Making effective use of data and analytics requires a strategy with clear goals and objectives that promote a coherent approach at all levels of the institution, as well as continuous learning to ensure impact. This chapter explores what it means for the ASF to take a “strategic approach” to data and analytics, focusing on assessing integrity risks. It covers both objective-setting at the institutional level, as well as the need for plans for the ASF to monitor its investment in analytics, drawing inspiration from other SAIs and OECD member countries.
Strengthening Analytics in Mexico’s Supreme Audit Institution
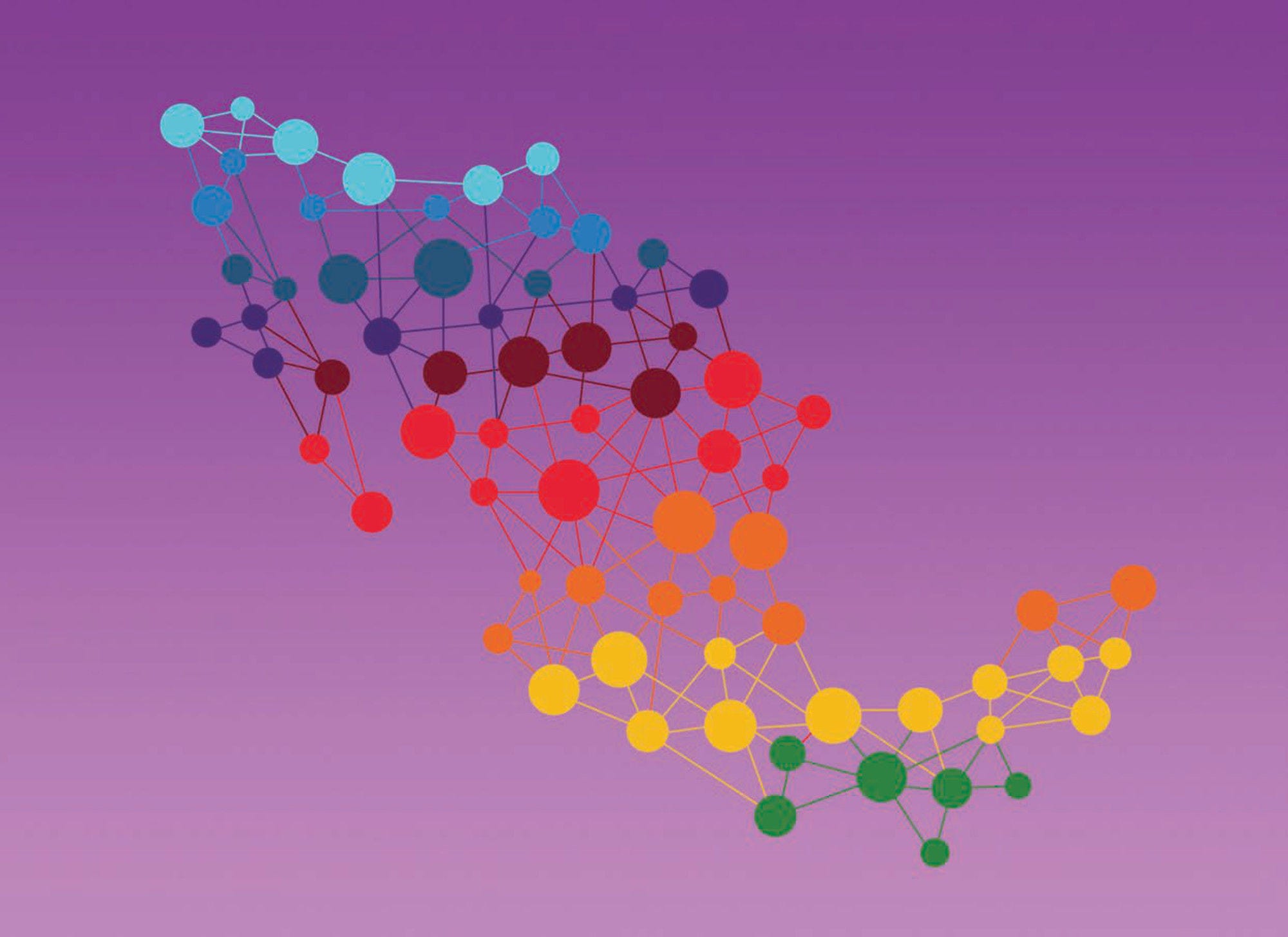
1. Strategic considerations for Mexico’s supreme audit institution to advance analytics
Abstract
1.1. Introduction
Making effective use of data and analytics requires more than simply introducing new tools, technologies or data sources to the work of audit institutions. It requires a strategy, with clear goals and objectives that all levels of the Supreme Audit Institution (SAI), particularly line managers and those charged with strategy implementation, are aware of and can support. A strategy helps the leadership of SAIs to be effective stewards of taxpayer money by ensuring that clear objectives guide investments and decisions. A strategy also provides incentives for continuous learning and aligning of data and analytics to long-term goals. Data and analytics serve institutional goals. Defining these goals and articulating them is a critical step in an organisation’s digital transformation and instilling a culture that promotes decision-driven analytics as opposed to data-driven decision making (MIT, 2020[1]).
For Mexico’s Superior Audit of the Federation (Auditoria Superior de la Federación, ASF), the digital strategy is best reflected in its digital transformation work programme, published in September 2020. The programme emphasises technical goals and objectives for equipping auditors with the infrastructure, architecture, skills and tools needed to effectively audit in a digital environment. By design, the programme focuses on many ASF-wide priorities, demonstrating the ASF’s commitment internally and externally to invest in its own modernisation to keep pace with the digital transformation occurring across the Mexican government and among peer SAIs. Moreover, the COVID-19 pandemic further highlighted the importance of ASF’s digital transformation, as reflected in different initiatives to enhance capacity for auditing remotely.
The digital transformation work programme sets the tone and a solid foundation for many activities. The document describing the programme notes that data can ultimately be used for real-time monitoring or for the use of artificial intelligence in auditing. However, it does not elaborate specifically on a key area of the ASF’s investment—data and analytics for preventing and detecting irregularities.1 As described in this report, the ASF has already developed several initiatives to collect data and assess integrity risks in support of its audits and investigations. However, without a clear institution-wide, unified strategy and objectives for leveraging data and analytics in this critical area of the ASF’s activities, the institutional investments and efforts in terms of data and analytics are at risk of being siloed, ad hoc and inefficient. This chapter explores the need for the ASF to enhance its “strategic approach” to data and analytics for assessing integrity risks in terms of both objective-setting at the institutional level as well as putting in place the plans for the ASF to monitor its investment in analytics, drawing inspiration from other SAIs and OECD member countries.2
1.2. Setting the foundations for effective use of analytics for assessing integrity risks
The International Organization of Supreme Audit Institutions (INTOSAI) promotes the modernisation of SAIs through the issuing of standards and guidance that emphasise the critical role of data for supporting SAIs’ missions. Specifically, INTOSAI calls on SAIs to take a strategic approach to how they use data. Several working groups and partners of INTOSAI, such as the Working Group on Information Technology Auditing and the INTOSAI Development Initiative, have issued practical guidance on how SAIs can accomplish this. Guidance for combating fraud and corruption also highlights the critical role that data and analytics can play, and the suggested policies, practices and tools for leveraging data in support of this specific objective. There is no single definition of “analytics” (used as shorthand for “data analytics” in this report). Analytics can be seen as the computation process of exploratory and confirmatory “data analysis”, including data collection, cleansing, analysing and deploying (INTOSAI, 2019[2]). This report adopts this broad definition and conceptualisation of analytics given the relevance for the ASF and the focus of the project.
Box 1.1. Analytics guidelines of the INTOSAI Working Group on IT Audit
Analytics is a broad field, and even among supreme audit institutions, practices can vary widely depending on desired outcomes, available raw data, and context. However, the INTOSAI Working Group on IT Audit, of which the ASF is a member, has developed a series of general guidelines to bear in mind when using analytics to support audit work. These recommendations are subdivided into five chronological steps per INTOSAI (Figure 1.1).
Initial stage: Identify the target or the objective, and a potential source of data to be used.
Data readiness: Take a careful look at the potential data– cleanse it, and check for data reliability.
Analytics creation: Decide how the data, once prepared, will be analysed. There are a number of different type of analytics methodologies, and it is critical the right one is selected for any specific project:
Descriptive analytics merely summarise the data available into simple percentages or fractions.
Diagnostic analytics are conducted to find out why something happened, or why something did not happen the way it should have.
Predictive analytics use data to estimate the likelihood of future events. Multiple linear regression or machine learning are well known examples. If a Machine Learning model is ultimately selected, an additional step known as training is also required at this stage. In training, the programme is taught what to look for and how to evaluate findings.
Analytics deployment: Apply the analytics programme to the cleansed and ready data. This step could be a one-off, frequent, or continuous, depending on the programme selected.
Business intelligence: Apply what was learned through the analytics programme to reporting and decision making.
Figure 1.1. INTOSAI’s Data Analytics Process
The ASF’s investment in its digital transformation aligns with broader trends in the Executive Branch of the federal government in recent years. In July 2019, the Federal Official Gazette (Diario Oficial de la Federación) published Mexico’s 2019-2024 National Development Plan (Plan Nacional de Desarrollo, PND), which sets out the national objectives, strategy, and priorities for Mexico’s development. From 2013 to 2018, the federal government pursued a National Digital Strategy (Estrategia Digital Nacional, EDN) that recognised the strategic value of digitalisation for the public sector and society in general (Government of Mexico, 2013[3]). The government did not implement a new strategy after 2018, although the National Digital Strategy Office (Coordinación de la Estrategia Digital Nacional, CEDN) at the Office of the President in Mexico is considering a new one (Coordination of the National Digital Strategy, Government of Mexico, 2021[4]). The “National Programme to Combat Corruption and Impunity, and to Improve Public Management” for 2019-2024—led by SFP, CEDN and the Ministry of Finance and Public Credit (Secretaría de Hacienda y Crédito Público, SHCP)—provides strategic direction and priorities that focus specifically on anti-corruption.
While Mexico lacks an updated National Digital Strategy, the National Development Plan and anti-corruption programmes provide direction for the strategic use of data. Data governance is at the core of many of the principles and policies that the Mexican government has adopted in recent years. Robust data governance promotes integration and systemic coherence, offering a common basis for organisations to effectively use data for a range of policy goals, including combatting corruption and fraud. The model described in Figure 1.2 highlights the values of all organisational, policy and technical aspects for successful data governance.
Figure 1.2. Aspects for successful data governance
The data governance model above is relevant from both a whole-of-government and institutional perspective. For audit institutions, data governance and data management are at the forefront of their everyday work. INTOSAI’s Moscow Declaration calls for SAIs to make better use of data and analytics in audits, including “adaptation strategies, such as planning for such audits, developing experienced teams for data analytics, and introducing new techniques into the practice of public audit” (INTOSAI, 2019[6]). Moreover, various INTOSAI regional and working groups, as well as individual SAIs, have raised the need for effective data governance as the “what” and “how” of auditing evolves with the digitalisation of government.3 Government entities beyond SAIs are grappling with the same issues and developing their own data governance framework (see the example from New Zealand in Box 1.2). The model in Figure 1.3 captures common principles and needs across these sources, including:
The strategic layer demonstrated that data strategies are a critical element of good data governance. Data strategies enable accountability and can help define leadership, expectations, roles and goals. The strategic layer also highlights how the formulation of data policies and/or strategies can benefit from open and participatory processes, thus integrating the inputs of actors from within ASF and externally.
The tactical layer enables the coherent implementation and steering of data policies, strategies and initiatives. It draws upon the value of auditors’ skills and competencies, and highlights people-centred activities like recruitment, communication, co-ordination, and collaboration as instruments for extracting value from data assets. It also takes into account the importance of formal and informal institutional networks, such as communities of practice. The tactical layer also includes data-related legislation and regulations that help to define and ensure compliance with the rules and policies guiding data management, including data openness, protection and sharing.
The delivery layer covers the day-to-day implementation of data strategies. It touches on different technical and policy aspects of the data value cycle across its different stages (from data production to reuse), the role and interaction of different actors in each stage (e.g. as data providers), and the inter-connection of data flows across stages. The adoption of technological solutions takes place in this layer with a linkage to strategic goals and objectives. It also relates, for instance, to the need for re-engineering legacy infrastructure, architecture and data management practices and processes. Addressing issues of data interoperability and standardisation also takes place at this level.
In interviews, ASF officials highlighted aspects of all six key elements—leadership and vision; capacity; regulation; data value cycle; data infrastructure; and data architecture—as challenges. In particular, several strategic priorities including establishing a unified vision for analytics for integrity, collaborating more closely with other institutions, and devising a cohesive action plan came to the forefront during fact-finding interviews with the OECD, as discussed below. These priorities are critical because they are the foundation for effective and efficient use of analytics that avoids creating data siloes and promotes efficiency across ASF on cross-cutting activities that are resource intensive, such as data management and cleaning. The subsequent sections take inspiration from these elements as a framework for identifying areas for ASF to improve its own approach to data and analytics for detecting integrity risks.
Box 1.2. Data Governance Framework of New Zealand
The leading agency for government-held data in New Zealand (Stats NZ) developed a new and improved data governance framework for the New Zealand government. The framework is part of the agency’s numerous efforts to promote better data management practices across the public sector, and to leverage data as a strategic asset for decision making. One of the central pillars of the framework is the adoption of a so-called “whole-of-data life cycle approach”, meaning public bodies and employees are encouraged to think more strategically about the governance, management, quality and accountability of their data, over the whole data life cycle (i.e. from the design and source of the data to its storing, publication and disposal).
Figure 1.3. Towards a holistic data governance framework
1.3. Approaching analytics with a strategic mindset
1.3.1. Establishing a unified vision for analytics
While the National Development Plan provides broader goals for government to navigate digital transformation, and ASF’s role in the NACS provides some inspiration, ASF can set objectives for digital transformation and analytics at its discretion. ASF’s strategic plan for 2018-2026 consists of 29 objectives that map across a theory of change for contributing to good governance and accountability in the public sector, as well as positioning ASF as a national and international example of high quality technical expertise. One of the main value propositions of ASF described in its strategic plan is to raise awareness among audited entities about the risks of irregularities. The plan also emphasises the need for risk analyses, “leading” technologies to support ASF’s functions, and the development of technical capabilities and the specialisation of staff (ASF, 2018[7]). However, the strategy does not articulate clear goals or objectives that set a unified vision or guide for teams within the ASF that have developed independent initiatives for using data and analytics to assess integrity risks.
Likewise, the ASF’s digital work programme does not provide a unified vision, objectives or direction. In addition to the strategic plan, ASF’s General Directorate of Systems (Dirección General de Sistemas, DGS) developed a work programme to guide the digital transformation. The DGS sits within the General Administration Unit (Unidad General de Administración), and among other roles, has a strategic cross-cutting function to establish IT standards, policies and systems within the ASF, as well as implement technical tools and provide technical assistance internally (Government of Mexico, 2021[8]). The digital transformation programme elaborates a multi-dimensional objective to:
Develop, regulate and implement projects of substantive and procedural processes with automated flows that consider inputs and outputs in digital format, from robotic automation, electronic signature and signature of the Tax Administration System (SAT) and time stamps of the Ministry of Economy (SE), to the storage of information and critical transactions in blockchain in order to promote the digital transformation in the Superior Audit of the Federation that ensures the availability of "state-of-the-art” technologies to strengthen the institution, stay at the forefront in use of existing technology and enhance the results of the audit of public resources (ASF, 2020[9]).
The programme also highlights several projects that are relevant for analytics, including the development of a central data warehouse, automation of data processes and information flow, and the digitalisation of the ASF’s audit process through the development of a Digital Mailbox (Buzón Digital) (ASF, 2020[9]).
The ASF’s strategy and digital work programme broadly allude to analytics and integrity risks, but they do not outline objectives that link analytics for identifying irregularities. Data and analytics enable teams across the ASF to achieve their objectives, and deliver on the institution’s broader value propositions as defined in its strategic plan. In interviews with senior ASF officials, establishing a unified vision for data and analytics, including one that reflects auditing and governance trends related to big data, was flagged as one of the ASF’s top strategic priorities. Addressing analytics at the strategic level could have a number of positive impacts on ASF’s digital transformation. For instance, in interviews, officials recognised that it would be useful to engage leadership, enhance co-ordination, promote data sharing internally and potentially facilitate the centralisation of key data activities (see section below). It could also help leadership to establish experimentation as a strategic objective, and frame efforts to innovate as part of the ASF’s culture and management’s commitment to invest in auditors’ skills and tools (see Chapter 2). Box 1.3 illustrates how the Australian National Audit Office (ANAO) addresses data and analytics in its corporate plan, which is the ANAO’s core document for setting its vision and strategic directions.
Box 1.3. The Australian National Audit Office’s strategic priorities for data and analytics
Taking a unified, cohesive, and strategic approach on data analytics is critically important for audit institutions hoping to maximise resources, improve decision making and achieve the organisation’s goals. With this in mind, the Australian National Audit Office (ANAO)’s yearly corporate plan sets out a clear and succinct framework for how data analytics and technology will be used at the organisation, the specific and relevant processes in place, and which teams across the organisation are responsible for each aspect. More specifically, the document identifies a stand-alone team dedicated to analytics, key areas in which the Office would like to use data to better meet its objectives, and ways in which it would like to improve data governance practices. The importance of data analytics to the organisation’s work is also highlighted by its mention in other related sections of the corporate plan, including those on human capital, budgeting and productivity.
1.3.2. Creating an action plan for analytics
The ASF’s approach to analytics can be characterised as bottom-up, with specialised areas developing capacities, tools and processes that serve their individual mandates, which include assessing integrity risks. Nonetheless, as noted above, the ASF does not have clearly defined objectives or processes for identifying integrity risks through analytics, and those that are in place are not unified across teams. Its expertise in data and analytics for this purpose is spread across four different departments—“Superior Audits”—and their General Directorates (Direcciones Generales, DG). This includes the Special Audit of Financial Compliance (Auditoría Especial de Cumplimiento Financiero, AECF) and within it, the General Directorate of Forensic Audits (Dirección General de Auditoría Forense, DGAF) and the General Directorate of Audit of Information and Communications Technology (Dirección General de Auditoría de Tecnologías de Información y Comunicaciones, DGATIC). These DGs have concentrated resources of data and analytics experts.
In addition, expertise in analytics can be found in other departments that have developed their own capacities independently, including the Special Audit of Federal Spending (Auditoría Especial de Gasto Federalizado, AEGF), as well as the Special Audit of Performance (Auditoría Especial de Desempeño, AED). In late August 2021, ASF issued amended internal regulations that introduced a DG under the auspices of the AEGF, called the DG of Forensic Audit and Federal Spending (Dirección General de Auditoría Forense del Gasto Federalizado, DGAFGF).4 The fourth department-level team in the ASF, the Special Audit of Monitoring, Reporting and Investigation (Auditoría Especial de Seguimiento, Informes e Investigación, AESII) plays a more indirect role, as it supports the follow-up of audits and referrals that use the analytics produced by other departments.
Both the AECF and the AEGF have also developed independent systems and approaches to analytics to support auditors within their respective departments, detailed further in Chapter 2. These systems leverage different databases, but they share many of the same processes and challenges concerning data management, and even share some of the same data sources. The AECF has also developed innovative applications of new technologies to support the ASF’s auditing across the institution. This includes the use of satellite imagery to enhance remote oversight, such as audits of infrastructure projects. It also involves the use of geographic information systems and geo-referencing tools to inform analysis of territorial gaps in auditing and organise the results of audits over the last 20 years to allow for filtering by geography. The AECF envisioned the enhancement of new tools in 2021, such as the use of drones to facilitate real-time auditing of public works and the development of dashboards with key performance indicators. The AECF’s work not only has wide implications for other departments in the ASF, but it is also addressing needs related to improving the use of data and analytics for detecting fraud, as discussed in Chapter 2.
As the name suggests, the mission of the DGAF is to conduct forensic audits and investigations, and according to the DGAF officials, the DG makes use of data and forensic tools for all of the audits it conducts. The DGAF also hosts the ASF’s Forensic Laboratory (Laboratorio Forense), which collects, analyses and safeguards the digital evidence in adherence with regulations and chain of custody procedures (ASF, 2021[11]). The Forensic Laboratory is not a centralised data and analytics function, although some activities include data analysis and it does support other DGs primarily within the AECF. The main objectives of the Forensic Laboratory are to 1) obtain and safeguard digital evidence; 2) analyse physical storage devices or digital information that may be considered as evidence or support for any alleged irregularity and subsequent sanction procedures; and 3) provide analysis and support for investigations. Its activities include:
Creation of forensic images of electronic devices in order to preserve and ensure the integrity of the information.
Recovery and analysis of digital information focused on the review of different digital data sources through the application of specific software.
Data analyses, including data matching and other techniques.
Network analysis and visualisations to support investigations.
Assessment of counterfeit documentation and fraudulent imitation of text in electronic files.
Data management and copying information between physical storage devices, and if necessary, identifying possible errors linked to corrupt data or anomalies.
Secure deletion of physical storage devices (ASF, 2021[11]).
To complement the strategic plan and work programme on digital transformation, the ASF could devise an action plan that focuses on institutional objectives for leveraging data and analytics to enhance audit work. The ASF’s own work programme for digital transformation covers several critical areas that could be reflected in the action plan. For instance, the work programme includes analyses of the ASF’s strengths, weaknesses, opportunities and threats (SWOT) across key components of the entity’s digital transformation objectives. This includes analyses of the ASF’s objectives related to software development, technological infrastructure, telecommunications, computer services and systems operations. All of these areas influence the ability of auditors to make use of data in their work. What differentiates an action plan from this work programme is the ability to hone in on applications of data and analytics in specific areas of ASF’s activities, which in turn helps to tailor digital transformation to the goals and needs of different teams. One of these areas could be data and analytics for assessing integrity risks, filling a critical gap in the attention the ASF currently pays to fraud and corruption in its strategy and digital transformation work programme.
The European Court of Auditors (ECA) is undergoing its own digital transformation. This includes efforts to exercise greater automation in its audit procedures, employ algorithms to detect irregularities in digital documents and make use of artificial intelligence to detect performance patterns in large datasets (The European Court of Auditors, 2019[12]). According to the ECA, such innovations can make it so auditors focus more on asking the right questions rather than spending all their time on verification and analyses. As ASF advances its own digital transformation, an action plan will help the ASF to go beyond its current approach to identify objectives that are specific, measurable, achievable, relevant and time-based relative to specific areas. The ECA’s road map, with a focus on short, medium and long-term objectives, may serve as a model for the ASF to set priorities and frame its own path forward in the action plan. Implicit in this figure is the notion that the ASF could consider a phased approach to its enhancement of analytics over the period of several years. This is similar to what the UK National Audit Office (NAO) has done to deliver its own strategic transformation. In the first year, the NAO completed a detailed plan and started to procure new audit software and rolled out a cloud-based data platform (i.e. the Audit Information Management System), while developing a data-driven methodology to assess risks related to audited entities. In 2021‑22, the NAO will pilot the methodology and will implement it fully the following year (UK National Audit Office, 2021[13]).
Figure 1.4. The European Court of Auditor’s roadmap towards digital audit
The value of developing an action plan lies in large part in the process itself. Given their mandates, the Directorate General of Planning and Evaluation (Dirección General de Planeación y Evaluación) or the DGS, would likely take leadership roles in this effort. What is critical, regardless of which Directorate steers the action plan, is that the DGs have direct reporting lines to senior leadership and an institution-wide mandate. For instance, when the UK National Audit Office enhanced its analytics and established an internal data service, one of the key enablers of its efforts was the leveraging of senior leadership (UK National Audit Office, 2018[14]). However, development of the action plan would be a vehicle for engaging and gaining support from a wider range of actors, including the departments and teams that are responsible for data management and analytics across the ASF, as described below. A collective effort to develop an analytics action plan would help to break down siloes between the different departments and teams that are working with similar data and using comparable techniques, as well as facing similar challenges related to capacity and data access (see more on co-ordination in Chapter 2). The action plan would also help to encourage staff to consider how data can support the planning and execution of audits, and create an organisational culture around the subject. In addition to engaging leadership early and often, other considerations for the ASF in terms of the development process and content of an action plan, some of which are elaborated on in Chapter 2, include:
Using the data governance framework described above to help frame key areas to focus on and identify priorities.
Considering implications and relevance for the entire audit cycle, including planning, implementation and follow-up.
Defining clear objectives as part of the action plan so that purpose comes before the data, and not the other way around.
Accounting for internal context by making objective and activities tailored and fit-for-purpose for individual team’s within the ASF.
Promoting learning, inclusivity and experimentation when using data and new techniques.
Establishing indicators and a theory of change for the ASF with regards to data and analytics, focusing on outcomes and not simply outputs (e.g. indicators that reflect fraud loss prevention, mitigation or recoveries as a result of data and analytics).
Developing a baseline for measuring the impact of the ASF’s investments in analytics, and a starting point for making changes in response to internal and external factors, such as changes in funding or the need to respond to emerging risks in the context of COVID-19.
Accounting for the external context and stakeholders (e.g. the ASF’s own digital transformation work programme describes key external stakeholders that would be relevant and the COVID-19 crisis can help to shape priorities).
Brazil’s supreme audit institution (Tribunal de Contas da União, TCU), developed its own analytics capacity in a similar way, albeit with a more deliberate strategic decision to decentralise its analytics capacities to audit teams. Individual departments trained auditors in analytics, encouraged them to seek data and technological support tools that would be useful for their audits, and form communities of practice. To illustrate for the ASF how the TCU approached a similar task, Box 1.4 describes the three key pillars it chose to structure its data analysis strategy—governance, a platform and information-based solutions.
Box 1.4. Pillars of data and analytics strategy of Brazil’s supreme audit institution
Organisations strive to work effectively and efficiently, which can sometimes be alternatively articulated as doing better, but also choosing what is done better. This same idea can be applied to audit institutions, which, given their constrained resources, frequently face steep opportunity costs on project selection decisions. Analytics can make these decisions easier, but often the increasingly vast amount of information and seemingly infinite possibilities for analysis can hamper efforts towards efficiency and effectiveness. Thus, institutions must formalise and implement work processes to ensure that analytics are indeed improving the body’s work – that the information being used is necessary and sufficient to fill its purpose. Brazil’s Supreme Audit institution, the Tribunal de Contas da União (TCU), did this by focusing on the three following main pillars.
Governance: the guidelines, support and direction that come from top management, including a cohesive organisational strategy and a plan of action. This can help create a culture within the organisation that encourages the use of analytics for control activities.
Platform: the tangible tools needed to execute analytics-related tasks – to derive useful insights from raw data. For example, TCU created and manages a virtual environment named Labcontas. Labcontas allows auditors to easily and independently access information from dozens of databases as a benefit of agreements signed between public institutions. This makes it easier to co‑ordinate efforts, and reduces the time and resources often needed to obtain information during audits.
Information-based solutions: the tools and the high-level structures put in place must result in actionable, timely and reliable insights. This kind of information is used in Brazil for a range of audit-related tasks, from holding managers accountable to flagging potential high-risk grant or procurement proposals submitted to the government.
Source: (Revista do TCU, 2016[15]).
1.3.3. Engaging the National Digital Platform and the Ministry of Public Administration
In July 2016, the Mexican government signed into law the National Anti-Corruption System (Sistema Nacional Anticorrupción, NACS). The NACS forms part of a series of broader reforms for improved governance in Mexico, and is closely linked with complementary initiatives that established the National Auditing and National Transparency System (NAS and NTS, respectively). Together, these “systems” of accountability and integrity actors were conceived in order to strengthen anti-corruption, oversight and transparency measures in the Mexican government. The General Law of the NACS (Ley General del Sistema Nacional Anticorrupción) provides that a representative of a Citizen Participation Committee (CPC) presides over the system’s Co-ordination Committee and Governing Board, composed of the heads of the Ministry of Public Administration (Secretaría de Función Pública, SFP); the ASF; the Federal Tribunal of Administrative Justice (Tribunal Federal de Justicia Administrativa); the Specialised Anticorruption Prosecutor (Fiscalía Especializada en Combate a la Corrupción); the National Institute for Transparency, Access to Information and the Protection of Personal Data (Instituto Nacional de Transparencia, Acceso a la Información y Protección de Datos Personales); and a representative from the Federal Judicial Council (Consejo de la Judicatura Federal) (Government of Mexico, 2021[16]).
The General Law also established the National Digital Platform (Plataforma Digital Nacional, PDN). The goal of the platform is to support the work of the authorities of the NACS with new technologies, methodologies, data science and artificial intelligence (National Anti-Corruption System, 2021[17]). The PDN also aims to reduce siloes of information so that data can be comparable, accessible, and reusable (Executive Secretary of the National Anti-Corruption System, Mexico, 2020[18]). The PDN aims to provide access to the following systems and sources of information:
asset and proof of presentation of tax declarations
public servants involved in public procurement procedures
sanctioned public servants and private individuals
information and communication of the NACS and NAS
complaints for administrative offenses and acts of corruption
public information on procurement.
In 2019, the OECD issued a follow-up report of a 2017 Integrity Review of Mexico that provided recommendations in a number of areas, including proposals for addressing the challenges in the design of the PDN (OECD, 2019[19]). The recommendations focused on ensuring the interoperability of databases and developing a strategy for using the platform effectively for risk analysis. By September 2019, the Executive Secretariat of the NACS (Secretaría Ejecutiva del Sistema Nacional Anticorrupción, SESNA) had launched the beta version of the PDN. At the time of drafting this report, the PDN is still in its first version, and the SESNA had successfully incorporated four of the six databases (National Anti-Corruption System, 2021[17]). The SESNA has yet to incorporate data on complaints and data related to information and communication of the NACS and the NAS into the PDN. This reflects what the SESNA describes as a significant ongoing challenge of accessing and collecting data from across government (Executive Secretary of the National Anti-Corruption System, Mexico, 2020[18]).
As a leader of both the NACS and NAS, the ASF is well-positioned to accelerate the improvements to the PDN described in the OECD’s 2017 report, including addressing gaps in data, enhancing the quality of data and helping to fulfil the promise of the PDN as an effective tool for risk analysis. Regarding data quality, ASF officials noted that auditors can use the PDN, but they still must substantiate their findings with certified information obtained directly from the relevant authority. The ASF could promote and support improvements to the quality of data on the PDN based on the experience of ASF’s auditors and their follow-up to certify data. In turn, this could help to improve the quality of the ASF’s (and others’) analyses based on the PDN.
Moreover, a deeper engagement to accelerate improvements to the PDN would also be a constructive avenue for the ASF to further engage with the NACS and the NAS, in accordance with its mandate under the General Law of the National Anticorruption System, while supporting the implementation of Mexico’s anti-corruption agenda.5 Recent media accounts have criticised the NACS for not fulfilling its mandate and in a recent study, Mexico scored poorly on implementation of anti-corruption laws. For instance, a 2020 study showed that while Mexico has a strong anti-corruption legal framework, it stands out relative to eight other countries in Latin America for its lack of implementation of laws and reduced institutional capacity (Lawyers Council for Civil and Economic Rights, 2020[20]). Initiatives related to data and analytics could help to address implementation challenges and generate positive attention for the NACS and the ASF among the general public. For instance, the ASF, the SFP and other members of the NACS and the NAS could also consider developing guidance for government to enhance data quality for the PDN, drawing inspiration from the United Kingdom’s National Fraud Initiative (NFI). The NFI’s platform operates differently from the PDN, since public bodies are required to submit data to the NFI on a regular basis. However, the underlying data issues the NFI faces are similar to the Mexican context. The NFI produces guidance that sets out data specifications in terms of how data should be formatted and the types of data checks entities can conduct. It also supports the ethical use of data through initiatives like the Code of Data Matching Practice, which promotes transparency and lays out principles and practices for protecting citizens’ right to privacy (see Box 1.5).
Box 1.5. The UK National Fraud Initiative’s Code of Data Matching Practice
The Audit Commission’s National Fraud Initiative was launched in 1996 as the United Kingdom’s largest data-matching exercise in relation to fraud. The Serious Crime Act of 2007 enabled bodies, other than those with a mandatory requirement to provide data for the National Fraud Initiative, to volunteer to participate by providing data to the commission (Government of the UK, 2007[21]).
The National Fraud Initiative has enabled participating organisations to prevent and detect more than GBP 300 million fraud and error in the period from April 2016 to March 2018. Approximately 1 200 public and private sector organisations participate in the initiative, including the public audit agencies in Scotland, Wales and Northern Ireland. Each national audit agency carries out data-matching under its own powers, but uses the National Fraud Initiative’s systems, processes and expertise.
To increase transparency around this massive data-matching exercise, the National Fraud Initiative has set out a Code of Data Matching Practice that is followed by all organisations that participate in the Cabinet Office’s data-matching exercises. The code “creates a balance between the important public policy objective of preventing and detecting fraud, and the need to pay due regard to the rights of those whose data are matched for this purpose.” To achieve this goal, the code was informed by the consultation of a range of stakeholders, with the Information Commissioner’s office providing input on data protection.
The code notably requires each institution to publish a privacy notice that informs citizens about the specific datasets used, the way they are collected, the purpose of this data-matching exercise and its legal basis, the institutions with which the data are shared, the retention period for the data, and the rights of citizens including complaint mechanisms.
This example illustrates both the necessity of transparency for integrity actors when implementing anti-fraud programmes and the value of their input to inform the creation of codes of practice that safeguard citizen’s rights.
The ASF could also consider enhancing its co-ordination with SFP concerning data use and reuse, and detecting integrity risks. The ASF focuses on ex post auditing whereas the SFP, as the internal audit function, provides monitoring and assurance throughout the year, so it is unlikely there is duplication, according to ASF officials. Officials also noted that they inform the SFP and the audited entity if they detect irregularities during an audit. However, as noted in the next chapter, communication between the ASF and the SFP as an objective of co-ordination is a low intensity effort. Building on this, the ASF and the SFP could work together to identify shared objectives and additional avenues for collaboration. For instance, in the context of analytics for assessing integrity risks, the ASF and the SFP could exchange risk registries and convene regular meetings to better co-ordinate responses and outreach to the Specialised Anticorruption Prosecutor, a unit within the National Prosecutor’s Office (Fiscalía General de la República, FGR), when irregularities are found. This process is currently lacking a co‑ordinated approach, ASF officials recognised.6 There are already precedents. According to officials, ASF has entered into agreements for the exchange of information with several institutions, including the Tax Administration Service (SAT), the SHCP and the Treasury of the Federation (TESOFE), among others.7
1.4. Planning and monitoring for continuous improvement
1.4.1. Developing a plan to monitor existing and new analytics initiatives
The ASF could develop a plan to ensure the relevance of its analytics initiatives and reliability of the results, particularly for new methodologies and systems that are under development. The ASF can lead by example and draw from its own standards for internal control (Marco Integrado de Control Interno). According to the standards, managers of government entities should use monitoring and evaluation to identify problems in a timely manner and implement corrective actions (ASF, 2014[23]). The ASF is primarily a consumer of data, and the models and systems it develops for running analytics are subject to changes over time. This can include changes to data quality or relevance, depending on the methodology. For instance, ASF officials highlighted plans within the AECF to develop a machine learning capacity. Machine learning relies on the use of historical data to develop algorithms and predictive models. Shifts in context, data reliability or data access can affect the accuracy and utility of the model. For this reason, continuous monitoring of the performance of the ASF’s data and analytics initiatives is a critical component of the aforementioned action plan, should ASF decide to develop one.
Monitoring makes up one of four key pillars in the GAO’s Artificial Intelligence (AI) Accountability Framework, which also includes governance, data and performance. GAO developed the AI Framework to support managers in using AI responsibly and to promote accountability in government AI programmes and processes. GAO identified key practices that focus on the design, development, deployment, and continuous monitoring of AI systems, organised into the four pillars. Many of the activities across each of the pillars support or serve a monitoring or evaluative purpose, which can offer the ASF insights into what it can monitor for its own analytics initiatives. For instance, the “Data” pillar highlights the need for government entities to document sources and origins used for data models, including documents on 1) the means of collecting, preparing, labelling and maintaining data; and 2) the means of monitoring data on a continual basis (US Government Accountability Office, 2021[24]). Box 1.6 provides further details on the AI Framework’s monitoring pillar.
Box 1.6. The monitoring pillar of the US Government Accountability Office’s Artificial Intelligence Accountability Framework
Like other government entities, audit bodies that use AI must continuously monitor these programmes to ensure that they are still conducting analysis the way they were intended to. The US Government Accountability Office (GAO) includes two components of this type of AI monitoring, continuous monitoring and assessing sustainment for expanded use of AI. As part of its AI framework, the GAO describes leading practices for each of these components.
Continuous monitoring of performance
When monitoring for performance, government entities should consider implementing plans that outline when and how the AI will be checked. In general, AI that has a high impact on an entity’s work should be subject to increased monitoring. Another possible step to produce accurate results would be to establish the range of data and model drift that is acceptable to ensure the AI system produces desired results. Input data and output from the AI also requires verification when monitoring AI. The GAO stipulates that entities should document findings and results from monitoring to promote transparency and accountability. Monitoring should also involve conducting interviews and consultations with key stakeholders.
Assessing for sustainment and expanded use
To assess sustainment, audit bodies should assess whether the AI programme is still relevant to the present context, and if it is still meeting organisational requirements. Novel areas in which use of the AI may be expanded should also be considered. This could involve conducting third party assessments.
Monitoring for continuous improvement can also help the ASF to identify the extent of false positives, and understand how to refine its analytics to ensure resources and control activities are targeted appropriately. No analytics technique is fail proof, and human error can be difficult to decipher from fraud or corruption based on what essentially amounts to a review of databases. Human biases can also seep into algorithms and analyses (see Box 1.7). The ASF’s auditors follow-up on irregularities (see next chapter), but capacity is limited and feedback loops so that auditors learn about the results of their work from stakeholders (e.g. SFP, prosecutors offices or law enforcement) are ad hoc. The monitoring plan for data and analytics can help to address this issue by promoting communication and engagement of stakeholders, which in turn can help the ASF to refine its algorithms and analytics based on actual results.
Box 1.7. Monitoring biases in algorithms
Independence is an important component of the work of any auditor, and this fundamental principle can be extended to AI programmes used in audits as well. Biased AI models can be created by purposeful or accidental action, and can disproportionately harm groups or individuals, leading to concerning lapses of fairness and equity. For example, an AI software developed by a major technology company to pre-screen job applicants was found to be biased against female candidates. Damages to fairness and equity caused by an AI can diminish trust in governments, and therefore, AI programmes must be monitored regularly. Monitoring AI presents a number of challenges. It is possible that local laws prohibit the collection or use of certain forms of demographic data, and even if biases are found, it can be difficult to find the right approach to mitigating them. However, as a countermeasure to these challenges, governments are increasingly taking steps to offer guidance on monitoring AI for biases, and auditing AI models.
For instance, in the United Kingdom, the Centre for Data Ethics and Innovation (CDEI) is a government entity under the Department for Digital, Culture, Media and Sport that is tasked with developing the right governance regime for data-driven technologies. Specifically, the CDEI offers guidance on effective use of AI in government. It recommends that organisations using AI should frequently compare the data that was used to train an AI model against the population from which it was taken to ensure the former is representative. Secondly, managers of AI programmes should analyse and mitigate any other sources of bias that are likely to lead to differences in outcome. Finally, it is important to understand and analyse the correlation between key model variables and demographic groups to understand how these might impact biases and outcomes.
1.4.2. Assess the return on investment of analytics initiatives
According to the INTOSAI Framework of Professional Pronouncements (INTOSAI-P 12 on the Value and Benefits of Supreme Audit Institutions), the extent to which SAIs can make a difference in the lives of citizens depends on 12 principles.8 One of these principles is that SAIs should be responsive to changing environments and emerging risks in order to demonstrate ongoing relevance to citizens, parliament and other stakeholders (INTOSAI, 2019[26]). To fulfil this principle, in part, SAIs should establish mechanisms for gathering information, making decisions and measuring performance. How SAIs do this varies, but the SAI Performance Measurement Framework, which the ASF helped to develop when it chaired the INTOSAI Working Group on the Value and Benefits of SAIs, provides general guidance for SAIs to assess results across a range of activities (INTOSAI, 2016[27]).
As described in the OECD’s Progress Report on the Implementation of the Mexican Superior Audit of the Federation’s Mandate, the ASF has a dedicated team in the Technical Unit called the Directorate of Analysis and Follow-up of Management (Dirección de Análisis y Seguimiento de la Gestión), which assesses the impact of ASF’s work (OECD, 2021[28]). A key metric the team developed frames the ASF’s impact as a function of recoveries relative to its overall budget (i.e. amount of recoveries from the audited public account divided by the modified budget assigned to the ASF for the fiscal year equals pesos recovered for each peso of modified budget). The sources of information for calculating this return on investment (ROI) are the annual General Executive Report that is delivered to the Surveillance Commission of the Chamber of Deputies (Comisión de Vigilancia de la Auditoría Superior de la Federación), as well as the institution’s budget data. Figure 1.5 depicts the results for the ASF over a 10-year period, based on the recovery formula. For instance, for every peso allocated to the ASF in 2012, the ASF recovered nearly 10 pesos. The overall trend for the 10-year period has been a decrease in ROI, according to this metric.
Figure 1.5. The ASF’s return on investment using recoveries as a metric
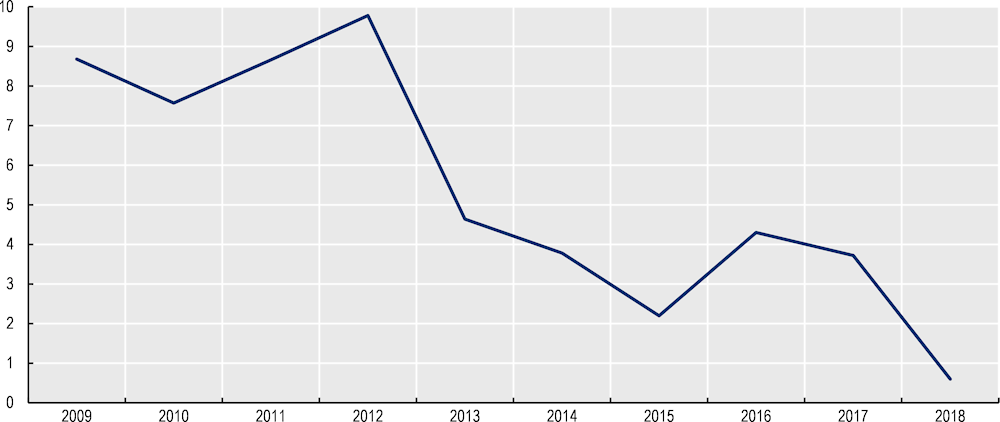
Note: The average ratio over this same period was 5.39; the median was 4.47.
Source: (OECD, 2021[28]).
The OECD’s Progress Report proposed that the ASF take additional steps to assess the impact of its audit work and communicate its value, including adding new indicators to supplement its assessment of recoveries each year. Building on the proposals in that report, the ASF could also consider measuring the performance of its analytics activities. With the development of new IT capabilities in recent years, and plans for new initiatives, some of which are described in this report, the ASF will continue to invest taxpayer money in its capacity to audit effectively in a digital age. It is beyond the scope of this report to analyse all initiatives and assess metrics; however, likely costs include salaries for staff and data experts, enhancements to the IT infrastructure or architecture, license fees for software and materials for trainings. In the integrity context, the ASF could enhance its assessments to consider not only output indicators (e.g. number of irregularities detected using analytics), but also short-term outcome indicators. For example, as part of the capacity-building on follow-up processes (see Chapter 2), the DGAF could consider enhancing its data collection, in collaboration with the Specialised Anticorruption Prosecutor, to understand the ultimate impact its analytics and referrals have on the outcomes of referred cases. Much of the information, feedback and data that would support improved performance measurement can be captured in the monitoring plan described previously, and tailored for different purposes. Moreover, having a baseline for ROI can help the ASF to make decisions about new investments and scaling up successful initiatives based on evidence and results.
1.5. Summary of the proposals for action
This chapter explores considerations for the ASF to elevate analytics for assessing integrity risks at the strategic level. Setting a strategic vision, objectives and a co-ordinated course of action would help to rally all levels of the ASF around a common understanding of its use of analytics for assessing integrity risks, and can inspire a cultural shift in which auditors naturally incorporate data and analytics into all phases of the audit cycle. A strategy and action plan for analytics for integrity risk assessments would also set the tone for leadership’s commitment to the professional development of auditors, while establishing a baseline for monitoring and measuring performance of investments in this area. Specifically, to advance a more strategic approach, the ASF could consider the following proposals for action:
Establish a unified vision for the ASF’s use of data and analytics for assessing integrity risks— Data and analytics enables teams across the ASF to achieve their objectives, and deliver on the institution’s goals outlined in its strategic plan. Senior ASF officials flagged the development of a unified vision for data and analytics, including one that reflects trends related to big data, as a top strategic priority. There is a similar need for a unified vision to guide the application of analytics for assessing integrity risks. ASF’s current strategy and digital transformation work programme do not emphasise this point, yet there are decentralised initiatives that have similar processes and needs in terms of capacities, skills and data requirements with long-term plans for development. Analytics plays a critical part in the ASF’s work across multiple directorates, including its efforts to combat fraud and corruption. Addressing data and analytics at the strategic level, whether in the ASF’s next strategy, subsequent iterations of its digital work programme, or elsewhere, would promote coherence and co-ordination across the organisation.
Create an action plan for analytics—With a more coherent vision in place, the ASF could take further steps to ensure internal initiatives are aligned vertically and horizontally. The ASF’s approach to analytics can be characterised as bottom-up, with specialised areas developing capacities, tools and processes that serve their individual mandates. While this model has its benefits in terms of specialisation, it also increases the risk of duplication as well as incoherent policies and procedures. The ASF could devise an action plan that focuses on institutional objectives for leveraging data and analytics to enhance audit work. A separate action plan for analytics would provide the necessary granularity for honing in on applications of data and analytics in specific areas of ASF’s activities. One of these areas could be data and analytics for integrity, filling a critical gap in the attention the ASF currently pays to fraud, corruption and integrity in its strategy and digital transformation work programme.
Engage the National Digital Platform (Plataforma Digital Nacional, PDN) and the Ministry of Public Administration (Secretaría de Función Pública, SFP)—The shared goals of the PDN and ASF in terms of enhancing the use of data to combat corruption and detect integrity risks suggests opportunities for improved co-ordination and collaboration. The ASF could help to accelerate improvements to the platform, including addressing current gaps related to information and data from the NACS and the NAS. The ASF could also promote and support improvements to the quality of data on the PDN based on the experience of the ASF’s auditors and their follow-up to certify data for audits. In turn, this could help to improve the quality of the ASF’s own subsequent analysis using the PDN. Moreover, the ASF could improve co-ordination and collaboration with the SFP, including exchange of risk registries and convene regular meetings to better co-ordinate responses and outreach to the Specialised Anticorruption Prosecutor when irregularities are found.
Develop a plan to monitor new and existing analytics initiatives—the ASF could develop a plan to ensure the relevance of its analytics initiatives and reliability of the results, particularly for new initiatives, in line with INTOSAI standards and international leading practices. Shifts in context, data reliability or data access can affect the accuracy and utility of various analytic techniques, including machine learning, which the ASF is developing. Continuous monitoring of the performance of the ASF’s data and analytics initiatives is a critical component of the aforementioned action plan, should ASF decide to develop one. One benefit of monitoring its analytics, including the underlying models and algorithms, is that it can help the ASF to identify the extent of false positives, and understand how to refine its analytics to ensure resources and control activities are targeted appropriately. No analytics technique is fail proof, and human error can be difficult to decipher from fraud or corruption based on what essentially amounts to a review of databases. Human biases can also seep into algorithms and analyses. The monitoring plan for data and analytics can help to address such issues and provide a constructive process for the ASF to engage stakeholders in the refinement of analytical procedures.
Asses the return on investment of its analytics initiatives—the OECD’s Progress Report on the Implementation of the Mexican Superior Audit of the Federation’s Mandate proposed that the ASF take additional steps to assess the impact of its audit work and communicate its value, including adding new indicators to supplement its assessment of recoveries each year. Building on the proposals in that report, the ASF could also consider measuring the performance of its analytics activities. With the development of new IT capabilities in recent years, and plans for new analytics initiatives, the ASF could enhance its assessments to consider not only output indicators, but also short-term outcome indicators. Having a baseline for return on investment (ROI) can help the ASF to make decisions about new investments and scaling up successful initiatives based on evidence and results.
References
[29] Angel, A. (2021), “More than 80% complaints from the ASF for deviations fall in three years; impunity persists”, Animal Politico, https://www.animalpolitico.com/2021/03/caen-denuncias-asf-desvios-impunidad-persiste/ (accessed on 17 November 2021).
[11] ASF (2021), Presentation by ASF Officials in the Director General of Forensic Audits, “Laboratorio de Cómputo Forense”.
[9] ASF (2020), El Programa de Transformación Digital (Digital Transformation Programme).
[7] ASF (2018), 2018-2026 Plan Estratégico Institucional (Strategic Institutional Plan), ASF.
[23] ASF (2014), Marco Integrado de Control Interno, https://www.asf.gob.mx/uploads/176_Marco_Integrado_de_Control/Marco_Integrado_de_Cont_Int_leyen.pdf.
[10] Australian National Audit Office (2020), Corporate Plan 2020-21, https://www.anao.gov.au/work/corporate/anao-corporate-plan-2020-21.
[22] Cabinet Office’s National Fraud Initiative, Government of the UK (2018), Code of Data Matching Practice, https://assets.publishing.service.gov.uk/government/uploads/system/uploads/attachment_data/file/750372/Code_of_Data_Matching_Practice.pdf.
[25] Centre for Data Ethics and Innovation (2020), Review into bias in algorithmic decision-making, https://assets.publishing.service.gov.uk/government/uploads/system/uploads/attachment_data/file/957259/Review_into_bias_in_algorithmic_decision-making.pdf.
[4] Coordination of the National Digital Strategy, Government of Mexico (2021), Planning Process for the Development of the National Digital Strategy and Technology Policy, https://www.gob.mx/cedn/documentos/proceso-de-planeacion-para-el-desarrollo-de-la-estrategia-digital-nacional-y-de-la-politica-tecnologica (accessed on 3 August 2021).
[18] Executive Secretary of the National Anti-Corruption System, Mexico (2020), The National Digital Platform: Data for the fight against corruption, https://contralacorrupcion.mx/la-plataforma-digital-nacional/#_ftnref1 (accessed on 2 July 2021).
[8] Government of Mexico (2021), “Diario Oficial de la Federación”, in Agreement amending, adding and repealing several provisions of the Internal Regulations of the Superior Audit Office of the Federation.
[16] Government of Mexico (2021), The General Law of the National Anticorruption System (Ley General Del Sistema Nacional Anticorrupción), http://www.diputados.gob.mx/LeyesBiblio/pdf/LGSNA_200521.pdf.
[3] Government of Mexico (2013), National Digital Strategy, https://www.gob.mx/cms/uploads/attachment/file/17083/Estrategia_Digital_Nacional.pdf.
[21] Government of the UK (2007), Serious Crime Act 2007, https://www.legislation.gov.uk/ukpga/2007/27/section/49 (accessed on 3 September 2021).
[2] INTOSAI (2019), Data Analytics Guideline Prepared for INTOSAI’s Working Group on IT Audit.
[26] INTOSAI (2019), INTOSAI-P - 12 - The Value and Benefits of Supreme Audit Institutions – making a difference to the lives of citizens, https://www.issai.org/pronouncements/intosai-p-12-the-value-and-benefits-of-supreme-audit-institutions-making-a-difference-to-the-lives-of-citizens/.
[6] INTOSAI (2019), Moscow Declaration, https://incosai2019.ru/en/documents/46?download=275 (accessed on 3 August 2021).
[27] INTOSAI (2016), Supreme Audit Institutions Performance Management Framework, https://www.idi.no/elibrary/well-governed-sais/sai-pmf/426-sai-pmf-2016-english/file.
[20] Lawyers Council for Civil and Economic Rights (2020), Latin America Anti-Corruption Assessment, Cyrus R. Vance Center for International Justice and the New York City Bar, https://www.vancecenter.org/wp-content/uploads/2021/05/Latin-America-Anticorruption-Assessment-2020.pdf.
[1] MIT (2020), “Leading With Decision-Driven Data Analytics”, MIT Sloan Management Review, https://sloanreview.mit.edu/article/leading-with-decision-driven-data-analytics/ (accessed on 3 August 2021).
[17] National Anti-Corruption System (2021), Plataforma Digital Nacional, https://www.plataformadigitalnacional.org/about (accessed on 5 July 2021).
[28] OECD (2021), Progress Report on the Implementation of the Mexican Superior Audit of the Federation’s Mandate, OECD, Paris, https://www.oecd.org/corruption/ethics/progress-report-on-the-implementation-of%20the-Mexican-Superior-Audit-of-the-Federation-s-mandate.pdf.
[19] OECD (2019), Follow up Report on the OECD Integrity Review of Mexico, OECD, Paris, https://www.oecd.org/corruption/ethics/follow-up-report-oecd-integrity-review-mexico.htm (accessed on 3 August 2021).
[5] OECD (2019), The Path to Becoming a Data-Driven Public Sector, OECD Digital Government Studies, OECD Publishing, Paris, https://doi.org/10.1787/059814a7-en.
[15] Revista do TCU (2016), “Evolution of Control in the”, Federal Court of Accounts Journal, https://revista.tcu.gov.br/ojs/index.php/RTCU/issue/view/68/102 (accessed on 3 August 2021).
[12] The European Court of Auditors (2019), “Developing data services for audit”, Presentation of Magdalena Cordero, Director of Information, Workplace and Innovation, https://ecademy.eca.europa.eu/pluginfile.php/260/mod_resource/content/1/Developing%20data%20services%20for%20auditors%20%E2%80%93%20the%20ECA%20experience.pdf (accessed on 3 August 20021).
[13] UK National Audit Office (2021), NAO strategy: progress update and estimate memorandum for 2021-22, https://www.nao.org.uk/wp-content/uploads/2021/04/NAO-strategy-Progress-update-and-estimate-memorandum-for-2021-22-1.pdf.
[14] UK National Audit Office (2018), Data Analytics at the National Audit Office (UK), https://www.intosaipas.org/wp-content/uploads/2020/02/Agenda-item_2A_Andy-Fisher_Data-Analytics-at-the-UK-NAO.pdf (accessed on 3 August 2021).
[24] US Government Accountability Office (2021), Artificial Intelligence: An Accountability Framework for Federal Agencies and Other Entities, https://www.gao.gov/assets/gao-21-519sp.pdf.
Notes
← 1. For purposes of this document, “irregularities” is used interchangeably with “integrity risks” to capture a broad set of risks related to fraud, corruption, waste, abuse and error.
← 2. While this report focuses on ASF’s leveraging of data and analytics for detecting irregularities, many of the issues and proposals for action raised in this report have broader implications for the ASF that can inform other analytics activities that support audits of programme performance, effectiveness and efficiency. There are tools available for the ASF to consider broader Information Technology (IT) assessments. For instance, at the time of drafting this report, the Deutsche Gesellschaft für Internationale Zusammenarbeit (GIZ) was developing and testing a Supreme Audit Institutions Information Technology Maturity Assessment.
← 3. For instance, see the African Organisation of Supreme Audit Institutions research report on integrating big data into public sector auditing (https://afrosai-e.org.za/wp-content/uploads/2020/12/Research-Paper-Integrating-Big-Data-in-Public-Sector-Auditing.pdf); the training tool on environmental data published by the INTOSAI Working Group on Environmental Auditing (https://www.environmental-auditing.org/media/113693/23g-wgea_environmental-data_2019-fin.pdf); or the experiences of the Netherlands Court of Audit in developing an audit framework for algorithms (http://intosaijournal.org/developing-an-audit-framework-for-algorithms/).
← 4. This DG was not taken into account in the OECD’s analysis and drafting, as it was only established after the fact-finding mission. Therefore, the potential areas for co-ordination and risks of overlap with DGAF are not addressed in this report.
← 5. The involvement of the ASF in the design, improvement and subscription to the PDN is established in Article 9, Sections XII and XIII of the General Law of the National Anticorruption System (Government of Mexico, 2021[16]).
← 6. According to media reports, the FGR’s success rate in terms of corruption complaints being prosecuted is low and actual convictions are non-existent. Recognising this, the ASF has adapted its strategy to reduce the number of cases referred to the FGR, while at the same time trying to improve the quality of its referrals (Angel, 2021[29]). Better data and information, including integrated information from enhanced ASF and SFP co-ordination, could help to improve the success rates of the FGR and facilitate the ASF’s own strategy.
← 7. The agreement with TESOFE was cancelled on 16 December 2020 by means of official letter 401-T-136/2020.
← 8. At the time of writing this report, the ASF was the leader of the Working Group on Value and Benefits of SAIs.