Human capital is widely regarded as a fundamental input in growth theory. Furthermore, recommendations to boost human capital feature prominently among structural policy priorities identified by the OECD for a number of countries. However, the empirical evidence linking human capital with macroeconomic outcomes has been problematic. This chapter first provides an overview of the role of human capital in determining economic outcomes and reviews existing evidence on this topic. It then presents a new measure of human capital based on OECD education data surveys, which better incorporates both quality and quantity dimensions. Drawing on the proposed measure of human capital, this chapter suggests a substantial scope for long‑run productivity gains from human capital, with a larger effect from quality as compared to quantity improvements, although the lags are typically much longer than for other policies that boost productivity.
Value for Money in School Education
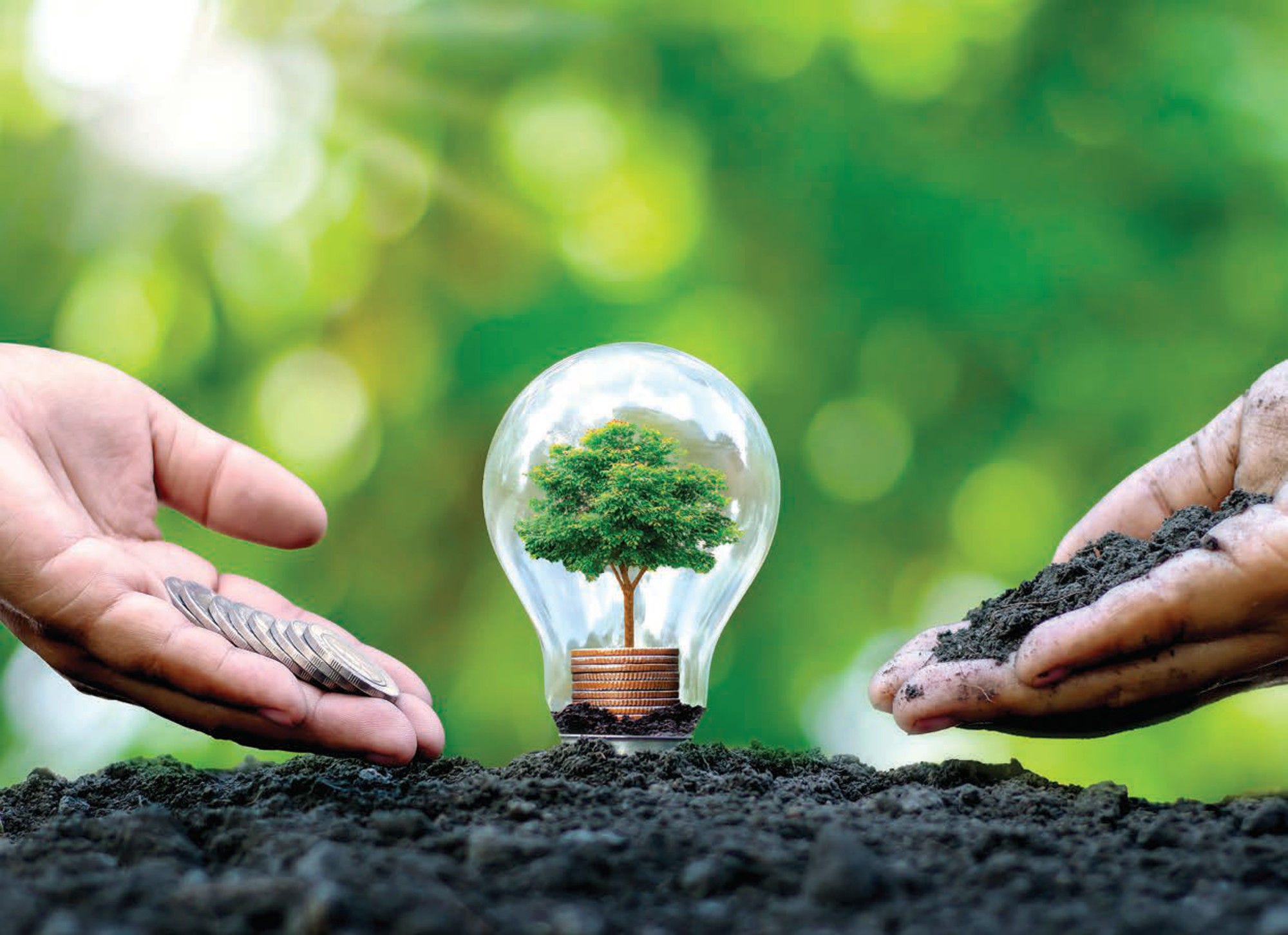
1. The importance of human capital for economic outcomes
Abstract
Introduction
The fiscal response of OECD governments to the COVID-19 crisis has been swift. Across the OECD, governments have committed billions of dollars to support public health systems, prevent massive business failures and protect households from the impact of the crisis. The additional spending (or forgone revenue) amounts to around 10% of Gross Domestic Product (GDP) on average in OECD countries over 2020-2021, with wide disparities across countries (IMF, 2022[1]). The aid to the health sector represents on average 1.5% of GDP. Public debt ratios in 2023 are projected to exceed 2019 levels considerably (by 15 percentage points in the median OECD economy) (OECD, 2022[2]). They will need to be adjusted over the medium term given future demands on public finances from long-term trends such as ageing populations (Guillemette and Turner, 2021[3]) and climate change. Boosting growth will help reduce the debt-to-GDP ratios. However, more recent developments related to the consequences of Russia’s war of aggression against Ukraine are likely to further strain public finances. While stagnating economic growth will lower fiscal revenues, increased expenditures are expected in many OECD countries: rocketing energy costs call for efforts to support households and small businesses, rising interest rates put pressure on public debt, and spending on defence gains new priority in the new geopolitical context (OECD, 2022[4]; NATO, 2022[5]).
Substantial public resources will continue to be needed to support the health sector further, to address the economic consequences of the new geopolitical situation and to accelerate the green and energy transitions in an effort to limit climate change and enhance energy sovereignty. Finance ministries will therefore be faced with complex choices and competing budgetary priorities in seeking to balance short-term and long-term economic, geopolitical, environmental and social goals. In this context, it is useful to undertake a critical assessment of the importance of various pillars of economic growth, and more specifically the importance of education, for economic outcomes.
From an economic perspective, sustained high-quality education constitutes a long-term investment in the knowledge, skills and competencies of people, leading to higher productivity, earnings and quality of life for individuals. At the macro level, a well-educated workforce is a key factor in achieving greater aggregate productivity, innovation and long-term economic growth. Yet, these economic benefits are not always straightforward to measure, in part due to data constraints.
Human capital is widely regarded as a fundamental input in the theoretical growth literature.1 Furthermore, recommendations to boost human capital feature prominently among structural policy priorities identified by the OECD for a number of countries (OECD, 2021[6]). However, despite this emphasis in both economic theory and policy practice, the empirical evidence linking human capital with economic outcomes has been problematic, especially at the macroeconomic level.
This chapter provides a short overview of the role of human capital in determining economic outcomes, including both microeconomic and macroeconomic evidence, and concludes by summarising a proposal for a new measure of human capital, which better incorporates both quality and quantity dimensions by using OECD data from the Programme for International Student Assessment (PISA) and the Survey of Adult Skills (PIAAC). Incorporating this new measure of human capital within the OECD’s standard framework for assessing structural reforms suggests substantial scope for long-run productivity gains from human capital, with greater potential from improvements in the quality than the quantity component of human capital, although the lags are typically much longer compared to other policies that boost productivity.
This chapter is organised around five sections:
First, the chapter provides a brief account of the literature on the role of human capital for economic outcomes, and its importance as a key structural policy priority in OECD countries.
Second, the chapter elaborates on the definition of human capital and the conceptual framework underpinning the concept.
Third, the chapter reviews the empirical microeconomic evidence on rates of return to education for individuals, in terms of employability premia and equilibrium effects.
Fourth, the chapter outlines the empirical macroeconomic evidence deriving from growth accounting and cross-country regression analyses.
Finally, the chapter offers a proposal for a new measure of human capital based on OECD PISA and PIAAC and mean years of schooling data and examines the effects of this new measure of human capital on multi-factor productivity.
Human capital is among the main OECD structural policy priorities
The OECD has consistently singled out education and skills development as a key structural policy priority in its economic reviews of OECD and non-OECD countries…
Boosting human capital is one of the main structural policy priorities recommended by the OECD for a large number of OECD and non-OECD countries (OECD (2021[6]) and Figure 1.1. ). In both cases, recommendations related to education and skills development account for the second largest share of total policy priorities behind product market reform. Further, the recommendations concern all levels of formal education and training in the workplace (Figure 1.2. ):
Expanding the quality of pre-school childcare. Evidence shows that early childhood education and care provide the basis for a child’s future skills development and learning. This is particularly true for disadvantaged children (OECD, 2018[7]). Expansion of pre-primary education improves educational attainment, labour market attachment and welfare dependency (Braga et al., 2013[8]; Havnes and Mogstad, 2011[9]).
Improving teacher quality. Within schools, teaching quality is the single most important factor that affects students learning (OECD, 2005[10]). The quality of teachers is an elusive concept that cannot be directly measured reliably, but it has been proxied, for instance, by the share of certified teachers, the PIAAC score of teachers, or teachers’ wages, which have all been found to show a positive association with student test scores (Hanushek, Piopiunik and Wiederhold, 2019[11]; Dolton and Marcenaro-Gutierrez, 2011[12]). These findings suggest that teacher quality does indeed matter, although the exact mechanisms at play remain to be investigated.
Improving tertiary education. Tertiary education has an impact on wage earnings of its graduates. Higher education institutions that have the autonomy to manage their financial resources, staff policies and the selection of students have been often shown to achieve better education outcomes (Oliveira Martins et al., 2007[13]).
Developing lifelong learning. While technology is evolving rapidly, workers need to adapt quickly to the new requirements of the labour market. Skills acquired in formal education or in previous jobs become obsolete more quickly. Lifelong learning is key to help workers upgrade and expand their skills and adapt to technological change (OECD, 2021[14]). On average across OECD countries, 57% of medium- and high-income adults participate in adult learning, while only 35% of low-income adults do so (OECD, 2019[15]). Policies should aim at raising the participation of people with low levels of education in lifelong learning. Denmark, Sweden and Norway managed to attain the same participation of workers regardless of income level (OECD, 2019[16]).
This emphasis on education and skills development is consistent with the prominence of human capital as a key driver of productivity and growth in economic theory
The prominence of education policy recommendations in structural reform priorities is not surprising considering the strong theoretical underpinnings of the importance in human capital in productivity and income developments. However, efforts at providing empirical evidence have been more mixed, not least because of the difficulty of developing a measure of human capital that can adequately capture intangible characteristics such as knowledge and skills. This is briefly reviewed in the next sections.
Figure 1.1. Human capital among the main structural policy priorities
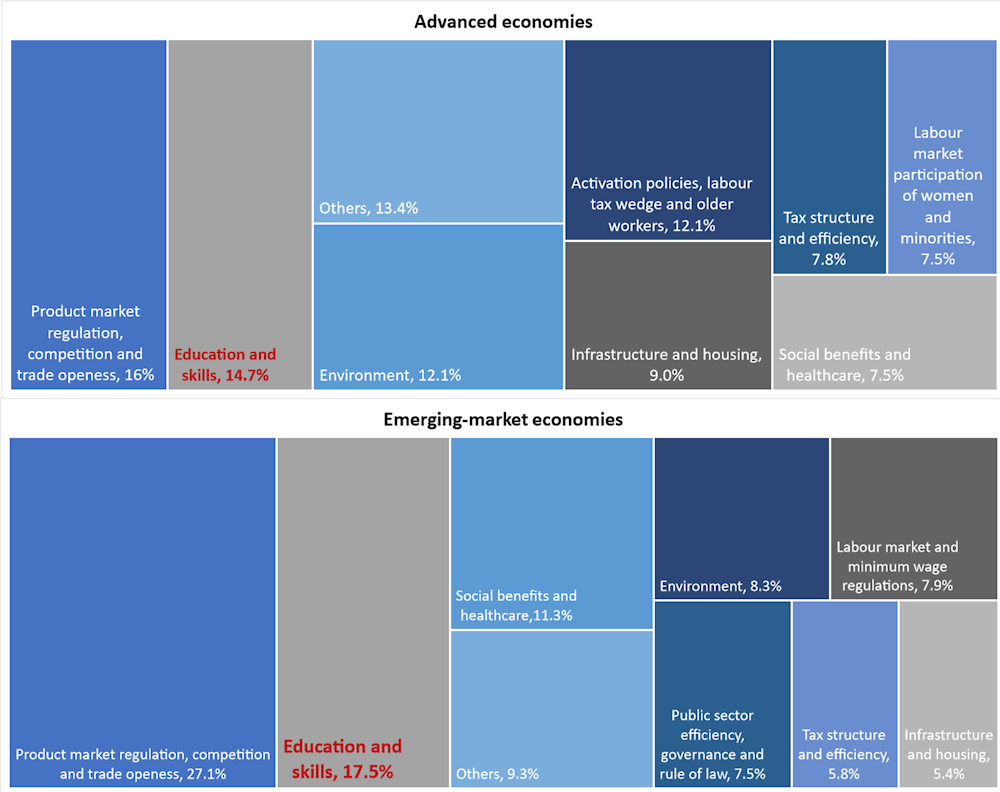
Source: Adapted from OECD, (2021[6]) Economic Policy Reforms 2021: Going for Growth: Shaping a Vibrant Recovery, https://doi.org/10.1787/18132723, Figure 2.
Figure 1.2. Policy recommendations regarding human capital
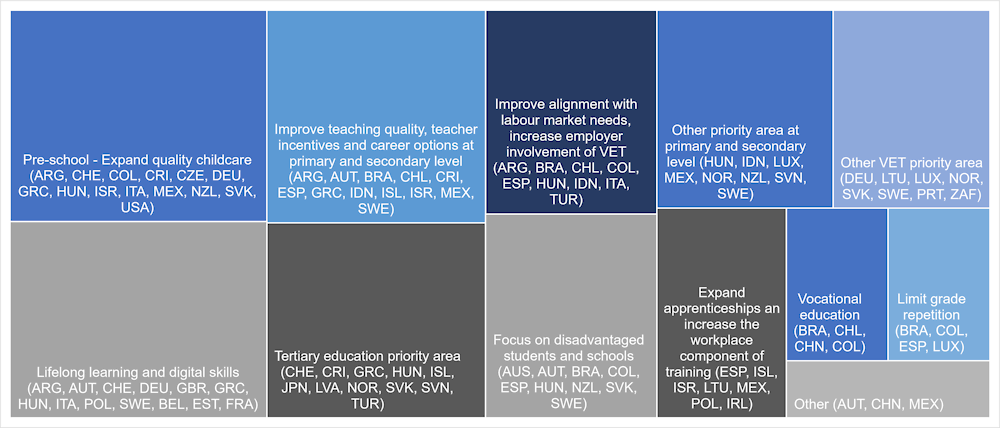
Source: OECD (2021[6]), Economic Policy Reforms 2021: Going for Growth: Shaping a Vibrant Recovery, https://doi.org/10.1787/18132723, Figure 1.A.8.
Human capital as a driver of economic outcomes: definition and conceptual framework
Economic theory has long envisaged human capital as a limited stock of knowledge and skills conceived as a production factor with a finite impact on GDP per capita
Human capital can be broadly defined as the stock of knowledge, skills and other personal characteristics embodied in people that help them to be more productive. Investment in human capital includes investment in formal education (early childhood, formal school and higher education system, adult training programmes), but also informal and on-the-job learning and work experience. A wider definition includes health as well.
Understanding the determinants of economic growth has occupied economists for decades. Starting with a standard aggregate production function where the output of the economy is a function of labour, capital and technological change (Solow, 1956[17]), the augmented neoclassical growth models incorporated human capital as a production factor (Mankiw, Romer and Weil, 1992[18]). In these models, the change in gross domestic product per worker is linked to the change in human capital, the latter having only a limited impact as the capacity for a country to invest in human capital faces natural constraints (Hanushek and Woessmann, 2021[19]).
But endogenous growth models have reinforced interest in human capital, arguing that it also contributes to long-term growth indirectly, through innovation diffusion and TFP2
By contrast, the endogenous growth literature argues that growth is eventually driven by innovation and that human capital is key to raise the innovative capacity of a country (Schumpeter (2006[20]), Lucas (1988[21]); Romer (1990[22]); Aghion and Howitt (1997[23])). In these models, the changes in GDP per worker are linked to the level of human capital, the latter influencing long-run growth rates. A last strand of literature argues that human capital facilitates the diffusion of technologies (Nelson and Phelps (1966[24]); Welch (1970[25]); Benhabib and Spiegel (2005[26])). In these models, human capital influences growth directly and indirectly through its impact on total factor productivity.
A key question is whether the relationship between human capital and growth is causal i.e. if higher human capital generates more growth or if higher growth leads to higher human capital. This question is essential as policy makers expect the policies directed to improve human capital to have a positive effect on long-term growth. The first studies analysing the link between the years of schooling and growth may have encountered reverse causality issues (Bils and Klenow, 2000[27]; Hanushek and Woessmann, 2021[19]). By contrast, using student performance as a measure of human capital should be less prone to misinterpretation, as it is unlikely that higher growth leads to higher student performance. Indeed, academic literature has found little impact of an increase in education spending on student performance, so there is little chance that higher growth-induced expenditure alters student performance (Hanushek and Woessmann, 2011[28]).
Empirical microeconomic evidence of the outcomes of education for individuals
In human capital theory (Becker, 1967[29]), schooling is seen as an optimising investment decision based on future benefits and the costs of education. While there are costs associated with the pursuit of studies, the individual returns to education can be large (Schultz, 1961[30]; Mincer, 1974[31]). More education is supposed to increase the productivity of individuals which will translate into higher salaries and higher employability probability.
Empirical estimates of rates of return to education show positive returns to more time spent in education
A general view is that the demand for education depends on the economic incentives associated with studying (Becker, 1967[29]; Freeman, 1986[32]). The common use of mean years of schooling (MYS) in many panel regressions as a proxy measure of human capital relies on two assumptions: i.) returns to education do not differ across countries and over time; ii.) returns increase linearly with the quantity (years) of education. The second assumption is based on microeconomic evidence from Mincerian wage equations according to which log wage earnings is a linear function of the time spent in the education system (and a positive, but decreasing, function of work experience). The specification is named after Jacob Mincer (1958[33]; 1974[31]) and has been described as "one of the most widely used models in empirical economics".
Against this background, empirical studies from the late 1990s started to question the assumption of linear returns to years of schooling and instead assumed decreasing marginal returns, so that primary education had the biggest marginal returns, followed by secondary education, with tertiary education having the lowest returns. A first wave of studies relied on piece-wise linearity assuming returns of 13.4%, 10.1% and 6.8% for primary, secondary and tertiary education, respectively (Hall and Jones, 1999[34]; Caselli, 2004[35]; Feenstra, Inklaar and Timmer, 2015[36]). A second wave relied on a polynomial specification, advocated by Morrisson and Murtin (2013[37]), which smoothed out the step decreases in the piece-wise linear form of decreasing returns.
The most recent and reliable data suggest that average returns to primary, secondary and tertiary education are U-shaped relative to the time spent in education (Psacharopoulos and Patrinos (2004[38]); Montenegro and Patrinos (2014[39])). The pattern of returns has important implications for measures of human capital. In particular, assuming U-shaped, increasing or decreasing returns yields considerable differences not only in the level, but also in the slope, of the human capital variable.
Education also translates into employability premia
Acquiring more education entails a lower risk of unemployment and a higher likelihood of labour market participation. The gap in unemployment risk across the different levels of education is particularly large for the young, and tends to narrow with age (Blöndal, Field and Girouard, 2002[40]). Using the Heckman two‑step method to assess and correct for the selection bias (Heckman, 1979[41]; Heckman, Lochner and Todd, 2005[42])), evidence shows that the estimated conditional probability of employment for a tertiary education holder is around two percentage points higher than for an upper-secondary degree holder (Boarini and Strauss, 2007[43]).
But microeconomic studies cannot easily integrate general equilibrium effects to estimate the overall macroeconomic impact of a policy change
A weakness of microeconomic studies is that they cannot easily integrate general equilibrium effects, as the returns to education are estimated on data corresponding to a given state of the economy, with given prices and policies. Thus, the estimated marginal impact on any individual’s income may not be representative of the overall macroeconomic impact on the economy if a new policy is applied widely.
Empirical macroeconomic evidence of the aggregate impact of education
While theoretically human capital is a key determinant of growth, finding an empirically robust relation between human capital and growth at the macroeconomic level is not an easy task because, among other things, there is no widely accepted definition of human capital.
In growth accounting studies, the contribution of human capital to cross-country differences in income depends on the measure of human capital used
The growth accounting methodology consists in decomposing income differences across countries into the variation of their components: physical capital, human capital and a residual, the total factor productivity, which represents technical progress. The exercise requires income and its components to be measured correctly and is dependent on the assumed functional form of the production function (Flabbi and Gatti, 2018[44]).
The contribution of human capital to cross-country differences in income depends on the measure of human capital used. Using secondary school enrolment, Mankiw et al. (1992[18]) find that the difference in human capital explains 50% of income differences. Using years of schooling attainment, Klenow and Rodriguez-Clare (1997[45]) find that human capital accounts for only 10 to 30% of income differences. Adjusting human capital for quality leads to broadly the same finding that human capital explains a smaller share of income differences (Caselli, 2004[35]). Under the assumption of perfect substitutability of skilled and unskilled workers, those studies aggregate the different types of human capital into a single measure.
Removing the perfect substitutability assumption leads to different results (Flabbi and Gatti, 2018[44]). Jones (2016[46]) suggests computing aggregates by taking into account the different returns of the different categories of human capital (skilled and unskilled workers) as a function of their relative scarcity and the possible complementarities between them. Based on the same data used by Caselli (2004[35]), he concludes that physical and human capital variations can fully explain output differences between countries. Removing not only the perfect substitutability assumption but also using a broader definition of skills, Malmberg (2016[47]) argues that human capital accounts for the bulk of income differences across countries.
Likewise, cross-country growth regressions provide mixed evidence on the relationship between quantity-based measures of human capital and economic outcomes
Macroeconomic cross-country growth regressions provide mixed evidence on the relationship between economic outcomes and quantity-based measures of human capital, including literacy, enrolment rates and mean years of schooling (Benos and Zotou, 2014[48]). Past OECD studies looking at OECD countries confirm the difficulty of finding a robust positive effect of human capital on income per capita or productivity levels. First, including many control variables in the regression analysis tends to reduce the size or significance of the effect identified. Human capital may be correlated with other variables, in particular those representing good governance, and may have indirect effects through such variables. Including these variables in the regression, is thus likely to weaken the estimated effect of human capital (Fournier and Johansson, 2016[49]). Second, using common time fixed effects appears to weaken the estimated effect of human capital as it has a similar time trend across OECD countries (Égert, 2017[50]). Thirdly, the estimated effect is sensitive to the measure of human capital and to the estimation method (Guillemette et al., 2017[51]).
One major criticism of many of these macroeconomic studies is that they use quantity-based measures of human capital that do not capture quality aspects. The same length of schooling does not entail the same amount of knowledge and skills if the quality of education differs across countries and evolves over time. In a first new strand of the literature, MYS was adjusted by the returns to education. However, the available estimates of returns to education may not capture this sufficiently, especially if they are averaged over countries or time, particularly because returns may differ due to other factors such as labour market conditions. Moreover, MYS, even if adjusted for work experience, neither account for training received after leaving the formal education system nor for a possible depreciation of skills.
Another strand of academic literature has attempted to model both quantity and quality dimensions of human capital to explain macroeconomic variables (such as productivity or GDP per capita) following two main approaches:
Including a measure of quality (e.g. student test scores) and quantity (MYS) as separate explanatory variables in panel or cross-country regressions (for example: Altinok (2007[52]); Hanushek and Kimko (2000[53]); Hanushek and Woessmann (2012[54]); Fournier and Johansson (2016[49]); Barro and Lee (2015[55])). However, such an approach usually results in either the quantity or (more often) the quality variable being dominant and the other being statistically insignificant and quantitatively unimportant. Moreover, such effects can become fragile if additional control variables are added (Fournier and Johansson, 2016[49]).
Another approach takes a combination (usually a product or geometric mean) of MYS and a variable representing the quality dimension, usually based on student test scores and often relative to a benchmark country (for example: Altinok (2007[52]), Fournier and Johansson (2016[49]), Rabiul, Ang and Madsen (2014[56])). The resulting composite variable is sometimes being referred to as learning‑adjusted years of schooling (LAYS). A weakness of these approaches is that the relative weighting of quality and quantity components is arbitrarily imposed during the construction of the composite variable (so that typically an equal percentage increase in the quality or quantity dimensions has the same effect on the composite measure).
A new measure of human capital to improve estimates of the macroeconomic outcomes of education
To address the shortcomings of previous empirical studies, a new measure of human capital is developed using PIAAC, PISA and MYS data to account for quality and quantity of education
A new stock measure of human capital has been developed recently by exploiting data from the OECD Programme for International Student Assessment (PISA) and the Programme for the International Assessment of Adult Competencies (PIAAC) (Égert, de la Maisonneuve and Turner, 2022[57]). It attempts to overcome inherent problems with using either in isolation: PIAAC provides a measure of skills for the entire adult working population, but has no time series and limited country coverage; PISA, especially when combined with similar international test scores, has a much longer time series and country coverage, but only applies to those aged 15. Moreover, conceptually, PISA measures the quality of education in primary or secondary schooling resulting in quality effects transmitted to the stock of working-age population with long lags.
The analysis shows that skills at the age of 15 (measured by student test scores) have a strong empirical relationship with skills (measured by PIAAC) observed later in adulthood of the same cohorts (Figure 1.3). Regression analysis estimates that, depending on the specification, the elasticity of cohort‑specific adult skills with respect to student test scores is three to four times higher than the elasticity with respect to mean years of schooling. Exploiting this link, a new stock measure of human capital, covering the working-age population and reflecting both the quality and quantity of education, is calculated as the cohort-weighted average of past student test scores and mean years of schooling of current cohorts.
Figure 1.3. Student and adult test scores are correlated
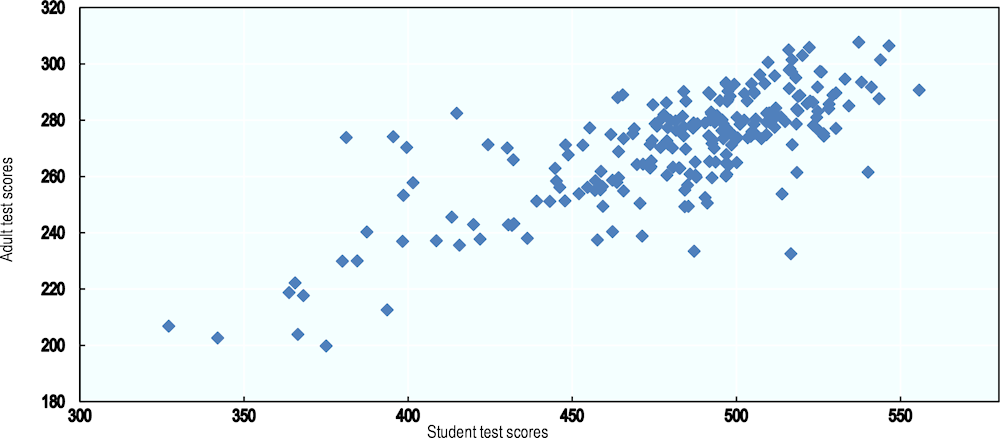
Note: Adult test scores of specific cohorts, obtained from surveys of adult skills, are matched with earlier student test scores of the same cohorts for the 35 countries for which both PIAAC and PISA scores are available. Student test scores denote the average scores for reading, maths and science. Adult test scores stand for the average of scores on literacy, numeracy and problem solving.
Source: Adapted from Égert, de la Maisonneuve and Turner (2022[57]), “A new macroeconomic measure of human capital exploiting PISA and PIAAC”, OECD Economics Department Working Papers, No. 1709, https://doi.org/10.1787/a1046e2e-en, Figure 1.
This new human capital measure shows a strongly significant correlation with multi-factor productivity…
The effect of the new measure of human capital is evaluated within the OECD Economics Department’s Quantitative Assessment of Structural Reforms framework (Égert and Gal, 2017[58]). The framework quantifies the impact of structural reforms on per capita income and includes a multi-factor productivity (MFP) regression. In addition to human capital, the policy determinants of MFP include an indicator of product market regulation; a measure of innovation intensity; trade openness (an intermediate policy outcome, which proxies trade policies) and the output gap as a cyclical control variable.
When added to the cross-country time-series regression, the new measure of human capital has a strongly significant correlation with MFP. To assess the potential productivity gains from improvements in human capital, the effect of closing the gap between the median OECD country and the top three performers, in both quantity and quality components of the new human capital variable are considered separately:
A sustained improvement in PISA student test scores by 5.1%, equivalent to an improvement by 25.5 points from the median OECD country (496.2, the average of the Czech Republic and Norway in 2018) to the average of the leading three countries (Estonia, Japan and Korea in 2018), is estimated to increase MFP by between 3.4% and 4.1 % in the long run (for details see Égert, de la Maisonneuve and Turner (2022[57])).
A sustained increase in mean years of schooling by 9.3% equivalent to an improvement by 1.2 years from the median OECD country (12.7 years, the average of the cohort of 20 to 24 years in Lithuania and Poland in 2020) to the leading three countries (Ireland, Australia and Japan), is estimated to increase MFP by between 1.8% and 2.2 % in the long run (Égert, de la Maisonneuve and Turner, 2022[57]).
… and much larger potential productivity gains from improvements in the quality of human capital than its quantity
These stylised calculations suggest that the potential for productivity gains is much greater from improvements in the quality than quantity component of human capital. Moreover, the magnitude of these potential gains in MFP is comparable to a similarly standardised improvement in product market regulation, which is the other key reform category advocated by the OECD next to investments in human capital (Figure 1.1. ). A similarly scaled improvement in the OECD’s product market regulation indicator (equal to the difference from the median to the top three performing countries) generates a long-run increase in MFP of 2.6% (for details see Égert, de la Maisonneuve and Turner (2022[57])).
However, while an improvement in student skills has a broadly similar long-run impact on multi-factor productivity as improving product market regulation, it may take four decades to fully show
There are, however, unusually long lags between policies that affect the skills of students in compulsory schooling and their long-run macroeconomic effect. The calculations described above all assume that improvements are sustained in successive cohorts of students, but it still takes nearly 50 years before these student cohorts are fully reflected in all cohorts of the working-age population. There is then a further lag before this upskilling is fully reflected in MFP. To underline these longer lags the effect of a policy that brings about a sustained improvement in student skills can be compared with a policy that leads to a stepwise improvement in product market regulation (in both cases the shocks are again calibrated to close the gap between the median and top three performing countries). Thus, while an improvement in student skills has a broadly similar long-run impact on MFP as improving product market regulation, it may take four decades to take effect (Figure 1.4.). These long lag times thus highlight the attractiveness of lifelong learning strategies aimed at improving the human capital of the existing workforce.
Figure 1.4. Comparing policy responses to improve skills and product market competition
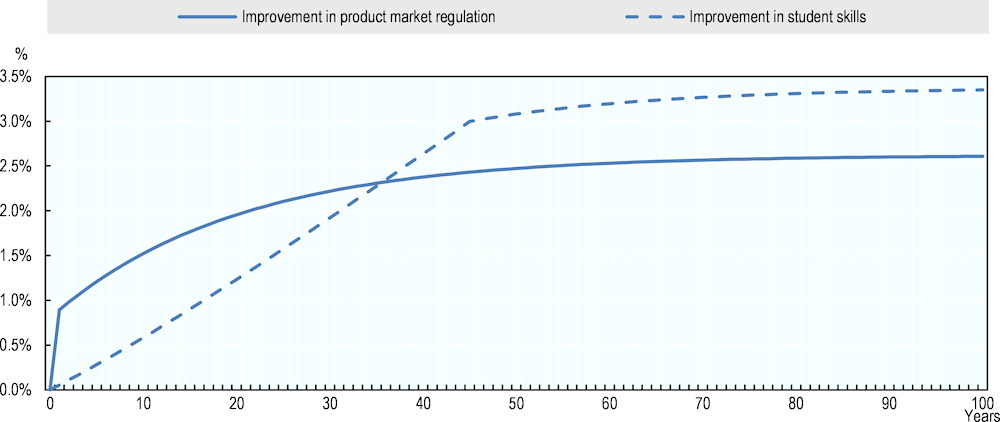
Note: The chart displays the dynamic response of mfp to a standardised shock to student skills and product market regulation. The shocks are standardised by calibrating the magnitude of the shock as the difference between the OECD median country and the top three performing countries in terms of the shocked indicator (see text for further details). The shock for human capital assumes that skills are upgraded gradually as students gradually enter the workforce.
Source: Adapted from Égert, de la Maisonneuve and Turner (2022[57]),“A new macroeconomic measure of human capital exploiting PISA and PIAAC”, OECD Economics Department Working Papers, No. 1709, https://doi.org/10.1787/a1046e2e-en, Figure 6.
Key Messages
Overall, this new measure of human capital based on Survey of Adult Skills (PIAAC) and PISA data to account for the quality of human capital in a more fine-grained manner confirms the importance of human capital improvements for multi-factor productivity, and ultimately economic growth. In doing so, this analysis sends a strong message to finance policy makers for continued public investments in education given the magnitude of this impact which is equivalent to improvements in product market regulation in the long run.
This new analysis also provides important messages for education policy makers, insofar as it shows stronger economic effects on multi-factor productivity deriving from quality improvements in human capital relative to gains in educational attainment and mean years of schooling (representing the quantity of human capital). For education authorities, these findings send a strong signal calling for sustained efforts to boost the learning outcomes of all students and to enhance the effectiveness and efficiency of their education systems, in order to realise these potential productivity gains and economic returns.
Lastly, a third important message for both finance and education policy makers relates to the time lags of human capital improvements, which take around 50 years to fully materialise. On the one hand, this underlines the urgency of tackling quality and equity challenges in education to maximise economic returns. On the other hand, it highlights the critical importance of a long-term commitment and non-partisan process of continued improvement in the education sphere, as its benefits will take far longer than the time horizon of electoral cycles to materialise.
References
[23] Aghion, P. and P. Howitt (1997), Endogenous Growth Theory, The MIT Press, https://EconPapers.repec.org/RePEc:mtp:titles:0262011662.
[52] Altinok, N. (2007), “Human Capital Quality and Economic Growth”, HAL, Working Papers.
[55] Barro, R. and J. Lee (2015), Education Matters, Oxford University Press, https://doi.org/10.1093/acprof:oso/9780199379231.001.0001.
[29] Becker, G. (1967), Human Capital and the Personal Distribution of Income: An Analytical Approach, Ann Arbor, Michigan: University of Michigan Press.
[26] Benhabib, J. and M. Spiegel (2005), “Human Capital and Technology Diffusion”, in Aghion, P. and S. Durlauf (eds.), Handbook of Economic Growth, Elsevier, https://EconPapers.repec.org/RePEc:eee:grochp:1-13.
[48] Benos, N. and S. Zotou (2014), “Education and Economic Growth: A Meta-Regression Analysis”, World Development, Vol. 64, pp. 669-689, https://doi.org/10.1016/j.worlddev.2014.06.034.
[27] Bils, M. and P. Klenow (2000), “Does Schooling Cause Growth?”, American Economic Review, Vol. 90/5, pp. 1160-1183, https://doi.org/10.1257/aer.90.5.1160.
[40] Blöndal, S., S. Field and N. Girouard (2002), “Investment in Human Capital through Upper-Secondary and Tertiary Education”, OECD Economic Studies.
[43] Boarini, R. and H. Strauss (2007), “The Private Internal Rates of Return to Tertiary Education: New Estimates for 21 OECD Countries”, OECD Economics Department Working Papers, No. 591, OECD Publishing, Paris, https://doi.org/10.1787/031008650733.
[8] Braga, M. et al. (2013), “Educational policies in a long-run perspective”, Economic Policy, Vol. 28/73, pp. 45-100, http://www.jstor.org/stable/24029622.
[35] Caselli, F. (2004), Accounting for Cross-Country Income Differences, https://EconPapers.repec.org/RePEc:nbr:nberwo:10828.
[12] Dolton, P. and O. Marcenaro-Gutierrez (2011), “If you pay peanuts do you get monkeys? A cross-country analysis of teacher pay and pupil performance”, Economic Policy, Vol. 26/65, pp. 5-55, https://EconPapers.repec.org/RePEc:oup:ecpoli:v:26:y:2011:i:65:p:5-55.
[28] E.A. Hanushek, S. (ed.) (2011), The economics of international diferences in educational achievement, North Holland.
[50] Égert, B. (2017), “Regulation, institutions and productivity: New macroeconomic evidence from OECD countries”, OECD Economics Department Working Papers, No. 1393, OECD Publishing, Paris, https://doi.org/10.1787/579ceba4-en.
[57] Égert, B., C. de la Maisonneuve and D. Turner (2022), “A new macroeconomic measure of human capital exploiting PISA and PIAAC: Linking education policies to productivity”, OECD Economics Department Working Papers, No. 1709, OECD Publishing, Paris, https://doi.org/10.1787/a1046e2e-en.
[58] Égert, B. and P. Gal (2017), “The quantification of structural reforms in OECD countries: A new framework”, OECD Economics Department Working Papers, No. 1354, OECD Publishing, Paris, https://doi.org/10.1787/2d887027-en.
[36] Feenstra, R., R. Inklaar and M. Timmer (2015), “The Next Generation of the Penn World Table”, American Economic Review, Vol. 105/10, pp. 3150-3182, https://doi.org/10.1257/aer.20130954.
[44] Flabbi, L. and R. Gatti (2018), A Primer on Human Capital, World Bank, Washington, DC, https://doi.org/10.1596/1813-9450-8309.
[49] Fournier, J. and Å. Johansson (2016), “The Effect of the Size and the Mix of Public Spending on Growth and Inequality”, OECD Economics Department Working Papers, No. 1344, OECD Publishing, Paris, https://doi.org/10.1787/f99f6b36-en.
[51] Guillemette, Y. et al. (2017), “A revised approach to productivity convergence in long-term scenarios”, OECD Economics Department Working Papers, No. 1385, OECD Publishing, Paris, https://doi.org/10.1787/0b8947e3-en.
[3] Guillemette, Y. and D. Turner (2021), “The long game: Fiscal outlooks to 2060 underline need for structural reform”, OECD Economic Policy Papers, No. 29, OECD Publishing, Paris, https://doi.org/10.1787/a112307e-en.
[34] Hall, R. and C. Jones (1999), “Why Do Some Countries Produce So Much More Per Worker Than Others?”, The Quaterly Journal of Economic, Vol. 1/114, pp. 83-116.
[53] Hanushek, E. and D. Kimko (2000), “Schooling, Labor-Force Quality, and the Growth of Nations”, American Economic Review, Vol. 90/5, pp. 1184-1208, https://doi.org/10.1257/aer.90.5.1184.
[11] Hanushek, E., M. Piopiunik and S. Wiederhold (2019), “The Value of Smarter Teachers”, Journal of Human Resources, Vol. 54/4, pp. 857-899, https://doi.org/10.3368/jhr.54.4.0317.8619R1.
[19] Hanushek, E. and L. Woessmann (2021), “Education and Economic Growth”, in Oxford Research Encyclopedia of Economics and Finance, Oxford University Press, https://doi.org/10.1093/acrefore/9780190625979.013.651.
[54] Hanushek, E. and L. Woessmann (2012), “Schooling, educational achievement, and the Latin American growth puzzle”, Journal of Development Economics, Vol. 99/2, pp. 497-512, https://doi.org/10.1016/j.jdeveco.2012.06.004.
[9] Havnes, T. and M. Mogstad (2011), “No Child Left Behind: Subsidized Children Care and Children’s Long-Run Outcomes”, American Economic Journal: Economic Policy, Vol. 3, pp. 97-129.
[41] Heckman, J. (1979), “Sample Selection Bias as a Specification Error”, Econometrica, Vol. 47/1, p. 153, https://doi.org/10.2307/1912352.
[42] Heckman, J., L. Lochner and P. Todd (2005), “Earnings Functions, Rates of Return and Treatment Effects: The Mincer Equation and Beyond”, p. 199, https://www.nber.org/system/files/working_papers/w11544/w11544.pdf (accessed on 18 July 2022).
[1] IMF (2022), Fiscal Monitor Database of Country Fiscal Measures in Response to the COVID-19 Pandemic, https://www.imf.org/en/Topics/imf-and-covid19/Fiscal-Policies-Database-in-Response-to-COVID-19 (accessed on 1 October 2021).
[46] Jones, C. (2016), “The Facts of Economic Growth”, in Handbook of Macroeconomics, https://doi.org/10.1016/bs.hesmac.2016.03.002.
[45] Klenow, P. and A. Rodriguez-Clare (1997), “Economic growth: A review essay”, Journal of Monetary Economics, Vol. 40/3, pp. 597-617, https://EconPapers.repec.org/RePEc:eee:moneco:v:40:y:1997:i:3:p:597-617.
[32] Layard, 0. (ed.) (1986), Demand for Education, Elsevier Pubs, Amsterdam, Netherlands.
[21] Lucas, R. (1988), “On the mechanics of economic development”, Journal of Monetary Economics, Vol. 22/1, pp. 3-42, https://doi.org/10.1016/0304-3932(88)90168-7.
[47] Malmberg, H. (2016), “Human Capital and Development Accounting Revisited”, Job Market Paper, p. 76, https://www.economics.utoronto.ca/index.php/index/research/downloadSeminarPaper/76746 (accessed on 18 July 2022).
[18] Mankiw, N., D. Romer and D. Weil (1992), “A Contribution to the Empirics of Economic Growth”, The Quarterly Journal of Economics, Vol. 107/2, pp. 407-437, https://doi.org/10.2307/2118477.
[31] Mincer, J. (1974), Schooling, Experience, and Earnings, National Bureau of Economic Research, Inc, https://EconPapers.repec.org/RePEc:nbr:nberbk:minc74-1.
[33] Mincer, J. (1958), “Investment in Human Capital and Personal Income Distribution”, Journal of Political Economy, Vol. 66/4, pp. 281-302, http://www.jstor.org/stable/1827422.
[39] Montenegro, C. and H. Patrinos (2014), Comparable Estimates of Returns to Schooling around the World, The World Bank, https://doi.org/10.1596/1813-9450-7020.
[37] Morrisson, C. and F. Murtin (2013), “The Kuznets curve of human capital inequality: 1870–2010”, The Journal of Economic Inequality, Vol. 11/3, pp. 283-301, https://doi.org/10.1007/s10888-012-9227-2.
[5] NATO (2022), “Defence Expenditure of NATO Countries (2014-2022)”, https://www.nato.int/nato_static_fl2014/assets/pdf/2022/6/pdf/220627-def-exp-2022-en.pdf (accessed on 22 October 2022).
[24] Nelson, R. and E. Phelps (1966), “Investment in Humans, Technological Diffusion, and Economic Growth”, The American Economic Review, Vol. 56/1/2, pp. 69-75, http://www.jstor.org/stable/1821269.
[4] OECD (2022), OECD Economic Outlook, Interim Report September 2022: Paying the Price of War, OECD Publishing, Paris, https://doi.org/10.1787/ae8c39ec-en.
[2] OECD (2022), OECD Economic Outlook, Volume 2022 Issue 1, No. 111, OECD Publishing, Paris, https://doi.org/10.1787/62d0ca31-en.
[6] OECD (2021), Economic Policy Reforms 2021: Going for Growth: Shaping a Vibrant Recovery, OECD Publishing, Paris, https://doi.org/10.1787/3c796721-en.
[14] OECD (2021), OECD Skills Outlook 2021: Learning for Life, OECD Publishing, Paris, https://doi.org/10.1787/0ae365b4-en.
[16] OECD (2019), Economic Policy Reforms 2019: Going for Growth, OECD Publishing, Paris, https://doi.org/10.1787/aec5b059-en.
[15] OECD (2019), OECD Skills Outlook 2019: Thriving in a Digital World, OECD Publishing, Paris, https://doi.org/10.1787/df80bc12-en.
[7] OECD (2018), Engaging Young Children: Lessons from Research about Quality in Early Childhood Education and Care, Starting Strong, OECD Publishing, Paris, https://doi.org/10.1787/9789264085145-en.
[10] OECD (2005), Teachers Matter: Attracting, Developing and Retaining Effective Teachers, Education and Training Policy, OECD Publishing, Paris, https://doi.org/10.1787/9789264018044-en.
[13] Oliveira Martins, J. et al. (2007), “The Policy Determinants of Investment in Tertiary Education”, OECD Economics Department Working Papers, No. 576, OECD Publishing, Paris, https://doi.org/10.1787/085530578031.
[38] Psacharopoulos, G. and H. Patrinos (2004), “Returns to investment in education: a further update”, Education Economics, Vol. 12/2, pp. 111-134, https://doi.org/10.1080/0964529042000239140.
[56] Rabiul, I., J. Ang and J. Madsen (2014), “Quality-adjusted human capital and productivity growth”, Economic Inquiry, Vol. 52/2, pp. 757-777, https://doi.org/10.1111/ecin.12052.
[22] Romer, P. (1990), “Endogenous Technological Change”, Journal of Political Economy, Vol. 98/5, pp. S71-S102, http://www.jstor.org/stable/2937632.
[30] Schultz, T. (1961), “Investment in Human Capital”, The American Economic Review, Vol. 51/1, pp. 1-17, http://www.jstor.org/stable/1818907.
[20] Schumpeter, J. (2006), Theorie der wirtschaftlichen entwicklung [The theory of economic development], Duncker & Humblot (original work published 1912).
[17] Solow, R. (1956), “A Contribution to the Theory of Economic Growth”, The Quarterly Journal of Economics, Vol. 70/1, p. 65, https://doi.org/10.2307/1884513.
[25] Welch, F. (1970), “Education in Production”, Journal of Political Economy, Vol. 78/1, pp. 35-59, https://doi.org/10.1086/259599.
Notes
← 1. For a recent survey of the economic growth literature, including the role played by human capital, see Jones (2016[46]).
← 2. Total Factor Productivity.