For Good Measure
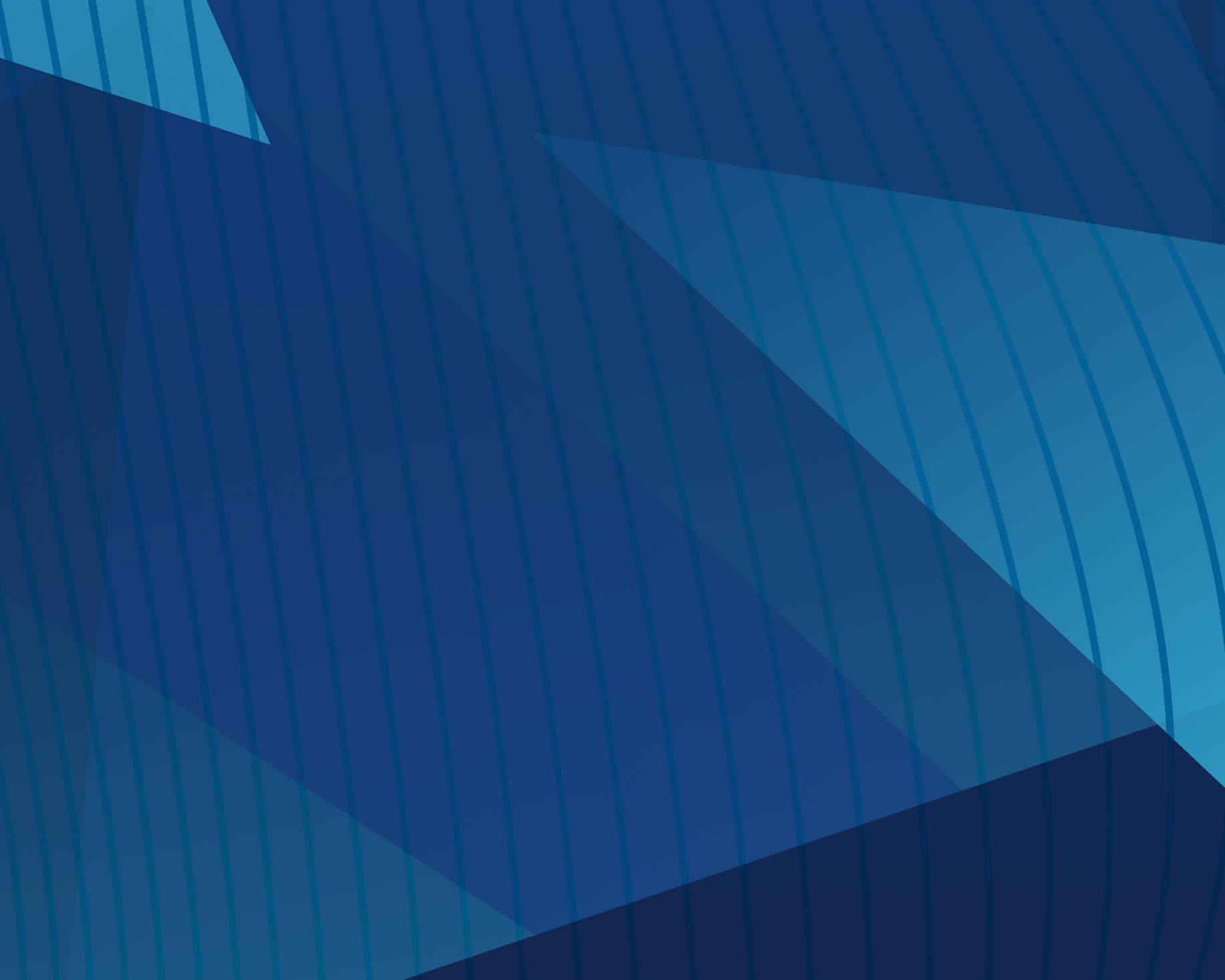
Chapter 8. Economic security
Abstract
This chapter examines what we know about economic security and what we still must learn. It defines economic security as vulnerability to economic loss, and treats “economic” as a description of the consequences (such as income loss) rather than the causes of insecurity. The chapter presents a series of recommendations for researchers and statistical agencies that would permit better measurement and analysis of economic security, both as a fundamental feature of economic life and as a major influence on subjective well-being and economic behaviour. It synthesises state-of-the-art theory and evidence regarding economic security and then lays out a small number of relatively well-developed measures. Based on this review and on the best available data, the chapter provides some preliminary evidence about the evolution of economic security in developed economies. The chapter then describes some of the continuing shortcomings of existing statistics in this field, and suggests ways to improve them.
Jacob S. Hacker is Director of the Institution for Social and Policy Studies and Stanley B. Resor Professor of Political Science at Yale University. The author wishes to thank Austin Nichols and especially Philipp Rehm – a true partner in conducting much of the research on which this chapter is based – as well as participants in the HLEG workshop on “Economic Insecurity: Forging an Agenda for Measurement and Analysis” held in New York, United States on 4 March 2016, organised in collaboration with the OECD, the Washington Center for Equitable Growth and the Yale Institution for Social and Policy Studies. Thanks to the Ford Foundation for funding and hosting the event. The author also thanks the HLEG members who commented on prior drafts.
The opinions expressed and arguments employed in the contributions below are those of the author(s) and do not necessarily reflect the official views of the OECD or of the governments of its member countries.
8.1. Introduction
In the wake of the financial crisis of the late 2000s, hundreds of millions of people in OECD countries faced significant economic dislocations, including unemployment, income volatility, and sharp drops in housing wealth and other assets. Opinion surveys showed a spike in people’s worries about these and other economic risks, as well as a deterioration in their confidence that political leaders and public policies could effectively address them. In a phrase, citizens of crisis-affected countries grew more concerned about their “economic security”, i.e. the degree to which they were vulnerable to hardship-causing economic losses. This heightened concern, in turn, influenced everything from their consumer and investment decisions, to their choices about family formation and geographic mobility, to their political behaviour.
This chapter examines what we know about economic security and what we still must learn. The 2009 Stiglitz-Sen-Fitoussi report devoted relatively limited attention to economic security, whether as an influence on subjective well-being or as an objective feature of individuals’ economic lives. It mentioned job security, health and medical spending security, and retirement security, but did not present measures of these phenomena. Its short section on economic security closed by arguing for a more comprehensive approach:
The many factors shaping economic security are reflected in the variety of approaches used to measure them. Some approaches try to quantify the frequency of specific risks, while others look at the consequences of a risk that materialises and at the means available to people to protect themselves from these risks (especially resources provided by social security programmes). A comprehensive measure of economic security would ideally account for both the frequency of each risk and its consequences, and some attempts in this direction have been made. A further problem is that of aggregating across the various risks that shape economic security, as the indicators that describe these risks lack a common metric to assess their severity. A final, even more intractable problem is that of accounting for the long-term consequences for quality of life of the various policies used to limit economic security (through their effects on unemployment and labour-force participation). (Stiglitz, Sen and Fitoussi, 2009; pp. 201-202)
It is fair to say that the recommendations of the Stiglitz-Sen-Fitoussi report with regard to economic security – unlike its recommendations with regard to income inequality and subjective well-being – did not spark the investments in statistics, theory and research necessary to achieve these ambitious goals. Given advances in concepts and data since 2009, however, the subject is ripe for a re-examination.
8.1.1. Key features of extant measures
Before examining these measures, a few final points are in order. First, most measures focus on economic shocks that are unexpected and largely beyond the control of individuals. In practice, however, it is often difficult to know whether shocks are unexpected or involuntary. One approach is to focus on consumption, on the assumption that unexpected and involuntary shocks will have larger consumption effects. Yet consumption data are not widely available, nor are consumption drops the only possible measure of involuntary losses.
Second, while analysts frequently refer to the “risk” of economic loss, which implies a prospective outlook, most measures are in fact retrospective (the exception is measures of perceived security that ask respondents to offer their own assessment of various future events). In these cases, the risk faced by individuals or households is assumed to reflect past experience – their own, that of people like them, or a combination of the two.
Finally, and related, some measures are specific to people or households, while others can only be used to examine aggregate outcomes (for example, levels of security within regional or occupational groupings or among specific types of households). Perceived security is usually measured at the respondent level. By contrast, many measures of observed security are available only for aggregate groups, as they require observing the incidence of outcomes within defined populations.
This last point raises a final issue: the proper unit of analysis for measures of economic security. In general, security is an individual-level phenomenon. People experience insecurity, not groups. However, many of the most important forces that shape economic security, including the buffers people have against it, operate at the household level or even at higher levels of aggregation (communities, firms, regions, countries and so on). Most measures are built up from either individual-level data or household-level data, with the choice depending on the specific measure used.1
8.2. What is the state of existing statistics on economic security?
This section reviews the leading measures used to chart observed and perceived economic security, moving back and forth between concepts and data. Because data limitations are such a significant constraint, each measure is discussed in the context of the major data sources that are required to produce it. The section begins with measures of perceived security; it then turns to measures of observed security.
8.2.1. Measures of perceived economic security
A wide range of surveys ask questions regarding people’s perceived economic security. The main benefit of such surveys is that they directly capture individuals’ perceptions of their personal and household economic experiences. To the extent that economic security is seen as synonymous with – or at least closely related to – individuals’ psychological response to economic risks, surveys provide information about subjective perceptions that data on individuals’ material circumstances cannot provide. And even if these perceptions are viewed “merely” as an effect or correlate of observed security, there is simply no way to understand the link between observed and perceived security without delving into these subjective responses.
Two key constraints, however, limit the utility of existing surveys for assessing perceived security. First, many survey questions are not asked repeatedly over any significant span of time, limiting the ability to examine changes in perceived security. Second, surprisingly few questions are replicated with similar wording in multiple national surveys, limiting the ability to examine cross-national differences in perceived security. The discussion that follows focuses on survey instruments that are available both over time and across at least a small subset of countries.
General assessments of the economy
Three broad categories of survey questions about perceived economic security appear regularly in major surveys. The first type is questions about how one feels about the economy or aspects of one’s economic situation in the past or present. The most famous are the questions on one’s own and the national financial situation used to compile the University of Michigan Consumer Sentiment Index (Carroll, Fuhrer and Wilcox, 1994). The most common of these questions, however, seem weakly related to economic security – in particular, its forward-looking aspect. In addition, substantial research has shown that many of these general economic perceptions are heavily coloured by assessments of incumbent parties, with partisans of the incumbent party offering more favourable assessments (Duch, Palmer and Anderson, 2000).
Perceptions of buffers
A second type of question that offers greater promise concerns individuals’ perceptions of their own ability to weather future economic shocks. Economic data often contain people’s answers to such questions as whether they have particular forms of insurance coverage or specific levels of wealth. These questions, however, do not assess perceived security – they are simply designed to elicit information about households’ material circumstances. Still, a number of surveys do include questions that involve a subjective component. The most common of such items concern the length of time the respondents’ believe they could remain economically comfortable if they were the victim of adverse economic shocks. Typically, these surveys find that the majority of individuals believe they could go only a limited amount of time before experiencing hardship – a reflection of the limited liquid wealth that many households have.
Similarly, some surveys ask people to assess whether they could rely on certain sources of support in the event of economic shocks, which also involves a subjective, forward-looking component. An example is the European Union’s Survey of Income and Living Conditions (EU-SILC) 2015 ad hoc module’s question on the possibility to rely on relatives, friends, or neighbours in case help (including financial help) is needed. Other examples of such questions are included in the EU-SILC – most notably, when respondents are asked about their ability to make ends meet (current), to face unexpected expenses (current, future), and their unmet needs (past) for medical and dental examination or treatment.
These questions contain a subjective component, and they provide an important indicator of individuals’ preparation against major economic risks. Still, they are best thought of as measures of buffers rather than of security more generally. They are most useful, therefore, when they are accompanied by questions about the likelihood that individuals will experience economic shocks.
Expectations regarding future shocks
This brings us to the third and final category of survey measures – namely, questions about one’s likely economic situation in the future. These forward-looking measures seem closer to the concept of economic security than either of the survey items just discussed. More important, they provide something that measures of observed security cannot: individuals’ own estimate of the risks they face.
Forward-looking questions fall into two broad subgroups.
The first are questions that ask about individuals’ worry or anxiety with regard to specific risks. Prior research shows that expressed worry is closely correlated with the expected probability of economic shocks; it also captures an emotional element of individuals’ responses that other measures tend to miss. Unfortunately, surveys with these sorts of questions tend to be country-specific – the most notable examples are US surveys, such as the Survey of Risk Perceptions and Insecurity (SERPI; Rehm, Hacker and Schlesinger, 2012; Hacker, Rehm and Schlesinger, 2013). Country-specific surveys obviously cannot be used to examine differences across nations though, when repeated, they can be used to look at changes over time.
The second type of survey item asks individuals about the likelihood of economic loss. Typically, these questions have asked people to rate the chance of an economic event occurring on the basis of an ordinal scale (for example, 1 to 5, with 5 denoting the most insecure status). The most common of these types of questions concern the risk of job loss. For example, the International Social Survey Program (ISSP) has repeatedly asked respondents to express how much they agree with the statement “my job is secure,” with four answer options ranging from “strongly agree” to “strongly disagree.” Several high-profile surveys, however, ask respondents to estimate the precise numerical probability that they or someone like them will experience a particular adverse economic shock (Manski, 2004; Hacker, Rehm and Schlesinger, 2013; Hendren, 2017).
Can we believe respondents’ estimates?
It might be wondered whether respondents can come up with meaningful estimates at the high-level of precision that a 0-100 scale requires. To address this concern, some surveys use categorical or more limited ordinal scales. However, Hacker, Rehm and Schlesinger (2013) show that with the appropriate survey instruments – in their work, a sliding scale that shows visually the proportion of the population that each percentage level represents – it is possible to get fine-grained estimates that do not exhibit substantial clumping at zero or 50%.
Moreover, their work and recent research by Rehm (2016) and by Hendren (2017) shows that individuals do a reasonably good job forecasting the likelihood of major economic shocks in the following year. A number of studies also show that shocks affect the probabilities that individuals attach to future economic losses, as well as their attitudes toward economic security more broadly. These findings strongly suggest that measures of perceived and observed security may not be as distinct as some analysts believe, at least when individual responses are averaged across larger groups.
Hendren’s analysis also shows that measures of perceived and observed security can be used in conjunction with each other to assess the welfare loss associated with economic shocks. In brief, he looks at the drop in consumption that occurs not only when individuals lose their job, but also when individuals come to believe they have a high probability of losing it. The former measure is often used as a proxy for the welfare loss of unemployment, but as Hendren points out, if individuals cut back their consumption prior to unemployment because they expect to become unemployed, this approach will understate the negative welfare effects of unemployment – according to Hendren, by a substantial amount. Though Hendren’s work does not consider the additional disutility associated with the fear or anxiety that such knowledge might produce, it does point to the promise of measures that utilise both perceived and observed security simultaneously.
8.2.2. Measures of observed economic security
While measures of perceived security rely on individuals’ own perceptions of their economic situation, measures of observed security draw on economic data that capture their material circumstances. Of course, much of this data comes from surveys of national populations (rather than, say, administrative sources). The difference is that these survey items are not designed to elicit information about respondents’ perceptions but rather about their experiences and circumstances.
Four classes of measures of observed security are prevalent in the literature: 1) measures of household and individual buffers; 2) estimates of the probabilities of economic shocks; 3) indexes of observed security; and 4) various measures of over-time economic (in)stability.
Some measures are hybrids of these. For example, Bossert and D’Ambrosio develop an indicator of insecurity that is a weighted combination of wealth levels (buffers) and past wealth dynamics (stability), while Hacker and his colleagues present a measure of the joint risk (probabilities) of income loss and medical spending shocks (stability) that includes a correction for household wealth (buffers). These hybrid measures are generally close to one of the major types (Bossert and D’Ambrosio, 2013; Hacker et al., 2014).2
Buffers
This class of measures looks at the adequacy of individuals’ or households’ savings, insurance or other buffers against major economic shocks.
A major category of these measures defines and assesses asset sufficiency, i.e. whether households could maintain an adequate standard of living for a specified period merely by drawing down their wealth (usually, but not always, excluding housing because it is relatively illiquid and households need a place to live). In effect, these are measures of household’s capacity for self-insurance. “Adequate” is defined differently in different studies, but a common metric in US analyses is the federal poverty line (a very low standard), with the specified period usually being 3 months (again, a low bar). Thus households are defined as “asset poor” when they have so little wealth they would not be able to support themselves at the US federal poverty line for at least three months if they lost their sources of income.
Cross-national analyses of asset poverty are rare, however, in part because of limited data. With regard to data, the Luxembourg Wealth Study (LWS) has augmented the pool of cross-national wealth data, drawing on national statistical offices’ micro-data regarding income and wealth. At the same time, the OECD and Eurostat have launched a project on household income consumption and wealth statistics, linking data on the distribution of each item based on micro-data. Yet these data are not always fully comparable cross-nationally, nor are high-quality wealth measures generally integrated into panel data, so wealth levels and vulnerability to shocks often cannot be examined inter-temporally side-by-side. Moreover, these wealth data generally do not do a good job measuring wealth at the top of the distribution – for that, specialized surveys that over-sample high-net-worth individuals like the US Survey of Consumer Finances (SCF) are best. However, this is not a significant problem when looking at asset poverty, since the focus is on the lower part of the distribution.3
In addition to data challenges, conceptual issues bedevil cross-national analysis. These include how to set the minimum standard of living that assets are expected to provide, including whether it should be relative to the national income distribution or an absolute standard that is common across countries. No less vexing is whether to include housing wealth in asset measures, as housing valuation and prevalence differ substantially across countries, as does the ease of unlocking housing wealth when experiencing economic shocks (by, for example, borrowing against accumulated home equity).
Nonetheless, measures of asset sufficiency contain valuable information and are an important part of measuring economic security across countries, especially since they can be developed with existing data. To illustrate their value, Figure 8.1 compares income and asset poverty and “economic vulnerability” across OECD countries, using a relative poverty standard and adjusting income for household size (“equivalising”). The income poor are those with equivalised income below 50% of the median income in each country. The income and asset poor are those with equivalised income below 50% of the median income and equivalised liquid financial wealth below 25% of the income poverty line (three-month buffer). The “economically vulnerable” are those who are not income poor but have equivalised liquid financial wealth below 25% of the income poverty line.
Three features of Figure 8.1 stand out. First, there is considerable overlap between income and asset poverty, though the degree of coincidence varies across countries. Most of the income poor are also asset poor. However, second, many of those who are not income poor lack adequate liquid financial wealth to weather economic shocks. Indeed, economic vulnerability is typically at least three times as high as income poverty. This suggests that vulnerability to economic shocks is a much broader phenomenon than economic deprivation. Third, levels of economic vulnerability vary greatly across countries. In Greece, for example, more than half the population lacks enough liquid financial wealth to maintain a poverty-level income for three months. By contrast, the share is roughly one in five in Austria and Norway.
Like other assessments of buffers, measures of asset sufficiency contain no information about the severity or character of the shocks that individuals or households face. Similar households with the same level of liquid wealth could experience very different levels of security based on their exposure to economic risks. Beyond this, asset measures do not typically take into account either borrowing capacity or informal sources of support, such as inter-household transfers. In principle, such measures could incorporate borrowing capacity through, for example, the use of credit ratings or (returning to perceived security) estimates of one’s ability to borrow in the event of adverse shocks. But so far the focus has been squarely on household wealth.
Another weakness is that, in their emphasis on self-insurance, these measures leave out various types of formal insurance against major risks, such as health insurance and retirement pensions – both public and private. The OECD and the International Labour Organization (ILO) collect extensive data on the breadth of such protections, including the level of benefits, scope of coverage, duration of support, and stringency of qualifying conditions. Typically, these measures are based on statutory programme rules or administrative data (or both). Based on these measures, analysts have produced indices of social programme generosity that allow for comparison across countries.
Figure 8.1. Income and asset-based poverty
Note: Countries are ranked in ascending order of the share of individuals who are “economically vulnerable”. The OECD average is the simple country average. The “income poor” are those with equivalised income below 50% of median income in each country. The “income and asset poor” are those with equivalised income below 50% of the median and equivalised liquid financial wealth below 25% of the income poverty line (3-month buffer). The “economically vulnerable” are those who are not “income poor” but have equivalised wealth below 25% of the income poverty line. Income poverty rates refer to household disposable income for Australia, Canada, Chile, Denmark, Finland, Italy, the Netherlands, New Zealand, Norway and the United States, and to household gross income for the remaining countries. Liquid financial wealth includes cash, quoted shares, mutual funds and bonds net of liabilities of own unincorporated enterprises.
Source: OECD (2017), OECD Wealth Distribution Database, https://stats.oecd.org/Index.aspx?DataSetCode=WEALTH.
Similarly, many countries collect data on the prevalence and characteristics of private insurance, such as commercial life and health insurance. These data are often collected at the household or individual level in surveys of income and wealth, and at the aggregate level in surveys of national savings and production (or in commercial statistics on the breadth and characteristics of particular types of insurance).
For all their value, however, measures of formal insurance protection exhibit two salient weaknesses. First, with the exception of estimates based on household surveys, they are not usually available as individual- or household-level data that allow for comparison of levels of economic security across individuals or households, rather than across countries (or other geographic units).
Second, the tendency to focus on formal policy characteristics leaves these measures open to substantial slippage between assessed and actual risk protection. For example, not all benefits are “taken up” by those with formal coverage, nor do public and private implementers always carry out programme instructions faithfully. With private benefits in particular, eligibility for specific benefits may be extremely complex to determine, as evidenced by US research on “surprise medical bills” (Cooper and Morton, 2016; Garmon and Chartock, 2016). Though actual protections are likely to be close to formal rules, important differences in economic security may still be missed, particularly when those most likely to experience economic dislocations – the poor, the young, the less educated – are most likely to fall through the gaps between promised and provided benefits.
Despite these caveats, measures of social programme generosity provide crucial information about the extent to which public policies are designed to increase economic security. These indicators can be used to assess how broad and deep such protections are, as well as – alongside other measures of observed or perceived insecurity – how effective they are at achieving their purposes. Table 8.1 shows the most recent results (2010) for one broadly used collection of indicators of social programme generosity, the Comparative Welfare Entitlements Dataset. The table shows the level (relative to the nation’s average production wage), duration and coverage for two important benefits: sickness pay and unemployment insurance. It orders the countries based on the dataset’s index of the comparative generosity of sickness pay, which normalizes these generosity indicators based on their historical (since 1980) and cross-country variation, equally weighting benefit levels, duration and coverage.4
Shock probabilities
Many students of political economy have examined discrete economic risks by looking at the cross-sectional prevalence of key economic shocks, most often unemployment. The goal is to calculate fine-grained measures of the risk of these shocks for particular groups, such as workers in different occupations. Rehm (2016), for example, develops “occupational unemployment rates,” which are “calculated exactly as national unemployment rates are, except that the calculations are performed for detailed occupations”, by dividing workers into up to 27 groups based on the International Standard Classification of Occupations (ISCO) developed by the ILO.
The logic – for which Rehm provides strong backing – is that “using a workers’ occupation as a reference group and approximating the probability of job loss by the unemployment rate of his or her occupation provides a good measure of risk exposure” in the employment domain. Figure 8.2 shows, for instance, that these rates are strongly correlated with individuals’ perceived job security. The figure pairs data from the US labour force survey and the US General Social Survey, showing the relationship between occupational unemployment rates (calculated for 27 occupations using labour force data) and whether a worker says it is “likely or very likely” they will lose his or her job in the coming year (calculated from the General Social Survey, with occupations assigned using the same ISCO standards). Given the imprecision of the survey question, the main point of interest is not the close numerical correspondence between the observed rate and perceived risk but the near-linear increase as occupational unemployment rates rise.
So far unemployment has been the main focus of such inquiries, but similar group-specific measures of risk could in principle be constructed for other outcomes, such as reductions in pay or hours, entry into poverty, and reported sickness.5
Table 8.1. Welfare state generosity, 2010
Country |
Unemployment Generosity Index |
Sickness Generosity Index |
---|---|---|
Norway |
14.2 |
15.9 |
Sweden |
8.2 |
14.9 |
Netherlands |
11.9 |
13.8 |
Belgium |
14.0 |
13.7 |
Germany |
10.0 |
13.3 |
Switzerland |
13.9 |
13.0 |
France |
11.1 |
12.3 |
Denmark |
9.5 |
12.2 |
Finland |
9.2 |
12.1 |
Ireland |
11.1 |
10.6 |
Austria |
10.4 |
10.3 |
Chinese Taipei |
5.4 |
10.3 |
Spain |
11.7 |
10.0 |
Italy |
5.7 |
9.6 |
Portugal |
10.6 |
9.5 |
United Kingdom |
8.3 |
8.0 |
Japan |
5.3 |
7.6 |
Greece |
7.3 |
7.3 |
New Zealand |
7.1 |
6.3 |
Australia |
7.1 |
6.3 |
Canada |
8.1 |
5.2 |
United States |
10.7 |
0.0 |
Korea |
4.2 |
0.0 |
Note: Country characteristic scores are standardised using z-scores with mean and distribution based on a reference period. (Extreme values, including unlimited benefit duration, are dropped and assigned a maximum or minimum z-score.) These standardised characteristics for each programme (unemployment, sick pay) are summed and the sum is multiplied by the coverage ratio. Thus, the sub-index is computed as: (Programme generosity score) = [2*z(Benefit Replacement rate)+ z(ln(Benefit Duration weeks))+ z(ln(Benefit Qualification weeks)+ z(Waiting days )+12.5]*Insurance Coverage.
Source: Scruggs L. (2014), Comparative Welfare Entitlements Dataset (CWED2), University of Connecticut & Detlef Jahn, University of Greifswald, http://cwed2.org/.
Figure 8.2. Occupational unemployment rates and perceptions of job security, United States, 1972-2010
Note: The dashed line is the 45-degree line; the solid line shows the predicted probability of respondent saying he or she is likely or very likely to lose his or her job within the next 12 months, based on the US Current Population Survey (occupational unemployment rates) from 1972 to 2010 and the US General Social Survey (perceptions of job security within occupational groups) from 1972 to 2010, controlling for income, age, gender, work status, education, race, region (South vs. outside South) and church attendance.
Source: Rehm, P. (2016), Risk Inequality and Welfare States: Social Policy Preferences, Development, and Dynamics, Cambridge University Press, Cambridge, MA.
These probability measures have the virtue of being readily understandable; in many cases, moreover, they do not require panel data and thus can build on the large number of cross-sectional surveys already used by policy-makers. Indeed, labour force surveys are among the most widely available and reliable sources of data for inter-temporally and cross-nationally comparable indicators.
For all their virtues, however, these probability estimates can only be calculated for specific outcomes, and many important outcomes (such as large out-of-pocket medical expenses) may be more difficult to see in standard economic data than is the case for unemployment. Furthermore, they can only be justified as estimates of the probability of these outcomes for a given individual or household with additional theory or evidence that supports the assumption that all members of an occupation or other group face risk more or less equal to the group’s average probability.
Finally, because these measures are based on cross-sectional data – and, indeed, such fine-grained measures require samples of a size rarely achieved by panel studies – they cannot speak to the cumulative character of economic shocks. A group-specific unemployment rate, for example, only captures the share of a group that is out of work at any given time or during any given period. Yet the experience of unemployment is very different if particular individuals or households experience it again and again over time, as opposed to its incidence being spread broadly across the population.
Indices
Until now, the focus has been on discrete indicators, but a major class of measures used in time-series and cross-national analysis attempts to bring together multiple indicators to “sum up” observed economic security. These indices range from the relatively simple measures of programme generosity just discussed to relatively elaborate measures that try to capture both the probability of shocks and the strength of buffers within specific domains.
Among the latter, the work of Lars Osberg stands out as exemplary of domain-specific indices, which he calls the “named risks” approach. Though its implementation has evolved, the basic idea is that economic security is a product of 1) the probability of an adverse event and 2) the average consequence of that event, which is mediated by 3) the strength of the buffers available to protect people. Thus, Osberg’s approach embodies the threefold distinction between shocks, losses, and buffers common to most definitions of economic security.
The four domain-specific indices developed by Osberg and his colleagues are meant to describe economic security for residents of a given country with regard to unemployment, family breakup, medical costs, and poverty in old age. In practice, the precise indices mix 1), 2), and 3) in different ways. With regard to unemployment, for example, the focus is on 1) and 3): “Security from employment” is defined as a product of the unemployment rate 1) and the breadth of and fraction of prior wages provided by unemployment benefits 3).6 With regard to family breakup, the focus is instead on 1) and 2): “Security from single-parent poverty” is a product of the divorce rate 1) and the incidence and depth of poverty among single-female-parent families 2). In two cases – “security in the event of sickness” and “security from poverty in old age” – the index is based solely on 2): economic security with regard to medical costs is conceived of as (the inverse of) the average share of income spent on un-reimbursed medical expenses 2); and economic security in old age is conceived of as (the inverse of) the incidence and depth of poverty within a country among those older than 65.
The next section on key results that emerge from current evidence will consider these indices more fully, as well as non-index integrated measures that attempt to capture economic security with a single indicator, such as the various measures of income volatility that have become so central to economic research over the last generation. For now, it suffices to lay out briefly the strengths and weaknesses of index measures in general and the index measures proposed by Osberg and his colleagues in particular (Osberg, 2015, 2010; Osberg and Sharpe, 2014, 2009).
A major strength of the domain-specific index approach is its ability to reflect the leading priorities embodied in public policy. That is, in this approach, which domains to cover and how to conceptualise security within them can – and, indeed, must – be tailored to the goals of specific policies. Osberg bases his list of “named risks” on Article 25 of the United Nation’s 1948 Universal Declaration of Human Rights, which reads “Everyone has the right to…security in the event of unemployment, sickness, disability, widowhood, old age or other loss of livelihood in circumstances beyond his control”. Consequently, Osberg’s measures focus on economic security with regard to “unemployment”, “sickness”, “widowhood” and “old age”.
This strength, however, is counterbalanced by a potential weakness. Basing measures on the priorities of public policy increases the chance that they will be relevant to policy-makers, but reduces the ability to assess whether those priorities are aligned with the realities of economic security. Implicitly, this is acknowledged in the substitution of “single parenthood” for “widowhood” in the index of family-related security: in an age in which most mothers work and family breakup is common, death of the (male) family breadwinner is no longer seen as the only or even most salient financial risk that parents face.
A concern common to all indices is the appropriateness of weighting. Domain-specific indices of economic security weight at two levels. The first is weighting done to construct the domain-specific index itself. In recent iterations of the index of “security in the event of unemployment”, for example, Osberg and his colleagues have weighted the unemployment rate more heavily than unemployment benefits on the grounds that it has a larger effect on well-being. While this justification has merit, it moves the index away from a relatively simple measure – the joint product of the unemployment rate, the average replacement rate, and the share of workers covered – towards one that is more complex and potentially ad hoc.
Second, to the extent that these domain-specific measures are to be combined into a comprehensive index, there is the question of how to weight these different and possibly even incommensurable measures. At least four options present themselves: equal weighting (as in the Human Development Index); weighting on the basis of some measure of policy priority, such as spending on particular social benefits; weighting on the basis of the impact of each domain on household well-being; and weighting on the basis of measures of perceived security, such as the importance that individuals ascribe to security within a given domain, or the impact of shocks within that domain on perceived security. The last two approaches have considerable merit, but for the most part the evidence they require is lacking. If that evidence were stronger, moreover, it might well allow for more direct measures of observed security than the indices under consideration.
Another great strength of these domain-specific indices is that they rely on easily obtained and generally reliable data. The flipside is that these aggregate-level data may not correspond closely with individual experience or lend themselves to finer-grained comparisons across groups. The potential slippage between formal benefit rules and actual implementation has already been discussed. Also problematic is the inconsistent treatment of public and private buffers. Some of the measures proposed – un-reimbursed medical expenses, old-age poverty – are designed to measure outcomes in the context of all public and private protections. In the case of unemployment, however, only public benefits are considered, even though personal savings, spousal labour supply and other private buffers may be an important source of additional support.
Finally, these indices were designed to measure economic security at the country level and are not always easy to apply to less highly aggregated groups. Certainly, it is not possibly to use them to say that particular individuals or households are economically secure since, like estimates of the probability of specific shocks, they require a reference group. Thus, they are less useful for calculating the distribution of economic security within countries than they are for comparing levels of security across countries.
Volatility
Over the past generation, a large literature on income and consumption volatility has accumulated, driven by advances in both data and theory (e.g. Gottschalk et al., 1994; Moffitt and Gottschalk, 2002; Hacker, 2008; Hacker and Jacobs, 2008; Dynan, Elmendorf and Sichel, 2008; Acs and Nichols, 2010; Nichols and Zimmerman, 2008; Nichols and Rehm, 2014; Kopczuk, Saez and Song, 2010; Gorbachev, 2011; Dogra and Gorbachev, 2016). With regard to data, the growth of high-quality panel studies has made it feasible to examine the over-time fluctuation of economic outcomes at the individual or household level in a way that was rarely possible in the past.
Using these data, scholars have proposed a range of innovative measures of such over-time fluctuations – most of them falling into the broad category of “volatility” estimates. Generally, volatility estimates are meant to describe the magnitude of inter-temporal fluctuation of some economic variable at the household or individual level. Typically, these measures focus on earnings or household income but sometimes they examine consumption. Moreover, they usually treat volatility as variance relative to longer-term trends, such as lifetime growth in earnings. Thus, volatility measures provide a rough estimate of income or consumption risk. Box 8.1 provides a more in-depth look at various volatility measures.
The primary implication of the burgeoning body of research on volatility is that income (and, to a lesser extent, consumption) varies enormously over time. Though most of the existing work has been done on the United States – which, it is becoming increasingly clear, has higher levels of volatility compared with other affluent countries – the conclusion holds across all countries for which panel data exists. People’s economic circumstances change a great deal over time – so much so that, according to one recent calculation using the US Panel Study of Income Dynamics (PSID), more than half of adult Americans between the ages of 25 to 60 experienced at least one year below 150% of the federal poverty line (around USD 12 000 for an individual in 2015) in the period 1968-2009 (Rank, Hirschl and Foster, 2014). This means, in turn, that many more people utilize social benefits aimed at cushioning major economic shocks during their lives than a static snapshot of the income distribution might suggest.
Whether income or consumption volatility has risen over time is a different question, which will be taken up in the next section. But the fact of volatility, hitherto hidden from view by the near-universal reliance on one-shot cross-sectional samples, is not in dispute.
Nonetheless, volatility is not the same as economic security for at least two reasons.
Volatility measures treat gains and losses as symmetrical (except insofar as models assume diminishing marginal utility of income). Yet economic security is focused on losses, which, again, likely loom much larger in subjective well-being than do comparable gains.
Volatility measures do not as a rule distinguish between different sources of losses (or gains). Yet not all losses threaten economic security. Losses that are freely incurred are different from less controllable shocks. For example, economic security is likely to be much more compromised by an involuntary job loss than by a planned hiatus from the workforce.
With regard to the source of economic shocks, some studies of volatility focus on outcomes that are unlikely to be voluntary, such as large shifts in consumption that are not explained by predictable changes in household needs. Most, however, treat all income or consumption changes similarly. Distinguishing voluntary changes from involuntary ones is difficult, since many life events involve elements of both. Early retirement, for example, is often associated with sickness or disability or with firms’ efforts to shed older workers. Even divorce, almost by definition a choice for at least one partner, involves involuntary aspects, as the large literature on the negative consequences of divorce (particularly for women) suggests. Thus, determining voluntariness with regard to particular shocks can be difficult, especially with existing data. And since volatility analysts are usually interested in changes over time rather than absolute levels, putting to the side this thorny issue does not compromise their findings so long as the relative proportion of voluntary versus involuntary changes remains stable over time.
The issue of voluntariness should also be distinguished from the question of whether economic shocks are foreseeable. Foreseeability, too, is a matter of degree, and economic security is almost certainly not related to it in any unvarying manner. We know that people dislike uncertainty (Knight, 1921) – that is, situations in which they cannot even assign probabilities to future outcomes – and will pay to reduce that uncertainty (e.g. Ellsberg, 1961; Camerer and Weber, 1992; Di Mauro and Maffioletti, 2004). Thus, less foreseeable risks may well pose a greater threat to economic security, or at least to perceived security, all else equal.
Box 8.1. Measures of volatility
Many approaches to measuring volatility decompose variance in incomes into “persistent” components that are relatively stable over time (variance in long-run incomes across people, or inequality), and “transitory” components measuring inter-temporal variability or “volatility.” These studies differ on many dimensions, including whether they focus on earnings or household income and whether they use administrative or panel data. A key difference is whether they use parametric or nonparametric models.
Nonparametric Models: The seminal nonparametric computations of permanent and transitory variation from Gottschalk et al. (1994) have been followed by “error components models” (ECM) or dynamic variance components models with persistent and transitory shocks. After the early work looking primarily at male earnings (often white male earning in US data), numerous authors decomposed changes in household income inequality into persistent and transitory parts. Many of these ECM identify a smaller role for transitory variation or volatility than for long-term or persistent differences across people; but even when transitory variation is small relative to persistent variation, the increase in transitory variation is often large.
Parametric Models: A more recent body of work uses parametric decomposition, with most estimates informed by a series of papers by Gottschalk and Moffitt, with the most prominent recent analysis using this approach being Kopczuk, Saez and Song (2010). By contrast, Nichols and Zimmerman (2008), Acs and Nichols (2010) and Nichols and Rehm (2014) write income (not log income) as the sum of a permanent (time-invariant) component, a person-specific linear time trend, and transitory variability around trend. Persistent variance is the “inequality” (I) in longer-run incomes; variation in trends is called “mobility risk” (M); and variation around the trend is called “volatility” (V) or “inter-temporal variability around trend”. Using a wide variety of data, Nichols and Rehm (2014) document large increases in volatility in the United States relative to Canada and other countries. They also conclude that tax and transfer programmes have lesser volatility-mitigating effects in the United States than in other countries, and that the United States are diverging from its peers in the extent of volatility mitigation of its tax and transfer system.
Persistent variation across individuals in family or household resources tends to be much larger than inter-temporal variation in family or household income. In the United States, however, both persistent and transitory variations seem to have increased at comparable rates in recent decades. The increase in inter-temporal variation in household income stems from many sources, and more work is needed to ascertain its welfare consequences, but a substantial rise in the prevalence of large income drops (paired with a much smaller rise in the prevalence of large income gains) indicates that welfare-lowering risk has increased over past decades in the United States. This view is supported by recent work on consumption volatility by Dogra and Gorbachev (2016), who show that unexplained consumption variation has increased alongside higher income volatility – with the rise driven by increases among households with liquidity constraints (proxied by zero or negative wealth).
Source: Courtesy of Austin Nichols.
However, we also know from Rehm’s research and related work (Rehm, 2016; Hendren, 2017) that people can more or less correctly anticipate many common economic shocks, at least to the extent that their perceptions of the likelihood correlate highly with observed measures of risk and that they can update these perceptions based on new information or experiences. Indeed, one reason why commercial insurance against salient economic shocks is often inadequate or unavailable is such private information, which creates adverse selection (only high-risk individuals demand coverage) that can destabilise or prevent the formation of viable private markets. This line of research suggests that economic security (or, at least, perceived security) depends not only on foreseeability but also on what is foreseen, with those who see the chance of a shock as high being less secure than those who are less certain or foresee a lower probability.
The third and final reason why volatility estimates are not direct measures of economic security is that they generally look only at household income, ignoring household wealth and major non-discretionary expenditures such as out-of-pocket medical costs.7 But liquid wealth is of course a major source of household protection against income volatility, and household well-being can be threatened by sharp spikes in non-discretionary spending as well as by sharp drops in income. The small literature on consumption volatility is in part a response to these difficulties, but it suffers from its own weaknesses – most notably, the scarcity of high-quality consumption data.
Though economic security and economic volatility are not synonymous, the extensive and increasingly sophisticated literature on volatility provides crucial guidance for the measurement of economic security. One of the great virtues of this research has been its consistent focus on the refinement of individual-level measures that can be used for analysis at multiple levels, from the worker or household, to demographic or educational groups, to countries as a whole. This micro-level focus distinguishes volatility measures from some of the indices of economic security just considered, which are, by construction, limited to macro- or meso-level analysis.
In addition to offering crucial conceptual and methodological guidance, the literature on volatility also provides many valuable clues about the evolution of citizens’ economic security. These central findings and their implications are discussed in the next section.
Hybrid measures
Responding to some of the shortcomings of volatility measures as measures of economic security, Hacker and his colleagues have developed an alternative measure called the Economic Security Index or ESI (Hacker et al., 2014).8 Despite the title, it is not truly an index; rather, it is a comprehensive measure of the incidence of large shocks to household economic standing that integrates multiple datasets covering income, wealth and medical spending. This measure has been implemented using three major US panel data sources: the PSID, the Survey of Income and Program Participation (SIPP), and the Current Population Survey (which re-contacts households that remain in the same residence, allowing the formation of two-year mini-panels through an algorithm-based matching of households across adjacent years). All three of these sources show similar trends and demographic differences.
Unlike measures of volatility, the ESI focuses only on drops – in this case, a 25% or greater decline in “available household income” from one year to the next. The 25% threshold was chosen based on a separate opinion survey, which found that the median US household said they could go roughly three months without income before suffering “real hardship”. (Other thresholds show similar trends, albeit at different levels.) The “one year to the next” criterion reflects the annual structure of most panel data, as well as the annual reporting and receipt of most public taxes and many public benefits.9
There are two other notable features of the ESI: first, it accounts for liquid financial wealth; second, it accounts for two of the most important non-discretionary expenditures faced by many households, i.e. out-of-pocket medical costs (including insurance premiums) and debt service. Liquid (not total) household wealth enters in the calculation of the ESI as an exclusion criterion: households who have adequate liquid financial wealth to fully buffer their cumulative expected losses are not treated as “economically insecure” even when they experience a 25% or greater year-over-year income drop.10 Medical costs and debt service enter in as constraints on income available for other consumption needs, i.e. these expenditures are subtracted from income when determining whether households experience a 25% or greater loss. Finally, household income is equivalised and then assigned equally to adult household members to provide an individual-level measure.
The ESI is thus a hybrid of the buffer and volatility approaches that produces a number similar to the estimates of the probability of adverse economic shocks discussed earlier. It is also a hybrid of an income-based and a consumption-based measure, since key non-discretionary expenditures are subtracted from income. Like occupational unemployment rates, the individual-level measure is binary (“drop” or “no drop”) and can only be turned into an estimate of economic security by looking at its incidence across a defined population. Though it would be possible to construct a truly individual-level measure with a long panel by looking solely at an individuals’ past history (e.g. Stettner, Cassidy and Wentworth, 2016), a probability estimate better captures the core aspect of economic security, namely that it involves the risk (but not certainty) of adverse outcomes. Like all observed measures, however, it is inherently backward-looking: future risk is assumed to be similar to (recent) past risk.
To bolster this approach, the research team that developed the ESI conducted a panel opinion survey that asked extensive questions about economic security, including whether individuals experienced large household income drops. These results allow not just for an independent verification of the estimates of the incidence of large drops made using the SIPP, PSID, and CPS. More important, given that none of these data sources contain questions about perceived economic security, they were used to verify that individuals experiencing large drops did in fact express lower levels of security. As already noted, they did: large income shocks were associated with much higher levels of worry, higher estimates of future shocks, and greater support for public policies designed to buffer these shocks. Wealth drops did not have such consistent effects, though the relatively small size of the sample – between 2 000 and 3 000 respondents – may have contributed to this (non)finding.
Nonetheless, the ESI has its own significant weaknesses. Like nearly every other measure discussed, it does not distinguish between voluntary and involuntary changes (although it does focus on sizable losses of 25% or greater that are least likely to be expected or voluntary). Nor does it capture all economic threats, including other salient non-discretionary expenditures, most notably the unavoidable costs that come with participation in the labour force, such as child care and transportation costs (although there is much less agreement about the extent to which these expenses are non-discretionary than there is with regard to medical costs.)
To date, no measure has been proposed that truly integrates over-time volatility with long-term risks such as retiring without adequate income. The ESI is no exception, though it does exclude earmarked retirement savings from the calculations of liquid financial wealth, on the grounds that spending down such savings jeopardizes household’s economic security with regard to retirement. There is a large literature that looks at consumption drops at retirement (e.g. Banks, Blundell and Tanner, 1998; Aguiar and Hurst, 2005; Haider and Stephens Jr., 2007), and this focus might be integrated into the ESI approach. But such an integration would require accounting for the reduced income needs of retirees.11
Finally, in its focus on changes, the ESI does not treat people facing persistent but stable deprivation as economically insecure – a feature shared by all volatility measures. In practice, low-income individuals experience much greater instability of income and non-discretionary spending than do higher-income individuals, and they have much more limited liquid financial wealth. Still, it remains true that volatility-based measures miss aspects of economic insecurity that do not involve economic instability.
In short, several measures have been proposed to assess economic security. Some of these measures are designed to capture only certain important aspects of economic security, such as the strength of household buffers, while others are closer to fully comprehensive measures. Even the latter, however, do not represent incontestable proxies, not least because the precise definition of economic security remains under discussion. Nonetheless, these measures of observed security clarify how analysts, policy-makers and statistical agencies could better assess a critical feature of citizens’ lives and a fundamental influence on their well-being. The next section turns to what these measures tell us about the character and evolution of economic security in recent decades.
8.3. What can we say about economic security based on the available evidence?
This section presents a small number of indicators that can be used to compare economic security across individuals, households, socio-demographic groups and countries. It focuses on observed economic security, and more specifically on two measures of observed security that are available or can be developed for multiple countries and time periods: Lars Osberg’s “named risk” approach, a hybrid of the probability-of-shock and prevalence-of-buffers approach; and new estimates based on the approach embodied in ESI, i.e. a volatility-based measure that focuses on large year-to-year income drops.
In presenting these results, this chapter draws on both published and unpublished research. In particular, the ESI-style estimates for countries other than the United States have been developed only recently and thus should be considered preliminary. Moreover, both these data and the evidence developed by Osberg and his colleagues are currently available only for affluent countries. As more and more countries develop high-quality economic data – and in particular panel data – it should become possible to develop similar measures for a broader range of countries.
8.3.1. The “Named Risk” approach
Osberg’s “named risk” measure of economic security is designed to capture the overall prevalence of and degree of protection against major economic shocks listed in the UN Universal Declaration of Human Rights, specifically unemployment, sickness, single-parent poverty and poverty in old age. Results are available from 1980 through 2009 for 14 affluent democracies: Australia, Belgium, Canada, Denmark, Finland, France, Germany, Italy, the Netherlands, Norway, Spain, Sweden, the United Kingdom and the United States.
In addition to the shock-specific measures, Osberg and his colleagues calculate an aggregate index of economic security, weighting each component based on the share of the population in each year presumed to face the relevant risk (i.e. everyone aged 15 to 64 years in the case of unemployment; the entire population in the case of sickness; married women with children under 18 in the case of single-parent poverty; and adults aged 45 to 64 in the case of old-age poverty).
For ease of exposition, this aggregate measure is used in Figure 8.3 to compare all 14 countries, with the dark bar indicating the beginning of the series (1980) and the lighter bar the end (2014).
Figure 8.3 points to several notable features.12 First, countries differ substantially in their overall level of economic security. In 2014, economic security was greatest in Norway, with a value of 0.83 (the indices are constrained to be between 0 and 1), followed by Denmark with a value of 0.81. By contrast, the United States had the lowest score at 0.48, followed by Spain (at 0.59).
Second, in most nations, the index does not change much between 1980 and 2014. Only three nations experienced a change in the index greater than 10%: Canada (12% increase), Spain (18% decline), and the United States (a 62% increase in economic security).
The relative stability of the aggregate index reflects relatively little change in most of the shock-specific indices.13 The most notable trend in the shock-specific indices is an overall increase in “security from single-parent poverty” and “security in old-age”. These indices improve because of reductions in the prevalence and severity of poverty among single-parent households and the elderly, respectively.
Whether poverty is a good measure of economic security is an important question, and one that will be taken up in the conclusions. For now, it is simply worth noting that levels of poverty are not necessarily the same as the risk of poverty. Assessing risk requires looking at the probability that any individual or group will enter poverty over some defined interval, which in turn requires considering income dynamics. It is to the topic of income dynamics – and specifically the prevalence of large income drops – that we now turn.
Figure 8.3. Osberg’s index of economic security, selected OECD countries, 1980 and 2009
Source: Centre for the Study of Living Standards (2016), Database of the Index of Economic Well-being for Selected OECD Countries and Alberta, 1980-2014, www.csls.ca/iwb/FinalIEWBAlbertaandOECD2014.xlsx.
8.3.2. The prevalence of major income drops
The second set of findings reviewed here is based on the Economic Security Index. Recall that the ESI is a hybrid measure constructed using panel data that captures the share of individuals experiencing a 25% or greater year-over-year decline in family-size-adjusted real household income net of out-of-pocket medical spending. The ESI excludes from this share both the very small portion of the population entering retirement between one year and the next and the larger (but still relatively small) proportion possessing liquid financial wealth sufficient to self-finance the loss.14
Unfortunately, neither out-of-pocket medical spending nor liquid financial wealth is reliably reported in many panel studies. Thus, what is presented here is a limited version of the ESI without the medical spending or wealth adjustments. This measure captures the share of the adult population experiencing a 25% or greater loss in (household-size-adjusted) individual income from one year to the next.15 It can be thought of as a measure of income risk, whether for the population as a whole (when looking at the prevalence of 25% or greater losses nationwide) or for specific sub-groups (when looking at the prevalence among regional, occupational or demographic groupings).
This measure is simple, feasible given existing data and scalable, with a larger absolute loss required as income rises. Given this last characteristic, it is also comparable across countries with different average per-capita income levels. As noted previously, the 25% threshold was based on US survey questions that asked how long respondents could go without their income before experiencing hardship. Whether responses to this sort of question would be similar in other countries is unclear. It is worth reporting, however, that within a plausible range neither over-time trends nor the rankings of countries are particularly sensitive to the exact threshold chosen.
This measure can be constructed for almost forty countries, reflecting the growth of reliable panel studies in the past two decades. Since panel data are a crucial precondition for any approach that tracks individuals or households over time, it makes sense to begin with a brief summary of the data. The next section discusses the changing scope and character of panel data in greater depth.
Producing these estimates required assembling various panel studies that cover more than a few years and have been more or less continuous since their creation. For many of these studies, the period that can be analysed is relatively brief. With the exception of a few pioneering panel studies – notably, the German Socio-Economic Panel (GSOEP), which starts in 1984, and the US PSID, which starts in 1968 – country-specific panel studies mostly date from the 1990s. These encompass Australia, Canada, Korea, Sweden, Switzerland and the United Kingdom. Another wave of studies began in the early 2000s with the launch of the European Union’s Survey of Income and Living Conditions (EU-SILC), eventually encompassing more than 20 countries.
For the United States, in addition to the PSID, panel data can be obtained from the Survey of Income and Program Participation (SIPP) and from the matched Current Population Survey (CPS) files. Though the SIPP, PSID, and CPS were all used to construct the Economic Security Index, the estimates presented here use matched CPS files, which produce the largest sample. The main drawback of matched CPS results is their short (two-year) panels, but they are sufficient to measure year-over-year income drops.16
Figure 8.4 provides a simple picture of the prevalence of large income losses in all the countries for which data are available, as well as changes over the most recent period encompassing the financial crisis (roughly from the early 2000s until the end of the data series). The length of the bars shows the range of prevalence estimates across the years (indicated next to the country abbreviation). The point marker shows that average for these estimates within each country across these years. Finally, if there is a trend in the data over the most recent period, this is indicated by an arrow showing the direction of the change. Thus, the figure shows the average, range and recent evolution of income risk in these countries.
As Figure 8.4 shows, the prevalence of large income losses varies substantially both across countries and within countries over time. Within the group considered by Figure 8.4, the countries with the greatest range and highest average prevalence of large income losses are generally those that were hit hardest by the financial crisis, including Spain, Greece and Iceland. The countries with the lowest average prevalence of large income losses include the Netherlands, Sweden, Switzerland, Denmark, Norway and Finland. The United States – the country that has been the focus of most analyses of income volatility – has a relatively high prevalence of large income losses.
Moreover, the ranking of countries is fairly consistent with the “named risk” approach, especially its measure of security in the event of unemployment. Figure 8.5 shows the correlation between this measure, averaged for 2006-08, and the average prevalence of large income losses over the same period in the 14 countries for which both estimates are available.
Figure 8.4. Average, range, and evolution of the incidence of large income losses
Notes: Based on the following panel data collections: ECHP, EU-SILC, CPS, CNEF (BHPS, SOEP, HILDA, KLIPS, SHP, SLID). For each country, the period covered is indicated on the horizontal axis. Arc-changes, unlike percentage changes, treat gains and losses symmetrically (e.g. an income gain from USD 50 to USD 100 implies a 100 per cent change but a 67 per cent arc-change; while an income loss from USD 100 to USD 50 implies a 50 per cent change but a 67 per cent arc-change); they are bound between +2 and -2.
Finally, while the prevalence of large income losses generally rose between the early and late 2000s, this trend was largely cancelled out as countries recovered from the crisis. Indeed, only a handful of countries – most notably the United States – have experienced a secular rise in the prevalence of large income losses. Most countries have seen little or no increase in the incidence of large income losses, and a few (e.g. Switzerland, Norway and Austria) witnessed a decline. Since these data encompass the period of the financial crisis and the slow recovery that followed, this is a notable finding, which suggests that some countries were able to reduce the fallout of the crisis and its aftermath, at least when it comes to income risk.
This hypothesis can be put to a more direct test by parsing the components of household income. In the literature on income inequality, researchers commonly distinguish between inequality before and after taxes and transfers, and use the difference between the two as a rough measure of how much taxes and transfers reduce inequality. More specifically, the standard approach is to calculate the difference between the Gini coefficient of the distribution of market income [Gini(MI)] and the Gini coefficient of the distribution of disposable income [Gini(DI)].17 Because Gini coefficients are summary indicators, this measure is necessarily calculated at the aggregate level.
When looking at the prevalence of large income losses, an equivalent approach would distinguish between losses in income before and after taxes and transfers, and assess how many fewer citizens experience large income losses when taxes and transfers are taken into account.18 In other words, the reduction of risk would be calculated as the difference between the aggregate prevalence of market income losses [Risk(MI)] and the aggregate prevalence of disposable income losses [Risk(DI)] as follows: [Risk(MI)-Risk(DI)]/Risk(MI).
Figure 8.5. The incidence of large income losses vs. Osberg’s scaled index of economic security
Note: Refer to Figure 8.3 “Osberg’s index of economic security, selected OECD countries”. Sample: AUS 2001-14, BEL 1993-2014, CAN 1993-2010, DEU 1984-2014, DNK 1993-2014, ESP 1993-2014, FIN 1995-2014, FRA 1993-2014, GBR 1992-2014, ITA 1993-2014, NLD 1993-2014, NOR 2002-14, SWE 2003-14, USA 1985-2012.
This approach, however, is less precise than necessary. Comparing summary measures such as Gini coefficients, there is no way to determine who changes rank as the income measure switches from market income to disposable income. By contrast, with the current measure of income risk, it is possible to know who is prevented from experiencing a large income loss by taxes and transfers. In particular, individuals can be classified into four categories based on whether they experience a qualifying drop in market income, in disposable income, or in both, as illustrated by the two-by-two matrix in Table 8.2.
Table 8.2. Incidence of risk (market income vs. disposable income)
|
|
Large loss in market income |
|
---|---|---|---|
No |
Yes |
||
Large loss in disposable income |
No |
[1] Secure (no income loss) 81% |
[3] Income loss mitigated 6% |
Yes |
[2] Income loss aggravated 2% |
[4] Risk (income loss) 10% |
Note: Based on the following panel data collections: ECHP, EU-SILC, CPS, CNEF (BHPS, SOEP, HILDA, KLIPS, SHP, SLID). Sample: AUS 2002-16, AUT 1995-2014, BEL 1994-2014, BGR 2006-14, CAN 1994-2010, CHE 2001-16, CZE 2005-14, DEU 1985-2015, DNK 1994-2014, ESP 1994-2014, EST 2004-14, FIN 1996-2014, FRA 1994-2014, GBR 1993-2015, GRC 1994-2014, HRV 2010-14, HUN 2005-14, IRL 1994-2014, ISL 2004-14, ITA 1994-2014, KOR 2004-14, LTU 2005-13, LUX 2004-14, LVA 2007-14, MLT 2006-14, NLD 1994-2014, NOR 2003-14, POL 2005-14, PRT 1994-2014, ROU 2007-14, SRB 2013-14, SVK 2005-14, SVN 2005-14, SWE 2004-14, USA 1986-2011.
The matrix shows the four possible combinations of market and disposable income dynamics. Those in the top-left cell [1] do not experience large income losses in either market income or disposable income. Pooling the data for all countries and years, about 81% of adults belong to that category. At the other extreme, those in the bottom-right cell [4] experience large income losses in both disposable income and market income. On average, about 10% of adults are in this unfortunate situation.
The top-right cell [3] refers to individuals who experience a large drop in market income, but not in disposable income. For these individuals, about 7% of adults, the tax and transfer system serves as a safety net that keeps them from crossing the 25% threshold. Finally, the bottom-left cell [2] indicates the peculiar situation in which the tax and transfer system actually exacerbates income losses. Fortunately, these cases are rare, roughly 2% of observations.
These cells suggest a more precise measure of the buffering role of the tax and transfer system: the share of adults who experience a large loss in market income but not in disposable income. The proportion is substantial: on average, the tax and transfer system mitigates large income drops for about 41% of individuals experiencing large income drops (7/(7+10) = 0.41). However, this number varies dramatically across countries and over time.
Figure 8.6 provides a summary picture of the role of taxes and transfers in reducing the prevalence of large income losses in our set of countries during the most recent period (again, roughly the early 2000s until the end of the relevant data series). The length of the bars shows the average share of adults who see their market income decline by 25% or more, but are prevented from experiencing a large disposable income loss by taxes and transfers. The arrows show whether this buffering role has increased or decreased over time.
The conclusion suggested by this figure is that countries with high-levels of income risk (as shown in Figure 8.4) do relatively little to cushion large market income losses through the tax and transfer system. Moreover, the recent evolution of this buffering role indicates that countries have, if anything, increased the degree to which they reduce large income losses during the recent crisis. That is to say, a growing share of those who experience large market income losses are prevented from experiencing large disposable income losses thanks to the tax and transfer system. Whether this reflects a conscious change in policy or, more likely, the resilience of countries’ safety nets during the economic crisis, it provides some reassurance to those who worry that national systems of social protection are becoming progressively less capable of covering contemporary risks to income. It remains to be seen whether this conclusion will continue to hold true once the deep employment effects of the crisis recede.
Finally, while all these estimates have looked at the adult population as a whole, they can be used to examine socio-demographic differences within countries. Figure 8.7 contains one such comparison looking at the average prevalence of large disposable income losses for adults with different levels of formal education. Not surprisingly, in most nations, income losses are much more likely among adults with limited formal education as compared with those with extensive formal education. Though not reported in Figure 8.7, income losses are also more prevalent among lower-income adults (when income is averaged across all available observations for each individual), workers who are not members of trade unions, heads of single-parent households, and younger adults.
Indeed, it is possible to look not only at differences across broadly defined groups. When panels are sufficiently long, it is also possible to construct individual-level or household-level measures for extended periods of time, as is done in the volatility literature.
Figure 8.6. Effect of taxes and transfers on the incidence of large income losses
Notes: Based on the following panel data collections: ECHP, EU-SILC, CPS, CNEF (BHPS, SOEP, HILDA, KLIPS, SHP, SLID). For each country, the period covered is indicated on the horizontal axis. Arc-changes, unlike percentage changes, treat gains and losses symmetrically (e.g. an income gain from USD 50 to USD 100 implies a 100 per cent change but a 67 per cent arc-change; while an income loss from USD 100 to USD 50 implies a 50 per cent change but a 67 per cent arc-change); they are bound between +2 and -2.
Figure 8.7. Incidence of income losses by education, pre- to post-secondary
Note: Based on the EU-SILC for 2012/2013.
Table 8.3, for example, shows the share of the working-age population experiencing one, two, three, or four or more large income losses over a decade.19 Over ten years, as the table shows, only a minority of working-age adults in Australia, Germany, the United Kingdom, Switzerland and the United States were fortunate enough to escape the experience of a year-over-year income loss of 25% or more. On the other side, roughly 23% of Americans experienced two drops, about 12% experienced three drops, and over 7% experienced 4 or more drops, indicating that close to half of Americans experienced two or more large income losses in that decade.
Table 8.3. Number of large year-to-year income drops experienced by individuals over a decade
Drops |
United States |
United Kingdom |
Switzerland |
Germany |
Australia |
---|---|---|---|---|---|
0 |
26.4% |
39.5% |
40.4% |
41.1% |
33.0% |
1 |
31.1% |
32.9% |
33.9% |
30.8% |
32.1% |
2 |
22.9% |
16.1% |
17.2% |
17.4% |
21.4% |
3 |
12.3% |
8.3% |
6.1% |
7.3% |
9.6% |
4+ |
7.4% |
3.3% |
2.4% |
3.4% |
4.0% |
Note: Based on the CNEF panel data collection (PSID, BHPS, SHP, SOEP, HILDA). Sample: AUS 2002-16, CHE 2001-16, DEU 1985-2015, GBR 1993-2006, USA 1971-97.
In sum, the prevalence of large income losses appears to be a sensible measure of economic security that varies across countries and individuals in ways generally consistent with other relevant findings (including, as discussed in the last section, measures of perceived economic security). Still, it remains incomplete in some key respects, and significant additional work will need to be done to develop a broader evidence base for measuring other elements of economic security (such as large consumption shocks) and extending these measures to a larger set of countries. The next section discusses this evidence base in greater depth.
8.4. Are available statistics adequate to inform policy?
As is clear from the discussion thus far, the availability of reliable and cross-nationally comparable data has been a crucial constraint on the development of improved measures of economic security. Three shortcomings of existing statistics stand out: the limited pool of long-term and cross-nationally comparable panel data; the weaknesses of most administrative data for tracing individuals over time; and the lack of regular questions about economic security in conventional random-sample opinion surveys and, to even greater extent, in panel data. Nonetheless, these data have been rapidly improving, as shown when reviewing each of these major sources.
8.4.1. Panel data
Measures that look at fluctuations in individuals’ economic standing over time generally require panel data. Historically, this has been a key constraint on the development of better metrics on insecurity.
To be sure, panel data are not always necessary. Cross-sectional data can be used to develop a number of the measures discussed in this chapter, including assessments of the buffers that individuals and households have and estimates of the cross-sectional incidence of key economic shocks, such as unemployment. The challenge, however, is that such data can only provide insight into the point-in-time incidence of shocks, rather than their over-time prevalence. Moreover, it is generally not possible to use cross-sectional data to estimate how buffers change for particular households or individuals, or whether shocks are concentrated among specific groups rather than broadly distributed across the population.
Fortunately, a wide range of panel datasets have been inaugurated in the last two decades (Box 8.2). One weakness of many of these sources, however, is their lack of simultaneous data on income, consumption, and wealth. In addition, most do not contain questions that allow researchers to assess perceived economic security alongside observed economic security. Finally, differences in question wording and survey design can make it difficult to compare results across different panels. The recommendations presented at the end of this chapter aim to remedy these problems, and to increase the number of countries for which panel data are available.
Box 8.2. Major panel data sources
For measuring economic insecurity, we would like multiple observations on income over a long period of time at a relatively high frequency. Typically, we have instead a short panel with high-frequency data collection, or a long panel with lower frequency.
In the United States, the Panel Study of Income Dynamics (PSID) has measured incomes on an annual basis back to 1968, but has not been refreshed to stay representative in every year, and it switched to biennial reporting of incomes in 1997. The Survey of Income and Program Participation (SIPP) has measured monthly incomes every four months since 1984, but starts new panels every few years and switched to annual reporting in 2016. The Current Population Survey (CPS) has annual income in March (matchable to the prior or subsequent March for a fraction of the sample) but does not follow movers. The Health and Retirement Survey and National Longitudinal Surveys are lower frequency and do not represent the full population.
Long panel surveys are available for many developed countries, including the Household Income and Labour Dynamics (HILDA) in Australia, the Canadian Survey of Labour and Income Dynamics (SLID), the German Socio-Economic Panel (GSOEP), the Korea Labour and Income Panel Study (KLIPS), the Swiss Household Panel (SHP), and the British Household Panel Study (BHPS), plus harmonised country panel datasets in the Cross-National Equivalent File (CNEF) and the European Union Statistics on Income and Living Conditions (EU-SILC). China and many less developed countries have or are soon to have panel household surveys as well.
Source: Courtesy of Austin Nichols.
8.4.2. Administrative data
New datasets linking tax and programme data are becoming available in a handful of countries. These can be superior to panel data because of the well-known problems of recall bias and attrition in panel data. However, most administrative datasets remain limited, and they suffer from their own problems, including significant restrictions on their use by researchers (Box 8.3). A promising new source of data is the large datasets being created by financial institutions. These data allow very fine-grained analysis of economic dynamics, but suffer from some serious problems, including limited availability, limited scope (often encompassing only transactions conducted through a single financial institution) and lack of representativeness of the individuals/households included.
Box 8.3. Major administrative data sources
Administrative data from tax records are sometimes hard to access, but offer the possibility of longer panels with annual measures of income. In the United States, for example, the Longitudinal Employment and Household Dynamics (LEHD) dataset, available in secure Census data facilities, links multiple administrative records on people and firms. Similarly, the Social Security Administration (SSA) hosts the Master Earnings File (MEF) containing all earnings reported to the SSA.
These sources offer long series of annual income thought to be of high quality because they are subject to auditing. Recently, efforts have been made to merge multiple administrative records with government survey data, as in the so-called “Gold Standard File” created by the US Census and the SSA. An important point to bear in mind with matched data sources is that merge rates are never 100%, and matched samples may be less representative of the population of interest.
Federal government sources offer large sample sizes, but so too does a new generation of corporate data sources, both from regular clients and special surveys such as those conducted by AC Nielsen. Credit reporting agencies and major banks have in some cases better coverage of annual totals for both household income and expenditures, thanks to aggregating transaction-level data for account holders. Many of these sources can also track income across country boundaries and may include transactions not subject to third-party reporting and therefore missing from government files. Nevertheless, many low-income or unbanked individuals will never be observed in these files.
Source: Courtesy of Austin Nichols.
8.4.3. Questions about economic security in conventional opinion surveys
As noted in the discussion of perceived security measures, opinion surveys sometimes include questions about economic security, particularly job security. These questions typically ask respondents to assess how likely it is that they will experience specific adverse economic events, or how well protected they would be if they experienced such events. Cross-nationally comparable survey questions are relatively rare, however, and overwhelmingly concern economic security in the domain of employment. Moreover, even within countries, panel surveys are relatively rare, making it difficult to assess the causes of changing perceptions of economic security at the individual level.
Nonetheless, a number of cross-sectional surveys do include questions regarding perceived job security. These include:
International Social Survey Program (ISSP) Work Orientations I-III,: “For each of these statements about your (main) job, please tick one box to show how much you agree or disagree that it applies to your job. My job is secure” (1989, 1997, 2005).
European Social Survey (ESS): “Using this card, please tell me how true each of the following statements is about your current job. My job is secure” (2004, 2010).
European Quality of Life Survey (EQLS): “Using this card, how likely or unlikely do you think it is that you might lose your job in the next 6 months?” (2003, 2007, 2011/12).
Eurobarometer (EB): “Here is a list of statements about your current job. For each of them, please tell me if it is very true, quite true, a little true, or not at all true? My job is secure” (1996, 2009).
Figure 8.8 shows the country-year averages for one such question in the European Working Conditions (EWCS): “How much do you agree or disagree with the following statements describing some aspects of your job? I might lose my job in the next 6 months” (2005 and 2010):
Figure 8.8. How insecure is my job?
Note: “I might lose my job in the next 6 months”. Percentage of respondents who “strongly agree” or “agree” (vs. “neither agree nor disagree”/“disagree”/ “strongly disagree”).
Source: Eurofound (2010), European Working Conditions Surveys (EWCS), 2005/2010, www.eurofound.europa.eu/surveys/european-working-conditions-surveys.
8.5. Conclusions
This chapter has reviewed and extended the growing literature on economic security, outlining a range of measures and data that are improving analysts’ and policy-makers’ understanding of this critical aspect of economic life. This final section provides a series of suggestions for promoting more and better research on economic security and improving the base of data on which this work rests.
The core point is that researchers, national statistical agencies and key international organisations should work together to improve and augment existing measures. No one doubts the fundamental importance of economic security. Yet few measures of this vital phenomenon are widely used and accepted. The goal of this chapter has been to lay out a small number of such measures that are consistent with available theory and evidence, can be relatively easily produced for multiple countries using extant data, and can guide policy-makers seeking to safeguard and increase economic security.
The task going forward will be to refine these measures to produce a small number of reliable indicators of economic security that can help experts and policy-makers evaluate economic well-being and social progress. These measures will necessarily be imperfect, and no single one will suffice on its own. Together, however, a small set of measures could provide a baseline for assessing and comparing economic security while creating the foundation for improved measures in the future.
8.5.1. Improving and encouraging research on economic security
The starting point for these efforts is a stronger conceptual foundation. Research on economic security requires a major collaborative programme spanning policy-makers, researchers and theorists to refine both theory and measurement. With regard to theory, the concept of economic security remains in its infancy. Substantial progress in the study of economic security will require its further refinement, with particular attention to the role of individual psychology. With regard to measurement, the development of stronger indicators will require grappling with the intertwined role of income, consumption and wealth, the causes of losses (including the difficult question of how to establish causality given the ubiquity of confounders in this realm), and the improvement of measures of perceived security. It will also require better integration of measures of perceived and observed security within major data sources, the next focus.
8.5.2. Improving and augmenting data related to economic security
Refinements in theory and measurement will only bear fruit if they rest on an adequate base of high-quality data. In particular, there is a pressing need for cross-national data efforts that bring together researchers and national statistical offices to develop panel data that are comparable across countries. These data should include perceived security measures alongside traditional panel economic variables, as is now done in a handful of panel studies. In addition, efforts should be made to link panel data with administrative data that incorporates public tax and programme datasets.
At the same time, researchers and national statistical offices, as well as interested private survey firms, should work together to create a small subset of “security monitors” that could be incorporated into opinion surveys. On the whole, data on the subjective experience of economic security that could allow for monitoring of over-time or cross-country trends remain sparse. Fielding better cross-nationally comparable surveys is therefore imperative. Prior to that, however, extant measures should be compared with measures of observed security to see how closely they correspond. (For that purpose, including more questions about perceived security in panel datasets would again be invaluable.)
Any security monitors based on opinion surveys must be closely tied to the best available research on how to gauge security through a limited number of survey instruments. Here, survey designers should draw on the experience gained by the large community of researchers working on subjective well-being. Moreover, questions should be designed to cover a broad range of risks, not just those related to employment – including risks related to retirement and family dissolution, as well as access to and affordability of food, housing and health care.
Finally, efforts should be directed at developing innovative new data sources that can be scaled up, including lab experiments that probe subjective risk perceptions and individuals’ willingness to pay to insure themselves against key economic risks. A central question here will be how to distinguish “economic security” from general risk aversion, since the former is broader than the latter. Collaboration with private businesses and other institutions that provide financial services should also be encouraged to obtain new data on individual economic dynamics that address the major current limits of proprietary sources. In all these efforts, data should be designed the capture the joint distribution of income, consumption and wealth, as argued elsewhere in this volume.
8.5.3. Identifying a small number of core measures of economic security
In the meantime, researchers, policy-makers and national statistical agencies should work to develop a handful of core measures of economic security that can be incorporated into a “dashboard” of indicators of well-being. In addition to refined ESI-style measures of income risk, these indicators should include one or a few perceived measures of economic security; one or a few measures of buffers (such as asset poverty); and one or a few “named-risk measures” (probability multiplied by severity) for a few major risks such as unemployment, uninsured medical expenses and inadequate retirement income.
There is a strong case, however, for not aggregating these various measures into a single index, since the logic of current weighting schemes is relatively weak. When employing Osberg’s named-risk approach, moreover, it would be ideal to have all such measures focus on the same basic outcome: the uninsured income loss in the event of a particular adverse shock. Ideally, these estimates would also be based on panel data, reducing the gap between measures of income loss and the “named-risk” approach.
With regard to ESI-style measure of income risk, the estimates developed this chapter indicates that it should be possible to develop reliable measures that can be used to compare multiple countries. Nonetheless, several questions remain. For starters, is it possible to incorporate out-of-pocket medical spending into such estimates, as is done for the United States in the ESI? Out-of-pocket medical costs represent a substantial financial burden for many households, so ignoring their effect on economic security leaves an incomplete picture. However, rising out-of-pocket costs may reflect improvements in the quality or volume of care, so their welfare effects are ambiguous. How to treat medical costs is a question that research on economic security needs to better address.
Additional questions must be addressed as well. Perhaps the most pressing is whether wealth and consumption data can be integrated into measures of income loss. Should, for example, such measures subtract debt service or treat wealthy individuals differently from those without wealth, as is done in the ESI? Can consumption effects be used as a test of whether income losses are involuntary? Can measures better account for the differing consumption needs of different households?
A large set of questions also concern the causes of income losses. With present data, it is not always possible to link large losses to discrete changes in the standing of workers or their families. Improved data and measurement techniques should make it possible to pinpoint the causes of large losses more precisely. It is worth keeping in mind, however, that large losses are often the result of multiple simultaneous shocks, which presents difficulties for those interested in parsing their causes. Moreover, even with the best available data, it is always difficult to distinguish correlation from causation in observational studies.
Over the past few decades, economic security has gained heightened salience due to major changes in the workplace and the family, as well as mounting pressures on public programmes and the erosion of key private protections. These changes – and the popular concerns they have generated – make it imperative that researchers and policy-makers better understand how economic security is changing, who is most affected by these changes, and what policies are best poised to address the resulting dislocations.
The findings and recommendations of this chapter point to major areas where our knowledge of economic security has improved. The chapter has also highlighted areas where work can and should be further advanced. Better concepts and measures would not only benefit economic research. They could also help policy-makers evaluate how well existing policies protect economic security and guide them as they sought to improve those policies. Few tasks are more vital today.
References
Acs, G. and A. Nichols (2010), America Insecure – Changes in the Economic Security of American Families, The Urban Institute, Washington D.C, www.urban.org/sites/default/files/publication/32906/412055-america-insecure.pdf.
Aguiar, M. and E. Hurst (2005), “Consumption versus expenditure”, The Journal of Political Economy, Vol. 113(5), pp. 919-948.
Banks, J., R. Blundell and S. Tanner (1998), “Is There a Retirement-Savings Puzzle?”, The American Economic Review, Vol. 88(4), pp. 769-788.
Bossert, W. and C. D’Ambrosio (2013), “Measuring economic insecurity”, The International Economic Review, Vol. 54(3), pp. 1017-1030.
Camerer, C. and M. Weber (1992), “Recent developments in modeling preferences: Uncertainty and ambiguity”, The Journal of Risk and Uncertainty, Vol. 5(4), pp. 325-370.
Carroll, C.D., J.C. Fuhrer and D.W. Wilcox (1994), “Does consumer sentiment forecast household spending? If so, why?”, The American Economic Review, Vol. 84(5), pp. 1397-1408.
Cazes, S., A. Hijzen and A. Saint-Martin (2015), “Measuring and assessing job quality: The OECD Job Quality Framework”, OECD Social, Employment and Migration Working Papers, No. 174, OECD Publishing, Paris, http://dx.doi.org/10.1787/5jrp02kjw1mr-en.
Cooper, Z. and F.S. Morton (2016), “Out-of-network emergency-physician bills – An unwelcome surprise”, The New England Journal of Medicine, Vol. 375(20), pp. 1915-1918.
Centre for the Study of Living Standards (2016), Database of the Index of Economic Well-being for Selected OECD Countries and Alberta, 1980-2014, www.csls.ca/iwb/FinalIEWBAlbertaandOECD2014.xlsx.
Deaton, A. (1992), Understanding Consumption, Oxford University Press, Oxford.
Di Mauro, C. and A. Maffioletti (2004), “Attitudes to risk and attitudes to uncertainty: Experimental evidence”, Applied Economics, Vol. 36(4), pp. 357-372.
Dogra, K. and O. Gorbachev (2016), “Consumption volatility, liquidity constraints and household welfare”, The Economic Journal, Vol. 126(597), pp. 2012-2037.
Doiron, D. and S. Mendolia (2012), “The impact of job loss on family dissolution”, The Journal of Population Economics, Vol. 25(1), pp. 367-398.
Duch, R.M., H.D. Palmer and C.J. Anderson (2000), “Heterogeneity in perceptions of national economic conditions”, The American Journal of Political Science, Vol. 44(4), pp. 635-652.
Dynan, K.E., D.W. Elmendorf and D.E. Sichel (2008), “The evolution of household income volatility”, Federal Reserve Board and Brookings Institution, Draft.
Ellsberg, D. (1961), “Risk, ambiguity, and the savage axioms”, The Quarterly Journal of Economics, Vol. 75(4), pp. 643-669.
Eurofound (2010), European Working Conditions Surveys (EWCS), 2005/2010, www.eurofound.europa.eu/surveys/european-working-conditions-surveys.
Friedman, M. (1957), “The permanent income hypothesis”, in A Theory of the Consumption Function, pp. 20-37, Princeton University Press, Princeton.
Garmon, C. and B. Chartock (2016), “One in five inpatient emergency department cases may lead to surprise bills”, Health Affairs, Vol. 36(1), pp. 177-181.
Gorbachev, O. (2011), “Did household consumption become more volatile?”, The American Economic Review, Vol. 101(5), pp. 2248-70, https://doi.org/10.1257/aer.101.5.2248.
Gottschalk, P. et al. (1994), “The growth of earnings instability in the US labor market”, Brookings Papers on Economic Activity, Vol. 1994, No. 2, pp. 217-72, www.jstor.org/stable/2534657.
Hacker, J.S. (2008), The Great Risk Shift: The New Economic Insecurity and the Decline of the American Dream, 2nd edition, Oxford University Press, New York.
Hacker, J.S. and E. Jacobs (2008), The Rising Instability of American Family Incomes, 1969-2004: Evidence from the Panel Study of Income Dynamics, Economic Policy Institute.
Hacker, J.S., P. Rehm and M. Schlesinger (2013), “The insecure american: Economic experiences, financial worries, and policy attitudes”, Perspectives on Politics, Vol. 11(1), pp. 23-49, https://doi.org/10.1017/S1537592712003647.
Hacker, J.S. et al. (2014), “The Economic Security Index: A New Measure for Research and Policy Analysis”, The Review of Income and Wealth, Vol. 60(S1).
Haider, S.J. and M. Stephens Jr. (2007), “Is there a retirement-consumption puzzle? Evidence using subjective retirement expectations”, The Review of Economics and Statistics, Vol. 89(2), pp. 247-264.
Hendren, N. (2017), “Knowledge of future job loss and implications for unemployment insurance”, The American Economic Review, Vol. 107(7), pp. 1778-1823.
Kahneman, D. and A. Tversky (2013), “Prospect theory: An analysis of decision under risk”, in Handbook of the Fundamentals of Financial Decision Making: Part I, pp. 99-127, World Scientific.
Knight, F.H. (1921), Risk, Uncertainty and Profit, Houghton Mifflin Company, Boston and New York.
Kopczuk, W., E. Saez and J. Song (2010), “Earnings inequality and mobility in the United States: Evidence from social security data since 1937”, The Quarterly Journal of Economics, Vol. 125(1), pp. 91-128.
Manski, C.F. (2004), “Measuring expectations”, Econometrica, Vol. 72(5), pp. 1329-76.
Moffitt, R. and P. Gottschalk (2002), “Trends in the transitory variance of earnings in the United States”, The Economic Journal, Vol. 112 (478), Conference Papers (March), pp. C68-C73.
Munnell, A., H.A. Webb and L. Delorme (2006), Retirements At Risk: A New National Retirement Risk Index, Center for Retirement Research, Trustees of Boston College, http://crr.bc.edu/wp-content/uploads/2011/09/NRRI.pdf.
Nichols, A. and P. Rehm (2014), “Income risk in 30 countries”, The Review of Income and Wealth, Vol. 60, pp. S98-116, https://doi.org/10.1111/roiw.12111.
Nichols, A. and S. Zimmerman (2008), Measuring Trends in Income Variability, The Urban Institute, Washington, D.C.
OECD (2017), How’s Life? 2017: Measuring Well-being, OECD Publishing, Paris, http://dx.doi.org/10.1787/how_life-2017-en.
OECD (2017), OECD Wealth Distribution Database, https://stats.oecd.org/Index.aspx?DataSetCode=WEALTH.
Osberg, L. (2015), “How should one measure economic insecurity?”, OECD Statistics Working Papers, No. 2015/01, OECD Publishing, Paris, http://dx.doi.org/10.1787/5js4t78q9lq7-en.
Osberg, L. (2010), “Measuring economic insecurity and vulnerability as part of economic well-being: Concepts and context”, in IARIW 31st General Conference, St. Gallen, Switzerland.
Osberg, L. and A. Sharpe (2014), “Measuring Economic Insecurity in Rich and Poor Nations”, The Review of Income and Wealth, Vol. 60(S1), https://doi.org/10.1111/roiw.12114.
Osberg, L. and A. Sharpe (2009), Measuring Economic Security in Insecure Times: New Perspectives, New Events, and the Index of Economic Well-Being, Centre for the Study of Living Standards, Ottawa.
Rank, M.R., T.A. Hirschl and K.A. Foster (2014), Chasing the American Dream: Understanding What Shapes Our Fortunes, Oxford University Press, Oxford.
Rehm, P. (2016), Risk Inequality and Welfare States: Social Policy Preferences, Development, and Dynamics, Cambridge University Press, Cambridge, MA.
Rehm, P., J.S. Hacker and M. Schlesinger (2012), “Insecure Alliances: Risk, Inequality, and Support for the Welfare State”, The American Political Science Review, Vol. 106(2), pp. 386-406, https://doi.org/10.1017/S0003055412000147.
Scruggs, L. (2014), “Social welfare generosity scores in CWED 2: A methodological genealogy”, CWED Working Paper Series.
Scruggs L. (2014), Comparative Welfare Entitlements Dataset (CWED2), University of Connecticut & Detlef Jahn, University of Greifswald, http://cwed2.org/.
Stettner, A., M. Cassidy and G. Wentworth (2016), “A new safety net for an era of unstable earnings”, The Century Foundation, New York, https://tcf.org/content/report/new-safety-net-for-an-era-of-unstable-earnings/.
Stiglitz, J.E., A. Sen and J.-P. Fitoussi (2009), Report by the Commission on the Measurement of Economic and Social Progress, http://ec.europa.eu/eurostat/documents/118025/118123/Fitoussi+Commission+report.
Sullivan, D. and T. von Wachter (2009), “Job displacement and mortality: An analysis using administrative data”, The Quarterly Journal of Economics, Vol. 124(3), pp. 1265-1306.
Tversky, A. and D. Kahneman (1992), “Advances in prospect theory: Cumulative representation of uncertainty”, The Journal of Risk and Uncertainty, Vol. 5(4), pp. 297-323.
Western, B. et al. (2012), “Economic Insecurity and Social Stratification”, The Annual Review of Sociology, Vol. 38, pp. 341-359.
Notes
← 1. One reason for looking at household data is that earnings – the main focus of individual-level analyses – is only one source of income for most people. With increased labour force participation of women, and reforms to social programmes that increase the incentive to work, there is good reason to think that the covariance of earnings and transfers has increased over time, and that the inter-temporal covariance of spouses’ incomes has increased as well. Such increased covariances can result in higher family or household income variability even in the absence of increasing earnings volatility. It is important to note, however, that assigning household resources to individuals, as is common in insecurity research, requires assumptions about how household resources are shared within the family. An important agenda for this research – one essential to understanding the gendered character of insecurity – is to examine more closely how resources are actually pooled within the family.
← 2. Indices, by design, encompass multiple indicators that can, and often do, involve elements of measures 1), 2), and 4).
← 3. The OECD Wealth Distribution Database compiles data on wealth distribution sourced from surveys and administrative registers based on common definitions and classifications. OECD (2017) presents data on the distribution of wealth for 28 OECD countries and 3 emerging economies.
← 4. Unemployment insurance covers national insurance provisions earned without income-testing. Sick pay covers benefits paid in the event of short-term non-occupational illness or injury. This includes provisions for mandatory private (employer-paid) benefits in addition to public insurance. Public pensions include only mandatory public programmes (except for the nominally private Finnish earnings-related fund). Data are also provided for replacement rates of minimum pensions for those without work history. Replacement rates, eligibility criteria and duration of benefits are calculated for a notional average production worker in manufacturing who is 40 years old and has been working for the 20 years preceding the loss of income or the benefit period. Two different household types are accounted for: single (living alone, no children or other dependents) and family (cohabiting with a spouse with no earnings, two children aged 7 and 12). Replacement rates are calculated by annualising the first half year of benefits (i.e., calculating the benefit for the first 26 weeks and multiplying by 2). The reference wage for replacement rates is the “average production worker wage.” General government cash transfers are accounted for when calculating the net wage; the replacement rate for families refers to income that includes child/family benefits (Scruggs, 2014a and 2014b).
← 5. A more elaborate example of a probability-estimate measure is the “Retirement Risk Index” developed by Munnell and colleagues to study changes in US economic security (Munnell, Webb and Delorme, 2006). This measure is designed to capture the probability that working-age Americans who have yet to claim public and private retirement benefits will retire without adequate income. In essence, this risk measure marries the buffer approach discussed previously with the group-specific probability estimates currently under consideration. It does so by calculating available wealth for retirement (based on present wealth and its forecasted growth, plus expected public and private benefits) and then comparing this household-specific total with the amount needed to purchase an actuarially fair annuity that offers an income-replacement rate judged sufficient by established models of retirement planning. What makes it a group-specific risk measure is that these numbers are then used to calculate the future probability of inadequate retirement income as just defined for various educational and income groups and age cohorts. (The main finding is that retirement preparedness has declined sharply overall, particularly so for younger and poorer Americans.) Thus, this measure is conceptually equivalent to Rehm’s – though it uses forecasted income rather than observed unemployment to assign probabilities.
← 6. Another example of such measure is the index of labour market security used by the OECD in the context of its OECD Job Quality Framework. This index is measured as the product of unemployment risk (the monthly probability of becoming unemployed times the average expected duration of completed unemployment spells in months) and (one minus) unemployment insurance (the coverage of the unemployment insurance/assistance times the replacement rates of public transfers received by the unemployed). See Cazes, Hijzen and Saint-Martin (2015).
← 7. How discretionary medical spending truly is remains a major topic of analysis, which is not discussed here; suffice it to say that the largest out-of-pocket expenditures are likely to be the least within the immediate control of individual patients.
← 8. Another volatility-related hybrid measure is the approach of Bossert and D’Ambrosio (2013), who measure economic security as a weighted sum of household wealth and its past volatility. Like other volatility measures, this approach provides a household-level estimate of economic insecurity (which can be translated into an individual-level estimate by assuming equal distribution of household-size-equivalised resources – the common approach in the volatility literature). Bossert and D’Ambrosio apply their approach to the US PSID and to the Italian Survey of Household Income and Wealth (SHIW). Without going into the precise characteristics of this measure – and, indeed, Bossert and D’Ambrosio say that exactly how to weight current wealth and past wealth volatility is presently unknown – its main strength is its integration of wealth levels and changes into a single measure. Its main weaknesses are, first, panel studies with high-quality wealth data are rare, certainly when compared with income data; and, second, there is limited evidence that changes in net worth are, by themselves, a major source of economic insecurity. In part, this is because wealth can change without any direct material hardship if asset prices fluctuate but individuals are not required to liquidate their wealth; in part, it reflects the aforementioned issue of how losses should be treated relative to gains (Bossert and D’Ambrosio are agnostic on this question, though they say that losses should be weighted at least as heavily as gains). By contrast, there is considerable evidence that large income losses make people feel less secure.
← 9. The SIPP has a shorter panel structure that allows assessment of whether the specific annual accounting period – i.e. what dates are considered as start and end points of a year – makes a significant difference to the results; it does not.
← 10. How much is required is to buffer a loss is determined by using the PSID to determine how long it takes for the median household with similar characteristics experiencing a similar-sized drop to return to their pre-drop income level, and then summing the cumulative income shortfall over this period.
← 11. This could be done either by adjusting household income at retirement or by accounting for the full range of work-related non-discretionary expenditures that retirees need not incur. At present, those retiring in the previous year are excluded from calculation of the ESI, so as not to confuse entrance into retirement with an adverse shock.
← 12. The next three paragraphs draw heavily on Osberg and Sharpe, 2014.
← 13. These trends in poverty, in turn, raise two questions: first, should poverty be assessed using country-specific poverty levels; and second, should these levels be absolute (i.e. the same across time or space) or relative (e.g. less than 50% percent of median income, the standard used by Osberg and his colleagues)? For comparing across countries, there is a strong argument for using country-specific thresholds. Poverty is commonly understood as deprivation relative to other members of a given society. It would be difficult, for example, to use the same standard for old-age poverty in Bangladesh as in Belgium; there would either be no poverty in the latter or near-universal poverty in the former. For over-time comparison, however, there is a stronger argument for using absolute poverty levels – for example, by fixing poverty levels for each nation at the beginning of a series – so as to separate out trends in economic security from trends in median income. This is particularly true when looking at short time intervals during which it is plausible to argue that the income levels that define poverty remain relatively constant.
← 14. Technically, the cumulative loss that occurs before income returns to its pre-drop level for a typical household with similar characteristics and a similarly sized loss.
← 15. Technically, the threshold is a 25 percent or greater arc-percent change. Arc-percent changes are calculated as 2*(Income[t]-Income [t-1])/[(Income[t]+Income[t-1])]. Unlike percent changes, arc-percent changes are bound by minus and plus 2, and they treat gains and losses symmetrically. For example, a respondent doubling her income from USD 50 to USD 100 experiences a 100 percent change (but a 67 arc-percent change). A respondent with a change in income from USD 100 to USD 50 experiences a 50 percent change (but a 67 arc-percent change). The arc-percent approach treats the change of USD 50 in a symmetric fashion.
← 16. All data were cleaned and standardised following established conventions. To deal with outliers as well as different top-coding and bottom-coding rules, income values were bottom-coded at 1 national currency unit (NCU) and top-coded at the 98th percentile. The main income variable used is total household income after taxes (including all cash benefits), adjusted by family size.
← 17. Usually, researchers calculate the percentage difference between the two [Gini(MI)-Gini(DI)]/Gini(MI)], though sometimes they use the absolute difference.
← 18. This approach raises issues that can only be touched upon here. The biggest one is the common assumption – embodied in the convention of calling pre-tax, pre-transfer income “market” income – that income before taxes and transfers is a pristine reflection of market forces, while disposable income after taxes and transfers captures the effects of government policy. In fact, taxes and transfers can greatly affect labour and capital markets, hence “market” income as well as disposable income. In addition, governments can and do attempt to shape market income through a range of tools besides taxes and transfers, including regulatory and macro-economic policies. For these reasons, it is best to think of the difference between market and disposable income as suggestive of the role of taxes and transfers but not, by itself, as offering a complete or definitive assessment.
← 19. The sample consists of respondents aged 25-60 with 10 consecutive years of non-missing information on income drops (most recent spell). Weights from the final year of the spell are applied.