This chapter uses longitudinal data from five countries (Australia, Canada, Denmark, Switzerland and the United States) to examine the relationship between cognitive competencies at age 15 and educational attainment and early labour market outcomes at age 25. It also explores different sources of disadvantage, related to a student’s home, school and social environment, which contribute to skills gaps during compulsory education and reduce the upward social mobility of children from less-educated families.
Equity in Education
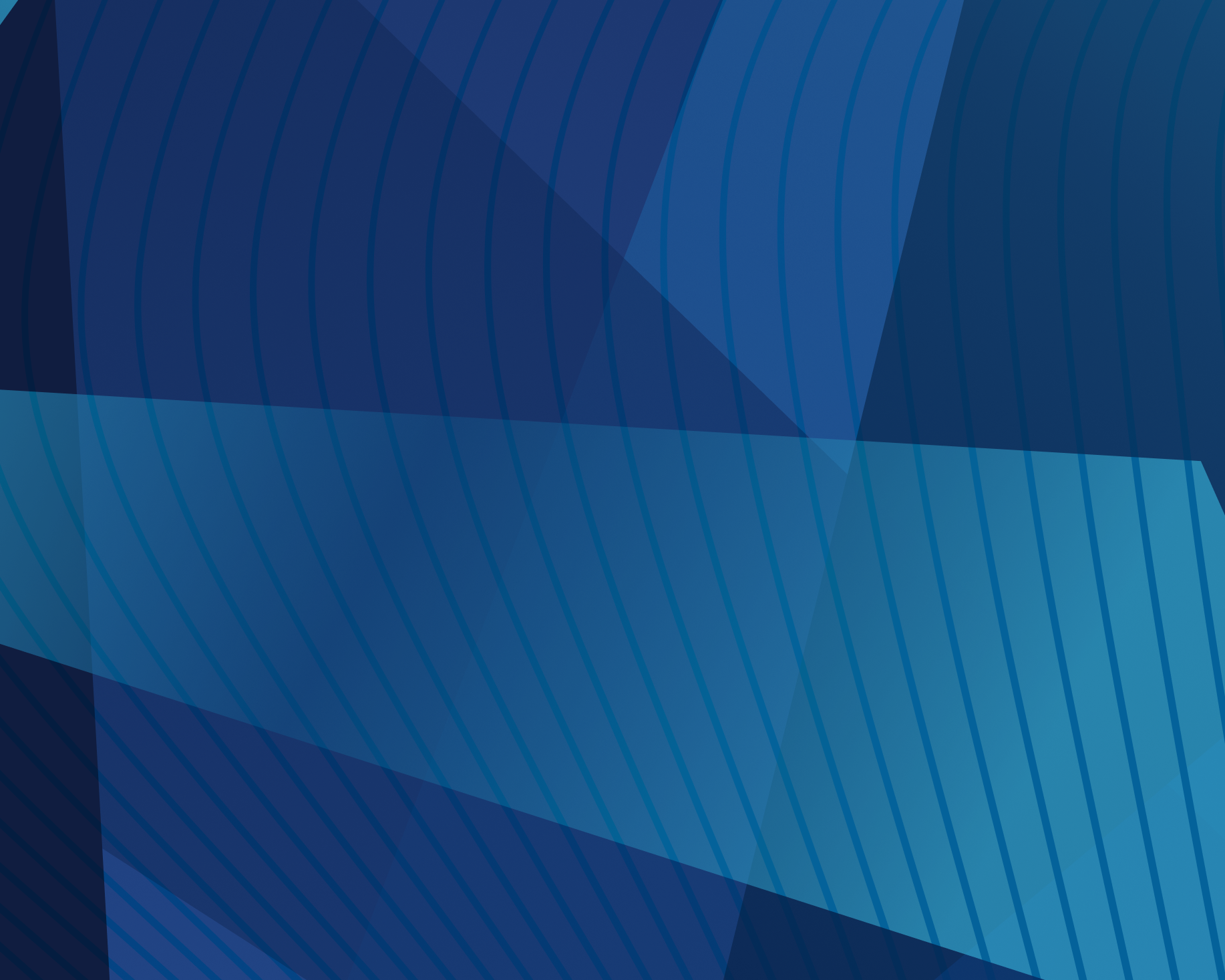
Chapter 5. Educational mobility and school-to-work transitions among disadvantaged students
Abstract
What the data tell us
Across the five countries analysed, students with at least one tertiary-educated parent are between 17 and 30 percentage points more likely to complete university than their peers without tertiary-educated parents.
Students who score in the top quarter in reading are between 38 and 53 percentage points more likely to complete university than students who score in the bottom quarter. Differences in 15-year-olds’ reading performance explain between 27% and 43% of the difference in university completion rates between students with and those without tertiary-educated parents. This suggests that reducing the gaps related to socio-economic status in what students learn during compulsory schooling could increase upward educational mobility.
Across the five countries considered, students with tertiary-educated parents are between 7 and 20 percentage points more likely to be employed in jobs requiring tertiary education at age 25 than students without tertiary-educated parents. Accounting for differences in PISA performance reduces this difference to between 4 and 13 percentage points.
Lower expectations, less family wealth, concentration in disadvantaged schools, and limited access to cultural activities represent certain characteristics of students from less-educated families that are related to the lower likelihood of completing a university education.
DEFINING SOCIAL AND EDUCATIONAL MOBILITY
Social mobility refers to a change in the economic or social status of individuals between their childhood and adult life. Children’s ability to attain a higher social status than their parents depends on multiple factors, including health, social and cultural capital, education and the evolution of the labour market (Nunn et al., 2007[1]). While individual effort can result in upward social mobility, the chances that someone from a disadvantaged background will succeed in life are also bound by where the person lives, and by the social and cultural group to which he or she belongs (Ololube, Onyekwere and Agbor, 2015[2]; Schaefer, 2005[3]).
Inequitable education and career opportunities – shaped by factors such as race, gender or socio-economic status – can create inefficiencies that hinder economic growth, as they lead to significant misallocations of skills and talent (Hsieh et al., 2013[4]).
Because of differences in family and social environments, children begin formal schooling with different levels of skills (Downey, Hippel and Broh, 2004[5]). Schools can play an important role in compensating for these initial inequalities. But education systems that fail to provide equal opportunities to all students can end up reinforcing, rather than helping to reduce, social inequalities that may threaten social cohesion (OECD, 2010[6]). In today’s shifting labour market, the gaps between low-qualified and high-qualified workers are expanding: less-educated adults tend to face the highest unemployment and inactivity rates, as well as the lowest and most rapidly declining relative wages, on average (OECD, 2017[7]). That, in turn, can lead to a wide range of social problems, including poverty, poor health and crime (Schoenfeld, 2002[8]).
How successful are today’s schools at counterbalancing the forces that perpetuate existing inequalities in society? Some insights into this question can be obtained by analysing the relationship between socio-economic gaps in cognitive outcomes near the end of compulsory schooling – a measure of equity in education – and socio-economic gaps in higher education and labour market outcomes in early adult life. This analysis can be conducted by studying longitudinal data that provide information on the same individuals at different points in time.
This chapter uses longitudinal data from five countries – Australia, Canada, Denmark, Switzerland and the United States (Box 5.1) – to analyse the relationship between performance at school and educational mobility, defined as the likelihood that a student without tertiary-educated parents will complete tertiary education.1 The chapter also explores certain dimensions of disadvantage (less family wealth, less cultural capital, negative dispositions towards education, and school segregation) that may help to explain the strong links between parents’ education, students’ reading proficiency at the end of compulsory schooling, and transitions into higher education and skilled employment.
Box 5.1. Longitudinal country data
Analyses in this chapter are based on longitudinal data, accessed by the OECD Secretariat in raw form or through collaboration with national researchers, from the following countries (Figure 5.1).1
Figure 5.1. Description of longitudinal data sets
1. The final survey for Switzerland was conducted in 2014, when participants were 29. However, the previous survey, conducted in 2010, was used for this analysis in order to obtain information on participants at the age of 25.
2. The public access version of the ELS data excludes school identifiers, as well as detailed information on student degree selection, employment, and post-secondary performance.
Each of the datasets collects baseline data on nationally representative samples of students when they are 15 years old. The datasets from Australia, Canada, Denmark and Switzerland followed the transition of early PISA cohorts into adulthood, and can therefore shed light on the power of PISA literacy measures to accurately predict social mobility. Nationally representative longitudinal data from the United States, while not based on PISA participants, are also used because the data include measures of literacy proficiency of students around the age of 15, for which a concordance with PISA scores has been developed. While the frequency and timeline of follow-up surveys vary by country, all the datasets collect information on young people when they are approximately 25 years old (the only exception is Denmark, where the analysis in this chapter refers to individuals who are either 26 or 27 years old).
The data for Denmark is derived from PISA and the Survey of Adult Skills (a product of the OECD Programme for the International Assessment of Adult Competencies [PIAAC]). Participants in the PISA 2000 cycle were tested and interviewed again in the 2012 Survey of Adult Skills (PIAAC).
Transitions from Education to Employment (TREE1)2 surveys the post-secondary education and labour market pathways of students in Switzerland, and is the country’s first longitudinal study of this type at the national level. TREE1, the project’s first cohort, is based on a sample of students who participated in PISA 2000. The sample was tracked for follow-up surveys annually from 2001 to 2007, and twice more (in 2010 and 2014).
The Longitudinal Study of Australian Youth (LSAY) tracks students annually for a period of 10 years as they move from school into further study, work and other destinations. While the first surveys began in 1995, participants have been recruited from Australian schools that have taken part in PISA since 2003 (Y03).
Canada designed the Youth in Transition Survey (YITS) to examine the patterns of, and influences on, major transitions in young people’s lives, particularly with respect to education, training and work. PISA/YITS is a special project that aligned the two survey programmes. The PISA 2000 cohort was selected to participate in YITS, and was surveyed in follow-up interviews every two years through 2010. These data have not been released for public access, so all estimates were obtained through collaboration with national researchers at Employment and Social Development Canada (ESDC), who conducted the analyses on the basis of statistical programmes prepared by the OECD.3
The Educational Longitudinal Study of 2002 (ELS) is the fourth in a series of American school-based longitudinal studies, focusing on the transitions of American youth from secondary school into higher education and the labour market. While the study does not involve PISA participants, it provides nationally representative data and records literacy measures for 15-year-old students that can be benchmarked to PISA. Follow-up surveys were conducted in 2004, 2006 and 2012/13. The public version of this dataset excludes access to school identifiers, as well as detailed reports of students’ degree type, employment and post-secondary transcripts.
1. One weakness of longitudinal data comes from attrition, the discontinued participation of original participants in a longitudinal study. Attrition is problematic for two main reasons. First, attrition reduces the size of the sample, and if the sample becomes too small, this can jeopardise statistical power. Second, if attrition is not random, it can lead to non-response bias. Attrition is not random if certain subgroups of the initial sample are more likely to drop out or not respond to a follow-up survey. If such is the case, this can mean that the sample no longer remains representative of the original population being studied, and thus can affect the validity of statistical findings. Two approaches are typically adopted to deal with this type of missing data: weighting survey responses to re-balance the data or imputing values for the missing information. In this chapter, country- and cycle-specific survey weights are used to correct for non-random attrition that occurred over the course of these longitudinal studies. This method places greater weight on individuals who may be under-represented in the final survey, based on a series of observable characteristics, in order to produce estimates from nationally representative samples.
2. The Swiss panel study TREE (Transitions from Education to Employment) is a social science data infrastructure mainly funded by the Swiss National Science Foundation (SNF) and located at the University of Bern.
3. Canadian results exclude observations from the province of Quebec due to the structural differences in primary and secondary education systems, in comparison to the other provinces in Canada.
PROGRESSION INTO HIGHER EDUCATION AND PISA PERFORMANCE AT AGE 15
This chapter focuses on upward educational mobility, defined as the likelihood that a student without tertiary-educated parents attains tertiary education. The percentage of students without a tertiary-educated parent ranges from 26% in Denmark to 62% in the United States (Table 5.36). The figures included in this chapter refer to advantaged and disadvantaged students as students with and without a tertiary-educated parent.
Figure 5.2 shows the rates at which students with and those without a tertiary-educated parent pass through various levels of education. In Australia, Canada and the United States, all students are enrolled in a comprehensive programme of secondary education that ends when they are about 18 years old. However, some students in these countries drop out during upper secondary education, as education is compulsory only until the student reaches the age of 16 (Table 5.35).
Figure 5.2. Highest level of education completed, by socio-economic status
Notes: Results based on students’ self-reports.
Values with statistically significant differences between advantaged and disadvantaged students are displayed in bold.
Advanced degree includes those who have completed or are currently attending a master’s or doctoral programme.
Advantaged students are those with at least one tertiary-educated parent; disadvantaged students are those without a tertiary-educated parent.
Tertiary vocational education refers to tertiary-level (ISCED 5B) vocational training.
Data from Canada did not allow for distinguishing between ISCED 4 and ISCED 5B vocational training, therefore country estimates include both levels of post-secondary vocational education.
Source: OECD, PISA 2000 and PISA 2003 Databases, Tables 5.1, 5.3, 5.5 and 5.37.
In Denmark and Switzerland, compulsory secondary education programmes end when students are between 15 and 16 years old. Students in these two countries then decide whether to continue secondary schooling and enter a separate, non-compulsory programme track. This more segmented course of studies may explain why the percentage of students completing secondary education is lower in Denmark and Switzerland than in Australia, Canada and the United States.
In all countries except Australia, students without tertiary-educated parents are less likely than students with a tertiary-educated parent to complete secondary education. However, when looking at the rates of university completion by age 25, a much larger gap related to parents’ education emerges in all countries. Denmark and Switzerland, with more prominent vocational tracks, have lower university completion rates overall. In Denmark, students with tertiary-educated parents are approximately 19 percentage points more likely than their peers with less-educated parents to complete university; in Switzerland, they are 16 percentage points more likely than their peers with less-educated parents to do so.2 Australia, Canada and the United States have higher rates of university completion overall, possibly because these countries do not separate students into academic and vocational tracks at the secondary level, so that students are often less prepared to enter the job market immediately after secondary education (Hanushek, Woessmann and Zhang, 2011[9]). Despite higher rates of university completion among students with less-educated parents, the absolute gaps between advantaged and disadvantaged groups are even larger in these countries, with a nearly 30 percentage-point difference in the United States, a 21 percentage-point difference in Australia, and a 17 percentage-point difference in Canada.
Across all countries, students without tertiary-educated parents are also less likely to pursue an advanced university degree (master’s or PhD) than their peers with tertiary-educated parents. In the United States, only 3% of students without a tertiary-educated parent – compared to 12% of students with a tertiary-educated parent – are enrolled in or have completed an advanced university degree when they are around 25 years old. For the other countries considered in this chapter, gaps in attainment of advanced degrees range from 5 to 17 percentage points between the two groups of students (Table 5.37).
PISA data show that differences related to socio-economic status in what students have learned and can do with their knowledge are large across all countries (OECD, 2016[10]). To what extent do these learning gaps explain educational mobility? If students score similarly in the PISA reading test, are there still differences between students from more- and less-educated families in how they progress through school after the age of 15? These questions are important because they can help identify the potential impact on educational and social mobility of policies that aim to reduce gaps in opportunities to learn during compulsory schooling.
Examining the relationship between university completion and PISA performance reveals significant differences in achievement across quarters of reading performance in all countries (Figure 5.3). In Switzerland, only 1% of students in the bottom quarter of reading performance, compared to 39% of students in the top quarter, complete university. In Canada, students in the top quarter of reading performance are 53 percentage points more likely than students in the bottom quarter to earn a university degree. In Australia, Denmark and the United States, differences between these two groups range from 44 to 51 percentage points.
Figure 5.3. University completion, by quarter of PISA reading performance
Notes: The difference between the top and the bottom quarters of reading performance is statistically significant in all countries.
Quarters of performance are computed for the final sample of each country longitudinal data set.
Countries are ranked in ascending order of the percentage of students in the bottom quarter of reading performance.
Source: OECD, PISA 2000 and PISA 2003 Databases, Table 5.4.
The relationship between 15-year-old students’ performance and completion of a tertiary degree holds across different measures of performance and different fields of study in tertiary education. For example, in Denmark, 15% of students in the top quarter of science performance complete a university degree in mathematics, engineering or science, while only 2% of students in the bottom quarter of science performance complete such degrees (Table 5.32). Across countries, no large difference in students’ choice of field of study at university, based on their parents’ education, is observed (Table 5.23). Only in Canada are students with tertiary-educated parents more likely than students with less-educated parents to choose to pursue science, mathematics or engineering degrees.
In Figure 5.4, the dark blue bars show the percentage-point difference in university completion rates between 25-year-old adults with and without tertiary-educated parents. The light blue bars show the difference after accounting for PISA reading performance, meaning the difference observed when comparing students with similar performance in PISA. Across all countries, 15-year-olds’ reading performance explains between 27% (in the United States) and 43% (in Denmark) of the gap in university completion rates between students from more- and less-educated families. This suggests that, while performance around the age of 15 is not a perfect predictor of future education trajectories, the cumulative effect of fewer or lost learning opportunities throughout the initial cycles of schooling is strongly related to students’ chances of surpassing their parents’ level of education.
Figure 5.4. Completed university education at age 25, by PISA reading performance and parents’ education
Notes: Results based on students’ self-reports.
All percentage-point differences are statistically significant.
Values above the country name represent the percentage of disadvantaged students who completed university by age 25.
Advantaged students are those with at least one tertiary-educated parent; disadvantaged students are those without a tertiary-educated parent.
Source: OECD, PISA 2000 and PISA 2003 Databases, Tables 5.3 and 5.4.
The link between performance at 15 and university completion has several possible explanations. One is that students who lag behind their peers in school believe that they do not have the academic skills to do well in higher education. In addition, students from disadvantaged backgrounds tend to be viewed by others as less capable of progressing in education (Croizet and Claire, 1998[11]). Poor grades and difficulties in keeping up with their classmates can reinforce the effects of negative social expectations on the students’ own perceptions of their capacity to participate in higher education (Owens and Massey, 2011[12]). It is also possible that academic performance among 15-year-old students is not directly related to decisions to pursue higher education; other factors associated with school performance, such as family income, may determine university completion rates. In this case, the observed relationship between performance in PISA and university completion should not be interpreted as causal.
Differences across education systems could also influence the relationship between performance at age 15 and attainment of higher education. In both Denmark and Switzerland, upper secondary schools offer multiple tracks that are designed to sort students based on academic ability and preferences. The highest-performing students often enrol in the academic track, while low performers typically continue with technical vocational education. However, access to university education is largely reserved for those who are selected into the academic track at the secondary level. Initial groupings based on performance can thus play a significant role in determining higher educational attainment in these types of systems (Hanushek and Woessmann, 2006[13]).
EARLY CAREER OUTCOMES AND PISA PERFORMANCE
Students from different socio-economic backgrounds often experience different school-to-work transitions. Advantaged students tend to begin working in less-intensive employment, either during secondary or post-secondary education, which gives them greater opportunity to prioritise academic performance and achievement. By contrast, disadvantaged students more commonly begin working at an earlier age and at greater intensity, which may be less conducive to attaining higher educational and career goals over the long term (Mortimer et al., 2008[14]; OECD, 2017[15]). Disadvantaged youth are more likely to accept lower-quality employment – in terms of compensation, job security and career development – placing them at higher risk of periods of unemployment and inactivity. Data on early career outcomes in this chapter show marked differences according to parents’ level of education.
Figure 5.5 shows that, in four out of five countries, significantly larger shares of students with tertiary-educated parents than students without tertiary-educated parents are enrolled in tertiary studies at the age of 25, and thus have not necessarily entered the labour market by this time.
Figure 5.5. Employment status at age 25, by socio-economic background
Notes: Results based on students’ self-reports.
Values with statistically significant differences between advantaged and disadvantaged students are displayed in bold.
Individuals who are studying can also be considered as working either part or full time, therefore the rows will not add up to 100%.
Individuals are considered to be studying at age 25 if they are enrolled in any tertiary education programme (ISCED 5B or above) at the time of the survey. The definition for Canada includes post-secondary studies (ISCED 4) as well.
Working part time is considered as employed from 1 to 20 hours per week.
Working full time is considered as employed for more than 20 hours per week.
Unemployed is defined as individuals who are not enrolled in education, have no job at the time of the survey, and are actively looking for work.
NEET is defined as individuals who are neither employed nor in education or training, and are not actively looking for work.
Advantaged students are those with at least one tertiary-educated parent; disadvantaged students are those without a tertiary-educated parent.
Source: OECD, PISA 2000 and PISA 2003 Databases, Tables 5.7, 5.13, 5.15, 5.17 and 5.21.
In Denmark, 25-year-olds with less-educated parents are more likely to be in full-time employment, while adults of the same age with tertiary-educated parents are more often employed part time while they continue with their studies. In the other countries, except the United States, similar patterns are observed, but the differences between the two groups are not statistically significant.
In all countries covered in this analysis, most 25-year-olds are either studying or working. In Denmark, larger shares of 25-year-olds with tertiary-educated parents are found in both education and part-time employment, while 25-year-olds with less-educated parents are often more likely to be NEET (neither employed nor in education or training, and not actively looking for work). In other countries, similar patterns are observed for advantaged and disadvantaged 25-year-olds, but the differences are not statistically significant.
In Australia, Canada, Denmark and the United States, 25-year-old women are significantly more likely than men of that age to be NEET. This can be related to the fact that many young women leave the labour market when they have children (OECD, 2017[16]). Similar patterns are observed in Switzerland, but the gender gap is not statistically significant. Only in Australia are first-generation immigrants significantly more likely than adults without an immigrant background to be NEET at the age of 25 (Table 5.15).
In Australia and Canada, inactivity, as measured by the NEET rate, is related to 15-year-olds’ literacy proficiency. In Canada, about 4% of students who were in the top quarter of reading performance when they were 15 are NEET at the age of 25; this is the case for 9% of students who scored in the bottom quarter of reading performance when they were 15 (Table 5.16). In Australia, 7% of students who were in the bottom quarter of performance when they were 15, but only 2% of students who were in the top quarter of performance are NEET at the age of 25. Similar relationships are observed in the other countries, but the differences are not statistically significant.
In Australia and the United States, unemployment rates reveal similar patterns. In the United States, 5% of students who were in the top quarter of PISA reading performance – but 14% of students in the bottom quarter – are unemployed at the age of 25 (Table 5.18). In Australia, 2% of students who were in the top quarter of PISA reading performance – but 6% of students in the bottom quarter – are unemployed at the age of 25. Such patterns are observed in the other countries, but are not statistically significant.
The relationships between performance at school and participation in the labour market suggest that targeting low performance during compulsory schooling may be a way to prevent social exclusion later on, particularly in countries where relatively large shares of young people are inactive or unemployed after leaving school.
When examining the percentages of students in skilled employment (a job requiring tertiary education) by quarters of performance in PISA (Table 5.20), the patterns that emerge are comparable to those concerning university completion in Figure 5.3. In Australia, only 14% of students who were in the bottom quarter of reading performance end up in skilled employment at the age of 25, while nearly 50% of students who were in the top quarter hold a skilled job at that age. In Denmark, students who were in the top quarter of performance at the age of 15 are 47 percentage points more likely than those in the bottom quarter to have a skilled job at the age of 25. Differences for the other countries considered in this chapter range from 23 to 25 percentage points. These results imply that performance during secondary school matters not only for later educational attainment, but also in shaping opportunities in the labour market during early adulthood.
Socio-economic segregation in the labour market – by which adults from less-educated families are concentrated in occupations requiring lower academic skills – is an obvious consequence of lower rates of tertiary attainment among disadvantaged students. Across countries, students with less-educated parents are between 7 and 20 percentage points less likely than students with tertiary-educated parents to be working in skilled jobs when they are 25 years old (Figure 5.6).
Figure 5.6. Skilled employment, by parents’ education and PISA reading performance
Notes: Results based on students’ self-reports.
Statistically significant percentage-point differences after accounting for reading performance are shown in light blue.
All percentage-point differences before accounting for reading performance are statistically significant.
Values above the country name represent the percentage of disadvantaged students who work in a skilled job at age 25.
Skilled employment is defined as employment that requires tertiary education, ISCED level 5A or above.
Advantaged students are those with at least one tertiary-educated parent; disadvantaged students are those without a tertiary-educated parent.
Source: OECD, PISA 2000 and PISA 2003 Databases, Tables 5.19 and 5.20.
Differences in performance at school between students from more- and less-educated families are associated with differences in access to skilled employment. When comparing students who performed similarly at the age of 15, the difference between 25-year-olds with and those without tertiary-educated parents in being employed in a skilled job shrinks to between 10 and 12 percentage points in Australia, Denmark, Switzerland and the United States, and is no longer significant in Canada. This suggests that disadvantages in learning opportunities during compulsory education are linked to early career outcomes.
Box 5.2. The Danish case: Linking PISA to PIAAC
Denmark provides a unique setting in which to study the factors that influence PISA students’ chances of success later in life. Denmark conducted the 2011-12 Survey of Adult Skills (a product of the OECD Programme for the International Assessment of Adult Competencies [PIAAC]) among a sample of students who had participated in the PISA 2000 assessment. The Survey of Adult Skills (PIAAC) measures adults’ proficiency in three key information-processing skills: literacy, numeracy and problem solving in technology-rich environments.
Longitudinal follow-ups of PISA students discussed in this chapter identify strong links between observable factors at age 15 – including cognitive proficiency, background characteristics and attitudes towards schooling – and higher education and labour market outcomes. In Denmark, it is also possible to examine how disparities in cognitive skills related to socio-economic status evolve between the ages of 15 and 26-27 by comparing performance in PISA with performance in the Survey of Adult Skills (PIAAC). While learning disparities between students of different socio-economic, cultural and demographic backgrounds have been extensively analysed in a large body of research (OECD, 2017[17]; Fruehwirth, Navarro and Takahashi, 2016[18]; Montt, 2016[19]), much less is known about the persistence of such differences into early adulthood.
About 31% of the variation in PIAAC literacy proficiency among 26-year-olds is explained by PISA performance in reading among 15-year-olds (Table 5.39a). There are similar correlations between performance in the PIAAC numeracy assessment and PISA performance in mathematics (Table 5.40a). Around 15% of the variation in literacy (and 12% in numeracy) among 26-year-olds is explained by the total number of years of education students have completed. These relationships suggest that the quality of education opportunities, and not just the quantity of education, influences how well citizens are equipped to participate in, and benefit from, increasingly knowledge-based societies.
However, earlier education is only one of the factors that shape individuals’ ability to process information and solve cognitive problems as adults. The tasks individuals engage in at work and their life experiences have, in fact, the potential to reshape cognitive capacity, because brains are “plastic” and change dramatically over a lifetime. Furthermore, differences in the attitudes of adolescents and their parents can also play a role in explaining cognitive development beyond the age of compulsory education (Chowdry, Crawford and Goodman, 2011[20]; Borgonovi et al., 2017[21]). Factors specific to family background, such as parents’ education, parents’ interest in school, cultural activities, and social communication between parents and students, also influence cognitive outcomes during school years (Borgonovi and Montt, 2012[22]; OECD, 2017[15]; Kaplan Toren, 2013[23]) and possibly beyond.
Figure 5.7 presents the percentage of variation in numeracy proficiency among 26-year-olds that can be accounted for by students’ attitudes towards learning, years of education and family background. Around 14% of the total variation in adults’ proficiency in numeracy, as measured by the PIAAC survey, is explained by attitudes towards learning reported at age 15 (Table 5.40a). Family background explains a similar percentage of the variation in performance (Table 5.40c). Accounting for performance in the PISA mathematics assessment significantly affects the strength of these relationships, suggesting that both family background and attitudes towards learning affect cognitive proficiency at age 26 by shaping learning opportunities early in life, up to age 15. In other words, neither family background nor 15-year-olds’ attitudes towards school greatly influence progress in cognitive achievement between the ages of 15 and 26 among students who scored similarly in PISA when they were 15 years old.
Figure 5.7. Variation in numeracy proficiency explained by student characteristics and educational attainment (Denmark)
Notes: Results based on students’ self-reports.
The results shown by the dark blue bars are obtained from the R squared of model 1 in Tables 5.40a, 5.40b and 5.40c.
The results shown by the light blue bars are obtained by subtracting the R squared of model 2 from the R squared of model 3 in Tables 5.40a, 5.40b and 5.40c.
Attitudes toward learning include: the index of student self-efficacy, the index of effort and perseverance, the index of interest in reading and the index of engagement in reading.
Family background variables include: the PISA index of economic, social and cultural status, the index of cultural communication, the index of social communication, the percentage of students whose father or mother helps with homework several times per month or more, and the percentage of students who reported that they discuss with their parents how well they are doing in school several times per month or more.
Source: OECD, PISA 2000 Database; PIAAC Database, Tables 5.40a, 5.40b and 5.40c.
Figure 5.8 shows that more than half of the gap in literacy proficiency between 26-year-olds with and those without tertiary-educated parents can be accounted for by reading performance at age 15. This suggests that students from less-educated families are not only less likely to perform well during secondary school, but they also face the risk of persistent poor performance and low skills. Only 5% of the gap is explained by the number of completed years of schooling (Table 5.41). This weaker relationship between educational attainment and cognitive performance in the Survey of Adult Skills (PIAAC) is partly explained by the fact that there is only limited variation in years of schooling in this sample.
Figure 5.8. Explaining disparities in literacy and numeracy proficiency in Denmark
Notes: Results based on students’ self-reports.
Advantaged 26-year olds are defined as those whose economic, social, and cultural status (ESCS) is in the top quarter; disadvantaged 26-year olds are defined as those whose economic, social, and cultural status (ESCS) is in the bottom quarter.
Values represent the percentage of the difference between advantaged and disadvantaged 26-year-olds in numeracy and literacy proficiency explained by predictors at age 15 and educational attainment at age 26.
Source: OECD, PISA 2000 Database; PIAAC Database, Table 5.41.
A similar picture emerges when considering proficiency in numeracy. Performance in PISA mathematics explains more than two-thirds of the gap in numeracy proficiency between advantaged and disadvantaged 26-year-olds, a relationship that is even stronger than that between PISA reading performance and literacy proficiency. Interestingly, differences in educational attainment account for a larger fraction of the variation in numeracy proficiency than in literacy proficiency. Proficiency in numeracy requires a complex combination of higher-order skills and subject knowledge that is mostly acquired at school, while reading skills can, arguably, be more easily acquired outside of school. This finding suggests that making opportunities to learn mathematics more equitable can have a large impact on adults’ capacity to solve problems (OECD, 2016[24]).
Overall, these results for Denmark confirm that students’ chances of succeeding later in life are shaped by a multitude of factors. Still, disparities in learning outcomes among 15-year-olds explain a considerable fraction of the variation in proficiency among 26-year-olds, which suggests that lifelong learning opportunities, while important, are no substitute for equitable learning opportunities early in life. Skills beget skills.
UNDERSTANDING THE FORCES BEHIND EDUCATIONAL MOBILITY AND SCHOOL-TO-WORK TRANSITIONS
The evidence on limited educational mobility in the previous section demands a better understanding of what it means to grow up in a disadvantaged environment. What types of inequities can be observed among 15-year-olds that are associated with their parents’ education, and how do these inequities influence higher education and early career outcomes?
The social sphere where disadvantaged students grow up differs in many ways from that enjoyed by more advantaged students (Putnam, 2015[25]). Students from disadvantaged families tend to face greater financial constraints and insecurity, live in more fragmented families, enjoy fewer opportunities to participate in cultural activities, and are exposed to less positive messages from their families, teachers and peers about the value of making an effort at school (Snellman et al., 2015[26]). This section explores three dimensions of disadvantage – student attitudes and behaviours, school characteristics and family background – all of which are related to parents’ education and can widen a divide in opportunities for social mobility by influencing cognitive and social development at school and beyond.
Understanding why students from less-educated families are less likely to pursue higher education can inform policy design. If environmental differences, rather than individual effort and inherited talent alone, determine education and career outcomes, then there is a case to be made for policy interventions that compensate for inequitable opportunities. To be effective, those interventions should target the sources of inequity. For example, if financial constraints are the main cause of early dropout among talented disadvantaged students, then establishing merit-based scholarship schemes can be an effective way to increase equity in participation in higher education (Belley and Lochner, 2007[27]). However, if the performance of disadvantaged students suffers due to peer influences, then policies that enable disadvantaged families to move to less-impoverished areas could be more effective than scholarships (Chetty and Hendren, 2015[28]).
Box 5.3. Can genes predict educational attainment?
The controversial debate of the role of nature vs. nurture in determining opportunities for success in life has attracted the attention of researchers across multiple fields – from behavioural geneticists and psychologists to sociologists and economists. One side of the argument claims that all people are born with equal capacities to succeed, and that talent is nurtured in specific environments. Others argue that people enter the world with different intellectual abilities, largely determined by genetic inheritance. Empirical evidence falls between these two extremes. Early evidence from behavioural genetics shows that academic potential is, to some extent, inherited, particularly with regard to specific outcome measures, such as adult IQ, which is about 50% heritable (Plomin et al., 2001[29]). Some evidence has also established a link between genetic endowments and educational attainment (Behrman and Taubman, 1989[30]; Behrman, Taubman and Wales, 1977[31]).
Social scientists recently shifted focus to a different method of calculating transmission coefficients from parents to natural children and to adopted children. Such studies have provided estimates of how much of the transmission of education, income or some other outcome takes place even in the absence of a genetic connection between parents and children. A study using data on a large sample of adopted Swedish children concluded that the transmission of earnings and education works strongly through both biological and environmental channels (Bjorklund, Lindahl and Plug, 2004[32]). In a subsequent study, data on Korean-American adopted children reveals somewhat less transmission from adoptive parents, yet concludes that both biological and adoptive parents transmit a great deal (Sacerdote, 2011[33]).
While empirical evidence supports a wide range of explanations for the role of genetics in life outcomes, most of these studies make clear that the nurturing environment plays an important role. Given that finding, it would be useful to identify the specific inequities in the environments in which advantaged and disadvantaged students grow up that widen gaps in educational attainment and labour market outcomes, and determine how these different types of inequities interact and accumulate.
Differences in 15-year-old students’ attitudes and behaviour
Students’ expectations
Adolescence is a time when students begin to think seriously about their future, when their aspirations become more closely aligned with their interests, abilities and the opportunities available to them, and when their vision of themselves can be influenced by the peers and adults around them (Beal and Crockett, 2010[34]; OECD, 2017[15]). For this reason, students’ aspirations for their future education and career can play a large role in determining their actual achievements (Nurmi, 2013[35]).
Fewer disadvantaged students either desire or expect to be able to attend university, and they are less likely to see themselves working in high-skilled jobs later in life (Gore et al., 2015[36]; Gale et al., 2013[37]). Yet high expectations can help disadvantaged students overcome barriers to pursuing higher education. Negative or ambivalent expectations are, instead, often associated with a sense of hopelessness (D’errico, Poggi and Correa, 2011[38]).
PISA data reveal significant differences in the expectations of 15-year-old advantaged and disadvantaged students (Chapter 2). Across the countries examined in this chapter, students with less-educated parents are between 6 and 23 percentage points more likely than students with tertiary-educated parents to expect to work in a skilled job later on in life (Table 5.9).
Longitudinal data allow for verifying the extent to which 15-year-old students hold realistic expectations for their future education and career. Table 5.10 shows that there is a mismatch between expectations and achievement. For example, 27% of students in Denmark who, at 15, did not expect to work in an occupation that requires tertiary education are, in fact, working in such an occupation when they are about 26 years old. However, in the four countries with available data, people who, as 15-year-old students, had expected to work in a high-skilled job are more likely to be doing so as young adults than those who had not held the same expectations when they were 15 (Figure 5.9). For example, in Australia, 40% of the 15-year-old students who had expected to work in a high-skilled occupation continue with their studies and are employed in such an occupation when they are 25 years old. By contrast, only 20% of Australian 15-year-old students who did not see themselves working in a high-skilled job when they were 15 are engaged in such work when they are 25.
Figure 5.9. Student expectations and skilled employment
Notes: Results based on students’ self-reports.
Statistically significant percentage-point differences after accounting for reading performance are shown in light blue.
All percentage-point differences before accounting for reading performance are statistically significant.
Data on career expectations are not available for the United States.
Source: OECD, PISA 2000 and PISA 2003 Databases, Table 5.10.
The positive relationship between expectations and achievement cannot be interpreted in causal terms, because several other student characteristics can be related to both expectations and progression through school. High-performing students tend to hold high expectations for their education and also have the intellectual capacity to complete higher education. It is possible to take some of these confounding factors into account by restricting the comparisons to students who had achieved the same level of performance at age 15. When comparing students who performed similarly in PISA, the difference between students who had high education expectations and those who had low education expectations in whether they are working in a skilled job at 25 is reduced by around a third in Canada and is no longer significant in Switzerland, but remains substantial in Australia and Denmark (Table 5.10).
At 15, students also seem to have a clear idea of what type of studies and career they would like to pursue. Table 5.12 shows that, in all countries, students who had expected a career in science, mathematics or engineering3 at the age of 15 are much more likely to hold this type of job at 25. This is especially true for boys. Girls, on the other hand, were much less likely than boys to expect a job in science, mathematics or engineering (Table 5.11). These low expectations are realised later on, with fewer women in science careers – even when comparing 15-year-old boys and girls who scored similarly on the PISA science test (Table 5.12).
The fact that differences in expectations related to socio-economic status are associated with subsequent career achievement, even after accounting for school performance, signals that both parents and educators should pay attention to how students think about their future. The parents and teachers of more advantaged students tend to have higher expectations for those students, which, in turn, shape the students’ own expectations (Buchmann and Dalton, 2002[39]; Sewell et al., 2003[40]). Academic and career counselling targeted to disadvantaged students may help these students develop expectations that are better aligned with their academic potential.
Effort and perseverance
Individual effort and perseverance have proven to be strong predictors of academic performance and higher levels of educational attainment (Chamorro-Premuzic and Furnham, 2003[41]; Trapmann et al., 2007[42]). Yet PISA data and other research have revealed significant disparities in effort and perseverance across students of different socio-economic status (OECD, 2013[43]).
It is likely that, with fewer resources and greater constraints, disadvantaged students are more likely to be discouraged. Even if disadvantaged students work hard, their education outcomes may not improve or be rewarded as much as those of other students, so they have less incentive to invest effort in their studies (Deluca and Rosenbaum, 2001[44]).
Figure 5.10. University completion rates, by effort and perseverance
Notes: Results based on students’ self-reports.
All percentage-point differences are statistically significant.
Comparable measures for effort and perseverance were not available for Australia or Canada.
Index of effort and perseverance from PISA 2000 survey was used, for Denmark and Switzerland, to identify 15-year-old students within the top and bottom quarters of effort and perseverance. Data from the United States provide a separate but comparable index of effort and perseverance, which was used to identify 15-year-old students within the top and bottom quarters.
Source: OECD, PISA 2000 and PISA 2003 Databases, Table 5.25.
The PISA index of effort and perseverance was derived using students’ responses to a series of questions about how they handle difficulty while studying. Students were asked how often they work as hard as possible; they keep working even when the material is difficult; they try to do their best to acquire the knowledge and skills taught; and they put forth their best effort. Students responded to each item in one of four ways, ranging from “almost never” to “almost always”.
Students who invest greater effort and perseverance (students in the top quarter of the index of effort and perseverance) are more likely to complete university than students who invest less effort and perseverance (students in the bottom quarter of the index). In Denmark, 35% of students who had reported high levels of perseverance, but only 19% of students who had reported low perseverance, had completed university by the age of 25. In Switzerland, 23% of highly perseverant students versus 11% of the least-perseverant students completed university; in the United States, 47% of highly perseverant students versus 26% of the least-perseverant students completed university by the age of 25. Even after accounting for parents’ education and student performance in reading, individual effort and perseverance explain a significant share of the difference in university completion rates across the three countries with available data (Table 5.25).
Other attitudes towards learning are also related to educational achievement. The 2000 round of PISA included an index of perceived self-efficacy, derived from students’ responses to a series of questions regarding their level of confidence in their academic abilities. Students were asked to report how often they are certain they can understand the most difficult material in the text; they are confident they can do an excellent job on assignments and tests; and they are certain they can master the skills being taught.
In Denmark and Switzerland, 15-year-old students without tertiary-educated parents reported lower levels of self-efficacy (Table 5.25) than their peers with tertiary-educated parents; and students with low self-efficacy (those who fall within the bottom quarter of the index) during secondary school were less likely to complete university than students with high self-efficacy. Yet, after accounting for PISA reading performance and parents’ education, the difference is significantly reduced in Denmark and no longer significant in Switzerland. This suggests that individual attitudes towards learning are strongly linked to academic performance and family characteristics. It is likely that confidence, effort and perseverance are more critical for students with less-educated parents, who often endure greater hardships to achieve the same outcomes as their peers from more advantaged backgrounds.
This evidence complements a growing body of literature that emphasises the importance of developing social skills and positive attitudes, both for cognitive growth and for longer-term labour market and social outcomes (Bowles, Gintis and Osborne, 2001[45]; Heckman, Stixrud and Urzua, 2006[46]). Research has suggested that non-cognitive skills, including attitudes and behaviours, are considerably less malleable at later ages (Cunha and Heckman, 2007[47]).
From a policy perspective, this highlights how teachers, schools and institutions can nurture disadvantaged students’ attitudes, aspirations and motivations. Teachers can help students develop academic mindsets and learning strategies that cultivate perseverance. Specific initial education and training programmes can help teachers identify and address lack of persistence and poor self-confidence among their students. These initiatives may be particularly relevant for teachers working in schools with large populations of disadvantaged students.
Involvement in cultural activities
Differences in the social environments surrounding advantaged and disadvantaged students can result not only in different attitudes towards schools but also in a different exposure to intellectually and culturally stimulating experiences. Exposure to the arts, music and other cultural expressions may be a particularly crucial form of socialisation during adolescence, influencing both early performance and attitudes at school, and later educational attainment and career outcomes.
PISA provides two measures for exploring differences in cultural experiences: an index of cultural activity (representing the incidence of 15-year-old students’ participation in various cultural activities over the previous year), and an index of cultural communication (representing parents’ engagement with students in cultural activities).
Research shows that cultural capital is distributed unequally across social classes and education, with the largest share enjoyed by wealthier and more highly educated individuals. Cultural capital is also something that is transmitted from parents to children, and primarily at home rather than in school (Sullivan, 2001[48]). Yet exposure to and participation in cultural activities can help students find meaning in what they do at school and appreciate the value of acquiring more education.
Figure 5.11. University completion rates, by frequency of cultural activity, parents’ education and PISA reading performance
Notes: Results based on students’ self-reports.
All percentage-point differences are statistically significant.
Comparable measures for cultural activity were not available for Australia or the United States.
Students engaged in frequent (infrequent) cultural activity are students in the top (bottom) quarter of the index of cultural activity.
Source: OECD, PISA 2000 Database, Table 5.28.
Fifteen-year-old students with tertiary-educated parents are significantly more likely than their peers with less-educated parents to have access to cultural activities (Table 5.28). For example, in Denmark and Switzerland, about one in four children of tertiary-educated parents reported that they had visited a museum or art gallery at least three or four times in the previous year; only one in ten children from less-educated families so reported. In Canada, students in the top quarter of the index of cultural activity are 26 percentage points more likely to complete university than students in the bottom quarter of the index. In Denmark, the gap in university completion rates between these two groups is 23 percentage points; in Switzerland, it is 17 percentage points.
When comparing students with similar levels of performance in the PISA reading assessment and whose parents have attained similar levels of education, the magnitude of this difference in university completion in Canada shrinks, but is still large (12 percentage points). In Denmark, the difference falls to 14 percentage points, and in Switzerland, it drops to 8 percentage points.
Tertiary-educated parents are more likely than less-educated parents to engage in cultural activities and conversations with their children (OECD, 2017[15]). For example, in Denmark and Switzerland, about one in two students with tertiary-educated parents reported that they discuss social and political issues with their parents several times per month (Table 5.27). By contrast, only around one in four students with less-educated parents so reported. In Denmark, around 40% of students who reported frequent cultural exchanges with their parents (those in the top quarter of the index of cultural communication) complete university education; only 14% of students who rarely engage in this type of communication with their parents (those in the bottom quarter of the index) reach that level of education. In Canada and Switzerland, gaps between these two groups of students range from 13 to 26 percentage points.
After accounting for parents’ education and PISA reading performance, gaps in university completion rates fall to 11 percentage points in Canada and 10 percentage points in Denmark, and are no longer significant in Switzerland (Table 5.27).
While not implying a causal relationship, these results suggest that cultural activities may be an additional mechanism through which more-educated families ensure an educational advantage for their children outside of school. Cultural activity can be viewed as yet another factor that explains differences between advantaged and disadvantaged students in socialisation and education opportunities.
Differences in family background
Family structure
Both falling marriage rates and increasing divorce rates have contributed to the increase in single-parent families (OECD, 2016[24]). Many studies have documented the challenges confronted by single parents and the disadvantages their children face relative to children raised in two-parent households (Mandara and Murray, 2006[49]). Children from single-parent homes tend to score lower on cognitive tests and complete fewer years of school when compared to children from two-parent homes (Milne et al., 1986[50]; Sigle-Rushton et al., 2004[51]).
Previous evidence from PISA has shown that family structure is related to performance at school (OECD, 2013[52]). Across OECD countries, the performance gap in the PISA 2012 mathematics assessment between students from single-parent families and those from other types of families was 15 score points – or the equivalent of almost half a year of schooling – before taking socio-economic status into account. In most countries, single-parent families tend to have lower socio-economic status than two-parent families (OECD, 2013[52]). After accounting for socio-economic status, the difference in PISA performance is reduced to five score points.
In Australia, 42% of students from two-parent families complete university while only 26% of students from single-parent families attain this level of education (Table 5.33). However, differences in other countries are much smaller, ranging from 7 to 8 percentage points, and are no longer significant after accounting for parents’ education and PISA reading performance. This suggests that family structure does not have the same influence on educational achievement in all countries, possibly because of differences in social and economic policies. Moreover, family structure may be related to academic performance up to age 15, but does not necessarily have a significant direct link to later decisions regarding further education and career choice. Yet in Australia, these gaps narrow only modestly when comparing students who perform similarly in PISA and whose parents have similar levels of education. While many single parents are perfectly capable of providing the support their children need to succeed in school and life, some may face difficulties as they act as both the primary care provider and income earner.
School systems and individual schools can consider how and what kinds of parental engagement are to be encouraged among single parents who have limited time to devote to school activities (Pong, Dronkers and Hampden-Thompson, 2003[53]). Given that single parents often struggle with limited time and financial resources, it is particularly important to evaluate the synergies that can be established between education policies and other policies, such as those related to welfare and childcare. It may also be important to identify the different challenges that are faced by mother-only versus father-only households (Nonoyama-Tarumi, 2017[54]) in order for schools to know how best to respond to individual circumstances.
Family wealth
Parents’ low education is often associated with less family wealth. Research in Sweden reveals that inequality in family wealth – even in a comparatively egalitarian context – has profound consequences for the distribution of opportunity across multiple generations (Hällsten and Pfeffer, 2017[55]). Parents’ wealth is strongly associated with a range of outcomes in early adulthood, and these associations are found to be stronger than those related to parents’ education in many cases (Karagiannaki, 2012[56]). Apart from the ability to afford more quality education and educational resources, family wealth also offers a level of security that cannot necessarily be substituted with financial aid for education. Students in wealthier families can focus on the academic, rather than the financial, challenges of higher education, and pay less attention to the short-term opportunity costs of pursuing additional years of schooling (Braga et al., 2017[57]).
The PISA index of family wealth was derived from students’ reports about the availability of various wealth-related possessions in their home. These questions identified whether a student has a room of his or her own, educational software, Internet access, and a dishwasher; and the number of cellular phones, televisions, computers, cars and bathrooms at home. For the countries with available measures of wealth, high-wealth students (students in the top quarter of the family wealth index) are between 8 and 16 percentage points more likely than low-wealth students (students in the bottom quarter of the family wealth index) to complete university (Figure 5.12). In Switzerland, the difference is no longer significant after accounting for parents’ education and performance in the PISA reading assessment. However, these gaps remain substantial in Canada and Denmark, suggesting that wealth can play an important role in both academic performance and educational attainment in certain settings.
Figure 5.12. University completion rates, by family wealth
Notes: Results based on students’ self-reports.
Statistically significant percentage-point differences after accounting for parents’ education and PISA reading performance are shown in light blue.
All percentage-point differences before accounting for parent’s education and PISA reading performance are statistically significant.
Data for comparable family-wealth indices were not available for Australia or the United States.
Source: OECD, PISA 2000 and PISA 2003 Databases, Table 5.26.
Looking at parents’ employment status is another way to identify the links between family resources and student outcomes. Students can face significant hardships when their parents are unemployed. They may perform poorly in school, be at risk of repeating a grade, and are less likely to finish secondary education (Mooi-Reci and Bakker, 2015[58]). These disadvantages tend to persist beyond the early years of schooling and can influence students’ chances of attaining higher levels of education and succeeding in the labour market. In Australia, Canada and Denmark, 15-year-old disadvantaged students are more likely than advantaged students to have unemployed parents (neither parent is employed because of unemployment or inactivity). Later outcomes reveal that 15-year-old students whose parents were unemployed at that time are between 12 and 13 percentage points less likely than students the same age whose parents were employed when their child was 15 to complete university by the age of 25 (Table 5.34). These gaps are no longer significant when comparing outcomes among students with similar PISA reading performance and parents’ education.
Inactivity of both parents is relatively rare for this sample of countries (only about 5%, on average, of the students considered in this chapter); thus it is difficult to estimate its precise relationship to student outcomes later in life. In addition, parents’ employment status and other family characteristics, such as low family wealth or coming from a single-parent household, are likely to be strongly correlated with performance outcomes observed at age 15. These indirectly influence later outcomes, and thus explain why certain disparities become insignificant after accounting for student performance. While this analysis provides a preliminary consideration of how family characteristics can influence educational attainment and early career achievement, it cannot fully identify the extent to which gaps in outcomes can be attributed to the direct or indirect effects.
Differences in school characteristics
School composition
An important factor behind limited educational mobility is residential segregation, whereby wealth and poverty are concentrated in particular geographic locations and neighbourhoods. Residential segregation is often accompanied by school segregation, where students from less-advantaged households are more likely both to attend lower-quality schools and be grouped with similarly disadvantaged peers. Schools in poorer neighbourhoods often suffer from limited resources, larger classes, inexperienced teachers and an inability to retain staff – all of which create fewer opportunities for students to excel. Teachers in disadvantaged schools tend to hold low expectations of their students, which reinforce the low expectations that students and their parents may already hold (Sparkes, 1999[59]). Some researchers (Wodtke, Harding and Elwert, 2011[60]; Crowder and South, 2011[61]) show that the fraction of childhood spent in high-poverty areas is negatively correlated with outcomes such as high school completion. Others (Chetty and Hendren, 2015[28]) have studied more than five million families that relocated and find that living in a poor neighbourhood has adverse effects on children’s outcomes.
Figure 5.13 shows that students with tertiary-educated parents who had attended disadvantaged schools (defined as schools where the share of students with tertiary-educated parents is in the bottom quarter of the national distribution) are slightly less likely to complete university than students with tertiary-educated parents who had attended advantaged schools (defined as schools where the share of students with tertiary-educated parents is in the top quarter of the national distribution), although most of these differences are statistically insignificant. This difference is marked in Australia, while it is almost negligible in Denmark.
Figure 5.13. University completion rates among advantaged students, by school composition and student performance
Notes: Results based on students’ self-reports.
Statistically significant percentage-point differences before accounting for reading performance are shown in a darker tone.
No percentage-point differences after accounting for reading performance are statistically significant.
Comparable school-level data were not available for the United States.
School composition is derived using shares of advantaged and disadvantaged students within each school to identify schools that fall in the top (advantaged) and bottom (disadvantaged) quarters.
Advantaged students are those with at least one tertiary-educated parent; disadvantaged students are those without a tertiary-educated parent.
Source: OECD, PISA 2000 and PISA 2003 Databases, Table 5.29.
The socio-economic composition of schools is more strongly related to disadvantaged students’ progression through school (Figure 5.14). In Australia, students with less-educated parents who had attended disadvantaged schools are 23 percentage points less likely to complete university than students with less-educated parents who had attended advantaged schools. In Canada, there is a 22 percentage-point difference between the two groups of students; in Switzerland, there is a 15 percentage-point difference.
Figure 5.14. University completion rates among disadvantaged students, by school composition and student performance
Notes: Results based on students’ self-reports.
Statistically significant percentage-point differences before accounting for reading performance are shown in a darker tone.
No percentage-point differences after accounting for reading performance are statistically significant.
Comparable school-level data were not available for the United States.
School composition is derived using shares of advantaged and disadvantaged students within each school to identify schools that fall in the top (advantaged) and bottom (disadvantaged) quarters.
Advantaged students are those with at least one tertiary-educated parent; disadvantaged students are those without a tertiary-educated parent.
Source: OECD, PISA 2000 and PISA 2003 Databases, Table 5.29.
In Australia, Canada and Denmark, significantly more students with tertiary-educated parents than students without complete university studies after attending disadvantaged secondary schools (Table 5.29). Among advantaged students who had attended disadvantaged schools, between 37% (Denmark) and 46% (Australia) complete university by age 25; but only between 15% (Denmark) and 22% (Australia) of disadvantaged students who had attended disadvantaged schools complete university by 25. It is possible that, for students with tertiary-educated parents, a number of other beneficial factors, including home environment, financial resources and family support compensate for different school environments. Students of less-educated parents, with fewer family resources and supplemental education opportunities, may be more sensitive to school quality and composition.
Peer expectations within schools
At the school level, peer expectations can also have a particularly strong influence both on a student’s own expectations and on academic achievement during secondary school (Sacerdote, 2011[62]). Students from low-income, less-educated households may be especially susceptible to peer influence during adolescence, particularly if these students lack nurturing experiences and mentors outside of school. As previously shown, students’ expectations play a key role in influencing later achievement. Peer expectations may influence students’ own expectations and thus their educational and social mobility.
In Australia, Denmark and Switzerland, students in advantaged schools are between 13 and 20 percentage points more likely to expect to work in a skilled occupation than students in disadvantaged schools (Table 5.30). These gaps become insignificant, in all countries except Switzerland, after accounting for school-level performance in reading. A possible explanation is that school performance reflects a wide range of factors associated with school quality, such as teacher quality, school resources and the learning environment – all of which are likely to affect education expectations within a particular school. Yet in Switzerland, some differences in students’ expectations between advantaged and disadvantaged schools are observed even when comparing schools that perform similarly.
Figure 5.15 shows that students who had attended schools with high student expectations (defined as those schools where the percentage of students who expect skilled employment is higher than the country average) are more likely to complete university than students who had attended schools with low student expectations (those schools where the percentage of students who expect skilled employment is lower than the country average). Apart from Denmark, this relationship between peer expectations and university completion remains significant even after accounting for school performance.
Figure 5.15. University completion rates, by peer expectations and school performance
Notes: Results based on students’ self-reports.
Statistically significant percentage-point differences after accounting for school performance are shown in light blue.
All percentage-point differences before accounting for school performance are statistically significant.
Comparable school-level data were not available for the United States.
Schools with high (low) expectations are those schools where the percentage of students who expect high-skilled employment is significantly higher (lower) than the country average.
Source: OECD, PISA 2000 and PISA 2003 Databases, Table 5.30.
When students with low expectations are grouped together in the same learning environment, their attitudes towards schooling and perceptions of future potential are reinforced. Research in sociology suggests that aspirations for further education tend to be higher when students attend a school where the average socio-cultural intake is also higher (Dupriez et al., 2012[63]). Though these results do not imply a causal link, they highlight the importance of considering the possible effects of school composition and social stratification on educational mobility.
The analysis of longitudinal data for a limited number of countries reveals that the difficulties disadvantaged students must overcome in order to move up the education ladder are numerous and varied, involving a combination of individual, family, school and even country-level factors. Therefore, it is crucial to identify these factors, and their contribution to the observed gaps in academic performance and educational mobility, in order to design efficient and integrated policies that address the needs of disadvantaged students. Greater efforts to develop longitudinal studies, or to link national and international assessments with administrative records, may prove a worthwhile investment in order to better understand how upward educational mobility works.
References
Beal, S. and L. Crockett (2010), “Adolescents’ Occupational and Educational Aspirations and Expectations: Links to High School Activities and Adult Educational Attainment”, Developmental Psychology, Vol. 46/1, pp. 258-265, http://dx.doi.org/10.1037/a0017416. [34]
Behrman, J. and P. Taubman (1989), Is Schooling Mostly in the Genes? Nature-Nurture Decomposition Using Data on Relatives, The University of Chicago Press, http://dx.doi.org/10.2307/1833246. [30]
Behrman, J., P. Taubman and T. Wales (1977), Controlling for and measuring the effects of genetics and family environment in equations for schooling and labor market success. Kinometrics: Determinants of Socioeconomic Success Within and Between Families., North-Holland Pub. Co, https://www.researchgate.net/publication/313444630_Controlling_for_and_measuring_the_effects_of_genetics_and_family_environment_in_equations_for_schooling_and_labor_market_success (accessed on 15 February 2018). [31]
Belley, P. and L. Lochner (2007), “The Changing Role of Family Income and Ability in Determining Educational Achievement”, Journal of Human Capital, Vol. 1/1, pp. 37-89, http://dx.doi.org/10.1086/524674. [27]
Bjorklund, A., M. Lindahl and E. Plug (2004), “Intergenerational Effects in Sweden: What Can We Learn from Adoption Data?”, IZA Discussion Paper No. 1194, https://papers.ssrn.com/sol3/papers.cfm?abstract_id=565401 (accessed on 15 February 2018). [32]
Borgonovi, F. and G. Montt (2012), “Parental Involvement in Selected PISA Countries and Economies”, OECD Education Working Papers, No. 73, OECD Publishing, Paris, http://dx.doi.org/10.1787/5k990rk0jsjj-en. [22]
Borgonovi, F. et al. (2017), “Youth in Transition: How do some of the cohorts participating in PISA fare in PIAAC?”, OECD Education Working Papers, No. 155, OECD Publishing, Paris, http://dx.doi.org/10.1787/19939019. [21]
Bowles, S., H. Gintis and M. Osborne (2001), “The Determinants of Earnings: A Behavioral Approach”, Journal of Economic Literature, Vol. 39/4, pp. 1137-1176, http://dx.doi.org/10.1257/jel.39.4.1137. [45]
Braga, B. et al. (2017), Wealth Inequality Is a Barrier to Education and Social Mobility, Urban Institute, Washington, DC, http://hdl.voced.edu.au/10707/444950. [57]
Buchmann, C. and B. Dalton (2002), “Interpersonal Influences and Educational Aspirations in 12 Countries: The Importance of Institutional Context”, Sociology of Education, Vol. 75/2, pp. 99-122, http://dx.doi.org/10.2307/3090287. [39]
Chamorro-Premuzic, T. and A. Furnham (2003), “Personality traits and academic examination performance”, European Journal of Personality, Vol. 17/3, pp. 237-250, http://dx.doi.org/10.1002/per.473. [41]
Chetty, R. and N. Hendren (2015), “The Effects of Exposure to Better Neighborhoods on Children: New Evidence from the Moving to Opportunity Experiment”, NBER Working Paper, No. 21156, http://dx.doi.org/10.3386/w21156. [28]
Chowdry, H., C. Crawford and A. Goodman (2011), “The role of attitudes and behaviours in explaining socio-economic differences in attainment at age 16”, Longitudinal and Life Course Studies, Vol. 2/1, pp. 59-76, http://www.llcsjournal.org/index.php/llcs/article/viewFile/141/119 (accessed on 02 March 2018). [20]
Croizet, J. and T. Claire (1998), “Extending the Concept of Stereotype Threat to Social Class: The Intellectual Underperformance of Students From Low Socioeconomic Backgrounds”, Personality and Social Psychology Bulletin, Vol. 24/6, pp. 588-594, https://doi.org/10.1177%2F0146167298246003. [11]
Crowder, K. and S. South (2011), “Spatial and Temporal Dimensions of Neighborhood Effects on High School Graduation”, Social Science Research, Vol. 40/1, pp. 87-106, http://dx.doi.org/10.1016/j.ssresearch.2010.04.013. [61]
Cunha, F. and J. Heckman (2007), “The Technology of Skill Formation”, American Economic Review, Vol. 97/2, pp. 31-47, http://dx.doi.org/10.1257/aer.97.2.31. [47]
Deluca, S. and J. Rosenbaum (2001), “Individual Agency and the Life Course: Do Low-SES Students Get Less Long-Term Payoff for Their School Efforts?”, Sociological Focus, Vol. 34:4, pp. 357-376, https://doi.org/10.1080/00380237.2001.10571208. [44]
D’Errico, F., I. Poggi and L. Correa (2011), “School and life for teenagers. Expectations and hopes in Italy and Brazil”, International Journal of Developmental and Educational Psychology, Vol. 1/2, pp. 433-442, www.redalyc.org/articulo.oa?id=349832329036. [38]
Downey, D., P. Hippel and B. Broh (2004), “Are Schools the Great Equalizer? School and Non-School Sources of Inequality in Cognitive Skills”, American Sociological Review, Vol. 69/5, pp. 613-635. [5]
Dupriez, V. et al. (2012), “Social Inequalities of Post-secondary Educational Aspirations: Influence of social background, school composition and institutional context”, European Educational Research Journal, Vol. 11/4, pp. 504-519, http://dx.doi.org/10.2304/eerj.2012.11.4.504. [63]
Fruehwirth, J., S. Navarro and Y. Takahashi (2016), “How the Timing of Grade Retention Affects Outcomes: Identification and Estimation of Time-Varying Treatment Effects”, Journal of Labor Economics, Vol. 34/4, pp. 979-1021, http://dx.doi.org/10.1086/686262. [18]
Gale, T. et al. (2013), Student Aspirations for Higher Education in Central Queensland: A Survey of Students’ Navigational Capacities, Centre for Research in Education Futures and Innovation (CREFI), Deakin University, Melbourne, Australia. [37]
Gore, J. et al. (2015), “Socioeconomic status and the career aspirations of Australian school students: Testing enduring assumptions”, Australian Educational Researcher, Vol. 42/2, pp. 155-177, http://dx.doi.org/10.1007/s13384-015-0172-5. [36]
Hällsten, M. and F. Pfeffer (2017), “Grand Advantage: Family Wealth and Grand Children’s Educational Achievement in Sweden”, American Sociological Review, Vol. 82/2, pp. 328-360, http://dx.doi.org/10.1177/0003122417695791. [55]
Hanushek, E. and L. Woessmann (2006), “Does Educational Tracking Affect Performance and Inequality? Difference-In-Differences Evidence Across Countries”, The Economic Journal, Vol. 116/510, pp. C63-C76, https://doi.org/10.1111/j.1468-0297.2006.01076.x. [13]
Hanushek, E., L. Woessmann and L. Zhang (2011), “General Education, Vocational Education, and Labor-Market Outcomes over the Life-Cycle”, NBER Working Paper, No. 17504, http://dx.doi.org/10.3386/w17504. [9]
Heckman, J., J. Stixrud and S. Urzua (2006), “The Effects of Cognitive and Noncognitive Abilities on Labor Market Outcomes and Social Behavior”, NBER Working Paper, No. 12006, http://dx.doi.org/10.3386/w12006. [46]
Hsieh, C. et al. (2013), “The Allocation of Talent and U.S. Economic Growth”, NBER Working Paper, No. 18693, http://dx.doi.org/10.3386/w18693. [4]
Kaplan Toren, N. (2013), “Multiple Dimensions of Parental Involvement and its Links to Young Adolescent Self-Evaluation and Academic Achievement”, Psychology in the Schools, Vol. 50/6, pp. 634-649, http://dx.doi.org/10.1002/pits.21698. [23]
Karagiannaki, E. (2012), “The effect of parental wealth on children’s outcomes in early adulthood”, CASEpaper 164, Centre for Analysis of Social Exclusion, London School of Economics. [56]
Mandara, J. and C. Murray (2006), “Father’s Absence and African American Adolescent Drug Use”, Journal of Divorce & Remarriage, Vol. 46/1-2, pp. 1-12, http://dx.doi.org/10.1300/J087v46n01_01. [49]
Milne, A. et al. (1986), “Single Parents, Working Mothers, and the Educational Achievement of School Children”, Sociology of Education, Vol. 59/3, pp. 125-139, http://dx.doi.org/10.2307/2112335. [50]
Montt, G. (2016), “Are Socioeconomically Integrated Schools Equally Effective for Advantaged and Disadvantaged Students?”, Comparative Education Review, Vol. 60/4, pp. 808-832, http://dx.doi.org/10.1086/688420. [19]
Mooi-Reci, I. and B. Bakker (2015), “Parental Unemployment: How Much and When Does it Matter for Children’s Educational Attainment?”, Life Course Centre Working Paper Series, Vol. 2015/03. [58]
Mortimer, J. et al. (2008), “Tracing the Timing of “Career” Acquisition in a Contemporary Youth Cohort”, Work and Occupations, Vol. 35/1, pp. 44-84, http://www.ncbi.nlm.nih.gov/pubmed/18542713 (accessed on 14 February 2018). [14]
Nonoyama-Tarumi, Y. (2017), “Educational Achievement of Children From Single-Mother and Single-Father Families: The Case of Japan”, Journal of Marriage and Family, Vol. 79, pp. 915-931, http://dx.doi.org/10.1111/jomf.12409. [54]
Nunn, A. et al. (2007), “Factors influencing social mobility”, Research Report, No. 450, Department for Work and Pensions, United Kingdom. [1]
Nurmi, J. (2013), “Socialization and Self-Development: Channeling, Selection, Adjustment, and Reflection”, in Handbook of Adolescent Psychology, John Wiley & Sons, Inc., http://dx.doi.org/10.1002/9780471726746.ch4. [35]
OECD (2017), Education at a Glance 2017: OECD Indicators, OECD Publishing, Paris, http://dx.doi.org/10.1787/eag-2017-en. [7]
OECD (2017), Educational Opportunity for All: Overcoming Inequality throughout the Life Course, Educational Research and Innovation, OECD Publishing, Paris, http://dx.doi.org/10.1787/9789264287457-en. [17]
OECD (2017), PISA 2015 Results (Volume III): Students’ Well-Being, PISA, OECD Publishing, Paris, http://dx.doi.org/10.1787/9789264273856-en. [15]
OECD (2017), The Pursuit of Gender Equality - An Uphill Battle, OECD Publishing, Paris, http://dx.doi.org/10.1787/9789264281318-en. [16]
OECD (2016), PISA 2015 Results (Volume I): Excellence and Equity in Education, PISA, OECD Publishing, Paris, http://dx.doi.org/10.1787/9789264266490-en. [10]
OECD (2016), Society at a Glance 2016: OECD Social Indicators, OECD Publishing, Paris, http://dx.doi.org/10.1787/9789264261488-en. [24]
OECD (2013), PISA 2012 Results: Excellence through Equity (Volume II): Giving Every Student the Chance to Succeed, PISA, OECD Publishing, Paris, http://dx.doi.org/10.1787/9789264201132-en. [52]
OECD (2013), PISA 2012 Results: Ready to Learn (Volume III): Students’ Engagement, Drive and Self-Beliefs, PISA, OECD Publishing, Paris, http://dx.doi.org/10.1787/9789264201170-en. [43]
OECD (2010), Improving Health and Social Cohesion through Education, Educational Research and Innovation, OECD Publishing, Paris, http://dx.doi.org/10.1787/9789264086319-en. [6]
Ololube, N., L. Onyekwere and C. Agbor (2015), “Social Stratification and Mobility: How Socio-Economic Status of Family Affects Children’s Educational Development and Management”, Education, Vol. 5/4, pp. 111-122, http://dx.doi.org/10.5923/j.edu.20150504.03. [2]
Owens, J. and D. Massey (2011), “Stereotype threat and college academic performance: A latent variables approach”, Social Science Research, Vol. 40/1, pp. 150-166, http://dx.doi.org/10.1016/j.ssresearch.2010.09.010. [12]
Plomin, R. et al. (2001), Behavioral Genetics, Worth Publishers, New York. [29]
Pong, S., J. Dronkers and G. Hampden-Thompson (2003), “Family policies and children’s school achievement in single- versus two-parent families”, Journal of Marriage and the Family, Vol. 65/19, pp. 681-699, https://pure.york.ac.uk/portal/en/publications/family-policies-and-childrens-school-achievement-in-single-versus-twoparent-families(9672f21f-51f8-4cd1-93ba-7539430458d4).html (accessed on 15 February 2018). [53]
Putnam, R. (2015), Our Kids: the American Dream in Crisis, Simon and Schuster, New York, NY. [25]
Sacerdote, B. (2011), “Nature and nurture effects on children’s outcomes: What have we learned from studies of twins and adoptees?”, Handbook of Social Economics, Vol. 1, pp.1-30, http://dx.doi.org/10.1016/B978-0-444-53187-2.00001-2. [33]
Sacerdote, B. (2011), “Peer Effects in Education: How might they work, how big are they and how much do we know thus Far?”, Handbook of the Economics of Education, Vol. 3, pp. 249-277, http://dx.doi.org/10.1016/B978-0-444-53429-3.00004-1. [62]
Schaefer, R. (2005), Sociology, McGraw-Hill, https://books.google.fr/books/about/Sociology.html?id=xYHq2vxI4QAC&redir_esc=y (accessed on 14 February 2018). [3]
Schoenfeld, A. (2002), “Making Mathematics Work for All Children: Issues of Standards, Testing, and Equity”, Educational Researcher, Vol. 31/1, pp. 13-25, https://gse.berkeley.edu/sites/default/files/users/alan-h.-schoenfeld/Schoenfeld_2002%20Making%20Math%20Work%20ER.pdf (accessed on 24 July 2018). [8]
Sewell, W. et al. (2003), “As We Age: A Review of the Wisconsin Longitudinal Study, 1957–2001”, Research in Social Stratification and Mobility, Vol. 20, pp. 3-111, http://dx.doi.org/10.1016/S0276-5624(03)20001-9. [40]
Sigle-Rushton, W. et al. (2004), Father Absence and Child Wellbeing: A Critical Review. The future of the family, Russell Sage Foundation, New York, http://eprints.lse.ac.uk/9589/ (accessed on 15 February 2018). [51]
Snellman, K. et al. (2015), “The Engagement Gap”, The ANNALS of the American Academy of Political and Social Science, Vol. 657/1, pp. 194-207, http://dx.doi.org/10.1177/0002716214548398. [26]
Sparkes, J. (1999), “Schools, Education and Social Exclusion”, CASEpaper 29, Centre for Analysis of Social Exclusion, London School of Economics, http://eprints.lse.ac.uk/6482/1/Schools,_Education_and_Social_Exclusion.pdf (accessed on 15 February 2018). [59]
Sullivan, A. (2001), “Cultural Capital and Educational Attainment”, Sociology, Vol. 35/04, pp. 893-912, http://dx.doi.org/10.1017/S0038038501008938. [48]
Trapmann, S. et al. (2007), “Meta-Analysis of the Relationship Between the Big Five and Academic Success at University”, Zeitschrift für Psychologie / Journal of Psychology, Vol. 215/2, pp. 132-151, http://dx.doi.org/10.1027/0044-3409.215.2.132. [42]
Wodtke, G., D. Harding and F. Elwert (2011), “Neighborhood Effects in Temporal Perspective: The Impact of Long-Term Exposure to Concentrated Disadvantage on High School Graduation”, American Sociological Review, Vol. 76/5, pp. 713-736, http://dx.doi.org/10.1177/0003122411420816. [60]
Notes
← 1. Upward mobility is one form of mobility to be explored in this analysis. Examining patterns regarding downward mobility and immobility are also possible with these data, but are not the focus of the analysis in this chapter.
← 2. The estimates in Figure 5.2 are presenting percentage-point differences between advantaged and disadvantaged students. This is not intended to identify the most- and least-equitable countries in this study, but rather highlight the inequities in educational mobility across socio-economic status. Odds ratios or risk ratios are required for comparing countries based on equitable outcomes.
← 3. In this chapter, STEM jobs are defined as jobs requiring tertiary education in the fields of science, technology, engineering and mathematics; they do not include healthcare-related employment.