivity is dividing the total output by the number of workers involved in the production. Total value added or volume of production can also be used alternatively, depending on the data at hand.
b. Nominal wages: Standard microeconomic theory suggests a clear relationship between wage growth and productivity growth in the short run (Borjas, 2012[15]). Typically, it is assumed that the firm’s capital stock is constant in the short run. When a firm’s output increases, given that capital stock is fixed, the marginal productivity of labour increases. If productivity per unit of labour input (or per worker) increases, while wages remain constant, this will increase labour demand because a further extension of production will increase profits. Given a fixed labour supply, the increased labour demand would result in higher pay, until a new profit-maximising equilibrium is reached at which wages again equal marginal productivity. Thus, a worker’s wage is equivalent to the marginal product of his/her labour, thus productivity.
Due to the difficulties of allocating GVA to the local level, local labour productivity estimates based on individual wages are considered more reliable than estimates based on GVA per worker. While this report uses primarily estimates based on nominal wages, estimates based on GVA per worker are used for international comparisons. Both measures show the same picture but deviate slightly from each other. These discrepancies are due to the abovementioned methodological differences and do not affect the general conclusions of the analysis.
2. Total factor productivity (TFP): Productivity can also be measured at the firm level as value-added output (real gross output less intermediate inputs). Using firm-level data on output, labour and capital, one can recover the role of TFP, the sum of factors that contribute to the production net of labour and capital inputs.
Measuring agglomeration economies: Wages vs. TFP
Both nominal wages and TFP can be used to measure productivity gains associated with agglomeration economies. However, the use of TFP for estimating agglomeration is problematic for multiple reasons. First, most of the firm-level data rely on surveys that are limited by the sample size, which is especially problematic for small size firms that are in most cases underrepresented. Second, the correct estimation of TFP requires precise data on certain variables such as value-added and intermediate inputs, which are, in many cases, unavailable (Gal, 2013[16]). Third, output, labour and other inputs that are used for TFP estimation are simultaneously determined by the firm, which causes a reverse causality issue that can potentially bias the estimated coefficient obtained from ordinary least squares (OLS).
With these caveats in mind, it is possible to use TFP to estimate agglomeration economies, although the economic interpretation of the elasticities obtained on the impact of local characteristics is slightly nuanced compared to those obtained when using wages. Following the standard established in the academic literature, this report uses wages as a measure of productivity as it provides a more precise estimation even for small geographical units and allows for controlling for individual sorting.
Source: (Australian Government Productivity Commission (2015[14]), “What is productivity and how is it measured?”, PC Insight 05/2015; Borjas, G. (2012[15]), Labor Economics, Higher Education, McGraw-Hill; Gal, P. (2013[16]), “Measuring Total Factor Productivity at the Firm Level using OECD-ORBIS”, https://doi.org/10.1787/5k46dsb25ls6-en.
Productivity levels have been diverging across Core Cities
While Core Cities as a group have GVA per worker levels at or just below the national average, there are important differences among them. Figure 2.6 compares Core Cities with the national average. GVA per worker levels across Core Cities range from 84% of the national average in Sheffield to 108% of the national average in Bristol. For comparison, GVA per worker in London is 144% of the national average.
Figure 2.6. Core Cities differ in their productivity levels and growth rates
Relative GVA per worker levels in 2016 and growth rate 2001-16

Note: Left axis is the GVA per worker of each Core City relative to the national average in 2016. Right axis is the annual growth rate in GVA per worker between 2001 and 2016, relative to national average growth in GVA per worker. Data refers to functional urban areas (FUAs).
Source: OECD calculations based on OECD (2019[13]), OECD Regional Statistics (database) (accessed August 2019).
Not surprisingly, cities with a higher GVA per worker in 2016 also had higher growth rates in GVA per worker during the period 2001-16 (Figure 2.6, right axis). These higher growth rates are one of the reasons why cities on the right-hand side of the chart have higher productivity levels. However, there is also evidence that cities with higher levels of GVA per worker in 2001 had higher growth rates subsequently. This nuance is important because the latter result indicates that there has been a divergence in productivity levels across Core Cities. It implies that between 2001 and 2016 the relatively more productive cities further increased their productivity advantage relative to the less productive cities.
Evidence for diverging growth rates can be found in Figure 2.7. It plots GVA per worker in Core Cities in 2001 and 2016 and shows an estimated regression line between the GVA per worker in those two years. If GVA per worker was growing at the same rate in all cities, the slope of the regression line would be 1. However, the estimated slope is 1.12, which indicates that cities with higher GVA per worker levels in 2001 grew faster subsequently.
Figure 2.7. Cities with higher GVA per worker in 2001 experienced faster subsequent growth
GVA per worker in 2001 and 2016, in USD PPP, 2010 prices

Note: Figure plots the annual GVA per worker in 2001 and 2016. Left-axis labour productivity (in USD) measured as GDP per worker in 2016, right-axis labour productivity growth between 2001 and 2016. Data refers to functional urban areas (FUAs).
Source: OECD calculations based on OECD (2019[13]), OECD Regional Statistics (database) (accessed August 2019).
Understanding the factors that drive productivity in cities
Cities are drivers of productivity growth
Cities play an important role in determining a country’s productivity. Across the OECD, metropolitan areas – urban agglomerations with more than half a million inhabitants – are home to over half of the population of OECD member countries and account for an even larger share of total GDP (OECD, 2015[17]). Thus, any change in productivity in cities will have important effects on aggregate productivity levels in a country.
Cities also matter for productivity growth for reasons beyond their sheer size. Urban areas are engines of innovation where new ideas are developed and tried out. This role of cities as innovation hubs is reflected in productivity levels in cities, which are usually significantly above the country average. Moreover, as new innovations eventually spread out from cities to the rest of the country, innovative activity in cities also benefits productivity in towns and rural areas.
Yet, it is not guaranteed that cities can play their role as drivers of productivity. Whether they are able to do so depends on a variety of circumstances. Among other factors, cities need high levels of human capital level in the workforce, the right sectoral composition of the economy, appropriate levels of infrastructure, effective public administrations, close links between businesses, schools and universities, and the right governance arrangements to align all actors in the policy areas. Moreover, cities are affected by a large number of national policies and regulations that are not targeted at cities specifically, but that have important consequences on the functioning of cities. This includes labour market regulations, fiscal policies (including tax and investment policies), environmental regulations and education policies.
To better understand the reasons for the observed pattern of low productivity in Core Cities, it is necessary to distinguish different factors that determine productivity. Three key factors can be identified; first, the skill level of the local workforce and the sectoral composition of the local economy, second, agglomeration economies; and third, place-specific factors related to local policy choices as well as natural characteristics, such as local geography. These factors are interconnected and have a complex structure, which makes it difficult but not impossible to identify each individual contribution.
Skills and industry composition matter for productivity
Skill levels of the workforce are among the most important determinants of a city’s productivity. Human capital not only has strong direct effects on a worker’s productivity. It also has positive spill-over effects on co-workers and others in his or her proximity.
Individual characteristics, such as age, education or experience, alone explain 57% of the variations in productivity across UK cities. The fact that individual characteristics matter for wages and productivity is now well documented for the UK (D’Costa and Overman, 2014[18]; Gibbons, Overman and Pelkonen, 2014[19]) and other developed countries such as France (Combes, Duranton and Gobillon, 2008[20]) or the US (Baum-Snow and Pavan, 2012[21]). Training, retaining and attracting skills in cities is an essential policy tool for boosting productivity levels in cities.
Skill composition in a city also matters for the industry structure of the local economy. Within the national economies, industries are unevenly distributed across regions and cities. Given that each industry requires workers with different skill sets, the demand for skills and types of jobs available in the local economy will also vary between locations. As a consequence, the average productivity in a city will vary depending on the type of skills demanded by the local industries. Industry composition matters because emerging evidence suggests that productivity growth in the UK has been concentrated in a few sectors (Riley, Rincon-Aznar and Samek, 2018[22]). Despite this evidence, further analysis is needed to get a comprehensive understanding of the influence of sector composition on productivity.
Larger and denser cities have a higher share of skill-intensive industries. If high-skilled workers are more likely to move into larger cities, then the share of skilled workers and thus the average productivity in these locations will be higher compared to smaller or less dense areas. Moreover, high-skilled workers may prefer living in larger cities as they offer more cultural amenities. If high-skilled workers value such amenities more than other skill groups, they would be more likely to live in large cities, thus increasing productivity in these areas.
Agglomeration economies
Another determinant of the productivity differences across cities is the agglomeration economies, the productivity gains that arise due to interactions between workers or firms that take place locally. Economist Alfred Marshall (1890[23]) was among the first to emphasise that the agglomeration of people and firms can increase productivity. In basic terms, agglomeration economies imply that a given worker in a given job will be more productive if he or she does the job in a large city rather than in a rural area.2 Thus, agglomeration economies are distinct from the productivity gains described above that occur because cities are home to higher-skilled workers and more productive industries.
Since the work by Marshall, considerable effort has been spent on identifying the mechanisms through which agglomeration economies emerge. While not all aspects related to the emergence of agglomeration economies are fully understood, three main mechanisms have been identified (see Box 2.3 for details). First, infrastructure and other inputs in the production process are more effectively shared among many users in cities than in rural areas. Second, because of the larger number of firms and workers in cities, workers are able to find jobs that are a better match for the skills that they possess. Third, innovations are generated and spread around at a faster pace in cities than in rural areas.
Local institutions, infrastructure and geography
Cities still have important differences in terms of productivity levels once the effects of skill levels, sectoral composition and agglomeration economies have been taken into account. These differences are due to several factors, some of which are man-made and some of which are due to natural endowments and geography. Natural advantages include access to a shore, temperate climate and strategic locations along trade routes can all contribute to higher productivity in a city.
There is an even broader range of man-made factors. They include infrastructures such as airports, highways and fast Internet connections. Effective urban planning that ensures compact and mixed-use developments to encourage the spread of ideas, but does not prevent urban growth also plays a role. The quality of local institutions, including whether public administrations that process requests from firms and residents quickly and efficiently matters strongly, too. Institutional quality can also determine, for example, whether the public sector, universities and businesses co‑operate well. Even the quality of strategic industries can play a major role. For example, a well-functioning local banking industry catering to local firms can provide a major advantage to highly productive firms in a city that is trying to scale up its operations.
The list above is by no means an exhaustive list of factors that influence productivity at the city level. Moreover, the way these policies affect productivity is complex and might work through some of the channels above. For example, better co‑operation between businesses and universities can have direct effects of productivity levels in a city and it might also have indirect effects because it attracts a larger number of high-skilled workers to a city.
Box 2.3. What are the agglomeration economies?
The mechanisms that create agglomeration economies can be broadly split into three groups: sharing, matching and learning. The discussion below follows Duranton and Puga’s contribution to the Handbook of Regional and Urban Economics (2004[24]) and builds on a long history of research, with early discussion of the concept of agglomeration benefits ranging back to the 19th-century economist Alfred Marshall and his “Principles of Economics” (Duranton and Puga, 2004[24]; Marshall, 2009[25]).
Sharing: Sharing of facilities or inputs by a large number of firms is one way of creating a critical mass for certain goods or facilities that require many beneficiaries to be efficient. For example, branching a river to provide a constant stream of fresh water for an industrial site involves large fixed costs that are only worth paying if there are enough firms benefitting from this investment. A similar argument applies to the provision of specialised goods and services. Such specialisation creates productivity gains but also requires a large enough demand to sustain the business model.
Matching: Larger labour markets result in better matches between employers and employees. A better match means that the person who is hired for a job is better suited for his or her position and hence more productive. Most people tend to look for jobs primarily within their city. In larger cities, they have more choice between different potential employers and are more likely to find a matching one.
Learning: Another cause that is often considered to be relevant is the so-called technology spill-overs. Businesses tend to learn from other nearby located businesses about the latest production methods. In larger cities, more businesses that are similar to each other exist. Therefore, there are more opportunities for them, e.g. enforced by labour market mobility, to learn about the most efficient production methods and to adapt accordingly.
In addition to these main mechanisms, another argument for the emergence of agglomeration economies is related to increased innovation that occurs when existing ideas and concepts are combined into new products and processes. While innovation can happen anywhere, it occurs predominantly in highly urbanised areas (Carlino and Kerr, 2015[26]). Lastly, cites might also be more productive because a larger number of businesses can increase the level of competition within a city. Fiercer competition ensures that unproductive businesses leave the market, which increases the average level of productivity within a city and raises its GDP.
Source: OECD (2015[17]), The Metropolitan Century: Understanding Urbanisation and its Consequences https://dx.doi.org/10.1787/9789264228733-en; Duranton, G. and D. Puga (2004[24]), “Micro-foundations of urban agglomeration economies”, in: Henderson, J.V. and J.F. Thisse (eds.), Handbook of Regional and Urban Economics, Vol. 4, Ch. 48, pp. 2063-2177; Marshall, A. (2009[25]), Principles of Economics: Unabridged Eight Edition, Cosimo, Inc; Carlino, G. and W. Kerr (2015[26]), Agglomeration and Innovation, http://dx.doi.org/10.1016/B978-0-444-59517-1.00006-4.
Productivity in Core Cities: An econometric analysis
Economists are especially interested in separating the influence of the workforce and firm composition from other factors. The idea behind this approach is to distinguish between factors that are mobile and can move from one city to another (i.e. firms and workers) and factors that are immobile and thus inherently associated to a particular city. From a policy perspective, such a distinction is important, too. While cities can increase their productivity levels by attracting highly productive firms and workers from other cities, policies to do so lead to a zero-sum competition between them. Thus, greater emphasis should be placed on policies that lead to an aggregate growth of productivity, such as strengthening education systems, improving local economic development policies, investing in infrastructure and making public administrations more efficient.
This section describes the results of an econometric analysis of more than 3.5 million individual records of workers over a span of 17 years. It provides the following insights:
First, it shows levels of productivity in cities that are due to their inherent characteristics. For example, the average productivity in banking, finance and business services industry in the UK is three times more productive than wholesale and retail trade or six times more productive than accommodation and food service activities. If there are two cities, similar in every sense except that for historical reasons one is specialised in financial services while the other in wholesale and retail, their average productivity levels would be different due to structural differences between these industries. The econometric analysis in this section can account for these differences and can provide insights on how city-specific characteristics drive productivity once these differences in productivity are factored out.
Second, the resulting estimates for all cities can be used to determine the magnitude of agglomeration economies in the UK. In other words, they provide insights into whether cities inherently become more productive as they grow in size. As discussed below in more detail, such a positive relation between city size and productivity exists in the UK but it is much weaker than in most other developed countries. Most economists would expect agglomeration economies to occur naturally in well-functioning cities. This raises the question of which factors prevent their emergence in the UK and how policy can address these factors. Third, estimates can also be used to analyse the influence of other factors, such as infrastructure provision, market access as well as any other city-specific characteristics or policies for which sufficient data at the city level exist.
Box 2.4. The advantages of working with microdata
The analysis in this chapter aims to quantify productivity levels across UK cities net of the effects of skill levels and sectoral composition in a city. For this purpose, the analysis covers 3.5 million observations of worker records and applies a two-step estimation approach (see Box 2.5). The use of such microdata has multiple advantages for identifying the sources of productivity differences compared to using aggregate data.
First, microdata allows quantifying the productivity levels that are net of compositional issues within industries and firms. As discussed above, differences in productivity across locations are driven by differences in the skill and occupational composition of the workers and the industrial structure of the local economy. Even within an industry, there are differences due to spatial divisions of production and labour. A company could locate its managers and professionals in large cities, such as London, whereas the workers undertaking routine production or delivering routine services are more prevalent in smaller cities. Although the same firm within the same industry would be operating in both locations, the value added of each plant and their average productivities will be different.
Moreover, individual workers have unobservable characteristics, such as their level of ambition. It cannot be ruled out that more ambitious workers move more frequently to some cities than to others. In such a case, workers in these cities might be on average more productive even though the inherent characteristics of these cities are not different from other cities. By using individual-level microdata, econometric analysis can account of this effect and net it out of city-specific productivity estimates.
Second, the use of microdata can address issues related to the measurement of productivity. Not all workers work the same number of hours. Workers can be working full-time or part-time jobs. Given that workers located in large cities are less likely to hold part-time jobs than workers who are located in smaller areas, the average productivity should be estimated while accounting for the differences in the hours worked. Again, if part-time work is more common in some cities than in others, this could distort productivity measurements that can be avoided by using microdata.
The remaining productivity differences across cities that captured once the factors above have been netted out can be driven by agglomeration economies but also by multiple other factors that affect the efficient functioning of a city. For example, an efficient urban infrastructure would reduce the cost of congestion and allow workers to find jobs that match their skills better (Puga, 2010[27]). The role of local governance and governmental fragmentation can also matter for productivity. For instance, Ahrend et al. (2017[28]) show that cities with a fragmented governance structure suffer from inefficiencies and are less productive. Similarly, workers in polluted cities are more likely to become ill and take sick leave more often, which also reduces productivity.
Source: Puga, D. (2010[27]), “The magnitude and causes of agglomeration economies”, Journal of Regional Science, Vol. 50/1, pp. 203‑219.; Ahrend, R. et al. (2017[28]), “What makes cities more productive? Evidence from five OECD countries on the role of urban governance”, Journal of Regional Science, Vol. 57/3, pp. 385-410.
Box 2.5. Empirical strategy
The analysis in this chapter uses individual-level microdata and applies a two-step estimation approach that has been used by other studies in the literature to estimate agglomeration economies (Ahrend et al., 2017[28]; Combes et al., 2019[29]; Combes, Duranton and Gobillon, 2008[20]; De la Roca and Puga, 2017[30]).
In the first step, the OECD functional urban area definition of cities is matched with large-scale survey-based microdata from the UK. The resulting data set is then used to estimate productivity differentials – net of individual skill and other individual-level observables (e.g. gender, age, occupation, full-time…) and industry composition – across cities using an OLS regression of the natural logarithm of wages on individual-level characteristics and a set of fixed effects.
denotes the natural logarithm of wages for individual 𝑖 in city 𝑎 at time 𝑡, 𝑋 a vector of individual observable characteristics, 𝑑 a vector of dummy variables that take the value 1 if the individual resides in the city 𝑎 at time 𝑡. is a sector fixed-effect that absorbs structural productivity differences between industries, is individual fixed effect which allows controlling for the “sorting bias” where individuals with higher unobservable abilities (e.g. higher levels of motivation) sort into larger cities. 𝜀 denotes the error term.
The city-year fixed effects () obtained in the first step capture productivity differential across cities, net of (observable and unobservable) skill differences and the industry structure of the local economy. The estimated productivity differentials () are used as the dependent variable in the second step, in which they are regressed on time-varying city characteristics (𝑄𝑎𝑡) such as population, the share of university graduates, the Herfindahl Index (two-digit SIC2003) and the various industrial shares. Additional year fixed effects control for national business cycles and country-specific inflation (the first step estimates nominal productivity differentials).
The standard errors in the OLS estimation are clustered at the city level to allow for heteroscedasticity and arbitrary autocorrelation over time (for each city) in the error term.
Data
The estimation of the first step is based on data from the UK Annual Survey of Hours and Earnings (ASHE) for 2000-18. The ASHE is the largest labour market survey and includes information on approximately 160 000 employees per year. It is a random sample of around 1% of the National Insurance pool, as it tracks employees whose national insurance ends with a specific pair of digits. ASHE provides detailed information on an individual’s earnings, hours worked, occupation, industry, whether the job is in the private or public sector, the worker’s age and gender. Information on education is not available via ASHE and thus it is imputed using the UK Quarterly Labour Force Survey for 2000‑17. Specifically, an individual’s years of schooling in ASHE are simulated using estimates of the coefficients of the best linear predictor of education from the Labour Force Survey over the same period. The final sample used in the estimation includes 3.5 million individual-year observations working across 96 FUAs.
Source: Ahrend, R. et al. (2017[28]), “What makes cities more productive? Evidence from five OECD countries on the role of urban governance”, Journal of Regional Science, Vol. 57/3, pp. 385-410; Combes, P. et al. (2019[29]), “Unequal migration and urbanisation gains in China”, http://dx.doi.org/10.1016/j.jdeveco.2019.01.009; Combes, P., G. Duranton and L. Gobillon (2008[20]), “Spatial wage disparities: Sorting matters!”, http://dx.doi.org/10.1016/j.jue.2007.04.004; De la Roca, J. and D. Puga (2017[30]), “Learning by working in big cities”, http://dx.doi.org/10.1093/restud/rdw031.
Core Cities generate agglomeration economies below their potential
Figure 2.8 plots the relationship between city size on the horizontal axis and productivity levels relative to the national average net of the effects of the workforce and sectoral composition for 2018.3 An important pattern in Figure 2.8 is the absence of any strong relationship between city size and productivity. The line depicting the estimated relationship has a slope of just 0.009 in 2018. Thus, there is very little evidence of agglomeration economies occurring in the UK. Compared to other OECD countries, this estimated relationship between city size and productivity is similar to 0.01 for Italy (Mion and Naticchioni, 2009[31]), but lower than 0.021 found for the Netherlands (Groot and de Groot, 2014[32]), 0.025 found for Spain (De la Roca and Puga, 2017[30]), or 0.03 for France (Combes, Duranton and Gobillon, 2008[20]) and Germany (Hirsch et al., 2019[33]).
Figure 2.8. Agglomeration economies in the UK, 2018
Productivity differences (net of the workforce and sectoral composition, 2018) and city size (2018)

Note: Hollow diamonds denote Core Cities, solid diamond denote other UK cities. The horizontal axis plots city population (log scale). The vertical axis plots productivity differences from the national average net of workforce and sector effects. The analysis is conducted at the functional urban area level. Estimates for Belfast come from econometric analysis using aggregate data, for details see endnote 3.
Source: OECD calculations based on the UK Annual Survey of Hours and Earnings (ASHE) microdata. Estimates for Belfast are based on aggregate data from National Official Labour Market Statistics (NOMIS[34]) and Northern Ireland Statistics and Research Agency (NISRA[35]) data (accessed August 2019).
It is important to stress that a considerable degree of uncertainty concerning the exact magnitude of agglomeration economies still exists despite the large number of studies on the topic. Largely, this is due to an ongoing academic debate on best methodological choices for estimating agglomeration economies. For example, estimates that are based on TFP measures of productivity (see Box 4.2) tend to produce larger agglomeration economies estimates than those based on wages (Melo, Graham and Noland, 2009[36]). Yet, despite these uncertainties, the abovementioned picture of not fully realised agglomeration economies in the UK is robust. All studies cited above use the same methodology as the analysis in this report, which ensures that differences in point estimates across countries are not driven by methodological differences. Moreover, the standard deviations of the point estimates are generally small (0.0021 in case of the UK). Thus, differences across countries are not due to statistical noise, either.
One possible explanation for the weak relationship between city size and productivity could be the high productivity levels of small cities in the South East which are close to London (shown on the top left side of Figure 2.8). Indeed, these cities benefit from their proximity to London, which generates positive productivity spill-overs (see the section on linking cities and regions for more details). However, if London and all the cities that are within 120 minutes driving distance from the centre of London are excluded,4 the estimated coefficient changes only very slightly from 0.009 to 0.01, a difference that is statistically insignificant.
Another notable pattern in Figure 2.8 is close proximity of Core Cities’ productivity to the national average once workforce and sector effects have been taken into account. This implies that a large part of the variation in productivity across Core Cities can be explained by their sector and workforce composition. In contrast, many smaller cities deviate strongly from their predicted productivity, given their population size. Those cities that are located below the trend line are less productive than expected given the estimated relationship, while those above the trend line are more productive.
Compared to 2007 shown in Figure 2.9, Core Cities are clustered even closer around the trend line, while smaller cities, as well as London, are slightly more dispersed. Yet, the overall picture remains remarkably stable. In particular, the estimated magnitude of agglomeration economies, which is represented by the slope of the trend line in both figures barely changed over the years and continues to remain exceptionally low.
Figure 2.9. Agglomeration economies in the UK, 2007
Productivity differences (net of the workforce and sectoral composition, 2007) and city size (2007)

Note: Hollow diamonds denote Core Cities, solid diamond denote other UK cities. The horizontal axis plots city population (log scale). The vertical axis plots productivity differences from the national average net of workforce and sector effects. The analysis is conducted at the functional urban area level. Belfast is excluded due to data availability.
Source: OECD calculations based on the UK Annual Survey of Hours and Earnings (ASHE) microdata.
Generating stronger agglomeration economies would yield significant productivity gains
The weak relationship between city size and productivity is one of the explanations behind the low levels of productivity in the UK. If large cities would use their potential to generate agglomeration economies, the productivity gains could be substantial. This can be illustrated by a hypothetical thought experiment, under which the relationship between city size and productivity gains in the UK is 0.03 or roughly 3 times stronger than it is today. This magnitude of agglomeration economies would be identical to the agglomeration economies observed in France and Germany. It would imply that a city, which is 10% larger than another has a productivity gain of 0.3% instead of 0.09%. While this does not sound much, Figure 2.10 shows that it can make a significant difference.
All Core Cities fall significantly below the productivity levels predicted according to the strong agglomeration economies scenario.5 If they moved up in the figure to the steeper line that represents the counterfactual scenario of strong agglomeration economies, their average productivity level would increase by 4.1 percentage points relative to the national average. Such productivity growth would raise output in Core Cities by GBP 19.8 billion per year. Given their importance for the UK economy, it would also increase aggregate productivity levels in the UK by close to 1% or nearly as much as the entire UK productivity growth between 2008 and 2016. Figure 2.11 shows the productivity gains of each Core City if it were to catch up to the strong agglomeration economies scenario.
Figure 2.10. Further agglomeration gains are possible

Note: Hollow diamonds denote Core Cities, solid diamond denote other UK cities. The horizontal axis plots city population (log scale). The vertical axis plots productivity differences from the national average net of workforce and sector effects. The trend line for the strong agglomeration economies scenario uses elasticities estimated by Combes, Duranton and Gobillon (2008[20]) for France and Hirsch et al. (2019[33]) for Germany. The analysis is conducted at the functional urban area level. Estimates for Belfast come from econometric analysis using aggregate data, for details see endnote 3. The analysis is conducted at the functional urban area level.
Source: OECD calculations based on the UK Annual Survey of Hours and Earnings (ASHE) microdata. Estimates for Belfast are based on aggregate data from National Official Labour Market Statistics (NOMIS[34]) and Northern Ireland Statistics and Research Agency (NISRA[35]) data (accessed August 2019).
Of course, such a thought exercise is an illustration of the potential productivity gains but should not be taken literally. Productivity distributions across cities are the outcome of decades of economic transformations and policy choices and cannot easily be replicated across countries. Moreover, given the size of Core Cities, any change in productivity levels of this magnitude would also have important macroeconomic consequences that cannot be taken into account in this thought exercise. From a purely mechanical point of view, such a movement would change the predicted relationship between city size and productivity itself, so that the estimated slope of the relationship between size and productivity would change.
Raising productivity in large cities in the UK to levels seen in other high-income countries could be achieved through two sets of policies. First, the factors that lead to the emergence of agglomeration economies could be strengthened. For example, in order to maximise the quality of matches between employers and employees, it is important that workers can reach as many potential workplaces as possible from their place of residence. Likewise, innovation diffusion to small firms can be strengthened by creating specialised on-the-job training programmes that teach the latest production technologies. Given the large number of potential participants, large cities are best suited to offer such programmes. Last but not least, it is important to upgrade existing infrastructure, such as telecommunication networks, rail lines and airports, to ensure that it benefits local economies to its full potential.
Figure 2.11. Productivity gains from stronger agglomeration economies
Productivity gains if Core Cities were catching up to a strong agglomeration economies scenario

Note: This chart reflects the differences between actual productivity levels in Core Cities (net of workforce and sectoral composition) and their expected productivity levels in a counterfactual scenario in which the UK generates agglomeration economies to the degree that France and Germany do (see Figure 2.10 for details).
Source: OECD calculations based on the UK Annual Survey of Hours and Earnings (ASHE) microdata. Estimates for Belfast are based on aggregate data from National Official Labour Market Statistics (NOMIS[34]) and Northern Ireland Statistics and Research Agency (NISRA[35]) data (accessed August 2019).
Second, agglomeration dis-economies can be reduced by mitigating factors that reduce productivity in large cities. Such factors include congestion, which leads to workers wasting time in traffic and pollution, which affects health levels, reduces workplace performance and increases time spent on sick leave. It also includes high real estate prices, which can lead businesses to locate in suboptimal locations to save rents. Such suboptimal firm locations can make it more difficult to access customers and to connect to universities. It can also limit the possibility for employees to meet with employees of other firms. Such random encounters are considered an important factor in the spread of innovations from one firm to another.
Comparing the impact of workforce and sector composition to the underutilised potential of agglomeration economies
The analysis above allows shedding light on how much workforce and sector composition affects productivity. Figure 2.12 shows the productivity gains for each Core City if workforce and sector composition were to catch up to the national average. The figure shows that in some cities, workforce and sector composition are primarily responsible for low productivity, while agglomeration economies play a more prominent role in other cities.
For example, Birmingham could increase its productivity by approximately seven percentage points if its workforce and sector composition were to adjust to the national average. In contrast, the productivity gain from catching up to the strong agglomeration economies scenario would benefit from five percentage points (Figure 2.11). In contrast, the picture for Bristol is the reverse. As its current workforce and industry composition is about as favourable as the national average, catching up to it would yield productivity gains of less than 0.5 percentage points. In contrast, the gains from generating more agglomeration economies and catching up to the strong agglomeration economies scenario would yield a productivity gain of two percentage points. Figure 2.13 provides the average productivity for all Core Cities. The bar on the left-hand side shows the potential gains from having a workforce and sector composition in line with the rest of the UK. The bar on the right-hand side shows the average gains from generating agglomeration economies to the same degree as French and German cities do.
Figure 2.12. Productivity effects from workforce characteristics and sector composition
Productivity gains if workforce characteristics and sector composition in Core Cities were to adjust to the national average

Note: The composition effect reflects the productivity gain that a city would realise if its workforce and sector composition adjusts to the national average. Estimates for Belfast come from econometric analysis using aggregate data. For details endnote 3.
Source: OECD calculations based on the UK Annual Survey of Hours and Earnings (ASHE) microdata. Estimates for Belfast are based on aggregate data from National Official Labour Market Statistics (NOMIS[34]) and Northern Ireland Statistics and Research Agency (NISRA[35]) data (accessed August 2019).
Figure 2.13. Composition effects and potential gains from strong agglomeration economies

Note: The figure presents gains associated with composition effects and potential gains from strong agglomeration economies for Core Cities. They are calculated as the weighted average across all Core Cities. Estimates for Belfast come from econometric analysis using aggregate data, for details see endnote 3.
Source: OECD calculations based on the UK Annual Survey of Hours and Earnings (ASHE) microdata. Estimates for Belfast are based on aggregate data from National Official Labour Market Statistics (NOMIS[34]) and Northern Ireland Statistics and Research Agency (NISRA[35]) data (accessed August 2019).
Importantly, Figure 2.13 should not be seen as an argument in favour of one set of policies or another. Policies to strengthen the skills of the workforce and policies to foster agglomeration economies do not conflict with each other and should be pursued in parallel. Moreover, there are positive spill-overs from one policy area to another. As discussed in the following section, increasing the education level of the workforce fosters the emergence of agglomeration economies, while cities that are able to generate positive agglomeration economies are likely to attract more skilled workers.
More generally, the same argument applies also to policies across levels of government. Productivity levels in cities are determined by structural factors that affect the entire country as well as by place-specific factors that affect individual cities. Thus, structural policies across the UK should be seen as complementary to place-based policies that aim at improving productivity in individual cities. Instead of prioritising one over the other, the UK Government should pursue both in a co-ordinated fashion.
Further evidence on the role of sectoral composition, infrastructure and connectivity for productivity
The section above made the argument that stronger agglomeration economies can not only contribute to increased productivity levels in Core Cities but also have significant effects on aggregate productivity in the entire UK. From a policy perspective, the important question is how to foster the emergence of agglomeration economies. This section will present tentative empirical evidence on these questions. The subsequent chapter contains a discussion of local economic development policies and subnational governance that provides concrete guidance based on the empirical results in this chapter.
Productivity and human capital
Human capital levels are one of the most important determinants of productivity. More educated people are more productive and are paid higher wages to account for this increased productivity. Yet, the education levels of cities’ workforce affect productivity through a second channel. More educated workers also have positive effects on the productivity of their co-workers, an effect that economists call a positive externality (Moretti, 1999[37]).
The econometric analysis discussed allows identifying this effect. It shows that a 10-percentage-point increase in the share of university graduates is associated with a 1.7% increase in productivity of the overall workforce, an effect that is statistically significant. It is important to note that this number captures only the positive spill-overs associated with having an educated workforce. It does not capture the individual gains that are associated with being educated. In other words, this productivity gain reflects the positive effects that a highly educated worker has on its co-workers and not the effects of education on the worker who received the education.
Connectivity of cities
Having better accessibility to other cities and regions is an important determinant of a city’s productivity. A city that is better connected to the rest of the country can provide better market access to firms and allows them to export at a lower cost (Krugman, 1980[38]). Likewise, it also reduces the local prices of imports, which increases efficiency and consumer welfare (Krugman and Venables, 2006[39]). As both factors boost productivity and thus give a competitive advantage, it also renders a place more attractive to investments. Moreover, better road access allows better and faster transportation of people which also improves their quality of life.
Empirically, the analysis shows that a 10-percentage-point increase in the road accessibility performance of a city increases the average productivity by 1.2%. Road accessibility is measured as the share of people that can be reached within a 90-minute drive from the city relative to the number of people living in the area that is potentially accessible (EC, 2018[40]). Cities that have better road infrastructure or good connections to motorway networks provide access to large population concentrations allowing higher accessibility. While the econometric analysis used road accessibility for data availability reasons, it is likely that accessibility by other modes of transport has equally important effects (Department for Transport, 2018[41]).
Industrial specialisation and diversity
Specialisation and diversification are two opposing factors that can potentially create agglomeration economies. Productivity gains from specialisation emerge because the workforce’s skills better match the needs of the industry and because innovations spread faster across firms within the same industry than across firms in different industries. In contrast to other benefits from agglomeration, the gains from specialisation are specific to the specialised industry (Özgüzel, 2019[42]). Many studies find that specialisation has a statistically significant, positive effect on productivity within the specialised industry, although that effect tends to be highly geographically localised (Combes and Gobillon, 2015[43]).
The idea that increasing diversity leads to productivity gains was proposed by journalist and sociologist Jane Jacobs (Jacobs, 1969[44]). Jacobs argued that the interactions of people in different occupations and industries facilitate a creative process that generates new ideas and innovation in cities (Glaeser et al., 1992[45]). The intuition of Jacobs was formalised by Duranton and Puga (2001[46]) and has been used to test whether industrial diversity in cities could drive productivity.
Empirically, it is not possible to identify statistically significant effects of either specialisation or diversification in UK cities. A potential explanation for this finding is that the benefits of specialisation and diversification are roughly identical and therefore cancel each other out. A productivity gain from increasing specialisation might lead to a productivity loss from decreasing diversification and vice versa.
Local and regional planning matters for productivity
The importance of place-making
Making cities attractive places to live and work is an important approach to making them more productive. Attractive cities are better in retaining skilled workers, which are one of the most important factors influencing productivity levels. They also generate investments from local, national and international businesses.
While competition across cities to attract skilled workers and capital might seem a zero-sum game and therefore a waste of resources from a public policy perspective, this is not the case for several reasons. First, and most importantly, public investment in the attractiveness of cities benefits residents independently from its economic impact because it increases quality of life. Second, attractive cities not only attract private investments that would have otherwise gone to other cities but they also generate additional investments that would not have been made otherwise. This is especially important given that low investment rates have been identified as one of the factors responsible for the weak productivity growth in the UK.
Many of the characteristics that are typically associated with attractive urban areas are also factors that facilitate the emergence of agglomeration economies. For example, walkable neighbourhoods with relatively high densities that combine a variety of uses are generally considered attractive places to live and real estate in these areas tends to be more valuable than in other parts of a city (Cortright, 2009[47]). At the same time, these are also neighbourhood characteristics that are known to foster the emergence of agglomeration economies through Jacobs’ externalities.6
Especially innovation in the creative sector is facilitated by the interactions that take place in dense, mixed-use neighbourhoods. Consequently, creative industries locate predominantly in attractive inner-urban spaces. In contrast, science industries, in which unstructured interactions of people in different professions play a less important role, are more frequently found in suburbs (Spencer, 2015[48]). For these industries, the more versatile built-environment and lower costs in more suburban location outweigh the benefits of being located in central urban areas.
From a policy perspective, this leads to a twofold conclusion. First, cities should consider investments in the attractiveness of their urban areas not only as a contribution to the quality of life of its residents but also as an economic development strategy. In particular, brownfield redevelopment and urban regeneration projects should factor in the economic spill-overs from creating attractive neighbourhoods that are dense, aesthetically appealing and feature a variety of uses. In the short term, it is often costlier to preserve and regenerate the existing building stock and intersperse it with new development. Yet, a regenerated area with an attractive building stock and a diverse mix of uses can yield large returns in the long term.
Second, cities should ensure that sufficient space for the development of research-intensive science industries is made available in less central neighbourhoods. Although such neighbourhoods often have a more functional character, it can still pay off to ensure that they are high-quality urban spaces that combine a variety of uses, are walkable and well-connected to public transport. As abovementioned, such characteristics are attractive for a highly skilled workforce and consequentially attract investments. Moreover, a strong shift of innovative activities from suburban to inner-urban environments has been taking place in the last two decades (Florida, Adler and Mellander, 2016[49]). As this trend is likely to continue, cities can ensure their future attractiveness for innovative activities by ensuring that commercial developments in these areas meet the growing need for neighbourhoods with urban characteristics.
Box 2.6. Cardiff Central Square Regeneration
The city’s Central Square regeneration scheme is Cardiff’s flagship economic development project. It seeks to address a perceived lack of agglomeration within the city by developing a cluster of knowledge-based business activity in and around the Central Square area. Anchored by the BBC Wales headquarter, the project has also provided for a high-quality environment for a range of commercial and higher education activity. In particular, the co-location of the Cardiff University School of Journalism next to the BBC Headquarters will support the development of the city’s creative sector, identified as a priority in Cardiff’s economic strategy.
Further effects are also driven by the location, with Cardiff Central Station at its heart. The station is both the focus of the rail routes that serve the city-region, as well as the sole interchange between these and the Great Western Mainline. Both the mainline and local services are subject to substantial upgrading, including electrification that will provide for a significant improvement in services, and hence greater reach and economic impact from the Central Square project.
Provision is made for the future expansion of the project south of the railway line, allowing for further agglomeration of activity within similar easy reach to the city-regions public transport infrastructure. In total, the scheme has the potential to provide for 30 000 jobs and leverage GBP 2 billion of investment over the next 10 to 15 years.
Source: Core Cities.
Linking Core Cities with surrounding regions
Agglomeration economies emerge because people learn from each other, innovate together, share infrastructure and specialise in a field of work. For this to happen, people must live and work in close proximity to each other. However, places further away from a large city can also benefit from the agglomeration economies it generates. While it is difficult to quantify the magnitude of these effects over greater distances precisely, recent research emphasises the importance of “borrowed agglomeration economies” (Meijers and Burger, 2016[50]).
The concept of “borrowed agglomeration economies” was developed by Alonso (2019a[51]) to describe the idea that smaller towns and cities can benefit from the positive agglomeration economies of larger neighbours. For example, businesses in a small town close to a large city benefit from the infrastructure of the larger city, such as airports and good public transport networks. If the town is close enough to the city to belong to the same local labour market, they can recruit from the same large pool of specialised workers. Moreover, businesses in such a town have easy access to specialised suppliers and a diverse customer base in the large city. Thus, it is not surprising that towns close to a large city can benefit from some of the agglomeration economies of the large city.
Borrowed agglomeration economies can most likely be observed in the UK, too. For example, urban areas that are within 90-minutes travel time to London have on average a 3.5% higher productivity than would be expected given the characteristics of their workforce, their sector mix and their population size. Yet, the emergence of borrowed agglomeration is not guaranteed, nor is the geographical distance over which they can occur fixed. Whether and over which distance borrowed agglomeration economies emerge depends on policy decisions in a variety of policy fields.
Most immediately, adequate transport connections are important. For example, good public transport connections on high-quality trains allow employees to work while travelling to customers, thus raising worker productivity immediately and also increasing the potential customer base. Likewise, a good public transport network increases the size of the local labour market, thereby improving the quality of matches between employers and employees and raising productivity through this channel.
Yet, it is not only infrastructure that matters for linking cities and regions. Effective spatial planning at the regional scale is similarly important. For example, good spatial planning can ensure that facilities of regional importance and major business districts are located in places that are easily accessible for the entire region. It also avoids costly duplication of facilities, co‑ordinates economic development policies across the boundaries of local jurisdictions and can foster the emergence of regional specialisations.
Other policies can also help to generate borrowed agglomeration economies. For example, universities are crucial actors in developing regional innovation ecosystems. They connect public and private actors, provide a skilled workforce to the region, and work directly with businesses to create innovations. In recent years, their importance for regional development has increased but there are important differences in how universities are engaged in regional co‑operations (Reichert, 2019[52]). Policymakers can facilitate the regional role that universities play through a variety of measures, ranging from specialised course offers in satellite locations to dedicated outreach programmes to small- and medium-sized enterprises (SMEs) within the region. By strengthening the innovative potential throughout the region, such policies contribute to raising productivity throughout the region.
Box 2.7. The Glasgow City-Region Regional Partnership
The Glasgow City-Region Regional Partnership builds on the strengths of the City Region City Deal partners to collaborate beyond the delivery of the City Deal Programme and brings together key players from the public and private sector to establish a partnership that reflects local economic circumstances, shared policy priorities and existing governance arrangements. The partnership comprises local, national and Scottish government, government agencies, the private sector and academia, united around a common purpose for inclusive economic growth and responsible for overseeing the delivery of a Regional Economic Strategy.
The development of Regional Partnerships was a key policy that emerged from the Scottish Government’s Enterprise and Skills Review. The overall purpose of the Regional Partnership is: to actively promote collaboration and partnership working between the eight member authorities, Scottish and UK Governments, their agencies, private sector and academia to deliver shared economic priorities; to maximise economic growth across all of the city-region geography ensuring that inclusive growth measures are incorporated into all activity; and to enable new ways of working to maximise outcomes. After 12 months, the Regional Partnership has matured to the level whereby it is co-producing a refreshed Regional Economic Strategy to incorporate spatial planning, investment priorities and actions across all of the partners to address shared priorities. The terms of reference for the partnership allow for other partners, for example, public health, to be co-opted as required.
Source: Core Cities.
While policies to link cities with regions are usually framed as benefitting regions, they are equally important for large cities. By global standards, Core Cities are comparatively small, just like most other European first- and second-tier cities. This is partly due to the early urbanisation in Europe, which favoured the emergence of many mid-sized cities and partly due to policy choices made subsequently.
In isolation, the size of Core Cities can be too small to provide the necessary critical mass of customers or suppliers to businesses, to use infrastructure such as an international airport efficiently, or to attract foreign direct investment (FDI). By linking the wider region closer to the city, the city gains additional mass that can help to increase productivity.
There are various approaches to link cities closer to their surrounding regions. For examples, most large cities in Germany have formed metropolitan associations that are responsible for varying policy areas but often cover issues such as regional spatial planning and business promotion (Ahrend, Gamper and Schumann, 2014[53]). These metropolitan associations are sometimes enshrined in state law, while in other instances they are entirely voluntary co‑operations between the involved local governments. Likewise, Rotterdam and The Hague in the Netherlands have formed a new metropolitan authority that is among other issues responsible for public transport provision and business promotion. The main motivation behind the creation of the metropolitan authority was the realisation that closer co‑operation is in the mutual interest of both cities (OECD, 2016[54]).
References
[28] Ahrend, R. et al. (2017), “What makes cities more productive? Evidence From five OECD countries on the role of urban governance”, Journal of Regional Science, Vol. 57/3, pp. 385-410, http://dx.doi.org/10.1111/jors.12334.
[53] Ahrend, R., C. Gamper and A. Schumann (2014), “The OECD Metropolitan Governance Survey: A Quantitative Description of Governance Structures in large Urban Agglomerations”, OECD Regional Development Working Papers, No. 2014/4, OECD Publishing, Paris, https://dx.doi.org/10.1787/5jz43zldh08p-en.
[55] Alonso, W. (1973), “Urban zero population growth”, Daedalus, Vol. 102/4, pp. 191-206.
[59] Alonso, W. (1973), “Urban Zero Population Growth”, Daedalus, Vol. 102/4, pp. 191-206.
[5] Andrews, D., C. Criscuolo and P. Gal (2016), “The Best versus the Rest: The Global Productivity Slowdown, Divergence across Firms and the Role of Public Policy”, OECD Productivity Working Papers, No. 5, OECD Publishing, Paris, https://doi.org/10.1787/63629cc9-en.
[14] Australian Government Productivity Commission (2015), “What is productivity and how is it measured?”, PC Insight 05/2015.
[8] Baily, M. and N. Montalbano (2016), Why Is US Productivity Growth So Slow? Possible Explanations and Policy Responses, https://www.brookings.edu/research/why-is-us-productivity-growth-so-slow-possible-explanations-and-policy-responses/ (accessed on 14 September 2019).
[3] Barnett, A. et al. (2014), The UK Productivity Puzzle, Bank of England Quarterly Bulletin 2014 Q2.
[21] Baum-Snow, N. and R. Pavan (2012), “Understanding the city size wage gap”, Review of Economic Studies, Vol. 79/1, pp. 88-127, http://dx.doi.org/10.1093/restud/rdr022.
[15] Borjas, G. (2012), Labor Economics, Higher Education, McGraw-Hill.
[26] Carlino, G. and W. Kerr (2015), Agglomeration and Innovation, Elsevier B.V., http://dx.doi.org/10.1016/B978-0-444-59517-1.00006-4.
[29] Combes, P. et al. (2019), “Unequal migration and urbanisation gains in China”, Journal of Development Economics, http://dx.doi.org/10.1016/j.jdeveco.2019.01.009.
[20] Combes, P., G. Duranton and L. Gobillon (2008), “Spatial wage disparities: Sorting matters!”, Journal of Urban Economics, Vol. 63/2, pp. 723-742, http://dx.doi.org/10.1016/j.jue.2007.04.004.
[43] Combes, P. and L. Gobillon (2015), The Empirics of Agglomeration Economies, Elsevier B.V., http://dx.doi.org/10.1016/B978-0-444-59517-1.00005-2.
[47] Cortright, J. (2009), How Walkability Raises Home Values in U.S. Cities, CEOs for Cities.
[18] D’Costa, S. and H. Overman (2014), “The urban wage growth premium: Sorting or learning?”, Regional Science and Urban Economics, Vol. 48, pp. 168-179, http://dx.doi.org/10.1016/j.regsciurbeco.2014.06.006.
[30] De la Roca, J. and D. Puga (2017), “Learning by working in big cities”, Review of Economic Studies, Vol. 84/1, pp. 106-142, http://dx.doi.org/10.1093/restud/rdw031.
[41] Department for Transport (2018), Transport Statistics Great Britain 2018.
[56] Diodato, D., F. Neffke and N. O’Clery (2018), “Why do industries coagglomerate? How Marshallian externalities differ by industry and have evolved over time”, Journal of Urban Economics, Vol. 106, pp. 1-26, http://dx.doi.org/10.1016/J.JUE.2018.05.002.
[24] Duranton, G. and D. Puga (2004), “Micro-foundations of urban agglomeration economies”, in Henderson, J. and J. Thisse (eds.), Handbook of Regional and Urban Economics, Elsevier.
[46] Duranton, G. and D. Puga (2001), “Nursery cities: Urban diversity, process innovation, and the life cycle of products”, American Economic Review, Vol. 91/5, pp. 1454-1477, http://dx.doi.org/10.1257/aer.91.5.1454.
[40] EC (2018), Road Transport Performance in Europe, European Commission.
[7] Feldstein, M. (2016), “Remarks at the Brookings Institution conference on productivity”, Slow Growth in Productivity: Causes, Consequences, and Policies, https://www.brookings.edu/wp-content/uploads/2016/08/feldstein-remarks.pdf.
[57] Florida, R., P. Adler and C. Mellander (2016), “The city as innovation machine”, Regional Studies, Vol. 51/1, pp. 86-96, http://dx.doi.org/10.1080/00343404.2016.1255324.
[60] Florida, R., P. Adler and C. Mellander (2016), “The city as innovation machine”, Regional Studies, Vol. 51/1, pp. 86-96, http://dx.doi.org/10.1080/00343404.2016.1255324.
[49] Florida, R., P. Adler and C. Mellander (2016), “The city as innovation machine”, Regional Studies, Vol. 51/1, pp. 86-96, http://dx.doi.org/10.1080/00343404.2016.1255324.
[16] Gal, P. (2013), “Measuring Total Factor Productivity at the Firm Level using OECD-ORBIS”, OECD Economics Department Working Papers, No. 1049, OECD Publishing, Paris, https://doi.org/10.1787/5k46dsb25ls6-en.
[19] Gibbons, S., H. Overman and P. Pelkonen (2014), “Area disparities in Britain: Understanding the contribution of people vs. place through variance decompositions”, Oxford Bulletin of Economics and Statistics, Vol. 76/5, pp. 745-763, http://dx.doi.org/10.1111/obes.12043.
[45] Glaeser, E. et al. (1992), “Growth in cities”, Journal of Political Economy, Vol. 100/6, pp. 1126-1152, http://dx.doi.org/10.1086/261856.
[32] Groot, S. and H. de Groot (2014), “Regional wage differences in the Netherlands: Micro evidence on agglomeration externalities”, Journal of Regional Science, Vol. 54/3, pp. 503-523, http://dx.doi.org/10.1111/jors.12070.
[4] Haldane, A. (2018), “The UK’s productivity problem: Hub no spokes”, Bank of England 06, pp. 1-40, https://www.bankofengland.co.uk/-/media/boe/files/speech/2018/the-uks-productivity-problem-hub-no-spokes-speech-by-andy-haldane.
[33] Hirsch, B. et al. (2019), “The urban wage premium in imperfect labour markets”, CEP Discussion Papers dp1608.
[44] Jacobs, J. (1969), The Economy of Cities, Vintage Books, New York, http://dx.doi.org/10.1057/9780230275010.
[38] Krugman, P. (1980), “Scale economies, product differentiation, and the pattern of trade”, American Economic Review, Vol. 70/5, pp. 950-959, http://dx.doi.org/10.1016/0022-1996(79)90017-5.
[39] Krugman, P. and A. Venables (2006), “Globalization and the inequality of nations”, The Quarterly Journal of Economics, Vol. 110/4, pp. 857-880, http://dx.doi.org/10.2307/2946642.
[25] Marshall, A. (2009), Principles of Economics: Unabridged Eight Edition, Cosimo, Inc.
[23] Marshall, A. (1890), Principles of Economics, Macmillan, London.
[50] Meijers, E. and M. Burger (2016), “Stretching the concept of ‘borrowed size’”, Urban Studies, Vol. 54/1, pp. 269-291, http://dx.doi.org/10.1177/0042098015597642.
[36] Melo, P., D. Graham and R. Noland (2009), “A meta-analysis of estimates of urban agglomeration economies”, Regional Science and Urban Economies, Vol. 39/3, pp. 332-342.
[31] Mion, G. and P. Naticchioni (2009), “The spatial sorting and matching of skills and firms”, Canadian Journal of Economics, Vol. 42/1, pp. 28-55, http://dx.doi.org/10.1111/j.1540-5982.2008.01498.x.
[37] Moretti, E. (1999), “Estimating the social return to education: Evidence from repeated cross-sectional and longitudinal data”, University of California Working Paper.
[35] NISRA (2019), Northern Ireland Statistics and Research Agency, https://www.nisra.gov.uk/.
[34] NOMIS (2019), National Official Labour Market Statistics, https://www.nomisweb.co.uk/sources.
[1] OECD (2019), OECD Productivity Statistics (database), https://doi.org/10.1787/pdtvy-data-en.
[12] OECD (2019), OECD Regional Outlook 2019: Leveraging Megatrends for Cities and Rural Areas, OECD Publishing, Paris, https://doi.org/10.1787/9789264312838-en.
[13] OECD (2019), OECD Regional Statistics (database).
[9] OECD (2017), OECD Economic Surveys: United Kingdom 2017, OECD Publishing, Paris, https://dx.doi.org/10.1787/eco_surveys-gbr-2017-en.
[54] OECD (2016), OECD Territorial Reviews: The Metropolitan Region of Rotterdam-The Hague, Netherlands, OECD Territorial Reviews, OECD Publishing, Paris, https://dx.doi.org/10.1787/9789264249387-en.
[11] OECD (2015), OECD Economic Surveys: United Kingdom 2015, OECD Publishing, Paris, https://dx.doi.org/10.1787/eco_surveys-gbr-2013-en.
[6] OECD (2015), The Future of Productivity, OECD Publishing, Paris, https://doi.org/10.1787/9789264248533-en.
[17] OECD (2015), The Metropolitan Century: Understanding Urbanisation and its Consequences, OECD Publishing, Paris, https://dx.doi.org/10.1787/9789264228733-en.
[51] OECD (2019a), Making Decentralisation Work: A Handbook for Policy-Makers, OECD Multi-level Governance Studies, OECD Publishing, Paris, https://dx.doi.org/10.1787/g2g9faa7-en.
[42] Özgüzel, C. (2019), “Agglomeration effects in a developing economy: Evidence from Turkey”, ERF Working Papers.
[2] Pessoa, J. and J. Van Reenen (2014), “The UK productivity and jobs puzzle: Does the answer lie in wage flexibility?”, Economic Journal, Vol. 124/576, pp. 433-452, http://dx.doi.org/10.1111/ecoj.12146.
[27] Puga, D. (2010), “The magnitude and causes of agglomeration economies”, Journal of Regional Science, Vol. 50/1, pp. 203-219.
[58] Reichert, S. (2019), “The role of universities in regional innovation ecosystems”, European University Association.
[52] Reichert, S. (2019), The Role of Universities in Regional Innovation Ecosystems, European University Association.
[22] Riley, R., A. Rincon-Aznar and L. Samek (2018), “Below the aggregate: A sectoral account of the UK productivity puzzle”, ESCoE Discussion, Vol. 06.
[48] Spencer, G. (2015), “Knowledge neighbourhoods: Urban form and evolutionary economic geography”, Regional Studies, Vol. 49/5, pp. 883-898, http://dx.doi.org/10.1080/00343404.2015.1019846.
[10] UK2070 Commission (2019), Fairer and Stronger: Rebalancing the UK Economy.
Notes
← 1. There are significant differences in estimated productivity levels depending on whether estimates are based on GVA per worker or on real hourly wages. Measures based on GVA per worker generally report larger differences than measures based on average real hourly wages. In line with the established consensus among urban economists, measures based on average real hourly wages obtained from microdata are preferred when they are available.
← 2. While all workers benefit from agglomeration economies, recent evidence suggests that the productivity of high-skilled workers benefits more strongly than the productivity of low-skilled workers (Diodato, Neffke and O’Clery, 2018[56]).
← 3. Due to access restrictions to the relevant microdata for Northern Ireland, estimates for Belfast were obtained using aggregate hourly wage data obtained from ONS and NISRA. In a first step, residuals from a regression of aggregate wage levels on workforce characteristics and industry composition were obtained for all UK cities. In a second step, the residuals were rescaled to match their variance to the variance of the city-level fixed effects of the first-stage microdata regression (see Box 2.4). The estimate for Belfast is based on the rescaled residual for Belfast.
← 4. Driving times are obtained using Google Maps, by taking the average of the range of predicted driving times for a Monday at 1pm.
← 5. It is noteworthy that London, the UK’s most productive city, falls below the trend line for the strong agglomeration economies scenario. This suggests that London’s productivity level, adjusted for the sectoral composition and the skill profile of its workforce, could be higher than it is. While this might seem surprising, the possibility of London underperforming, too, is not implausible given the fact that labour productivity in Paris is 15% higher than London.
← 6. The term Jacobs’ externalities describes the productivity-enhancing innovative processes that emerge from interaction of people in different professions.