This chapter provides guidance on the use of innovation data for constructing indicators as well as statistical and econometric analysis. The chapter provides a blueprint for the production of innovation indicators by thematic areas, drawing on the recommendations in previous chapters. Although targeted to official organisations and other users of innovation data, such as policy analysts and academics, the guidance in this chapter also seeks to promote better understanding among innovation data producers about how their data are or might be used. The chapter provides suggestions for future experimentation and the use of innovation data in policy analysis and evaluation. The ultimate objective is to ensure that innovation data, indicators and analysis provide useful information for decision makers in government and industry while ensuring that trust and confidentiality are preserved.
Oslo Manual 2018
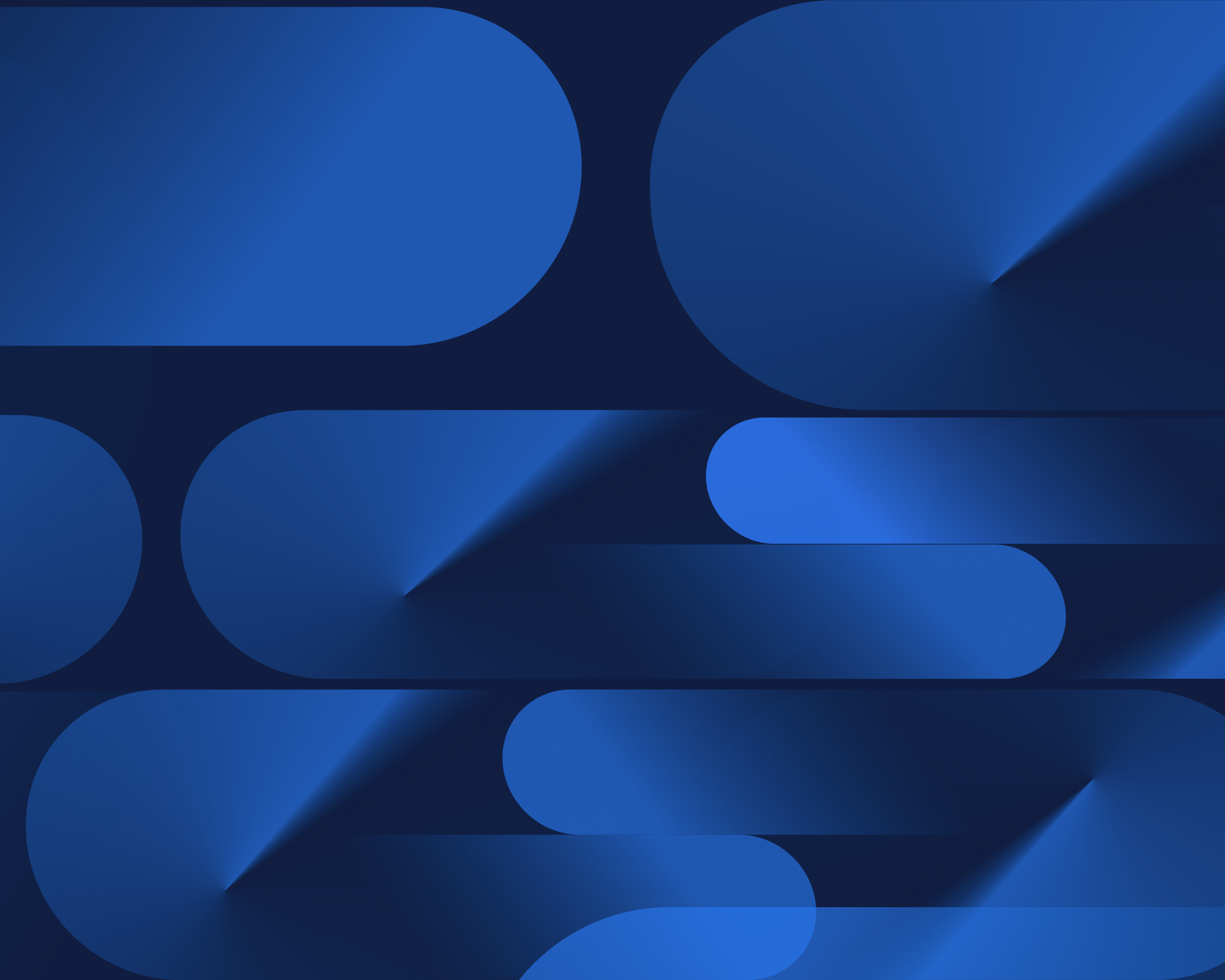
Chapter 11. Use of innovation data for statistical indicators and analysis
Abstract
11.1. Introduction
11.1. Innovation data can be used to construct indicators and for multivariate analysis of innovation behaviour and performance. Innovation indicators provide statistical information on innovation activities, innovations, the circumstances under which innovations emerge, and the consequences of innovations for innovative firms and for the economy. These indicators are useful for exploratory analysis of innovation activities, for tracking innovation performance over time and for comparing the innovation performance of countries, regions, and industries. Multivariate analysis can identify the significance of different factors that drive innovation decisions, outputs and outcomes. Indicators are more accessible to the general public and to many policy makers than multivariate analysis and are often used in media coverage of innovation issues. This can influence public and policy discussions on innovation and create demand for additional information.
11.2. This chapter provides guidance on the production, use, and limitations of innovation indicators, both for official organisations and for other users of innovation data, such as policy analysts and academics who wish to better understand innovation indicators or produce new indicators themselves. The discussion of multivariate analyses is relevant to researchers with access to microdata on innovation and to policy analysts. The chapter also includes suggestions for future experimentation. The ultimate objective is to ensure that innovation data, indicators and analysis provide useful information for decision makers in both government and industry, as discussed in Chapters 1 and 2.
11.3. Most of the discussion in this chapter focuses on data collected through innovation surveys (see Chapter 9). However, the guidelines and suggestions for indicators and analysis also apply to data obtained from other sources. For some topics, data from other sources can substantially improve analysis, such as for research on the effects of innovation activities on outcomes (see Chapter 8) or the effect of the firm’s external environment on innovation (see Chapters 6 and 7).
11.4. Section 11.2 below introduces the concepts of statistical data and indicators relating to business innovation, and discusses desirable properties and the main data resources available. Section 11.3 covers methodologies for constructing innovation indicators and aggregating them using dashboards, scoreboards and composite indexes. Section 11.4 presents a blueprint for the production of innovation indicators by thematic areas, drawing on the recommendations in previous chapters. Section 11.5 covers multivariate analyses of innovation data, with a focus on the analysis of innovation outcomes and policy evaluation.
11.2. Data and indicators on business innovation
11.2.1. What are innovation indicators and what are they for?
11.5. An innovation indicator is a statistical summary measure of an innovation phenomenon (activity, output, expenditure, etc.) observed in a population or a sample thereof for a specified time or place. Indicators are usually corrected (or standardised) to permit comparisons across units that differ in size or other characteristics. For example, an aggregate indicator for national innovation expenditures as a percentage of gross domestic product (GDP) corrects for the size of different economies (Eurostat, 2014; UNECE, 2000).
11.6. Official statistics are produced by organisations that are part of a national statistical system (NSS) or by international organisations. An NSS produces official statistics for government. These statistics are usually compiled within a legal framework and in accordance with basic principles that ensure minimum professional standards, independence and objectivity. Organisations that are part of an NSS can also publish unofficial statistics, such as the results of experimental surveys. Statistics about innovation and related phenomena have progressively become a core element of the NSS of many countries, even when not compiled by national statistical organisations (NSOs).
11.7. Innovation indicators can be constructed from multiple data sources, including some that were not explicitly designed to support the statistical measurement of innovation. Relevant sources for constructing innovation indicators include innovation and related surveys, administrative data, trade publications, the Internet, etc. (see Chapter 9). The use of multiple data sources to construct innovation indicators is likely to increase in the future due to the growing abundance of data generated or made available on line and through other digital environments. The increasing ability to automate the collection, codification and analysis of data is another key factor expanding the possibilities for data sourcing strategies.
11.8. Although increasingly used within companies and for other purposes, indicators of business innovation, especially those from official sources, are usually designed to inform policy and societal discussions, for example to monitor progress towards a related policy target (National Research Council, 2014). Indicators themselves can also influence business behaviour, including how managers respond to surveys. An evaluation of multiple innovation indicators, along with other types of information, can assist users in better understanding a wider range of innovation phenomena.
11.2.2. Desirable properties of innovation indicators
11.9. The desirable properties of innovation indicators include relevance, accuracy, reliability, timeliness, coherence and accessibility, as summarised in Table 11.1. The properties of innovation indicators are determined by choices made throughout all phases of statistical production, especially in the design and implementation of innovation surveys, which can greatly affect data quality (see Chapter 9). To be useful, indicators must have multiple quality characteristics (Gault [ed.], 2013). For example, accurate, reliable and accessible indicators will be of limited relevance if a delay in timeliness means that they are not considered in policy discussions or decisions.
Table 11.1. Desirable properties of business innovation indicators
Feature |
Description |
Comments |
---|---|---|
Relevance |
Serve the needs of actual and potential users |
Innovation involves change, leading to changes in the needs of data users. Relevance can be reduced if potential users are unaware of available data or data producers are unaware of users’ needs. |
Accuracy/ validity |
Provide an unbiased representation of innovation phenomena |
There may be systematic differences in how respondents provide information depending on the collection method or respondent characteristics. Indicators can fail to capture all relevant phenomena of interest. |
Reliability/precision |
Results of measurement should be identical when repeated. High signal-to-noise ratio |
Results can differ by the choice of respondent within a firm. Reliability can decline if respondents guess the answer to a question or if sample sizes are too small (e.g. in some industries). |
Timeliness |
Available on a sufficiently timely basis to be useful for decision-making |
Lack of timeliness reduces the value of indicators during periods of fast economic change. Timeliness can be improved through nowcasting or collecting data on intentions. However, some aspects of innovation are structural and change slowly. For these, timeliness is less of a concern. |
Coherence/comparability |
Logically connected and mutually consistent |
|
Additive or decomposable at different aggregation levels |
High levels of aggregation can improve reliability/precision, but reduce usefulness for policy analysis. Low levels of aggregation can influence strategic behaviour and distort measurement. |
|
Decomposable by characteristics |
For example, by constructing indicators for different types of firms according to innovations or innovation activities, etc. |
|
Coherence over time |
Use of time series data should be promoted. Breaks in series can sometimes be addressed through backward revisions if robustly justified and explained. |
|
Coherence across sectors, regions or countries, including international comparability |
Comparability across regions or countries requires standardisation to account for differences in size or industrial structure of economies. |
|
Accessibility and clarity |
Widely available and easy to understand, with supporting metadata and guidance for interpretation |
Challenges to ensure that the intended audience understands the indicators and that they “stir the imagination of the public” (EC, 2010). |
11.2.3. Recommendations and resources for innovation indicators
Basic principles
11.10. In line with general statistical principles (UN, 2004), business innovation statistics must be useful and made publicly available on an impartial basis. It is recommended that NSOs and other agencies that collect innovation data use a consistent schema for presenting aggregated results and apply this to data obtained from business innovation surveys. The data should be disaggregated by industry and firm size, as long as confidentiality and quality requirements are met. These data are the basic building blocks for constructing indicators.
International comparisons
11.11. User interest in benchmarking requires internationally comparable statistics. The adoption by statistical agencies of the concepts, classifications and methods contained in this manual will further promote comparability. Country participation in periodical data reporting exercises to international organisations such as Eurostat, the OECD and the United Nations can also contribute to building comparable innovation data.
11.12. As discussed in Chapter 9, international comparability of innovation indicators based on survey data can be reduced by differences in survey design and implementation (Wilhelmsen, 2012). These include differences between mandatory and voluntary surveys, survey and questionnaire design, follow-up practices, and the length of the observation period. Innovation indicators based on other types of data sources are also subject to comparability problems, for example in terms of coverage and reporting incentives.
11.13. Another factor affecting comparability stems from national differences in innovation characteristics, such as the average novelty of innovations and the predominant types of markets served by firms. These contextual differences also call for caution in interpreting indicator data for multiple countries.
11.14. Some of the issues caused by differences in methodology or innovation characteristics can be addressed through data analysis. For example, a country with a one-year observation period can (if available) use panel data to estimate indicators for a three-year period. Other research has developed “profile” indicators (see subsection 3.6.2) that improve the comparability of national differences in the novelty of innovations and markets on headline indicators such as the share of innovative firms (Arundel and Hollanders, 2005).
11.15. Where possible and relevant, it is recommended to develop methods for improving the international comparability of indicators, in particular for widely used headline indicators.
International resources
11.16. Box 11.1 lists three sources of internationally comparable indicators on innovation that follow, in whole or in part, Oslo Manual guidelines and are available at the time of publishing this manual.
Box 11.1. Major resources for international innovation data using Oslo Manual guidelines
Eurostat Community Innovation Survey (CIS) indicator database
Innovation indicators from the CIS for selected member states of the European Statistical System (ESS): http://ec.europa.eu/eurostat/web/science-technology-innovation/data/database.
Ibero-American/Inter-American Network of Science and Technology Indicators (RICYT)
Innovation indicators for manufacturing and service industries for selected Ibero-American countries: www.ricyt.org/indicadores.
OECD Innovation Statistics Database
Innovation indicators for selected industries for OECD member countries and partner economies, including countries featured in the OECD Science, Technology and Industry Scoreboard: http://oe.cd/inno-stats.
UNESCO Institute for Statistics (UIS) Innovation Data
Global database of innovation statistics focused on manufacturing industries: http://uis.unesco.org/en/topic/innovation-data.
The NEPAD (New Partnership for Africa’s Development) for the African Union is also active in promoting the use of comparable indicators in Africa. Online links to this manual will provide up-to-date links to international and national sources of statistical data and indicators on innovation.
11.3. Methodologies for constructing business innovation indicators
11.3.1. Aggregation of statistical indicators
11.17. Table 11.2 summarises different types of descriptive statistics and methods used to construct indicators. Relevant statistics include measures of central tendency, dispersion, association, and dimension reduction techniques.
Micro and macro indicators
11.18. Indicators can be constructed from various sources at any level of aggregation equal to or higher than the statistical unit for which data are collected. For survey and many types of administrative data, confidentiality restrictions often require indicators to be based on a sufficient level of aggregation so that users of those indicators cannot identify values for individual units. Indicators can also be constructed from previously aggregated data.
11.19. Common characteristics for aggregation include the country and region where the firm is located and characteristics of the firm itself, such as its industry and size (using size categories such as 10 to 49 persons employed, etc.). Aggregation of business-level data requires an understanding of the underlying statistical data and the ability to unequivocally assign a firm to a given category. For example, regional indicators require an ability to assign or apportion a firm or its activities to a region. Establishment data are easily assigned to a single region, but enterprises can be active in several regions, requiring spatial imputation methods to divide activities between regions.
11.20. Indicators at a low level of aggregation can provide detailed information that is of greater value to policy or understanding than aggregated indicators alone. For example, an indicator for the share of firms by industry with a product innovation will provide more useful information than an indicator for all industries combined.
Table 11.2. Descriptive statistics and methods for constructing innovation indicators
Generic examples |
Innovation examples |
|
---|---|---|
Types of indicators |
||
Statistical measures of frequency |
Counts, conditional counts |
Counts of product innovators |
Measures of position, order or rank |
Ranking by percentile or quartiles |
Firms in the top decile of innovation expenditure distribution |
Measures of central tendency |
Mean, median, mode |
Share of firms with a service innovation, median share of income/ turnover from product innovations |
Measures of dispersion |
Interquartile ranges, variance, standard deviation, coefficient of variation |
Coefficient of variation presented for error margins, standard deviation of innovation expenditures |
Indicators of association for multidimensional data |
||
Statistical measures of association |
Cross-tabulations, correlation/covariance |
Jaccard measures of co-occurrence of different innovation types |
Visual association |
Scatter plots, heat maps and related visuals |
Heat maps to show propensity to innovate compared across groups defined by two dimensions |
Adjustments to data for indicators |
||
Indicators based on data transformations |
Logs, inverse |
Log of innovation expenditures |
Weighting |
Weighting of the importance of indicators when constructing composite indicators, by major variables etc. |
Indicators weighted by firm size or adjusted for industry structure |
Normalisation |
Ratios, scaling by size, turnover, etc. |
Percent of employees that work for an innovative firm, etc. |
Dimension reduction techniques |
||
Simple central tendency methods |
Average of normalised indicators |
Composite innovation indexes |
Other indicator methods |
Max or min indicators |
Firms introducing at least one type of innovation out of multiple types |
Statistical dimension reduction and classification methods |
Principal component analysis, multidimensional scaling, clustering |
Studies of “modes” of innovation, e.g. Frenz and Lambert (2012) |
Dimensionality reduction for indicators
11.21. Surveys often collect information on multiple related factors, such as different knowledge sources, innovation objectives, or types of innovation activities. This can provide a complex set of data that is difficult to interpret. A common approach is to reduce the number of variables (dimensionality reduction) while maintaining the information content. Several statistical procedures ranging from simple addition to factor analysis can be used for this purpose.
11.22. Many indicators are calculated as averages, sums, or maximum values across a range of variables (see Table 11.2). These methods are useful for summarising related nominal, ordinal, or categorical variables that are commonly found in innovation surveys. For example, a firm that reports at least one type of innovation out of a list of eight innovation types (two products and six business processes) is defined as an innovative firm. This derived variable can be used to construct an aggregate indicator for the average share of innovative firms by industry. This is an example of an indicator where only one positive value out of multiple variables is required for the indicator to be positive. The opposite is an indicator that is only positive when a firm gives a positive response to all relevant variables.
11.23. Composite indicators are another method for reducing dimensionality. They combine multiple indicators into a single index based on an underlying conceptual model (OECD/JRC, 2008). Composite indicators can combine indicators for the same dimension (for instance total expenditures on different types of innovation activities), or indicators measured along multiple dimensions (for example indicators of framework conditions, innovation investments, innovation activities, and innovation impacts).
11.24. The number of dimensions can also be reduced through statistical methods such as cluster analysis and principal component analysis. Several studies have applied these techniques to microdata to identify typologies of innovation behaviour and to assess the extent to which different types of behaviour can predict innovation outcomes (de Jong and Marsili, 2006; Frenz and Lambert, 2012; OECD, 2013).
11.3.2. Indicator development and presentation for international comparisons
11.25. The selection of innovation indicators reflects a prioritisation of different types of information about innovation. The ability to construct indicators from microdata creates greater opportunities for indicator construction, but this is rarely an option for experts or organisations without access to microdata. The alternative is to construct indicators from aggregated data, usually at the country, sector, or regional level.
11.26. Reports that use multiple innovation indicators for international comparisons tend to share a number of common features (Arundel and Hollanders, 2008; Hollanders and Janz, 2013) such as:
The selection of specific innovation indicators at a country, sector, or regional level is usually guided by innovation systems theory.
The selection is also partly guided by conceptual and face validity considerations, although this is constrained by data availability.
Indicators are presented by thematic area, with themes grouped within a hierarchical structure, such as innovation inputs, capabilities, and outputs.
Varying levels of contextual and qualitative information for policy making are provided, as well as methodological information.
11.27. NSS organisations and most international organisations tend to address user requests for international comparisons through reports or dashboards based on official statistics, often drawing attention to headline indicators. The advantage of reports and dashboards is that they provide a fairly objective and detailed overview of the available information. However, due to the large amount of data presented, it can be difficult to identify the key issues. Composite innovation indexes, presented in scoreboards that rank the performance of countries or regions, were developed to address the limitations of dashboards. They are mostly produced by consultants, research institutes, think tanks and policy institutions that lack access to microdata, with the composite indexes constructed by aggregating existing indicators.
11.28. Compared to simple indicators used in dashboards, the construction of composite innovation indexes requires two additional steps:
The normalisation of multiple indicators, measured on different scales (nominal, counts, percentages, expenditures, etc.), into a single scale. Normalisation can be based on standard deviations, the min-max method, or other options.
The aggregation of normalised indicators into one or more composite indexes. The aggregation can give an identical weight to all normalised indicators or use different weights. The weighting determines the relative contribution of each indicator to the composite index.
11.29. Composite indexes provide a number of advantages as well as challenges over simple indicators (OECD/JRC, 2008). The main advantages are a reduction in the number of indicators and simplicity, both of which are desirable attributes that facilitate communication with a wider user base (i.e. policy makers, media, and citizens). The disadvantages of composite indexes are as follows:
With few exceptions, the theoretical basis for a composite index is limited. This can result in problematic combinations of indicators, such as indicators for inputs and outputs.
Only the aggregate covariance structure of underlying indicators can be used to build the composite index, if used at all.
The relative importance or weighting of different indicators is often dependent on the subjective views of those constructing the composite index. Factors that are minor contributors to innovation can be given as much weight as major ones.
Aside from basic normalisation, structural differences between countries are seldom taken into account when calculating composite performance indexes.
Aggregation results in a loss of detail, which can hide potential weaknesses and increase the difficulty in identifying remedial action.
11.30. Due to these disadvantages, composite indicators need to be accompanied by guidance on how to interpret them. Otherwise, they can mislead readers into supporting simple solutions to complex policy issues.
11.31. The various innovation dashboards, scoreboards and composite indexes that are currently available change frequently. Box 11.2 provides examples that have been published on a regular basis.
11.32. The combination of a lack of innovation data for many countries, plus concerns over the comparability of innovation survey data, has meant that many innovation rankings rely on widely available indicators that capture only a fraction of innovation activities, such as R&D expenditures or IP rights registrations, at the expense of other relevant dimensions.
Box 11.2. Examples of innovation scoreboards and innovation indexes
OECD Science, Technology and Innovation (STI) Scoreboard
The OECD STI Scoreboard (www.oecd.org/sti/scoreboard.htm) is a biennial flagship publication by the OECD Directorate for Science, Technology and Innovation. Despite its name, it is closer to a dashboard. A large number of indicators are provided, including indicators based on innovation survey data, but no rankings based on composite indexes for innovation themes are included. Composite indicators are only used for narrowly defined constructs such as scientific publications or patent quality with weights constructed from auxiliary data related to the construct.
European Innovation Scoreboard (EIS)
The EIS is published by the European Commission (EC) and produced by consultants with inputs from various EC services. It is intended as a performance scoreboard (see: http://ec.europa.eu/growth/industry/innovation/facts-figures/scoreboards_en). The EIS produces a hierarchical composite index (Summary Innovation Index) that is used to assign countries into four performance groups (innovation leaders, strong innovators, moderate innovators, modest innovators). The index uses a range of data sources, including innovation survey indicators. The European Commission also publishes a related Regional Innovation Scoreboard.
Global Innovation Index (GII)
The Global Innovation Index (www.globalinnovationindex.org) is published by Cornell University, INSEAD and the World Intellectual Property Organization (WIPO). The GII is a hierarchical composite index with input and output dimensions that are related to different aspects of innovation. The GII aims to cover as many middle- and low-income economies as possible. It uses research and experimental development (R&D) and education statistics, administrative data such as intellectual property (IP) statistics and selected World Economic Forum indicators that aggregate subjective expert opinions about topics such as innovation linkages. The GII does not currently use indicators derived from innovation surveys.
11.3.3. Firm-level innovation rankings
11.33. A number of research institutes and consultants produce rankings of individual firms on the basis of selected innovation activities by constructing composite indicators from publicly available data, such as company annual reports or administrative data provided by companies subject to specific reporting obligations, for example those listed on a public stock exchange. Notwithstanding data curation efforts, these data are generally neither complete nor fully comparable across firms in the broad population. Privately owned firms are not required to report some types of administrative data while commercially sensitive data on innovation are unlikely to be included in an annual report unless disclosure supports the strategic interests or public relations goals of the firm (Hill, 2013). Consequently, there can be a strong self-selection bias in publicly available innovation data for firms. Furthermore, reported data can be misleading. For example, creative media content development activities or other technology-related activities may be reported as R&D without matching the OECD definition of R&D (OECD, 2015).
11.34. Despite these self-selection biases (see Chapter 9), publicly available firm-level data from annual reports or websites offer opportunities for constructing new experimental innovation indicators provided that the data meet basic quality requirements for the intended analytical purposes.
11.4. A blueprint for indicators on business innovation
11.35. This section provides guidelines on the types of innovation indicators that can be produced by NSOs and other organisations with access to innovation microdata. Many of these indicators are in widespread use and based on data collected in accordance with previous editions of this manual. Indicators are also suggested for new types of data discussed in Chapters 3 to 8. Other types of indicators can be constructed to respond to changes in user needs or when new data become available.
11.36. Producers of innovation indicators can use answers to the following questions to guide the construction and presentation of indicators:
What do users want to know and why? What are the relevant concepts?
What indicators are most suitable for representing a concept of interest?
What available data are appropriate for constructing an indicator?
What do users need to know to interpret an indicator?
11.37. The relevance of a given set of indicators depends on user needs and how the indicators are used (OECD, 2010). Indicators are useful for identifying differences in innovation activities across categories of interest, such as industry or firm size, or to track performance over time. Conversely, indicators should not be used to identify causal relationships, such as the factors that influence innovation performance. This requires analytical methods, as described in section 11.5 below.
11.4.1. Choice of innovation indicators
11.38. Chapters 3 to 8 cover thematic areas that can guide the construction of innovation indicators. The main thematic areas, the relevant chapter in this manual that discusses each theme, and the main data sources for constructing indicators are summarised in Table 11.3. Indicators for many of the thematic areas can also be constructed using object-based methods as discussed in Chapter 10, but these indicators will be limited to specific types of innovations.
Table 11.3. Thematic areas for business innovation indicators
Thematic area |
Main data sources |
Relevant OM4 chapters |
---|---|---|
Incidence of innovations and their characteristics (e.g. type, novelty) |
Innovation surveys, administrative or commercial data (e.g. product databases) |
3 |
Innovation activity and investment (types of activity and resources for each activity) |
Innovation surveys, administrative data, IP data (patents, trademarks, etc.) |
4 |
Innovation capabilities within firms1 |
Innovation surveys, administrative data |
5 |
Innovation linkages and knowledge flows |
Innovation surveys, administrative data, bilateral international statistics (trade, etc.), data on technology alliances |
6 |
External influences on innovation (including public policies) and framework conditions for business innovation (including knowledge infrastructure)1 |
Innovation surveys, administrative data, expert assessments, public opinion polls, etc. |
6,7 |
Outputs of innovation activities |
Innovation surveys, administrative data |
6,8 |
Economic and social outcomes of business innovation |
Innovation surveys, administrative data |
8 |
1. New thematic area for this edition of the manual (OM4).
11.39. Table 11.4 provides a list of proposed indicators for measuring the incidence of innovation that can be mostly produced using nominal data from innovation surveys, as discussed in Chapter 3. These indicators describe the innovation status of firms and the characteristics of their innovations.
Table 11.4. Indicators of innovation incidence and characteristics
General topic |
Indicator |
Computation notes |
---|---|---|
Product innovations |
Share of firms with one or more types of product innovations |
Based on a list of product innovation types. Can be disaggregated by type of product (good or service) |
New-to-market (NTM) product innovations |
Share of firms with one or more NTM product innovations (can also focus on new-to-world product innovations) |
Depending on the purpose, can be computed as the ratio to all firms or innovative firms only |
Method of developing product innovations |
Share of firms with one or more types of product innovations that developed these innovations through imitation, adaptation, collaboration, or entirely in-house |
Based on Chapter 6 guidance. Categories for how innovations were developed must be mutually exclusive *Relevant to innovative firms only |
Other product innovation features |
Depending on question items, indicators can capture attributes of product innovations (changes to function, design, experiences etc.) |
*Not relevant to all firms |
Business process Innovations |
Share of firms with one or more types of business process innovations |
Based on a list of types of business process innovations. Can be disaggregated by type of business process |
NTM business process innovations |
Share of firms with one or more NTM business process innovations |
Depending on the purpose, can be computed as the ratio to all firms or innovative firms only |
Method of developing business process innovations |
Share of firms with one or more types of business process innovations that developed these innovations through imitation, adaptation, collaboration, or entirely in-house |
Based on Chapter 6. Categories for how innovations were developed must be mutually exclusive *Only relevant to firms with a business process innovation |
Product and business process innovations |
Share of firms with both product and business process innovations |
Co-occurrence of specific types of innovations |
Innovative firms |
Share of firms with at least one innovation of any type |
Total number of firms with a product innovation or a business process innovation |
Ongoing/abandoned innovation activities |
Share of firms with ongoing innovation activities or with activities abandoned or put on hold |
Can be limited to firms that only had ongoing/abandoned activities, with no innovations |
Innovation-active firms |
Share of firms with one or more types of innovation activities |
All firms with completed, ongoing or abandoned innovation activities *Can only be calculated for all firms |
Note: All indicators refer to activities within the survey observation period. Indicators for innovation rates can also be calculated as shares of employment or turnover, for instance the share of total employees that work for an innovative firm, or the share of total sales earned by innovative firms. Unless otherwise noted with an “*” before a computation note, all indicators can be computed using all firms, innovation-active firms only, or innovative firms only as the denominator. See section 3.5 for a definition of firm types.
11.40. Table 11.5 lists proposed indicators of knowledge-based activities as discussed in Chapter 4. With a few exceptions, most of these indicators can be calculated for all firms, regardless of their innovation status (see Chapter 3).
Table 11.5. Indicators of knowledge-based capital/innovation activities
General topic |
Indicator |
Computation notes |
---|---|---|
Knowledge-based capital (KBC) activities |
Share of firms reporting KBC activities that are potentially related to innovation |
Share of firms reporting at least one KBC activity (Table 4.1, column 2) *Can only be calculated for all firms |
KBC activities for innovation |
Share of firms reporting KBC activities for innovation |
Share of firms reporting at least one KBC activity for innovation (Table 4.1, columns 2 or 3) Can calculate separately for in-house (column 2) and external (column 3) investments |
Expenditures on KBC |
Total expenditures on KBC activities potentially related to innovation |
Total expenditures on KBC (Table 4.2, column 2) as a share of total turnover (or equivalent) |
Expenditures on KBC for innovation |
Total expenditures on KBC activities for innovation |
Total expenditures for innovation (Table 4.2, column 3) as a share of total turnover (or equivalent) |
Innovation expenditure share for each type of activity |
Share of expenditures for innovation for each of seven types of innovation activities |
Total expenditures for each innovation activity (Table 4.2, columns 2 and 3) as a share of total innovation expenditures *Not useful to calculate for all firms |
Innovation expenditures by accounting category |
Total expenditures for innovation activities by accounting category |
Total expenditures for each of five accounting categories (Table 4.3, column 3) as a share of total turnover (or equivalent) |
Innovation projects |
Number of innovation projects |
Median or average number of innovation projects per firm (see subsection 4.5.2) *Not useful to calculate for all firms |
Follow-on innovation activities |
Share of firms with ongoing follow-on innovation activities |
Any of three follow-on activities (see subsection 4.5.3) *Only calculate for innovative firms |
Innovation plans |
Share of firms planning to increase (reduce) their innovation expenditures in the (current) next period |
See subsection 4.5.4 |
Notes: Indicators derived from Table 4.1 refer to the survey observation period. Expenditure indicators derived from Table 4.2 and Table 4.3 only refer to the survey reference period. Unless otherwise noted with an “*” before a computation note, all indicators can be computed using all firms, innovation-active firms only, or innovative firms only as the denominator. See section 3.5 for a definition of firm types.
11.41. Table 11.6 lists potential indicators of business capabilities for innovation following Chapter 5. All indicators of innovation capability are relevant to all firms, regardless of their innovation status. The microdata can also be used to generate synthetic indexes on the propensity of firms to innovate.
Table 11.6. Indicators of potential or actual innovation capabilities
General topic |
Indicator |
Computation notes |
---|---|---|
Innovation management |
Share of firms adopting advanced general and innovation management practices |
Based on list of practices (see subsections 5.3.2 and 5.3.4) |
IP rights strategy |
Share of firms using different types of IP rights |
See subsection 5.3.5 |
Workforce skills |
Share of firms employing highly qualified personnel, by level of educational attainment or by fields of education |
Average or median share of highly qualified individuals |
Advanced technology use |
Share of firms using advanced, enabling or emerging technologies |
This may be relevant for specific sectors only (see subsection 5.5.1) |
Technical development |
Share of firms developing advanced, enabling or emerging technologies |
This may be relevant for specific sectors only (see subsection 5.5.1) |
Design capabilities |
Share of firms with employees with design skills |
See subsection 5.5.2 |
Design centrality |
Share of firms with design activity at different levels of strategic importance (Design Ladder) |
See subsection 5.5.2 |
Design thinking |
Share of firms using design thinking tools and practices |
See subsection 5.5.2 |
Digital capabilities |
Share of firms using advanced digital tools and methods |
See subsection 5.5.3 |
Digital platforms |
Share of firms using digital platforms to sell or buy goods or services Share of firms providing digital platform services |
See subsections 5.5.3 and 7.4.4 |
Notes: All indicators refer to activities within the survey observation period. All indicators can be computed using all firms, innovation-active firms only, or innovative firms only as the denominator. See section 3.5 for a definition of firm types.
11.42. Table 11.7 provides indicators of knowledge flows for innovation, following guidance in Chapter 6 on both inbound and outbound flows. With a few exceptions, most of these indicators are relevant to all firms.
Table 11.7. Indicators of knowledge flows and innovation
General topic |
Indicator |
Computation notes |
---|---|---|
Collaboration |
Share of firms that collaborated with other parties on innovation activities (by type of partner or partner location) |
See Table 6.5 *Not useful to calculate for all firms |
Main collaboration partner |
Share of firms indicating a given partner type as most important |
See Table 6.5 and Chapter 10 *Not useful to calculate for all firms |
Knowledge sources |
Share of firms making use of a range of information sources |
See Table 6.6 |
Licensing-out |
Share of firms with outbound licensing activities |
See Table 6.4 |
Knowledge services providers |
Share of firms with a contract to develop products or business processes for other firms or organisations |
See Table 6.4 |
Knowledge disclosure |
Share of firms that disclosed useful knowledge for the product or business process innovations of other firms or organisations |
See Table 6.4 |
Knowledge exchange with higher education institutions (HEIs) and public research institutions (PRIs) |
Share of firms engaged in specific knowledge exchange activities with HEIs or PRIs |
See Table 6.6 |
Challenges to knowledge exchange |
Share of firms reporting barriers to interacting with other parties in the production or exchange of knowledge |
See Table 6.8 |
Note: All indicators refer to activities within the survey observation period. Indicators on the role of other parties in the firm’s innovations are included in Table 11.4 above. Unless otherwise noted with an “*” before a computation note, all indicators can be computed using all firms, innovation-active firms only, or innovative firms only as the denominator. See section 3.5 for a definition of firm types.
11.43. Table 11.8 provides a list of indicators for external factors that can potentially influence innovation, as discussed in Chapter 7. With the exception of drivers of innovation, all of these indicators can be calculated for all firms.
Table 11.8. Indicators of external factors influencing innovation
General topic |
Indicator |
Computation notes |
---|---|---|
Customer type |
Share of firms selling to specific types of customers (other businesses, government, consumers) |
See subsection 7.4.1 |
Geographic market |
Share of firms selling products in international markets |
See subsection 7.4.1 |
Nature of competition |
Share of firms reporting specific competition conditions that influence innovation |
See Table 7.2 |
Standards |
Share of firms engaged in standard setting activities |
See subsection 7.4.2 |
Social context for innovation |
Share of firms reporting more than N social characteristics that are potentially conducive to innovation |
Can calculate as a score for different items (see Table 7.7) |
Public support for innovation |
Share of firms that received public support for the development or exploitation of innovations (by type of support) |
See subsection 7.5.2 |
Innovation drivers |
Share of firms reporting selected items as a driver of innovation |
See Table 7.8 *Not useful to calculate for all firms |
Public infrastructure |
Share of firms reporting selected types of infrastructure of high relevance to their innovation activities |
See Table 7.6 |
Innovation barriers |
Share of firms reporting selected items as barriers to innovation |
See Table 7.8 |
Note: All indicators refer to activities within the survey observation period. Unless otherwise noted with an “*” before a computation note, all indicators can be computed using all firms, innovation-active firms only, or innovative firms only as the denominator. See section 3.5 for a definition of firm types.
11.44. Table 11.9 lists simple outcome (or objective) indicators, based on either nominal or ordinal survey questions, as proposed in Chapter 8. The objectives are applicable to all innovation-active firms, while questions on outcomes are only relevant to innovative firms.
Table 11.9. Indicators of innovation objectives and outcomes
General topic |
Indicator |
Computation notes |
---|---|---|
General business objectives |
Share of firms reporting selected items as general objectives1 |
See Tables 8.1 and 8.2 |
Innovation objectives |
Share of firms reporting selected items as objectives for innovation activities1 |
See Tables 8.1 and 8.2 *Not useful to calculate for all firms |
Innovation outcomes |
Shares of firms attaining a given objective through their innovation activity1 |
See Tables 8.1 and 8.2 *Not useful to calculate for all firms |
Sales from new products |
Share of turnover from product innovations and new-to-market product innovations |
See subsection 8.3.1 |
Number of product innovations |
Number of new products (median and average) |
See subsection 8.3.1, preferably normalised by total number of product lines |
Changes to unit cost of sales |
Share of firms reporting different levels of changes to unit costs from business process innovations |
See subsection 8.3.2 *Calculate for firms with business process innovations only |
Innovation success |
Share of firms reporting that innovations met expectations |
See section 8.3 *Calculate for innovative firms only |
1. These indicators can be calculated by thematic area (e.g. production efficiency, markets, environment, etc.).
Note: All indicators refer to activities within the survey observation period. Unless otherwise noted with an “*” before a computation note, all indicators can be computed using all firms, innovation-active firms only, or innovative firms only as the denominator. See section 3.5 for a definition of firm types.
11.4.2. Breakdown categories, scaling, and typologies
11.45. Depending on user requirements, indicators can be provided for several breakdown characteristics. Data on each characteristic can be collected through a survey or by linking a survey to other sources such as business registers and administrative data, in line with guidance provided in Chapter 9. Breakdown characteristics of interest include:
Enterprise size by the number of persons employed, or other size measures such as sales or assets.
Industry of main economic activity, in line with international standard classifications (see Chapter 9). Combinations of two- to three-digit International Standard Industrial Classification (ISIC) classes can provide results for policy-relevant groups of firms (e.g. firms in information and communication technology industries).
Administrative region.
Group affiliation and ownership, for instance if an enterprise is independent, part of a domestic enterprise group, or part of a multinational enterprise. Breakdowns for multinationals are of value to research on the globalisation of innovation activities.
Age, measured as the time elapsed since the creation of the enterprise. A breakdown by age will help differentiate between older and more recently established firms. This is of interest to research on business dynamism and entrepreneurship (see Chapter 5).
R&D status, if the firm performs R&D in-house, funds R&D performed by other units, or is not engaged in any R&D activities (see Chapter 4). The innovation activities of firms vary considerably depending on their R&D status.
11.46. The level of aggregation for these different dimensions will depend on what the data represent, how they are collected and their intended uses. Stratification decisions in the data collection (see Chapter 9) will determine the maximum level that can be reported.
11.47. To avoid scale effects, many innovation input, output, intensity and expenditure variables can be standardised by a measure of the size of each firm, such as total expenditures, total investment, total sales, or the total number of employed persons.
11.48. A frequently used indicator of innovation input intensity is total innovation expenditures as a percentage of total turnover (sales). Alternative input intensity measures include the innovation expenditure per worker (Crespi and Zuñiga, 2010) and the share of human resources (in headcounts) dedicated to innovation relative to the total workforce.
11.49. For output indicators, the share of total sales revenue from product innovations is frequently used. In principle, this type of indicator should also be provided for specific industries because of different rates of product obsolescence. Data by industry can be used to identify industries with low product innovation rates and low innovation efficiency relative to their investments in innovation.
11.50. Standardised indicators for the number of IP rights registrations, or measures of scientific output (invention disclosures, publications, etc.) should also be presented by industry, since the relevance of these activities varies considerably. Indicators based on IP rights such as patented inventions can be interpreted as measures of knowledge appropriation strategies (see Chapter 5). Their use depends on factors such as the industry and the type of protectable knowledge (OECD, 2009a). Measures of scientific outputs of the Business enterprise sector such as publications are mostly relevant to science-based industries (OECD and SCImago Research Group, 2016). Furthermore, depending on a firm’s industry and strategy, there may be large gaps between a firm’s scientific and technological outputs and what it decides to disclose.
11.51. Indicators of innovation intensity (summing all innovation expenditures and dividing by total expenditures) can be calculated at the level of industry, region, and country. Intensity indicators avoid the need to standardise by measures of firm size.
Typologies of innovative/innovation-active firms
11.52. A major drawback of many of the indicators provided above is that they do not provide a measure of the intensity of efforts to attain product or business process innovations. The ability to identify firms by different levels of effort or innovation capabilities can be of great value for innovation policy analysis and design (Bloch and López-Bassols, 2009). This can be achieved by combining selected nominal indicators with innovation activity measures (see Table 11.5) and possibly innovation outcome measures (see Table 11.9). Several studies have combined multiple indicators to create complex indicators for different “profiles”, “modes” or taxonomies of firms, according to their innovation efforts (see Tether, 2001; Arundel and Hollanders, 2005; Frenz and Lambert, 2012).
11.53. Key priorities for constructing indicators of innovation effort or capability include incorporating data on the degree of novelty of innovations (for whom the innovation is new), the extent to which the business has drawn on its own resources to develop the concepts used in the innovation, and the economic significance for the firm of its innovations and innovation efforts.
11.4.3. Choice of statistical data for innovation indicators
11.54. The choice of data for constructing innovation indicators is necessarily determined by the purpose of the indicator and data quality requirements.
Official versus non-official sources
11.55. Where possible, indicator construction should use data from official sources that comply with basic quality requirements. This includes both survey and administrative data. For both types of data, it is important to determine if all relevant types of firms are included, if records cover all relevant data, and if record keeping is consistent across different jurisdictions (if comparisons are intended). For indicators that are constructed on a regular basis, information should also be available on any breaks in series, so that corrections can be made (where possible) to maintain comparability over time.
11.56. The same criteria apply to commercial data or data from other sources such as one-off academic studies. Commercial data sources often do not provide full details for the sample selection method or survey response rates. A lack of sufficient methodological information regarding commercial and other sources of data, as well as licensing fees for data access, have traditionally posed restrictions on their use by NSS organisations. The use of commercial data by NSS organisations can also create problems if the data provider stands to obtain a commercial advantage over its competitors.
Suitability of innovation survey data for constructing statistical indicators
11.57. Survey data are self-reported by the respondent. Some potential users of innovation data object to innovation surveys because they believe that self-reports result in subjective results. This criticism confuses self-reporting with subjectivity. Survey respondents are capable of providing an objective response to many factual questions, such as whether their firm implemented a business process innovation or collaborated with a university. These are similar to factual questions from household surveys that are used to determine unemployment rates. Subjective assessments are rarely problematic if they refer to factual behaviours.
11.58. A valid concern for users of innovation data is the variable nature of innovation. Because innovation is defined from the perspective of the firm, there are enormous differences between different innovations, which means that a simple indicator such as the share of innovative firms within a country has a very low discriminatory value. The solution is not to reject innovation indicators, but to construct indicators that can discriminate between firms of different levels of capability or innovation investments, and to provide these indicators by different breakdown categories, such as for different industries or firm size classes. Profiles, as described above, can significantly improve the discriminatory and explanatory value of indicators.
11.59. Another common concern is poor discriminatory power for many nominal or ordinal variables versus continuous variables. Data for the latter are often unattainable because respondents are unable to provide accurate answers. Under these conditions, it is recommended to identify which non-continuous variables are relevant to constructs of interest and to use information from multiple variables to estimate the construct.
Change versus current capabilities
11.60. The main indicators on the incidence of innovation (see Table 11.4) capture activities that derive from or induce change in a firm. However, a firm is not necessarily more innovative than another over the long term if the former has introduced an innovation in a given period and the latter has not. The latter could have introduced the same innovation several years before and have similar current capabilities for innovation. Indicators of capability, such as knowledge capital stocks within the firm, can be constructed using administrative sources or survey data that capture a firm’s level of readiness or competence in a given domain (see Table 11.6). Evidence on the most important innovations (see Chapter 10) can also be useful for measuring current capabilities.
11.5. Using data on innovation to analyse innovation performance, policies and their impacts
11.61. Policy and business decisions can benefit from a thorough understanding of the factors that affect the performance of an innovation system. Innovation indicators provide useful information on the current state of the system, including bottlenecks, deficiencies and weaknesses, and can help track changes over time. However, this is insufficient: decision makers also need to know how conditions in one part of the system influence other parts, and how the system works to create outcomes of interest, including the effects of policy interventions.
11.62. This section examines how innovation data can be used to evaluate the links between innovation, capability-building activities, and outcomes of interest (Mairesse and Mohnen, 2010). Relevant research has extensively covered productivity (Hall, 2011; Harrison et al., 2014), management (Bloom and Van Reenen, 2007), employment effects (Griffith et al., 2006), knowledge sourcing (Laursen and Salter, 2006), profitability (Geroski, Machin and Van Reenen, 1993), market share and market value (Blundell, Griffith and Van Reenen, 1999), competition (Aghion et al., 2005), and policy impacts (Czarnitzki, Hanel and Rosa, 2011).
11.5.1. Modelling dependencies and associations
11.63. Associations between the components of an innovation system can be identified through descriptive and exploratory analysis. Multivariate regression provides a useful tool for exploring the covariation of two variables, for example innovation outputs and inputs, conditional on other characteristics such as firm size, age and industry of main economic activity. Regression is a commonly used tool of innovation analysts and its outputs a recurrent feature in research papers on innovation.
11.64. The appropriate multivariate technique depends on the type of data, particularly for dependent variables. Innovation surveys produce mostly nominal or ordinal variables with only a few continuous variables. Ordered regression models are appropriate for ordinal dependent variables on the degree of novelty or the level of complexity in the use of a technology or business practice (Galindo-Rueda and Millot, 2015). Multinomial choice models are relevant when managers can choose between three or more exclusive states, for example between different knowledge sources or collaboration partners.
11.65. Machine learning techniques also open new areas of analysis having to do with classification, pattern identification and regression. Their use in innovation statistics is likely to increase over time.
11.5.2. Inference of causal effects in innovation analysis
11.66. Statistical association between two variables (for instance an input to innovation and a performance output) does not imply causation without additional evidence, such as a plausible time gap between an input and an output, replication in several studies, and the ability to control for all confounding variables. Unless these conditions are met (which is rare in exploratory analyses), a study should not assume causality.
11.67. Research on policy interventions must also manage self-selection and plausible counterfactuals: what would have happened in the absence of a policy intervention? The effects of a policy intervention should ideally be identified using experimental methods such as randomised trials, but the scope for experimentation in innovation policy, although increasing in recent years (Nesta, 2016), is still limited. Consequently, alternative methods are frequently used.
Impact analysis and evaluation terminology
11.68. The innovation literature commonly distinguishes between different stages of an innovation process, beginning with inputs (resources for an activity), activities, outputs (what is generated by activities), and outcomes (the effects of outputs). In a policy context, a logic model provides a simplified, linear relationship between resources, activities, outputs and outcomes. Figure 11.1 presents a generic logic model for the innovation process. Refinements to the model include multiple feedback loops.
11.69. Outputs include specific types of innovations, while outcomes are the effect of innovation on firm performance (sales, profits, market share etc.), or the effect of innovation on conditions external to the firm (environment, market structure, etc.). Impacts refer to the difference between potential outcomes under observed and unobserved counterfactual treatments. An example of a counterfactual outcome would be the sales of the firm if the resources expended for innovation had been used for a different purpose, for instance an intensive marketing campaign. In the absence of experimental data, impacts cannot be directly observed and must be inferred through other means.
Figure 11.1. Logic model used in evaluation literature applied to innovation

Source: Adapted from McLaughlin and Jordan (1999), “Logic models: A tool for telling your program’s performance story”.
11.70. In innovation policy design, the innovation logic model as described in Figure 11.1 is a useful tool for identifying what is presumed to be necessary for the achievement of desired outcomes. Measurement can capture evidence of events, conditions and behaviours that can be treated as proxies of potential inputs and outputs of the innovation process. Outcomes can be measured directly or indirectly. The evaluation of innovation policy using innovation data is discussed below.
Direct and indirect measurement of outcomes
11.71. Direct measurement asks respondents to identify whether an event is the result (at least in part) of one or more activities. For example, respondents can be asked if business process innovations reduced their unit costs, and if so, to estimate the percentage reduction. Direct measurement creates significant validity problems. For example, respondents might be able to determine with some degree of accuracy whether business process innovations were followed by cost reductions on a “yes” or “no” basis. However, the influence of multiple factors on process costs could make it very difficult for respondents to estimate the percentage reduction attributable to innovation (although they might be able to make an estimate for their most important business process innovation). Furthermore, respondents will find it easier to identify and report actual events than to speculate and assign causes to outcomes or vice versa. Business managers are likely to use heuristics to answer impact-related questions that conceptually require a counterfactual.
11.72. Non-experimental, indirect measurement collects data on inputs and outcomes and uses statistical analysis to evaluate the correlations between them, after controlling for potential confounding variables. However, there are also several challenges to using indirect methods for evaluating the factors that affect innovation outcomes.
Challenges for indirect measurement of outcomes
11.73. Innovation inputs, outputs and outcomes are related through non-linear processes of transformation and development. Analysis has to identify appropriate dependent and independent variables and potential confounding variables that provide alternative routes to the same outcome.
11.74. In the presence of random measurement error for independent variables, analysis of the relationship between the independent and dependent variables will be affected by attenuation bias, such that relationships will appear to be weaker than they actually are. In addition, endogeneity is a serious issue that can result from a failure to control for confounders, or when the dependent variable affects one or more independent variables (reverse causality). Careful analysis is required to avoid both possible causes of endogeneity.
11.75. Other conditions can increase the difficulty of identifying causality. In research on knowledge flows, linkages across actors and the importance of both intended and unintended knowledge diffusion can create challenges for identifying the effect of specific knowledge sources on outcomes. Important channels could exist for which there are no data. As noted in Chapter 6, the analysis of knowledge flows would benefit from social network graphs of the business enterprise to help identify the most relevant channels. A statistical implication of highly connected innovation systems is that the observed values are not independently distributed: competition and collaboration generate outcome dependences across firms that affect estimation outcomes.
11.76. Furthermore, dynamic effects require time series data and an appropriate model of evolving relationships in an innovation system, for example between inputs in a given period (t) and outputs in later periods (t+1). In some industries, economic results are only obtained after several years of investment in innovation. Dynamic analysis could also require data on changes in the actors in an innovation system, for instance through mergers and acquisitions. Business deaths can create a strong selection effect, with only surviving businesses available for analysis.
Matching estimators
11.77. Complementing regression analysis, matching is a method that can be used for estimating the average effect of business innovation decisions as well as policy interventions (see subsection 11.5.3 below). Matching imposes no functional form specifications on the data but assumes that there is a set of observed characteristics such that outcomes are independent of the treatment conditional on those characteristics (Todd, 2010). Under this assumption, the impact of innovation activity on an outcome of interest can be estimated from comparing the performance of innovators with a weighted average of the performance of non-innovators. The weights need to replicate the observable characteristics of the innovators in the sample. Under some conditions, the weights can be estimated from predicted innovation probabilities using discrete analysis (matching based on innovation propensity scores).
11.78. In many cases, there can be systematic differences between the outcomes of treated and untreated groups, even after conditioning on observables, which could lead to a violation of the identification conditions required for matching. Independence assumptions can be more valid for changes in the variable of interest over time. When longitudinal data are available, the “difference in differences” method can be used. An example is an analysis of productivity growth that compares firms that introduced innovations in the reference period with those that did not. Further bias reduction can be attained by using information on past innovation and economic performance.
11.79. Matching estimators and related regression analysis are particularly useful for the analysis of reduced-form causal relationship models. Reduced-form models have fewer requirements than structural models, but are less informative in articulating the mechanisms that underpin the relationship between different variables.
Structural analysis of innovation data: The CDM model
11.80. The model, developed by Crépon, Duguet and Mairesse (1998) (hence the name CDM), builds on Griliches’ (1990) path diagram of the knowledge production function and is widely used in empirical research on innovation and productivity (Lööf, Mairesse and Mohnen, 2016). The CDM framework is suitable for cross-sectional innovation survey data obtained by following this manual’s recommendations, including data not necessarily collected for indicator production purposes. It provides a structural model that explains productivity by innovation output and corrects for the selectivity and endogeneity inherent in survey data. It includes the following sub-models (Criscuolo, 2009):
1. Propensity among all firms to undertake innovation: This key step requires good quality information on all firms. This requirement provides a motivation for collecting data from all firms, regardless of their innovation status, as recommended in Chapters 4 and 5.
2. Intensity of innovation effort among innovation-active firms: The model recognises that there is an underlying degree of innovation effort for each firm that is only observed among those that undertake innovation activities. Therefore, the model controls for the selective nature of the sample.
3. Scale of innovation output: This is observed only for innovative firms. This model uses the predicted level of innovation effort identified in model 2 and a control for the self-selected nature of the sample.
4. Relationship between labour productivity and innovation effort: This is estimated by incorporating information about the drivers of the innovation outcome variable (using its predicted value) and the selective nature of the sample.
11.81. Policy variables can be included in a CDM model, provided they display sufficient variability in the sample and satisfy the independence assumptions (including no self-selection bias) required for identification.
11.82. The CDM framework has been further developed to work with repeated cross-sectional and panel data, increasing the value of consistent longitudinal data at the micro level. Data and modelling methods require additional development before CDM and CDM-related frameworks can fully address several questions of interest, such as the competing roles of R&D versus non-R&D types of innovation activity, or the relative importance or complementarity of innovation activities versus generic competence and capability development activities. Improvements in data quality for variables on non-R&D activities and capabilities would facilitate the use of extended CDM models.
11.5.3. Analysing the impact of public innovation policies
11.83. Understanding the impact of public innovation policies is one of the main user interests for innovation statistics and analysis. This section draws attention to some of the basic procedures and requirements that analysts and practitioners need to consider.
The policy evaluation problem
11.84. Figure 11.2 illustrates the missing counterfactual data problem in identifying the causal impacts of policies. This is done by means of an example where the policy “treatment” is support for innovation activities, for instance a grant to support the development and launch of a new product. Some firms receive support whereas others do not. The true impact of support is likely to vary across firms. The evaluation problem is one of missing information. The researcher cannot observe, for supported firms, what would have been their performance had they not been supported. The same applies to non-supported firms. The light grey boxes in the figure represent what is not directly observable through measurement. The arrows indicate comparisons and how they relate to measuring impacts.
11.85. The main challenge in constructing valid counterfactuals is that the potential effect of policy support is likely to be related to choices made in assigning support to some firms and not to others. For example, some programme managers may have incentives to select businesses that would have performed well even in the absence of support, and businesses themselves have incentives to apply according to their potential to benefit from policy support after taking into account potential costs.
11.86. The diagonal arrow in Figure 11.2 shows which empirical comparisons are possible and how they do not necessarily represent causal effects or impacts when the treated and non-treated groups differ from each other in ways that relate to the outcomes (i.e. a failure to control for confounding variables).
Figure 11.2. The innovation policy evaluation problem to identifying causal effects

Source: Based on Rubin (1974), “Estimating causal effects of treatments in randomized and nonrandomized studies”.
Data requirements and randomisation
11.87. Policy evaluation requires linking data on the innovation performance of firms with data on their exposure to a policy treatment. Innovation surveys usually collect insufficient information for this purpose on the use of innovation policies by firms. An alternative (see Chapter 7) is to link innovation survey data at the firm level with administrative data, such as government procurement and regulatory databases, or data on firms that neither applied for nor obtained policy support. The same applies to data on whether firms were subject to a specific regulatory regime. The quality of the resulting microdata will depend on the completeness of data on policy “exposure” (e.g. are data only available for some types of policy support and not others?) and the accuracy of the matching method.
11.88. Experiments that randomly assign participants to a treatment or control group provide the most accurate and reliable information on the impact of innovation policies (Nesta, 2016). Programme impact is estimated by comparing the behaviour and outcomes of the two groups, using outcome data collected from a dedicated survey or other sources (Edovald and Firpo, 2016).
11.89. Randomisation eliminates selection bias, so that both groups are comparable and any differences between them are the result of the intervention. Randomised trials are sometimes viewed as politically unfeasible because potential beneficiaries are excluded from treatment, at least temporarily. However, randomisation can often be justified on the basis of its potential for policy learning when uncertainty is largest. Furthermore, a selection procedure is required in the presence of budgetary resource limitations that prevent all firms from benefiting from innovation support.
Policy evaluation without randomisation
11.90. In ex ante or ex post non-randomisation evaluation exercises, it is important to account for the possibility that observed correlations between policy treatment and innovation performance could be due to confounding by unobserved factors that influence both. This can be a serious issue for evaluations of discretionary policies where firms must apply for support. This requires a double selection process whereby the firm self-selects to submit an application, and programme administrators then make a decision on whether to fund the applicant. This second selection can be influenced by policy criteria to support applicants with the highest probability of success, which could create a bias in favour of previously successful applicants. Both types of selection create a challenge for accurately identifying the additionality of public support for innovation. To address selection issues, it is necessary to gather information on the potential eligibility of business enterprises that apply for and do not receive funding, apply for and receive funding, and for a control group of non-applicants.
11.91. Comprehensive data on the policy of interest and how it has been implemented are also useful for evaluation. This includes information on the assessment rating for each application, which can be used to evaluate the effect of variations in application quality on outcomes. Changes in eligibility requirements over time and across firms provide a potentially useful source of exogenous variation.
11.92. The available microdata for policy use is often limited to firms that participated in government programmes. In this case it is necessary to construct a control group of non-applicants using other data sources. Innovation survey data can also help identify counterfactuals. Administrative data can be used to identify firms that apply for and ultimately benefit from different types of government programmes to support innovation and other activities (see subsection 7.5.2). The regression, matching and structural estimation methods discussed above can all be applied in this policy analysis and evaluation context.
Procedures
11.93. With few exceptions, NSOs rarely have a mandate to conduct policy evaluations. However, it is widely accepted that their infrastructures can greatly facilitate such work in conditions that do not contravene the confidentiality obligations to businesses reporting data for statistical purposes. Evaluations are usually left to academics, researchers or consultants with experience in causal analysis as well as the independence to make critical comments on public policy issues. This requires providing researchers with access to microdata under sufficiently secure conditions (see subsection 9.8.2). There have been considerable advances to minimise the burden associated with secure access to microdata for analysis. Of note, international organisations such as the Inter-American Development Bank have contributed to comparative analysis by requiring the development of adequate and accessible microdata as a condition of funding for an innovation (or related) survey.
11.94. Government agencies that commission policy evaluations using innovation and other related survey data require basic capabilities in evaluation methodologies in order to scrutinise and assess the methodologies used by contractors or researchers and to interpret and communicate the results. Replicability is an important requirement for ensuring quality, and the programming code used for statistical analysis should thus be included as one of the evaluation’s deliverables. Linked databases that are created for publicly funded evaluation studies should also be safely stored and made available to other researchers after a reasonable time lapse, as long as they do not include confidential data.
11.5.4. Co-ordinated analysis of innovation microdata across countries
11.95. When non-discretionary policies are implemented at the national level, it can be very difficult to identify appropriate control groups. For example, all firms within a country are subject to identical competition regulations. A solution is to use innovation data from across countries with different policy environments.
11.96. The main constraint for cross-country policy evaluation is access to microdata for all the countries included in the analysis. Microdata access is essential for accounting for a large number of business and contextual features and for testing counterfactuals. Microdata can be combined with data at the macro level to control for differences by country.
Analysis with pooled microdata
11.97. The optimal solution is to include microdata from multiple countries in a single database. This minimises differences in data manipulation and provides researchers with access to the full sample. This is a requirement for the estimation of multi-level models with combined micro- and country-level effects. An example is a model that analyses innovation performance as a function of business characteristics and national policies.
11.98. The construction of a single database for microdata from multiple countries is constrained by regulations governing data collection and access. National legislation to protect confidentiality can bar non-nationals from accessing data or the use of data abroad. However, legally compliant solutions have been found when there is consensus on the importance of co-ordinated international analysis. An example is the European Commission’s legislative arrangements to provide access to approved researchers to the CIS microdata at Eurostat’s Safe Centre for agreed research projects. This resource for pooled data from different countries has made a substantial contribution to international comparative analysis, although at present it is not possible to link the Safe Centre CIS data to other data.
Distributed, multi-country microdata analysis
11.99. When microdata cannot be remotely accessed or combined in a single database for confidentiality or other reasons, other methods can be used by focussing on the non-confidential outputs. The distributed approach to microdata analysis involves, in first instance, the design and implementation of a common data analysis programming code by individuals with access to their national microdata. The code is designed to return non-confidential outputs such as descriptive indicators or coefficients from multivariate analyses that are as similar as possible across countries. The data can then be compared and further analysed by the collective of individuals involved in the project or by authorised third parties.
11.100. The use of distributed methods for the analysis of innovation began as researcher-led initiatives involving a limited group of countries (Griffith et al., 2006). Since then, the distributed approach has been increasingly adopted for comparative analysis by international organisations such as the OECD (OECD, 2009b). In addition, national teams can produce estimates of parameters for use in further comparative analysis (Criscuolo, 2009), adopting tools similar to those used in quantitative meta-analysis.
11.101. One possible application of a distributed approach to microdata analysis is the construction of a multi-country micro-moments database (MMD) that includes a set of statistical indicators, drawn from national microdata, and captures attributes of the joint distribution of variables within each country. The database comprises a number M of m-moments corresponding to different multivariate statistics, where the moments have been estimated within each country for each combination of business group g (e.g. size and industry) and for each period t. The pooled MMD database for the group of participating countries enables not only tabulations of indicators but also meso- and macro-level analysis to which additional policy and other variables can be added. The ability to build a MMD depends on the comparability of the underlying data and the use of identical protocols to construct the national MMD components (Bartelsman, Hagsten and Polder, 2017).
11.6. Conclusions
11.102. This chapter has reviewed a number of issues relating to the use of innovation data for constructing indicators as well as in statistical and econometric analysis. The recommendations in this chapter are aimed not only at those producing indicators in an official capacity, but also at other interested users of innovation data. The chapter seeks to guide the work of those involved in the design, production and use of innovation indicators. It also contributes to address a broader range of user evidence needs that cannot be met by indicators alone. The chapter has thus described methods for analysing innovation data, with a focus on assessing the impacts of innovation and the empirical evaluation of government innovation policies. It is intended to guide existing data collection and analysis, as well as to encourage future experimentation which will enhance the quality, visibility, and usefulness of data and indicators derived from innovation surveys, a key objective of this manual.
References
Aghion, P. et al. (2005), “Competition and innovation: An inverted-U relationship”, The Quarterly Journal of Economics, Vol. 120/2, pp. 701-728.
Arundel, A. and H. Hollanders (2008), “Innovation scoreboards: Indicators and policy use” in Innovation Policy in Europe: Measurement and Strategy, Edward Elgar, Cheltenham, pp. 29-52.
Arundel, A. and H. Hollanders (2005), “EXIS: An Exploratory Approach to Innovation Scoreboards”, European Trend Chart on Innovation, DG Enterprise, European Commission, Brussels, http://digitalarchive.maastrichtuniversity.nl/fedora/get/guid:25cbd28f-efcf-4850-a43c-ab25393fcca7/ASSET1 (accessed on 9 August 2018).
Bartelsman, E.J., E. Hagsten and M. Polder (2017), “Micro Moments Database for cross-country analysis of ICT, innovation, and economic outcomes”, Tinbergen Institute Discussion Papers, No. 2017-003/VI, http://dx.doi.org/10.2139/ssrn.2898860.
Bloch, C. and V. López-Bassols (2009), “Innovation indicators”, in Innovation in Firms: A Microeconomic Perspective, OECD Publishing, Paris, https://doi.org/10.1787/9789264056213-en.
Bloom, N. and J. Van Reenen (2007), “Measuring and explaining management practices across countries”, The Quarterly Journal of Economics, Vol. 122/4, pp. 1351-1408.
Blundell, R., R. Griffith and J. Van Reenen (1999), “Market share, market value and innovation in a panel of British manufacturing firms”, The Review of Economic Studies, Vol. 66/3, pp. 529-554.
Crépon, B., E. Duguet and J. Mairesse (1998), “Research, innovation and productivity: An econometric analysis at the firm level”, Economics of Innovation and New Technology, Vol. 7/2, pp. 115-158.
Crespi, G. and P. Zuñiga (2010), “Innovation and productivity: Evidence from six Latin American countries”, IDB Working Papers, No. IDB-WP-218, Inter-American Development Bank, Washington DC.
Criscuolo, C. (2009), “Innovation and productivity: Estimating the core model across 18 countries”, in Innovation in Firms: A Microeconomic Perspective, OECD Publishing, Paris, https://doi.org/10.1787/9789264056213-en.
Czarnitzki, D., P. Hanel and J.M. Rosa (2011), “Evaluating the impact of R&D tax credits on innovation: A microeconometric study on Canadian firms”, Research Policy, Vol. 40/2, pp. 217-229.
de Jong, J.P.J. and O. Marsili (2006), “The fruit flies of innovations: A taxonomy of innovative small firms”, Research Policy, Vol.35/2, pp. 213-229.
EC (2010), Elements for the Setting-up of Headline Indicators for Innovation in Support of the Europe 2020 Strategy, Report of the High Level Panel on the Measurement of Innovation, DG Research and Innovation, European Commission, Brussels.
Edovald, T. and T. Firpo (2016), “Running randomised controlled trials in innovation, entrepreneurship and growth: An introductory guide”, Innovation Growth Lab, Nesta, London, https://media.nesta.org.uk/documents/a_guide_to_rcts_-_igl_09aKzWa.pdf (accessed on 9 August 2018).
Eurostat (2014), Glossary of Statistical Terms, http://ec.europa.eu/eurostat/statistics-explained/index.php/Glossary:Statistical_indicator (accessed on 9 August 2018).
Frenz, M. and R. Lambert (2012), “Mixed modes of innovation: An empiric approach to capturing firms’ innovation behaviour”, OECD Science, Technology and Industry Working Papers, No. 2012/06, OECD Publishing, Paris, https://doi.org/10.1787/5k8x6l0bp3bp-en.
Galindo-Rueda, F. and V. Millot (2015), “Measuring design and its role in innovation”, OECD Science, Technology and Industry Working Papers, No. 2015/01, OECD Publishing, Paris, https://doi.org/10.1787/5js7p6lj6zq6-en.
Gault, F. (ed.) (2013), Handbook of Innovation Indicators and Measurement, Edward Elgar, Cheltenham.
Geroski, P., S. Machin and J. Van Reenen (1993), “The profitability of innovating firms”, The RAND Journal of Economics, Vol. 24/2, pp. 198-211.
Griffith, R. et al. (2006), “Innovation and productivity across four European countries”, Oxford Review of Economic Policy, Vol. 22/4, pp. 483-498.
Griliches, Z. (1990), “Patent statistics as economic indicators: A survey”, Journal of Economic Literature, Vol. 28/4, pp. 1661-1707.
Hall, B.H. (2011), “Innovation and productivity”, NBER Working Papers, No. 17178, National Bureau of Economic Research (NBER), Cambridge, MA, www.nber.org/papers/w17178.
Harrison, R. et al. (2014), “Does innovation stimulate employment? A firm-level analysis using comparable micro-data from four European countries”, International Journal of Industrial Organization, Vol. 35, pp. 29-43.
Hill, C.T. (2013), “US innovation strategy and policy: An indicators perspective”, in Handbook of Innovation Indicators and Measurement, Edward Elgar, Cheltenham, pp. 333-346.
Hollanders, H. and N. Janz (2013), “Scoreboards and indicator reports”, in Handbook of Innovation Indicators and Measurement, Edward Elgar, Cheltenham, pp. 279-297.
Laursen, K, and A. Salter (2006), “Open for innovation: the role of openness in explaining innovation performance among UK manufacturing firms”, Strategic Management Journal, Vol. 27/2, pp. 131-150.
Lööf, H., J. Mairesse and P. Mohnen (2016), “CDM 20 years after”, CESIS Electronic Working Papers, No. 442, Centre of Excellence for Science and Innovation Studies (CESIS), KTH Royal Institute of Technology, Stockholm, https://static.sys.kth.se/itm/wp/cesis/cesiswp442.pdf.
Mairesse, J. and P. Mohnen (2010), “Using innovation surveys for econometric analysis”, in Handbook of the Economics of Innovation, Vol. 2, Elsevier.
McLaughlin, J.A. and G.B. Jordan (1999), “Logic models: A tool for telling your program’s performance story”, Evaluation and Program Planning, Vol. 22/1, pp. 65-72.
National Research Council (2014), Capturing Change in Science, Technology, and Innovation: Improving Indicators to Inform Policy, National Academies Press, Washington, DC, https://doi.org/10.17226/18606.
Nesta (2016), “Experimental innovation and growth policy: Why do we need it?”, Innovation Growth Lab, Nesta, London, https://media.nesta.org.uk/documents/experimental_innovation_and_growth_policy_why_do_we_need_it.pdf (accessed on 9 August 2018).
OECD (2015), Frascati Manual 2015: Guidelines for Collecting and Reporting Data on Research and Experimental Development, The Measurement of Scientific, Technological and Innovation Activities, OECD Publishing, Paris, http://oe.cd/frascati.
OECD (2013), “Knowledge networks and markets”, OECD Science, Technology and Industry Policy Papers, No. 7, OECD Publishing, Paris, https://doi.org/10.1787/5k44wzw9q5zv-en.
OECD (2010), Measuring Innovation: A New Perspective, OECD Publishing, Paris, https://doi.org/10.1787/9789264059474-en.
OECD (2009a), OECD Patent Statistics Manual, OECD Publishing, Paris, https://doi.org/10.1787/9789264056442-en.
OECD (2009b), Innovation in Firms: A Microeconomic Perspective, OECD Publishing, Paris, https://doi.org/10.1787/9789264056213-en.
OECD/JRC (2008), Handbook on Constructing Composite Indicators - Methodology and User Guide, OECD Publishing, Paris, www.oecd.org/sdd/42495745.pdf.
OECD and SCImago Research Group (CSIC) (2016), Compendium of Bibliometric Science Indicators, OECD, Paris, www.oecd.org/sti/inno/Bibliometrics-Compendium.pdf.
Rubin, D.B. (1974), “Estimating causal effects of treatments in randomized and nonrandomized studies”, Journal of Educational Psychology, Vol. 66/5, pp. 688-701.
Tether, B. (2001), “Identifying innovation, innovators, and innovation behaviours: A critical assessment of the Community Innovation Survey (CIS)”, CRIC Discussion Papers, No. 48, Centre for Research on Innovation and Competition, University of Manchester, Manchester.
Todd, P.E. (2010), “Matching estimators”, in Microeconometrics, The New Palgrave Economics Collection, Palgrave Macmillan, London, pp. 108-121.
UN (2004), Implementation of the Fundamental Principles of Official Statistics; Report of the Secretary-General, E/CN.3/2004/21, UN Statistical Commission, New York, https://unstats.un.org/unsd/statcom/doc04/2004-21e.pdf.
UNECE (2000), “Terminology on statistical metadata”, Statistical Standards and Studies, No. 53, Conference of European Statisticians, UN Statistical Commission and UN Economic Commission for Europe, Geneva, www.unece.org/fileadmin/DAM/stats/publications/53metadaterminology.pdf.
Wilhelmsen, L. (2012), “A question of context: Assessing the impact of a separate innovation survey and of response rate on the measurement of innovation activity in Norway”, Documents, No. 51/2012, Statistics Norway, Oslo, www.ssb.no/a/english/publikasjoner/pdf/doc_201251_en/doc_201251_en.pdf.