COVID-19 has put pressure on regional labour markets in Australia, as it has around the world. The number of employed people dropped by almost a million between March and May 2020. Employment started to rebound in June 2020 and total employment across Australia recovered to pre-pandemic levels by February 2021. COVID-19 is also likely to accelerate the adoption of automation, as firms seek to increase their productivity. The risk of automation varies at the local level in Australia, with some regions more at risk than others. This chapter looks at how automation could impact people and places across Australia, with a special focus on the regions of Sydney-South West (New South Wales) and Warrnambool and South West (Victoria).
Preparing for the Future of Work Across Australia
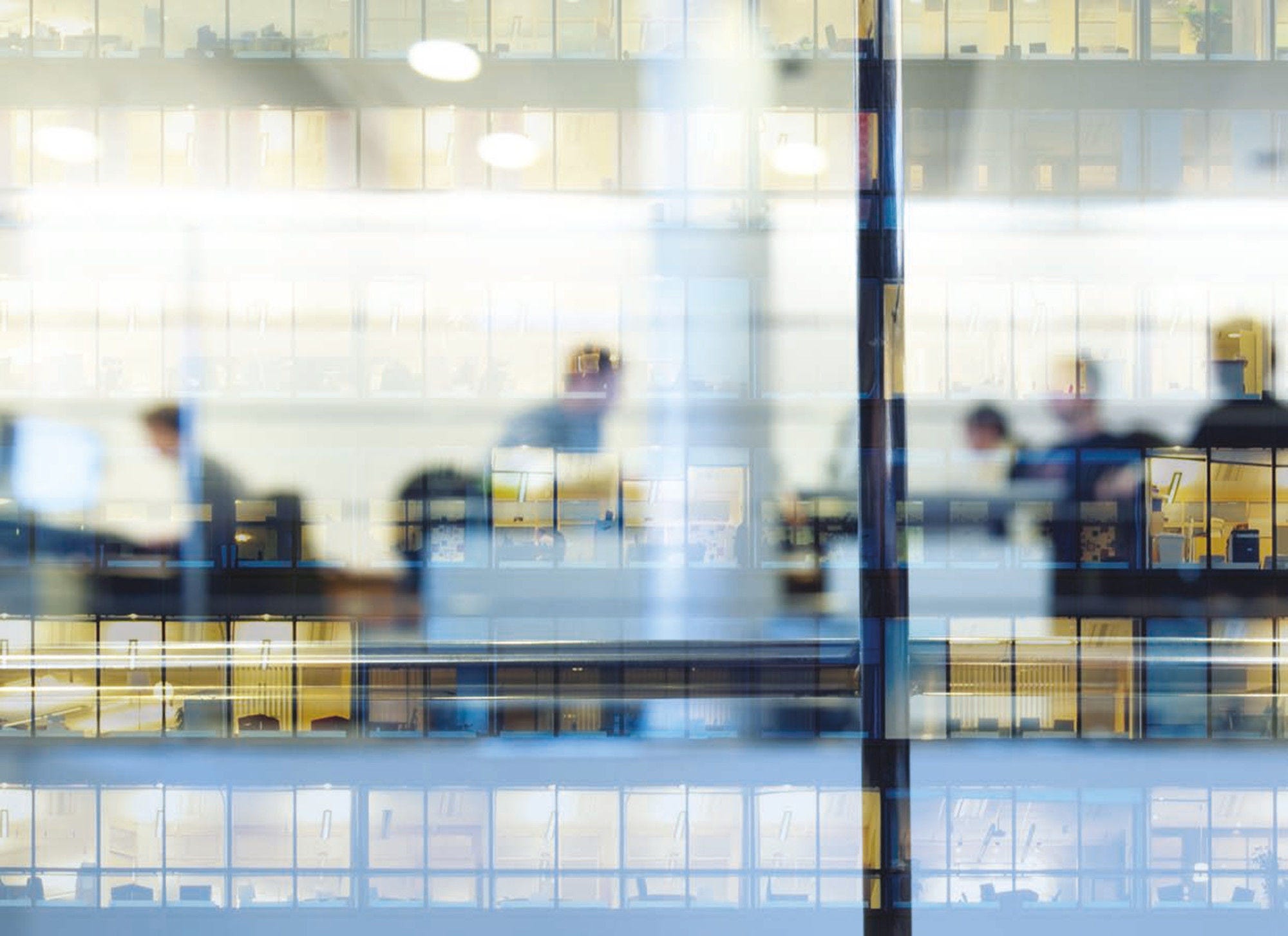
2. The local impacts of automation in Australia
Abstract
In Brief
COVID-19 has hit labour markets in Australia as around the world. The number of employed people in Australia dropped by almost a million between March and May 2020. A gradual recovery started in June and continued in the following months. In February 2021, total employment in Australia reached its pre-pandemic level at 13 million. The participation rate, reflecting the share of working age population participating in the labour market, has increased after a drop in the immediate aftermath of the pandemic. As of June 2021, unemployment rates dropped below pre-pandemic levels across most states and territories, partly reflecting improving participation rates as more and more people re-enter the labour market in Australia, either with a job or looking for one.
The risk of automation varies at the local level in Australia, with COVID-19 likely to accelerate automation in the workplace. Australia overall faces a lower risk of losing jobs to automation than on average across the OECD (about 36% vs. 46%). However, regional differences in the risk of automation exist within all Australian states, with some showing higher regional variation than others. For example, in Queensland, the risk of automation varies from 41.2% in Mackay - Isaac - Whitsunday to 27.7% in Brisbane Inner City.
The drivers of the risk of automation depend on the occupational profile of local labour markets. In rural regions, the risk of losing jobs to automation relates to the prevalence of low-skilled occupations in Agriculture. This is the case of South Australia-South East (South Australia), where 40.3% of jobs are at risk. In some regions, the risk is driven by occupations which are common to the Mining sector, such as in Mackay-Isaac-Whitsunday (Queensland). Regions at risk of automation also tend to have high employment shares in Manufacturing. Other regions are characterised by the prevalence of jobs in occupations at risk across sectors.
Sydney-South West (New South Wales) and Warrnambool and South West (Victoria) are examples of two regions at high risk for different reasons. Sydney-South West is characterised by a diversified local economy, with the high risk of automation deriving from high employment shares in a range of occupations at high risk. In Warrnambool and South West, Agriculture is the largest employer and drives the risk of automation. Both regions have shifted towards occupations at lower-risk of automation between 2006 and 2016.
Introduction
Megatrends related to digitalisation, automation and artificial intelligence (AI) are having a profound impact on local labour markets across the OECD. Some people and places are more at risk than others. The COVID-19 pandemic is posing unprecedented challenges to local labour markets in Australia, and accelerating a pre-existing trend towards automation. This chapter is structured as follows. Section 2.1 provides an overview of local labour market trends in Australia. Sections 2.2 and 2.3 analyse the impact of automation at the local level in Australia. Section 2.4 dives into the characteristics and impacts of automaton in two case study regions: Sydney-South West (New South Wales) and Warrnambool and South West (Victoria).
2.1. COVID-19 is posing unprecedented challenges to local labour markets, but Australia remains resilient
2.1.1. COVID-19 hit some places harder than others
The first COVID-19 case was confirmed in Australia in late January 2020. As of August 2021, the total number of COVID-19 confirmed cases in Australia stands at about 49 000, with the highest numbers being reported in New South Wales (22 000) and in Victoria (21 000). Since the start of the pandemic, states and territories in Australia have alternated periods of tightening and easing of restrictions, mirroring the changing spread of the virus at the local level. The number of new cases initially grew sharply in Australia, then levelled out at about 350 per day and started falling at the beginning of April 2020 to under 20 cases per day by the end of the month. A second wave of infections in Victoria commenced in late June 2020, leading to new localised lockdowns and the declaration of state of disaster in Victoria. In mid-September, Victoria introduced a roadmap for reducing restrictions and, at the end of October, the state recorded zero new COVID-19 cases and deaths for the first time since June 2020. In November and December 2020, local COVID-19 outbreaks were identified in South Australia and New South Wales, leading to the re-imposition of localised restrictions. Localised restrictions in response to local outbreaks were introduced in Western Australia and Victoria in January and February 2021. COVID-19 hit Australia immediately after its worst bushfire season, which ravaged homes, businesses, and entire towns across the country between 2019 and 2020.
The pandemic had an immediate impact on the Australian labour market, hitting vulnerable segments of the population more than others. Employment fell by over 857 000 jobs from March to May 2020. Data from the National Skills Commission (NSC) shows that young people were greatly impacted by COVID-19, with youth employment declining by 107 400 in May 2020 following a record decrease of 214 500 in April 2020. Young people accounted for about 45% of the total decline in employment in May, despite representing only 16% of the population. Since then, youth employment has rebounded somewhat to 1.9 million in June 2021, which is still slightly lower than the level recorded in March 2020. There was also a decrease in female employment, falling by 143 700 jobs in May following a fall of 327 700 in April (this being the largest monthly decline on record) (National Skills Commission, 2020[1]). Initially, COVID-19 had a disproportionate impact on women, due to their over-representation in industries that were most severely affected, such as accommodation and food services, and the fact that they were more likely to be employed on a casual basis, where job losses have been greater (National Skills Commission, 2020[2]). During COVID-19, many workers have experienced a decline in working hours, which fell by a record 163.9 million hours between March and April 2020. The decline in working hours also disproportionately affected working women (Australian Bureau of Statistics, 2020[3]). By February 2021, female employment increased to 6.2 million, which is above the level seen just before the pandemic.
A report released by the Australian Government National Skills Commission showed the immediate impacts of COVID-19 on business activity and confidence. Results of a survey of more than 250 businesses undertaken between 27 March and 3 April 2020 showed that the COVID-19 crisis affected the majority of businesses with the demand for their goods and services and cash flow considerably reduced. Consequently, many businesses reported reducing staff hours or letting staff go. Some 58% of businesses reported that COVID-19 had affected their business ‘a great deal’ with another 22% reporting it affected their business ‘somewhat’. The most commonly reported impacts of the COVID-19 pandemic were a lower demand for goods and services (67% of impacted businesses), cash flow issues (37%) and having to change business practices (33%) (Australian Government Department of Education, Skills and Employment, 2020[4]).
COVID-19 had different impacts at the local level in Australia throughout 2020. Some jurisdictions were more severely affected than others due to a range of factors, including ongoing COVID-19 cases, each jurisdiction’s industry composition, demographics (population size and age structure) and differences in the way each state and territory has managed the pandemic (National Skills Commission, 2020[2]). For example, between March and May 2020, the unemployment rate increased by more than 2 percentage points in Western Australia, while it increased by less than 1 percentage point in the Australian Capital Territory. Not surprisingly, reflecting the outbreak of COVID-19 cases in Victoria from late June 2020 and the subsequent restrictions, labour market indicators in Victoria worsened in the following months, with the unemployment rate hitting 7.3% in October 2020. Payroll and wage data, collected through the Weekly Payroll Jobs and Wages in Australia series, also add to the regional variation of labour market conditions in Australia throughout the pandemic. For example, between 1 February and 25 April 2020, Bunbury (WA) was the region experiencing the largest decline in payroll jobs (-9.4%), followed by South East (TAS) (-9.3%) and Gold Coast (QLD) (-9.1%). Payroll jobs returned to their pre-pandemic levels in most regions by the first quarter of 2021.
The jobactive caseload, which includes people participating in the New Employment Services Trial, dramatically increased in the first half of 2020 as a consequence of COVID-19. jobactive and the New Employment Services Trial are employment programs managed by the Australian Government. They are a network of employment service providers operating in 1 600 locations across Australia. Under jobactive and the New Employment Services Trial, job seekers receive assistance to improve their job readiness, and employability skills and employment applications. Service providers match job seekers with suitable work experience placements, including through compulsory activities such as the Work for the Dole program. The development of the new employment services model involved extensive consultation with more than 1 400 stakeholders including job seekers, employment services providers, industry representatives, employers and peak bodies, and independent advice delivered by the Employment Services Expert Advisory Panel in its report to Government. The new model is being trialled in two regions from July 2019 before being rolled out nationally from July 2022. The total jobactive caseload, which includes people participating in the New Employment Services Trial, reached a record high of 1.434 million people in the second quarter of 2020, up from 757 316 in the previous quarter. The caseload continued to increase in the third quarter, standing at 1.488 million, before declining to 1.326 million in the last quarter of 2020. The second quarter 2021 active caseload, however, remained almost double that of fourth quarter 2019 (see Figure 2.1).
From June 2020 onwards, Australia started experiencing gains in total employment, and by March 2021, it was back to pre-pandemic levels (see Figure 2.2). While employment fell by 856 900 between March and May 2020, it increased by 1 million over the following 13 months. Signs of recovery in the labour market also emerged from increasing participation rates and employment rates in Australia. As more people re-entered the labour force, Australia’s unemployment rate initially increased, reaching a peak of 7.4% in July 2020, before starting to decline. As of July 2021, unemployment rates dropped below pre-pandemic levels in all states and territories except the Australian Capital Territory (see Figure 2.3).
Figure 2.1. The total jobactive caseload almost doubled in light of COVID-19 and is now steadily declining

Source: jobactive Caseload Data, Australia Labour Market Information Portal.
Figure 2.2. Total employment in Australia started growing in June 2020 and recovered to pre-pandemic levels by February 2021

Source: Australian Bureau of Statistics (ABS), Labour Force Survey, July 2021.
Figure 2.3. Unemployment rates recovered to pre-pandemic levels across most Australian states and territories

Source: Australian Bureau of Statistics (ABS), Labour Force Survey, July 2021
Box 2.1. Government support for businesses, employees and job seekers during COVID-19
The Australian Government has announced a suite of measures to support businesses, employees and job seekers during the COVID-19 pandemic. As an example, the JobKeeper Payment scheme was introduced to provide a temporary subsidy for businesses significantly affected by COVID-19. Eligible employers, sole traders and other entities can apply to receive AUD 1 500 per eligible employee per fortnight. On 21 July, the government announced proposed changes to JobKeeper including an extension through to 28 March 2021. From 28 September 2020, eligibility for the JobKeeper Payment was based on actual turnover in the relevant periods. The payment has also been stepped down and is paid at two rates. Further changes were announced on 7 August 2020 to adjust the reference date for employee eligibility and make it easier for organisations to qualify for the JobKeeper Payment extension from 28 September 2020.
In addition, temporary cash flow payments up to AUD 100 000 have been made available to keep eligible small to medium-sized businesses operating, paying bills and retain staff. Under the Coronavirus SME Guarantee Scheme, the government is providing a guarantee of 50% to SME lenders to support new short-term unsecured loans to SMEs. This aims to provide businesses with funding to meet cash flow needs, by further enhancing lenders’ willingness and ability to provide credit. A safety net will apply to lessen the threat of actions that could unnecessarily push businesses to being wound up or forced into insolvency or bankruptcy during this time. Finally, the instant asset write-off threshold has been increased from AUD 30 000 to AUD 150 000 for businesses with an aggregated annual turnover of less than AUD 500 million, with the objective of supporting business purchase of new equipment. This applies from 12 March 2020 until 30 June 2021, providing the asset is purchased by 31 December 2020.
As the economy recovers from COVID-19, the Australian Government has introduced other measures to support skills development and job creation. As an example, in July 2020, the Australian Government announced the establishment of a new AUD 1 billion JobTrainer fund to give hundreds of thousands of Australians access to new skills by retraining and upskilling them into sectors with job opportunities. In partnership with the states and territories, more Australians will have access to free, or low cost, training places in areas of identified skills. This includes AUD 500 million from the Australian Government in 2020, with a matched contribution being sought from state and territory governments. The JobTrainer fund will provide for around 320 000 additional training places to help school leavers and job seekers gain the skills they need to get a job.
Going forward, the economic consequences of the pandemic are likely to affect some sectors more than others, with tourist destinations and large cities particularly affected. Across the OECD, regions have different sectoral specialisations, exposure to global value chains, focus on tradable vs. non-tradable sectors, and shares of non-standard employment. Consequently, some regions are likely to suffer more than others, facing a steeper economic recession and larger shares of jobs at risk. OECD estimates show that the risk is high in most Australian states, while it is slightly lower in the Northern Territory. The estimates refer to activities directly affected by the shutdowns, but a significant share of those may still be at risk even after the pandemic is under control (OECD, 2020[7]). The need to quickly reallocate workers from shrinking to expanding industries is a more important aspect of the current episode than other downturns (Borland, 2020[8]).
COVID-19 has led to a substantial shift towards teleworking in Australia as across the OECD, but the ability to perform jobs remotely varies at the local level. Teleworking –“work-from-home” or “home-office” – has been a necessary practice for many firms and workers during the lockdown period of the COVID-19 crisis. Communities have undergone a large scale “forced experiment” where sectors, firms and workers have continued to operate while being physically separated, provided they had the necessary technological, legal and digital security conditions (OECD, 2020[9]). The potential of teleworking varies greatly both between and within countries, depending on the occupational profile of local labour markets (OECD, 2021[10]). Across the OECD, the possibility of remote working correlates strongly with the skills requirement of the occupation. As a result, rates of potential remote working across regions reflect the skill composition of the local workforce. Other factors also come into play, such as the industrial composition of local economies (OECD, 2020[11]). Looking at Australia, OECD work shows that the Australian Capital Territory has the highest share of jobs that can potentially be performed remotely (56.2%), followed by Victoria (49.9%) and New South Wales (47.6%). On the other hand, only 36.1% of jobs can be performed remotely in South Australia (OECD, forth.) (see Figure 2.4). Even though a job may be amenable to teleworking, the worker, in all likelihood, must still have adequate access to a sufficiently fast internet service. If not, this creates a digital divide between urban and rural areas. Ensuring access to high-speed broadband in rural areas could help mitigate this divide (OECD, 2021[12]).
Figure 2.4. COVID-19 has highlighted spatial differences in the ability to perform remote working
Percentage of jobs amenable to remote working, Australian states and territories
2.1.2. Local labour markets across Australia had experienced diverging trends before the pandemic
Before COVID-19, the Australian labour market witnessed positive developments, but the unemployment rate remained higher than the pre-Global Financial Crisis (GFC) level. OECD data shows that the labour force participation rate, accounting for labour force participants as a share of the working age (15-64 year -old) population, has been above the OECD average over the past decade, and amounted to 78.5% in 2019, compared to the OECD average of 72.8%. Total employment in Australia was 20% higher in 2019 than it was in 2008, compared to the OECD average increase of 10%, However, like many other OECD countries, Australia’s unemployment rate in 2019 remained higher (5.2%) than before the 2008 GFC (4.2%).
The unemployment rate had increased in all Australian states and territories between 2008 and 2019, except for New South Wales (see Figure 2.5). Increases in the unemployment rate have coincided with improving labour force participation in Australia between 2008 and 2019. The labour force participation rate among the working age population (15-64 year old) increased from 76.5% to around 78.5% over that period. On average during 2019, the monthly unemployment rate stood at 6.3% in Tasmania and South Australia, while it was 3.4% in the Australian Capital Territory. Between 2008 and 2019, the unemployment rate increased in all Australian States except for New South Wales, where it decreased by about 0.3 percentage points. The increase in the unemployment rate was largest in Western Australia and Queensland, where it increased by 2.8 and 2.5 percentage points, from 3.1% to 5.9% and from 3.7% to about 6.1% respectively between 2008 and 2019. Over the last few decades, some states were consistently strong performers while others continued to face challenges in terms of high unemployment rates (OECD, 2019[14]). The large increase in the unemployment rate in Western Australia and Queensland was partly due to the ending of the Mining boom and its transition from an investment to production phase. The net-zero carbon transition could lead to more job losses in sectors such as mining, which may exacerbate existing regional labour market disparities (OECD, 2021[12]).
Regions within states and territories have experienced diverging trends, which reflect place-specific labour market dynamics (see Figure 2.6). For example, looking at New South Wales, the unemployment rate in 2019 was highest in Southern Highlands and Shoalhaven, standing at 8.5%, while it was lowest in Sydney – Sutherland, averaging 2.3% in the same year. Southern Highlands and Shoalhaven is also one of the few regions within New South Wales having witnessed an increase in unemployment between 2008 and 2019, by about 2.3 percentage points. Similar dynamics have taken place in other Australian states and territories, suggesting the importance of local labour market dynamics. As an example, in Victoria the unemployment rate was highest in Melbourne - North West, standing at 6.2% in 2019, and this is also the region that experienced the sharpest increase in the unemployment rate between 2008 and 2019 (+2.6 percentage points). On the other hand, Bendigo has among the lowest unemployment rates in Victoria and the rate has decreased by 3.7 percentage points between 2008 and 2019.
Figure 2.5. Before COVID-19, the unemployment rate increased in all Australian states and territories except for New South Wales between 2008 and 2019
Annual unemployment rates, Australian States and Territories, 2008 and 2019

Note: Unemployment rates for 2008 and 2019 are averages of 12-month rates.
Source: Australia Bureau of Statistics.
Figure 2.6. Many Australian regions had not recovered in 2019 to pre-2008 unemployment rate levels
Share of regions within each State or Territory, with higher unemployment rates in 2019 and 2008

Note: The numbers in each column represent the number of SA4 regions within each State and Territory. Data does not include the Australian Capital Territory.
Source: OECD elaboration on Australian Bureau of Statistics Labour Force Surveys.
2.1.3. Prior to COVID-19, job creation had been driven by capital city regions
Most Australian states and territories have experienced employment growth in recent years (see Figure 2.7). Victoria, New South Wales, and Australian Capital Territory experienced the largest growth, with total employment growing by 16.7%, 14.1%, and 11.2% between February 2015 and 2020 (or 490 400, 511 800, and 23 600 jobs respectively). Total employment has grown more moderately in other states and territories. Employment growth was negative only in the Northern Territory (-1.7%).
Employment dynamics however vary at the regional level, even within states and territories that have created jobs over the past years. For example, in New South Wales, Sydney-South West is the region that has experienced the largest employment growth over the past five years, adding about 56 000 jobs. This has been driven by sectors such as Retail Trade, Health Care and Social Assistance, Construction and Professional, Scientific and Technical Services. On the other hand, Coffs Harbour-Grafton has witnessed the sharpest job loss in New South Wales (-3 000 jobs), driven by losses in Agriculture, Manufacturing and Transport, Postal and Warehousing. Structural change is a driver of employment trends in regions, as regions dominated by services, especially knowledge-intensive ones, are likely to witness employment growth, while employment growth is likely to be lower and waning in regions dominated by goods-based industries (which are on a long term declining trend in Australia). In addition, employment trends have also been influenced by population trends. Sydney-South West is the region in New South Wales that has experienced the second largest population growth between 2015 and 2020 (13% or about 50 000 new residents). On the other hand, in Coffs Harbour-Grafton, population residents increased by only 3%, or 4 000 people, over the same period.
Job creation has been mainly driven by capital city regions within each state and territory (see Figure 2.8). This can be explained by the fact that regions closer to capital cities have stronger access to skills, finance and capital, as well as better infrastructure and can easily capitalise on agglomeration benefits. In Tasmania, Hobart accounts for 78.0% of net job creation (or 11 000 jobs) between 2015 and 2020. Similarly, in Western Australia, Perth-North West and Perth-South West account for 47.7% and 34.6% of net job creation over the same period, corresponding to about 19 400 and 14 100 jobs respectively. In South Australia, Adelaide-South and Adelaide-North created 37.5% and 31.6% of state employment (about 19 200 and 16 200 jobs).
Figure 2.7. Number of jobs increased in most states and territories over the five-year period leading up to the COVID-19 pandemic
Total employment change, February 2015 to February 2020

Source: Australian Bureau of Statistics Labour Force Survey, four-quarter average.
Figure 2.8. Capital city regions have driven job creation in each state and territory
SA4 region within each state accounting for the largest share of job creation between February 2015 and 2020

Source: Australian Bureau of Statistics Labour Force Survey, four-quarter average.
2.1.4. The sectoral composition of the Australian labour market has changed over the past decades, but regional differences persist
As in all OECD economies, the sectoral composition of the economy has shifted dramatically over the long term, away from goods production and towards services (OECD, 2018[15]). With higher incomes, consumers typically spend a greater share of their income on services compared to goods, and changing consumer preferences and population ageing have also shaped demand. The shift to services - in particular business services – also reflects shifts in how goods are produced. Supply chains have lengthened with goods producing industries becoming more focused on core activities and outsourcing non-core activities, and production has become more fragmented across countries with the rise of global value chains (GVCs) (Adeney, 2018[16]) (Timmer et al., 2014[17]). As a consequence of falling international trade costs and the entrance of emerging markets such as China, a greater share of the production stages of supply chains now occurs overseas (Kelly and La Cava, 2014[18]). In addition to the progressive opening up of the economy to international competition, the decline in Manufacturing jobs has also been due to technological development (Garnett, 2018[19]). In Australia, the end of the Mining boom has been coupled with an ongoing decline in the Manufacturing industry, while the Construction and Services sectors have grown (OECD, 2018[20]).
The share of manufacturing employment in Australia has dropped over the past decades. It accounted for about 12.1% of total employment in Australia in the early 2000. The share of manufacturing employment dropped to 9.0% in 2010 and 7.1% in 2020 (see Figure 2.9). More than a million people were employed in Manufacturing in 2000, while about 923 000 were employed in this sector in February 2020, prior to the pandemic based on trend data from the Australian Bureau of Statistics Labour Force Survey. The contraction in Manufacturing employment may be attributed to a variety of factors, including the persistently high Australian dollar, increased competition from low cost countries such as China and India, and a switch to more capital intensive production processes (Georgeson and Harrison, 2015[21]), as well as high energy costs. Similarly, employment in Agriculture, Forestry and Fishing has halved since 2000, when it amounted to about 4.9%. In 2020, Agriculture employment stands at 2.5% of total employment in Australia. Australia envisions revitalizing its manufacturing base through its Modern Manufacturing Strategy to help manufacturing scale-up, become more competitive and resilient. The strategy involves getting the economic conditions right for business, making science and technology work for industry, focusing on areas of advantage, and building national resilience for a strong economy.
Health Care and Social Assistance has emerged as the largest sector, while other services sectors have also increased their share of total employment. These trends are broadly consistent with what seen in other high-income economies over recent decades. Health Care and Social Assistance accounted for 13.8% of total employment in February 2020, up from 9.3% in 2000 and 10.9% in 2010. Professional, scientific and technical services and education and training today employ 8.9% and 8.6% of total workers respectively, up from 6.5% and 7.1% in 2000. The relative importance of the construction sector has also grown, as it today employs 9.1% of total workers, up from 7.8% in 2000. Health care and social Assistance is also the largest sector by employment in all Australian states and territories, except for the Australian Capital Territory, where the largest majority of workers are employed in public administration. Over the same period, Education and Training and Professional, Scientific and Technical Services added 196 000 and 184 100 jobs respectively. Construction was also a sector that experienced employment growth, adding more than 160 000 jobs.
However, Manufacturing still represents an important source of employment in many regions across Australia, and it has been growing over recent years. For example, it accounts for 12.5% in Shepparton (Victoria) and 12.4% in Melbourne-South East (Victoria), which have added about 1 300 and 2 300 Manufacturing jobs over the past five years. Richmond-Tweed (New South Wales), where Manufacturing employment amounts to about 9.7% of total employment, has experienced the strongest Manufacturing job creation over the past five years, as Manufacturing employment has increased by 7 100. Melbourne-South East accounts for about 4% of total Manufacturing employment in Australia, while the other three regions mentioned above account for 1% each. However, the net-zero carbon transition may lead to a number of manufacturing job losses which may contribute to regional labour market disparities (OECD, 2021[12]). Agriculture, Forestry and Fishing continues to represent a key source of employment in some regions. For example, it is as high as 31.2% of total employment in Queensland-Outback (Queensland) and 24.0% in Darling Downs-Maranoa (Queensland), 22.5% in Warrnambool and South West (Victoria) and 22.2% in Western Australia-Wheat Belt (Western Australia). Each of these regions accounts for about 4% of total Australian jobs in agriculture, forestry and fishing. These regions have experienced a net growth in Agriculture employment over the past five years, by 8 900, 9 000, 4 100 and 7 400 respectively.
Mining, accounting for about 1.9% of total employment nationally, is the main source of employment in some regions, which rely on resource extraction. It stands at about 21.5% of total employment (or 9 600 jobs) in Mandurah (Western Australia), 18.1% (or 19 800 jobs) in Western Australia-Outback (North and South) and 17.4% (or 15 600 jobs) in Mackay-Isaac-Whitsunday (Queensland).
Figure 2.9. Manufacturing employment has steadily declined in Australia
Manufacturing employment as a share of total employment, 2000, 2010 and 2020

Source: Australian Bureau of Statistics, Labour Force Survey – Employment by Industry time series.
Figure 2.10. In some regions, Manufacturing still accounts for more than 10% of total employment
Manufacturing employment as a share of total regional employment, selected regions, February 2020

Source: Australian Bureau of Statistics, Labour Force Survey – Employment by Industry time series, four-quarter average.
2.1.5. Labour productivity growth has slowed down, especially in some territories
Australia has a relatively high productivity compared to other OECD countries. Australian labour productivity in 2019 was over USD 55.05 per hour worked. This places Australia as being mid-range across the OECD. Top performing OECD countries include Ireland (USD 102.69 per hour), Luxembourg (94.75) and Norway (84.27). Australian productivity growth between 2001 and 2017 exceeded all G7 economies other than the United States. Capital deepening linked to the Mining boom is considered to be the largest driver of productivity growth in Australia (Australian Government Productivity Commission, 2019[22]). Catch-up to the productivity level of the United States has proven elusive over the past five decades, partly because of Australia’s relative remoteness and low population density have been a barrier to achieving efficient scale in Manufacturing and goods distribution (Australian Government Productivity Commission, 2020[23]).
Productivity has grown over the past decades in Australia, but it has slowed down in recent years. Technology has been a driver of productivity growth for many economies, including Australia, underpinning growth in wages, GDP per capita and well-being, with improvements in health, infrastructure and educational attainment (OECD, 2018[15]). In the 2000s, most developed countries, including Australia, have experienced a slowdown in productivity. Potential drivers of slowdowns include falling rates of innovation, the fading impacts of ICT and past economic reforms, and sectoral shifts to lower productivity sectors (Carmody, 2019[24]). While many of the factors that might explain a global productivity slowdown also apply to Australia, the high reliance on commodity exports in Australia is likely to have counteracted these (Australian Government Productivity Commission, 2019[22]). Australia’s advantage in Mining in particular has enabled it to complement the fast growing Chinese and Indian economies more so than most developed economies, as the higher growth rates in these economies increased demand for Australian iron ore, coal, natural gas and other commodities (ibid.).
Productivity differentials across states and territories within Australia reflect the sectoral composition of local economies. States and territories that rely heavily on utilities and resource extraction, such as Western Australia and the Northern Territory, have naturally higher productivity levels. These are capital-intensive sectors and employ a relatively small share of labour which results in naturally higher labour productivity compared to other industries. On the other hand, productivity is lowest in Tasmania. One reason for the lower productivity in Tasmania is that most of the industries which have intrinsically very high levels of labour productivity – because they are capital intensive, or because they rely heavily on very skilled labour where capital-intensive industries – are under-represented in Tasmania’s economy (Eslake, 2017[25]).
Figure 2.11. Labour productivity is highest in states and territories relying on utilities and resource extraction
Gross value added per worker, thousands, constant prices, constant PPP, base year 2015

Source: Calculations based on OECD Regional statistics database.
2.2. The risk of automation in Australia
2.2.1. Overall, Australia faces a lower risk of losing jobs to automation than on average across the OECD
Megatrends related to digitalisation, automation and the emergence of the gig economy are impacting labour markets across the OECD. Broad evidence supports concerns that automation could cause job displacement, as the tasks that machines are able to perform are increasing rapidly. However, technological development could also contribute to generating more jobs than it destroys within a given industry. By increasing productivity and reducing prices, certain technologies could have a positive impact on employment in industries other than the ones where they are deployed. Automation can also lower input costs for downstream industries, leading to output and employment growth in those industries (OECD, 2019[26]).
COVID-19 could accelerate the pace of automation in the workplace. Automation has historically taken place in bursts, especially around times of economic shocks, when human labour become relatively more expensive as firms’ revenues rapidly decline. In these periods, employers are more likely to shed less-skilled workers and replace them with technology and higher-skilled workers, increasing labour productivity in the face of recessions (Muro, Maxim and Whiton, 2020[27]). More than a quarter of Australian businesses reported an increase in take-up of new technology and automation due to COVID-19, with businesses in the Australian Capital Territory and Victoria reporting more than a 35% increase (see Figure 2.12). The automation of Australia’s industries has been in the works for some time, with Australia being a world leader in adopting mining equipment automation. A potential driver of automation in Australia in light of the pandemic might be a desire to become more self-sufficient in supplying goods and services, with local supply chains that are less susceptible to global shocks. The need to reduce the frequency and duration of human-to-human contact, especially as experts warn of the increasing threat of future pandemics, might be another driver of automation (Roberts, 2020[28]).
Digitalisation and automation have had an overall positive effect on the Australian economy, bringing rises in living standards, but some jobs are at risk. Automation offers the opportunity to increase productivity and competitiveness, helping Australia resume the faster and more widespread income growth of the long boom’s early decades (McKinsey & Company, 2019[29]). However, new technologies traditionally also have a potential for disruption, due to their labour saving effect and their uneven impacts across sectors and segments of the population. This is also the case for automation. New technologies are contributing to changing the supply and demand for products, services, labour and skills, producing a reallocation of economic activity across the globe. Some industries, such as Manufacturing, have therefore been a source of jobs and incomes decline, while others, such as business services, have risen. New technologies and structural change also require new skills and tasks, creating demand for some jobs rather than others (OECD, 2018[15]). Employment growth over the past decades in Australia has been concentrated in highly skilled jobs, which are typically less likely to be automated and could instead benefit from technological change (Heath, 2020[30]). High-skill workers tend to specialise in non-routine tasks that are less likely to be performed by a machine. OECD work finds that the correlation between skill content and routine intensity is indeed negative, i.e. that more routine-intensive occupations tend to require lower level skills, although this correlation is not necessarily very strong. High-skill workers may be more difficult to substitute and can be more easily re-employed in non-routine tasks (Marcolin, Miroudot and Squicciarini, 2016[31]). However, new algorithms in combination with the increasing processing power of computers have made it possible for machines to take care of tasks that until now only humans could do (for example, self-checkouts in stores, packing and moving of goods in warehouses, web-scraping data, automated voice receptionists, etc.). This has raised the spectre that specific functions, which have been impossible to automate until now, could be performed by machines instead of people in the future. This also affects high-skilled jobs that were in the past largely shielded from automation (OECD, 2018[32]).
Australia faces a lower risk of losing jobs to automation than on average across the OECD. This points to an occupational and sectoral structure within Australia’s economy where jobs entailing tasks at risk of automation are less prevalent than in other OECD countries (see Figure 2.13). OECD work finds that about 36% of jobs are at risk in Australia, compared to the OECD average of about 46% (OECD, 2019[26]). The OECD methodology estimates the risk of automation for individual jobs based on the Survey of Adult Skills (PIAAC) and building on the framework developed by Frey and Osborne (2013[33]) (see Box 2.2).
Figure 2.12. More than a quarter of Australian businesses reported an increase in take-up of new technology and automation due to COVID-19 in 2020

Note: The sample consists of 666 businesses of which 24% were from New South Wales, 20% were from Victoria, 19% were from Queensland, 12% were from South Australia, 12% were from Western Australia, 6% were from Tasmania, 4% were from the Australian Capital Territory, and 4% were from the Northern Territory.
Source: OECD survey of Australian firms, 2020.
Figure 2.13. Risk of automation in Australia is lower than the OECD average

Note: High risk of automation corresponds to a likelihood of automation of 70% or more. Jobs at risk of significant change are those with likelihood of automation between 50 and 70%. Data refer to 2012.
Source: OECD (2019[26]).
Box 2.2. Estimating the risk of automation across OECD countries
Frey and Osborne (FO) estimated the number of occupations at high risk of automation in the United States using a two-step methodology. They conducted a workshop with a group of experts in machine learning, whom they provided with a list of 70 occupations and their corresponding O*NET task descriptions. Experts were asked “Can the tasks of this job be sufficiently specified, conditional on the availability of big data, to be performed by state of the art computer-controlled equipment?”. This allowed for the coding of each occupation as automatable or non-automatable. They then used a machine learning algorithm to find out more about the links between the coding to automate and the list of O*NET variables.
FO were able to identify those variables and their associated bottlenecks with higher prediction power. High scores on these bottlenecks are likely to mean that an occupation is safe from automation. They could then compute a ‘probability of computerisation’ for each occupation in the US, leading to the aggregate estimate that 47% of US jobs have a probability of automation of more than 70%.
The FO model also had its critics. It has been argued that this approach overestimated the likely impact of technological change, while the focus should be on the automatability of the discrete tasks that make up an occupation (Arntz, Gregory and Zierahn, 2016[34]).
Table 2.1. Bottlenecks to automation
Computerisation bottleneck |
O*NET variable |
---|---|
Perception and Manipulation |
Finger dexterity Manual dexterity Cramped workspace; awkward positions |
Creative intelligence |
Originality Fine arts |
Social intelligence |
Social perceptiveness Negotiation Persuasion Assisting and caring for others |
Note: Please refer to Frey and Osborne (2013[33]) for further details on the definition of automation bottlenecks.
Source: Frey and Osborne (2013[33]).
Building on and improving the FO model, Nedelkoska and Quintini (2018[35]) (NQ) calculated the risk of automation across 32 OECD countries. The methodology is based on individual-level data from the OECD Survey of Adult Skills (PIAAC), providing information on the skills composition of each person’s job and their skillset. While drawing on FO, this methodology presents four key differences: (i) training data in the NQ model is taken from Canada to exploit the country’s large sample in PIAAC; (ii) O*NET occupational data for FO’s 70 original occupations were manually recoded into the International Standard Classification of Occupations (ISCO); (iii) NQ uses a logistic regression compared to FO’s Gaussian process classifier; (iv) NQ found equivalents in PIAAC to match FO’s bottlenecks (see Table 2.2). While PIAAC includes variables addressing the bottlenecks identified by FO, no perfect match exists for each variable. No question in PIAAC could be identified to account for job elements related to “assisting and caring for others”, related to occupations in health and social services. This implies that risks of automation based on NQ could be slightly overestimated.
Table 2.2. Automation bottleneck correspondence
FO computerisation bottleneck |
PIAAC variable |
---|---|
Perception and manipulation |
Finger dexterity |
Creative intelligence |
Problem solving (simple) Problem solving (complex) |
Social intelligence |
Teaching Advising Planning for others Communication Negotiation Influence Sales |
Note: Please refer to Nedelkoska and Quintini (2018[35]) for further details on the definition of the PIAAC variables.
Source: OECD (2018[32]); Nedelkoska and Quintini (2018[35]).
Recent studies have pointed out the difficulty in predicting the risk of automation, as different models and variables comes into play. Frey and Osborne’s original examination of the impact of automation on jobs was focused on machine learning and mobile robotics, but these are not the only key technological developments likely to impact the future of skills. Others have identified the rise of various forms of telepresence and virtual/augmented/mixed forms of reality, as well as the expansion of digital platforms as trends that will have important impacts on the future. The inherent unpredictability of technological progress means that within the growing literature, estimates of the jobs at risk of automation can vary widely, and the timeframes within which these impacts are predicted to occur are similarly broad, ranging from 10 to 50 years. Both the shape disruption will take, and its extent, are uncertain. What is certain is that workers will need to learn new skills and develop new competencies to adapt to changes are on their way (Crawford Urban and Johal, 2020[36]).
2.2.2. Automation could affect many workers in some occupations in Australia
Food Preparation Assistants, Handicraft and Printing Workers and Stationary Plant and Machine Operators are the three occupations facing the highest average risk of automation in Australia (see Figure 2.15). Workers employed in those occupations are therefore at a higher risk of losing their job or experiencing substantial change in terms of the skills required to perform that job. On the other hand, some occupations traditionally face a lower risk of being impacted by automation, including for example Teaching Professionals and Chief Executives, Senior Officials and Legislators. Jobs facing a higher risk of automation entail a relatively higher share of routine, repetitive and manual tasks that are more susceptible to being replicable by machines.
Based on the OECD methodology and given Australia’s occupational structure, personal service workers is the occupation that employs the largest number of workers at risk, with almost 315 000 workers who might be impacted by automation. Sales workers, drivers and mobile plant operators and customer services clerks are also occupations that risk facing substantial disruption in the coming years. Figure 2.14 provides an overview of the occupations where labour markets in Australia might face the most disruption. Jobs in these occupations are more likely to be automated on a large scale than in any other occupation. For this reason, automation may prove more disruptive for individual workers - especially if automation occurs quickly and on a large scale - as affected workers will face significant competition from other laid-off workers. Indeed, they will have to compete with a large number of other workers with similar profiles and skill sets who are also looking for new jobs. It should however be noted that there are contextual factors in Australia which might limit the impact of automation on these jobs. There might be country-specific factors which might limit the risk of automation for some occupations. In the case of Australia for example, geographical distance, market fragmentation and low population density are factors that might limit competitive pressures, potentially reducing incentives for businesses to cut costs and automate, and therefore reducing the risk for certain occupations.
Figure 2.14. Which occupations in Australia might be most impacted by automation?
Top 10 occupations with the largest number of workers at risk

Note: Figure includes persons in high or significant risk. Numbers are based on SA4 level calculations by occupation aggregated to obtain a national estimate.
Source: OECD calculations on Census 2016 data.
The risk of automation for each occupation depends on the tasks that are performed as part of it. Based on the OECD methodology, “bottlenecks to automation” are those tasks that, given the current state of knowledge, are difficult to automate. Bottlenecks include finger dexterity, simple and complex problem solving, teaching, advising, planning for others, communication, negotiation, influence and sales. Occupations where these tasks are frequent face a low risk of automation, while occupations where these tasks are less frequent, face a high risk. For more information about the bottlenecks to automation and their relation with the risk of automation (see Box 2.2).
Figure 2.15 presents the ten occupations facing the highest and lowest risk of automation in Australia, and the frequency of a selected number of bottlenecks to automation. Food Preparation Assistants, the occupation facing the highest risk of automation in Australia, show the lowest frequency of bottlenecks tasks such as planning and influence in Australia. The frequency of complex problems and advising is lowest for Cleaners and Helpers, while that of teaching is lowest for Refuse Workers and Other Elementary Workers. On the other hand, among occupations facing the lowest average risk of automation in Australia, teaching and influence are most frequent for Teaching Professionals. Complex problems are most common for Administrative and Commercial Managers, planning for Chief Executives, and advising for Health Professionals.
Figure 2.15. Jobs at risk of automation entail a low frequency of tasks safe from automation
Frequency of selected bottlenecks to automation, occupations facing the highest and lowest average risk of automation in Australia

Source: OECD calculations on PIAAC.
2.2.3. Some segments of the population face a higher risk than others
While both men and women in Australia are employed in occupations that might be impacted by automation, Australian men face a higher risk of automation than women (37.1% and 32.0% respectively), as they are more likely to be employed in occupations involving routine and repetitive tasks (see Figure 2.16). For example, as many as 256 000 men working as drivers and mobile plant operators in Australia are at risk of being affected by automation, compared to 17 000 women. Similarly, almost 200 000 men working as building and related trades workers (excluding electricians), more than 150 000 working as metal, Mmachinery and related trades workers, and more than 150 000 labourers in mining, construction, manufacturing and transport are at risk, compared to 15 000, 2 000 and 36 000 women. On the other hand, however, slightly more women than men face a risk of automation in occupations such as personal pervice workers (221 000 and 93 000 workers respectively) and sales workers (185 000 and 111 000).
Indigenous Australians often work in jobs requiring lower levels of skills, which face the highest risk of being automated over the long-term. They have lower employment shares than the total population in occupations such as managers and professionals. On the other hand, they have higher employment shares in machinery operators and drivers and labourers as well as other low-skill occupations (see Figure 2.17). In addition, efforts to improve labour market conditions of Indigenous Australians could be instrumental in tackling their risk of being impacted by automation. Between 2006 and 2016, Indigenous labour force participation, including the proportion of the 15-64 year-old population employed or seeking employment, had slightly increased from 56.8% to 57.1%, but remained lower than for the rest of the population (75.5% and 77.0% in 2006 and 2016 respectively) and experienced fewer percentage point gains. In addition, Indigenous Australians continue to face higher unemployment rates than the rest of the population, and their conditions seem to have deteriorated between 2006 and 2016. In 2006, the unemployment rate for Indigenous people stood at 15.7% (compared to 5.1% for the rest of the population), while in 2016 it amounted to 18.4% (compared to 6.8% for the rest of the population) (OECD, 2019[37]). The social economy could play a key role in improving labour market outcomes of Indigenous Australians.
Figure 2.16. Australian men face a higher risk of automation than women
Share of jobs at risk of automation by gender, 2016

Source: OECD calculations on Census 2016 data.
Figure 2.17. Indigenous Australians tend to work in lower-skill occupations, which have a higher probability of being automated
Employment share by occupation, Indigenous and total population in Australia

Note: Non-Indigenous Identity also includes persons who did not specify an identity within the 2016 Census (the category "Indigenous Status Not Stated").
Source: Australian Bureau of Statistics, Census of Population and Housing, 2016.
2.3. What are the local impacts of automation in Australia?
2.3.1. The risk of automation has a regional dimension in Australia
The risk of automation depends on the occupational profile of local labour markets and is therefore asymmetric across places. The geographic dimension of automation has important implications for policy makers trying to design policies that ensure the availability of good quality jobs. Automatable tasks are more prevalent in certain occupations and sectors, and neither occupations nor sectors are evenly distributed within national borders. Thus, areas with a higher proportion of jobs relying on routine tasks are likely to experience more disruption, whereas places where more jobs require tacit skills will face lower levels of risk. Tacit skills are based on experience and intuition instead of formal rules. They are therefore more difficult to replicate through mechanical processes or standard algorithms (OECD, 2018[32]).
The Australian Capital Territory faces the lowest risk of automation across states and territories in Australia. The Australian Capital Territory has a relatively high share of high-skill professionals compared to other states and territories, and it is predominantly a service-based economy. A total 29.3% of jobs are at risk of automation in the Australian Capital Territory – 21.3% facing high risk and 8.0% vulnerable to significant change. Across states and territories, the share of jobs at risk of automation is relatively low also in New South Wales (33.9%), Victoria (34.0%) and the Northern Territory (34.5%).
However, regional differences in the risk of automation exist within all Australian states and territories, with some showing higher regional variation than others. For example, regional variation in the share of jobs at risk of automation amounts to more than 15 percentage points in New South Wales, ranging from 40.2% in Hunter Valley exc. Newcastle to 24.6% in Sydney - North Sydney and Hornsby. On the other hand, regional differences in the risk of automation amount to about 1% in Northern Territory (34.9% in Darwin and 33.6% in the Northern Territory – Outback) (see Figure 2.19). Across regions, the risk of automation is highest in Mackay - Isaac - Whitsunday in Queensland (41.2%), followed by South Australia – South East in South Australia (40.3%). On the other hand, Sydney – North Sydney and Hornsby and Sydney – Eastern Suburbs have the lowest risk of losing jobs to automation in Australia, standing at 24.6% and 25.5% respectively. Looking at the total number of jobs at risk, this is highest in Melbourne-South East (Victoria) and Melbourne-West (Victoria), where about 125 000 and 120 000 jobs are at risk of automation respectively.
Regions with the lowest risk of losing jobs to automation in each state are often those surrounding the state’s capital. This is not surprising, as capital cities generally have higher employment shares in occupations requiring a higher-skill level, which face a lower risk of being impacted by automation. Capital cities also have a larger population base and higher incomes, which may mean that they are more likely to have less automatable professions such as teaching, health care and hospitality.
Looking at Queensland and Victoria, Brisbane Inner City and Melbourne Inner show the lowest risk among the states’ regions. Similarly, Adelaide Central and Hills shows the lowest risk in South Australia, Perth Inner in Western Australia, Sydney – North Sydney and Hornsby in New South Wales, and Hobart in Tasmania.
Figure 2.18. The share of jobs at risk of automation is lowest in the Australian Capital Territory
Share of jobs at risk of automation, Australian states and territories, 2016

Source: OECD calculations on Census 2016 data.
Table 2.3. Which Australian regions have the largest share of jobs at risk of automation?
State |
SA4 region |
Share of jobs at risk of automation |
Number of jobs at risk of automation |
---|---|---|---|
Queensland |
Mackay - Isaac - Whitsunday |
41.2 |
32 000 |
South Australia |
South Australia - South East |
40.3 |
31 000 |
Queensland |
Logan - Beaudesert |
40.2 |
54 000 |
Queensland |
Darling Downs - Maranoa |
40.2 |
22 000 |
Source: OECD calculations on Census 2016 data.
Table 2.4. Which Australian regions have the lowest share of jobs at risk of automation?
State |
SA4 region |
Share of jobs at risk of automation |
Number of jobs at risk of automation |
---|---|---|---|
New South Wales |
Sydney - North Sydney and Hornsby |
24.6 |
50 000 |
New South Wales |
Sydney - Eastern Suburbs |
25.5 |
34 000 |
Western Australia |
Perth - Inner |
26.2 |
22 000 |
Victoria |
Melbourne - Inner |
26.4 |
83 000 |
Source: OECD calculations on Census 2016 data.
Figure 2.19. The share of jobs at risk of automation varies substantially within most states and territories
Share of jobs at risk of automation, regions facing the highest and lowest shares within each state

Note: Data from ABS/Census 2016. Only states and territories with two or more SA4 regions are included.
Source: OECD calculations on Census 2016 data.
Box 2.3. How is the regional risk of automation calculated?
In order to produce sub-national estimates of the risk of automation, the results of Nedelkoska and Quintini (2018[35]) are applied at the state/territory and Statistical Area 4 (SA4) level in Australia. For each state/territory and SA4 region, the share of jobs at risk of automation is calculated using data on provincial and regional employment by occupation and the estimated probabilities of automfigureation from Nedelkoska and Quintini (2018[35]). As an approximation, the method assumes that jobs within the same job category have the same risk of automation across all states/territories and SA4 regions of Australia. Detailed estimates of the risk of automation, including the share and number of jobs at high and significant risk for all Australian SA4 regions, are presented in the Annex 2.A.
Importantly, the methodology to estimate the risk of automation in an occupation can account for differences in the risk across countries but it cannot show how the risk of automation within an occupation changes over time. This introduces an important caveat. Countries or regions might respond to the threat of automation not only by shifting into economic sectors that have a lower risk of automation, but also by adapting the task profile of occupations so that they become less susceptible to automation. With the current methodology, it is impossible to capture the latter effect. Estimates that show how the number of jobs at risk of automation changes over time are based on how the number of jobs in different occupations changes over time. In other words, they show how regions gain or lose jobs in risky and less risky occupations.
TThe mean, high and significant risk of automation by occupation in Australia and the number of workers affected are presented in the Annex 2.A. These probabilities are defined for each occupation based on the Nedelkoska and Quintini (2018[35]) methodology using PIAAC. Occupations with the highest mean risk of automation typically do not require specific skills or training, and include food preparation assistants, assemblers, labourers, refuse workers, cleaners and helpers. At the other end of the spectrum are occupations that require high level of education and training and which involve high degree of social interaction, creativity, problem-solving and caring for others. The high and significant risk of automation probabilities for each occupation in Australia are used to calculate the number of people at risk in each economic region in the country.
Source: Nedelkoska and Quintini (2018[35]).
2.3.2. The drivers of the risk of automation vary from one region to the other
The drivers of job loss due to automation differ across regions, even when they face similar risks of automation, and depend on the specific characteristics of the local economy. Therefore, there is not one single explanation of the risk of automation at the local level, and policies to reduce the risk of automation will depend on the specific features of local economies, including their sectoral composition and the workforce skills. In some regions, where Agriculture is a prevalent source of income, the risk of losing jobs to automation might be mainly related to the prevalence of skilled occupations in agriculture, which could be potentially replaced by machines. This might be the example of Darling Downs – Maranoa and Queensland – Outback in Queensland, among others. On the other hand, the prevalence of Manufacturing, construction or administrative jobs in cities and urban regions, might cause the risk of automation in these regions to be related mainly to those occupations. This might for example be the case of Mornington Peninsula in Victoria.
However, Australian regions facing a high risk tend to have some sectoral characteristics in common, such as relatively high employment shares in Manufacturing (see Figure 2.20). Traditional Manufacturing jobs tend to entail routine and repetitive tasks that might be vulnerable to automation. Research conducted for example by Autor (2015[38]) argues that a large proportion of middle-wage occupations, such as Manufacturing or clerical jobs, are highly reliant on easily automatable routine tasks. The prevalence of Manufacturing employment is also a characteristic of regions facing a higher risk of automation across the OECD (OECD, 2018[32]).
Figure 2.20. The risk of automation tends to be higher in Australian regions with higher employment shares in Manufacturing
Manufacturing employment and share of jobs at risk of automation, Australia SA4 regions

Source: OECD calculations on Census 2016 and Australian Bureau of Statistics.
In some Australian regions the risk of automation is driven by occupations which are common to the Mining sector. This is the case of Mackay-Isaac-Whitsunday (Queensland), which is the region facing the highest share of jobs at risk of automation in Australia (41.2%). Here, the regional risk of automation is driven by large employment shares in occupations such as Stationary Plant and Machine Operators, Drivers and Mobile Plant Operators, and Metal, Machinery and Related Trades Workers. A large number of these workers are employed within the Mining sector. In Mackay-Isaac-Whitsunday, about 15 600 people work in the Mining sector, which accounts for 17.4% of total employment.
In other regions, it is large employment shares in Agriculture that contribute to a high risk of automation. South Australia-South East (South Australia) faces one of the highest risk of automation in Australia (40.3%). In the region, the largest amount of jobs at risk of automation are Market-oriented Skilled Agricultural Workers (almost 3 000 workers at risk). South Australia-South East has a high employment share in Agriculture, which is the largest sector in region, accounting for 14.5% of total employment. Similarly, in Darling Downs (Queensland), which faces among the highest risk of automation across regions (40.2%), most workers at risk of automation are employed as Market-oriented Skilled Agricultural Workers (about 2 500 workers at risk). In the region, Agriculture accounts for 24.0% of total employment.
In several other regions, the high risk of automation derives from a mix of occupations in Personal Services, Retail Trade, Construction and Manufacturing. This is the case for example of Logan-Beaudesert (Queensland), which is the region facing the third highest share of jobs at risk of automation (40.2%). About 6 000 Mobile Plant and Machine Operators are at risk, followed by about 4 400 Labourers in Mining, Construction, Manufacturing and Transport, 4 000 Building and Related Trades Workers (excluding Electricians), 4 000 Sales Workers and 3 500 Personal Service Workers. This reflects large regional employment shares in sectors that face a high risk. In the region, Construction accounts for about 14.0% of total employment, followed by Health Care and Social Assistance (12.8%), Manufacturing (10.1%) and Retail Trade (8.4%). Tourism might also be the driver of the risk of automation in some regions (see Box 2.4).
Box 2.4. How will the future of work impact the tourism sector?
COVID-19 has heavily impacted the Tourism industry, in Australia as around the word. Tourism has continued to be one of the sectors hardest hit by the coronavirus pandemic and the outlook remains highly uncertain. While positive news on vaccines has boosted the hopes of Tourism businesses and travellers alike, challenges remain. Vaccine roll out will take some time, and the sector is potentially facing stop/start cycles for some time. The United Nations World Tourism Organization (UNWTO) foresees a decline in international arrivals close to 70%, with recovery to pre-pandemic levels not expected before 2023 (OECD, 2020[39]).
Prior to the pandemic, automation and new technologies were increasingly seen as new opportunities for both tourists and Tourism sector companies. Real-time translation software is making it more convenient for tourists to travel to areas in which they do not speak the language. Indeed, translation apps are now able to translate signage and are expected to go beyond that capability into translating verbal statements in coming years. Companies in the sector are starting to use big data and predictive analytics to increase knowledge of consumer behaviour and customise travel experiences accordingly. Autonomous vehicles (AVs) are already successfully being piloted on the roads in a number of jurisdictions and it is anticipated that the application of this technology on a broader scale could happen by 2025 or sooner.
While Tourism jobs are still recovering from the impacts of COVID-19, going forward automation is also likely to significantly impact some jobs in the sector, reshaping local labour markets that highly rely on Tourism as a source of employment. Jobs that include routine and repetitive tasks, such as moving objects, information processing, calculations, standardised communications, might be automated through computer programs, mobile applications, kiosks, chatbots, robots, autonomous vehicles, and other automation technologies. Within Australia, in Sunshine Coast (Queensland), where Accommodation and Food Services account for 11.5% of total employment, the largest number of workers at risk of automation are Personal Service Workers and Sales Workers (about 5 600 and 4 800 respectively). Similarly, in Gold Coast (also Queensland), where Accommodation and Food Services stand at 10.6% of total employment, there are about 10 000 Personal Service Workers and 9 000 Sales Workers at risk of being impacted by automation, higher than any other occupation. Going forward it will be important to ensure that the adoption of automation technologies in key local industries is matched with strategies to re-skill workers and diversify the economy. It should however also be noted that, given that the majority of businesses operating in the Tourism sector in Australia are SMEs, they might be less able to adopt and invest in automation technologies.
Automation will also change the type of skills needed for some jobs and create entirely new jobs in the Tourism sector. As an example, a marketing specialist would need to update the information in a chatbot and modify its AI rules as necessary. Chefs might need to get acquainted with restaurant information systems and smart fridges. Hotel receptionists might not need to give keys or issue cards to hotel guests, as access to hotel rooms might be with facial recognition technologies. Automation could also create the need for completely new job positions in Tourism companies that are currently largely neglected – e.g. for robot maintenance and repair, kiosks maintenance and repair, big data analytics, machine learning, automation process planning and control.
Rebuilding tourism is a priority, but the sector must become more sustainable and resilient. Seamless travel was identified as a pillar within the tourism agenda for the G20 at the start of 2020 but COVID-19 led to a massive drop in tourism during the year in Australia and around the world. As a result, the sector experienced job losses. As vaccinations roll out and procedures for safe and seamless travel are put in place, jobs are picking up in many locations. However, the crisis is an opportunity to rethink tourism for the future, as measures put in place today will shape the sustainable tourism of tomorrow.
Source: OECD (2018[40]; 2020[41]); Ivanov (2020[42]); (OECD, 2020[43]); (OECD, 2020[44]).
2.3.3. However, regions facing a high risk have some characteristics in common, such as poorer employment and skills outcomes
Regions facing a higher risk of losing jobs to automation in Australia tend to have higher unemployment rates (see Figure 2.21). The unemployment rate was highest in Queensland-Outback (Queensland), standing at 11.8% in 2019. The region has a share of jobs at risk of automation of 39.4%, among the highest across Australia regions. In Mackay - Isaac – Whitsunday, also in Queensland, the region with the highest share of jobs at risk of automation in Australia, the unemployment rate stands at 5.9%, above the average across regions. On the other hand, in Sydney-Inner West (New South Wales), where the unemployment rate is lowest, standing at 2.0%, only 28.2% of jobs are at risk of automation. Sydney - North Sydney and Hornsby (New South Wales), the region with the lowest share of jobs at risk of automation (24.6%), has an unemployment rate of only 3.5%.
Figure 2.21. Regions with a higher share of jobs at risk of automation tend to have higher unemployment rates
Unemployment rates and share of jobs at risk of automation, Australia SA4 regions

Source: OECD calculations on Census 2016 and Australian Bureau of Statistics.
The challenge for regions with high unemployment rates is that they often have lower productivity levels. These regions therefore face a dilemma as on the one hand, they have to provide jobs in the short term. On the other hand, they also have to encourage efforts to increase labour productivity to ensure high employment levels and prosperity in the long term. In many cases, this requires increasing automation, which can harm employment in the short run (OECD, 2018[32]).
The share of jobs at risk of automation also tends to be higher in regions with a lower share of tertiary-educated population (see Figure 2.22). Sydney - North Sydney and Hornsby (New South Wales) is the region with the highest share of tertiary-educated population and the lowest share of jobs at risk of automation, amounting to 30.4% and 24.6% respectively. The share of population with a bachelor’s degree is lowest in Wide Bay (Queensland), standing at 6.8%. This region also has among the highest share of jobs at risk in Australia (39.3%). Extensive research documents that places with highly educated workforces are less affected by automation. With some exceptions, the risk of automation decreases as educational attainment required for the job increases. Regions that have the highest share of jobs at risk of automation also have the lowest share of workers with tertiary education. Reducing the risk of automation in those regions will therefore require efforts in training and education (OECD, 2018[32]). Another key consideration is age demographics. About 39% of the population in Wide Bay is aged over 55. This is compared to 28% nationally. On the other hand, only 25% of the population in north Sydney is aged over 55. It is more common for younger people in Australia to hold a bachelor’s degree with a shift in focus towards higher education.
Figure 2.22. The share of jobs at risk of automation is higher in regions with lower levels of skills
Population with a Bachelor Degree and share of jobs at risk of automation, Australia SA4 regions

Source: OECD calculations on Census 2016.
2.3.4. The large majority of Australian regions have created jobs in less risky occupations over the past decade
About 81 regions in Australia, accounting for 91% of the total number of regions in the country, have created jobs predominantly in less risky occupations between 2006 and 2016. This suggests that the largest majority of Australian regions have created jobs reducing their vulnerability to automation over the past decade. The occupations that have grown the most in terms of total employment at the national level reducing the risk of automation include health professionals, teaching professionals, production and specialised services managers, business and administration professionals, among others. These factors are part of the structural adjustment taking place: shift in economic activity toward services, knowledge-based occupations etc. Given structural adjustment is likely to continue and highly unlikely to reverse, the point that automation potential may fall overtime, especially in regions where the said industries and occupations are growing, needs to be made.
However, some Australian regions have experienced increases in their exposure to automation, by shifting their employment structure towards jobs at a higher risk. It should be noted however that this risk varies at the local level, being sensible to the specific local context. Six Australian regions (7% of total regions) lost jobs between 2006 and 2016 predominantly in less risky occupations. These include Northern Territory-Outback (Northern Territory), West and North West (Tasmania), South Australia-Outback (South Australia), Queensland-Outback (Queensland), Brisbane-West (Queensland), and Far West and Orana (New South Wales). These regions might face significant challenges, as they are losing jobs and increasing their vulnerability to automation. The loss of jobs at a lower risk of automation in these regions compounds longer-term trends and challenges faced by these regions. Extreme regional areas in Australia face declining population growth, an aging demographic, skills drain, among others. These factors alone may limit job opportunities to a much greater extent than automation. Two other regions in Australia, including Barossa-Yorke-Mid North (South Australia) and Western Australia-Wheat Belt (Western Australia) are creating jobs, predominantly in risky occupations, therefore increasing their exposure to future labour market disruption.
Figure 2.23. Most Australian regions have created jobs predominantly in less risky occupations since 2006
Percentage of SA4 regions creating/losing jobs, 2006 to 2016

Source: OECD calculations on 2006 and 2016 Censuses.
Box 2.5. A regional typology for employment creation in the face of digitalisation and automation
Regions can be classified into four categories depending on whether they gain or lose jobs and whether the gains or losses occur in sectors with high or low risk of automation. Australian regions are classified according to whether they created jobs in the period 2006 to 2016, and further divided according to the type of occupation in which they created or shed employment. This classification provides insights into the short-term and long-term employment situation of a region (OECD, 2018[32]).
Table 2.5. The typology
Creating jobs |
Losing jobs |
||
---|---|---|---|
Predominantly in less risky occupations |
Predominantly in riskier occupations |
Predominantly in less risky occupations |
Predominantly in riskier occupations |
These regions improve their job situation in the short term and also reduce their long-term risk of unemployment from automation |
These regions improve their short-term job situation, but do so at the expense of moving towards a riskier job profile in the future |
These regions face the greatest challenge. They suffer current job losses combined with an increasing risk of further job losses in the future due to automation |
These regions have the typical profile of regions in the process of undergoing a structural change caused by automation. While jobs are being lost to automation today, the risk of further job losses due to automation decreases |
Source: OECD (2018[32]).
2.4. Special focus on Sydney-South West (New South Wales) and Warrnambool and South West (Victoria)
The regions of Sydney-South West (New South Wales) and Warrnambool and South West (Victoria) were chosen as case study areas for this report. They were selected because they face a higher risk of automation than on average in Australia and are among those at highest risk within their state. Sydney-South West is characterised by a relatively diversified local economy, with the high risk of automation deriving from high employment shares in a range of occupations at high risk. On the other hand, in Warrnambool and South West, Agriculture is the largest employer and drives the risk of automation in the region.
2.4.1. Sydney-South West: a widespread risk of automation adds to existing labour market challenges, but there are opportunities ahead
Sydney-South West is a populous region within New South Wales that faced more challenging labour market conditions than the rest of the state over the past decade. The region had a population of 405 686 inhabitants according to the 2016 Census, corresponding to about 5.4% of total population in New South Wales. The unemployment rate stood at 6.1% in 2019, among the highest in New South Wales. However, the rate had declined over the past decade and reached lower levels than in 2008. The labour force participation rate stood at 61.8% in 2019, compared to 65.6% across New South Wales. Only 13.8% of the population aged 15 and more has a bachelor’s degree level and above in Sydney-South West, compared to 23.4% in New South Wales. Over a fifth of the population (21.4%) has attained Year 12 education as their highest level of education (Australian Bureau of Statistics, 2019[45]). Sydney-South West has a younger population than New South Wales overall, which can contribute to differences in the share of population attaining a bachelor’s degree. Sydney-South West has a median age of 35, and about 14.6% of the population is aged 15-24. This compares to a median age of 38 in New South Wales and 12.5% of the population being 15-24 years-old.
Sydney-South West faces among the highest risk of automation across regions in New South Wales (see Figure 2.24). About 39.6% of jobs are at risk of automation in the region, the second highest among all 28 New South Wales regions. Only Hunter Valley exc. Newcastle faces a higher risk (40.2%). The risk of automation in Sydney-South West consists of 12.3% of jobs at high risk of automation and 27.3% at significant risk of change. The region has the highest share of jobs at high risk of automation in New South Wales.
This reflects the occupational profile of Sydney-South West and its differences with New South Wales as a whole. Looking at the sectoral composition of employment, Retail Trade, Health Care and Social Assistance, Manufacturing and Construction are the largest employers in Sydney-South West, accounting for 12.2%, 10.7%, 10.5% and 9.9% of total employment respectively. Sydney-South West has relatively high employment shares in many occupations facing a high risk of automation (see Table 2.6). For example, the region has higher employment shares in occupations such as Food Preparation Assistants, Handicraft and Printing Workers, Stationary Plant and Machine Operators, Cleaners and Helpers (i.e. those performing various tasks including keeping the interiors and fixtures clean or hand-laundering and pressing in private households, hotels, offices, etc.), and General and Keyboard Clerks, which are those facing the highest risk of automation in Australia. Differences in employment shares between Sydney-South West and New South Wales are highest for Drivers and Mobile Plant Operators and Labourers in Mining, Construction, Manufacturing and Transport, amounting to 2.3 and 2.5 percentage points respectively.
Most workers at risk of automation in Sydney-South West are Drivers and Mobile Plant Operators and Labourers in Mining, Construction, Manufacturing and Transport. About 6 400 people at risk are employed as Drivers and Mobile Plant Operators, followed by 5 300 Labourers in Mining, Construction, Manufacturing and Transport. Other occupations where the region risks losing many jobs include: Personal Service Workers, Sales Workers, Building and Related Trades Workers (excluding Electricians), where about 4 400, 4 400 and 4 300 jobs risk being affected.
Compared to a decade ago, Sydney-South West has however reduced its risk of automation, having created jobs predominantly in less risky occupations, between 2006 and 2016 (see Figure 2.25). For example, the region has added almost 2 500 Teaching Professionals, 2 000 Health Professionals and 1 600 Production and Specialised Services Managers, which are occupations facing a low risk of automation. At the same time, several occupations facing a higher risk have shed jobs over 2006 to 2016. For example, Stationary Plant and Machine Operators have shed about 500 jobs, Handicraft and Printing Workers about 340, General and Keyboard Clerks about 260. Some occupations at higher risk have however experienced jobs growth between 2006 and 2016 in Sydney-South West. A prominent example is given by Drivers and Mobile Plant Operators, which have added more than 2 200 jobs over that period. Going forward, it will be important for the region to ensure that future jobs growth is not driven by higher-risk occupations.
Figure 2.24. Sydney-South West faces the second highest risk of automation in New South Wales
Share of jobs at risk of automation, SA4 regions in New South Wales, 2016

Source: OECD calculations on Census 2016.
Table 2.6. Sydney-South West has high employment shares in occupations at high risk
Occupation |
Total employment |
Occupation share of total employment |
Jobs at significant risk of change |
Jobs at high risk of automation |
Food Preparation Assistants |
2 000 |
1.4% |
32.7% |
48.7% |
Handicraft and Printing Workers |
1 000 |
0.4% |
50.4% |
32.0% |
Stationary Plant and Machine Operators |
6 000 |
3.8% |
39.4% |
32.1% |
Cleaners and Helpers |
2 000 |
1.5% |
54.4% |
20.5% |
General and Keyboard Clerks |
5 000 |
3.1% |
39.6% |
24.0% |
Drivers and Mobile Plant Operators |
10 000 |
6.3% |
44.4% |
20.8% |
Labourers in Mining, Construction, Manufacturing and Transport |
8 000 |
5.3% |
32.9% |
31.3% |
Refuse Workers and Other Elementary Workers |
2 000 |
1.0% |
24.6% |
28.8% |
Agricultural, Forestry and Fishery Labourers |
1 000 |
0.4% |
45.1% |
10.4% |
Other Clerical Support Workers |
1 000 |
0.8% |
40.5% |
20.2% |
Note: total employment numbers are rounded.
Source: OECD calculations on Census 2016.
Figure 2.25. Sydney-South West has created jobs mainly in occupations at lower risk of automation
Change in number of jobs by occupation, 2006 to 2016

Note: Dark blue represents high-skill jobs, light blue represents middle-skill and gray represents low-skill. The number in the bubbles denotes the occupation ISCO-08 code.
Source: OECD calculations on 2006 and 2016 Census.
2.4.2. Warrnambool and South West: Agriculture drives the risk of automation, but employment has shifted towards lower-risk occupations recently
The unemployment rate in Warrnambool and South West has traditionally been lower than elsewhere in Victoria. The rate stood at about 3.0% in 2019, compared to 4.7% on average in Victoria, and the lowest among all Victorian SA4 regions. Already in 2008, the unemployment rate was lowest in Warrnambool and South West compared to other regions in the state. Warrnambool and South West has a total population of about 126 000 people in 2020, accounting for about 2% of Victoria’s total population. According to Census data, Aboriginal and Torres Strait Islander people make up 1.5% of the local population, compared to 0.8% in Victoria. The share of people attaining bachelor’s degree level and above is substantially lower in Warrnambool and South West compared to Victoria on average (12.7% and 24.3% respectively). The largest share of the population in Warrnambool and South West has a Certificate level III qualification (15.5%) (Australian Bureau of Statistics, 2019[46]).
Agriculture is the main driver of local employment in Warrnambool and South West. Agriculture, Forestry and Fishing accounts for 22.5% of total employment (or 15 200 jobs). Employment in Agriculture has also been growing over the past five years, adding about 4 100 jobs between 2015 and 2020. Health Care and Social Assistance is the second largest sector, employing 10.7% of the local workforce (7 200 people), followed by Education and Training and Accommodation and Food Services, accounting for 9.3% of total employment respectively, or 6 300 jobs.
Driven by occupations in Agriculture, the risk of automation is substantially higher in Warrnambool and South West than elsewhere in the state of Victoria (see Figure 2.26). About 38.4% of jobs are at risk, compared to 34.0% on average in the state. The high share of jobs at risk of automation in the region is driven by Agriculture, as about 2 250 workers employed as Market-oriented Skilled Agricultural Workers are at risk of being impacted by automation. This is followed by about 2 000 Drivers and Mobile Plant Operators, 1 800 Personal Service Workers and 1 500 Sales Workers.
Overall, the region has created jobs in less risky occupations between 2006 and 2016 (see Figure 2.27). As an example, the region has generated about 400 Health Professionals over this period, which face a low risk of automation. At the same time, the region has lost jobs in riskier occupations, such as Labourers in Mining, Construction, Manufacturing (-450 jobs) and Transport and Market-oriented Skilled Agricultural Workers (-550 jobs). However, it also created jobs in occupations facing a higher risk, which potentially poses challenges for the longer term might these trends continue or intensify. For example, the region has added about 300 Food Preparation Assistants and 300 Drivers and Mobile Plant Operators.
Figure 2.26. In Warrnambool and South West, the risk of automation is among the highest in Victoria
Share of jobs at risk of automation, SA4 regions in Victoria, 2016

Source: OECD calculations on 2016 Census
Table 2.7. Warrnambool and South West tends to have higher employer shares in occupations at risk than Victoria
Occupation |
Total employment |
Occupation share of total employment |
Jobs at significant risk of change |
Jobs at high risk of automation |
Food Preparation Assistants |
1 000 |
1.9% |
32.7% |
48.7% |
Handicraft and Printing Workers |
100 |
0.2% |
50.4% |
32.0% |
Stationary Plant and Machine Operators |
2 000 |
2.8% |
39.4% |
32.1% |
Cleaners and Helpers |
1 000 |
2.0% |
54.4% |
20.5% |
General and Keyboard Clerks |
1 000 |
2.1% |
39.6% |
24.0% |
Drivers and Mobile Plant Operators |
3 000 |
5.5% |
44.4% |
20.8% |
Labourers in Mining, Construction, Manufacturing and Transport |
1 000 |
2.5% |
32.9% |
31.3% |
Refuse Workers and Other Elementary Workers |
400 |
0.7% |
24.6% |
28.8% |
Agricultural, Forestry and Fishery Labourers |
1 000 |
2.2% |
45.1% |
10.4% |
Other Clerical Support Workers |
300 |
0.6% |
40.5% |
20.2% |
Note: total employment numbers are rounded.
Source: OECD calculations on 2016 Census.
Figure 2.27. Warrnambool and South West has created jobs mainly in occupations at lower risk of automation
Change in number of jobs by occupation, 2006 to 2016

Note: Dark blue represents high-skill jobs, light blue represents middle-skill and gray represents low-skill. The number in the bubbles denotes the occupation ISCO-08 code.
Source: OECD calculations on 2006 and 2016 Census.
References
[16] Adeney, R. (2018), Structural Change in the Australian Economy, https://www.rba.gov.au/publications/bulletin/2018/mar/pdf/structural-change-in-the-australian-economy.pdf (accessed on 2 June 2020).
[34] Arntz, M., T. Gregory and U. Zierahn (2016), “The Risk of Automation for Jobs in OECD Countries: A Comparative Analysis”, OECD Social, Employment and Migration Working Papers, No. 189, OECD Publishing, Paris, https://dx.doi.org/10.1787/5jlz9h56dvq7-en.
[3] Australian Bureau of Statistics (2020), 6202.0 - Labour Force, Australia, Apr 2020, https://www.abs.gov.au/ausstats/abs@.nsf/0/6050C537617B613BCA25836800102753?Opendocument (accessed on 18 May 2020).
[45] Australian Bureau of Statistics (2019), 2016 Census QuickStats: Sydney - South West, https://quickstats.censusdata.abs.gov.au/census_services/getproduct/census/2016/quickstat/127?opendocument (accessed on 15 June 2020).
[46] Australian Bureau of Statistics (2019), 2016 Census QuickStats: Warrnambool and South West, https://quickstats.censusdata.abs.gov.au/census_services/getproduct/census/2016/quickstat/217?opendocument (accessed on 15 June 2020).
[48] Australian Government (2020), 3 Step Framework for a COVID Safe Australia, https://www.pm.gov.au/sites/default/files/files/three-step-framework-covidsafe-australia.pdf (accessed on 18 May 2020).
[4] Australian Government Department of Education, Skills and Employment (2020), Impact of COVID-19 on Australian businesses - part one, https://www.employment.gov.au/newsroom/impact-covid-19-australian-businesses-part-one (accessed on 18 May 2020).
[5] Australian Government Department of Education, Skills and Employment (2020), JobTrainer package announced, https://www.dese.gov.au/news/jobtrainer-package-announced (accessed on 24 July 2020).
[23] Australian Government Productivity Commission (2020), PC Productivity Insights: Can Australia become a productivity leader?, https://www.pc.gov.au/research/ongoing/productivity-insights/australia-leader/productivity-insights-2020-australia-leader.pdf (accessed on 4 June 2020).
[22] Australian Government Productivity Commission (2019), PC Productivity Bulletin, https://www.pc.gov.au/research/ongoing/productivity-insights/2019/productivity-bulletin-2019.pdf (accessed on 4 June 2020).
[6] Australian Government Treasury (2020), Economic Response to the Coronavirus, https://treasury.gov.au/coronavirus/businesses (accessed on 29 July 2020).
[38] Autor, D. (2015), “Why Are There Still So Many Jobs? The History and Future of Workplace Automation”, Journal of Economic Perspectives, Vol. 29/3, pp. 3-30, http://dx.doi.org/10.1257/jep.29.3.3.
[8] Borland, J. (2020), Labour market snapshot #56 April 2020, https://cdn.theconversation.com/static_files/files/906/lmsapr20new_%281%29.pdf?1585212052 (accessed on 15 June 2020).
[24] Carmody, C. (2019), Slowing productivity growth-a developed economy comparison.
[36] Crawford Urban, M. and S. Johal (2020), Understanding the Future of Skills: Trends and Global Policy Responses, https://fsc-ccf.ca/wp-content/uploads/2020/01/UnderstandingTheFutureOfSkills-PPF-JAN2020-EN.pdf (accessed on 14 May 2020).
[25] Eslake, S. (2017), Education, Productivity and Economic Performance: Tasmania, then, now and tomorrow, http://dx.doi.org/10.13140/RG.2.2.16614.73288.
[33] Frey, C. and M. Osborne (2013), THE FUTURE OF EMPLOYMENT: HOW SUSCEPTIBLE ARE JOBS TO COMPUTERISATION?, University of Oxford, https://www.oxfordmartin.ox.ac.uk/downloads/academic/The_Future_of_Employment.pdf (accessed on 10 July 2019).
[19] Garnett, A. (2018), The Changes and Challenges Facing Regional Labour Markets, https://businesslaw.curtin.edu.au/wp-content/uploads/sites/5/2019/06/AJLE-v21n2-Garnett.pdf (accessed on 3 June 2020).
[21] Georgeson, C. and A. Harrison (2015), Regional impacts of the accelerated decline of the manufacturing sector in Australia, http://www.industry.gov.au/OCE (accessed on 2 June 2020).
[47] Gilfillan, G. (2020), COVID-19: Labour market impacts on key demographic groups, industries and regions, https://www.aph.gov.au/About_Parliament/Parliamentary_Departments/Parliamentary_Library/pubs/rp/rp2021/COVID-19-Stat_Snapshot#_Toc54358710.
[30] Heath, A. (2020), Skills, Technology and the Future of Work, https://www.rba.gov.au/speeches/2020/sp-so-2020-03-16.html (accessed on 15 June 2020).
[42] Ivanov, S. (2020), “The impact of automation on tourism and hospitality jobs”, Information Technology and Tourism, http://dx.doi.org/10.1007/s40558-020-00175-1.
[18] Kelly, G. and G. La Cava (2014), International Trade Costs, Global Supply Chains and Value-added Trade in Australia, https://www.rba.gov.au/publications/rdp/2014/pdf/rdp2014-07.pdf (accessed on 2 June 2020).
[31] Marcolin, L., S. Miroudot and M. Squicciarini (2016), “Routine jobs, employment and technological innovation in global value chains”, OECD Science, Technology and Industry Working Papers, No. 2016/1, OECD Publishing, Paris, https://dx.doi.org/10.1787/5jm5dcz2d26j-en.
[29] McKinsey & Company (2019), Australia’s automation opportunity: Reigniting productivity and inclusive income growth, https://www.mckinsey.com/~/media/McKinsey/Featured%20Insights/Future%20of%20Organizations/Australias%20automation%20opportunity%20Reigniting%20productivity%20and%20inclusive%20income%20growth/Australia-automation-opportunity-vF.ashx (accessed on 8 June 2020).
[27] Muro, M., R. Maxim and J. Whiton (2020), The robots are ready as the COVID-19 recession spreads, The Brookings Institution, https://www.brookings.edu/blog/the-avenue/2020/03/24/the-robots-are-ready-as-the-covid-19-recession-spreads/ (accessed on 22 April 2020).
[1] National Skills Commission (2020), A snapshot in time: The Australian labour market and COVID-19, https://www.nationalskillscommission.gov.au/sites/default/files/2020-06/NSC_a_snapshot_in_time_report.pdf (accessed on 22 July 2020).
[2] National Skills Commission (2020), The shape of Australia’s post COVID-19 workforce, https://www.nationalskillscommission.gov.au/sites/default/files/2020-12/NSC%20Shape%20of%20Australias%20post%20COVID-19%20workforce.pdf.
[35] Nedelkoska, L. and G. Quintini (2018), “Automation, skills use and training”, OECD Social, Employment and Migration Working Papers, No. 202, OECD Publishing, Paris, https://dx.doi.org/10.1787/2e2f4eea-en.
[10] OECD (2021), Implications of Remote Working Adoption on Place Based Policies: A Focus on G7 Countries, OECD Publishing, Paris, https://dx.doi.org/10.1787/b12f6b85-en.
[12] OECD (2021), OECD Regional Outlook 2021: Addressing COVID-19 and Moving to Net Zero Greenhouse Gas Emissions, OECD Publishing, Paris, https://dx.doi.org/10.1787/17017efe-en.
[11] OECD (2020), Capacity for remote working can affect lockdown costs differently across places, https://read.oecd-ilibrary.org/view/?ref=134_134296-u9iq2m67ag&title=Capacity-for-remote-working-can-affect-lockdown-costs-differently-across-places (accessed on 22 July 2020).
[7] OECD (2020), From pandemic to recovery: Local employment and economic development, https://read.oecd-ilibrary.org/view/?ref=130_130810-m60ml0s4wf&title=From-pandemic-to-recovery-Local-employment-and-economic-development (accessed on 12 May 2020).
[39] OECD (2020), “Mitigating the impact of COVID-19 on tourism and supporting recovery”, OECD Tourism Papers, No. 2020/03, OECD Publishing, Paris, https://dx.doi.org/10.1787/47045bae-en.
[13] OECD (2020), OECD Regions and Cities at a Glance 2020, OECD Publishing, Paris, https://dx.doi.org/10.1787/959d5ba0-en.
[41] OECD (2020), OECD Tourism Trends and Policies 2020, OECD Publishing, Paris, https://dx.doi.org/10.1787/6b47b985-en.
[9] OECD (2020), Productivity gains from teleworking in the post COVID-19 era, https://read.oecd-ilibrary.org/view/?ref=135_135250-u15liwp4jd&title=Productivity-gains-from-teleworking-in-the-post-COVID-19-era (accessed on 22 July 2020).
[43] OECD (2020), Rebuilding tourism for the future: COVID-19 policy responses and recovery, OECD Publishing, Paris, https://www.oecd.org/coronavirus/policy-responses/rebuilding-tourism-for-the-future-covid-19-policy-responses-and-recovery-bced9859.
[44] OECD (2020), “Safe and seamless travel and improved traveller experience: OECD Report to G20 Tourism Working Group”, OECD Tourism Papers, No. 2020/02, OECD Publishing, Paris, https://dx.doi.org/10.1787/d717feea-en.
[14] OECD (2019), Engaging Employers and Developing Skills at the Local Level in Australia, OECD Reviews on Local Job Creation, OECD Publishing, Paris, https://dx.doi.org/10.1787/9789264304888-en.
[37] OECD (2019), Indigenous Employment and Skills Strategies in Australia, OECD Reviews on Local Job Creation, OECD Publishing, Paris, https://dx.doi.org/10.1787/dd1029ea-en.
[26] OECD (2019), OECD Employment Outlook 2019: The Future of Work, OECD Publishing, Paris, https://dx.doi.org/10.1787/9ee00155-en.
[20] OECD (2018), Getting Skills Right: Australia, Getting Skills Right, OECD Publishing, Paris, https://dx.doi.org/10.1787/9789264303539-en.
[32] OECD (2018), Job Creation and Local Economic Development 2018: Preparing for the Future of Work, OECD Publishing, Paris, https://dx.doi.org/10.1787/9789264305342-en.
[15] OECD (2018), OECD Economic Surveys: Australia 2018, OECD Publishing, Paris, https://dx.doi.org/10.1787/eco_surveys-aus-2018-en.
[40] OECD (2018), OECD Tourism Trends and Policies 2018, OECD Publishing, Paris, https://dx.doi.org/10.1787/tour-2018-en.
[28] Roberts, J. (2020), Will COVID-19 mark the rise of automation?, https://australiascience.tv/will-covid-19-mark-the-rise-of-automation/ (accessed on 11 June 2020).
[17] Timmer, M. et al. (2014), “Slicing Up Global Value Chains”, Journal of Economic Perspectives, Vol. 28/2, pp. 99-118, http://dx.doi.org/10.1257/jep.28.2.99.
Annex 2.A. Jobs at risk of automation
Annexe Table 2.A.1. Share of jobs facing significant risk of change, high risk of automation, and average risk of automation
Occupation code (ISCO-08) |
Occupation title |
Significant risk of change |
High risk of automation |
Average risk of automation |
---|---|---|---|---|
11 |
Chief Executives, Senior Officials and Legislators |
1.5% |
0.0% |
26.3% |
12 |
Administrative and Commercial Managers |
5.9% |
1.6% |
30.4% |
13 |
Production and Specialized Services Managers |
4.0% |
1.0% |
27.3% |
14 |
Hospitality, Retail and Other Services Managers |
10.1% |
0.2% |
31.8% |
21 |
Science and Engineering Professionals |
12.8% |
7.0% |
38.3% |
22 |
Health Professionals |
11.6% |
1.1% |
29.2% |
23 |
Teaching Professionals |
1.7% |
0.0% |
23.1% |
24 |
Business and Administration Professionals |
14.6% |
1.0% |
34.0% |
25 |
Information and Communications Technology Professionals |
18.9% |
4.8% |
36.7% |
26 |
Legal, Social and Cultural Professionals |
15.1% |
2.5% |
33.7% |
31 |
Science and Engineering Associate Professionals |
25.7% |
7.9% |
38.0% |
32 |
Health Associate Professionals |
19.1% |
3.8% |
37.3% |
33 |
Business and Administration Associate Professionals |
18.3% |
3.9% |
36.7% |
34 |
Legal, Social, Cultural and Related Associate Professionals |
16.3% |
5.3% |
35.7% |
35 |
Information and Communications Technicians |
20.1% |
6.7% |
42.9% |
41 |
General and Keyboard Clerks |
39.6% |
24.0% |
54.9% |
42 |
Customer Services Clerks |
33.4$ |
9.6% |
44.6% |
43 |
Numerical and Material Recording Clerks |
33.9% |
13.4% |
47.0% |
44 |
Other Clerical Support Workers |
40.5% |
20.2% |
52.8% |
51 |
Personal Service Workers |
35.2% |
14.3% |
48.6% |
52 |
Sales Workers |
37.2% |
13.3% |
49.6% |
53 |
Personal Care Workers |
21.2% |
5.6% |
35.8% |
54 |
Protective Services Workers |
19.7% |
6.5% |
37.8% |
61 |
Market-oriented Skilled Agricultural Workers |
40.4% |
11.1% |
48.6% |
71 |
Building and Related Trades Workers (excluding Electricians) |
32.2% |
15.9% |
47.5% |
72 |
Metal, Machinery and Related Trades Workers |
40.2% |
11.7% |
46.0% |
73 |
Handicraft and Printing Workers |
50.4% |
32.0% |
59.8% |
74 |
Electrical and Electronic Trades Workers |
18.8% |
7.5% |
40.1% |
75 |
Food Processing, Woodworking, Garment and Other Craft and Related Trades Workers |
40.0% |
14.5% |
52.2% |
81 |
Stationary Plant and Machine Operators |
39.4% |
32.1% |
58.4% |
83 |
Drivers and Mobile Plant Operators |
44.4% |
20.8% |
54.9% |
91 |
Cleaners and Helpers |
54.4% |
20.5% |
56.7% |
92 |
Agricultural, Forestry and Fishery Labourers |
45.1% |
10.4% |
53.6% |
93 |
Labourers in Mining, Construction, Manufacturing and Transport |
32.9% |
31.3% |
54.6% |
94 |
Food Preparation Assistants |
32.7% |
48.7% |
62.7% |
96 |
Refuse Workers and Other Elementary Workers |
24.6% |
28.8% |
53.6% |
Source: OECD calculations on PIAAC.
Annex Figure 2.A.1. Share of jobs facing risk significant change or high risk of automation in the Australian Capital Territory

Source: OECD calculations on PIAAC and 2016 Australian Census.
Annexe Table 2.A.2. Number and share of jobs facing risk of significant change or high risk of automation in the Australian Capital Territory
Region |
Total employment (2016) |
Share of jobs at high risk of automation |
Number of jobs at high risk of automation |
Share of jobs at significant risk of change |
Number of jobs vulnerable to significant change |
Total share of jobs at risk of automation |
Total number of jobs at risk of automation |
---|---|---|---|---|---|---|---|
Australian Capital Territory |
197 000 |
8.0% |
16 000 |
21.3% |
42 000 |
29.3% |
58 000 |
Note: numbers are rounded to the nearest ‘000.
Source: OECD calculations on PIAAC and 2016 Australian Census.
Annexe Figure 2.A.2. Share of jobs facing risk of significant change or high risk of automation across New South Wales

Source: OECD calculations on PIAAC and 2016 Australian Census.
Annexe Table 2.A.3. Number and share of jobs facing risk of significant change or high risk of automation across New South Wales
Region |
Total employment (2016) |
Share of jobs at high risk of automation |
Number of jobs at high risk of automation |
Share of jobs at significant risk of change |
Number of jobs vulnerable to significant change |
Total share of jobs at risk of automation |
Total number of jobs at risk of automation |
---|---|---|---|---|---|---|---|
Hunter Valley exc. Newcastle |
108 000 |
12.2% |
13 000 |
28.0% |
30 000 |
40.2% |
43 000 |
Sydney - South West |
155 000 |
12.3% |
19 000 |
27.3% |
42 000 |
39.6% |
61 000 |
Riverina |
67 000 |
11.2% |
8 000 |
27.2% |
18 000 |
38.4% |
26 000 |
Central West |
84 000 |
11.3% |
10 000 |
26.9% |
23 000 |
38.2% |
32 000 |
Sydney - Outer South West |
119 000 |
11.5% |
14 000 |
26.5% |
32 000 |
38.1% |
45 000 |
New England and North West |
74 000 |
11.0% |
8 000 |
27.0% |
20 000 |
37.9% |
28 000 |
Murray |
49 000 |
10.9% |
5 000 |
26.9% |
13 000 |
37.8% |
18 000 |
Far West and Orana |
45 000 |
10.9% |
5 000 |
26.7% |
12 000 |
37.6% |
17 000 |
Sydney - Blacktown |
149 000 |
11.4% |
17 000 |
26.2% |
39 000 |
37.6% |
56 000 |
Mid North Coast |
73 000 |
11.0% |
8 000 |
26.4% |
19 000 |
37.4% |
27 000 |
Coffs Harbour - Grafton |
51 000 |
10.9% |
6 000 |
26.3% |
13 000 |
37.2% |
19 000 |
Sydney - Outer West and Blue Mountains |
144 000 |
11.0% |
16 000 |
25.8% |
37 000 |
36.8% |
53 000 |
Southern Highlands and Shoalhaven |
55 000 |
10.7% |
56 000 |
25.9% |
14 000 |
36.6% |
20 000 |
Richmond - Tweed |
94 000 |
10.6% |
10 000 |
25.9% |
24 000 |
36.5% |
34 000 |
Capital Region |
94 000 |
10.5% |
10 000 |
25.9% |
24 000 |
36.4% |
34 000 |
Central Coast |
137 000 |
10.6% |
15 000 |
25.4% |
35 000 |
36.0% |
49 000 |
Sydney - Inner South West |
241 000 |
10.7% |
26 000 |
25.2% |
61 000 |
35.9% |
87 000 |
Illawarra |
125 000 |
10.4% |
13 000 |
24.9% |
31 000 |
35.4% |
44 000 |
Sydney - Parramatta |
186 000 |
10.4% |
19 000 |
24.7% |
46 000 |
35.1% |
65 000 |
Newcastle and Lake Macquarie |
157 000 |
10.2% |
16 000 |
24.7% |
39 000 |
34.9% |
55 000 |
Sydney - Sutherland |
110 000 |
8.7% |
10 000 |
22.4% |
25 000 |
31.1% |
34 000 |
Sydney - Baulkham Hills and Hawkesbury |
113 000 |
8.2% |
9 000 |
21.7% |
25 000 |
29.9% |
34 000 |
Sydney - Northern Beaches |
127 000 |
7.7% |
10 000 |
20.9% |
27 000 |
28.6% |
36 000 |
Sydney - Inner West |
147 000 |
7.6% |
11 000 |
20.6% |
30 000 |
28.2% |
41 000 |
Sydney - City and Inner South |
170 000 |
7.5% |
13 000 |
20.5% |
35 000 |
28.1% |
48 000 |
Sydney - Ryde |
87 000 |
7.5% |
6 000 |
20.6% |
18 000 |
28.1% |
24 000 |
Sydney - Eastern Suburbs |
133 000 |
6.5% |
9 000 |
19.0% |
25 000 |
25.5% |
34 000 |
Sydney - North Sydney and Hornsby |
202 000 |
6.1% |
12 000 |
18.5% |
37 000 |
24.6% |
50 000 |
Note: numbers are rounded to the nearest ‘000.
Source: OECD calculations on PIAAC and 2016 Australian Census.
Annexe Figure 2.A.3. Share of jobs facing risk of significant change or high risk of automation across the Northern Territory

Source: OECD calculations on PIAAC and 2016 Australian Census.
Annexe Table 2.A.4. Number and share of jobs facing risk of significant change or high risk of automation across the Northern Territory
Region |
Total employment (2016) |
Share of jobs at high risk of automation |
Number of jobs at high risk of automation |
Share of jobs at significant risk of change |
Number of jobs vulnerable to significant change |
Total share of jobs at risk of automation |
Total number of jobs at risk of automation |
---|---|---|---|---|---|---|---|
Darwin |
68 000 |
10.1% |
7 000 |
24.8% |
17 000 |
34.9% |
24 000 |
Northern Territory - Outback |
29 000 |
9.6% |
3 000 |
24.0% |
7 000 |
33.6% |
10 000 |
Note: numbers are rounded to the nearest ‘000.
Source: OECD calculations on PIAAC and 2016 Australian Census.
Annexe Figure 2.A.4. Share of jobs facing risk of significant change or high risk of automation across Queensland

Source: OECD calculations on PIAAC and 2016 Australian Census.
Annexe Table 2.A.5. Number and share of jobs facing risk of significant change or high risk of automation across Queensland
Region |
Total employment (2016) |
Share of jobs at high risk of automation |
Number of jobs at high risk of automation |
Share of jobs at significant risk of change |
Number of jobs vulnerable to significant change |
Total share of jobs at risk of automation |
Total number of jobs at risk of automation |
---|---|---|---|---|---|---|---|
Mackay - Isaac - Whitsunday |
77 000 |
12.6% |
10 000 |
28.6% |
22 000 |
41.2% |
32 000 |
Logan - Beaudesert |
134 000 |
12.3% |
17 000 |
27.9% |
38 000 |
40.2% |
54 000 |
Darling Downs - Maranoa |
54 000 |
11.6% |
6 000 |
28.6% |
15 000 |
40.2% |
22 000 |
Central Queensland |
96 000 |
12.1% |
12 000 |
27.9% |
27 000 |
40.0% |
38 000 |
Moreton Bay - North |
93 000 |
12.0% |
11 000 |
27.7% |
26 000 |
39.7% |
37 000 |
Queensland - Outback |
33 000 |
11.6% |
4 000 |
27.8% |
9 000 |
39.4% |
13 000 |
Wide Bay |
98 000 |
11.6% |
11 000 |
27.7% |
27 000 |
39.3% |
38 000 |
Ipswich |
133 000 |
11.8% |
16 000 |
27.4% |
36 000 |
39.2% |
52 000 |
Townsville |
99 000 |
11.1% |
11 000 |
26.3% |
26 000 |
37.4% |
37 000 |
Cairns |
104 000 |
10.8% |
11 000 |
26.5% |
27 000 |
37.2% |
39 000 |
Toowoomba |
65 000 |
10.8% |
7 000 |
26.1% |
17 000 |
36.8% |
24 000 |
Sunshine Coast |
149 000 |
10.3% |
15 000 |
25.3% |
38 000 |
35.75 |
53 000 |
Gold Coast |
262 000 |
10.3% |
27 000 |
25.1% |
66 000 |
35.4% |
93 000 |
Brisbane - East |
106 000 |
10.3% |
11 000 |
24.8% |
26 000 |
35.1% |
37 000 |
Moreton Bay - South |
94 000 |
10.2% |
10 000 |
24.7% |
23 000 |
35.0% |
33 000 |
Brisbane - North |
102 000 |
9.6% |
10 000 |
23.7% |
24 000 |
33.4% |
34 000 |
Brisbane - South |
165 000 |
9.2% |
15 000 |
22.9% |
38 000 |
32.1% |
53 000 |
Brisbane - West |
85 000 |
7.6% |
6 000 |
20.4% |
17 000 |
28.0% |
24 000 |
Brisbane Inner City |
138 000 |
7.45 |
10 000 |
20.3% |
28 000 |
27.7% |
38 000 |
Note: numbers are rounded to the nearest ‘000.
Source: OECD calculations on PIAAC and 2016 Australian Census.
Annexe Figure 2.A.5. Share of jobs facing risk of significant change or high risk of automation across South Australia

Source: OECD calculations on PIAAC and 2016 Australian Census.
Annexe Table 2.A.6. Number and share of jobs facing risk of significant change or high risk of automation
Region |
Total employment (2016) |
Share of jobs at high risk of automation |
Number of jobs at high risk of automation |
Share of jobs at significant risk of change |
Number of jobs vulnerable to significant change |
Total share of jobs at risk of automation |
Total number of jobs at risk of automation |
---|---|---|---|---|---|---|---|
South Australia - South East |
76 000 |
11.8% |
9 000 |
28.6% |
22 000 |
40.3% |
31 000 |
Barossa - Yorke - Mid North |
45 000 |
11.7% |
5 000 |
27.8% |
12 000 |
39.5% |
18 000 |
South Australia - Outback |
33 000 |
11.6% |
4 000 |
27.7% |
9 000 |
39.3% |
13 000 |
Adelaide - North |
176 000 |
11.8% |
21 000 |
27.1% |
48 000 |
38.9% |
68 000 |
Adelaide - West |
103 000 |
10.5% |
11 000 |
24.9% |
26 000 |
35.4% |
36 000 |
Adelaide - South |
162 000 |
9.9% |
16 000 |
24.3% |
39 000 |
34.2% |
55 000 |
Adelaide - Central and Hills |
135 000 |
8.2% |
11 000 |
21.5% |
29 000 |
29.7% |
40 000 |
Note: numbers are rounded to the nearest ‘000.
Source: OECD calculations on PIAAC and 2016 Australian Census.
Annexe Figure 2.A.6. Share of jobs facing risk of significant change or high risk of automation across Tasmania

Source: OECD calculations on PIAAC and 2016 Australian Census.
Annexe Table 2.A.7. Number and share of jobs facing risk of significant change or high risk of automation
Region |
Total employment (2016) |
Share of jobs at high risk of automation |
Number of jobs at high risk of automation |
Share of jobs at significant risk of change |
Number of jobs vulnerable to significant change |
Total share of jobs at risk of automation |
Total number of jobs at risk of automation |
---|---|---|---|---|---|---|---|
West and North West |
43 000 |
12.1% |
5 000 |
28.2% |
12 000 |
40.2% |
17 000 |
South East |
14 000 |
11.4% |
2 000 |
28.2% |
4 000 |
39.7% |
6 000 |
Launceston and North East |
57 000 |
11.0% |
6 000 |
26.6% |
15 000 |
37.5% |
21 000 |
Hobart |
97 000 |
10.0% |
10 000 |
24.5% |
24 000 |
34.5% |
33 000 |
Note: numbers are rounded to the nearest ‘000.
Source: OECD calculations on PIAAC and 2016 Australian Census.
Annexe Figure 2.A.7. Share of jobs facing risk of significant change or high risk of automation across Victoria

Source: OECD calculations on PIAAC and 2016 Australian Census.
Annexe Table 2.A.8. Number and share of jobs facing significant risk of change or high risk of automation
Region |
Total employment (2016) |
Share of jobs at high risk of automation |
Number of jobs at high risk of automation |
Share of jobs at significant risk of change |
Number of jobs at significant risk of change |
Total share of jobs at risk of automation |
Total number of jobs at risk of automation |
---|---|---|---|---|---|---|---|
Shepparton |
53 000 |
11.3% |
6 000 |
27.3% |
15 000 |
38.6% |
21 000 |
Warrnambool and South West |
54 000 |
11.2% |
6 000 |
27.2% |
15 000 |
38.4% |
21 000 |
Latrobe - Gippsland |
108 000 |
11.2% |
12 000 |
26.9% |
29 000 |
38.0% |
41 000 |
North West |
62 000 |
10.9% |
7 000 |
27.1% |
17 000 |
38.0% |
24 000 |
Hume |
73 000 |
11.1% |
8 000 |
26.6% |
19 000 |
37.7% |
28 000 |
Melbourne - South East |
329 000 |
11.3% |
37 000 |
26.1% |
86 000 |
37.5% |
123 000 |
Melbourne - West |
319 000 |
11.2% |
36 000 |
25.9% |
83 000 |
37.1% |
118 000 |
Melbourne - North West |
153 000 |
11.0% |
17 000 |
25.6% |
39 000 |
36.6% |
56 000 |
Ballarat |
66 000 |
10.7% |
7 000 |
25.7% |
17 000 |
36.3% |
24 000 |
Bendigo |
65 000 |
10.7% |
7 000 |
25.4% |
17 000 |
36.1% |
23 000 |
Mornington Peninsula |
128 000 |
10.4% |
13 000 |
25.2% |
32 000 |
35.6% |
45 000 |
Geelong |
123 000 |
10.3% |
13 000 |
24.9% |
30 000 |
35.2% |
43 000 |
Melbourne - North East |
227 000 |
10.0% |
23 000 |
24.3% |
55 000 |
34.3% |
78 000 |
Melbourne - Outer East |
244 000 |
9.8% |
24 000 |
24.0% |
59 000 |
33.8% |
82 000 |
Melbourne - Inner South |
195 000 |
7.3% |
14 000 |
20.2% |
39 000 |
27.6% |
54 000 |
Melbourne - Inner East |
166 000 |
7.2% |
12 000 |
20.0% |
33 000 |
27.3% |
45 000 |
Melbourne - Inner |
315 000 |
6.9% |
22 000 |
19.5% |
61 000 |
26.5% |
83 000 |
Note: numbers are rounded to the nearest ‘000.
Source: OECD calculations on PIAAC and 2016 Australian Census.
Annexe Figure 2.A.8. Share of jobs facing risk of significant change or high risk of automation across Western Australia

Source: OECD calculations on PIAAC and 2016 Australian Census.
Annexe Table 2.A.9. Number and share of jobs facing risk of significant change or high risk of automation across Western Australia
Region |
Total employment (2016) |
Share of jobs at high risk of automation |
Number of jobs at high risk of automation |
Share of jobs at significant risk of change |
Number of jobs at significant risk of change |
Total share of jobs at risk of automation |
Total number of jobs at risk of automation |
---|---|---|---|---|---|---|---|
Western Australia - Outback (South) |
52 000 |
12.1% |
6 000 |
28.0% |
14 000 |
40.0% |
21 000 |
Western Australia - Outback (North) |
44 000 |
12.2% |
5 000 |
27.7% |
12 000 |
39.9% |
18 000 |
Mandurah |
36 000 |
12.1% |
4 000 |
27.7% |
10 000 |
39.8% |
14 000 |
Bunbury |
76 000 |
11.7% |
9 000 |
27.6% |
21 000 |
39.4% |
30 000 |
Western Australia - Wheat Belt |
58 000 |
11.1% |
6 000 |
27.8% |
16 000 |
38.9% |
22 000 |
Perth - North East |
117 000 |
11.0% |
13 000 |
26.0% |
31 000 |
37.0% |
43 000 |
Perth - South East |
224 000 |
10.9% |
25 000 |
25.8% |
58 000 |
36.7% |
82 000 |
Perth - South West |
184 000 |
10.1% |
19 000 |
24.6% |
45 000 |
34.75 |
64 000 |
Perth - North West |
257 000 |
10.0% |
26 000 |
24.4% |
63 000 |
34.45 |
88 000 |
Perth - Inner |
83 000 |
7.0% |
6 000 |
19.2% |
16 000 |
26.2% |
22 000 |
Note: numbers are rounded to the nearest ‘000.
Source: OECD calculations on PIAAC and 2016 Australian Census.