This chapter examines the structural effects of COVID-19 on the labour markets in a set of G7 countries. It starts by presenting evidence on the different workers' potential to shift from presence to remote working amid lockdowns and social distancing measures in Canada, Europe and the U.S. Then, for the United States, it documents the uneven effects of the COVID-crisis across groups of workers in the labour market and the importance of remote work for attenuating the adverse effects during the period. Lastly, it discusses the possible repercussions of the crisis on the inequalities in the labour market in the short and medium run.
Implications of Remote Working Adoption on Place Based Policies
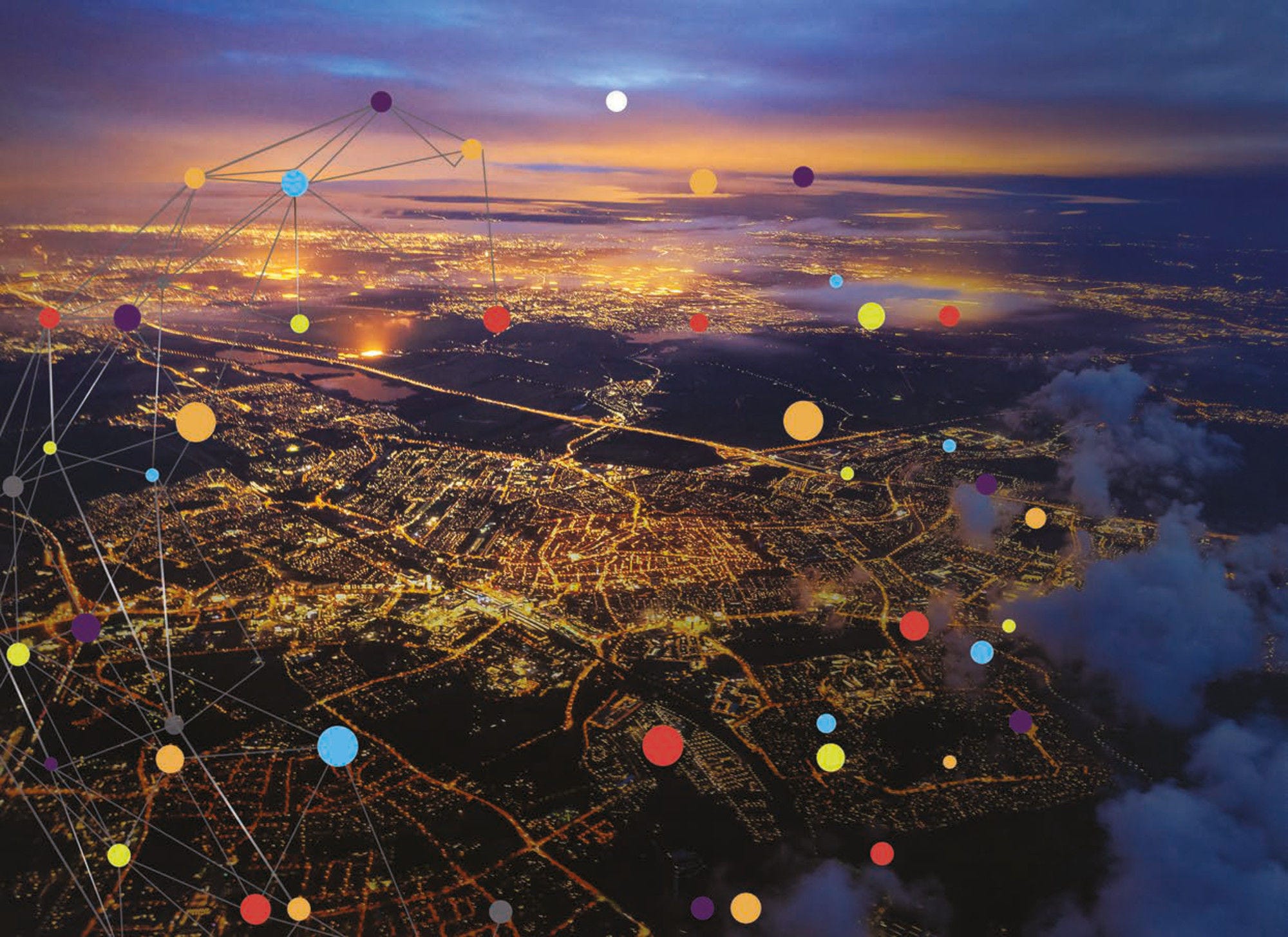
3. Labour markets in the new normality
Abstract
Introduction
COVID-19 has ushered in a new normal where remote working is increasingly widespread. To deal with the spread of COVID-19, governments have implemented strict containment measures such as social distancing and stay-at-home policies to deal with the spread of COVID-19. Such measures have required closing many workplaces, with most of the labour force obliged to work from home. In order to adapt to the sudden change of circumstances, firms have undergone a technological transition and reorganisation to allow their workers to operate from home. Thanks to this rapid transformation, some workers were able to continue working remotely, at least partially, sometimes with almost no activity reduction.
Differences in remote working potential can exacerbate existing inequalities. The possibility to keep working remotely while the pandemic containment measures are in place is mainly determined by workers' occupations, which are in turn highly correlated with workers' education or income. This means that some groups of workers (e.g., low-skilled, low-income, female, young, etc.) are less likely to work remotely. In that case, these groups may be hit harder by the economic crisis, further widening already existing inequalities within labour markets. Identifying the specific groups that are more vulnerable to the crisis could help governments develop policies that aim to minimise the income losses in the short run but also help develop medium-run policies that encourage the return of these groups to the labour market and training to equip them with necessary skills allowing them to adapt to the new normal.
This chapter is composed of three sections. The first section provides an assessment of the remote working capacity of workers employed across 31 countries in Europe, Canada and the U.S. to shed light on lockdowns' uneven effect along socio-demographic dimensions. The second section examines the short-term consequences of COVID-19 on employment and wages in the United States. It shows that the labour market effect of COVID-19 has been uneven across different groups. In particular, young, non-white, and less-educated workers suffered sharper increases in their unemployment rates and a more significant decline in working hours and labour force participation. The second section shows that workers who could work remotely were affected less severely than those who cannot. The final section discusses the medium- and long-term implications of the labour market effects of the crisis. It highlights the importance of ensuring an inclusive recovery in the labour market.
Who can work from home?
The COVID-19 pandemic forced many workplaces and most of the workforce to work from home. Governments have implemented strict containment measures and stay-at-home policies to reduce the spread of the virus and the pressure on the health system. As the measures required closing many workplaces, most of the labour force was forced to stay home and start working remotely.
However, not all workers are able to work from home. Due to differences in the nature of each occupation's daily tasks, remote working potential is significantly different across occupations (see Box 3.1 for a detailed explanation). For example, jobs requiring workers to be outdoors (e.g., food delivery person) or to use heavy equipment (e.g., a vehicle) are unlikely to be worked from home. In contrast, occupations requiring only a laptop and an internet connection (e.g., an accountant, finance specialist, etc.) will have a high potential to work remotely. There is a strong positive correlation between the skill level required for an occupation and its remote working potential. Managers, lawyers and I.T. workers are easily adaptable to remote working, in contrast with other occupations such as farmers, construction workers and artisans. Overall, the probability of holding a job requiring a physical presence is higher in specific social groups, such as low-skilled and low-wage workers.
During the pandemic, workers who could work from home faced lower risks of job and income losses. As discussed in the next section, workers who could work remotely were also significantly less likely to report COVID-19 unemployment and work absences. In contrast, workers who had to be physically present were much more likely to report COVID-19 unemployment and absences. The distribution of new job postings reflects similar trends. While the number of job postings decreased dramatically across European capitals during the pandemic, the drop was more tempered for jobs with high remote working potential (Adrjan and Kleine-Rueschkamp, 2021[1]).
Beyond the employment advantages, remote working also helped workers stay healthy. Working from home enabled workers to avoid face-to-face contacts in offices and public transportation, which reduced their probability of contracting the virus (Alipour, Fadinger and Schymik, 2021[2]). Consequently, workers who could work remotely faced lower risks of exposure and COVID-19 infection risk compared to those who could not work from home (Angelucci et al., 2020[3]).
The following section details the extent to which different groups of workers could shift to remote work following the containment measures imposed by governments after the start of the pandemic. Distinguishing the capacity to work remotely across different groups is essential for identifying those with higher vulnerability during the crisis.
Box 3.1. Assessing the share of jobs amenable to remote working
There are two main methods for measuring the remote working potential of workers and firms. The first method measures the actual or potential rate through surveys conducted at the worker or firm level (Bloom ve et al., 2015; van der Lippe and Lippenyi, 2019; Hensvik et al., 2020; Adams-Prassl et al., 2020; Irlacher and Koch, 2020). Such surveys would include questions on the number of days of remote work practised by the workers, whether they could or would like to do it more, what factors limit their remote working practice or potential. Such surveys have the advantage of presenting precise information on the actual remote work practises adopted by the worker or the firms. On the other hand, small sample sizes in such surveys raise issues regarding representativeness or flexibility in the analysis due to statistical power. They also make international comparisons challenging.
The second method measures workers' capacity to work remotely by their occupation. It classifies each occupation based on the tasks required and according to the degree to which those tasks can be performed remotely (Dingel and Neiman (2020[4]), Saltiel (2020[5]) or Gottlieb, Grobovsek and Poschke (2020[6]). For example, occupations requiring workers to be outdoors (e.g., food delivery person) or to use heavy equipment (e.g., a vehicle) are considered to have a low potential of remote working. In contrast, occupations requiring only a laptop and an internet connection (e.g., an accountant, finance specialist, etc.) will have a high potential to work remotely. This method allows the use of the most recent administrative datasets that have broad coverage and allows international comparisons.
The analysis in this chapter classifies occupations based on a study by Dingel and Neiman (2020[4]) which is built from the O*NET surveys conducted in the U.S. As the occupations are classified according to the U.S. Standard Occupational Classification system (SOC), this note uses crosswalk to the International Standard Classification of Occupations (ISCO) to associate each occupation with a level of remote working potential in other countries (OECD, 2020[7]). Merging the occupation level information with labour force surveys makes it possible to assess the remote working potential of different groups (e..g, industries, income groups, etc.).
Sources: Adams-Prassl, A., Boneva, T., Golin, M., & Rauh, C. (2020). Inequality in the Impact of the Coronavirus Shock: New Survey Evidence for the UK.; Bloom, N., Liang, J., Roberts, J., & Ying, Z. J. (2015). Does working from home work? Evidence from a Chinese experiment. The Quarterly Journal of Economics, 130(1), 165-218; Hensvik, L., Le Barbanchon, T., & Rathelot, R. (2020). Which jobs are done from home? Evidence from the American Time Use Survey;. Irlacher, M; Koch, M. (2020). Working from Home, Wages,and Regional Inequality in the Light of COVID-19, CESifo Working Paper, No. 8232, Center for Economic Studies and ifo Institute (CESifo), Munich; van der Lippe, T., & Lippenyi, Z. (2020). Co‐workers working from home and individual and team performance. New Technology, Work and Employment, 35(1), 60-79.
Remote working capacity varies significantly across industries and occupations
Remote working potential varies significantly across types of occupations. Due to the differences in the daily tasks, some jobs are more suitable for working from home. Figure 3.1 presents the share of workers who can work from home across the main occupation groups. For instance, amenability to remote working reaches 70% for managers and professionals, decreasing to less than 10% for elementary occupations, skilled agricultural, forestry and fishery workers, and craftspeople and related trades.
Figure 3.1. Remote working potential varies significantly across occupations

Note: The number of workers who can work remotely as the percentage of total workers in the occupation. Occupations are ranked in ascending order by the remote working potential in each occupation.
Source: OECD calculations based on European Labour Force Survey (2019), American Community Survey (2019), Canadian Labour Force Survey (2019) and Occupational Information Network data (accessed in March 2021).
The share of jobs amenable to remote working also changes across industries. Industries' remote working potential depends on the occupational composition of their workers. For instance, while 75% of workers employed in the information and communication sector can work from home, this possibility decreases to 20% in construction, accommodation and food services activities, or agriculture. Overall, knowledge-intensive sectors that use digital tools have a higher potential to operate remotely, while those requiring physical effort or face-to-face interactions suffer larger disruptions.1
Figure 3.2. Some industries are more suitable than others to remote work

Note: The number of workers who can work remotely as the percentage of total workers in the industry. Industries are ranked in descending order by the remote working potential in each industry.
Source: OECD calculations based on European Labour Force Survey (2019), American Community Survey (2019), Canadian Labour Force Survey (2019) and Occupational Information Network data (accessed in March 2021).
Education of the workforce and its remote working potential go hand in hand
The possibility of remote working correlates strongly with the skill requirement of the occupation. Workers with higher educational attainments are more likely to work in knowledge-intensive occupations not requiring a physical presence, which allows them to work remotely. Figure 3.2 illustrates the relationship between the share of workers with tertiary education (horizontal axis) and the share of workers who can work remotely (vertical axis) in the 2-digit ISCO occupation. The trend line shows that as the share of workers with tertiary education in the occupation increases, the share of jobs suitable to remote working also increases.
Differences in remote working potential across occupations are not only driven by skills. For example, science and engineering professionals or health professionals (e.g., doctors) are located below the trend line, indicating that the share of jobs amenable to remote working in these occupations is lower than expected, given the workforce's education levels. The technical equipment needs of science and engineering professionals, or the face-to-face interactions of health professionals, are important drivers of the observed differences after education is accounted for. On the other hand, managers or information and communications technicians are above the trend line and have higher rates of jobs amenable to remote working than expected from the workforce's skill composition.
Figure 3.3. Remote working potential increases with skill level in the occupation

Note: The number of workers who can work remotely as a percentage of total workers in the occupation (vertical axis), and the share of workers with tertiary education in the same occupation (horizontal axis).
Source: OECD calculations based on European Labour Force Survey (2019), American Community Survey (2019), Canadian Labour Force Survey (2019) and Occupational Information Network data (accessed in March 2021).
In Europe, the remote working potential is higher in more densely populated areas compared to other areas. Due to their industry structure and larger share of the high-skilled labour force, cities and dense urban areas are more likely to host occupations that can accommodate remote working. Indeed, using the "Degree of Urbanisation" (see Box 3.2 for more details) to distinguish different types of settlement for European countries, OECD evidence (2020[7]) demonstrates that cities (above 50 000 inhabitants) have a 13-percentage point higher share of jobs amenable to remote working than rural areas. Interestingly, the potential for remote working in towns and semi-dense areas seems to be somewhat closer to rural areas than to cities.
On average, cities and rural areas provide the highest and lowest remote working potential for workers of all skill groups. For example, while 58% of high-skilled workers can work remotely in cities, the share declines to 53% and 51% in towns and semi-dense areas or rural areas, respectively. Similar differences also exist for medium-skilled and low-skilled workers. The remote working gaps across regions are due to differences in the industrial structure, affecting occupational composition in the area.
Interestingly, the remote working potential gap between cities and rural areas is the smallest for high-skilled workers. For instance, the remote working potential of high-skilled workers in rural areas is 13% lower than those who work in cities. The gap is larger for low- and medium-skilled employees, at 26 and 30%, respectively. These differences suggest that the local economy's industrial structure affects the remote working potential of workers who are not high-skilled. On the other hand, the high-skilled workers are able to work remotely regardless of the industry that employs them.
Box 3.2. Defining skill groups
The analysis in this section classifies workers into three skill levels based on occupations (OECD, 2019):
Low skilled: Jobs in sales and services and elementary occupations (ISCO 5 and 9)
Medium skilled: Jobs such as clerks, craft workers, plant and machine operators and assemblers (ISCO 4, 7 and 8)
High skilled: Jobs in managerial, professional, technical and associated professional occupations (ISCO 1, 2 and 3)
It is important to note this definition groups workers by their occupations' skill requirement and do not necessarily reflect the actual skill levels defined by workers' formal education. As shown in the literature, migrants often downgrade in the labour market, meaning that they work in occupations that are below their skill levels. This effect is possibly stronger for migrants arriving from non-EU countries as they face additional difficulties in degree recognition or residence permits.
Source: OECD (2019), Under Pressure: The Squeezed Middle Class, OECD Publishing, Paris, https://dx.doi.org/10.1787/689afed1-en.
Figure 3.4. Remote working is higher in cities for all skill groups

Note: The number of workers who can work remotely as a percentage of total workers in the skill group by the degree of urbanisation.
Source: OECD calculations based on European Labour Force Survey (2019), American Community Survey (2019), Canadian Labour Force Survey (2019) and Occupational Information Network data (accessed in March 2021).
Remote working potential increases with wages
Remote working potential and wages are positively correlated. On average, occupations with higher remote working potential tend to have higher average wages. This is not surprising given that workers who can work remotely also tend to have higher education levels, as shown previously. Figure 3.5 presents the share of workers who can work remotely by income decile. Only one out of four workers who are in the first income decile (i.e., the 10% of workers earning the lowest salary) work in occupations that are amenable to remote working while this share increases to 61% in the 10th decile (i.e., the 10% of workers who are earning the highest salaries).
Box 3.3. The Degree of Urbanisation
The Degree of Urbanisation is a methodology to classify cities, towns and semi-dense areas, and rural areas for international comparison purposes. The method proposes three types of areas reflecting the urban-rural continuum instead of the traditional urban–rural dichotomy.
1. Cities: Densely populated areas with at least 50% of the population living in urban centres
2. Towns and semi-dense areas: Intermediate density areas where less than 50% of the population lives in rural areas and less than 50% of the population lives in urban centres
3. Rural areas: Thinly populated areas with more than 50% of the population living in rural areas
Source: Eurostat (2013), Urban-Rural Typology, http://ec.europa.eu/eurostat/web/rural-development/methodology, OECD (2020), OECD Regions and Cities at a Glance 2020, OECD Publishing, Paris, https://doi.org/10.1787/959d5ba0-en.
Figure 3.5. Better paid workers also have a higher remote working potential

Note: The number of workers who can work remotely as a percentage of total workers in the income decile.
Source: OECD calculations based on European Labour Force Survey (2019), American Community Survey (2019), Canadian Labour Force Survey (2019) and Occupational Information Network data (accessed in March 2021).
Unequal labour market effects of the pandemic: the case of the United States
The COVID-19 pandemic affected the United States labour market deeply, raising unemployment numbers and reducing labour force participation, wages, and hours worked. While the crisis has impacted the whole labour market, some workers have been affected more than others. To assess the impact of the pandemic on these labour market outcomes and explore its uneven effect across different types of workers, this section presents results from an econometric analysis for the United States. The analysis relies on data from the Current Population Survey (CPS), which includes more than 7 million individual records of workers over 2016-2021 and compares the evolution of labour market outcomes before and during the pandemic (see Box 3.4 for more details on the econometric analysis). First, the analysis compares the changes in workers' labour market outcomes with similar characteristics before and after the COVID shock and across different demographic groups. Second, it explores the differences in the outcomes between workers who were employed in occupations that are amenable to remote working and those who do not have jobs suitable to remote working.
The pandemic affected the U.S. labour market deeply
The pandemic affected all major labour market indicators in the U.S. Figure 3.6 presents the trend in labour market outcomes (vertical axis) over the period January 2018 to February 2021 (horizontal axis). The visuals show very large effects of COVID-19 on the U.S. labour markets, with a remarkable increase in the unemployment rate in March 2020 followed by a drastic increase in April 2020. More precisely, the unemployment rate increased from about 5% to 15% from February to April 2020. Following its peak in April 2020, the unemployment rate started declining gradually and dropped as low as 6% in December 2020.
The labour force participation also declined during the pandemic. Instead of entering unemployment, some workers dropped out of the labour force. More specifically, the participation rate decreased by 3 percentage points, from 62% to 59%, between March and April 2020, which corresponds to the largest monthly drop ever recorded in the U.S. (Lee, Park and Shin, 2021[8]). Interestingly, the sudden drop in the labour force participation rate turned out to be temporary. In fact, by July 2020, the participation rate had already bounced back to pre-crisis levels. However, since then, the participation rate has started its slow yet sustained decline, suggesting that some people who became unemployed might be discouraged to remain in the labour market. As these individuals exit the labour force, they reduce both the unemployment rate and labour force participation.
Since March 2020, the hours worked have slightly increased while the hourly wage has remained unchanged. One possible reason why hourly wages did not react to lower labour demand might be the slow adjustment of wages in the short run. Second, it is possible that workers with lower wages were the first ones to lose their jobs (Verdugo, 2016[9]). As these workers exit employment, the average wages of the remaining workers could have increased. If the wages dropped due to the lower labour demand during the crisis, the worker composition changes (i.e., where workers with lower salaries leave employment) and may have offset the decline as in the previous crisis. On the other hand, average working time per week increased by 1 hour, although it is difficult to understand whether it is due to the fact that workers who kept their jobs started working longer hours as in other recessions (Lazear, Shaw and Stanton, 2016[10]) or if those who worked in jobs requiring long hours of work managed to keep their jobs.
Figure 3.6. Covid-19 deeply affected the U.S. labour market

Note: The unemployment rate is calculated as the number of individuals who are unemployed as a percentage of the labour force. The labour force participation rate corresponds to the share of índividuals at work, who held a job but were temporarily absent from work due to factors like vacation or illness, were seeking work, or were temporarily laid off from a job during the reference period as a percentage of the noninstitutionalised civilian population. The number of hours worked for civilians aged 16-70 who are employed and either at work or absent from work during the survey week, all jobs. Trimmed to exclude values below 1st percentile and above 99th percentile. Hourly wages cover civilians aged 16-70 currently employed as wage/salary workers, paid hourly, and were in outgoing rotation groups. Excludes self-employed persons. Trimmed to exclude values below the 1st percentile and above the 99th.
Source: OECD calculations based on the Current Population Survey (CPS).
The short-run impact of COVID-19: An econometric analysis
Descriptive evidence presented in the previous section indicates that the COVID-19 crisis affected the labour market outcomes. While informative, these figures only provide suggestive evidence of the average effects across the labour market. However, early reporting on the labour market effects of the crisis suggests uneven consequences across different groups of workers. To address these questions, this section presents results from an econometric analysis as explained in Box 3.4
Using individual-level data, the regression analysis measures the change in individuals' labour market outcomes due to the COVID-19 pandemic. Figure 3.7 presents the regression results for the four labour market indicators, namely labour force participation rate, unemployment rate, average hours worked, and average hourly wages. Each panel presents results for a different labour market outcome, while each marker corresponds to the change since March 2020 (or Post-COVID) relative to the levels in the same month in the previous years for each population group. All the regressions take into account individual characteristics (such as education, age or sex) that may affect labour market outcomes. The analysis also accounts for the residence of the individual, as the crisis had an uneven effect across U.S. states. As such, the results should be seen as the change in the labour market outcomes for different groups, relative to the previous year and other groups that are also located within the same regional labour market.
The analysis shows that, since the beginning of the pandemic, there has been a substantial increase in the unemployment rate, a decrease in labour force participation, and a decrease in hours of work for all workers (Grey Marker) compared to the previous years. In contrast, no significant changes were observed for hourly wages. These effects, however, were uneven across groups. Young (aged 16 to 34), old (55 and above), low-skilled or migrant workers suffered the highest unemployment rate drops. While the unemployment rate increased by 3 percentage points for the overall population, the increase was larger, between 4-5 percentage points, for these most affected groups.
Similarly, these groups suffered the highest drops in labour force participation. As discussed above, while the hourly wages do not indicate any statistically significant change, the hours worked per week declined for all workers, more strongly for migrants and non-white minorities. Overall, these results suggest an increase in labour market inequalities.
Workers located in non-metropolitan areas were more than twice as likely to face unemployment compared to those located in metropolitan areas. The COVID-19 initiated discussions on whether the crisis would affect metropolitan areas more severely due to their higher reliance on the service sector, which requires face-to-face interactions (OECD, 2020[7]). Results from the econometric analysis (see Appendix ) suggest that individuals located in non-metropolitan areas were more than twice as likely to be unemployed compared to those located in the same U.S. state yet in a metropolitan area.2 On the other hand, the other labour market outcomes do not indicate any differential effect.
Figure 3.7. Unemployment increased, while labour force participation and hours worked declined

Note: Each panel presents regression results for a different labour market outcome. Each market corresponds to the coefficient (Post-COVID dummy) that captures the change in the labour market outcome since March 2020, relative to the levels in the previous years. Estimates are obtained from separate subgroup estimations. When possible, regressions control for individual characteristics (e.g., age, gender, education, marital status, race), state fixed-effects, month fixed-effects, year fixed-effects. Standard errors are clustered by U.S. states. The time period is January 2016 – January 2021.
Source: OECD calculations based on the Current Population Survey (CPS).
Box 3.4. Empirical strategy
The analysis in this section uses individual-level microdata and applies a simple pre/post strategy that has been used by other studies to study the effects of COVID-19 (Bacher-Hicks et al. 2020, Brodeur et al., 2021).
The estimation strategy relies on identifying the effects of the COVID-19 shock by comparing the evolution of labour market indicators for workers with similar observable characteristics (e.g., age, sex, education, etc.), before and after the COVID pandemic. The analysis uses the following model:
denotes the economic outcome for individual i, in state s¸ and month t. The four main outcome variables are the unemployment rate, labour force participation, hours of work, hourly wages
is an indicator equal to one for March and April 2020, and zero for all preceding months. The time period is January 2016 to January 2021
is a vector of demographic controls including age, gender, marital status and race
is a state fixed-effect that absorbs structural differences between states
is the time fixed-effect to account for cyclical differences by absorbing differences across months
Standard errors clustered at the state-level
Data
The estimation is based on data from the Current Population Survey (CPS), collected by the Bureau of Labor Statistics (BLS), and provided by the Integrated Public Use Micro Samples (IPUMS). The CPS is a monthly survey of 60 000 eligible households. The CPS provides a large sample size of workers and individual characteristics such as age, education, race, and marital status and labour market characteristics such as labour force participation, employment status, hours of work, occupation and industry. The survey questions refer to activities during the week that includes the 12th of the month.
Source: U.S. Bureau of Labor Statistics: 2020, Frequently asked questions: The impact of the coronavirus (COVID-19) pandemic on The Employment Situation for March 2020. April 3, 2020. Bacher-Hicks, A, Goodman, J., Mulhern, C., 2021. "Inequality in household adaptation to schooling shocks: Covid-induced online learning engagement in real time" Journal of Public Economics, vol. 193. Brodeur, A., Clark, A.E., Fleche, S. and Powdthavee, N., 2021. "COVID-19, lockdowns and well-being: Evidence from Google Trends". Journal of Public Economics, vol. 193.
Remote working shielded workers against the adverse effect of the crisis
Prior to the COVID-19 pandemic, only a tiny share of workers made use of remote working opportunities. Remote working was considered an option that would provide workers flexibility, allowing them to work beyond office hours or to occasionally adjust their schedule depending on their family needs such as elderly or childcare. Prior to the COVID-19 pandemic, only one-fourth of workers using digital equipment at work had worked from home at least once a week in the E.U. (OECD, 2019[11]). In April 2020, close to 40% of workers in the E.U. were working from home on a full-time basis (Eurofound, 2020[12]). According to surveys, numerous companies worldwide consider shifting to mass teleworking permanently even after the COVID-19 health crisis will have abated (OECD, 2020[13]).
There are arguments based on economic theory suggesting that workers who could work from home during the pandemic face lower risks of job and income losses. Individuals who are able to keep their professional activities going are more likely to stay employed as they are able to produce and generate revenues for their enterprises. In other words, labour demand for occupations that can work remotely is likely to drop less severely than those which cannot. Evidence from jobs postings in European capitals indicates a consistent trend. While the number of job postings decreased dramatically across European capitals during the pandemic, the drop was more tempered for jobs with high remote working potential (Adrjan and Kleine-Rueschkamp, 2021[1]).
Despite the relevance of the issue, little is known about the importance of the remote working capacity for individuals' employment outcomes during the crisis. The remainder of this section explores the uneven effect of the crisis on workers who can work remotely versus those who cannot. First, it presents descriptive evidence on changes in the primary labour market indicators separately for both groups. In a second step, it presents more detailed evidence based on the econometric analysis.
Individuals with higher remote working potential were affected less severely
Before moving to the regression analysis, visualising the overall trends is important to get an initial idea about the differences in the labour market outcomes of workers who can and cannot work remotely. Figure 3.8 presents the evolution of labour market outcomes (vertical axis) over the period of January 2018 to February 2021 (horizontal axis) for workers by remote working potential. For the sake of simplicity, workers who are employed in occupations that have a remote working score above the mean are considered to be able to remotely work, while those below the mean are considered to be unable to work from home.
Before the crisis, labour market outcomes for both groups evolved similarly and labour market indicators exhibited different levels driven by the nature of occupations. On average, individuals working in jobs with high remote working potential participated more in the labour market, were less likely to experience unemployment, earned higher hourly wages and worked longer hours. Despite their differences, the visuals show that the two groups' labour market outcomes evolved in parallel trends until the crisis.
Labour market outcomes for both groups diverged during the crisis. The visuals show that the parallel change in both groups' trends was disrupted during the pandemic, and the outcomes started diverging. While both groups were affected by the crisis, workers with a below-average capacity of remote working were affected more severely. For example, while both groups experienced an increase in their unemployment rates by April 2020, the increase was significantly larger for those who cannot work from home. Similarly, a larger share of these workers dropped out of the labour force compared to workers who can work remotely.
Figure 3.8. Covid-19 triggered divergence in labour market outcomes between workers with high- and low-remote working potential

Note: Panels plot the evolution of labour market indicators separately for workers who can work remotely vs. those who cannot. Workers are categorised following the occupational classification of Dingel and Neiman (2020[4]). The unemployment rate is calculated as the number of individuals who are unemployed as a percentage of the labour force. The labour force participation rate is the share of individuals at work, held a job but were temporarily absent from work due to factors like vacation or illness; were seeking work, or were temporarily laid off from a job during the reference period as a percentage of the noninstitutionalised civilian population. The number of hours worked for civilians aged 16-70 who are employed and either at work or absent from work during the survey week, all jobs. Trimmed to exclude values below the 1st percentile and above the 99th percentile. Hourly wages cover civilians aged 16-70 currently employed as wage/salaried workers, paid hourly, and were in outgoing rotation groups. Excludes self-employed persons. Trimmed to exclude values below the 1st percentile and above the 99th.
Source: OECD calculations based on the Current Population Survey (CPS)
The econometric analysis also confirms the divergence in labour market outcomes. Figure 3.9 presents the regression analysis for the four labour market indicators separately for workers with high and low remote working capacity. Each panel shows results for a different labour market outcome, while each marker corresponds to the change since March 2020 (or Post-COVID) relative to the levels in the same month in the previous years for each population group.3 Results indicate that workers who cannot work remotely are twice as likely to be unemployed and 75% more likely to drop out of the labour force than those who can work. In contrast, there is no statistically significant difference in wages and hours worked.
Figure 3.9. Those who can work remotely were less affected by the crisis

Note: Each panel presents regression results for a different labour market outcome separately for workers who can work remotely vs. those who cannot. Workers are categorised following the occupational classification of Dingel and Neiman (2020[2]). Each market corresponds to the coefficient (Post-COVID dummy) that captures the change in the labour market outcome since March 2020, relative to the levels in the previous years. Estimates are obtained from separate subgroup estimations. When possible, regressions control for individual characteristics (e.g., age, gender, education, marital status, race), state fixed-effects, month fixed-effects, year fixed-effects. Standard errors are clustered by U.S. states. The time period is January 2016 – January 2021.
Source:OECD calculations based on the Current Population Survey (CPS)
Going beyond the pandemic: Rebuilding an inclusive labour market
The COVID-19 crisis has further exacerbated, in certain cases, pre-existing labour market inequalities. The labour market impact of the crisis has been highly uneven across workers. Similar to the previous crisis, once again, the most disadvantaged groups of workers, including low-skilled, low-wage workers, migrants and young people, have suffered the most in many OECD countries (Adams-Prassl et al., 2020[14]; Alstadsaeter et al., 2020[15]; Beland, Brodeur and Wright, 2020[16]; Belot et al., 2020[17]). Furthermore, these groups also lack the financial resources to deal with income losses, which make them vulnerable. As the workers who suffer from income losses also have little capacity to mitigate those shocks, income support can be an important rescue in the short run.
Young people have again been hit hard relative to the rest of the population, like during the global financial crisis (OECD, 2016[18]). This year's graduates, sometimes referred to as the "Class of Corona", are leaving schools and universities with often very poor chances of quickly finding employment or work experience. Meanwhile, their older peers are already experiencing the second heavy economic crisis in their still-young careers. The initial labour market experience profoundly influences the later working life, and a crisis can have long-lasting scarring effects on employment and earnings perspectives.
Job losses during the COVID-19 crisis will have effects that go beyond short-term income losses leading to negative long-term labour market outcomes. Individuals who experience employment losses during a large crisis suffer longer unemployment periods or must cope with lower-quality jobs (Davis and Von Wachter, 2011[19]). Many will find it hard to find a new job with similar responsibility, pay and career opportunity as the lost job. Workers who lose their jobs during a crisis, such as the current one, are also more likely to leave the labour market and become inactive. Looking at the Great Recession in the US, Yagan (2019[20]) shows that while unemployment rates recovered to their pre-crisis levels, the labour force participation rates declined and never recovered to their pre-crisis levels even six years after the end of the crisis. Thus, it is essential to develop active labour market policies that support workers who have lost their jobs during this period by encouraging their return to the labour market while helping them find good quality jobs.
Remote working will be part of the future
While containment measures have restricted economic activity in some sectors, the rapid expansion of remote working has helped maintain other jobs during the COVID-19 crisis. The disruptions to existing working arrangements caused by COVID-19 have led to a major increase in remote working out of necessity. Early in the crisis, Dingel and Neiman (2020[4]) suggested that some occupations might have greater remote working potential.4 Evidence presented in the previous section suggests that workers with the capacity to work remotely are likely to have significantly lower risks of deterioration in labour market outcomes.
Remote working will be part of the future of work. However, predicting the actual share of remote workers once the health crisis will be over remains speculative. Remote working has many advantages for workers and firms, which indicates that there are reasons to believe that it will be part of the future. For instance, employees who work from home save time and money on commuting. Flexibility in working hours could also be preferable as it makes it possible to adjust working hours to the person's private needs, such as childcare. From the employer's perspective, such flexibility would reduce costs associated with renting or owning office space. Fewer people working on-site would allow firms to reduce rental costs, electricity and heating bills. In large cities such as London, New York or Tokyo, where office rents are high, office space reduction could substantially increase savings. In fact, surveys conducted across countries indicate that both employers and employees want to integrate remote working into their work-life even after the pandemic has receded (Taneja, Mizen and Bloom, 2021[21]).
Being able to work remotely will not benefit everyone equally, especially low-skilled workers. Individuals with a low level of formal education have little chance to work remotely due to the nature of their jobs' tasks. Such workers would not be able to seize such an opportunity, at least in the short run. While active labour market policies should be offered to help those who lost their jobs during the crisis back into employment, training programmes should be developed in parallel to upskill and prepare workers who are not able to work remotely.
Conclusion
The COVID-19 pandemic has led to a health crisis and to a labour market shock, putting unprecedented pressure on people, places, and firms. While people were forced to stay home under lockdowns, economic activity was interrupted, leading to declining GDP and rising unemployment. In many countries, the adverse labour market effects were particularly strong for already disadvantaged groups of workers, often overrepresented outside dense cities.
Relying on in-depth analysis on the U.S., the chapter also shows that workers living outside metropolitan areas were twice as likely to face unemployment as workers living in metropolitan areas. Without measures to support the large portions of workers hard hit by the pandemic, the current crisis stands to worsen further the conditions of already vulnerable groups and areas, potentially exacerbating already stark regional inequalities.
Remote working will be part of the future. As remote working becomes a permanent component of professional life, it will present new opportunities and bring about certain changes. As shown in Chapter 2, remote working has a strong regional dimension, with significantly higher concentrations of occupations amenable to remote working in dense regions or regions with easier access to cities. This chapter looks at how remote working could potentially influence the future through the lens of several individual characteristics, which are likely causing the observed spatial gaps, notably between cities and many areas with a lower population density. These characteristics include education, skills, and labour market outcomes. For example, highly skilled people are more likely to have jobs that are compatible with remote working, but rural areas offer lower remote working potential across all types of skills compared to cities.
How governments address the new normality of remote working will affect both territorial inequalities and those based on socio-economic conditions. It is essential that policies encourage the return to the labour market jointly address the unemployment crisis and the transition to remote working while simultaneously taking into consideration the socio-economic characteristics of the labour force within territories.
References
[14] Adams-Prassl, A. et al. (2020), “Inequality in the Impact ofthe Coronavirus Shock: Evidence from Real Time Surveys”, CEPR Discussion Paper 14665.
[1] Adrjan, P. and L. Kleine-Rueschkamp (2021), After the Boom? COVID-19 and European City Labour Market, HiringLab, https://www.hiringlab.org/uk/blog/2021/02/19/after-the-boom-covid-19-and-european-city-labour-markets/.
[2] Alipour, J., H. Fadinger and J. Schymik (2021), “My home is my castle – The benefits of working from home during a pandemic crisis”, Journal of Public Economics, Vol. 196, p. 104373, http://dx.doi.org/10.1016/j.jpubeco.2021.104373.
[15] Alstadsaeter, A. et al. (2020), “The First Weeks of the Coronavirus Crisis: Who Got Hit, When and Why? Evidence from Norway”, NBER Working Paper 2131.
[3] Angelucci, M. et al. (2020), “Remote Work and the Heterogeneous Impact of COVID-19 on Employment and Health”, National Bureau of Economic Research, http://dx.doi.org/10.3386/w27749.
[16] Beland, L., A. Brodeur and T. Wright (2020), “COVID-19, Stay-at-Home Orders and Employment: Evidence from CPS Data”, IZA Discussion Paper 13282.
[17] Belot, M. et al. (2020), “Unequal Consequences of COVID-19 across Age and Income: Representative Evidence from Six Countries”, IZA Working paper 13366.
[22] Bick, A., A. Blandin and K. Mertens (2020), “Work from Home After the COVID-19 Outbreak”, Federal Reserve Bank of Dallas, Working Papers, Vol. 2020/2017, http://dx.doi.org/10.24149/wp2017r1.
[19] Davis, S. and T. Von Wachter (2011), “Recessions and the cost of job loss”.
[4] Dingel, J. and B. Neiman (2020), “How Many Jobs Can be Done at Home?”, Becker Friedman Institute White Paper March, https://bfi.uchicago.edu/working-paper/how-many-jobs-can-be-done-at-home/.
[12] Eurofound (2020), Living, working and COVID-19: First findings – April 2020, https://www.eurofound.europa.eu/publications/report/2020/living-working-and-covid-19-firstfindings-%0Aapril-2020.
[6] Gottlieb, C., J. Grobovsek and M. Poschke (2020), “Working from home across countries”, COVID economics, Vol. 8.
[10] Lazear, E., K. Shaw and C. Stanton (2016), “Making Do with Less: Working Harder during Recessions”, Journal of Labor Economics, Vol. 34/2.
[8] Lee, S., M. Park and Y. Shin (2021), “Hit Harder, Recover Slower? Unequal Employment Effects of the Covid-19 Shock”, http://dx.doi.org/10.3386/W28354.
[7] OECD (2020), “Capacity for remote working can affect shutdowns’ costs differently across places”, OECD COVID-19 Policy Note, http://www.oecd.org/coronavirus/policy-responses/capacity-for-remote-working-can-affect-lockdown-costs-differently-across-places-0e85740e/.
[13] OECD (2020), “Exploring policy options on teleworking: Steering local economic and employment development in the time of remote work”, OECD Local Economic and Employment Development (LEED) Papers, Vol. 2020/10.
[11] OECD (2019), Measuring the Digital Transformation: A Roadmap for the Future, OECD Publishing, Paris, https://dx.doi.org/10.1787/9789264311992-en.
[18] OECD (2016), Society at a Glance 2016: OECD Social Indicators, OECD Publishing, Paris.
[5] Saltiel, F. (2020), “Home working in developing countries”, COVID economics, Vol. 6.
[23] Scarpetta, S., A. Sonnet and T. Manfredi (2010), “Rising youth unemployment during the crisis: How to prevent negative long-term consequences on a generation?”, OECD Social, Employment and Migration Working Papers, No. 106, Vol. 106/106, pp. 1-47, http://thefamilywatch.org/doc/doc-0077-es.pdf.
[21] Taneja, S., P. Mizen and N. Bloom (2021), “Working from home: The polarising workplace”, Vox EU blogpiece, https://voxeu.org/article/working-home-revolutionising-uk-labour-market.
[9] Verdugo, G. (2016), “Real wage cyclicality in the Eurozone before and during the Great Recession: Evidence from micro data”, European Economic Review, Vol. 82, pp. 46-69, http://dx.doi.org/10.1016/j.euroecorev.2015.11.001.
[20] Yagan, D. (2019), “Is the Great Recession Really Over? Longitudinal Evidence of Enduring Employment Impacts”, Journal of Political Economy (Forthcoming).
Annex 3.A. Technical Appendix
Definitions of variables
The unemployment rate: The number of persons unemployed as a percentage of the total labour force.
Labor force participation: Individuals in the labour force who are currently employed; held a job but were temporarily absent from work due to factors like vacation or illness; were seeking work; or were temporarily laid off from a job during the reference period.
Hours of work: Computed for civilians aged 16-70 who are employed and either at work or absent from work during the survey week, including all types of jobs. Hours of work is trimmed to exclude values below the 1st percentile and above the 99th percentile.
Hourly wages: The hourly wages (in 2018 constant dollars) is computed for civilians aged 16-70 currently employed as wage/salary workers, paid hourly and were in outgoing rotation groups. It excludes self-employed persons, and we trim to exclude values below the 1st percentile and above the 99th percentile.
Descriptive statistics
Annex Table 3.A.1. Descriptive Statistics
No. of observations |
Mean |
S.D |
Max |
Min |
|
---|---|---|---|---|---|
Labour force participation |
5 229 284 |
0.62 |
0.48 |
0 |
1 |
Unemployed |
3 195 149 |
004 |
0.21 |
0 |
1 |
Real hourly wages (2018 dollars) |
352 931 |
19.1 |
10.35 |
9.08 |
101.73 |
Hours worked last week |
2 695 661 |
39.13 |
12.12 |
1 |
100 |
Note: The labour force participation rate corresponds to the share of individuals currently employed, held a job but were temporarily absent from work due to factors like vacation or illness; were seeking work, or were temporarily laid off from a job during the reference period as a percentag of the noninstitutionalised civilian population. Number of hours worked for civilians aged 16-70 who are employed and either at work or absent from work during the survey week, all jobs. Trimmed to exclude values below the 1st percentile and above the 99th percentile. Hourly wages covers civilians aged 16-70 currently employed as wage/salary workers, paid hourly, and were in outgoing rotation groups. Excludes self-employed persons. Trimmed to exclude values below the 1st percentile and above the 99th.
Source: OECD calculations based on the Current Population Survey (CPS)
Regression results
Annex Table 3.A.2. The impact of COVID-19 on labour market outcomes by different worker groups
Unemployment |
Labour Force Participation |
Hourly wage |
Hours worked |
|
---|---|---|---|---|
All |
0.0569 (0.003)*** |
-0.0194 (0.002)*** |
0.1178 (0.154) |
-0.9856 (0.066)*** |
Young |
0.0858 (0.006)*** |
-0.0350 (0.005)*** |
0.0133 (0.136) |
-0.6834 (0.163)*** |
Old |
0.0540 (0.004)*** |
-0.0119 (0.004)*** |
0.4709 (0.421) |
-0.6435 (0.144)*** |
High-Skilled |
0.0370 (0.003)*** |
-0.0163 (0.003)*** |
0.6195 (0.393) |
-0.9495 (0.108)*** |
Low-Skilled |
0.0697 (0.004)*** |
-0.0208 (0.003)*** |
-0.0284 (0.139) |
-0.9888 (0.090)*** |
Married |
0.0448 (0.003)*** |
-0.0156 (0.002)*** |
0.2122 (0.256) |
-0.9687 (0.100)*** |
White |
0.0532 (0.003)*** |
-0.0174 (0.002)*** |
0.0800 (0.173) |
-1.0062 (0.095)*** |
Black |
0.0688 (0.005)*** |
-0.0270 (0.006)*** |
0.0846 (0.306) |
-1.0710 (0.164)*** |
Hispanic |
0.0766 (0.006)*** |
-0.0252 (0.006)*** |
0.4020 (0.233)* |
-1.2262 (0.108)*** |
Migrants |
0.0769 (0.007)*** |
-0.0283 (0.007)*** |
0.1551 (0.405) |
-1.5544 (0.205)*** |
Observations |
3 653 951 |
6 003 971 |
399 658 |
3 024 769 |
Individual characteristics |
Yes |
Yes |
Yes |
Yes |
State Fixed-Effect |
Yes |
Yes |
Yes |
Yes |
Month Fixed-Effect |
Yes |
Yes |
Yes |
Yes |
Year Fixed-Effect |
Yes |
Yes |
Yes |
Yes |
Note: Each column presents regression results for a different labour market outcome, while each row corresponds to the estimates for each worker group. The coefficients correspond to the Post-COVID dummy that has captured the change in the labour market outcome since March 2020, relative to the levels in the previous years. Estimates are obtained from separate subgroup estimations. When possible, regressions control for individual characteristics (e.g., age, gender, education, marital status, race), state fixed-effects, month fixed-effects, year fixed-effects. Standard errors are clustered by U.S. states. The time period is January 2016 – January 2021. The number of observations refers to the regressions with the entire sample.
Source: OECD calculations based on the Current Population Survey (CPS)
Annex Figure 3.A.1. Labour market outcomes for metropolitan and non-metro areas

Note:Each panel presents regression results for a different labour market outcome separately for workers located in metropolitan areas vs. those who do not live in a metropolitan area. Each market corresponds to the coefficient (Post-COVID dummy) that has captured the change in the labour market outcome since March 2020, relative to the levels in the previous years. Estimates are obtained from separate subgroup estimations. When possible, regressions control for individual characteristics (e.g., age, gender, education, marital status, race), state fixed-effects, month fixed-effects, year fixed-effects. Standard errors are clustered by U.S. states. The time period is January 2016 – January 2021.
Source:OECD calculations based on the Current Population Survey (CPS)
Annex Table 3.A.3. The impact of COVID-19 on labour market outcomes by different remote working potential
Unemployment |
Labour Force Participation |
Hourly wage |
Hours worked |
|
---|---|---|---|---|
High remote work |
0.0383 (0.003)*** |
-0.0037 (0.001)*** |
0.2834 (0.249) |
-1.0128 (0.076)*** |
Low remote work |
0.0788 (0.005)*** |
-0.0066 (0.001)*** |
0.3551 (0.218) |
-1.0534 (0.114)*** |
Observations |
3 346 375 |
3 363 894 |
376 941 |
2 791 579 |
Individual characteristics |
Yes |
Yes |
Yes |
Yes |
State Fixed-Effect |
Yes |
Yes |
Yes |
Yes |
Month Fixed-Effect |
Yes |
Yes |
Yes |
Yes |
Year Fixed-Effect |
Yes |
Yes |
Yes |
Yes |
Note: Each column presents regression results for a different labour market outcome separately for workers who can work remotely vs. those who cannot. Workers are categorised following the occupational classification of Dingel and Neiman (2020[2]). Each market corresponds to the coefficient (Post-COVID dummy) that has captured the change in the labour market outcome since March 2020, relative to the levels in the previous years. Estimates are obtained from separate subgroup estimations. When possible, regressions control for individual characteristics (e.g., age, gender, education, marital status, race), state fixed-effects, month fixed-effects, year fixed-effects. Standard errors are clustered by U.S. states. The time period is January 2016 – January 2021.
Source: OECD calculations based on the Current Population Survey (CPS)
Notes
← 1. Remote working is viable only assuming the continuation of the activity in the sector as a whole. However, if demand in a specific sector collapses completely, all workers employed in the sector will suffer and may have to cease working, regardless of whether their occupation could be performed remotely or not (OECD, 2020[7]).
← 2. Individuals are grouped into metropolitan and non-metropolitan areas based on the variable METRO provided in the CPS data, which refers to the household location.
← 3. Like in the previous analysis, all the regressions take into account individual characteristics (such as education, age or sex) that may affect the labour market outcomes, and the residence of the individual as the crisis had uneven affect across states. As such, the results should be seen as the change in the labour market outcomes for both groups, relative to the previous years and to one another within the same local labour market.
← 4. Data on actual remote working in the US during the crisis confirmed that Dingel and Neiman’s occupational classification captured well the remote working potential (Bick, Blandin and Mertens, 2020[22]).