Artificial intelligence (AI) is increasingly deployed by financial services providers across industries within the financial sector. It has the potential to transform business models and markets for trading, credit and blockchain-based finance, generate efficiencies, reduce friction and enhance the product offerings. With this potential comes the concern that AI could also amplify risks already present in financial markets, or give rise to new challenges and risks. This is becoming more of a preoccupation amidst the high growth of AI applications in finance. This chapter examines how policy makers can support responsible AI innovation in the financial sector, while ensuring that investors and financial consumers are duly protected and that the markets around such products and services remain fair, orderly and transparent. The chapter reviews benefits and challenges associated with data management; explainability and the robustness and resilience of machine learning models and their governance. It suggests policy recommendations to mitigate such risks and promote the safe development of AI use-cases in finance.
OECD Business and Finance Outlook 2021
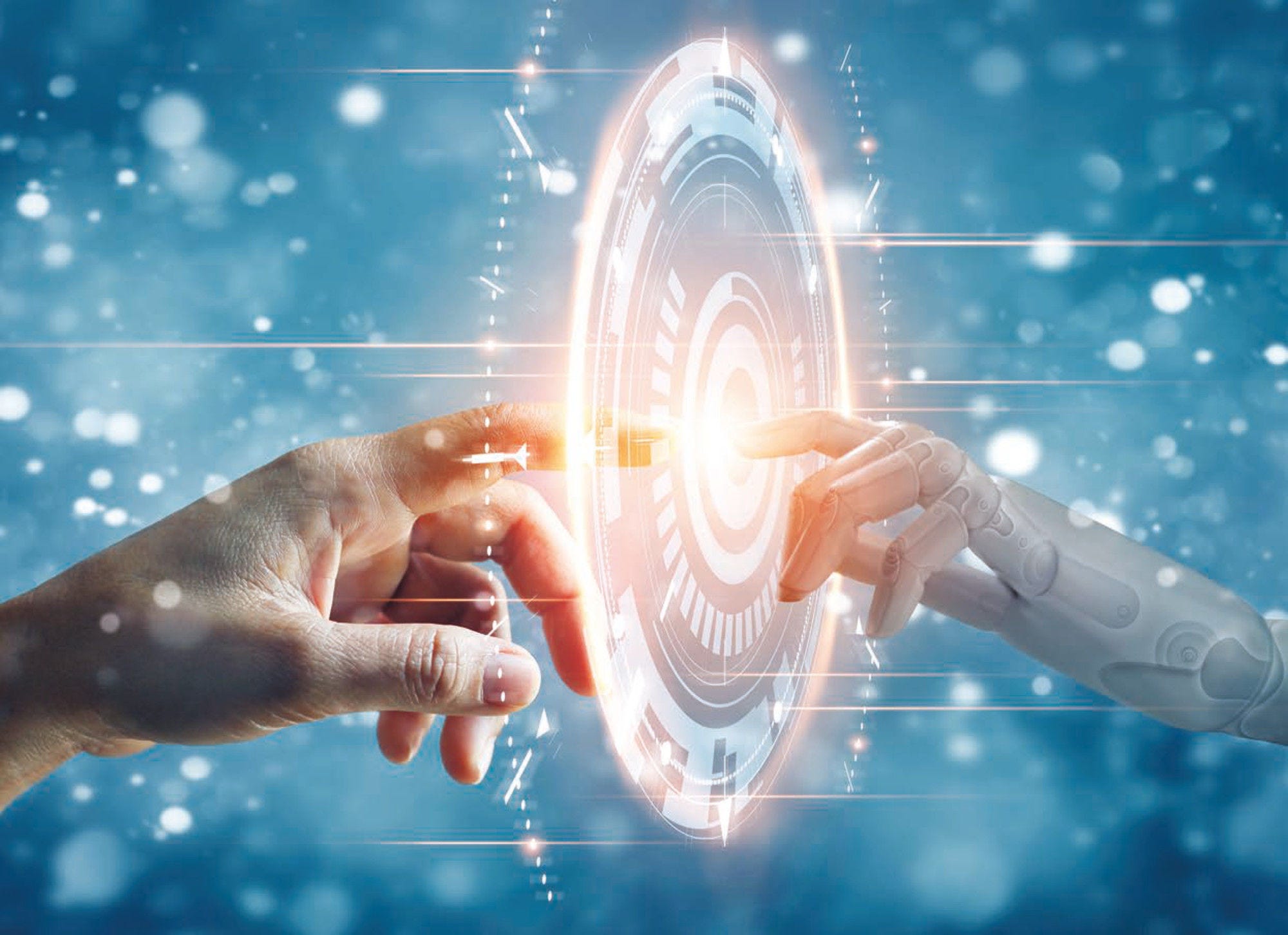
2. AI in finance
Copy link to 2. AI in financeAbstract
2.1. Introduction
Copy link to 2.1. IntroductionThe adoption of artificial intelligence (AI)1 systems and techniques in finance has grown substantially, enabled by the abundance of available data and the increase in the affordability of computing capacity. This trend is expected to persist and some estimates forecast that global spending on AI will double over the period 2020-24, growing from USD50.1bn in 2020 to more than USD110bn in 2024 (IDC, 2020[1]).
AI is increasingly deployed by financial services providers across industries within the financial sector: in retail and corporate banking (tailored products, chat-bots for client service, credit scoring and credit underwriting decision-making, credit loss forecasting, anti-money laundering (AML), fraud monitoring and detection, customer service, natural language processing (NLP) for sentiment analysis); asset management (robo-advice, management of portfolio strategies, risk management); trading (AI-driven algorithmic trading, automated execution, process optimisation, back-office); insurance (robo-advice, claims management). Importantly, AI is also being deployed in RegTech and SupTech applications by financial authorities and the public sector (see Chapter 5).
The deployment of AI techniques in finance can generate efficiencies by reducing friction costs (e.g. commissions and fees related to transaction execution) and improving productivity levels, which in turn leads to higher profitability. In particular, the use of automation and technology-enabled cost reduction allows for capacity reallocation, spending effectiveness and improved transparency in decision-making. AI applications for financial service provision can also enhance the quality of services and products offered to financial consumers, increase the tailoring and personalisation of such products and diversify the product offering. The use of AI mechanisms can unlock insights from data to inform investment strategies, while it can also potentially enhance financial inclusion by allowing for the analysis of creditworthiness of clients with limited credit history (e.g. thin file SMEs).
At the same time, the use of AI could amplify risks already present in financial markets, or give rise to new challenges and risks (OECD, 2021[2]). This aspect is becoming more of a preoccupation as the deployment of AI in finance is expected to further grow in importance and ubiquitous-ness. The inappropriate use of data or the use of poor quality data could create or perpetuate biases and lead to discriminatory and unfair results at the expense of financial consumers, for example, by unintentionally replicating or enhancing existing biases in practices or data. The use of the same models or datasets can lead to convergence and herding behaviour, increasing volatility and amplifying liquidity shortages in times of market stress. Growing dependencies on third party providers and outsourcing of AI models or datasets raise issues around governance and accountability, while concentration issues and dependence on few large dominant players may also arise, given the important investment required for the deployment of AI techniques is based on in-house capabilities rather than outsourcing. Existing model governance frameworks may be insufficiently addressing risks associated with AI, while the absence of clear accountability frameworks may give rise to market integrity and compliance risks. Novel risks arise from the difficulty in understanding how AI-based models generate results, what is generally referred to as ‘explainability’, and the lack of explainability can give rise to incompatibilities with existing regulatory and supervisory requirements. The increased use of AI in finance could also lead to potential increased interconnectedness in the markets, while a number of operational risks related to such techniques could pose threat to the resilience of the financial system in times of stress.
Against this backdrop, this chapter examines how policy makers can support responsible AI innovation in the financial sector, while ensuring that investors and financial consumers are duly protected, and the markets around such products and services remain fair, orderly and transparent. The chapter reviews the potential transformative effect of AI on certain financial market activities, key benefits, emerging challenges and risks from the use of such techniques, and discusses associated policy implications.
Section one provides an overview of the use of AI in certain parts of the financial markets, and examines how the deployment of AI techniques could affect the business models of specific financial market activity: asset management, trading, credit intermediation and blockchain-based financial services. It highlights the expected benefits and potential unintended consequences of AI use-cases in these areas of finance, and examines how risks stemming from AI interact with existing risks.
Section two reviews some of the main challenges emerging from the deployment of AI in finance. It focuses on data-related issues, the lack of explainability of AI-based systems; robustness and resilience of AI models and governance considerations.
Section three offers policy implications from the increased deployment of AI in finance, and policy considerations that support the use of AI in finance while addressing emerging risks. It provides policy recommendations that can assist policy makers in supporting AI innovation in finance, while sharpening their existing arsenal of defences against risks emerging from, or exacerbated by, the use of AI.
2.2. AI and financial activity use-cases
Copy link to 2.2. AI and financial activity use-casesAI is increasingly adopted by financial firms trying to benefit from the abundance of available big data datasets and the growing affordability of computing capacity, both of which are basic ingredients of machine learning (ML) models. Financial service providers use these models to identify signals and capture underlying relationships in data in a way that is beyond the ability of humans. However, the use-cases of AI in finance are not restricted to ML models for decision-making and expand throughout the spectrum of financial market activities (Figure 2.1). Research published in 2018 by Autonomous NEXT estimates that implementing AI has the potential to cut operating costs in the financial services industry by 22% by 2030.
This section looks at how AI and big data can influence the business models and activities of financial firms in the areas of asset management and investing; trading; lending; and blockchain applications in finance.
Figure 2.1. Examples of AI applications in financial market activities
Copy link to Figure 2.1. Examples of AI applications in financial market activities
2.2.1. Asset management2 and the buy-side
Copy link to 2.2.1. Asset management<a id="back-endnotea2z3" href="/content/oecd/en/publications/2021/09/oecd-business-and-finance-outlook-2021_377c2c18/full-report/component-6.html#endnotea2z3" style="vertical-align: top;font-size: 0.8em;">2</a> and the buy-sideAsset managers and the buy-side of the market have used AI for a number of years already, mainly for portfolio allocation, but also to strengthen risk management and back-office operations. The use of AI techniques has the potential to create efficiencies at the operational workflow level by reducing back-office costs of investment managers, automating reconciliations and increasing the speed of operations, ultimately reducing friction (direct and indirect transaction costs) and enhancing overall performance by reducing noise (irrelevant features and information) in decision-making (Blackrock, 2019[3]) (Deloitte, 2019[4]). AI is also used by asset managers and other institutional investors to enhance risk management, as ML allow for the cost-effective monitoring of thousands of risk parameters on a daily basis, and for the simulation of portfolio performance under thousands of market/economic scenarios.
The main use-case of AI in asset management is for the generation of strategies that influence decision-making around portfolio allocation, and relies on the use of big data and ML models trained on such datasets. Information has historically been at the core of the asset management industry and the investment community as a whole, and data has been the cornerstone of many investment strategies before the advent of AI (e.g. fundamental analysis, quantitative strategies or sentiment analysis). The abundance of vast amounts of raw or unstructured data, coupled with the predictive power of ML models, provides a new informational edge to investors who use AI to digest such vast datasets and unlock insights that then inform their strategies at very short timeframes.
Figure 2.2. Use of AI techniques by hedge funds (H1 2018)
Copy link to Figure 2.2. Use of AI techniques by hedge funds (H1 2018)Given the investment required by firms for the deployment of AI strategies, there is potential risk of concentration in a small number of large financial services firms, as bigger and more powerful players may outpace some of their smaller rivals (Financial Times, 2020[6]). Such investment is not constrained in monetary resources required to be invested in AI technologies but also relates to talent and staff skills involved in such techniques. Such risk of concentration is somewhat curbed by the use of third-party vendors; however, such practice raises other challenges related to governance, accountability and dependencies on third parties (including concentration risk when outsourcing is involved) (see Section 2.3.5).
Importantly, the use of the same AI algorithms or models by a large number of market participants could lead to increased homogeneity in the market, leading to herding behaviour and one-way markets, and giving rise to new sources of vulnerabilities. This, in turn, translates into increased volatility in times of stress, exacerbated through the simultaneous execution of large sales or purchases by many market participants, creating bouts of illiquidity and affecting the stability of the system in times of market stress.
2.2.2. Algorithmic Trading
Copy link to 2.2.2. Algorithmic TradingAI in trading is used for core aspects of trading strategies, as well as at the back-office for risk management purposes. Traders can use AI to identify and define trading strategies; make decisions based on predictions provided by AI-driven models; execute transactions without human intervention; but also manage liquidity, enhance risk management, better organise order flows and streamline execution. When used for risk management purposes, AI tools allow traders to track their risk exposure and adjust or exit positions depending on predefined objectives and environmental parameters, without (or with minimal) human intervention. In terms of order flow management, traders can better control fees and/or liquidity allocation to different pockets of brokers (e.g. regional market-preferences, currency determinations or other parameters of an order handling) (Bloomberg, 2019[7]).
Strategies based on deep neural networks can provide the best order placement and execution style that can minimise market impact (JPMorgan, 2019[8]). Deep neural networks mimic the human brain through a set of algorithms designed to recognise patterns, and are less dependent on human intervention to function and learn (IBM, 2020[9]). Traders can execute large orders with minimum market impact by optimising size, duration and order size of trades in a dynamic manner based on market conditions. The use of such techniques can be beneficial for market makers in enhancing the management of their inventory, reducing the cost of their balance sheet.
AI tools and big data are augmenting the capabilities of traders to perform sentiment analysis so as to identify themes, trends, patterns in data and trading signals based on which they devise trading strategies. While non-financial information has long been used by traders to understand and predict stock price impact, the use of AI techniques such as NLP brings such analysis to a different level. Text mining and analysis of non-financial big data (such as social media posts or satellite data) with AI allows for automated data analysis at a scale that exceeds human capabilities. Considering the interconnectedness of asset classes and geographic regions in today’s financial markets, the use of AI improves significantly the predictive capacity of algorithms used for trading strategies.
The most disruptive potential of AI in trading comes from the use of AI techniques such as evolutionary computation, deep learning and probabilistic logic for the identification of trading strategies and their automated execution without human intervention. Although algorithmic trading has been around for some time (see Figure 2.4), AI-powered algorithms add a layer of development and complexity to traditional algorithmic trading, evolving into fully automated, computer-programmed algorithms that learn from the data input used and rely less on human intervention. Contrary to systematic trading, reinforcement learning allows the model to adjust to changing market conditions, when traditional systematic strategies would take longer to adjust parameters due to the heavy human involvement.
Figure 2.3. Historical evolution of trading and AI
Copy link to Figure 2.3. Historical evolution of trading and AI
What is more, the use of ML models shifts the analysis towards prediction and real-time trend analysis instead of conventional back-testing strategies based on historical data, for example through the use of ‘walk forward’ tests3 instead of back testing.4 Such tests predict and adapt to trends in real time to reduce over-fitting in back tests based on historical data and trends (Liew, 2020[10]), and overcome the limitation of predictions based on historical data when previously identified trends break down.
While conventional algorithms have been used to detect ‘high informational events’ and provide speed of execution (e.g. in high frequency trading or HFT), more advanced forms of AI-based algorithms are currently being used to identify signals from ‘low informational value’ events in flow-based trading.5 Such events consist of harder to identify events that are difficult to extract value from. As such, rather than provide speed of execution to front-run trades, AI at this stage is being used to extract signal from noise in data and convert this information into trade decisions. As AI techniques develop, however, it is expected that these algos will allow for the amplification of ‘traditional’ algorithm capabilities particularly at the execution phase. AI could serve the entire chain of action around a trade, from picking up signal, to devising strategies, and automatically executing them without any human intervention, with implications for financial markets.
AI algorithms, HFT and potential unintended consequences
Copy link to AI algorithms, HFT and potential unintended consequencesThe application of AI techniques in algorithmic and high-frequency trading (HFT) trading can increase market volatility and create bouts of illiquidity or even flash crashes, with possible implications for the stability of the market and for liquidity conditions particularly during periods of acute stress. Although HFT is an important source of liquidity for the markets under normal market conditions, improving market efficiency, any disruption in their operation can lead to the opposite results with liquidity being pulled out of the market, amplifying stress in the market and potentially affecting market resilience.
The possible simultaneous execution of large sales or purchases by traders using the similar AI-based models could give rise to new sources of vulnerabilities (FSB, 2017[11]). Indeed, some algo-HFT strategies appear to have contributed to extreme market volatility, reduced liquidity and exacerbated flash crashes that have occurred with growing frequency over the past several years (OECD, 2019[12]) . In addition, the use of ‘off-the-shelf’ algorithms by a large part of the market could prompt herding behaviour, convergence and one-way markets, further amplifying volatility risks, pro-cyclicality, and unexpected changes in the market both in terms of scale and in terms of direction. In the absence of market makers willing to act as shock-absorbers by taking on the opposite side of transactions, such herding behaviour may lead to bouts of illiquidity, particularly in times of stress when liquidity is most important.
At the single trader level, the lack of explainability of ML models used to devise trading strategies makes it difficult to understand what drives the decision and adjust the strategy as needed in times of poor performance. Given that AI-based models do not follow linear processes (input A caused trading strategy B to be executed) which can be traced and interpreted, users cannot decompose the decision/model output into its underlying drivers to adjust or correct it. Similarly, in times of over-performance, users are unable to understand why the successful trading decision was made, and therefore cannot identify whether such performance is due to the model’s superiority and ability to capture underlying relationships in the data or to other unrelated factors. That said, there is no formal requirement for explainability for human-initiated trading strategies, although the rational underpinning these can be easily expressed by the trader involved.
It should be noted that the massive take-up of third-party or outsourced AI models or datasets by traders could benefit consumers by reducing available arbitrage opportunities, driving down margins and reducing bid-ask spreads. At the same time, the use of the same or similar standardised models by a large number of traders could lead to convergence in strategies and could contribute to amplification of stress in the markets, as discussed above. Such convergence could also increase the risk of cyber-attacks, as it becomes easier for cyber-criminals to influence agents acting in the same way rather than autonomous agents with distinct behaviour (ACPR, 2018[13]).
Box 2.1. Safeguarding mechanisms built in trading systems
Copy link to Box 2.1. Safeguarding mechanisms built in trading systemsA number of defences are available to traders wishing to mitigate some of the unintended consequences of AI-driven algorithmic trading, such as automated control mechanisms, referred to as ‘kill switches’. These mechanisms are the ultimate line of defence of traders, and instantly switch off the model and replace technology with human handling when the algorithm goes beyond the risk system and do not behave in accordance with the intended purpose. In Canada, for instance, firms are required to have built-in ‘override’ functionalities that automatically disengage the operation of the system or allows the firm to do so remotely, should need be (IIROC, 2012[14]).
Kill switches and other similar control mechanisms need to be tested and monitored themselves, to ensure that firms can rely on them in case of need. Nevertheless, such mechanisms could be considered suboptimal from a policy perspective, as they switch off the operation of the systems when it is most needed in times of stress, giving rise to operational vulnerabilities.
In the UK, for example, firm are expected to have manual and automated controls that stop trading or prevent user access, and with manual intervention required to restart trading (referred to as ‘kill-switch’ controls) (Bank of England, 2018[15]). A firm, at a minimum, is expected to: (a) have a governance process around the use of kill-switch controls; (b) detail the action to be taken in respect of outstanding and placed orders when kill-switch controls are activated; and (c) periodically assess kill-switch controls to ensure that they operate as intended. This includes an assessment of the speed at which the procedure can be affected (Bank of England, 2018[15]).Safeguards are also built in pre-trading risk management systems, aiming to prevent and stop potential misuse of AI-based systems. Defences could also be applied at the exchange level where the trading is executed, and could include automatic cancellation of orders when the AI system is switched off for some reason and methods that provide resistance to sophisticated manipulation enabled by technology. Circuit breakers, currently triggered by massive drops between trades, could perhaps be adjusted to also identify and be triggered by large numbers of smaller trades performed by AI-driven systems, with the same effect.
What is more, the deployment of AI by traders could amplify the interconnectedness of financial markets and institutions in unexpected ways, potentially increasing correlations and dependencies of previously unrelated variables (FSB, 2017[11]). The scaling up of the use of algorithms that generate uncorrelated profits or returns may generate correlation in unrelated variables if their use reaches a sufficiently important scale. It can also amplify network effects, such as unexpected changes in the scale and direction of market moves.
Potential consequences of the use of AI in trading are also observed in the competition field (see Chapter 4). Traders may intentionally add to the general lack of transparency and explainability in proprietary ML models so as to retain their competitive edge. This, in turn, can raise issues related to the supervision of ML models and algorithms. In addition, the use of algorithms in trading can also make collusive outcomes easier to sustain and more likely to be observed in digital markets (OECD, 2017[16]). AI-driven systems may exacerbate illegal practices aiming to manipulate the markets, such as ‘spoofing’6, by making it more difficult for supervisors to identify such practices if collusion among machines is in place.
Similar considerations apply to trading desks of central banks, which aim to provide temporary market liquidity in times of market stress or to provide insurance against temporary deviations from an explicit target. As outliers could move the market into states with significant systematic risk or even systemic risk, a certain level of human intervention in AI-based automated systems could be necessary in order to manage such risks and introduce adequate safeguards.
2.2.3. Credit intermediation and assessment of creditworthiness
Copy link to 2.2.3. Credit intermediation and assessment of creditworthinessAI is being used by banks and fintech lenders in a variety of back-office and client-facing use-cases. Chat-bots powered by AI are deployed in client on-boarding and customer service, AI techniques are used for KYC, AML/CFT checks, ML models help recognise abnormal transactions and identify suspicious and/or fraudulent activity, while AI is also used for risk management purposes. When it comes to credit risk management of loan portfolios, ML models used to predict corporate defaults have been shown to produce superior results compared to standard statistical models (e.g. logic regressions) when limited information is available (Bank of Italy, 2019[17]). AI-based systems can also help analyse the degree of interconnectedness between borrowers, allowing for better risk management of lending portfolios.
The AI use case with the most transformational effect on credit intermediation is the assessment of creditworthiness of prospective borrowers for credit underwriting. Advanced AI-based analytics models can increase the speed and reduce the cost of underwriting through automation and associated efficiencies. More importantly, credit scoring models powered by big data and AI allow for the analysis of creditworthiness of clients with limited credit history or insufficient collateral, referred to as ‘thin-files’ through a combination of conventional credit information with big data not intuitively related to creditworthiness (e.g. social media data, digital footprints, and transactional data accessible through Open Banking initiatives).
The use of AI and big data has the potential to promote greater financial inclusion by enabling the extension of credit to unbanked parts of the population or to underbanked clients, such as near-prime customers or SMEs. This is particularly important for those SMEs that are viable but unable to provide historical performance data or pledge tangible collateral and who have historically faced financing gaps in some economies. Ultimately, the use of AI could support the growth of the real economy by alleviating financing constraints to SMEs. Nevertheless, it should be noted that AI-based credit scoring models remain untested over longer credit cycles or in case of a market downturn.
Risks of bias and disparate impact in credit outcomes
Copy link to Risks of bias and disparate impact in credit outcomesThe use of ML models and big data for credit underwriting raises risks of disparate impact in credit outcomes and the potential for discriminatory or unfair lending (US Treasury, 2016[18]).7 Biased, unfair or discriminatory lending decisions can stem from the inadequate or inappropriate use of data or the use of poor quality or unsuitable data, as well as the lack of transparency or explainability of AI-based models. Similar to all models using data, the risk of ‘garbage in, garbage out’ exists in ML-based models for risk scoring. Inadequate data may include poorly labelled or inaccurate data, data that reflects underlying human prejudices, or incomplete data (S&P, 2019[19]). A neutral machine learning model that is trained with inadequate data, risks producing inaccurate results even when fed with ‘good’ data. Equally, a neural network8 trained on high-quality data, which is fed inadequate data, will produce a questionable output, despite the well-trained underlying algorithm.
The difficulty in comprehending, following or replicating the decision-making process, referred to as lack of explainability, raises important challenges in lending, while making it harder to detect inappropriate use of data or the use of unsuitable data by the model. Such lack of transparency is particularly pertinent in lending decisions, as lenders are accountable for their decisions and must be able to explain the basis for denials of credit extension. The lack of explainability also means that lenders have limited ability to explain how a credit decision has been made, while consumers have little chance to understand what steps they should take to improve their credit rating or seek redress for potential discrimination. Importantly, the lack of explainability makes discrimination in credit allocation even harder to find (Brookings, 2020[20]).
Biased or discriminatory outcomes of AI credit rating models can be unintentional: well-intentioned but poorly designed and controlled models can inadvertently generate biased conclusions, discriminate against protected classes of people (e.g. based on race, sex, religion) or reinforce existing biases. Algorithms may combine facially neutral data points and treat them as proxies for immutable characteristics such as race or gender, thereby circumventing existing non-discrimination laws (Hurley, 2017[21]). For example, while a credit officer may be diligent not to include gender-based variants as input to the model, the model can infer the gender based on transaction activity, and use such knowledge in the assessment of creditworthiness. Biases may also be inherent in the data used as variables and, given that the model trains itself on such data, it may perpetuate historical biases incorporated in the data used to train it.
Safeguarding mechanisms to mitigate risks of disparate treatment and bias
Copy link to Safeguarding mechanisms to mitigate risks of disparate treatment and biasDeveloped economies have regulations in place to ensure that specific types of data are not being used in the credit risk analysis (e.g. US regulation around race data or zip code data, protected category data in the United Kingdom). Regulation promoting anti-discrimination principles, such as the US fair lending laws, exists in many jurisdictions, and regulators are globally considering the risk of potential bias and discrimination risk that AI/ML and algorithms can pose (White & Case, 2017[22]).
In some jurisdictions, comparative evidence of disparate treatment, such as lower average credit limits for members of protected groups than for members of other groups, is considered discrimination regardless of whether there was intent to discriminate. Potential mitigants against such risks are the existence of auditing mechanisms that sense check the results of the model against baseline datasets; testing of such scoring systems to ensure their fairness and accuracy (Citron and Pasquale, 2014[23]); disclosure to the customer and opt-in procedures; and governance frameworks for AI-enabled products and services and assignment of accountability to the human parameter of the project, to name a few (see Section 1.4).
Box 2.2. AI and Big Data in financial services provided by BigTech in certain jurisdictions
Copy link to Box 2.2. AI and Big Data in financial services provided by BigTech in certain jurisdictionsThe use of AI by BigTech is amplifying the use of massive datasets of customer information that is already leveraged by such firms to provide tailored financial services, and intensifies ensuing risks particularly in certain jurisdictions where BigTech is very active in financial service provision (e.g. China). Such risks are associated with data privacy considerations and concerns around the collection, storage and use of personal data for commercial gain and which could disadvantage customers through discriminatory practices related to credit (or other services) availability and pricing. Financial consumers risk receiving discriminatory product offering, pricing or advice, while the lack of explainability of AI-based techniques makes it increasingly difficult for supervisors to access and audit the activities provided by such firms.
AI techniques could further strengthen the ability of BigTech to provide novel and customised services, reinforcing their competitive advantage over traditional financial services firms and potentially allowing BigTech to dominate in certain parts of the market. The data advantage of BigTech could in theory allow them to build monopolistic positions, both in relation to client acquisition (for example through effective price discrimination) and through the introduction of high barriers to entry for smaller players.
Excessive market concentration and the dependence of the market on a few large firms could have possible systemic implications depending on their scale and scope (FSB, 2017[11]). A related risk of potential anti-competitive behaviours and market concentration is associated with the technological aspect of the service provision by BigTech (e.g. cloud computing service providers) and the possible emergence of a small number of key players in markets for AI solutions and/or services incorporating AI technologies, evidence of which is already observed in some parts of the world (ACPR, 2018[13]).
At the end of 2020, the European Union and the UK published regulatory proposals, the Digital Markets Act, that seek to establish an ex ante framework to govern ‘Gatekeeper’ digital platforms such as BigTech, aiming to mitigate some of the above risks and ensure fair and open digital markets (European Commission, 2020[24]). Some of the obligations proposed include the requirement for such Gatekeepers to provide business users with access to the data generated by their activities and provide data portability, while prohibiting them from using data obtained from business users to compete with these business users (to address dual role risks). The proposal also provides for solutions addressing self-preferencing, parity and ranking requirements to ensure no favourable treatment to the services offered by the Gatekeeper itself against those of third parties.
2.2.4. AI in blockchain9-based financial services
Copy link to 2.2.4. AI in blockchain<a id="back-endnotea2z10" href="/content/oecd/en/publications/2021/09/oecd-business-and-finance-outlook-2021_377c2c18/full-report/component-6.html#endnotea2z10" style="vertical-align: top;font-size: 0.8em;">9</a>-based financial servicesDistributed ledger technologies (DLT) are increasingly being used in finance, supported by their purported benefits of speed, efficiency and transparency, driven by automation and disintermediation (OECD, 2020[25]). Major applications of DLTs in financial services include issuance and post-trade/clearing and settlement of securities; payments; central bank digital currencies and fiat-backed stablecoins; and the tokenisation of assets more broadly. Merging AI models, criticised for their opaque and ‘black box’ nature, with blockchain technologies, known for their transparency, sounds counter-intuitive in the first instance.
Although a convergence of AI and DLTs in blockchain-based finance is promoted by the industry as a way to yield better results in such systems, this is not observed in practice at this stage. Increased automation amplifies efficiencies claimed by DLT-based systems, however, the actual level of AI implementation in DLT-based projects does not appear to be sufficiently large at this stage to justify claims of convergence between the two technologies. Instead, what is currently observed is the use of specific AI applications in blockchain-based systems (e.g. for the curation of data to the blockchain) or the use of DLT systems for the purposes of AI models (e.g. for data storage and sharing).
DLT solutions are used for the data management aspect of AI techniques, benefiting from the immutable and trust-less characteristics of the blockchain, while also allowing for the sharing of confidential information on a zero-knowledge basis without breaching confidentiality and privacy requirements. In the future, the use of DLTs in AI mechanisms is expected to allow users of such systems to monetise their data used by AI-driven systems through the use of Internet of Things (IoT) applications, for instance.
The implementation of AI applications in blockchain systems is currently concentrated in use-cases related to risk management, detection of fraud and compliance processes, including through the introduction of automated restrictions to a network. AI can be used to reduce (but not eliminate) security susceptibilities and help protect against compromising of the network, for example in payment applications, by identifying irregular activities for instance.. Similarly, AI applications can improve on-boarding processes on a network (e.g. biometrics for AI identification), as well as AML/CFT checks in the provision of any kind of DLT-based financial services. AI applications can also provide wallet-address analysis results that can be used for regulatory compliance purposes or for an internal risk-based assessment of transaction parties (Ziqi Chen et al., 2020[26]).
AI could also be used to improve the functioning of third party off-chain nodes, such as so-called ‘Oracles’10, nodes feeding external data into the network. The use of Oracles in DLT networks carries the risk of erroneous or inadequate data feeds into the network by underperforming or malicious third-party off-chain nodes (OECD, 2020[25]). As the responsibility of data curation shifts from third party nodes to independent, automated AI-powered systems that are more difficult to manipulate, the robustness of information recording and sharing could be strengthened. In a hypothetical scenario, the use of AI could further increase disintermediation by bringing AI inference directly on-chain, which would render Oracles redundant. In theory, it could act as a safeguard by testing the veracity of the data provided by the Oracles and prevent Oracle manipulation. Nevertheless, the introduction of AI in DLT-based networks does not necessarily resolve the ‘garbage in, garbage out’ conundrum as the problem of poor quality or inadequate data inputs is a challenge observed equally in AI-based applications.
Using AI to augment the capabilities of smart contracts
Copy link to Using AI to augment the capabilities of smart contractsThe largest potential of AI in DLT-based finance lies in its use in smart contracts11, with practical implications around their governance and risk management and with numerous hypothetical (and yet untested) effects on roles and processes of DLT-based networks. Smart contracts rely on simple software code and have existed long before the advent of AI. Currently, most smart contracts used in a material way do not have ties to AI techniques. As such, many of the suggested benefits from the use of AI in DLT systems remains theoretical, and industry claims around convergence of AI and DLTs functionalities in marketed products should be treated with caution.
That said, some AI use-cases are proving helpful in augmenting smart contract capabilities, particularly when it comes to risk management and the identification of flaws in the code of the smart contract. AI techniques such as NLP12 are already being tested for use in the analysis of patterns in smart contract execution so as to detect fraudulent activity and enhance the security of the network. Importantly, AI can test the code in ways that human code reviewers cannot, both in terms of speed and in terms of level of detail. Given that code is the underlying basis of any smart contract, flawless coding is fundamental for the robustness of smart contracts.
Box 2.4. AI and decentralised finance (DeFi)
Copy link to Box 2.4. AI and decentralised finance (DeFi)Smart contracts are at the core of the decentralised finance (DeFi) market, which is based on a user-to-smart contract or smart-contract to smart-contract transaction model. User accounts in DeFi applications interact with smart contracts by submitting transactions that execute a function defined on the smart contract.
Smart contracts facilitate the disintermediation from which DLT-based networks can benefit, and are one of the major source of efficiencies that such networks claim to offer. They allow for the full automation of actions such as payments or transfer of assets upon triggering of certain conditions, which are pre-defined and registered in the code.
AI integration in blockchains could in theory support decentralised applications in the DeFi space through use-cases that could increase automation and efficiencies in the provision of certain financial services. Indicatively, the introduction of AI models can support the third-party private sector provision of customised recommendations across products and services; credit scoring based on users’ online data; investment advisory services and trading based on financial data; as well as other reinforcement learning1 applications on blockchain-based processes (Ziqi Chen et al., 2020[26]). Researchers suggest that, in the future, AI could also be integrated for forecasting and automating in ‘self-learned’ smart contracts, similar to models applying reinforcement learning AI techniques (Almasoud et al., 2020[27]). In other words, AI can be used to extract and process information of real-time systems and feed such information into smart contracts. As in other blockchain-based financial applications, the deployment of AI in DeFi augments the capabilities of the DLT use-case by providing additional functionalities; however, it is not expected to radically affect any of the business models involved in DeFi applications.
The use of AI to build fully autonomous chains would raise important challenges and risks to its users and the wider ecosystem. In such environments, AI contracts rather than humans execute decisions and operate the systems and there is no human intervention in the decision-making or operation of the system. In addition, the introduction of automated mechanisms that switch off the model instantaneously (such as kill switches) is very difficult in such networks, not least because of the decentralised nature of the network.
1. Reinforcement learning involves the learning of the algorithm through interaction and feedback. It is based on neural networks and may be applied to unstructured data like images or voice.
In theory, using AI in smart contracts could further enhance their automation, by increasing their autonomy and allowing the underlying code to be dynamically adjusted according to market conditions. The use of NLP could improve the analytical reach of smart contracts that are linked to traditional contracts, legislation and court decisions, going even further in analysing the intent of the parties involved (The Technolawgist, 2020[28]). It should be noted, however, that such applications of AI for smart contracts are purely theoretical at this stage and remain to be tested in real-life examples.
Operational challenges relating to compatibility and interoperability of conventional infrastructure with DLT-based one and AI technologies remain to be resolved for such applications to come to life. In particular, AI techniques such as deep learning require significant amounts of computational resources, which may pose an obstacle to performing well on the Blockchain (Hackernoon, 2020[29]). It has been argued that at this stage of development of the infrastructure, storing data off chain would be a better option for real time recommendation engines to prevent latency and reduce costs (Almasoud et al., 2020[27]). Challenges also exist with regards to the legal status of smart contracts, as these are still not considered to be legal contracts in most jurisdictions (OECD, 2020[25]). Until it is clarified whether contract law applies to smart contracts, enforceability and financial protection issues will persist.
Box 2.3. Innovation in infrastructure
Copy link to Box 2.3. Innovation in infrastructureThe provision of infrastructure systems and services like transportation, energy, water and waste management are at the heart of meeting significant challenges facing societies such as demographics, migration, urbanisation, water scarcity and climate change. Modernising existing infrastructure stock, while conceiving and building infrastructure to address these challenges and providing a basis for economic growth and development is essential to meet future needs.
The role of technology and innovation in achieving these policy objectives is an important topic for policy makers. For example, embracing new technologies that enable drastic reductions in greenhouse gas (GHG) emissions when building and operating infrastructure will be a crucial element to net zero emissions. This could be from the type of cement that is used to installation of energy efficient charging stations for electric vehicles. Governments, in cooperation with diverse stakeholders, could benefit from sharing good practices related to technology and innovation in infrastructure, while also setting supportive policy frameworks to harness the benefits while mitigating risks.
The G20 Riyadh Infratech Agenda, endorsed by Leaders in 2020, provides high-level policy guidance for national authorities and the international community to advance the adoption of new and existing technologies in infrastructure. This work highlights the important role technology can play in helping countries make well-informed decisions and achieve more efficient financial outlays, by mobilising private sector investment, by enhancing service delivery and by achieving environmental, social and economic benefits.
While infratech can include a number of technologies, AI and ML applications are of note, particularly as digital technologies become more integrated into structures, changing the nature of infrastructure from simple hard assets to dynamic information systems (G20 Saudi Arabia, 2020[30]). For example, AI can be a powerful tool to optimise windmill operations and safety, analyse traffic patterns in transportation, and improve operations in energy grids.
Source: (G20 Saudi Arabia, 2020[30]).
2.3. Emerging risks and challenges from the deployment of AI in finance
Copy link to 2.3. Emerging risks and challenges from the deployment of AI in financeAs the use of AI in finance grows in size and spectrum, a number of challenges and risks associated with such techniques are being identified and deserve further consideration by policy makers. This section examines some of these challenges, and touches upon potential risk mitigation tools. Challenges discussed relate to data management and use; risk of bias and discrimination; explainability; robustness and resilience of AI models; governance and accountability in AI systems; regulatory considerations; employment risks and skills.
2.3.1. Data management, privacy/confidentiality and concentration risks
Copy link to 2.3.1. Data management, privacy/confidentiality and concentration risksData is the cornerstone of any AI application, but the inappropriate use of data in AI-powered applications or the use of inadequate data introduces an important source of non-financial risk to firms using AI techniques. Such risk relates to the veracity of the data used; challenges around data privacy and confidentiality; fairness considerations and potential concentration and broader competition issues.
The quality of the data used by AI models is fundamental to their appropriate functioning, however, when it comes to big data, there is some uncertainty around of the level of truthfulness, or veracity, of big data (IBM, 2020[31]). Together with characteristics such as exhaustivity (how wide the scope is) and extensionality (how easy is it to add or change fields), veracity is key for the use of big data in finance, as it may prove difficult for users of AI-powered systems to assess whether the dataset used is complete and can be trusted. Correct labelling and structuring of big data is another pre-requisite for ML models to be able to successfully identify what a signal is, distinguish signal from noise and recognise patterns in data (S&P, 2019[19]). Different methods are being developed to reduce the existence of irrelevant features or ‘noise’ in datasets and improve ML model performance, such as the creation of artificial or ‘synthetic’ datasets generated and employed for the purposes of ML modelling. These can be extremely useful for model testing and validation purposes in case the existing datasets lack scale or diversity (see Section 1.3.4).
Synthetic datasets can also allow financial firms to secure non-disclosive computation to protect consumer privacy, another of the important challenges of data use in AI, by creating anonymous datasets that comply with privacy requirements. Traditional data anonymisation approaches do not provide rigorous privacy guarantees, as ML models have the power to make inferences in big datasets. The use of big data by AI-powered models could expand the universe of data that is considered sensitive, as such models can become highly proficient in identifying users individually (US Treasury, 2018[32]). Facial recognition technology or data around the customer profile can be used by the model to identify users or infer other characteristics, such as gender, when joined up with other information.
Data privacy can be safeguarded through the use of ‘notification and consent’ practices, which may not necessarily be the norm in ML models. For example, when observed data is not provided by the customer (e.g. geolocation data or credit card transaction data) notification and consent protections are difficult to implement. The same holds when it comes to tracking of online activity with advanced modes of tracking, or to data sharing by third party providers. In addition, to the extent that consumers are not necessarily educated on how their data is handled and where it is being used, their data may be used without their understanding and well informed consent (US Treasury, 2018[32]).
Additional concerns are raised around data connectivity and the economics of data used by ML models in finance. Given the critical importance of the ability to aggregate, store, process, and transmit data across borders for financial sector development, the importance of appropriate data governance safeguards and rules is becoming increasingly important (Hardoon, 2020[33]). At the same time, the economics of data use are being redefined: A small number of alternative dataset players have emerged, exploiting the surge in demand for datasets that inform AI techniques, with limited visibility and overseeing over their activity at this stage. Increased compliance costs of regulations aiming to protect consumers may further redefine the economics of the use of big data for financial market providers and, by consequence, their approach in the use of AI and big data.
Access to customer data by firms that fall outside the regulatory perimeter, such as BigTech, raises risks of concentrations and dependencies on a few large players. Unequal access to data and potential dominance in the sourcing of big data by few big BigTech in particular, could reduce the capacity of smaller players to compete in the market for AI-based products/services. The strength and nature of the competitive advantages created by advances in AI could potentially harm the operations of efficient and competitive markets if consumers’ ability to make informed decisions is constrained by high concentrations amongst market providers (US Treasury, 2018[32]).
Box 2.4. Financial Consumer Protection and AI: OECD Policy responses to protect and support financial consumers
Copy link to Box 2.4. Financial Consumer Protection and AI: OECD Policy responses to protect and support financial consumersThe OECD has undertaken significant work in the area of digitalisation to understand and address the benefits, risks and potential policy responses for protecting and supporting financial consumers. The OECD has done this via its leading global policy work on financial education and financial consumer protection.
Financial education
Copy link to Financial educationThe OECD and its International Network on Financial Education (OECD INFE) developed research and policy tools to empower consumers with respect to the increasing digitalisation of retail financial services, including the implications of a greater application of AI to financial services.
The G20/OECD INFE Policy Guidance on Digitalisation and Financial Literacy, developed by the OECD/INFE in the framework of Argentina’s G20 Presidency provides non-binding policy directions to policy makers and other relevant stakeholders and is aimed at identifying and promoting effective initiatives that enhance digital and financial literacy of consumers and entrepreneurs, supporting their evaluation and dissemination, and promoting a responsible and beneficial development of digitalisation.
The Policy Guidance supports the development of core competencies on digital financial literacy to build trust and promote a safe use of digital financial services, protect consumers from digital crime and misselling, and support those at risk of over-reliance on digital credit.
The Guidance takes into account the increasing use of algorithms in determining decisions about credit or insurance, and how this can extend provision but also lead to new forms of exclusion for sectors of the population, and identifies core competencies to empower consumers to counter new kinds of digital exclusion. These competencies include:
Awareness of the different types of financial products and services delivered through digital means for personal or business purposes, including their benefits and risks.
Knowledge of consumer rights and obligations in the digital world.
Encourage consumers to know where to check, when possible, that a digital financial service provider is authorised by the relevant national financial authorities.
Prompting consumers to appropriately manage their digital footprint to the extent possible, avoid engaging in risky behaviours involving their personal data, and understand the consequences of sharing or disclosing personal data.
It invites policy makers to foster behaviours that can protect consumers and entrepreneurs from any negative consequences of these developments, and to prompt them in particular to:
Appropriately manage their digital footprint to the extent possible and avoid engaging in risky behaviours involving their personal data, and understand the consequences of sharing personal identification numbers, account or personal information whether digitally or through other channels.
Assess the kind of information that is requested by (financial) service providers to decide whether it is relevant and understand how it may be stored and used.
Policy aimed at financial service providers would also benefit consumers, so the onus of financial literacy is not entirely on the consumer.
The G20 OECD INFE Policy Guidance has been complemented by specific work conducted by the OECD/INFE on personal data and financial literacy and on the implications of artificial intelligence and machine learning for retail consumers. This work led to the release in 2020 of the report Personal Data Use in Financial Services and the Role of Financial Education: A consumer-centric analysis. The report reviews the risks and benefits brought by the technological innovations that have increased the capacity to capture, store, combine and analyse customer data, presents consumer attitudes to data sharing, and suggests policy options to support consumer awareness with respect to personal data use.
It encourages financial education policy makers to cooperate with the authorities in charge of personal data protection frameworks and it identifies additional elements pertaining to personal data to complement the core competencies identified in the G20 OECD INFE Policy Guidance note. It notably calls on policy makers to increase awareness among consumers of the analytical possibilities of big data and of their rights over personal data, for them to take steps to manage digital footprints and protect their data online.
The report invites policy makers to take a targeted approach and address the needs of the least technologically-savvy, who are most at risk given their low familiarity with online transactions, and of the groups willing to share more personal information in exchange for personalised products and service, such as younger generations.
Financial consumer protection
Copy link to Financial consumer protectionThe G20/OECD High Level Principles on Financial Consumer Protection (the Principles) are designed to assist G20, OECD and FSB jurisdictions as well as all other interested economies to enhance financial consumer protection. The Principles are administered by the G20/OECD Task Force on Financial Consumer Protection which has developed guidance for policy makers and oversight authorities to apply the Principles in the context of an increasingly digital environment.1
Key financial consumer protection policy responses relating to selected Principles
Copy link to Key financial consumer protection policy responses relating to selected PrinciplesPrinciple 2: Oversight Bodies
Copy link to Principle 2: Oversight BodiesTechnological developments present a range of challenges and opportunities for oversight bodies responsible for supervising and enforcing financial consumer protection laws. These include balancing the development of FinTech innovations while ensuring the appropriate level of consumer protection; and ensuring the adequacy of supervisory tools, resources and capabilities to oversee digital financial services. As set out in the G20/OECD Policy Guidance on Financial Consumer Protection Approaches in the Digital Age, oversight bodies can seek to address these challenges and opportunities in a number of ways, including:
Ensure that regulatory and supervisory resources, tools and methods are appropriate and adapted to the digital environment, which includes having access to data and exploring the use of technology to assist in market supervision.
Ensure they have adequate knowledge of the financial services market, including by engaging with businesses, industry representatives and consumers to understand new digital products and services and identify market trends and issues.
Ensure capability to deal effectively with technological innovation issues while ensuring appropriate consumer protections are maintained, for example, through regulatory sandboxes, innovation hubs, dedicated regulatory guidance or support for new entrants etc.
Principle 4: Disclosure & Transparency
Copy link to Principle 4: Disclosure & TransparencyNew types of disclosure challenges emerge in the context of digitalisation, associated with complex interfaces, limited space in digital devices or opaque terms, changes to consumer behaviour in an online or mobile setting, conditions and fees, especially regarding complex digital products. Set out in the G20/OECD Policy Guidance on Financial Consumer Protection Approaches in the Digital Age, to address these challenges, oversight bodies responsible for financial consumer protection can seek to:
Ensure that disclosure and transparency requirements are applicable and adequate to the provision of information through all channels relevant to digital financial services and covering all relevant stages of the product lifecycle.
Support consumer communications that are clear and simple to understand regardless of the channel of communication.
Embed an understanding of consumer decision-making and the impact of behavioural biases in the development of policies to ensure a customer-centric approach.
Encourage financial services providers to test digital disclosure approaches to ensure their effectiveness and recognise that there may be consumers in the target audience for the product or service who are not digitally literate.
Principle 7: Protection of Consumer Assets
Copy link to Principle 7: Protection of Consumer AssetsAI is underpinned by the explosion in recent times in the generation, collection, storage, sharing and use of personal and transactional data. Protection of consumer assets is a fundamental part of an overall financial consumer protection framework and includes covering fraudulent or unauthorised payments, segregation of consumer assets and procedures for protecting and recovering unclaimed assets. As outlined in the Financial Consumer Protection Policy Approaches in the Digital Age Protecting consumers' assets, data and privacy policy makers and oversight bodies responsible for financial consumer protection can seek to:
Ensure they have the necessary technological capacity and supervisory tools to mitigate digital security risks and react to such risks where the financial assets of a consumer are at risk.
Work collaboratively with industry, stakeholders, other regulatory and supervisory authorities and foreign counterparts to share information and understand emerging trends relating to digital financial risks.
Ensure that financial services providers are required to continuously assess the digital security risk to the services they provide and adopt appropriate security measures to reduce the risks.
Principle 8: Protection of Consumer Data & Privacy
Copy link to Principle 8: Protection of Consumer Data & PrivacyConsumers’ financial and personal information should be protected through appropriate control and protection mechanisms. These mechanisms should define the purposes for which the data may be collected, processed, held, used and disclosed (especially to third parties). Also outlined in the Financial Consumer Protection Policy Approaches in the Digital Age Protecting consumers' assets, data and privacy, policy makers and oversight bodies responsible for financial consumer protection should:
Ensure that the legal, regulatory and supervisory framework for financial consumer protection has appropriate safeguards and measures relating to the protection of consumer data and privacy, including a definition of “personal data”.
Liaise with data protection authorities to ensure understanding and application of data protection laws and regulations to financial services providers.
Ensure financial services providers have robust and transparent governance, accountability, risk management and control systems relating to use of digital capabilities (particularly AI, algorithms and machine learning technology).
1. The Task Force is currently conducting a strategic Review of the Principles to identify new or emerging developments in financial consumer protection policies or approaches over the last 10 years that may warrant updates to the Principles to ensure they are fully up to date. The Review will include considering digital developments and their impacts on the provision of financial services to consumers.
2.3.2. Algorithmic bias and discrimination in AI
Copy link to 2.3.2. Algorithmic bias and discrimination in AIDepending on how they are used, AI algorithms have the potential to help avoid discrimination based on human interactions, or intensify biases, unfair treatment and discrimination in financial services. The risk of unintended bias and discrimination of parts of the population is very much linked to the misuse of data and to the use of inappropriate data by ML model (e.g. in credit underwriting, see Section 1.2.3). AI applications can potentially compound existing biases found in the data; models trained with biased data will perpetuate biases; and the identification of spurious correlations may add another layer of such risk of unfair treatment (US Treasury, 2018[32]). Biased or discriminatory outcomes of ML models are not necessarily intentional and can even occur with strong quality, well-labelled data, through inference and proxies, or given the fact that correlations between sensitive and ‘non-sensitive’ variables may be difficult to detect in vast databases (Goodman and Flaxman, 2016[34]).
Careful design, diligent auditing and testing of ML models can further assist in avoiding potential biases. Inadequately designed and controlled AI/ML models carry a risk of exacerbating or reinforcing existing biases while at the same time making discrimination even harder to observe (Klein, 2020[35]). Auditing mechanisms of the model and the algorithm that sense check the results of the model against baseline datasets can help ensure that there is no unfair treatment or discrimination by the technology. Ideally, users and supervisors should be able to test scoring systems to ensure their fairness and accuracy (Citron and Pasquale, 2014[23]). Tests can also be run based on whether protected classes can be inferred from other attributes in the data, and a number of techniques can be applied to identify and/or rectify discrimination in ML models (Feldman et al., 2015[36]).
The human parameter is critical both at the data input stage and at the query input stage and a degree of scepticism in the evaluation of the model results can be critical in minimising the risks of biased model decision-making. Human intervention is necessary so as to identify and correct for biases built into the data or in the model design, and to explain the output of the model, although the extent to which all this is feasible remains an open question, particularly given the lack of interpretability or explainability of advanced ML models. Human judgement is also important so as to avoid interpreting meaningless correlations observed from patterns as causal relationships, resulting in false or biased decision-making.
2.3.3. The explainability conundrum
Copy link to 2.3.3. The explainability conundrumThe difficulty in decomposing the output of a ML model into the underlying drivers of its decision, referred to as explainability, is the most pressing challenge in AI-based models used in finance. In addition to the inherent complexity of AI-based models, market participants may intentionally conceal the mechanics of their AI models to protect their intellectual property, further obscuring the techniques. The gap in technical literacy of most end-user consumers, coupled with the mismatch between the complexity characterising AI models and the demands of human-scale reasoning further aggravates the problem (Burrell, 2016[37]).
In the most advanced AI techniques, even if the underlying mathematical principles of such models can be explained, they still lack ‘explicit declarative knowledge’ (Holzinger, 2018[38]). This makes them incompatible with existing regulation that may require algorithms to be fully understood and explainable throughout their lifecycle (IOSCO, 2020[39]). Similarly, the lack of explainability is incompatible with regulations granting citizens a ‘right to explanation’ for decisions made by algorithms and information on the logic involved, such as the EU’s General Data Protection Regulation (GDPR)13 applied in credit decisions or insurance pricing, for instance. Another example is the potential use of ML in the calculation of regulatory requirements (e.g. risk-weighted assets (RWA) for credit risk), where the existing rules require that the model be explainable or at least subject to human oversight and judgement (e.g. Basel Framework for Calculation of RWA for credit risk – Use of models 36.33).
Lack of interpretability of AI and ML algorithms could become a macro-level risk if not appropriately supervised by micro prudential supervisors, as it becomes difficult for both firms and supervisors to predict how models will affect markets (FSB, 2017[11]). In the absence of an understanding of the detailed mechanics underlying a model, users have limited room to predict how their models affect market conditions, and whether they contribute to market shocks. Users are also unable to adjust their strategies in time of poor performance or in times of stress, leading to potential episodes of exacerbated market volatility and bouts of illiquidity during periods of acute stress, aggravating flash crash type of events (see Section 1.2.2). Risks of market manipulation or tacit collusions are also present in non-explainable AI models.
Interestingly, AI applications risk being held to a higher standard and thus subjected to a more onerous explainability requirement as compared to other technologies or complex mathematical models in finance, with negative repercussions for innovation (Hardoon, 2020[33]). The objective of the explainability analysis at committee level should focus on the underlying risks that the model might be exposing the firm to, and whether these are manageable, instead of its underlying mathematical promise. A minimum level of explainability would still need to be ensured for a model committee to be able to analyse the model brought to the committee and be comfortable with its deployment.
Given the trade-off between explainability and performance of the model, financial services providers need to strike the right balance between explainability of the model and accuracy/performance. It should also be highlighted that there is no need for a single principle or one-size-fits-all approach for explaining ML models, and explainability will depend to a large extent on the context (Brainard, 2020[40]) (Hardoon, 2020[33]). Importantly, ensuring the explainability of the model does not by itself guarantee that the model is reliable (Brainard, 2020[40]). Contextual alignment of explainability with the audience needs to be coupled with a shift of the focus towards ‘explainability of the risk’, i.e. understanding the resulting risk exposure from the use of the model instead of the methodology underlying such model. Recent guidance issued by the UK Information Commissioner’s Office suggests using five contextual factors to help in assessing the type of explanation needed: domain, impact, data used, urgency, and audience (UK Information Commissioner’s Office, 2020[41]).
Improving the explainability levels of AI applications can contribute to maintaining the level of trust by financial consumers and regulators/supervisors, particularly in critical financial services (FSB, 2017[11]). Research suggests that explainability that is ‘human-meaningful’ can significantly affect the users’ perception of a system’s accuracy, independent of the actual accuracy observed (Nourani et al., 2020[42]). When less human-meaningful explanations are provided, the accuracy of the technique that does not operate on human-understandable rationale is less likely to be accurately judged by the users.
Auditability and disclosure of AI techniques used by financial service providers
Copy link to Auditability and disclosure of AI techniques used by financial service providersThe opacity of algorithm-based systems could be addressed through transparency requirements, ensuring that clear information is provided as to the AI system’s capabilities and limitations (European Commission, 2020[43]). Separate disclosure should inform consumers about the use of AI system in the delivery of a product and their interaction with an AI system instead of a human being (e.g. robo-advisors), to allow customers to make conscious choices among competing products. Suitability requirements, such as the ones applicable to the sale of investment products, might help firms better assess whether the prospective clients have a solid understanding of how the use of AI affects the delivery of the product/service. To date, there is no commonly accepted practice as to the level of disclosure that should be provided to investors and financial consumers and potential proportionality in such information.
In the absence of explainability about the model workings, financial service providers find it hard to document the model process of AI-enabled models used for supervisory purposes (Bank of England and FCA, 2020[44]). Some jurisdictions have proposed a two-pronged approach to AI model supervision: (i) analytical: combining analysis of the source code and of the data with methods (if possible based on standards) for documenting AI algorithms, predictive models and datasets; and (ii) empirical: leveraging methods providing explanations for an individual decision or for the overall algorithm’s behaviour, and relying on two techniques for testing an algorithm as a black box: challenger models (to compare against the model under test) and benchmarking datasets, both curated by the auditor (ACPR, 2020[45]).
Documentation of the logic behind the algorithm, to the extent feasible, is being used by some regulators as a way to ensure that the outcomes produced by the model are explainable, traceable and repeatable (FSRA, 2019[46]). The EU, for instance, is considering requirements around disclosure documentation of programming and training methodologies, processes and techniques used to build, test, and validate AI systems, including documentation on the algorithm (what the model shall optimise for, which weights are designed to certain parameters at the outset etc.) (European Commission, 2020[43]). The US Public Policy Council of the Association for Computing Machinery (USACM) has proposed a set of principles targeting inter alia transparency and auditability in the use of algorithms, suggesting that models, data, algos and decisions be recorded so as to be available for audit where harm is suspected (ACM US Public Policy Council, 2017[47]). The Federal Reserve’s guidance for model risk management includes also documentation of model development and validation that is sufficiently detailed to allow parties unfamiliar with a model to understand how the model operates, its limitations and key assumptions (Federal Reserve, 2011[48]).
2.3.4. Training, validation and testing of AI models to promote their robustness and resilience
Copy link to 2.3.4. Training, validation and testing of AI models to promote their robustness and resilienceAppropriate training of ML models is fundamental for their performance, and the datasets used for that purpose need to be large enough to capture non-linear relationships and tail events in the data. This, however, is hard to achieve in practice, given that tail events are rare and the dataset may not be robust enough for optimal outcomes. The inability of the industry to train models on datasets that include tail events is creating a significant vulnerability for the financial system, weakening the reliability of such models in times of unpredicted crisis and rendering AI a tool that can be used only when market conditions are stable.
The validation of ML models using different datasets than the ones used to train the model, helps assess the accuracy of the model, optimise its parameters, and mitigate the risk of over-fitting. The latter occurs when a trained model performs extremely well on the samples used for training but performs poorly on new unknown samples, i.e. the model does not generalise well (Xu and Goodacre, 2018[49]). Validation sets contain samples with known provenance, but these classifications are not known to the model, therefore, predictions on the validation set allow the operator to assess model accuracy. Based on the errors on the validation set, the optimal model parameters set is determined using the one with the lowest validation error (Xu and Goodacre, 2018[49]). Validation processes go beyond the simple back testing of a model using historical data to examine ex-post its predictive capabilities, and ensure that the model’s outcomes are reproducible.
Synthetic datasets and alternative data are being artificially generated to serve as test sets for validation, used to confirm that the model is being used and performs as intended. Synthetic databases provide an interesting alternative given that they can provide inexhaustible amounts of simulated data, and a potentially cheaper way of improving the predictive power and enhancing the robustness of ML models, especially where real data is scarce and expensive. Some regulators require, in some instances, the evaluation of the results produced by AI models in test scenarios set by the supervisory authorities (e.g. Germany) (IOSCO, 2020[39]).
Box 2.5. AI and tail risk: learnings from the COVID-19 pandemic
Copy link to Box 2.5. AI and tail risk: learnings from the COVID-19 pandemicIn spite of the dynamic nature of AI models and their evolution through learning from new data, they may not be able to perform under idiosyncratic one-time events not reflected in the data used to train the model, such as the COVID-19 pandemic. Evidence based on a survey conducted in UK banks suggest that around 35% of banks experienced a negative impact on ML model performance during the pandemic (Bholat, Gharbawi and Thew, 2020[50]). This is likely because the pandemic has created major movements in macroeconomic variables, such as rising unemployment and mortgage forbearance, which required ML (as well as traditional) models to be recalibrated.
Tail and unforeseen events, such as the recent pandemic, give rise to discontinuity in the datasets, which in turn creates model drift that undermine the models’ predictive capacity. Tail events cause unexpected changes in the behaviour of the target variable that the model is looking to predict, and previously undocumented changes to the data structure and underlying patterns of the dataset used by the model, both caused by a shift in market dynamics during such events. These are naturally not captured by the initial dataset on which the model was trained and are likely to result in performance degradation.
Synthetic datasets generated to train the models could going forward incorporate tail events of the same nature, in addition to data from the COVID-19 period, with a view to retrain and redeploy redundant models. Ongoing testing of models with (synthetic) validation datasets that incorporate extreme scenarios and continuous monitoring for model drifts is therefore of paramount importance to mitigate risks encountered in times of stress.
Ongoing monitoring and validation of models throughout their life is foundational for the appropriate risk management of any type of model (Federal Reserve, 2011[48]) and is the most effective way to identify and address ‘model drift’. Model drift comes in the form of concept drifts or data drifts: Concept drifts describe situations where the statistical properties of the target variable studied by the model change, which changes the very concept of what the model is trying to predict (Widmer, 1996[51]). For example, the definition of fraud or the way it shows up in the data could evolve over time with new ways of conducting illegal activity, such a change would result in concept drift. Data drifts occur when statistical properties of the input data change, affecting the model’s predictive power. The major shift of consumer attitudes and preferences towards e-commerce and digital banking is a good example of such data drifts not captured by the initial dataset on which the model was trained and result in performance degradation.
2.3.5. Governance of AI systems and accountability
Copy link to 2.3.5. Governance of AI systems and accountabilitySolid governance arrangements and clear accountability mechanisms are indispensable, particularly as AI models are increasingly deployed in high-value decision-making use-cases (e.g. credit allocation). Organisations and individuals developing, deploying or operating AI systems should be held accountable for their proper functioning (OECD, 2019[52]). Importantly, intended outcomes for consumers would need to be incorporated in any governance framework, together with an assessment of whether and how such outcomes are reached using AI technologies.
In advanced deep learning models, issues may arise concerning the ultimate control of the model, as AI could unintentionally behave in a way that is contrary to consumer interests (e.g. biased results in credit underwriting). In addition, the autonomous behaviour of some AI systems during their life cycle may entail important product changes having an impact on safety, which may require a new risk assessment (European Commission, 2020[43]). Human oversight from the product design and throughout the lifecycle of the AI products and systems may be needed as a safeguard (European Commission, 2020[43]).
Currently, financial market participants rely on existing governance and oversight arrangements for the use of AI techniques, as AI-based algorithms are not considered to be fundamentally different from conventional ones (IOSCO, 2020[39]). Model governance best practices have been adopted by financial firms since the emergence of traditional statistical models for credit and other consumer finance decisions. In accordance with such best practices, financial service providers must ensure that models are built using appropriate datasets; that certain data is not used in the models; that data that is a proxy for a protected class is not used; that models are rigorously tested and validated (sometimes by independent validators); and that when models are used in production, the production input data is consistent with the data used to build the model. Documentation and audit trails are also held around deployment decisions, design, and production processes.
The increasing use of complex AI-based techniques and ML models will warrant the adjustment, and possible upgrade, of existing governance and oversight arrangements to accommodate for the complexities of AI techniques. Explicit governance frameworks that designate clear lines of responsibility for the development and overseeing of AI-based systems throughout their lifecycle, from development to deployment, will further strengthen existing arrangements for operations related to AI. Internal governance frameworks could include minimum standards or best practice guidelines and approaches for the implementation of such guidelines (Bank of England and FCA, 2020[44]).
Currently existing model governance frameworks have yet to address how to handle AI models in finance, which exist only ephemerally, and change very frequently, although the need for such remains debatable in some jurisdictions. Model governance frameworks should provide that models must be monitored to ensure they do not produce results that constitute comparative evidence of disparate treatment. However, there are challenges to testing that premise: since many ML models are non-deterministic, there is no guarantee that even with the same input data the same model will be produced.
Box 2.6. Governance considerations when outsourcing and third party providers are involved
Copy link to Box 2.6. Governance considerations when outsourcing and third party providers are involvedPossible risks of concentration of certain third-party providers may rise in terms of data collection and management (e.g. dataset providers) or in the area of technology (e.g. third party model providers) and infrastructure (e.g. cloud providers) provision. AI models and techniques are being commoditised through cloud adoption, and the risk of dependency on providers of outsourced solutions raises new challenges for competitive dynamics and potential oligopolistic market structures in such services.
In addition to concentration and dependency risks, the outsourcing of AI techniques or enabling technologies and infrastructure raises challenges in terms of accountability. Governance arrangements and contractual modalities are important in managing risks related to outsourcing, similar to those applying in any other type of services. Finance providers need to have the skills necessary to audit and perform due diligence over the services provided by third parties. Over-reliance on outsourcing may also give rise to increased risk of disruption of service with potential systemic impact in the markets. Similar to other types of models, contingency and security plans need to be in place, as needed (in particular related to whether the model is critical or not), to allow business to function as usual if any vulnerability materialises.
The ease of use of standardised, off-the-shelf AI tools may encourage non-regulated entities to provide investment advisory or other services without proper certification/licensing in a non-compliant way. Such regulatory arbitrage is also happening with mainly BigTech entities making use of datasets they have access to from their primary activity.
2.3.6. Other sources of risks in AI use-cases in finance: regulatory considerations, employment and skills
Copy link to 2.3.6. Other sources of risks in AI use-cases in finance: regulatory considerations, employment and skillsAlthough many countries have dedicated AI strategies (OECD, 2019[52]), a very small number of jurisdictions have current requirements that are specifically targeting AI-based algorithms and models. In most cases, regulation and supervision of ML applications are based on overarching requirements for systems and controls (IOSCO, 2020[39]). These consist primarily of rigorous testing of the algorithms used before they are deployed in the market, and continuous monitoring of their performance throughout their lifecycle.
The technology-neutral approach that is being applied by most jurisdictions to regulate financial market products (in relation to risk management, governance, and controls over the use of algorithms) may be challenged by the rising complexity of some innovative use-cases in finance. Given the depth of technological advances in AI areas such as deep learning, existing financial sector regulatory regimes could fall short in addressing the systemic risks posed by a potential broad adoption of such techniques in finance (Gensler and Bailey, 2020[53]). The complex nature of AI could give rise to potential incompatibilities with existing financial rules and regulations (e.g. due to the lack of explainability, see Section 1.3.3).14
Industry participants note a potential risk of fragmentation of the regulatory landscape with respect to AI at the national, international and sectoral level, and the need for more consistency to ensure that these techniques can function across borders (Bank of England and FCA, 2020[44]). In addition to existing regulation that is applicable to AI models and systems, a multitude of published AI principles, guidance, and best practice have been developed in recent years although views differ over their practical value and the difficulty of translating such principles into effective practical guidance (e.g. through real life examples) (Bank of England and FCA, 2020[44]).
Employment and skills
Copy link to Employment and skillsThe widespread adoption of AI and ML by the financial industry may give rise to some employment challenges and needs to upgrade skills, both for market participants and for policy makers alike. Demand for employees with applicable skills in AI methods, advanced mathematics, software engineering and data science is rising, while the application of such technologies may result in potentially significant job losses across the industry (Noonan, 1998[54]) (US Treasury, 2018[32]). Such loss of jobs replaced by machines may result in an over-reliance in fully automated AI systems, which could, in turn, lead to increased risk of disruption of service with potential systemic impact in the markets. If markets dependent on such systems face technical or other disruptions, financial service providers need to ensure that from a human resources perspective, they are ready to substitute the automated AI systems with well-trained humans acting as a human safety net and capable of ensuring there is no disruption in the markets.
Skills and technical expertise becomes increasingly important for regulators and supervisors who need to keep pace with the technology and enhance the skills necessary to effectively supervise AI-based applications in finance. Enforcement authorities need to be technically capable of inspecting AI-based systems and empowered to intervene when required (European Commission, 2020[43]). The upskilling of policy makers will also allow them to expand their own use of AI in RegTech and SupTech, an important area of application of innovation in the official sector (see Chapter 5).
AI in finance should be seen as a technology that augments human capabilities instead of replacing them. It could be argued that a combination of ‘man and machine’, where AI informs human judgment rather than replaces it (decision aid instead of decision maker), could allow for the benefits of the technology to materialise, while maintaining safeguards of accountability and control as to the ultimate decision-making. At the current stage of maturity of AI solutions, and to ensure that vulnerabilities and risks arising from the use of AI-driven techniques are minimised, some level of human supervision of AI-techniques is still necessary. The identification of converging points, where human and AI are integrated, will be critical for the practical implementation of such a combined ‘man and machine’ approach (‘human in the loop’).
2.4. Policy considerations
Copy link to 2.4. Policy considerationsAI use-cases in finance have potential to deliver significant benefits to financial consumers and market participants, by improving the quality of services offered and producing efficiencies to financial firms, reducing friction and transaction costs. At the same time, the deployment of AI in finance gives rise to new challenges, while it could also amplify pre-existing risks in financial markets (OECD, 2021[2]).
Policy makers and regulators have a role in ensuring that the use of AI in finance is consistent with promoting financial stability, protecting financial consumers, and promoting market integrity and competition. Emerging risks from the deployment of AI techniques need to be identified and mitigated to support and promote the use of responsible AI without stifling innovation. Existing regulatory and supervisory requirements may need to be clarified and sometimes adjusted to address some of the perceived incompatibilities of existing arrangements with AI applications.
One such source of potential incompatibility with existing laws and regulations is associated with the lack of explainability in AI, and more efforts are needed to overcome these both at the policy and industry levels. The difficulty in understanding how and why AI models produce their outputs and the ensuing inability of users to adjust their strategies in times of stress may lead to exacerbated market volatility and bouts of illiquidity during periods of market stress, and to flash crashes. Risks related to pro-cyclicality, convergence, and increased market volatility through simultaneous purchases and sales of large quantities can further amplify systemic risks. Overcoming or improving the explainability conundrum will help promote trust of users and supervisors around AI applications.
The application of regulatory and supervisory requirements on AI techniques could be looked at under a contextual and proportional framework, depending on the criticality of the application and the potential impact on the consumer involved (OECD, 2021[2]). In particular, policy makers may need to sharpen their existing arsenal of defences against risks emerging from, or exacerbated by, the use of AI, in a number of areas:
Sharpen the policy focus on better data governance by financial firms, aiming to reinforce consumer protection across AI use-cases in finance. Some of the most important risks raised in AI use-cases in finance relate to data management: data privacy, confidentiality, concentration of data and possible impact on the competitive dynamics of the market, but also risk of data drifts. The importance of data is undisputed when it comes to training, testing and validation of ML models, but also when defining their capacity to retain their predictive powers in tail events. Policy makers could consider the introduction of specific requirements or best practices for data management in AI-based techniques. These could touch upon data quality, adequacy of the datasets used depending on the intended use of the AI model, as well as tools to monitor and correct for conceptual drifts. When it comes to databases purchased by third party providers, additional vigilance may be required by financial firms and only databases approved for use and compliant with data governance requirements should be permitted. Requirements for additional transparency over the use of personal data and opt-out options for the use of personal data could also be considered by authorities.
Promote practices that will help overcome risk of unintended bias and discrimination. In addition to efforts around data quality, safeguards could be put in place to provide assurance about the robustness of the model when it comes to avoiding potential biases. Appropriate sense checking of model results against baseline datasets and other tests based on whether protected classes can be inferred from other attributes in the data are two examples of best practices to mitigate risks of discrimination. The validation of the appropriateness of variables used by the model could reduce a source of potential biases.
Consider disclosure requirements around the use of AI techniques in finance when these have an impact on the customer outcome. Financial consumers should be informed about the use of AI techniques in the delivery of a product, as well as potential interaction with an AI system instead of a human being, to be able to make conscious choices among competing products. Clear information around the AI system’s capabilities and limitations may need to be included in such disclosure. Suitability requirements for AI-driven financial services, similar to the ones applicable to the sale of investment products, could be considered by authorities for the sounder assessment of prospective clients’ understanding of the impact on AI in the delivery of the product. Policy makers might consider mandating that financial services providers use active disclosure (e.g. giving potential customers information and explanation directly, having a dedicated question line or FAQ) as opposed to simply passive disclosure to ensure maximum understanding by consumers.
Strengthen model governance and accountability mechanisms. Policy makers should consider requiring clear and explicit governance frameworks and attribution of accountability to the human element to help build trust in AI-driven systems. Designation of clear lines of responsibility for the development and overseeing of AI-based systems throughout their lifecycle, from development to deployment, may need to be put in place by financial services providers so as to strengthen existing arrangements for operations related to AI, particularly when third party providers and outsourcing are involved. Currently applicable frameworks for model governance may need to be adjusted for AI, and although audit trails of processes are helpful for model oversight, the supervisory focus could be shifted from documentation of the development process to model behaviour and outcomes. Supervisors may also wish to look into more technical ways of managing risk, such as adversarial model stress testing or outcome-based metrics (Gensler and Bailey, 2020[53]).
Consider requirements for firms to provide confidence around the robustness and resilience of AI models: The provision of increased assurance by financial firms around the robustness and resilience of AI models is fundamental as policy makers seek to guard against build-up of systemic risks, and will help AI applications in finance gain trust. The performance of models needs to be tested in extreme market conditions, to prevent systemic risks and vulnerabilities that may arise in times of stress. The introduction of automatic control mechanisms (such as kill switches) that trigger alerts or switch off models in times of stress could further assist in mitigating risks, although they expose the firm to new operational risks and could amplify market stress. Back-up plans, models and processes should be in place to ensure business continuity in case the models fails or acts in unexpected ways. Further, regulators could consider add-on or minimum buffers if banks were to determine risk weights or capital based on AI algorithms (Gensler and Bailey, 2020[53]). The importance of cybersecurity should also be considered for the generation of robust technological AI systems and the importance of cyber resilience for financial services.
Consider the introduction or reinforcement of frameworks for appropriate training, retraining and rigorous testing of AI models. Such processes help ensure that ML model-based decisioning is operating as intended and in compliance with applicable rules and regulations. Datasets used for training must be large enough to capture non-linear relationships and tail events in the data, even if synthetic, so as to improve model reliability in times of unprecedented crisis.
Promote the ongoing monitoring and validation of AI models as the most effective way to improve model resilience, prevent, and address model drifts. Best practices around standardised procedures for continuous monitoring and validation throughout the lifetime of a model could assist in improving model resilience, and identify whether the model necessitates adjustment, redevelopment, or replacement. Model validation processes may need to be separated from model development ones and documented as best possible for supervisory purposes. The frequency of testing and validation may need to be defined depending on the complexity of the model and the materiality of the decisions made by such model.
Place emphasis in human primacy in decision making for higher-value use-cases (e.g. lending). Appropriate emphasis could be placed on human primacy in decision making when it comes to higher-value use-cases (e.g. lending decisions) which have a significant impact on consumers. Authorities could consider the introduction of processes that can allow customers to challenge the outcome of AI models and seek redress, such as the ones introduced by GDPR (right of individuals ‘to obtain human intervention’ and to contest the decision made by an algorithm (EU, 2016[55])). Public communication by the official sector that clearly sets expectations could further build confidence in AI applications in finance.
Deploy resources to keep pace with advances in technology, investing in research and in the upscaling of skills for financial sector participants and policy makers alike. Given the increasing technical complexity of AI, investment in research could allow some of the issues around explainability and unintended consequences of AI techniques to be resolved. Investment in skills for both finance sector participants and policy makers would allow them to follow advancements in technology and maintain a multidisciplinary dialogue at operational, regulatory and supervisory level. Enforcement authorities in particular will need to be technically capable of inspecting AI-based systems and empowered to intervene when required, but also to enjoy the benefits of this technology by deploying AI in RegTech/SupTech applications.
Promote multidisciplinary dialogue between policy makers and the industry at national and international level, including whether the application of existing rules is sufficient to cater for emerging risks linked to the innovative nature of such technologies. Software engineers, data scientists, modelers, operational and front office executives from the industry as well as academics and supervisors need to engage in a continuous dialogue and exchange to promote a better understanding of the opportunities and limitations of AI’s use in finance. Given the ease of cross-border provision of financial services, dialogue between the different stakeholders involved should be fostered and maintained at domestic and global levels. There is a role for multilateral organisations in facilitating such dialogue and sharing best practices among countries.
Oversee financial industry use of AI so as to indirectly foster trust in AI: The role of policy makers is important in supporting innovation in the sector while ensuring that financial consumers and investors are duly protected and the markets around such products and services remain fair, orderly and transparent. Efforts to mitigate emerging risks could help instil trust and confidence and promote the adoption of such innovative techniques.
References
[47] ACM US Public Policy Council (2017), Principles for Algorithmic Transparency and Accountability, https://www.acm.org/binaries/content/assets/public-policy/2017_usacm_statement_algorithms.pdf.
[45] ACPR (2020), Governance of Artificial Intelligence in Finance, https://acpr.banque-france.fr/sites/default/files/medias/documents/20200612_ai_governance_finance.pdf.
[13] ACPR (2018), Artificial intelligence: challenges for the financial sector, https://acpr.banque-france.fr/sites/default/files/medias/documents/2018_12_20_intelligence_artificielle_en.pdf.
[27] Almasoud, A. et al. (2020), Toward a self-learned Smart Contracts, https://www.researchgate.net/publication/330009052_Toward_a_self-learned_Smart_Contracts.
[15] Bank of England (2018), “Algorithmic trading - Supervisory statement SS5/18”, https://www.bankofengland.co.uk/-/media/boe/files/prudential-regulation/supervisory-statement/2018/ss518.
[44] Bank of England and FCA (2020), Minutes: Artificial Intelligence Public-Private Forum-First meeting, https://www.bankofengland.co.uk/minutes/2020/artificial-intelligence-public-private-forum-minutes.
[17] Bank of Italy (2019), Corporate default forecasting with machine learning, https://www.bancaditalia.it/pubblicazioni/temi-discussione/2019/2019-1256/en_Tema_1256.pdf?language_id=1.
[5] BarclayHedge (2018), BarclayHedge Survey: Majority of Hedge Fund Pros Use AI/Machine Learning in Investment Strategies., https://www.barclayhedge.com/insider/barclayhedge-survey-majority-of-hedge-fund-pros-use-ai-machine-learning-in-investment-strategies.
[50] Bholat, D., M. Gharbawi and O. Thew (2020), The impact of Covid on machine learning and data science in UK banking | Bank of England, Bank of England Quarterly Bulletin Q4 2020, https://www.bankofengland.co.uk/quarterly-bulletin/2020/2020-q4/the-impact-of-covid-on-machine-learning-and-data-science-in-uk-banking.
[3] Blackrock (2019), Artificial intelligence and machine learning in asset management Background, https://www.blackrock.com/corporate/literature/whitepaper/viewpoint-artificial-intelligence-machine-learning-asset-management-october-2019.pdf.
[7] Bloomberg (2019), What’s an “Algo Wheel?” And why should you care? | Bloomberg Professional Services, https://www.bloomberg.com/professional/blog/whats-algo-wheel-care/.
[40] Brainard (2020), Speech by Governor Brainard on supporting responsible use of AI and equitable outcomes in financial services - Federal Reserve Board, https://www.federalreserve.gov/newsevents/speech/brainard20210112a.htm.
[20] Brookings (2020), Reducing bias in AI-based financial services, https://www.brookings.edu/research/reducing-bias-in-ai-based-financial-services/.
[37] Burrell, J. (2016), “How the machine ’thinks’: Understanding opacity in machine learning algorithms”, http://dx.doi.org/10.1177/2053951715622512.
[23] Citron, D. and F. Pasquale (2014), “The Scored Society: Due Process for Automated Predictions”, https://papers.ssrn.com/abstract=2376209.
[4] Deloitte (2019), Artificial intelligence The next frontier for investment management firms.
[55] EU (2016), EUR-Lex - 32016R0679 - EN - EUR-Lex, https://eur-lex.europa.eu/eli/reg/2016/679/oj.
[24] European Commission (2020), Digital Services Act, https://ec.europa.eu/digital-single-market/en/digital-services-act-package.
[43] European Commission (2020), On Artificial Intelligence - A European Approach To Excellence and Trust White Paper on Artificial Intelligence, https://ec.europa.eu/commission/sites/beta-political/files/political-guidelines-next-commission_en.pdf.
[48] Federal Reserve (2011), The Fed - Supervisory Letter SR 11-7 on guidance on Model Risk Management -- April 4, 2011, https://www.federalreserve.gov/supervisionreg/srletters/sr1107.htm.
[36] Feldman, M. et al. (2015), Certifying and removing disparate impact, Association for Computing Machinery, New York, NY, USA, http://dx.doi.org/10.1145/2783258.2783311.
[6] Financial Times (2020), Hedge funds: no market for small firms | Financial Times, https://www.ft.com/content/d94760ec-56c4-4051-965d-1fe2b35e4d71.
[11] FSB (2017), Artificial Intelligence and Machine Learning In Financial Services Market Developments and Financial Stability Implications, https://www.fsb.org/wp-content/uploads/P011117.pdf.
[46] FSRA (2019), Supplementary Guidance-Authorisation of Digital Investment Management (“Robo-advisory”) Activities.
[30] G20 Saudi Arabia (2020), G20 Riyadh InfraTech Agenda, https://cdn.gihub.org/umbraco/media/3008/g20-riyadh-infratech-agenda.pdf.
[53] Gensler, G. and L. Bailey (2020), “Deep Learning and Financial Stability”, SSRN Electronic Journal, http://dx.doi.org/10.2139/ssrn.3723132.
[34] Goodman, B. and S. Flaxman (2016), European Union regulations on algorithmic decision-making and a ``right to explanation’’, http://dx.doi.org/10.1609/aimag.v38i3.2741.
[29] Hackernoon (2020), Running Artificial Intelligence on the Blockchain | Hacker Noon, https://hackernoon.com/running-artificial-intelligence-on-the-blockchain-77490d37e616.
[33] Hardoon, D. (2020), Contextual Explainability, https://davidroihardoon.com/blog/f/contextual-explainability?blogcategory=Explainability (accessed on 15 March 2021).
[38] Holzinger, A. (2018), “From Machine Learning to Explainable AI”, https://www.researchgate.net/profile/Andreas_Holzinger/publication/328309811_From_Machine_Learning_to_Explainable_AI/links/5c3cd032a6fdccd6b5ac71e6/From-Machine-Learning-to-Explainable-AI.pdf.
[21] Hurley, M. (2017), Credit scoring in the era of big data, Yale Journal of Law and Technology, https://yjolt.org/sites/default/files/hurley_18yjolt136_jz_proofedits_final_7aug16_clean_0.pdf.
[9] IBM (2020), AI vs. Machine Learning vs. Deep Learning vs. Neural Networks: What’s the Difference? | IBM, https://www.ibm.com/cloud/blog/ai-vs-machine-learning-vs-deep-learning-vs-neural-networks.
[31] IBM (2020), The Four V’s of Big Data | IBM Big Data & Analytics Hub, https://www.ibmbigdatahub.com/infographic/four-vs-big-data.
[1] IDC (2020), Worldwide Spending on Artificial Intelligence Is Expected to Double in Four Years, Reaching $110 Billion in 2024, According to New IDC Spending Guide, https://www.idc.com/getdoc.jsp?containerId=prUS46794720.
[14] IIROC (2012), Rules Notice Guidance Note Guidance Respecting Electronic Trading, https://www.iiroc.ca/news-and-publications/notices-and-guidance/guidance-respecting-electronic-trading.
[39] IOSCO (2020), “The use of artificial intelligence and machine learning by market intermediaries and asset managers Consultation Report INTERNATIONAL ORGANIZATION OF SECURITIES COMMISSIONS”, http://www.iosco.org (accessed on 14 September 2021).
[8] JPMorgan (2019), Machine Learning in FX, https://www.jpmorgan.com/solutions/cib/markets/machine-learning-fx.
[35] Klein, A. (2020), Reducing bias in AI-based financial services, Brookings, https://www.brookings.edu/research/reducing-bias-in-ai-based-financial-services/.
[10] Liew, L. (2020), What is a Walk-Forward Optimization and How to Run It? - AlgoTrading101 Blog, Algotrading101, https://algotrading101.com/learn/walk-forward-optimization/.
[54] Noonan (1998), AI in banking: the reality behind the hype | Financial Times, Financial Times, https://www.ft.com/content/b497a134-2d21-11e8-a34a-7e7563b0b0f4.
[42] Nourani, M. et al. (2020), The Effects of Meaningful and Meaningless Explanations on Trust and Perceived System Accuracy in Intelligent Systems, http://www.aaai.org.
[2] OECD (2021), Artificial Intelligence, Machine Learning and Big Data in Finance: Opportunities, Challenges and Implications for Policy Makers, https://www.oecd.org/finance/financial-markets/Artificial-intelligence-machine-learning-big-data-in-finance.pdf.
[25] OECD (2020), The Tokenisation of Assets and Potential Implications for Financial Markets, OECD Paris, https://www.oecd.org/finance/The-Tokenisation-of-Assets-and-Potential-Implications-for-Financial-Markets.htm.
[52] OECD (2019), Artificial Intelligence in Society, OECD Publishing, Paris, https://dx.doi.org/10.1787/eedfee77-en.
[12] OECD (2019), OECD Business and Finance Outlook 2019: Strengthening Trust in Business, OECD Publishing, Paris, https://doi.org/10.1787/af784794-en.
[16] OECD (2017), Algorithms and Collusion: Competition Policy In the Digital Age, https://www.oecd.org/daf/competition/Algorithms-and-colllusion-competition-policy-in-the-digital-age.pdf.
[19] S&P (2019), Avoiding Garbage in Machine Learning, https://www.spglobal.com/en/research-insights/articles/avoiding-garbage-in-machine-learning-shell.
[28] The Technolawgist (2020), Does the future of smart contracts depend on artificial intelligence? - The Technolawgist, https://www.thetechnolawgist.com/2020/12/07/does-the-future-of-smart-contracts-depend-on-artificial-intelligence/.
[41] UK Information Commissioner’s Office (2020), What are the contextual factors?, https://ico.org.uk/for-organisations/guide-to-data-protection/key-data-protection-themes/explaining-decisions-made-with-artificial-intelligence/part-1-the-basics-of-explaining-ai/what-are-the-contextual-factors/.
[32] US Treasury (2018), A Financial System That Creates Economic Opportunities Nonbank Financials, Fintech, and Innovation Report to President Donald J. Trump Executive Order 13772 on Core Principles for Regulating the United States Financial System Counselor to the Secretary, https://home.treasury.gov/sites/default/files/2018-08/A-Financial-System-that-Creates-Economic-Opportunities---Nonbank-Financials-Fintech-and-Innovation.pdf.
[18] US Treasury (2016), Opportunities and Challenges in Online Marketplace Lending, https://www.treasury.gov/connect/blog/Pages/Opportunities-and-Challenges-in-Online-Marketplace-Lending.aspx.
[22] White & Case (2017), Algorithms and bias: What lenders need to know, https://www.jdsupra.com/legalnews/algorithms-and-bias-what-lenders-need-67308/.
[51] Widmer, G. (1996), Learning in the Presence of Concept Drift and Hidden Contexts.
[49] Xu, Y. and R. Goodacre (2018), “On Splitting Training and Validation Set: A Comparative Study of Cross-Validation, Bootstrap and Systematic Sampling for Estimating the Generalization Performance of Supervised Learning”, Journal of Analysis and Testing, Vol. 2/3, pp. 249-262, http://dx.doi.org/10.1007/s41664-018-0068-2.
[26] Ziqi Chen et al. (2020), Cortex Blockchain Whitepaper, https://www.cortexlabs.ai/cortex-blockchain.
Notes
Copy link to Notes← 1. The use of the term AI in this note includes AI and its applications through ML models and the use of big data.
← 2. For the purposes of this section, asset managers include traditional and alternative asset managers (hedge funds).
← 3. Walk forward optimisation is a process for testing a trading strategy by finding its optimal trading parameters in a certain time period (called the in-sample or training data) and checking the performance of those parameters in the following time period (called the out-of-sample or testing data) (Liew, 2020[10]).
← 4. Such tools can also be used in high frequency trading to the extent that investors use them to place trades ahead of competition.
← 5. As opposed to value-based trade, which focuses on fundamentals.
← 6. Spoofing is an illegal market manipulation practice that involves placing bids to buy or offers to sell securities or commodities with the intent of cancelling the bids or offers prior to the deal’s execution. It is designed to create a false sense of investor demand in the market, thereby manipulating the behaviour and actions of other market participants and allowing the spoofer to profit from these changes by reacting to the fluctuations.
← 7. It should be noted, however, that the risk of discrimination and unfair bias exists equally in traditional, manual credit rating mechanisms, where the human parameter could allow for conscious or unconscious biases.
← 8. Inspired by the functionality of human brains where hundreds of billions of interconnected neurons process information in parallel, neural networks are composed of basic units somewhat analogous to human neurons, with units linked to each other by connections whose strength is modifiable as a result of a learning process or algorithm. Deep learning neural networks are modelling the way neurons interact in the brain with many (‘deep’) layers of simulated interconnectedness (OECD, 2021[2]).
← 9. Blockchain and distributed ledger technologies are terms used interchangeably in this Chapter.
← 10. Oracles feed external data into the blockchain. They can be external service providers in the form of an API endpoint, or actual nodes of the chain. They respond to queries of the network with specific data points that they bring from sources external to the network.
← 11. Smart contracts are distributed applications written as code on Blockchain ledgers, automatically executed upon reaching pre-defined trigger events written in the code (OECD, 2020[25]).
← 12. Natural Language Processing (NLP), a subset of AI, is the ability of a computer program to understand human language as it is spoken and written (referred to as natural language).
← 13. In cases of credit decisions, this also includes information on factors, including personal data that have influenced the applicant’s credit scoring. In certain jurisdictions, such as Poland, information should also be provided to the applicant on measures that the applicant can take to improve their creditworthiness.
← 14. Regulatory sandboxes specifically targeting AI applications could be a way to understand some of these potential incompatibilities, as was the case in Colombia.