This chapter discusses the key features of two active labour market measures: vocational training and employment subsidies offered to unemployed people by the public employment service of Lithuania. The chapter includes a description of the characteristics of the individuals and employers who participate in the programmes. It also describes the rich, individual level administrative data that provide the foundation for the empirical analysis and the econometric approach used in the counterfactual impact evaluation of these two measures in the following chapters of this review. In addition to outcomes commonly examined in impact evaluations of active labour market policies, such as employment probabilities, this chapter describes additional outcomes examined, most notably career progression. For the latter, the chapter outlines the construction of an occupational index calculated based on the observed wages of individuals by detailed occupational codes.
Impact Evaluation of Vocational Training and Employment Subsidies for the Unemployed in Lithuania

3. Counterfactual impact evaluation approach and outcomes examined
Abstract
3.1. Introduction
As discussed in the previous chapter, Lithuania devotes a relatively limited amount of resources to active labour market policies (ALMPs): spending on ALMPs amounts to less than half of the OECD average and the share of its labour force participating in ALMPs is only one fifth of the OECD average. Only a minority of jobseekers enter ALMPs in Lithuania: in 2019, for example, less than 18% of jobseekers participated in any ALMP.1 At the same time, Lithuania has relatively large disparities in employment rates across educational and geographic divides. In addition, the Lithuanian Employment Service (LES) also has established contacts with a relatively large share of the unemployed population, given that a relatively large share of jobseekers registers with them. These contrasting facts point to the need to carefully consider questions relating to the content and targeting of Lithuania’s ALMPs.
To what extent are Lithuania’s ALMPs successful in bringing jobseekers back into employment – and which programmes work best for whom? Which aspects of ALMPs work well and which ones could be improved? When faced with such questions, policy makers often turn to key performance indicators – job placement rates, participant satisfaction – or rely on feedback from staff or jobseekers. Both of these sources of information can play an important role in assessing the merits of a policy. For example, key performance indicators can be useful in understanding which ALMPs have the highest post-participation employment probabilities, for examining to what extent these have improved over time or for monitoring the performance of specific training providers in real-time. Similarly, subjective feedback can help provide a nuanced view of the benefits and drawbacks of a certain programme as well as concrete suggestions for improvements. At the same time, however, such approaches cannot provide a rigorous answer to the crucial question of what is the precise impact of a programme or policy – this requires accounting for what would have happened to individuals in the absence of the programme or policy. This is the motivation for conducting counterfactual impact evaluations (CIEs) such as the one outlined in this chapter.
The impact evaluation illustrated in this chapter focuses on two of Lithuania’s main ALMPs: vocational training and employment subsidies for the unemployed. The two programmes provide, respectively, training lasting several months intended to fill gaps in jobseekers’ skills, and subsidies of generally up to six months to offset part of the employers’ wage costs associated with hiring workers from disadvantaged groups. In addition to analysing outcomes typically examined in CIEs of ALMPs, such as employment probability or earnings, the analysis examines another important question: the effect of participation in ALMPs on occupational mobility. These outcomes are tracked continuously over up to the three‑year period starting with the beginning of the programme. The empirical analysis relies on rich and comprehensive data that allow for the wide set of outcomes to be analysed, as well as for accounting for a number of different jobseeker attributes. Several types of data are used in this evaluation: unemployment registry data, ALMP participation data, data on employment and earnings, as well as data on employer characteristics.
The chapter begins with a description of the two programmes analysed and the characteristics of the individuals and employers who participate in the programmes. It then also describes the rich, individual level administrative data that provide the foundation for the empirical analysis, as well as the econometric approach used in the counterfactual impact evaluation of these two measures in the following chapters of this review. The final sections describe the labour market outcomes examined in the impact evaluation. In addition to outcomes commonly examined in impact evaluations of active labour market policies, such as employment probabilities, this chapter describes additional outcomes examined, most notably career progression. For the latter, the chapter details the construction of an occupational index calculated based on the observed wages of individuals by detailed occupational codes.
3.2. Vocational training and employment subsidy programmes are two of the main ALMPs in Lithuania
The impact evaluation focuses on two of Lithuania’s main active labour market programmes (ALMPs): vocational training for the unemployed and employment subsidies. These measures together account for roughly one‑third of Lithuania’s ALMP participant numbers and half of expenditures on ALMPs during the 2014‑20 period, excluding the provision of public employment service (PES) counselling and job brokerage services as well as wage subsidy measures introduced during the COVID‑19 crisis.
The key parameters of the two ALMPs are as follows:
Vocational training. This is voucher-based training where jobseekers can select from accredited training providers. Anticipated duration is generally three months for formal training (but can be up to eight months) and one month for non-formal training (but can be up to three months). In practice, individuals generally enter training after being unemployed for three to five months for formal training and after two to three months for non-formal training, although individuals can enter training immediately after becoming unemployed. Average total subsidy (subsidija in Lithuanian) amounts during 2014‑20 were EUR 1 865 for formal training (which generally leads to an accreditation or certificate), and EUR 1 148 for non-formal training. With the rare exception of very expensive training programmes, all training expenses are covered by the LES. Individuals can enter tripartite agreements with employers in advance of their training, whereby employers promise employment to individuals successfully completing their vocational training.
Employment subsidies. The programme subsidises 50% of participant’s wage costs (up to 75% for individuals with disabilities), with a ceiling amounting to twice the statutory minimum wage during the 2017‑19 period and one and a half statutory minimum wages thereafter. The maximum programme duration is generally six months.2 Individuals may enter the programme at any point in their unemployment spell, and the exact time of entry varies considerably in practice (the median unemployment duration at entry is five months). The average subsidy amount varied from EUR 1 004 in 2013 to roughly EUR 1 550 from 2016 onwards.
Additional statistics on the two programmes are provided in Table 3.1. Vocational training programmes tend to be shorter than employment subsidies, but they tend to be more expensive. Training entered with tripartite agreement with employers – a unique feature of the Lithuanian ALMPs described in greater detail below – tend to be the shortest and also least expensive.
Table 3.1. Length of participation and costs vary across programmes in Lithuania
Duration of programme participation in months and average cost in EUR
Programme |
Distribution of duration |
Average costs |
|||||
---|---|---|---|---|---|---|---|
5th |
25th |
Median |
75th |
95th |
Mean |
||
Vocational training |
0.3 |
0.9 |
1.8 |
4.6 |
7.5 |
2.8 |
1 715 |
- of which, without tripartite agreement |
0.5 |
1.1 |
2.8 |
5.1 |
7.6 |
3.2 |
1 901 |
- of which, with tripartite agreement |
0.1 |
0.8 |
1.0 |
1.7 |
5.7 |
1.7 |
1 203 |
Employment subsidies |
0.9 |
3.0 |
5.0 |
6.0 |
9.2 |
7.7 |
1 460 |
Note: Statistics are calculated for all entrants during the 2014‑20 period. Programme costs are expressed in nominal amounts and include direct costs paid to the vocational training provider (in the case of vocational training) or the employer (in the case of employment subsidies).
Source: OECD calculations based on data from the Lithuanian Employment Service.
Vocational training programmes cover a multitude of different topics and vary considerably in terms of their length. During the 2014‑20 period, jobseekers entered into 2001 different training programmes, with many of these having only a small number of participants. The most commonly entered training programme was a one‑month training course for obtaining a commercial motor vehicle license. This programme accounted for slightly over 10% of all vocational training participants during this period. Examples of longer vocational training programmes include an eight‑month training programme for chefs, a seven‑month training programme for hairdressers and a seven‑month training programme for plumbers. Note that these training programmes do not involve on-the‑job training at specific employers – such training is offered via an apprenticeship programme, which was introduced in 2017. Vocational training includes both formal and non-formal training programmes. Formal training programmes are more likely to be – but not exclusively – aimed towards obtaining accreditation or a license during the course of the training.
Employers receiving employment subsidies are required to retain workers hired through the subsidy programme for at least six months after the end of the subsidy.3 If they terminate the contract of a worker before this period, they are not eligible for receiving new employment subsidies for the following 12 months. Given that employment subsidies are six months in duration, this requirement effectively means that employers must retain workers for one year after they first hire them. While this may make them less attractive to employers, such stipulations may help narrow the scope for strategic behaviour from firms to exploit the subsidies (for a detailed analysis of this question, see Chapter 5). On the other hand, one feature that has made the employment subsidies more attractive to employers in recent years is the automated exchange of information on gross wages from administrative sources, which has lowered the reporting requirements of participating firms. Because employment subsidies are paid as a proportion of gross wages, this information is necessary for calculating the payments made to firms.
A notable feature of vocational training in Lithuania – and one that is analysed in this evaluation – is the possibility of having employers promise employment to individuals successfully completing their vocational training. Such tripartite agreements – which are entered into force between the jobseeker, the LES and the prospective employer – stipulate that after completing the vocational training (which is nevertheless financed by the LES), the future employer will employ a person with the acquired qualification or competence for at least six months after the training, and the vocational training participant will stay with the intended employer for at least six months. From the perspective of the jobseeker, such agreements provide an assurance that the time and effort devoted to the training will result in guaranteed employment, thus presumably offering an additional motivation to complete the training course. For individuals deciding on which training to undertake, it offers reassurance that they will not be subject to the potentially changing short-term needs of employers. From the perspective of the employer, the agreements provide a way to acquire workers with skills that it anticipates will be in demand, and are potentially helpful particularly in cases where labour with relevant skills is not locally available. But such planning from an employer also requires anticipating the level of product demand in the medium term, which can be difficult in periods of high uncertainty, such as that induced by the COVID‑19 crisis.
Both participation and expenditures on the two ALMPs examined varied considerably over the period examined in the impact evaluation (Table 3.2). In terms of total number of participants, roughly one-fifth more individuals participated in vocational training than in employment subsidies; expenditures on vocational training were roughly two‑fifths higher. From an evaluation perspective, the total number of participants in both programmes is large enough to permit a detailed evaluation. Individuals with tripartite agreements accounted for 26.5% of all vocational training participants during the period, although this share varied considerably over time. Individuals entered into vocational training or employment subsidies in 7.5% of unemployment spells during this period.
Table 3.2. Participation in vocational training and employment subsidy programmes in Lithuania varied considerably during the 2014‑20 period
Programme |
2014 |
2015 |
2016 |
2017 |
2018 |
2019 |
2020 |
Total |
---|---|---|---|---|---|---|---|---|
Number of participants (thousands) |
||||||||
Vocational training |
5.8 |
19.1 |
17.2 |
21.4 |
16.6 |
8.2 |
5.4 |
93.8 |
-of which, individuals with tripartite agreements |
3.7 |
9.0 |
3.2 |
2.9 |
2.3 |
2.4 |
1.4 |
24.9 |
Employment Subsidies |
20.3 |
14.1 |
7.1 |
9.1 |
10.7 |
7.2 |
11.2 |
79.7 |
Expenditures (millions of EUR) |
||||||||
Vocational training |
9.4 |
26.8 |
30.2 |
36.4 |
29.5 |
17.5 |
10.6 |
160.4 |
-of which, individuals with tripartite agreements |
4.7 |
8.5 |
4.0 |
3.5 |
3.0 |
4.2 |
2.0 |
29.9 |
Employment Subsidies |
25.4 |
17.6 |
10.5 |
13.9 |
16.9 |
11.1 |
19.9 |
115.4 |
Note: Participants and expenditures are calculated based on year of entry into programme. Expenditures are in nominal amounts.
Source: OECD calculations based on data from the Lithuanian Employment Service.
3.3. Counsellors’ discretion plays a role in targeting ALMPs
Eligibility and need for different ALMPs, including vocational training and employment subsidies is established when the jobseeker and their LES counsellor first discuss and assess the jobseeker’s employment opportunities and needs for support. Since the end of 2021, the counsellors are supported by a digital jobseeker profiling tool that uses statistical methods, machine learning and administrative data to predict jobseekers’ probability of long-term unemployment and needs for support. Previously (during the period evaluated in this report), the LES profiling tool was based on 22 questions about employment opportunities, health issues, willingness to work and other topics that the counsellor asked from the jobseeker (generally indirectly to fully understand the circumstances). This profiling tool segmented jobseekers to three categories according to their distance to employment and five groups of support needs. The final decision on support needs is taken by the counsellor and the jobseeker in a mutual agreement, not necessarily fully adhering to the suggestions by the (current or previous) profiling tool, enabling them to take into account further individual circumstances of the jobseeker. Subsequently, an individual action plan is agreed between the counsellor and the jobseeker to set the pathway for employment establishing the responsibilities for both the LES and the jobseeker. Among other measures, the individual action plan can establish the necessity of acquiring a qualification or competency (and thus participation in vocational training) or eligibility for employment subsidies. The individual action plan is revisited during each counselling session and fully re‑assessed after six months and subsequently every three months. As such, a need for an ALMP can be established later on and not necessarily during the first assessment of employment opportunities and needs for support.
In the case of employment subsidies, an individual then notes their eligibility for employment subsidies when applying to job vacancies. Furthermore, employers can note that they would like to hire an individual via the employment subsidies and request assistance from the LES; the decision of whom they hire is ultimately the decision of the employer, who can also specify that they would like to hire a certain individual.
In the case of vocational training, once the type of vocational training has been agreed upon by the jobseeker and LES counsellor, the vocational training provider is chosen by the jobseeker, possibly in conjunction with a future employer. Prior to the introduction of the voucher system in 2012, there were longer public tenders for the purchase of training services, with the procurement procedure for acquiring training providers generally taking three months (and longer in specific cases). The new system arguably enables training to better adapt to the changing skills demanded by employers compared to the previous system.
The Lithuanian Law on Employment specifies the following target groups of unemployed individuals that are to be the primary beneficiaries of ALMPs (Republic of Lithuania, 2016[1]):
individuals without professional qualifications or education (or lacking ones recognised in Lithuania),
long-term unemployed (defined as at least 12 months for those 25 years of age or older and six months otherwise),
unemployed over 50 years of age,
unemployed of 29 years or younger or without previous employment experience, and
individuals with a disability.
Furthermore, guidelines specify which programmes should be preferentially applied to each of the specific groups (Ministry of Social Security and Labour, Lithuania, 2017[2]). These specify, for example, that workers with disabilities are to receive a wide array of support via training and subsidies relating to employment, including ones dedicated to supported employment and rehabilitation (which are not the subject of the impact evaluation in this report). For this reason, individuals with disabilities who have an assessed working capacity 25% or less are not included in the subsequent analysis. Among the variables coded in the data by LES counsellors, the highest shares of participants fulfil the age criteria for target groups (see Table 3.3 below).
Table 3.3. Age criteria in Lithuania are most prominent for participation in vocational training and employment subsidy programmes
Share of individuals participating in either the vocational training or employment subsidy programmes belonging to specific target groups
Target group |
Share of vocational training participants fulfilling criterion |
Share of employment subsidy participants fulfilling criterion |
---|---|---|
Persons up to 29 years of age |
39.9 |
37.6 |
Persons over 50 years of age |
23.3 |
39.5 |
Raising a child under the age of eight |
11.8 |
10.0 |
Unskilled unemployed (individuals without professional qualifications or education recognised in Lithuania) |
5.4 |
5.8 |
Working-age persons with a disability and a level of working capacity of 45‑55% |
2.8 |
4.3 |
Unemployed persons starting work for the first time after having acquired their current qualifications |
2.8 |
1.9 |
Working-age persons with a disability and a level of working capacity of 30‑40% |
0.7 |
2.3 |
Other categories |
0.0 |
1.5 |
Note: Categories are presented as coded in the LES database and statistics are calculated based on entrants during the 2014‑20 period. Categories are not mutually exclusive.
Source: OECD calculations based on data from the Lithuanian Employment Service.
Taking into account the above guidelines, it is important to note that the law gives LES counsellors the right to exercise discretion in deciding whether to refer an individual to a specific measure. LES counsellors appear to exercise this discretion in practice. To the extent that these target groups can be accurately gleaned from the administrative data, a sizable share of individuals do not meet any of the above criteria in practice. In fact, for any given calendar year during the 2014‑20 period, 5 to 19% of individuals entering either vocational training or subsidised employment did not meet any of these criteria. This fact informs the choice of the econometric procedure used, with comparisons made based on detailed information on individual’s observed characteristics (for details on the methodology, see Section 3.6.
3.4. People closer to the labour market are more likely to get support, particularly vocational training
This section examines the characteristics of individuals and firms participating in vocational training and employment subsidies. The analysis across individuals focusses on differences across gender, age, duration of unemployment, education level and location. In order to provide a sense of the extent to which specific categories of individuals are likely to enter ALMPs, the characteristics of the ALMP participants are contrasted with the characteristics of all individuals who are registered as unemployed with LES, taking the averages of monthly unemployment stocks during the 2014‑20 period. These comparisons are presented in Figure 3.1.
Men are disproportionally likely to enter the vocational training or employment subsidy programme, particularly vocational training without tripartite agreements. Men accounted for 78% of vocational training participants, even though men and women were roughly evenly represented amongst the registered unemployed during the 2014‑20 period (their shares were 50.7% and 49.3%, respectively). Among training participants with tripartite agreements, their share was exceeding female participants less, at 55%. This may indicate that women are more likely to engage in vocational training if they are given a guarantee of employment upon the successful completion of their training. The gender disparities could also be influenced by the availability of courses as the most popular courses, like obtaining a licence to drive a commercial vehicle, have mostly male participants.
Jobseekers under the age of 30, particularly men, are disproportionally likely to enter vocational training or the employment subsidy programme. Women aged 30 or over, on the other hand, are disproportionally less likely to enter vocational training or the employment subsidy programme, with women over 50 over four times less likely to enter vocational training than their share of the unemployed would suggest (if individuals were to enter in proportion to their representation among the unemployed). The share of women over 50 entering training with tripartite agreements is considerably higher than the share entering without tripartite agreements. For men over 50, employment subsidies are disproportionally prevalent relative to their share of the unemployed. The participant statistics by age and gender also indicates that some creaming might be taking place regarding vocational training (i.e. those groups that already have a better access to the labour market receive additional support) as young and prime‑age men are particularly likely to receive this measure.
The pattern of participation in ALMPs by duration of unemployment is likely affected by the assessment process of jobseeker needs and the development of the individual action plans, which are conducted in the very beginning of the job search period. This approach leads to a “train-first” strategy for some group of jobseekers in Lithuania, but not necessarily those that benefit from the measure the most if the assessment is not sufficiently accurate and not frequently enough revisited. Earlier on in their unemployment spell, individuals who enter an ALMP are disproportionally likely to enter vocational training. For those unemployed seven months or more, individuals who enter one of the two ALMPs examined are more likely to enter into subsidised employment. This appears consistent with the guidelines on the application of ALMP measures (Ministry of Social Security and Labour, Lithuania, 2017[2]), which advocate for training as a priority intervention for most categories of jobseekers, although access to training is still very limited due to budget constraints.
Low-skilled jobseekers are disproportionally less likely to enter either of the two ALMPs studied.4 This likely reflects the fact that people without any qualification were not eligible for training programmes for some periods within the timeframe analysed – rather, such individuals were to be referred to formal education programmes before potentially being eligible for training programmes targeted towards the unemployed. This approach again leads to creaming as upskilling is more likely provided to those with already higher qualification.
Interestingly, in terms of the urban location of jobseekers entering the ALMPs examined, individuals from non-urban areas are slightly more likely to participate.5 This is the case also for vocational training, where consultations with stakeholders have indicated that finding a suitable training provider can be more of a challenge in practice than in the larger urban regions. While individuals outside large urban areas may thus have fewer options for vocational training, this apparently does not directly translate into lower rates of vocational training.
Figure 3.1. Groups such as younger jobseekers and men are disproportionally included in vocational training or employment subsidies
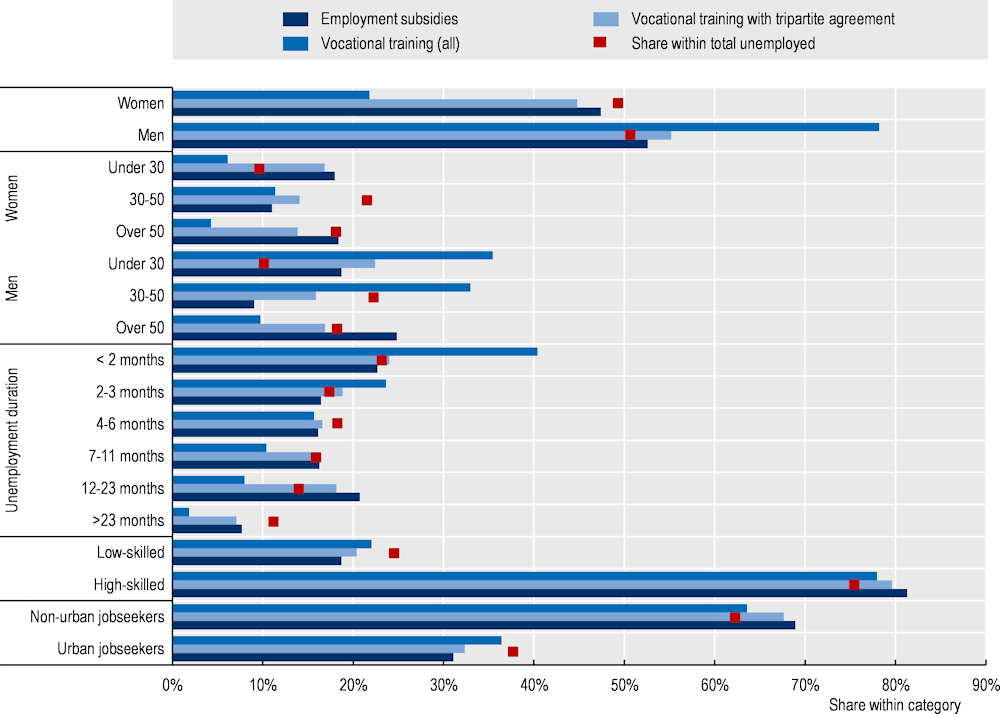
Note: Statistics for stocks of all unemployed are calculated based on averages of monthly statistics during the 2014‑20 period. Participant numbers refer to totals during the 2014‑20 period for individuals entering either vocational training (without or without tripartite agreements) or employment subsidies. Statistics for vocational training include individuals who enter training both with and without tripartite agreements.
Source: OECD calculations based on data from the Lithuanian Employment Service.
While the discussion so far has focused on the characteristics of jobseekers engaging in ALMPs, another interesting aspect concerns the characteristics of the firms who entered tripartite contracts to hire participants of vocational training or hired jobseekers via employment subsidies. Figure 3.2 shows the distribution of firms within size categories for these two ALMPs. Expressed as a share of their total employment, small firms make disproportionately large use of employment subsidies and, to a much smaller extent, vocational training with a tripartite agreement. During the 2018‑20 period, their annual intake of employment subsidy programme participants amounted to 1.5% of their average employment (note that given that such employment subsidies generally last for six months, the corresponding share of employees for whom employment subsidies are being paid at any given point would amount to half of this amount). Smaller firms also accounted for the largest share of total participants: in terms of the total share among employment, firms with less than 50 employees employed 75% of employment subsidy participants and 58% of vocational training participants who entered into a tripartite agreement. However, this finding does not mean that larger firms are not making use of these ALMPs to hire workers. In fact, expressed as a share of firms who hired someone via the two ALMPs, the share is greater among larger firms. These two findings can be reconciled by the fact that there are a considerably larger number of small firms.
Figure 3.2. Incidence of vocational training with tripartite agreement and employment subsidies varies considerably across firm size categories in Lithuania
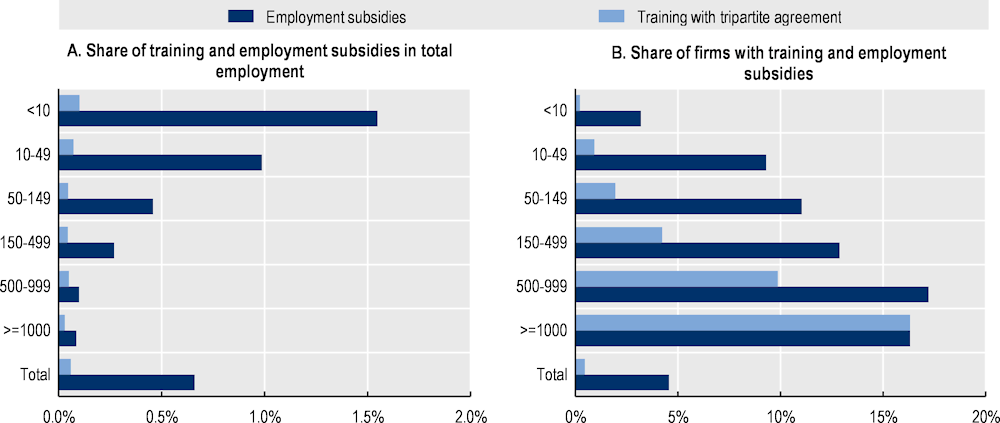
Note: Statistics refer to the 2018‑20 period and do not include sole‑proprietorships (which can also employ workers). Statistics on employment shares refer to annual averages during the 2018‑20 period; statistics on share of firms relate to the share of firms having made use of the ALMP at any time during 2018‑20.
Source: OECD calculations based on data from the Lithuanian Employment Service, Lithuanian State Social Insurance Fund Board and State Enterprise Centre of Registers data.
Another interesting question concerns the age profile of firms engaging with ALMP participants. Start-up firms and young firms play an important role in job creation, even if they account for a minority of total employment: across the OECD, firms five years old or younger account for only 21% of total employment, but are responsible for 47% of job creation (OECD, 2016[3]). They are also generally less likely to be making a profit (Calvino, Criscuolo and Menon, 2016[4]) and thus have a stronger incentive to seek out employment subsidies. As illustrated in Figure 3.3, Panel A, the youngest firms indeed have the highest share of individuals who are employed via employment subsidies. However, the relationship appears V-shaped, with firms ten years or older disproportionally availing themselves of such employment subsidies. For training, the relationship is more straightforward: older firms tend to enter into tripartite agreements with a fewer share of the workers they employ. These findings are similar when examining the distribution across firms instead of the individuals they employ (Figure 3.3, Panel B).
Figure 3.3. Incidence of vocational training with tripartite agreement and employment subsidies varies slightly across firm age categories in Lithuania

Note: Statistics refer to the 2018‑20 period and do not include sole‑proprietorships. Statistics on employment shares refer to annual averages during the 2018‑20 period; statistics on share of firms relate to the share of firms having made use of the ALMP at any time during 2018‑20. Shares reported in both Panels are shares within the size category.
Source: OECD calculations based on data from the Lithuanian Employment Service), Lithuanian State Social Insurance Fund Board and State Enterprise Centre of Registers data.
In terms of the sectors of economic activity of firms making use of the ALMPs examined, several sectors stand out in terms of their relative use. A disproportionally large share of employment in agricultural and manufacturing firms involves hiring unemployed individuals via employment subsidies – on average hiring workers accounting for 2.6% and 1.1% of their employment during the 2018‑20 period. For vocational training with tripartite agreements, two sectors that stand out are agriculture as well as administrative and support service activities, both using such an arrangement with 0.2% of their employment during the 2018‑20 period. In terms of absolute numbers of individuals hired with employment subsidies, the most prominent sectors are manufacturing as well as the wholesale and retail trade sectors. For vocational training with tripartite agreements, the transport sector stands out in terms of absolute number of participants.
Sectors making extensive use of vocational training through tripartite agreements may be disproportionally facing labour shortages, likely due to a combination of shortages of workers with adequate skills and possibly more challenging working conditions. As discussed in Chapter 4, while the presence of tripartite agreements in vocational training has a positive effect on employment outcomes, it also has a negative effect on occupational mobility. The reasons for making disproportionately extensive use of employment subsidies are likely varied and complex, but as discussed in Chapter 5, there is no evidence of them being used to displace existing workers.
3.5. The rich administrative data provide a detailed information on unemployed individuals and their labour market outcomes
Evaluating the effectiveness of ALMPs requires rich data with detailed information on jobseekers characteristics, their participation in ALMPs and their employment outcomes. The data used to conduct the evaluation in this report come from several sources, as outlined in Table 3.4, and span the period from January 2014 to December 2020. Unique individual identifiers allow the data to be combined, providing a rich understanding of individuals’ participation in ALMPs, their background characteristics – both from the LES registry – and their labour market outcomes and wages from a number of different sources. This is complemented with additional employment data, covering also those individuals who were not in unemployment, as well as with firm-level data containing information on the attributes of firms where individuals became employed.
Table 3.4. Several data sources are used in the evaluation
Data source |
Information available |
Periodicity |
Sample |
Coverage |
---|---|---|---|---|
Lithuanian Employment Service |
Detailed background characteristics of registered unemployed, participation in ALMPs and unemployment benefits |
Start and end dates of unemployment spells, participation in ALMPs and unemployment benefit receipt |
Registered unemployed |
2014‑20 |
Board of the State Social Insurance Fund under the Ministry of Social Security and Labour (SODRA) |
Employment outcomes and earnings |
Start and end dates of employment spells |
Individuals who were unemployed at some point during 2014‑20 |
2014‑20 |
Board of the State Social Insurance Fund under the Ministry of Social Security and Labour (SODRA) |
Employment outcomes and earnings |
Monthly |
Individuals who were never unemployed at some point during 2014‑20 |
2018‑20 |
State Enterprise Centre of Registers (Register of Legal Entities) |
Business registry data |
Changes as reported by legal entities |
Registered legal entities |
2018‑20 |
Note: Although the business registry data contains information also on sole proprietorships, the absence of unique firm identifiers in some of the other data means that they cannot be consistently included in the statistics.
The resulting database contains detailed information on the 947 185 unique individuals who were registered as unemployed at any point during the 2014‑20 period. These individuals experienced 2.1 million distinct unemployment spells in total. It also contains detailed information on the 79 700 entries for employment subsidy programme participation and 93 800 entries into vocational training. Individuals entered into vocational training and/or employment subsidies in 7.5% of unemployment spells during this period. The data generally span the period from January 2014 to December 2020. Unique individual identifiers allow the data from the different sources to be combined.6
One potential problem often encountered in impact evaluations of ALMPs concerns the question of how to deal with multiple, sequential entries into ALMPs. In the presence of multiple interventions and possible overlap between different ALMPs, identifying the precise effects of one specific ALMP presents an important challenge. In the case of Lithuania, this is not a major concern: during the vast majority of unemployment spells (92.5%), individuals entered into only one ALMP during their entire unemployment spell. In the remaining 7.5% of cases, individuals entered either two or three ALMPs in total. Of the latter, a sizable proportion involved short ALMP durations (e.g. less than one month). For the purposes of the evaluation, we focus on the first ALMP entered during an unemployment spell.7The exception to this rule concerns the cases where individuals enter an ALMP for less than a month and enter another ALMP within the same month. In this case, we examine the effect of the ALMP which is of longer duration.
Despite the richness of the data on which this evaluation draws, two limitations relating to the data are notable. First, the employment data do not contain information on hours worked. This is worth bearing in mind when interpreting the results, particularly in terms of the outcomes relating to days worked and daily wages. For example, if participating in an ALMP increases the probability that an individual will become employed on a part-time instead of a full-time basis, this would produce a bias on the estimated results: actual hours worked would be lower than suggested by the observed days worked, whereas hourly wages would be higher than suggested by the observed daily wages. In practice, this may not be a problem given the low prevalence of part-time work in Lithuania: in 2020, Lithuania’s part-time employment rate of 5.5% was one of the lowest in the OECD, where it averaged 16.7% (OECD, 2022[5]). Second, data on training do not include information on the target occupations of the training. For this reason, examining whether individuals enter the occupations for which they underwent training is outside the scope of the analysis.
Additional questions related to data will be discussed in a technical report to be produced in addition to this review (OECD, forthcoming[6]). This report will discuss the data in more detail, identifying how the analysis could be enriched with additional databases and discussing ways to make better use of data in the future.
3.6. The impact evaluation methodology accounts for counterfactual outcomes
Assessing the impact of an ALMP requires comparing ALMP participants’ labour market outcomes, such as employment or earnings, with the outcomes that would have occurred had they not participated in the ALMP. Because the latter, “counterfactual” outcomes cannot be observed, it is necessary to find some way of constructing them from the data. A simple way to do this would be to compare the outcomes of those individuals that participated in training (or other ALMP) and those that did not. However, as will be discussed in detail below, in the absence of random assignment in the programme, such groups are likely not comparable, and making simple such comparisons may result in selection bias which would not yield accurate estimates of the true effect of the programme.
In the case of ALMPs in Lithuania, several sources of selection bias may be present. For example, it may be the case that certain types of individuals (e.g. more motivated individuals) are more likely to participate in training and have better employment outcomes for reasons besides their participation in the training. Conversely, certain individuals who face additional barriers to employment – and therefore have worse employment outcomes – may be more likely to be directed towards ALMPs by caseworkers. Many of those who do not participate in an ALMP may not be included simply by virtue of the fact they find a job quickly (and exit unemployment) without support from the LES. This latter group of individuals may have better future employment outcomes than ALMP participants by construction: if they exit unemployment again quickly they have a good chance of keeping that job, and are much more likely to be employed in several years or months than if they had remained unemployed. Additionally, LES counsellors are less likely to view such individuals as someone needing the support of an ALMP such as training, given that doing so could mechanically extend their unemployment duration for the duration of a course.
To address such sources of bias, the approach in this report controls for differences in demographic characteristics ( e.g. gender, education, age, etc.), observed skills and barriers to employment between ALMP participants and non-participants. Such an approach is then be used to produce an estimate of the “treatment effect” by comparing individuals that appear similar in terms of their observable characteristics. The outcomes of participants (the “treatment” or “intervention” group) are compared with a similar group of non-participants (the “control” or “comparison” group).
The econometric approach employs several techniques in order to ensure the comparability of the treatment and control groups and to provide unbiased results:
Only individuals with similar unemployment duration are compared with each other. This compares the labour market outcomes of those who enter an ALMP in a given month with those who have not (yet) entered an ALMP at a similar unemployment duration. The application of this “dynamic selection-on-observables” methodology – initially adopted by Sianesi (2004[7]) – is explained in greater detail in Box 3.1.
Individuals are also compared with each other only if they have identical values of several additional attributes. In addition to comparing individuals or similar unemployment duration, comparison individuals by construction have the exact same characteristics along a number of additional dimensions: calendar month and year of entry into the programme, age group, and whether they are receiving unemployment benefits (i.e, individuals not receiving unemployment benefits are only compared with other individuals not receiving unemployment benefits).
A rich set of additional personal characteristics are used to identify individuals with similar probabilities of entering the ALMP studied. Within the precise groups mentioned above, individuals are further matched with similar individuals based on an estimate of the probability that they enter into the ALMP studied. Such an approach – based on a so-called propensity score – is commonly used in the literature to tackle for the difficulty of otherwise accounting for a wide array of additional personal characteristics (Card, Kluve and Weber, 2018[8]). The propensity score is a measure of the probability of participating in the policy under analysis. The calculations of the propensity score take into account the following factors: (i) each individual’s employment history (earnings, duration of employment), (ii) employment barriers (as identified and coded by LES counsellors), (iii) demographic characteristics (education, gender), (iv) skills (foreign language, ICT skills, etc.), and (v) municipality of residence. The definition of the age categories parallel the target groups for inclusion in the ALMPs described in Section 3.2. Details on these attributes will be presented in the accompanying technical report (OECD, forthcoming[6]).
The outcomes presented are measured relative to individual-specific pre‑treatment outcomes. Individuals participating in ALMPs at different times throughout their unemployment spells may have different unobservable characteristics – aspects that are important for their probability of entering employment but are not observed in the data. For this reason, the estimates presented in this impact analysis account for individual-specific factors by comparing differences in outcomes before and after ALMP participation for specific individuals, for both individuals in the treatment and control groups (the so-called “difference‑in-difference” approach). This controls for an additional potential source of bias.
The choice of the research design is dictated by the relatively broad eligibility criteria of the Lithuanian ALMPs and the availability of rich administrative data. In the case of Lithuania, it is not possible to apply a research design that would exploit strict eligibility criteria, such as an age threshold. In such a research design, groups of individuals who are not eligible for a programme – for example, in the case of a programme targeted toward youth, because they have just crossed an age threshold – could serve as the natural basis for establishing what would have occurred to participants had they not participated (the so‑called counterfactual outcomes). Instead, in the case of this evaluation, in the absence of a strict eligibility criteria based on a threshold, the research design makes use of the rich available administrative data to match individuals along a number of dimensions, including their unemployment duration at the precise calendar month when an individual enters an ALMP. Such an approach is often used in impact evaluations and was also employed in a recent OECD evaluation of ALMPs in Latvia (OECD, 2019[9]).
Box 3.1. Econometric approach: Dynamic selection-on-observables
When individuals begin ALMP measures (are “treated”) at different times throughout their unemployment spells, selecting “dynamically” into such measures, the set of individuals who were never treated does not serve as a suitable comparison group for those who were treated. Individuals only become available for treatment if they stay in unemployment long enough. Conversely, one of the main reasons that some individuals do not get treated is because they are able to find jobs and exit unemployment quickly. This motivates an approach that does not simply compare the ever treated with the never treated, but rather compares those who begin treatment at a given point in their unemployment spell with those who have been unemployed for a similar duration but have not yet entered an ALMP. Note that individuals in both the treatment and control groups are potentially eligible to enter treatment, but individuals in the control group who are paired with individuals in the treatment group do not enter treatment in the same month as the individual entering treatment (although they may subsequently enter treatment). This ensures that the probability distribution of individuals subsequently finding a job or of later joining a programme is the same as the distribution for the similar treated individuals had they decided to wait longer as well. This “dynamic selection-on-observables” method was developed by Sianesi (2004[7]).
In this report, the dynamic selection-on-observables approach is implemented in conjunction with nearest neighbour propensity score matching. This entails the following:
1. Calculating propensity scores based on a rich set of covariates – each individual’s employment history (earnings, duration of employment), employment barriers (as identified and coded by LES counsellors), demographic characteristics (education, gender), skills (foreign language, ICT skills, etc.), and municipality of residence. The scores are calculated separately for each combination of programme and calendar year.
2. Matching exactly on each pre‑treatment duration (, the amount of time between registration and the start of treatment), calendar month and year of entry into the programme, age group, and whether individuals are receiving unemployment benefits; in other words, grouping individuals with exactly the same values of characteristics.
3. Within the groups defined in the second step, conducting nearest neighbour matching – pairing individuals with similar propensity scores.
4. Estimating treatment effects separately for each time horizon of interest (, the amount of time elapsed since the start of the ALMP measure, when the employment and earnings are measured). Denoting potential labour market outcomes (such as employment or earnings) for an individual () as , where under treatment and otherwise, the average treatment effect on the treated (for each is then:
The s are the key treatment effects reported for labour market outcomes in this analysis, looking at individuals every 3 months from month 3 to 36 after entering treatment (for individuals in the treatment group) or after being matched to an individual in the treatment group (for individuals not entering treatment). Given the exact matching on calendar month and year and the nearest neighbour matching, any time‑specific effects are differenced out by construction.
A key identifying assumption in propensity score matching is that all outcome‑relevant differences between programme participants and non-participants are captured in their observed characteristics. In other words, conditional on observed covariates, the selection into the treatment can be considered random (e.g. Imbens (2000[10])). If selection into programme is governed not only by observables but also by unobservable individual characteristics correlated with the potential outcomes, then propensity score matching cannot produce unbiased estimates of treatment effects. To address this issue, the evaluation method in this report combines propensity score matching with a difference‑in-differences approach (Heckman et al. (1998[11]); Smith and Todd (2005[12])). Intertemporal changes in outcomes between participants are compared to changes in outcomes for the comparison group, where changes are measured relative to a pre‑programme benchmark period.
Given the large sample sizes involved in the analysis, with several thousand participants per year in each of the programmes analysed, the analysis use direct matching estimators. This has the benefit of exhibiting the smallest bias for all sample sizes, even though nearest neighbour matching has the drawback of higher variance estimates (Huber, Lechner and Wunsch, 2013[13]). With large sample sizes, the superior bias properties gain in importance given that the absolute difference in precision relative to more efficient estimators diminishes as the variances tend to zero asymptotically. The standard errors are estimated using the adjustment outlined by Abadie and Imbens (2016[14]), which take into account the fact that the propensity scores are also estimated.
Under this framework, the dynamic selection-on-observables approach can only be used to estimate the treatment effect of the first ALMP measure in which individuals participate. Everything that happens after starting participation in the first ALMP measure is effectively treated as part of individuals’ outcomes, even if that entails not working due to further participations in ALMP measures.
3.7. A rich set of labour market outcomes are evaluated
CIEs of ALMPs typically examine outcomes such as the change in the probability of becoming employed for ALMP participants compared to similar non-participants. The effects of ALMPs on employment probability have been widely studied, with a meta‑analysis by Card, Kluve and Weber (2018[8]) including employment probability estimates from 111 impact evaluations of ALMPs. While this outcome is certainly important given one ultimate aim of ALMPs is to help individuals become employed, the focus on this outcome may also be partly dictated by data availability: data on other outcomes is often more difficult to obtain.
In the case of Lithuania, the rich and comprehensive data available enable the analysis to track a wide set of outcomes in evaluating the programmes studied and over a relatively long period. The outcomes are tracked continuously over up to the three‑year period starting with the beginning of the programme. Outcome values are calculated on a monthly basis and tracked over time relative to a reference month, which is defined either as the month when an individual enters an ALMP (for the treatment group) or that same calendar month for an individual in the control group who is matched to someone in the treatment group. Details on the calculations of these outcomes will be provided in a forthcoming technical report which is to accompany this review (OECD, forthcoming[6]).
The following outcomes are examined:
Probability of entering employment. This probability is measured using a binary outcome variable which is equal to 1 if individual is employed at certain time, and equal to 0 otherwise.
Cumulative employment duration. This measures the cumulative duration of all jobs held during the observation time, after the reference month.
Wages. This is calculated as earnings per calendar day, i.e. monthly earnings divided by the number of days in the month during which an individual was employed in constant 2015 prices. Due to data limitations, it is not possible to calculate an hourly rate.
Occupational mobility. The analysis maps the occupation of individuals entering employment onto an occupational index, which can be interpreted as a “job ladder”. The construction of the index is detailed in Section 3.8.
Cumulative earnings. This measures total earnings, gross of taxes and contributions, in constant 2015 prices, received in all jobs held during the observation time.
Cumulative earnings net of subsidies. In order to assess the cost effectiveness of the studied ALMPs, the analysis also compares the benefits of each programme as expressed by cumulative earnings premium over the three year time horizon, gross of taxes and contributions, with the direct costs incurred by the programme. All amounts are in constant 2015 prices.
Including cumulative earnings net of subsidies as an individual labour market outcome warrants additional discussion. Most of the outcomes examined – such as employment probability or days worked – are directly relevant both for programme participants and for the policy makers designing and implementing the measures. Cumulative earnings net of subsidies, on the other hand, are relevant only to the policy maker given that the participant does not bear the direct training costs. However, similar to the measure of cumulative gross earnings, this outcome presents a measure of earnings that differs from the earnings net of taxes and contributions that an individual ultimately receives. Note that like the other outcomes, cumulative earnings net of subsidies are calculated at the level of individual participants. This is necessary because the precise subsidy amounts vary considerably across individuals. In the case of vocational training, the training costs depend on the duration and type of training offered. Employment subsidies are linked to the level of wages paid by the employer (subject to certain ceilings).
In addition to aggregate effects, results are presented across sub-groups of individuals and, in the case of vocational training, by selected programme attributes. The results examine sub-groups of workers based on their gender, age, education level, urban or rural location and unemployment duration. For the vocational training programmes, the results also examine the role of tripartite agreements, the aim of the training (qualifications and/or competencies), and across formal or non-formal training.
3.8. Looking beyond employment prospects to analyse occupational mobility
In addition to analysing outcomes typically examined in CIEs of ALMPs, such as employment probability or earnings, the work with Lithuania aims to address another important question: the effect of participation in ALMPs on occupational mobility. A large body of empirical evidence has documented the “scarring” effect of job loss, with measurable effects on wages that can persist long after an individual becomes re‑employed (for example, Lachowska, Mas and Woodbury (2020[15])). Empirical evidence also shows that jobseekers exiting employment tend to disproportionally enter (or return to) low-skills occupations compared to the employed population (Bisello, Maccarrone and Fernández-Macías, 2020[16]). ALMPs may help counteract these effects, mitigating or conceivably even reversing the typically observed negative effects of job loss on an individual’s career trajectory. Training programmes may offer the opportunity to acquire skills or credentials necessary for employment in more high skills occupations. Employment subsidies may make employers more willing to hire a given jobseeker and possibly invest in on-the‑job training.
In order to provide a tractable measure of occupational mobility, the analysis relies on an occupational index, which is calculated from observed wages. Following the approach adopted by Laporšek et al. (2021[17]), a wage index is calculated for each detailed occupational code using data on the wages and employment of all employed individuals in Lithuania during the 2018‑20 period.8 This index maps each of the 440 distinct occupational codes observed in the data into an index that has an intuitive and practical interpretation: an occupation whose index value is one unit greater than another occupation’s index value has an average real monthly wage that is one euro greater. Furthermore, increases and decreases in the index can be interpreted, respectively, as positive and negative changes in an individual’s occupation: climbing up or down the occupational ladder.
The occupational index distribution for Lithuania shows that following unemployment, individuals who become re‑employed disproportionally enter lower-ranked occupations (Figure 3.4). Following an unemployment spell, a larger share of individuals become employed in occupations whose mean monthly wages are below EUR 1 000; conversely, prior to becoming unemployed, a proportionally larger share of individuals were employed in higher ranked occupations. On average, individuals becoming re‑employed have an occupational index that is approximately EUR 100 lower, corresponding with a roughly 5 percentage point drop down the distribution of the occupational index.
Figure 3.4. Individuals who become re‑employed after unemployment disproportionally enter lower-paid occupations
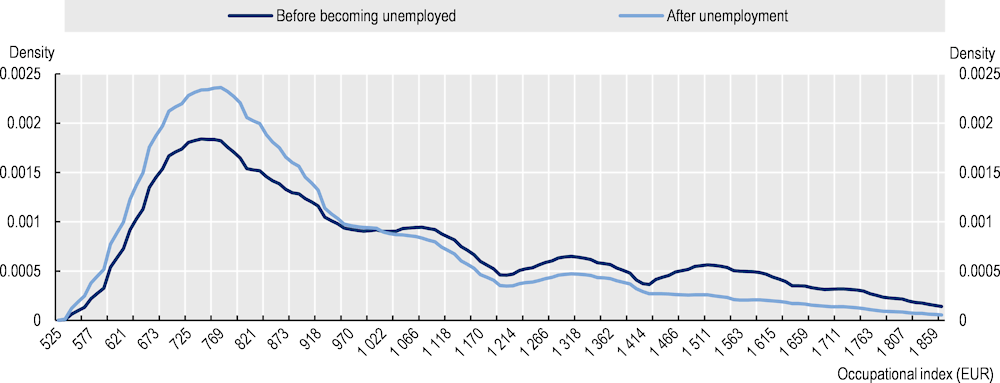
Note: The heights of the lines indicate the relative share of individuals in occupations whose average wages are on the horizontal axis. The distributions are calculated for all individuals who were registered as unemployed at some point during the 2014‑20 period. Observations with index value above EUR 1 866 are excluded from the kernel density chart. The chart includes persons who were registered as unemployed during the 2014‑20 period.
Source: OECD calculations based on data from the Lithuanian Employment Service and Lithuanian State Social Insurance Fund Board.
While a descriptive analysis of occupational index distributions as presented in Figure 3.4 is instructive for understanding the underlying data, it does not account for a multitude of possible underlying factors that could explain the differences in the distributions. For instance, differences in the occupational index distributions before and after unemployment may be subject to composition effects, with a subset of individuals more likely to become re‑employed. To account for such factors, as outlined in Section 3.5 above, the impact evaluation results in the next chapters take into account counterfactual outcomes of participants had they not participated in the programmes.
References
[12] A. Smith, J. and P. E. Todd (2005), “Does matching overcome LaLonde’s critique of nonexperimental estimators?”, Journal of Econometrics, Vol. 125/1-2, pp. 305-353, https://doi.org/10.1016/j.jeconom.2004.04.011.
[14] Abadie, A. and G. Imbens (2016), “Matching on the Estimated Propensity Score”, Econometrica, Vol. 84/2, pp. 781-807, https://doi.org/10.3982/ecta11293.
[16] Bisello, M., V. Maccarrone and E. Fernández-Macías (2020), “Occupational mobility, employment transitions and job quality in Europe: The impact of the Great Recession”, Economic and Industrial Democracy, p. 0143831X2093193, https://doi.org/10.1177/0143831x20931936.
[4] Calvino, F., C. Criscuolo and C. Menon (2016), “No Country for Young Firms?: Start-up Dynamics and National Policies”, OECD Science, Technology and Industry Policy Papers, No. 29, OECD Publishing, Paris, https://doi.org/10.1787/5jm22p40c8mw-en.
[8] Card, D., J. Kluve and A. Weber (2018), “What Works? A Meta Analysis of Recent Active Labor Market Program Evaluations”, Journal of the European Economic Association, Vol. 16/3, pp. 894–931, https://doi.org/10.1093/jeea/jvx028.
[18] European Commission (2022), Databases and indicators - The LMP database, Directorate General for Employment, Social Affairs & Inclusion, https://ec.europa.eu/social/main.jsp?catId=1143&intPageId=3227&langId=en.
[11] Heckman, J. et al. (1998), “Characterizing Selection Bias Using Experimental Data”, Econometrica, Vol. 66/5, p. 1017, https://doi.org/10.2307/2999630.
[13] Huber, M., M. Lechner and C. Wunsch (2013), “The performance of estimators based on the propensity score”, Journal of Econometrics, Vol. 175/1, pp. 1-21, https://doi.org/10.1016/j.jeconom.2012.11.006.
[10] Imbens, G. (2000), “The role of the propensity score in estimating dose-response functions”, Biometrika, Vol. 87/3, pp. 706-710, https://doi.org/10.1093/biomet/87.3.706.
[15] Lachowska, M., A. Mas and S. Woodbury (2020), “Sources of Displaced Workers’ Long-Term Earnings Losses”, American Economic Review, Vol. 110/10, pp. 3231-3266, https://doi.org/10.1257/aer.20180652.
[17] Laporšek, S. et al. (2021), “Winners and losers after 25 years of transition: Decreasing wage inequality in Slovenia”, Economic Systems, Vol. 45/2, p. 100856, https://doi.org/10.1016/j.ecosys.2021.100856.
[2] Ministry of Social Security and Labour, Lithuania (2017), Įsakymas dėl užimtumo rėmimo priemonių įgyvendinimo sąlygų ir tvarkos aprašo patvirtinimo [Order on the approval of the conditions and procedure for the implementation of employment support measures].
[5] OECD (2022), Part-time employment rate (indicator), https://doi.org/10.1787/f2ad596c-en (accessed on 5 April 2022).
[9] OECD (2019), Evaluating Latvia’s Active Labour Market Policies, Connecting People with Jobs, OECD Publishing, Paris, https://doi.org/10.1787/6037200a-en.
[3] OECD (2016), “No Country for Young Firms? Policy failures and regulations are a greater obstacle for start-ups”, OECD, Paris, https://www.oecd.org/sti/ind/Policy-Note-No-Country-For-Young-Firms.pdf.
[6] OECD (forthcoming), “Technical report: Impact Evaluation of Vocational Training and Employment Subsidies for the Unemployed in Lithuania”, OECD, Paris.
[1] Republic of Lithuania (2016), Law on employment, https://e-seimas.lrs.lt/portal/legalAct/en/TAD/5f0be3809c2011e68adcda1bb2f432d1.
[7] Sianesi, B. (2004), “An Evaluation of the Swedish System of Active Labor Market Programs in the 1990s”, Review of Economics and Statistics, Vol. 86/1, pp. 133-155.
Notes
← 1. This share of registered unemployed is calculated based on the number of participants in any ALMP according to data from the European Commission’s ALMP database (European Commission, 2022[18]) and the total number of individuals registered as unemployed at any point during 2019.
← 2. For jobseekers with grave disabilities or working capacity up to 25%, the subsidy may be paid until the end of employment (indefinitely). For jobseekers with medium disabilities or working capacity between 30% to 40%, the subsidy may be paid up to 24 months. During the 2014‑20 period, over 95% of individuals received the subsidies for six months or less.
← 3. This requirement applies to no-fault dismissals. Separations are permissible in certain cases, e.g. at-fault dismissals due to gross negligence on the part of the worker.
← 4. The “low-skills” category contains individuals with have completed up to a lower secondary education.
← 5. Note that the urban category corresponds to the following municipalities where the city and the district are separate entities: Vilniaus, Kauno, Klaipėdos, Šiaulių and Panevėžio. The category “Urban” thus constitutes the largest five urban municipalities, while the category “non-urban” corresponds to areas that are not large urban municipalities.
← 6. More information on the data used and how it was processed will be available in the technical report accompanying this publication (OECD, forthcoming[6]).
← 7. For individuals entering multiple ALMPs during an unemployment spell, the first ALMP is typically much longer than the second one (spells with three or four ALMPs are extremely rare, occurring in less than 0.7% of unemployment spells). The average duration of the first ALMP entered amounted to 227 days compared to 87 for the second spell. This is largely because individuals entering a second ALMP typically enter a very short-term vocational training: on average, vocational training as the second ALMP lasted 27 days, compared to 97 days for vocational training entered as the first ALMP.
← 8. The analysis uses 4‑digit ISCO‑08 codes and is calculated from real monthly wages at constant 2015 prices. Further restrictions are made in calculating the index, such as excluding individuals who are self-employed, individuals with earnings below the statutory minimum wage, and outliers with extremely high reported wages.