This section provides a practical instrument for policy officials working in ministries, departments and public agencies on what is the process through which the behavioural aspects of a problem can be identified, scoped and addressed.
Tools and Ethics for Applied Behavioural Insights: The BASIC Toolkit
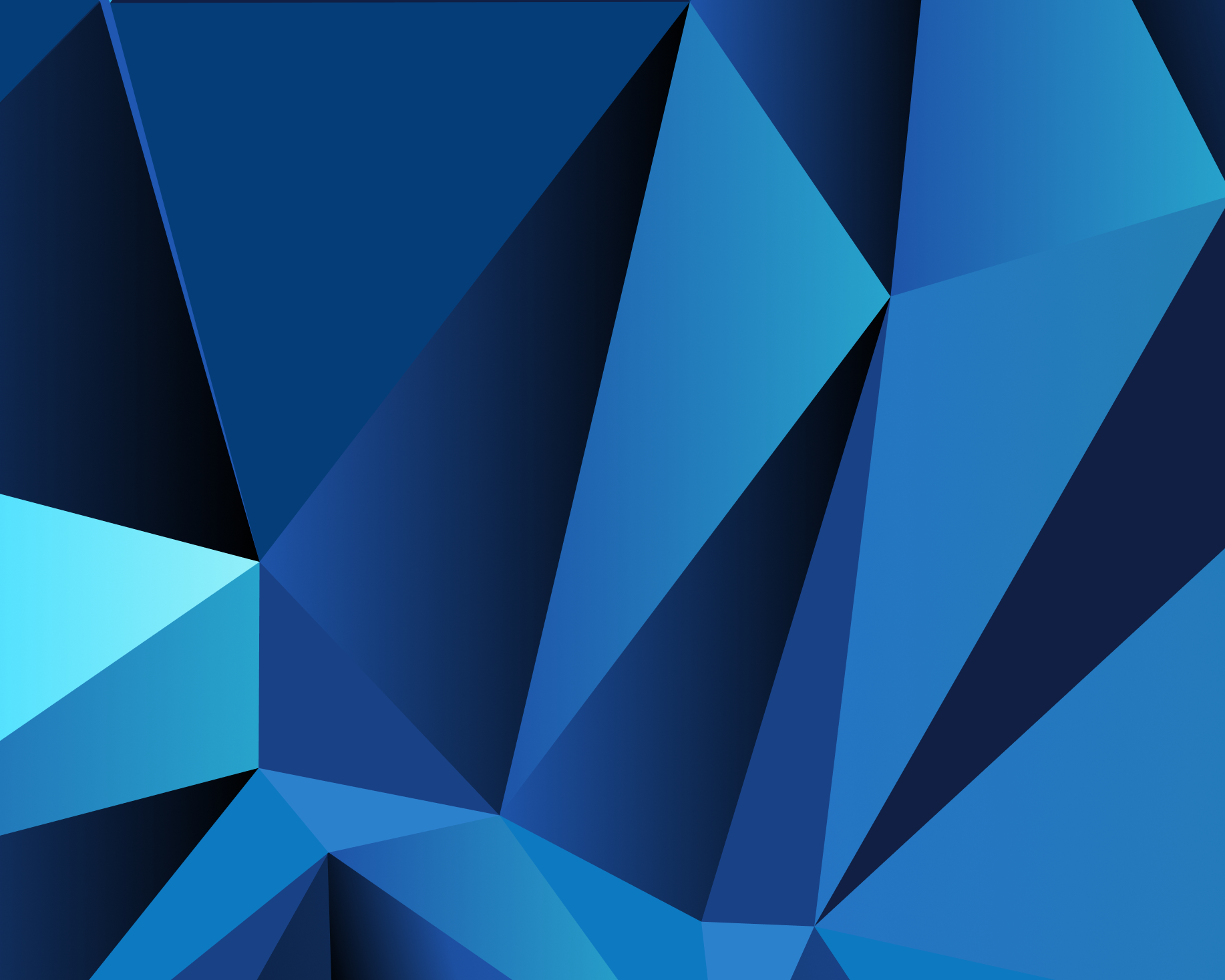
Chapter 1. Introductory guide to BASIC
Abstract
Introduction
Have you ever grabbed a chocolate bar at the check-out line only to regret it later? Filled up your entire bowl with pasta even though you intended to only take a small portion? Found yourself sticking with the side of chips or fries, instead of substituting for a salad?
It is easy to make unhealthy choices even when you choose to be healthy. These everyday choices add up. Today, more than one in two adults are overweight or obese in OECD countries (OECD, 2017b). Around the world, obesity-related illness is estimated to cost USD 1.2 trillion by 2025 (WOF, 2015). Why do people make such choices? Before making assumptions, it is important to consider what drives decision-making given the specific context.
This is where behavioural insights (BI) can help. BI is a tool based on the idea that context and behavioural biases influence our decision-making. As a policymaker, you can use BI to address “wicked problems” like obesity to better design and deliver policy outcomes.
To integrate BI into your day-to-day work, you can use the BASIC process to analyse Behaviours, conduct an Analysis, develop Strategies, test them with Interventions, and scale up results for policy Change.1 For example, if your desired policy outcome is to lower adult obesity rates, then you can start by selecting a relevant, specific behaviour (i.e. proportion of healthy items ordered from restaurant menus).
Say you learn that 60% of residents who eat out frequently intend to take the healthy options but end up choosing burgers. You start by writing your assumptions to explain why:
Information: Residents do not know exactly how calorific burgers are.
Cost: Residents find burgers to be cheaper than the healthier options.
Access: Residents cannot easily access restaurants that serve healthy options.
At first, you may consider traditional policy instruments to address each cause:
Require calorie labelling on restaurant menus.
Implement a junk food tax on burgers to make them less affordable.
Provide a tax credit to restaurants that provide healthy alternatives to increase availability.
In theory, better information, prices, or access should lead to healthier eating habits. This is aligned with classical economic theory that assumes individuals will choose the rational decision that maximises their utility. People use information to make better decisions so you may assume that the more information they have about how unhealthy burgers are, the more likely they will choose a healthier option that will benefit them in the long-run.
Unfortunately, we know, even from our own personal experience, that this is not always the case. This is the central idea behind BI, which is built on extensive research from the field of behavioural economics and the behavioural sciences that have repeatedly found that people systematically deviate from traditionally explained rational behaviour. This is not to say individuals are irrational but rather that you cannot always rely on your assumptions that people will always make the decision that leads to the best outcome for them.
By understanding how people actually react and behave in different situations, you can better anticipate the behavioural consequences of your policy and ultimately design policies that can help citizens make the healthy choice. Below in italics, you will find examples of how you can use existing BI research to complement traditional policy instruments:
Require calories labelling on restaurant menus that put calorie counts before the food item because people give disproportionate weight to the first piece of information they see. Dallas (2019) found that displaying calories first resulted in a 16.31% decrease in ordered calories
Implement a junk food tax that requires the price hike of burgers to be clearly marked on the menu because a price difference is more salient at the point of decision-making. Chetty (2009) found that tax-inclusive prices reduced demand by 8%.
Provide a tax credit to restaurants that only provide healthy options because adding healthy items next to burgers can vicariously fulfil healthy-eating goals and increase indulgent eating habits. Wilcox (2009) found that adding a healthy alternative increased unhealthy ordering by 230%.
At this point, you can choose which solution(s) is/are the most appropriate in your context, and test which is the most effective in increasing the proportion of healthy items ordered from restaurant menus. Through testing, you will gain evidence-based results to inform your policy to lower adult obesity rates before setting policy and full-scale implementation.
This approach is not only limited to healthy eating or complementing traditional policy levers. By integrating BI from the start of the policy cycle, policymakers can design behaviourally informed policies on a variety of issues that go with the grain of how people actually behave rather than go against it, and ultimately improve outcomes without compromising people’s autonomy. This guidebook helps you get started by breaking a policy issue down to its behavioural components and identifying potential behavioural barriers that can undermine the intended policy outcome or enablers that can ultimately enhance the effectiveness of the policy. It uses a process that guides the policymaker through Behaviours, Analysis, Strategies, Interventions and Change (abbreviated “BASIC”) to apply BI to any policy problem from start to finish (see Figure 1.1).
Figure 1.1. The BASIC Framework
What is BASIC?
BASIC is a toolkit that equips the policymaker with best practice tools, methods and ethical guidelines for conducting BI projects from the beginning to the end of a public policy cycle. Earlier BI frameworks have primarily focused on the end stages of the policy cycle such as experimentation or compliance while less emphasis is placed on the behavioural analysis of a policy problem (OECD, 2017a). BASIC aims to bridge this gap by providing guidance on how to apply to BI to ex ante appraisal as well as the ex post evaluation stage of a policy cycle. This approach is reflected in the five stages of BASIC (Table 1.1). By understanding how and under what circumstances BI can be applied to cause behaviour change, policymakers are far more likely to design and deliver more effective policies.
Table 1.1. Applying BASIC to increasing enrolment in pension plans
Stage |
Description |
Example |
---|---|---|
Behaviour |
Identify and better understand your policy problem. |
Increase pension savings by encouraging more citizens to enrol in pension plans. |
Analysis |
Review the available evidence to identify the behavioural drivers of the problem. |
Individuals tend to stick with defaults and choose inaction over action. |
Strategy |
Translate the analysis to behaviourally informed strategies. |
Change the default. Automatically enrol individuals into pension plans and allow them to opt-out. |
Intervention |
Design and implement an intervention to test which strategy best addresses the problem. |
Test whether allowing individuals to opt-out increases pension savings rather than the current practice of opt-in. |
Change |
Develop plans to scale and sustain behaviour. |
Share results with citizens, apply findings to system-wide reminders and monitor long-term consequences of the intervention. |
Source: Adapted from Thaler, R.H. and S. Benartzi (2004), “Save more tomorrow︎: Using behavioral economics to increase employee saving”, Journal of Political Economy, Vol. 112(1), University of Chicago.
As you read through the BASIC Guidebook, you will gain an introduction to behaviourally informed policymaking and a brief overview of testing and implementation. This is geared towards policymakers who know the policy problem and context but have limited or even no experience with BI. You can find approaches, proofs of concepts and details on methods for designing and implementing a behaviourally informed policy intervention in the BASIC Manual accompanying by an introductory guide.
Specifically, the guide will give you:
A practical and in-depth look into the first three sections, Behaviour,2 Analysis and Strategies to identify a behaviour that is driving the policy issue and why, and design actionable strategies based on the behavioural analyses.
Outline of the Intervention section that provides general guidance on engaging with behavioural experts and stakeholders at the testing stage.
High-level recommendations for the Change section so policymakers can make an informed decision when planning to scale and disseminate results after testing.
Ethical considerations for each step of BASIC.
What you need to know before you keep reading
Integrating BI throughout the policy cycle can enhance the design and delivery of policy outcomes, but it has several areas that you should consider as a policymaker before moving forward.
First, ethics should be a priority from the onset. The BI approach has specific ethical concerns that are different from traditional public policy because it often involves the use of primary data of individual- or group-level behaviours and leverages behavioural biases to inform policies. As public policy operates within a transparent setting and has far‑reaching implications, it is important to integrate ethical considerations when applying BI from the start to the end of the policy cycle. The final section includes overall ethical considerations and specific guidelines for every stage of BASIC.
Second, you should be aware of both the benefits and limitations of BI. Table 1.2 gives a high-level summary of considerations before deciding if BI is the right fit for your project.
Table 1.2. Considerations before applying Behavioural Insights (BI)
What BI is |
What BI is not |
---|---|
Problem-solving method BI is a powerful method to better understand policy problems and pre-test solutions before they are implemented across a wide range of policy issues. |
Silver bullet BI is not a silver bullet that solves all policy challenges. Some policy issues may benefit more from traditional policy levers (i.e. financial, regulatory or awareness-raising approaches) or alternative non-traditional tools (i.e. human centred design or machine learning). |
Way to learn “what works” The BI culture of empirically testing solutions and disseminating results allows practitioners and academics to exchange evidence on lessons learned to inform policymaking. |
One-size-fits-all Replicating what works in one environment does not guarantee success in another environment. Ethical considerations should also be adapted to the context. Pre‑testing solutions in the context where you plan to implement the policy minimises this risk. |
Beyond nudging 1.0 BI goes beyond nudging or small policy tweaks. BI represents a wide range of tools to use evidence to diagnose problems, bridge the gap between research and practice, and inform comprehensive policy solutions. |
Only for behavioural experts BI is not limited to behavioural experts. A multi-disciplinary approach is key for BI projects. BI brings together diverse expertise such as knowledge of the policy context, behavioural science and first-hand experience with public service. |
Policy tool BI should be considered every time you are designing or evaluating a policy. Even in cases where you may not be able to start with a behavioural analysis or run a full experiment, BI can still be used to complement traditional policy tools and levers throughout the policy cycle. |
Irrationality BI does not suggest that humans are fundamentally irrational creatures. Rather, it argues that deviations from “traditionally explained rational” behaviour are not the result of flawed reasoning but rather adaptive forms of reasoning that can also constitute efficient heuristics (i.e. mental shortcuts or intuitive judgments) in an uncertain world. |
As a reminder, the guide is by no means the only resource to apply BI to policymaking. In addition to the BASIC Manual, there are other useful frameworks and reports to aid you in your BI project. Box 1.1 shares some key resources that can provide additional tools and examples to complement the approach provided by BASIC.
Box 1.1. Additional BI resources
With the rise of BI around the world, a number of useful frameworks have been developed by both government and non-government agencies. BASIC has been developed to fill a need in the community for how to implement behaviourally informed public policy.
Below is a non-exhaustive list of widely referenced frameworks that complement BASIC and could be a resource for policymakers looking for different ways to analyse a behavioural problem.
MINDSPACE (The Behavioural Insights Team, 2010): Provided an early checklist for thinking about how nine well-evidenced behavioural insights may inform public policy development, design and delivery.
Test, Learn, and Adapt (The Behavioural Insights Team, 2013): Gave an accessible introduction to the basics of using randomised controlled trials in policy evaluation.
EAST Framework (The Behavioural Insights Team, 2014): Provided a simple framework considering how behavioural insights may help design policies based on leveraging convenience, social aspects of decision-making and the attractiveness and timeliness of policies.
World Development Report Mind, Society, and Behavior (World Bank, 2015): Gave a comprehensive overview of how the BI perspective on human decision-making is of relevance to development policy.
Define, Diagnose, Design, Test (ideas42, 2017): Provided a practical framework for thinking through a problem and identifying behaviourally informed solutions.
US Internal Revenue Service (IRS) Behavioral Insights Toolkit (IRS, 2017): Created to be a practical resource for use by IRS employees and researchers who are looking to use BI in their work.
Assess, Aim, Action, Amend (BEAR, 2018): Presented a playbook developed for applying BI in organisations outlining four steps for applying BI.
Stage 1: Behaviour
BI is more effective the earlier you integrate it into your policy initiative. The first stage, Behaviour, focuses on problem definition. Applying BI at this early stage is ideal because it is less likely that concrete solutions are developed and more likely that innovative approaches are welcomed. As a starting point, this section provides you with the tools to think through four key questions to help identify and define the behavioural aspects of the policy issue:
1. What are the behaviours driving the policy issue?
2. Which behaviour(s) should you target?
3. What is your desired policy outcome?
4. What is the context shaping target behaviours?
What are the behaviours driving the policy issue?
Before applying BI to any policy issue, it is important to define in as much detail as possible the behavioural elements of the problem. You can start brainstorming by using a Behavioural Reduction tool to identify relevant concrete behaviours that are relevant to your policy issue. Engaging stakeholders, citizens and/or behavioural experts even at this early stage can help generate insights into the behavioural aspects of the problem that you may have not been aware of as a policy official (Figure 1.2).
Throughout this process, it is important to remember that every item may not be behavioural (i.e. generate interest in composting). Although these are important to identify, the focus of this exercise should be on behaviour(s) that can be measured (i.e. register for composting programme) and not on opinions, values or structural aspects of the problem.
Figure 1.2. Example of a behavioural reduction
Which behaviour(s) should you target?
When choosing which relevant behaviour to pursue, in addition to financial and political feasibility, there are several considerations that are specific to BI projects. The priority filter questionnaire can be a guide to generate a more holistic discussion on which elements are important to the project and apply this to the selection of the target behaviour.
The priority filter questionnaire is a decision-tool composed of weighted questions that reflect important considerations for the success of the behavioural project. For each question, you can rate the target behaviour (i.e. on a scale from 1 = “definitely not” to 5 = “definitely”), pre-determine a cut-off (i.e. questions that score at least a 4.8 will be considered) and calculate the overall score for each. Although each questionnaire is tailored to the project, Table 1.3 presents some general questions to consider. This can be an iterative process, so keep coming back to these questions as priorities may change as you learn more about the behaviour, context and relevant behavioural biases.
Table 1.3. Sample questions for the priority filter questionnaire
Areas |
Sample questions |
---|---|
Importance |
Is a change in behaviour an institutional priority? |
Ethics |
Are there any potential risks or unintended consequences when pursuing the desired behaviour? Are there uneven risks (i.e. positive for the majority but harmful risks for minority groups)? |
Impact |
Will changing the target individual behaviour translate to a significant societal impact? |
Feasibility |
Is it politically feasible? Are resources available? Is it controversial? |
Data access |
Is baseline data readily available? Can you collect individual or group-level prospective data? |
Frequency |
Does the behaviour occur frequently? Is there a reasonable base rate for the preferred behaviour? |
What is your desired policy outcome?
As you begin to narrow down your target behaviour, it is also important to start defining a roadmap for your policy initiative. One way is to write down what a meaningful outcome would look like for your policy initiative. You can craft a well-defined outcome specific to your target behaviour(s) by defining the following criteria (Figure 1.3):
Specific to the target behaviour.
Measurable to assess and quantify results.
Assignable to a specific group of individuals.
Realistic given the time, budget and resources available for the project.
Time-related to ensure outcomes are achieved within a specified time period.
Figure 1.3. Example of a “SMART” outcome
Source: Adapted from Doran, G.T. (1981), “There’s a SMART way to write management’s goals and objectives”, Management Review, Vol. 70(11), pp. 35-36.
Box 1.2. BI is a data-driven process
Knowing which data can be used and generated will better prepare you for the Intervention section. When defining your outcome, consider what kind of data can be collected, how much resources it costs and ways to ensure that collection does not compromise data privacy. For example, determine if you can link who received which phone notifications (intervention) with who transferred part of their income towards savings (outcome). Finally, understand the ethical considerations or preparations (i.e. ethical review board) necessary to protect user privacy and your organisation.
What is the context shaping target behaviours?
Gaining a deeper understanding of where the target behaviour occurs or is the most likely to occur can shed light on how this affects your desired policy outcome. A process map that outlines decision points immediately before, during and after the target behaviour can help pinpoint areas where you can potentially design a behavioural intervention.
A process map is a visual tool that identifies touchpoints for the key actors engaging in your target behaviour(s). Although this is not an exhaustive list, Table 1.4 shares a number of popular process tools available, especially from the fields of psychology and design research.
Table 1.4. Process tools to map behaviours
Tool |
Description |
Key features |
---|---|---|
Behavioural flowchart |
Detailed process chart that places key decision points in a broader process. |
Identifies potential loose ends and friction points that inhibit the efficiency and reliability of the process. |
Behavioural mapping |
Map that reflects patterns of movement and behaviour within a given environment. |
Allows you to relate various observed behaviours to particular locations, types of users and over time. |
User journey mapping |
Timeline which outlines each touchpoint that the user experiences to perform the key behaviour. |
Reflects the actions, mind-sets and emotions of users through visualisation and storytelling. Commonly used in UX design for products and services. |
Service blueprinting |
Extension of the user journey map that visualises the relationship between different service components. |
Clarifies interactions between service users, digital touchpoints and activities that are both seen and unseen by the user. |
Sources: Ng, C.F. (2016), “Behavioural mapping and tracking”, Research Methods for Environmental Psychology, Vol. 29; Gibbons, S. (2018), Journey Mapping 101, https://www.nngroup.com/articles/journey-mapping-101/; OECD/OPSI (n.d.), Practical Service Blueprinting – The Guide, https://oecd-opsi.org/toolkits/practical-service-blueprinting-the-guide/.
Box 1.3. Map the “actual” behaviour
The process map should reflect how people “actually” behave rather than how they should behave. Speaking to or surveying relevant stakeholders and target individuals can generate helpful insights. Observations can expose new insights because people may not provide honest answers, not remember past behaviour or not be consciously aware of their own behaviours or biases (Ng, 2016). If you are using a user journey map, go to actual users, observe their process and listen to their real-time feedback. If you are using the behavioural flowchart, observe friction points such as delays to know how long the delays really are.
Stage 2: Analysis
Once you have identified the behavioural problems at the heart of your policy issue, it is important to understand why people behave as they do. The second section, Analysis, aims to examine, through the lens of BI, which psychological and cognitive factors are causing the targeted behaviours.
Introduction to “slow” and “fast” thinking
Analysing these behaviours starts by drawing a distinction between “slow” and “fast” thinking (Kahneman, 2011; Figure 1.4). In broad terms, behavioural science shows that we rely on two types of cognitive processes – a cognitive process that is slow, deliberate and conscious (i.e. reason through a math problem) and a cognitive process that is fast, automatic, intuitive and by and large unconscious (i.e. recognise the emotion on the face of a friend).
Figure 1.4. Distinction between “slow” and “fast” thinking
Source: Adapted from Kahneman, D. (2011), Thinking, Fast and Slow, Macmillan.
Many of the behavioural problems that BI tackles are the result of intuitions which we form when we “think fast”. In particular, we can identify four main aspects of behaviour that tend to cause the biases involved in behavioural problems: Attention; Belief formation; Choice; and Determination (ABCD). This “ABCD framework” assists you in analysing and diagnosing behavioural problems. The framework, and how each aspect departs from rational choice theory, is summarised in Table 1.5.
Table 1.5. Overview of ABCD framework
Aspect |
What rationality says |
What BI shows |
Example |
---|---|---|---|
Attention |
People should focus on what is most important in light of their knowledge and preferences. |
People’s attention is limited and easily distracted. |
Forgetting an appointment. |
Belief formation |
People should form their beliefs according to the rules of logic and probability. |
People rely on mental shortcuts or intuitive judgments and often over/underestimate outcomes and probabilities. |
Underestimating how long a task will take. |
Choice |
People should choose so as to maximise their expected utility. |
People are influenced by the framing and the social as well as situational context of choices. |
Being influenced by what our social circle thinks is the right thing to do rather than choosing the rational option. |
Determination |
Provided that one decides to pursue certain long-term goals, one should stick to the plan. |
People’s willpower is limited and subject to psychological biases. |
Failing to quit smoking. |
Tip: Familiarise with the behaviour
Before you delve into the Analysis section using ABCD, use the process map that you created from the Behaviour section to make sure you are familiar with the target behaviour. If a process map is not feasible, it is still advisable to examine past data on the behaviour or find ways to locally observe or engage in the behaviour.
Reminder: Flexible methodologies
When studying behaviours, you can adopt flexible research designs. This means that the type and number of methods used in your study might vary as data collection continues.
Attention
Have you ever failed to take medication or to file taxes on time? These are everyday examples of attentional problems that originate from our cognitive limitations. Humans have a finite ability to sift through a large amount of information and to focus on what is important.
Attention is often scarce, easily distracted and quickly overwhelmed. This results in individuals having a hard time making choices based on relevant information and aligning their decisions with their intrinsic preferences. Common examples of inattention are forgetting commitments and overlooking non-obvious information (or “salient,” in BI terms); as well as falling prey to distractions while working.
As we will see in the Strategies section, behaviourally informed policy solutions can target attention problems by focusing on making the key information salient and understandable, seizing the person’s attention and planning for inattention in the decision-making process. In particular, getting the timing of an intervention right can really make the difference between the success or failure of a policy.
Guiding questions for attention biases
1. Is the targeted decision point well-timed3 and placed in a context where people are in a suitable state of mind?
2. What is seizing people’s attention in that particular context?
3. What happens if people are inattentive at the decision point? Is there a default safety mechanism in place?
Box 1.4. How are attention biases relevant to policymaking?
Attention biases have been shown to affect numerous domains of relevance for policymakers. For example, they can affect the number of people who enrol in pensions (Thaler and Benartzi, 2004) or donate organs (Johnston and Goldstein, 2004) when they do not actively exercise their attention and just choose the default option that a system offers them.
Equally, forgetfulness can have important effects on important health and legal effects if, for instance, people do not show up to court (Ideas42, 2018) or doctor’s appointments because of memory limitations.
Sources: Thaler, R.H. and S. Benartzi (2004), “Save more tomorrow︎: Using behavioral economics to increase employee saving”, Journal of Political Economy, Vol. 112(1), University of Chicago; Johnson, E.J. and D.G. Goldstein (2004), “Defaults and donation decisions”, Transplantation, Vol. 78(12), pp. 1713–6; ideas42 (2018), Using Behavioral Science to Improve Criminal Justice Outcomes Preventing Failures to Appear in Court, https://urbanlabs.uchicago.edu/attachments/store/9c86b123e3b00a5da58318f438a6e787dd01d66d0efad54d66aa232a6473/I42‑954_NYCSummonsPaper_Final_Mar2018.pdf.
Belief formation
It is a well-replicated finding in the social psychology literature that, when asked to compare their driving skills to other people, the majority of participants (up to around 90%, as in Svenson (1981), rank themselves in the top 50% – which cannot possibly be mathematically true.
This form of overconfidence is in line with the behavioural findings on belief formation, which show that individuals tend to rely on a coherent worldview to make predictions and decisions. In doing so, they ignore relevant information that goes against their views or only accept information that confirms these beliefs. The consequence can be over/underestimation of outcomes, missing relevant information and relying “too much” on heuristics (i.e. mental shortcuts or intuitive judgments) to make decisions.
As we will see in the Strategies section, behaviourally informed solutions provide processes and tools to move away from this confirmation bias with a view of supporting good judgment and accurately assessing probabilities.
Guiding questions for belief formation biases:
1. What are people’s pre-existing beliefs? What questions direct their search?
2. How does context interact with belief formation?
Box 1.5. How are belief formation biases relevant to policymaking?
While they are only rarely within the scope of policy discussions, erroneous beliefs can be a real threat to policymakers. For example, if a population misperceives the probability of high-consequence events such as natural catastrophes or terrorist attacks (Sunstein, 2003), this might affect the appropriateness of the time and resources their community spends to prevent them.
Moreover, cognitive traits linked to belief formation, and notably overconfidence, have been linked to issues of high priority for policy, such as financial crises (Lo, 2013) or people’s beliefs about whether climate change will personally impact them (Gifford et al., 2009).
Sources: Sunstein, C.R. (2003), “Terrorism and probability neglect”, Journal of Risk and Uncertainty, Vol. 26(283), pp. 121-136; Lo, A.W. (2013), “Fear, greed, and financial crises: A cognitive neurosciences perspective”, in Handbook of Systemic Risk, Cambridge University Press; Gifford, R. et al. (2009), “Temporal pessimism and spatial optimism in environmental assessments: An 18-nation study”, Journal of Environmental Psychology, Vol. 29, pp. 1–12.
Choice
Have you ever been confronted with too many options for you to possibly choose the best one? This phenomenon is defined as choice overload and is one of the ways in which our decision-making is influenced by behavioural factors.
The context and moment in which we make choices have a distinct upshot on whether we will choose the best option according to our preferences. Often, people value more intrinsic factors and motivation like the feeling of “doing the right thing” than purely material or economic incentives, running sometimes counter to traditional economic models. Failing to think of all rational and irrational aspects of choice can lead to policies that miss the driver of individuals’ decisions.
Behaviourally informed solutions test possible choice mechanisms and use the results to inform decisions.
Guiding questions for choice biases:
1. What makes a given choice attractive to people?
2. How are choices framed?
Box 1.6. How are choice biases relevant to policymaking?
Biases in choices can have negative consequences on important policy outcomes. Think, for example, of how biases are used to sell us things we do not want or guide us to certain choices over others. Instances include restaurants that structure their menus strategically or airline companies that exploit people’s inattention and set the purchase of flight insurance as a default option (European Commission, 2014). Having awareness of these choice biases is fundamental in order to create responsive regulatory regimes that effectively protect consumers in the market. It is in this context that behaviourally informed interventions can be leveraged for “better information disclosure, access to customer service, usage and consumption of data and understanding of bundled services” (OECD, 2016).
Sources: European Commission (2014), “Taking consumer rights into the digital age: over 507 million citizens will benefit as of today”, Press Release, http://europa.eu/rapid/press-release_IP-14-655_en.htm (accessed on 07 November 2018); OECD (2016), Protecting Consumers through Behavioural Insights: Regulating the Communications Market in Colombia, http://dx.doi.org/10.1787/9789264255463-en.
Determination
Taking the right decision is not enough, as anyone who has ever subscribed to a gym and then hardly attended can easily understand! Individuals can have a hard time sticking to some of their choices over time because of issues with will-power, self-regulation, self-control and choice architectures that guide people away from their decisions.
Problems with determination involve the psychological discomfort of not being able to achieve a long-term goal and guide people to search for immediate gratification. Determination challenges can also create mental taxation or exhaustion, which has been shown to decrease our decision-making abilities. Finally, the determination biases can create a climate of inertia and procrastination and eventually lead to excessive self-directed blame.
As the Strategies section suggests, behaviourally informed solutions take into consideration these commitment biases and provide plans and feedback to increase determination, for example by using reminders and commitment devices.
Guiding questions for determination biases:
1. What are the points of friction relative to the desired behaviour? Is it too easy to do the wrong thing?
2. Do people have plans and are they given feedback?
3. How do performance and goal achievement interact with the social context? For example, do people commit to their long-term goals privately or publicly? And what kind of expectations do such commitments create in other people?
Box 1.7. How are determination biases relevant to policymaking?
Intention-action gaps can bring about many policy problems and make existing policies ineffective. For example, they have clear implications for public health, which is hugely impacted by people’s limited ability to act upon their desire to lead a healthy lifestyle, in terms of exercising and eating healthy but also, for instance, quitting smoking.
Similarly, it may impact people’s ability to think long terms, in terms of monetary savings as well as in the case of sustainable behaviour.
Stage 3: Strategies
Building on your behavioural analysis, the next step is to identify behaviourally informed strategies that will effectively change the identified behaviours that you wish to or can address, at the root of your policy problem.
Translating your analysis to actionable strategies inevitably requires some level of familiarity with previous research in the behavioural field, so it is advisable to refer to the expertise of a behavioural team or expert. Nevertheless, having a preliminary understanding of the key principles behind the most common behavioural strategies will facilitate your relationship with behavioural experts and allow you to better understand how to reduce the behavioural barriers at stake.
The goal of this chapter is to get overview of the concepts that you can use to generate behaviourally informed solutions. Because these strategies are strictly grounded in the first two stages of BASIC, the section will also follow the structure of the ABCD framework.
Targeting attention
Attentional issues are rarely at the centre of the development, design and delivery of public policies. Yet, as mentioned in the Analysis stage, inattention is widespread and it can make the difference between failure and success of a policy. For this reason, it may prove effective to revise and design policy interventions so that they become more relevant, seize attention and, if this is not possible, so that they plan for inattention.
Make it relevant
For interventions to work effectively, it is first of all important to engage with people in impactful ways. This means engaging with them at the right time, in the right place and at the point where they are most willing to enact the behaviour that you aim to promote. Some behavioural insights to keep in mind in this regard:
State of mind: People’s abilities and motivation are not constants (Loewenstein, 1996) but rather are influenced by their current state of mind. For example, if you are hungry or tired, you are more likely to make mistakes, make worse decisions and eat bad food.
Timing and placement of an intervention play a huge role in whether people will pay attention. For instance, to increase the likelihood of people paying fines, it might be strategic to time the deadlines of fines and charges relative to when people receive their pay. Similarly, placing healthy food at eye-level rather than far from sight could positively impact people’s choices (Thaler and Sunstein, 2008).
Seize attention
One of the ingredients that can make an intervention successful is simply making sure to seize people’s attention. Behavioural insights shows us that people often fail to attend to what may be perceived to be important in a given context. In light of this knowledge, you should always carefully consider how to design the details of your policy intervention so that people will not overlook what is important for the intervention to succeed. Three BI strategies can help to do so:
Make it salient: Activate, guide or retain focus on a particular aspect of the choice architecture so that people attend to it. A famous example of this principle is a behavioural experiment in the Schiphol Airport in Amsterdam, where engraving silhouettes of flies into the urinals reduced spillage by 80% and cleaning costs by 8% (Evans-Pritchard, 2013).
Reminders: Send reminders and trigger an association in people’s memory. Reminders are becoming increasingly relevant due to digitisation and have been shown to successfully induce behaviour change in a vast array of domains, including health outcomes (Stubbs et al., 2012).
Prompts: Using prompts is another powerful way of grabbing people’s attention. Prompts are now especially common in the online universe in the form of pop-up boxes and they work by interrupting people’s ongoing action and forcing them to make a decision before being able to proceed.
Plan for inattention
Examining what happens when attention fails and then planning and designing for inattention is also a central strategy for dealing with attentional problems.
Defaults
We increasingly rely on defaults or pre-set choices to decide for us when we do not have the time or capacity to carefully examine the vast array of choices available. It is, therefore, crucial to get the arrangement of defaults right and to prevent their misuse. In particular, if your policy issue involves defaults, ensure that these are well aligned with individual and societal preferences, in order to guarantee compliance with ethical standards. It is also important to consider active decision-making, by sometimes still offering a choice, otherwise, defaults could backfire.
Targeting belief formation
What is the best way of preventing the formation of erroneous beliefs and inaccurate interpretations of probabilities? Effective strategies include guiding search, making inferences intuitive and supporting judgments.
Guide search
Problems in belief formation usually go hand in hand with vast amounts of information and possibilities: people have too much information and too little time to process it. Tips for avoiding this include:
Searching by aspects: Allow people to use filters to partition the set of options they are given. This kind of “search by aspects” has proven useful to guide people through complex informational sets, such as in the online search for flights, hotels or job-openings where people can prioritise which features are most important in their search.
Question trees: We are all familiar with call centres guiding us to the right service section by using prompts such as “press 1 for English”. Using this type of Q&A tools (sometimes called “question trees”) is another powerful way to guide users to the right answer and help people find their way around vast and complex sets of information. In the public policy domain, this could, for example, be applied to guide citizens to the information they need when interacting with public bodies.
Make it intuitive
If you want to help people navigate information, it is also important to structure information in a way that is easy for them to understand. The concept of “intuitive coding” might prove crucial for putting in place user-centric interfaces in public policy, such as creating medical prescription forms that are intuitive for any citizen and will help them adopt the right behaviour (King et al., 2014).
Similarly, adjusting information architecture and layout on public websites so that, in BI terms, it conforms to their “mental models” (i.e. resembles what they are used to, searching for, or browse most frequently) may significantly improve the functionality and experience of the service.
Support judgment
People inform their beliefs from pre-existing beliefs. In doing so, people use their intuition and an array of mental shortcuts or intuitive judgments (i.e. heuristics) to make decisions in uncertain contexts. This plays a part in forming beliefs in specific contexts. This knowledge can be used to design effective Strategies:
Adapt to heuristics: Your policy interventions should strive to present information in a way that allows for the appropriate application of heuristics. The setup of the intervention should match, rather than conflict with, people’s intuitions.
Leverage social proof: When people are uncertain about what is the right thing to do in a given context, they often look at the behaviour of others in an attempt to make sense of the world. By highlighting a positive behavioural norm, practitioners may support judgment by “de-biasing” the existing misperception or potentially promote the misperception that the positive behaviour is more common than it actually is, which might result in people adopting the desired behaviour.
Targeting choice
Make it attractive
“The fundamental law of choice is that of attraction” (Hansen, 2018). When faced with a set of options, people usually select the option that they find most attractive. If you want to make your desired policy outcome more appealing, consider the following insights:
Consider motives: What are people’s drivers and incentives in your policy context? Getting a sense of their extrinsic and intrinsic preferences will allow you to align your intervention with people’s strongest motives.
Create perspectives: When people hold weak preferences over options, highlighting an attribute that may provide a secondary motive for choosing among options might be an effective way of helping people make choices.
Trigger emotions: Our emotions are a fundamental factor when navigating choices. To choose, we internally simulate the consequences of making one choice over another. Anticipating your public’s emotions can thus help you understand what kind of strategies will work best in your environment. Ethics is very important in this strategy.
Frame prospects
Whenever we face a set of options, we are also confronted with a set of possible futures, i.e. prospects. Arrangement and formulation of these options matters in determining which choice will be chosen.
Figure 1.5. Arranging choices – Which do you prefer?
Source: Produced by the OECD with images obtained free of copyright from Pixabay user TKaucic (2017) https://pixabay.com/vectors/coffee-cup-cup-of-coffee-drink-2819815/.
To illustrate the potential effect of arranging choices, consider the choice options in the two arrangements in Figure 1.5. As can be understood intuitively, some people who prefer the small coffee in the first setting actually have a preference for the medium size in the second setting, as people have a tendency to choose the middle option rather than extremes (the compromise effect).
Prospect theory, one of the theoretical pillars of behavioural economics, provides an explanation for this and shows that people think in terms of expected utility relative to a reference point rather than absolute terms. Furthermore, the theory indicates that “losses loom larger than gains”, meaning that people are more strongly affected by the prospect of loss than the prospect of gains.
Practitioners might use prospect theory when deciding how to formulate simple prospects, such as those faced by citizens when making everyday decisions in their interaction with public bodies.
Make it social
Humans are, first and foremost, social animals. Yet, this is often overlooked in public policy, where they are treated as individual citizens and consumers. Connecting with the social identities and norms that informally regulate human groups is an invaluable strategy to spark behaviour change. Two main insights to do so include:
Connect with social identities and consider peer comparison: This mechanism is what drives people’s sense of status, recognition and identification with a group and a powerful force behind their behaviours.
Create a sense of community: Would you rather go to a popular theatre or restaurant or to an empty one? Most of people’s choices ultimately have deeply ingrained social dimensions to them. Observing the role that a sense of community may play for how people make choices and creating a sense of community around certain activities may hold the key for influencing and creating behaviour change. This is evidenced by big marathon events, communal eating events, and charity fundraising.
Targeting determination
Finally, we overview Strategies to overcome the intention-action gap. Simply, if you want people to achieve a goal, you should strive to make it easy for them to achieve it. Otherwise, even relatively small obstacles may become a reason for people to postpone action.
Work with friction: Reducing the hassle-factor and simplification can make it easier to take up a preferable service or performing an action. This might translate into reducing the number of actions, clicks or questions people need to go through to achieve a goal. The simpler it is to perform an action, the more likely it is that people will do it.
Provide plans and feedback: As anyone who has been on a diet can understand, behaviour change requires that goal-directed behaviours are not just initiated or considered once or twice, but are also continuously maintained over time. Mental taxation and balancing of competing goals can easily lead to failure. On the contrary, making concrete and specific action plans towards a goal can help people harness their inner resources (boost) and accomplish their goal.
Commitment devices: Make use of strategies that will help people follow their plan of action. For example, encourage people to publicly, rather than privately, commit to a certain goal so they will have the added incentive of maintaining their reputation.
Leverage social norms: These are the mutual expectations that govern the behaviour of members of groups and societies. These can lead people to forego self-serving behaviour in favour of fairness and persist even when everyone in the group would prefer the norm did not exist. In some situations, these norms can help achieve policy goals – especially when promoting pro-social behaviour – but care should be taken as they intervene and make use of the structures and foundations of society.
Stage 4: Intervention
After developing your BI strategies, the next stage is to participate in the design of an intervention that will test which strategies are the most effective to reach your policy outcome. Your understanding of the context and relationships with key stakeholders are essential to identify where, when and among whom the intervention can take place. In parallel, this is another opportunity to rely on behavioural experts who have rigorous research skills and subject matter expertise to lead the intervention design, consult on its implementation and conduct analyses of the results.
At this stage, you can work with stakeholders and behavioural experts to choose which BI strategies are the most relevant because testing too many strategies can become expensive and messy. To increase the likelihood of a successful behavioural intervention, below are some preliminary considerations to discuss with your key stakeholders and behavioural experts. This will enable you to align expectations and make necessary changes early on in the experimental design.
Define success. There no single definition for a successful BI project. Take the time to understand what success looks like for you, your stakeholders and the experts at the start of your project to manage expectations.
Involve user-tests early on when piloting BI strategies. When moving into the research and design stage, a first step is to involve users in testing aspects of the solutions that the strategies give rise to.
Explore research designs. Seek consultation on whether an experiment or another design is the best fit for the project. Explore digital platforms that may make it easier to randomly assign and deliver the intervention in a cost-effective way. If testing in a real-life setting (field trial) is not possible, discuss possibilities of conducting a laboratory or online experiment.
Know your sample size. The bigger the sample size, the better, but it comes at a cost. Work with stakeholders to determine what you both find to be a meaningful difference. Consult the experts who can calculate how many participants are necessary to achieve it and what is the statistically meaningful difference.
Assess the risks of the intervention. Conduct a risks assessment with stakeholders and experts to manage risks and potential unintended outcomes. This may include a null result where no intervention has an effect.
Be realistic about the timeline and budget. In addition to necessary approvals, consult the experts on how much time and resources are necessary for your intervention to have its desired effect (or not). Learn from stakeholders on what is necessary to generate the desired sample size. Build this into your timeline and budget.
Secure legal, ethical and other approvals. Seek necessary approvals required to carry out the experimental design. If you are working with external experts understand what is required to obtain their institutions’ approvals to consult on the project (i.e. institutional review board).
Be transparent about data sharing and publications. When engaging with external experts such as those from academic institutions that value novel research and scientific publications, it is important to be explicit about what data can be shared and the user-rights of results for external publications.
Consider options for communicating results, long-term monitoring and scaling. Outline a game plan on how to move forward after data collection and analyses, accounting for the possibility of null or negative results. Refer to the Change section for guidelines.
Ways to know “what works”
Randomised controlled trials (RCTs)
How do you know whether your new strategy works or if other uncontrollable factors are driving your policy outcome? A randomised controlled trial (RCT) is the ideal way to solve this dilemma. The key feature of an RCT is the use of a random assignment to create at least two groups that closely resemble each other. The only difference is that one group is exposed to the new strategy while the other does not. By comparing identical groups, chosen at random, an RCT enables you to understand which strategies, if any, are working, and eliminate pre-existing or external factors that normally complicate the evaluation process. You may be more familiar with RCTs in medicine where RCTs are used to compare a new treatment with the current one or a placebo.
Often you may want to determine whether a new policy is more effective in achieving your measurable policy outcome compared to the status quo. Say you implemented a city-wide school breakfast programme with the aim of increasing school attendance. The current policy requires schools to provide free breakfast in the cafeteria before school starts, but student participation remains low. Your behaviourally informed strategy is to “make it relevant” by changing the placement and timing. Your new policy is to offer breakfast in the classroom at the start of the school day.
To test the effectiveness of the new strategy against the existing policy, you identify 300 schools (“sample size”) who already run the school breakfast programme and have agreed to take part in the pilot. From there, you randomly assign 150 schools to offer breakfast in the classroom (“the treatment group”) and the other half to the normal practice of breakfast in the cafeteria (“the control group”) (Figure 1.6).
The beauty of random assignment is that it eliminates uncontrollable pre-existing or external factors (i.e. improvements in public transportation) that may affect school attendance because the schools in the treatment group are not systematically different from those in the control group. At the end of your experiment when you compare school attendance, the difference between the two groups should only be a result of your intervention. If you are testing multiple strategies, it is still necessary to include a control group to know how the new strategies did against the status quo. In the above example, you can see that four schools saw an increase in school attendance while one school saw a decrease. How do you know if you are better or worse off under the new policy? The control group shows us that only two schools saw an improvement while two schools saw a decline in attendance. So, you can say that the classroom breakfast policy led to an overall improvement in school attendance compared to the cafeteria breakfast policy. If the new policy was no better than the current one, you would have seen a similar pattern in both the treatment and the control groups.
Figure 1.6. Simple randomised controlled trial (RCT) example
Alternative to RCTs
RCTs are the ideal way to determine if your intervention led to your desired policy outcome but random allocation may not always be logistically, ethically or politically feasible. In this case, consult a behavioural expert about conducting a quasi-experiment – an experimental approach that is similar to an RCT, but participants are not randomised into control and treatment groups (Campbell and Stanley, 2015). You can no longer eliminate pre-existing or external factors that may influence your outcome but you can still generate evidence to learn which strategies are more effective. Examples of valuable quasi-experimental designs include:
Regression discontinuity (RD): where participants are assigned to treatment and control groups based on a cut point of an assignment variable. The discontinuity between the treatment and control trends is then measured.
Propensity score matching (PSM): where participants in the treatment group are paired to participants in the control group based on the similarity of their scores to account for selection bias.
Difference in differences: where the effect of a treatment or of a policy is estimated by comparing the pre- and post-treatment differences in the outcome in the treatment and control group.
Stage 5: Change
Your team has implemented the Intervention and examined the findings – now what? You want to avoid stopping at the proof-of-practice stage if indeed the findings suggest that the intervention could have an impact. The fifth and final stage, Change, is the time for you to look back at your BI exercise and think of its long-term implications.
By this stage, you will know whether the tests have produced promising results and a behavioural insight can be scaled up into a full policy intervention – or whether repeated failure brings the project to an end and the community can learn from what did not work. Change is a crucial decision point for policymakers – at this time, you really have the decision power to determine which direction to take the project.
The goal of Change is to inform public policies on the basis of the results of the project and to ensure that society gains the broadest possible value from the insights of the experiment. BASIC suggests five final considerations on this note:
1. Revisiting the political context and project level. Are the interventions aligned with the current context such as political, and technological, state of affairs? Are the results sufficiently promising to motivate policy action, in light of the current legal landscape? Would the intervention be in line with public opinion or feasible in the current climate? Are the resources in place to implement the intervention in the long term?
2. Implementing and scaling behaviourally informed policies. How can you best implement and scale up your intervention? Should you change a law, regulation or regulatory regime (OECD, 2016)? Is it possible that the results might fail to generalise when scaled? Keep in mind that through the implementation and scaling up of a behaviourally informed policy, certain groups could be more or less affected than what was suggested by the intervention. This, in turn, may lead to further iterations and tweaks in the design of the policy in question.
3. Setting up monitoring of long-term and potential side effects. Because most BI experiments are limited in time span, plan to have specific plans for monitoring the scaled-up policy in the long term as well as its potential side effects. This may be done by integrating an ex post evaluation or review of a given policy as a required step of the policymaking process. In this way, evaluations or reviews will help ensure the quality of policy over time.
4. Maintaining the policy initiative. While crucial to avoid watering down behavioural policies, sometimes maintenance of BI interventions may be neglected. This can happen because BI features may appear as unimportant or may be in conflict with what seems necessary from a more rational perspective. Therefore, it is important to have instructions for the proper maintenance – physical or systemic – of the policy. To avoid problems with maintaining a policy initiative over time, practitioners should consider what audiences need to be involved in the maintenance and produce material and instructions that fit these audiences and the situations in which this material is to be used (Hansen, 2018).
5. Disseminating knowledge widely. While the idea of disseminating results is common in the behavioural community, it is still not so in most public institutions. As a result, many early BI projects were not reported at all or only for internal use. In particular, null results have not been widely publicised leading to publication bias. Likewise, the lack of standards has led to non-transparent reporting; reporting without moderators; reporting only in local languages; overstatement of effects, savings and revenues; and understatement of true costs (for examples, see OECD, 2017a, and Osman et al., 2018). For this reason, it is crucial that researchers and practitioners participate, support and systematically share and report their work in national as well as international networks of both researchers and policymakers.
Ethical considerations
Applying BI to public policy can raise specific ethical concerns as BI uses data on individual or group behaviours, as well as experimental methods for testing these theories at small scales before implementing more broadly. As a result, issues related to privacy, consent or the ethics of applying certain solutions to only some groups arise.
First, consider some general principles to dispel some misconceptions on the ethics of BI:
While we are always being behaviourally influenced, this does not exempt behaviourally informed interventions from ethical evaluation. When applying BI, you are intentionally trying to intervene to change the behaviour of citizens. This means citizens will experience influences that they would not otherwise, requiring an ethical evaluation.
Public acceptance of BI does not make it always ethically permissible. What is acceptable is not necessarily what should be acceptable and ethical considerations should be discussed for each intervention.
While people may avoid a behavioural intervention in principle, this does not mean that they can in practice. BI interventions neither force individuals to act a certain way nor sanction them for not acting a certain way. This does not mean they are always free to choose how they want and issues related to consent and awareness needs to be carefully considered since individual are inherently not ideally rational.
You should then discuss and consider the ethical implications of the intervention.
Before starting a behaviourally informed intervention
Consider establishing an ethical review board from day one. If time and resources do not allow it, then outline the ethical issues associated with the project, how to address them and continuously consider where ethical approval may be required. A university ethical review board may be considered for expert advice and the use of established ethical approval process can be used.
Appoint ethical supervision of data collection, use and storage. BI often involves data collection and analysis that goes beyond what is standard in public policymaking. Consider appointing at least one member – either a member of the ethical review board or the team working on the behaviourally informed intervention – to supervise ethical aspects of data collection, use and storage.
Observe existing ethical guidelines and codes of conduct. Make sure all team members observe ethical guidelines and codes of conduct, which are often already present in public institutions. Where existing standards are not sufficient for BI, flag these issues and establish procedures for these instances. Ensure appropriate procedures are in place to protect whistleblowing and ensure anonymity is respected.
When going through each of the BASIC steps
Behaviour
Observe the limits of legitimate public policy interventions. Not all behaviours driving a policy problem fall within the legitimate confines of policymaking. Make sure that you refrain from targeting and changing behaviours that cannot be defended as being in the public interest or aligned with government priorities.
Secure acceptance when targeting behaviours. Policymakers suffer biases too, which can influence the decision to target certain behaviour(s). To avoid these biases, always evaluate the existing evidence for targeting a given behaviour change.
Beware not to simplify behaviour too much. Behavioural analysis of policy problems aggregate patterns of groups, yet individuals usually hold distinct preferences. Distributional impacts may also result in some citizens being influenced differently than others. Always consider how to minimise potential side effects and protect individual rights, values and liberties when targeting behaviour change.
Analysis
Behavioural analyses usually observe or study human behaviour close up and often in their individuals’ everyday environments, running the risk of affecting participants’ personal lives and colliding with people’s privacy.
Seek ethical approvals and competencies where necessary. Use the ethical review board or relevant authorities within which the behaviour is studied to grant approval. If using a third party to conduct the study, this ethical responsibility cannot be transferred. Ensure appropriate training to develop sufficient competencies for data use and analysis.
Consider what guidelines must be followed when studying behaviour up close. These include collecting and documenting consent, revealing the purpose of the study, ensuring participants are voluntarily participating and additional safeguards are in place when studying vulnerable populations.
Only collect data that is necessary and ensure secure handling. Ensure that those handling the data are properly instructed in the secure collection and handling of data.
Strategies
Some behavioural insights rely on mechanisms that are not fully accessible to consciousness or under people’s conscious control, while others involve counter-intuitive and theoretical insights whereby moral intuitions are not well adapted.
To ensure the responsible use of BI in public policy, you should always evaluate the morality of a policy strategy with regards to transparency and “avoidability”. Transparent insights are when citizens can identify: i) who is trying to influence them; ii) what this means; and iii) what purpose is being achieved. Alternatively, behaviours that people cannot control are referred to as unavoidable, while those that make possible or depend on conscious control are referred to as avoidable.
When assessing the transparency and “avoidability” of policy intervention, keep in mind the following considerations:
Prioritise transparency. Is your intervention clearly communicated, including being transparent about its purpose and nature?
Offer a way out. Can citizens avoid the intervention? Does the intervention offer easy pathways to objections and complaints?
Ensure the policy intervention serves the public interest. Is it in line with public sentiments? Does it prevent harm against others?
Ensure citizens are not being held responsible for consequences that they did not consciously select. In your context, are they able to fully understand the implications of their choices? Are they considered legally accountable for these?
Intervention
Be aware that interventions unavoidably intervene in people’s lives. Experiments intentionally give one group a treatment that is believed to have a positive impact, while withholding this treatment intentionally from another group. Consider three sources for help ensuring interventions are carried out ethically: i) ethical codes of conduct; ii) informed consent; and iii) institutional review boards. These sources may not be uniformly applicable to all experimental research. You must orient yourself within the standard ethical guidelines and codes that fit into the special circumstances of the specific behavioural interventions.
Consider whether legal permission is required and demonstrate the necessity of the experiment. You should consider if the laws in your country deem experimentation as legally permissible in public service. It may also be necessary to demonstrate that the intervention will improve a policy situation, reveal knowledge not currently known, provide necessary data, be used to inform policy and protect the rights of individuals.
Always consult experience. Make sure that experiments are conducted by people with experience in experimental design, intervention and reporting to ensure proper protocols are followed.
Ensure justice, fairness and distributional impacts are considered. You need to consider and address the potential ethical issues that arise from one group receiving treatment, and the other not. This may require deploying safety valves for discontinuing the experiment for ethical reasons or compensating/offsetting groups after the experiment.
Take all measures to protect confidentiality and ensure ethical data analysis. You should carefully consider using procedures and protocols that ensure the confidentiality of participants’ responses, e.g. by using randomised response methods or determining not to collect or connect any data about potential identifiers. Ethical data analysis can be strengthened by pre-registering studies, over accounting for data outliers and truthfully reporting on attrition, to strictly follow standards of statistics and their representation.
Change
Adhere to principles of proper stakeholder engagement. Make sure to involve public bodies, staff, citizens, businesses and other affected parties are properly consulted and the results of this consultation are clearly communicated.
Follow principles of transparency and accountability. Results of experiments and consultations should be shared with executive and legislative branches, as well as with broader society. This includes ensuring proper credit is given to the policymakers and government agencies who ran the experiments.
Report on what works and what does not. Other policymakers, society and the research community should learn from your efforts. This includes reporting on null results and unexpected effects to avoid exposing citizens to interventions that have already been shown to fail.
Monitor long‑term and side effects. In implementing behaviourally informed interventions, you also have the responsibility for developing plans for monitoring the effects of the interventions to protect citizens from the potential negative consequences.
Conclusions
What are the ingredients of good behavioural public policies? When and how should BI be applied to policymaking? Can we use our knowledge of how people think and make decisions to create more effective interventions? The BASIC Guidebook addresses these fundamental questions and provides a starting point to learn about the process of incorporating BI into policymaking.
The guide offers an introductory look at how to identify, scope and address the behavioural aspects of a policy problem and emphasises the importance of applying BI from the beginning to the end of the policy cycle. It aims to show how a nuanced understanding of human behaviour can provide new tools for policymakers. Introducing subtle changes to choice of architecture, factoring in people’s social preferences, working to facilitate people’s decision-making, can all be powerful drivers of behaviour change.
This introductory guide provides a tool for incorporating these insights when designing and implementing public policies, by overviewing the basic steps and best practices of behavioural insights. Once you have gained an initial understanding of these through the guide, we recommend referring to the BASIC Manual for a more detailed and comprehensive depiction of the BI approach, that will allow you to fully grasp the rationale and mechanisms of the BASIC framework.
References
Campbell, D.T. and J.C. Stanley (2015). Experimental and Quasi-experimental Designs for Research. Ravenio Books.
Chetty, R., A. Looney and K. Kroft (2009), “Salience and taxation: Theory and evidence”, American Economic Review, Vol. 99(4), pp. 1145-77.
Dallas, S.K., P.J. Liu and P.A. Ubel (2019), “Don’t count calorie labelling out: Calorie counts on the Left side of menu items lead to lower calorie food choices”, Journal of Consumer Psychology, Vol. 29(1), pp. 60-69.
Doran, G.T. (1981), “There’s a SMART way to write management’s goals and objectives”, Management Review, Vol. 70(11), pp. 35-36.
European Commission (2014), “Taking consumer rights into the digital age: over 507 million citizens will benefit as of today”, Press Release, http://europa.eu/rapid/press-release_IP-14-655_en.htm (accessed on 07 November 2018).
Gibbons, S. (2018), Journey Mapping 101, Nielsen Norman Group, https://www.nngroup.com/articles/journey-mapping-101/.
Gifford, R. et al. (2009), “Temporal pessimism and spatial optimism in environmental assessments: An 18-nation study”, Journal of Environmental Psychology, Vol. 29, pp. 1–12.
Hallsworth, M. et al. (2015), “Stating appointment costs in SMS reminders reduces missed hospital appointments: findings from two randomised controlled trials”, PLOS One, Vol. 10(9), https://doi.org/10.1371/journal.pone.0137306.
Hertwig, R. (2017), “When to consider boosting: Some rules for policy-makers”, Behavioural Public Policy, Vol. 1(2), pp. 143-161.
ideas42 (2018), Using Behavioral Science to Improve Criminal Justice Outcomes Preventing Failures to Appear in Court, https://urbanlabs.uchicago.edu/attachments/store/9c86b123e3b00a5da58318f438a6e787dd01d66d0efad54d66aa232a6473/I42-954_NYCSummonsPaper_Final_Mar2018.pdf.
ideas42 (2017), Define, Diagnose, Design, Test, http://www.ideas42.org/blog/first-step-towards-solution-beta-project/ (accessed on 06 November 2018).
IRS (2017), Behavioral Insights Toolkit, US Internal Revenue Service, https://www.irs.gov/pub/irs-soi/17rpirsbehavioralinsights.pdf.
Johnson, E.J. and D.G. Goldstein (2004), “Defaults and donation decisions”, Transplantation, Vol. 78(12), pp. 1713–6.
Kahneman, D. (2011), Thinking, Fast and Slow, Macmillan.
Lo, A.W. (2013), “Fear, greed, and financial crises: A cognitive neurosciences perspective”, in Handbook of Systemic Risk, Cambridge University Press.
Ng, C.F. (2016), “Behavioural mapping and tracking”, Research Methods for Environmental Psychology, Vol. 29.
OECD (2017a), Behavioural Insights and Public Policy: Lessons from Around the World, OECD Publishing, Paris, http://dx.doi.org/10.1787/9789264270480-en.
OECD (2017b), Obesity Update 2017, OECD Publishing, Paris, http://www.oecd.org/health/health-systems/Obesity-Update-2017.pdf
OECD (2016), Protecting Consumers through Behavioural Insights: Regulating the Communications Market in Colombia, OECD Publishing, Paris, http://dx.doi.org/10.1787/9789264255463-en.
OECD/OPSI (n.d.), Practical Service Blueprinting – The Guide, Practical Service Design, OECD Observatory for Public Sector Innovation, https://oecd-opsi.org/toolkits/practical-service-blueprinting-the-guide/.
Osman, M. et al. (2018), “Learning lessons: How to practice nudging around the world”, Journal of Risk Research, pp. 1-9, http://dx.doi.org/10.1080/13669877.2018.1517127.
Sunstein, C.R. (2003), “Terrorism and probability neglect”, Journal of Risk and Uncertainty, Vol. 26(283), pp. 121-136.
Svenson, O. (1981), “Are we all less risky and more skilful than our fellow drivers?”, Acta Psychologica, Vol. 47(2), pp. 143-148, http://dx.doi.org/10.1016/0001-6918(81)90005-6.
Thaler, R.H. and S. Benartzi (2004), “Save more tomorrow︎: Using behavioral economics to increase employee saving”, Journal of Political Economy, Vol. 112(1), University of Chicago.
Thaler, R.H. and C.R. Sunstein (2009), Nudge: Improving Decisions about Health, Wealth, and Happiness, Penguin Books, New York.
The Behavioural Insights Team (2014), EAST Four Simple Ways to Apply Behavioural Insights, The Behavioural Insights Team, https://38r8om2xjhhl25mw24492dir-wpengine.netdna-ssl.com/wp-content/uploads/2015/07/BIT-Publication-EAST_FA_WEB.pdf.
The Behavioural Insights Team (2013), Test, Learn, Adapt: Developing Public Policy with Randomised Controlled Trials, The Behavioural Insights Team, https://38r8om2xjhhl25mw24492dir-wpengine.netdna-ssl.com/wp-content/uploads/2015/07/TLA-1906126.pdf.
The Behavioural Insights Team (2010), MINDSPACE, The Behavioural Insights Team, https://www.behaviouralinsights.co.uk/publications/mindspace/ (accessed on 11 April 2019).
Wilcox, K. et al. (2009), “Vicarious goal fulfilment: When the mere presence of a healthy option leads to an ironically indulgent decision”, Journal of Consumer Research, Vol. 36(3), pp. 380-393.
World Bank (2015), The World Development Report 2015: Mind, Society and Behaviour, World Bank, Washington, DC, http://www.worldbank.org/content/dam/worldbank/publications/wdr/wdr%202015/wdr-2015-full-report.pdf.
World Obesity Federation (2015), About Obesity, World Obesity Federation, England and Wales.
Notes
← 1. Behaviour, Analysis, Strategic, Intervention and Change are the five stages of the BASIC process for applying behavioural insights to public policy.
← 2. In the BASIC manual, the core stages of BASIC are referred to in small caps (i.e. “Behaviour”) to distinguish the stage from the regular use of the word (i.e. in Behaviour you diagnose the behaviour problems).
← 3. Italics in guiding questions in this section refer to behavioural strategies developed in Stage 3.