A pre-requisite to reinvigorate the productivity engine is to identify its underlying driving forces. This chapter reviews the most important drivers of multifactor productivity growth: those that boost innovation and experimentation of new knowledge and technologies, such as research and development, digitalisation and investment in intangible assets; those that contribute to the diffusion of existing knowledge and technologies, including human capital and public infrastructure; and those that facilitate the allocation of resources within or between sectors and firms, such as competition, globalisation and financial development. This chapter also discusses the importance of good governance and institutions, which emerge as a cross-cutting factor affecting all three dimensions.
Identifying the Main Drivers of Productivity Growth
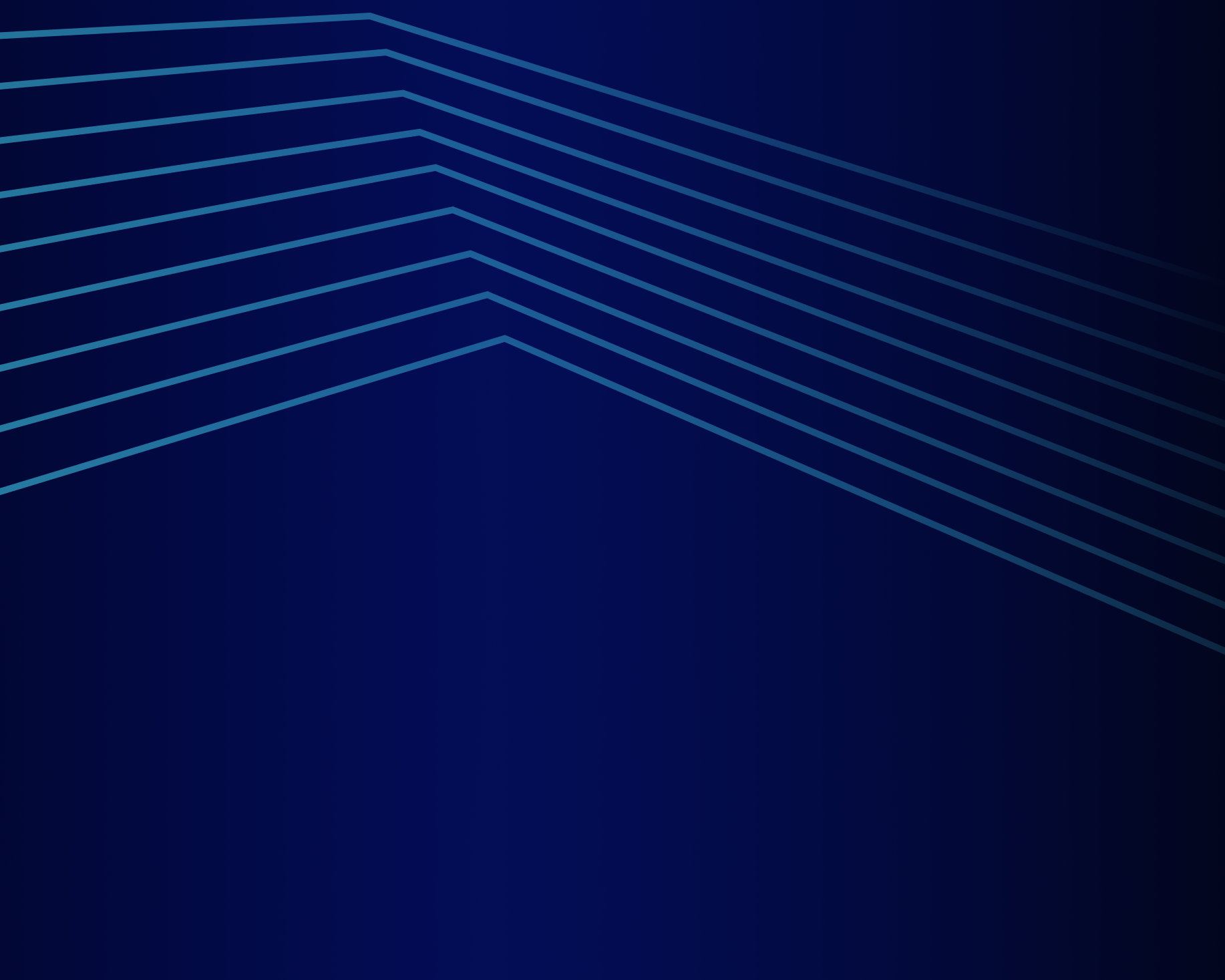
3. The key drivers of MFP growth
Abstract
Introduction
A pre-requisite to reinvigorate the productivity engine is to understand its role, on economic growth, as well as the underlying forces driving its potential. Multifactor productivity (MFP) is a complex, multifaceted concept whose developments can be influenced by a wide range of policy and institutions. A simple and illustrative framework, inspired by the analysis set out in 2015 in The Future of Productivity (OECD, 2015), helps to classify the MFP drivers into three categories:
Those that boost innovation and experimentation of new knowledge and technologies: Research and Development (R&D), digitalisation and investment in intangible assets;
Those that contribute to the diffusion of existing knowledge and technologies: skills and qualifications, and public infrastructure; and
Those that facilitate the allocation of resources within or between sectors and firms: competition and business dynamics, globalisation and financial development.
In practice, this allocation is somewhat arbitrary with some of the drivers belonging potentially to several categories. For instance public infrastructure can arguably boost innovation and promote the diffusion of existing technologies. Governance and institutions are a cross-cutting factor affecting all three dimensions. In addition, very often MFP drivers interact and complement each other. Complementarities and spillovers across the different drivers need to be accounted for when designing economic policies to maximise their impact on MFP and in turn growth and living standards.
This chapter scrutinises the economic literature to review each of these factors, the challenges affecting their measurement and the impact they have on MFP growth.
Research and development
R&D is thought to be an important determinant of productivity as a major source of innovation and knowledge accumulation in an economy (Coe and Helpman, 1995). In a globalised economy with international trade in goods and services and foreign direct investment, a country’s productivity may depend on both domestic R&D as well as the R&D efforts of trading partners and neighbours. Domestic R&D produces traded and non-traded goods that promote a more effective use of resources, while enhancing the country’s capacity to absorb and benefit from foreign technological advances. Meanwhile, foreign R&D has the potential to yield both direct and indirect benefits. Direct benefits may come from learning about new technologies, materials, production processes or organisational systems. Indirect benefits may arise from imports of goods and services that have been developed by trading partners.
The System of National Accounts 2008 (2008 SNA) introduced the treatment of R&D expenditures as investment. R&D is therefore treated identically to all other fixed assets, contributing to the stock of capital in a country and providing production services together with all other fixed assets. As a result, the direct contribution of R&D to GDP growth is included in the overall contribution of capital input and excluded from the residual MFP growth.
Nonetheless, R&D may still have indirect effects on MFP growth, for example through its interaction with other production factors, i.e. spillover effects. Barro and Sala-i-Martin (2004) identified two main avenues for such spillovers from R&D to MFP growth. First, through an expansion in the range of available inputs and raising the stock of knowledge thereby reducing future R&D costs (i.e. varieties models: Griliches and Lichtenberg, 1984; Coe and Helpman, 1995). Second, through improvements in the quality of intermediate inputs, or reductions in the cost of providing inputs of a given quality, enabling future innovators to begin their own improvements from a higher quality level (i.e. quality ladder models: Grossman and Helpman, 1991; Aghion and Howitt, 1992).
Much of the empirical literature demonstrates the MFP growth boosting properties of R&D (Griliches, 1980; Coe and Helpman, 1995; Guellec and Van Pottelsberghe, 2004; Wieser, 2005; Coe, Helpman and Hoffmaister, 2009; Hall, 2011; Ang and Madsen, 2013; Herzer, 2022). That said, some recent literature points to a less straightforward relationship between R&D and productivity, indicating that innovation (part of which related to R&D) has a positive effect on productivity in developed countries, but not necessarily less developed economies (Hammar and Belarbi, 2021). Recently empirical studies have suggested that although R&D is an important driver of productivity, the efficiency of R&D activity has declined over the past decades, with more targeted support for innovation being required to foster productivity growth (Miyagawa and Ishikawa, 2019; Bloom et al., 2020).
Even with recent trends pointing to a weakening of R&D efficiency, there is an impetus for governments to fund or otherwise encourage R&D. However, different countries may need to prioritise different types of R&D investments. For example, smaller countries which constitute a lower share of the global R&D stock and countries further from the technology frontier appear to have relatively more to gain from assimilating new technologies from the international knowledge stock (Guellec and Van Pottelsberghe, 2004; Coe, Helpman and Hoffmaister, 2009). Indeed, the direct costs of absorbing foreign technology when domestic conditions are right must be lower than the cost of inventing similar technology. For these countries, creating the right domestic conditions through free flowing international trade and development of high-skill human capital are essential to make the most out of the international knowledge stock. That being said, some results show that domestic R&D intensity is positively related to the impact of foreign R&D on MFP growth, meaning that some domestic R&D is important to foster absorptive capacity (Guellec and Van Pottelsberghe, 2004). On the other hand, larger countries and those closer to the technology frontier have a lot more to gain from increasing investment in domestic R&D efforts.
This section will begin with a discussion of the key measurement challenges of R&D, before summarising the empirics on the relationship between R&D and productivity, highlighting the complementarities with other determinants of MFP growth and addressing issues related with the causation between R&D and MFP.
Measuring R&D is conceptually challenging
With the implementation of the 2008 SNA, R&D expenditure is now treated as an investment that gives rise to a knowledge-based asset. Together with all other capital assets, R&D is accounted for in terms of its provision of inputs into production in the form of capital services. In other words, R&D capital is included within the measure of capital input, and affects MFP growth directly. Treating R&D as a capital asset in the growth accounting framework implies that the higher the contribution from R&D capital to GDP growth, the lower the (residually derived) growth in MFP.
While national accountants have treated R&D expenditure as investment since the introduction of the 2008 SNA, it is likely that R&D contributes to economic and productivity growth beyond its direct effect via the stock of capital and thereby derived R&D productive (capital) services. Indeed, MFP captures all kinds of improvements in the way inputs are combined to produce output, including spillover effects from one production factor to another, and can therefore be strongly influenced by R&D and innovation.
Finding an adequate measure of R&D to analyse its impact on MFP brings with it some key conceptual challenges (Griliches, 1979). First, R&D takes time and once complete may not be implemented immediately, meaning current expenditures may not be expected to affect measured productivity for some time, necessitating assumptions about the relevant lag structure. Second, past R&D investments depreciate and eventually become obsolete as technology progresses, meaning the R&D stock is not just the accumulation of all past expenditures. Third, the level of knowledge in one sector or country is not only derived from its own R&D investments, but is also affected by spillovers from other firms, industries, or countries.
Recent evidence supports the use of the standard approach to calculate R&D capital services (APO-OECD, 2021). Diewert and Huang (2011) referred to the stock of R&D capital as a technology that “locates the economy’s production frontier (so that) an increase in the stock of R&D shifts the production frontier outwards”. This implies that R&D assets work as a technology index that affects the working of all other inputs. Nevertheless, Schreyer and Zinni (2021) compared the results of an econometric approach allowing R&D to work like a technology index (i.e. quasi-fixed input) shifting the production frontier outwards with the results of a standard approach where R&D is treated as all other fixed assets (i.e. variable input) in a sample of 20 OECD countries, and found very similar results between the two approaches.
Many different measures have been employed to capture the relationship between R&D and productivity. In his seminal work, Griliches (1980) explored three firm-level measures of R&D growth in United States’ manufacturing companies: growth in total company expenditures on R&D, growth in company-financed R&D expenditures (excluding federally supported) and the growth rate in the number of research scientists and engineers engaged in R&D.
Some empirical studies used instead measures of the R&D capital stock (often both domestic and foreign) as their measure of R&D (Coe, Helpman and Hoffmaister, 2009). Such measures generally use data on R&D expenditures and are calculated using a perpetual inventory method, which accumulates past purchases of capital assets (in this case R&D expenditures) adjusted for depreciation.
While R&D expenditures can be considered as a measure of R&D input, patents have been used as a measure by some studies as a proxy for R&D output. Ang and Madsen (2013), tested both R&D expenditures and patents as their measure of foreign knowledge stock to investigate the impact of international knowledge spillovers on MFP. These two measures each come with their own benefits and pitfalls. Patent data are decomposed into those filed by residents and non-residents allowing studies to distinguish between domestic and foreign innovations. Furthermore, patents are often filed based on “informal R&D” which would not be captured by a measure of R&D expenditure. The key disadvantages of using patent data as a measure of innovative activity are twofold. First, many innovations are not patented (e.g. non-codifiable innovations). Second, the value of patents varies substantially, though with enough data the law of large numbers may alleviate this problem. The use of R&D expenditures is also an effective solution to this second problem, under the assumption that the importance and value of individual innovations are, on average, proportional to the resources engaged in their development.
Estimates of returns to R&D are generally positive but vary widely
The empirical literature refers to returns from R&D from a number of sources, that can be summarised as private returns to R&D (from R&D investment within the firm) and social returns to R&D, which can occur through both domestic (inter or intra-industry) and international spillovers.
Firms can benefit directly from their R&D investments
Despite the considerable variation in the estimated returns to R&D between studies, there is evidence of a strong positive relationship between R&D expenditures and growth of output and MFP (Table 3.1).
Griliches (1980) estimated private returns to R&D, by exploiting microdata for about nine hundred large R&D conducting companies in the manufacturing sector of the United States, including variables on total R&D firm expenditures, R&D expenditure financed by the company, the number of research scientists and engineers, total company employment and sales, as well as matched data on value added, assets and other economic variables for the period 1957-1965. R&D total company expenditure growth is estimated to have had a strong and positive relationship to productivity growth (proxied by the difference between the estimated rate of growth of total company sales and the product of the rate of growth of total company employment and the average share of labour in sales). The source of the funding was not estimated to be of particular importance in explaining productivity developments.
Table 3.1. Returns to R&D in selected studies
Coverage |
Private returns |
Domestic returns |
International spillovers |
|
---|---|---|---|---|
Griliches (1980) |
United States Manufacturing 1957-1965 |
Total R&D exp: 7.6% Company R&D exp: 6.3% Scientists & engineers: 8.7% |
||
Wieser (2005) |
A survey of empirical evidence at the firm level |
Rate of return: 7%-69% (median 27%) Elasticity: 3%-38% (median 10%) |
||
Coe and Helpman (1995) |
21 OECD countries plus Israel 1971-1990 |
G7 countries: 13.4% Non-G7 countries: 8.9% |
Full sample: 6.0% |
|
Coe, Helpman and Hoffmaister (2009) |
24 OECD countries 1971-2004 |
G7 countries: 1.7% Non-G7 countries: 9.6% |
Full sample:20.6% |
|
Guellec and Van Pottelsberghe (2004) |
16 countries 1980-1998 |
Long-term elasticity of MFP with respect to business R&D (13%) and public research (17%) |
Long-term elasticity of MFP with respect to foreign R&D (45%) |
|
Herzer (2022) |
82 developing economies 1995-2016 |
Results across specifications 24.3%-31% |
Results across specifications 0.7%-6.1% |
|
Ang and Madsen (2013) |
China, India, Japan, Korea, Singapore 1955-2006 |
Import channel: 23.5% Export channel: 18.9 FDI channel: 13.5% Patent channel: 16.4% Proximity channel: 11.5% Other channel: 14.0 |
Import channel: 8.2% Export channel: 6.5% FDI channel: 12.2% Patent channel: 6.6% Proximity channel: 30.1% Other channel: 26.1 |
Note: Domestic returns correspond to returns to private/business plus public R&D, unless stipulated otherwise. These form one part of social returns, which incorporate both domestic returns and international spillovers. See reference list for full references.
Wieser (2005) surveyed an expansive literature on R&D’s contribution to productivity growth at the firm level. Across 31 studies with significant results the overall average private rate of return to R&D was 28.3%, with a range of 7-69% and a standard deviation of 13 percentage points.
The relationship between R&D and productivity at the firm level is complex. For example, there is significant uncertainty surrounding the outcome of R&D expenditure, as compared to the return on physical capital investment. Doraszelski and Jaumandreu (2013) modeled firm productivity growth as a consequence of R&D expenditures with uncertain outcomes and found that for a panel of Spanish firms R&D does explain a significant proportion of productivity growth. However, their estimates also suggest that engaging in R&D approximately doubles the degree of uncertainty surrounding the evolution of a firm’s productivity level. Another issue in the empirical literature is the difficulty in differentiating correlation from causation (see below).
R&D investments can spillover at home and abroad
Private rates of return to R&D are generally considered to be the tip of the iceberg, with the estimated “social” rates of return often being much greater. The term “social” means they implicitly account for the direct impact of R&D (i.e. the private rate of return at the firm level) and the externalities (i.e. inter firm R&D spillovers) generated by such innovative activities (Guellec and Van Pottelsberghe, 2004). In an increasingly globalised world an economy’s productivity is affected by both domestic R&D efforts, as well as spillovers from those of other countries (Coe and Helpman, 1995; Coe, Helpman and Hoffmaister, 2009). Therefore, social returns to R&D include both returns to domestic R&D investments and those that spillover from foreign economies, especially key trading partners. The channels through which these spillovers might impact productivity are multiple, for example, through learning about new technologies, materials or production processes, each of which facilitates more effective use of current resources and therefore results in productivity growth.
Both domestic and foreign R&D capital stock are closely related to MFP in developed economies, with the elasticity of MFP with respect to the domestic R&D capital stock being greater in larger countries, while in smaller countries the elasticity is greater with respect to the foreign R&D capital stock (Coe, Helpman and Hoffmaister, 2009; Coe and Helpman, 1995). Furthermore, domestic R&D capital and foreign R&D capital are found to be cointegrated (i.e. have one common trend that combines them in the long run), and are both significant determinants of MFP.
Returns to R&D vary depending on the source of investment. Guellec and Van Pottelsberghe (2004) analyse the long-term impact on MFP growth of business R&D, public R&D (performed in government laboratories and universities) and foreign R&D for a sample of 16 major OECD economies and found a relatively modest long-term elasticity of MFP with respect to domestic business R&D. This is on the lower end of estimates elsewhere in the literature, though this may be accounted for by the fact that public and foreign R&D are included as explanatory variables. Indeed, they found that the long-term elasticity of MFP with respect to public R&D was slightly greater, likely because the latter tends to focus on basic research (often performed by universities to enhance the stock of knowledge available to society as a whole), which is associated with more uncertainty and a higher social return than applied research.
Furthermore, foreign R&D was found to have a long-term elasticity of MFP around three times greater than either of the domestic R&D sources (Coe and Helpman, 1995; Guellec and Van Pottelsberghe, 2004). While this may seem unexpectedly high, it reflects the impact of low cost technology for the domestic economy. Indeed, the direct cost of absorbing foreign technology when domestic conditions are right are likely to be substantially lower than the cost of inventing it.
However, certain conditions appear to enhance or reduce the estimated elasticities across countries (Coe and Helpman, 1995). There seems to exist a positive relationship between a country’s R&D intensity and the elasticity of MFP with respect to the business R&D capital stock. The implication is that there are increasing returns to investment in research, possibly due to firms reaping internal returns to scale and complementarities between lines of research. This relationship might also reflect an increased ability to absorb the knowledge generated by other firms, industries or by the public sector (e.g. Griffith, Redding and Van Reenen, 2000). The share of government funding was found to have a negative effect on the elasticity of MFP with respect to business R&D, driven by defence-related public funding. A potential explanation is that this may lead to a crowding out effect.
Turning to the impact of foreign R&D on productivity growth, country size (as measured by GDP) matters (Guellec and Van Pottelsberghe, 2004). Smaller countries benefit more in productivity terms from foreign R&D than larger ones as smaller countries have less researchers, making collaboration with foreign researchers more important. Similarly to the results for business R&D, fostering a high absorptive capacity is important in order to adopt or improve foreign technology. On the same note, the impact of public R&D on MFP was found to be greater when business R&D intensity (measured as the ratio of business R&D expenses to business GDP) in the economy is higher. This implies that part of the effect of public research is indirect, with some of the benefits being realised through the use of its discoveries in the business sector. Many governments and international organisations are already trying to enhance this effect through the creation of stronger links between public and private research (Asian Development Bank, 2022).
The impact of public R&D on MFP was also found to be positively affected by the share of universities, as opposed to government laboratories, in public research (Guellec and Van Pottelsberghe, 2004). This may be partially explained by the fact that government research is often aimed towards public missions that do not have a direct impact on productivity, whereas universities perform basic research that could eventually be exploited by the industry towards technological innovation. Another explanation comes from the allocation of funding (project based or institutional) which may be more flexible and faster to adapt in universities. However, Elnasri and Fox (2015) found that in Australia both government research agencies and higher education offer greater potential gains to public funding as compared to the provision of tax incentives to firms for R&D investment. Similarly, Guellec and Van Pottelsberghe (2004) found that the impact of public research was decreasing with the share of industry funding of the higher education sector. They claimed that partnerships between firms and universities often involve more applied R&D than usual university research, and applied R&D has a lower potential long-term effect on growth than basic research.
While many studies focused on OECD or other predominantly developed economies, a few studies presented empirical evidence on the impact of R&D on MFP growth in developing and transition economies (Krammer, 2010, Madsen et al., 2010; Goñi and Maloney, 2017; Hammar and Belarbi, 2021; Herzer, 2022). It appears that while many imported goods and services are not a channel for international R&D spillovers, machinery and equipment imports are important for technology transfer from developed to developing economies, even if they occur predominantly between developed and middle-income economies, with low-income economies often not benefitting from the R&D conducted in more developed countries (Herzer, 2022).
However, there is some heterogeneity in the results found by these studies exploring the relationship between R&D and MFP in developing economies. While some of these studies found that foreign R&D (import related spillovers) has a greater impact on MFP than domestic R&D in transition economies (Krammer, 2010) and developing economies (Madsen et al., 2010), others found that domestic R&D has a greater effect on domestic MFP in both middle-income and low-income economies than foreign R&D (Herzer, 2022). Still, the greater effect of domestic R&D on MFP as compared with foreign R&D was more relevant among middle-income economies than for low-developing economies. Potential explanations for this difference include lower levels of human capital, less developed financial markets, political and economic instability, and poor protection of physical and intellectual property rights in low-income economies, all factors that may hinder the creation, adoption, and diffusion of knowledge and technology (Goñi and Maloney, 2017). Finally, some of the studies bringing together the developed and developing economies, found a less straightforward relationship between R&D and productivity, indicating that innovation (part of which related to R&D) has a positive effect on productivity in developed countries, but not necessarily less developed economies (Hammar and Belarbi, 2021).
Looking to the Asian economies, both international knowledge spillovers and domestic R&D appear to play an important role. Ang and Madsen (2013) examined the importance of the domestic R&D capital stock and foreign knowledge spillovers to MFP in high performing Asian economies (China, India, Japan, Korea, Singapore) over the period 1955-2006. They identified productivity effects of international knowledge spillovers through a number of channels: imports, exports, inward foreign direct investment (FDI), patents, geographical proximity and a general channel (whereby knowledge can spillover automatically without passing through any specific channel). Using R&D expenditures to measure knowledge stock, the results showed that MFP, domestic R&D capital stock, and international knowledge stock are cointegrated and that growth rates of both domestic and international knowledge stock are potentially important determinants of productivity growth. The average elasticity of MFP with respect to domestic R&D capital stock (0.16) was found to be only slightly higher than that for foreign knowledge spillovers (0.15). The results when using patents as the measure of knowledge stock produced almost identical conclusions, implying that both R&D expenditures and patents are reliable measures of innovation. Their estimates highlighted that knowledge has been transmitted through all identified channels, but that the import channel and the non-specific general channel have been the most important to these Asian economies. The impact of the domestic R&D knowledge stock is mostly higher than the corresponding estimates for OECD countries (i.e. Coe and Helpman 1995; Guellec and Van Pottelsberghe, 2004; Coe, Helpman, and Hoffmaister 2009). This likely reflects the fact that the contribution of R&D to productivity is higher in the “take-off” phase during which a substantial fraction of R&D activity is used to adapt and improve the technology that has been developed at the frontier.
However more recent estimates present a more reserved outlook. For example, Miyagawa and Ishikawa (2019) pointed to a decline in the efficiency of R&D in Japan over the past decade. Even if they found that the R&D efficiency is positive, they saw a decline, in particular, in the services sector. Therefore, R&D efficiency cannot be considered as constant. A key implication is that instead of viewing the scale of R&D expenditures as a single objective, countries may benefit from a more targeted approach to government support, possibly accounting for movements and industry differences in R&D efficiency. This reduction in efficiency might be considered intuitive and a good example of Moore’s law: the more innovation has already taken place, the less there is left to discover. Indeed, recent evidence for the United States points to the concept that ideas are getting harder to find, with research productivity falling in half every 13 years, meaning that research effort needs to be doubled just to sustain the same research output (Bloom et al., 2020). On the other hand, it could be argued that new advances and innovations have in fact opened up the range of possibilities, creating new and unexplored avenues for research.
The benefits to R&D investment are greater when the right complements are available
The R&D capital stock does not determine MFP growth in isolation and instead fits into a larger picture with other complementary variables. Therefore, much of the literature on the relationship between R&D and MFP investigates or controls for the role of other variables.
The inclusion of human capital, alongside domestic and foreign R&D stock, tend to reduce the impact of the R&D, without impacting their statistical significance (Frantzen, 2000; Cameron, Proudman and Redding, 2005). This highlights the complementarity between human capital and R&D, implying that part of the role of R&D in MFP growth is actually associated with the human capital engaged in adopting the resulting flow of innovations. As discussed above, human capital is considered to be an instrumental determinant in MFP growth, often facilitating returns from other determinants. Spillovers from R&D depend in part on skilled workers who can understand and build upon existing innovations (i.e. quality ladders), accelerating the diffusion of new technologies.
Institutions are also likely to play a key role in determining the effectiveness with which R&D is able to enhance MFP growth. Countries where it is relatively easy to do business or where the quality of tertiary education is relatively higher tend to benefit more from their own R&D efforts, from international R&D spillovers and from their own investment in human capital formation than other countries (Coe, Helpman and Hoffmaister, 2009). Patent protection may also affect MFP indirectly through its impact on R&D, encouraging firms to invest in riskier projects with higher returns (Coe, Helpman and Hoffmaister, 2009). Indeed, countries with stronger patent protection benefit relatively more from a given level of domestic R&D, with the same being true but to a lesser extent for foreign R&D capital. In addition, no significant difference is found between countries with legal systems originating in English or German law, but countries with legal systems based in French or Scandinavian law benefitted relatively less from a given level of R&D capital. More recently, Su, Wang and Peng (2021) found that the association between intellectual property rights (IPR) protection and MFP is not uniform across countries, but follows an inverted U-shaped form. However, the optimal level of IPR for MFP purposes is greater for developed than developing economies.
As indicated by the wealth of literature on international knowledge spillovers, and as highlighted in the section covering Globalisation, the role of international trade in technology diffusion, and hence R&D international spillovers, is key, especially for countries further from the technological frontier. Cameron, Proudman and Redding (2005) identified innovation and technology transfer as two avenues for productivity growth for manufacturing firms behind the technological frontier in the United Kingdom, with international trade augmenting the speed of technology transfer while R&D advances innovation. Aw, Roberts and Xu (2009) also addressed the intersection between R&D investments and international trade, here using data for electronics exporters. They find that a firm’s decision to export and to invest in R&D are interdependent, with the probability of the latter being increased by prior exporting activity. This is consistent with the idea that the returns to R&D investment are greater when they can be spread across a larger market.
Finally, both R&D and ICT use play a role in determining innovativeness and labour productivity (Martin and Nguyen-Thi, 2015). R&D and ICT investments act as “innovation enablers”, but not all increases in these inputs translate into equivalent increases in equivalent increases in a firm’s capacity to innovate. In addition, there is no evidence that product innovation has a significant effect on productivity levels when only R&D activities are taken into account as an innovation input, whereas productivity levels are slightly elevated when both ICT use and R&D activities are introduced.
Does R&D boost MFP growth, or vice versa?
The results presented so far have demonstrated the clear relationship between R&D and MFP, but have not approached the question of causality. This leaves it open to interpretation whether R&D investment really causes MFP growth and whether there is any sign of reverse causality. While it might be intuitive to assume a one way relationship from R&D investment to MFP growth, there may be some interaction in the opposite direction. For example, there may be a positive response of R&D spending in reaction to shifting demand patterns – i.e. productivity improvements may increase incomes which can then impact demand and so R&D spending.
A number of studies have investigated this relationship and have both confirmed a causal link from R&D to productivity and that the link is principally in this direction (Rouvinen, 2002; Frantzen, 2003; Lu, Chen and Wang, 2006). Frantzen (2003) analysed the causality between productivity and domestic and foreign R&D using panel data for different manufacturing sectors across a set of OECD countries over the period 1972-1994. For both the panel as a whole and the individual industries, Granger causality tests indicated that although there are feedbacks, the causation runs mainly from R&D to MFP and not vice versa, and that this causation is primarily long run in nature. This supports the results of Rouvinen (2002). These results are also upheld in Lu, Chen and Wang (2006), who found that the direction of causality is from the R&D capital stock and R&D spatial spillovers to MFP growth using panel data for a number of electronics firms during the 1990s.
Digitalisation, including ICT and other intangibles
The past two decades have brought with them new and disruptive technologies, giving rise to new forms of intermediation, service provision and consumption (Ahmad and Schreyer, 2016). Digitalisation has drastically changed the way people interact, consume and work. These innovations were expected to stimulate a new wave of productivity growth, similar to that seen following electrification and the invention of internal combustion engines at the end of the 1800’s. However, these gains from digitalisation have not yet materialised, with productivity growth in most of the world having been relatively subdued even before the 2007-2008 financial crisis (Fernald, 2014). This has raised a number of questions regarding potential lagged effects of these new technologies, structural versus cyclical factors and, of course, measurement.
The OECD Going Digital Toolkit identifies digital technologies and data as factors that hold promise to boost economic and productivity growth (OECD, 2022). Digital technologies and data have the potential to spur innovation, generate efficiencies and facilitate knowledge spillovers, helping to drive productivity growth. On average, information industries outperform other non-agriculture business sector activities in labour productivity terms. For example, in the OECD area the labour productivity of information industries was 162% of other non-agricultural business sector activities in 2018, with an even greater difference in the United States and Korea, at 237% and 280%, respectively (OECD, 2022).
Much of the empirical evidence on ICT and productivity has noted a strong relationship (Timmer and van Ark, 2005; Bloom, Sadun and Van Reenen, 2012; Venturini, 2015, Gal et al., 2019), though some studies have signposted more moderate effects (Hawash and Lang, 2020). Many papers highlight the importance of complementarities between ICT and human capital, with sufficient and adequate skills being necessary for the efficient adoption of ICT and the realisation of the benefits to productivity growth (Gal et al., 2019). The productivity gap between the United States and the United Kingdom highlights further complementarities relating to organisational structure and flexibility, which are key to yielding ICT-related productivity gains (Bloom, Sadun and Van Reenen, 2012). In the same direction, some level of domestic ICT production is an important ingredient in creating an environment conducive to the effective adoption of ICT and this cannot be substituted through ICT imports. It is also important to note that not all ICT is created equal and different types of ICT are important for different types of firms, with enterprise resource planning being more beneficial to large firms and cloud computing being more beneficial for small firms.
A number of studies suggest that intangible assets make up more than half of all capital in some countries (Corrado, Hulten and Sichel, 2005; Haskel and Westlake, 2017; Martin, 2019). While they are not straightforward to measure (Haskel and Westlake, 2017; Martin, 2019), the empirical evidence indicates that intangibles play an important role in productivity growth (Corrado, Hulten and Sichel, 2009; European Investment Bank, 2016), with economic competencies being a large contributor (Jona-Lasino et al., 2011; Hintzmann et al., 2021) and complementarities between different intangibles yielding substantial benefits (Crass and Peters, 2014; Raknerud et al., 2020). Data has become a particularly important intangible asset over the past decade, constituting a social and economic resource, including for value creation, decision-making, innovation and production (Mitchell, Ker and Lesher, 2021). While important, data is another intangible asset that is difficult to measure, with a number of approaches having been used over time (Ker and Mazzini, 2020; Li and Chi, 2021). So far, most experimental estimates have relied on a cost-based approach, using the cost of producing the information or know-how derived from the data (Mitchell, Ker and Lesher, 2021).
Regarding automation and AI, the need for improved measurement is paramount, requiring an adjustment of economic measurement frameworks to fully and better account for these types of additions to the intangible capital stock. Improved measures of automation would also be an asset for growth and productivity analysis, especially where these data allow for a link to be made with increasing capital shares. While the productivity gains to AI are currently somewhat subdued, the evidence shows that there is substantial potential for future gains.
This section will first outline some background on the measurement of the digital economy, before focusing on three main strands of the literature: the rising importance and productivity effects of intangible capital; the relation between information and communications technologies (ICT) and productivity; and the interaction between artificial intelligence (AI), labour displacement and productivity.
The growing digital economy needs to be accurately reflected in economic statistics
The measurement of the digital economy has long been a point of contention in the literature and was outlined at some length in APO/OECD (2021). Therefore, this report will revisit only briefly the key components of the digital economy and the measurement challenges therein.
One of the core manifestations of the digital economy has been the substantial increase in peer-to-peer transactions facilitated by online intermediaries in the corporate sector, which has given rise to the term sharing economy. These services come in a variety of forms, but can be generally placed into four categories: i) Dwelling services (e.g. AirBnB), ii) Business and transportation services (e.g. Uber, Lyft, Bolt), iii) Distribution services (e.g. Amazon, eBay) and iv) Financial intermediation services (e.g. GoFundMe, Kickstarter). It should be noted that the underlying transactions which make up the sharing economy are not in themselves new, with households having long engaged in peer-to-peer transactions such as the provision of rental services, taxi services, often unlicensed, and the sale of second-hand goods.
On the conceptual side, all of these peer-to-peer transactions fall within the national accounts’ production boundary, and therefore should be captured by GDP. However, digitalisation has increased the scale of these transactions, facilitated by new types of intermediaries. Therefore, while these transactions sit within the conceptual boundary of GDP, the question is whether the compilation practices currently employed to measure peer-to-peer transactions, and which were designed to measure low-scale, relatively insignificant, sums, are sufficiently robust to accurately measure them at much larger scale (Ahmad and Schreyer, 2016). However, the very cause of the increased size of the problem (the new intermediaries) may also be a source of the solution, in that they provide potential access to new administrative data or business accounts that record what were previously largely invisible (non-observed) transactions. This could not only contribute to solving the current compilation question, but also illuminates information on previously non-observed transactions.
Other forms of digitalisation have also become an established feature in many economies. One such incarnation is the increasing role of consumers as own-account producers, with widespread internet access allowing more and more households to provide services to themselves that used to be produced by private companies. For example, households are now able to use search engines and travel websites to book flights and plan holidays, while this would previously have required a dedicated travel agent. Other examples include the self-check in at airports, self-service payment at supermarkets, and on-line banking. All these examples suggest that households are increasingly involved in activities previously performed by producers and therefore included in GDP. Conceptually, this is not new to the system of national accounts, as it joins the traditional discussion regarding unpaid household activities, such as childcare, the preparation of meals, and gardening (Ahmad and Schreyer, 2016).
Own-account production of services, with the exception of owner-occupied housing services, is excluded from the national accounts’ production boundary. This is due to the importance of the third-party criterion used in the accounts and the importance that GDP retains its primary focus as a tool to guide macro-economic policy making, as well as valuation difficulties. For example, valuing households’ own-account production at replacement costs (based on market prices for comparable products) as opposed to an alternative valuation based on opportunity costs (based on the foregone salary of households when they produce this output) can lead to very different results (Ahmad and Koh, 2011). Hence, even though the substitution of own-account production for market production has the potential to distort cross-country and temporal comparisons of GDP and to affect the output and productivity of some industries, solving this issue by imputing a value for households’ own-account production could make the measurement issue even worse. The recommended solution is therefore to monitor the development of households’ own account production in a satellite account rather than in the central framework of national accounts.
Free and subsidised consumer products, such as free mobile phone applications or search engines, may be considered as other possible sources of GDP underestimation. These products are usually financed through advertising, and/or through the collection of data generated by users of these digital products. So far, national accountants value the production of these service providers based on the advertising revenues they generate and treat this production as an intermediate consumption of advertising agencies. Exactly the same accounting treatment is applied to radio and TV service providers. While the national accounts community is currently discussing the potential to treat (part of) the output of these service providers as final rather than intermediate consumption, the limited size of advertising services in GDP means the resulting effect on the value of output is expected to be small (Byrne et al., 2016; Ahmad et al., 2017).
Finally, digitalisation and the rapid pace at which ICT products (e.g. computers, mobile phones, etc.) are renewed over time creates significant challenges for the price and volume measurement of output and investment. The large share of new ICT products introduced every period requires that price statisticians rely on efficient quality adjustment methods to measure price inflation for these products. The NSOs of APO members are encouraged to keep abreast of the statistical literature on the price measurement of ICT products and to compare their deflators with those used in OECD countries. Since these goods are largely traded across countries, there should only be limited differences in their price levels and evolutions. Therefore, any significant difference in ICT price deflators across countries should be analysed with great care.
Ahmad et al. (2017) estimate the potential scale of mismeasurement in ICT price changes and its impact on GDP measures in selected OECD countries. They start by selecting the lowest average annual growth rate (“lower bound”) in national price indices of ICT equipment, computer software databases and communications services across the selected countries over the period 2010-2015. In the next step, they replace each country’s own price index for each of the three products, adjusted for the differences in general inflation rates in the country, by the selected “lower bound” growth in assets’ prices and estimate the impact on measured GDP depending on whether the affected products are used for final or intermediate use and on whether they are imported or domestically produced. The authors consider three scenarios to test the sensitivity of the results to variations in the use (final or intermediate) and origin (domestically produced or imported) of these assets. The results indicate that adjustments for potential mismeasurement of prices of ICT products can be expected to add on average 0.2% per annum to GDP growth rate across the selected OECD countries. Adjustments are larger if all ICT products are assumed to be domestically produced and used for final demand because in such cases the mismeasurement of ICT prices directly affects GDP; however, these assumptions are particularly extreme, if not unrealistic.
While Ahmad et al. (2017) show that the mismeasurement of ICT prices is unlikely to severely bias output and productivity measures in OECD countries, further work is needed to determine the impact on non-OECD economies, as the extent of potential ICT price mismeasurement could be more severe and, for many, the contribution of ICT production and exports more significant (OECD, 2018; OECD, 2019).
ICT has a positive impact on productivity, but how large?
Since the early 2000’s, many empirical studies have looked to information and communication technology (ICT) as a potential source of productivity growth. Indeed, the movement from paper record keeping and human “computers” to accessing information at the press of a button and automating countless manual processes is a clear source of productivity gains across all industries and a potential cause of productivity divergence across economies. However, some studies have noted that productivity improvements induced by ICT adoption may only materialise with delays of 5-15 years depending on the availability of and investment in complementary inputs, such as skilled labour, capacity to innovate and organisational changes (OECD, 2004; Corrado et al., 2017).
A number of empirical studies, including Timmer and van Ark (2005), identified the adoption of ICT as a potential cause for the gap in labour productivity growth between the United States and the European Union (EU) in the mid to late 1990’s and early 2000’s. While labour productivity growth in the United States accelerated from 1.3% during the period 1980–1995 to 1.9% during 1995–2003, EU labour productivity growth declined from 2.3% to 1.3%.. During the period 1985-1995 the superior performance of the European Union was driven by higher contributions from capital deepening in non-ICT assets and faster MFP growth. However, from 1995 onwards the United States saw increases in the contributions from capital deepening in both ICT and non-ICT assets and MFP growth, while the contributions from ICT capital deepening were more modest in the European Union and contributions from non-ICT capital deepening and MFP growth in the latter actually declined. For the period 1995-2001 the United States’ larger ICT-producing sector added 0.2 percentage point more per year to aggregate MFP growth than that of the European Union. Taken together these two effects explain almost all of the difference in labour productivity growth between the United States and the European Union between 1995 and 2001.
Bloom, Sadun and Van Reenen (2012) conducted a similar study comparing the United States and the United Kingdom, highlighting the role of the intensity of use and productivity of ICT in the observed productivity gap. They found that the mean value added per worker in establishments with above average IT capital per worker was 34% higher than in those with below average IT capital per worker in United States establishments, while the same figure was just 24% for establishments owned by non-US multinationals. One potential explanation could be selection bias, whereby multinationals from the United States cherry pick the most productive United Kingdom establishments, as opposed to United States ownership causing higher ICT productivity. Econometrics tests suggest no selection bias. However, a transfer of ownership from the domestic firm to the multinational enterprise was associated with an increase in productivity, particularly for a move to United States ownership.
After takeover by a United States multinational, an establishment benefits from significantly higher ICT-related productivity than a similar establishment taken over by a non-United States multinational (Bloom, Sadun and Van Reenen, 2012). The intuition is that United States firms benefit from lower adjustment costs, possibly due to more flexible labour regulations, allowing them to re-organise more quickly and take advantage of new ICT enabled innovations. In the case of Europe, Corrado et al. (2017) explore the channels through which intangible assets affect productivity growth using a combination of INTAN-Invest and EUKLEMS data for ten member states of the European Union between 1998 and 2007. Investment in the right intangible capital, especially organisational change and worker training, is key to fully exploiting the returns to ICT capital. The authors found that the output elasticity of intangible capital depends on ICT intensity, suggesting complementarities between ICT and intangible capital in production.
Using a sample of OECD countries, Venturini (2015) presents evidence that ICT capital is an important source of MFP spillovers, owing to its ability to create networking effects and enable knowledge externalities. The author’s baseline econometric results indicated a positive and significant relationship between ICT capital and MFP growth and between business enterprise R&D stock (but not public research capital) and MFP growth. Focusing on specialisation in ICT production, they found that while the ICT producing sector is relatively small (between 1 and 3% of business sector employment and value added), it performs around 20% of private research and accounts for around 25% of aggregate productivity spillovers from knowledge-generating activities, with the rest coming from the non-ICT producing industry.
The externalities derived from increases in regional ICT capital on firm productivity tend to be larger than the direct effects on firm productivity of raising that firm’s own ICT investment. Results show a relatively large gap, ranging from a ratio of around 1.5:1 up to 3:1 (Riley and Robinson, 2011; Geppert and Neumann, 2011).
Industry-level digital adoption is associated with significant productivity returns at the firm level, with little sensitivity to the inclusion of common drivers of adoption and productivity (e.g. skills or regulatory environment), or adoption rates being included at a lag versus at the beginning of the sample period (Gal et al., 2019; Cette et al., 2020). These analyses rely on the combination of industry-level cross-country data on the adoption of a range of digital technologies with firm-level cross-country data on MFP in an empirical framework allowing for productivity heterogeneity across firms. Furthermore, productivity gains are found to be greatest for high productivity firms, indicating that digital adoption may contribute to increase productivity dispersion across firms in an industry. In addition, if firms are grouped by size, it is observed that enterprise resource planning is more beneficial to large firms, whereas cloud computing is more beneficial for small firms, reflecting the efficiency gains for small firms related to not having to invest in large and expensive IT infrastructure. Further, these analyses suggest that digitalisation is, on average, most beneficial to manufacturing rather than services industries, and more broadly in industries relatively intensive in routine tasks.
However, the scale of the impact of ICT on MFP growth is found to differ in different types of economies. Hawash and Lang (2020) use data for a panel of 76 developing countries between 1991 and 2014, including information on ICT capacity and ICT usage. For these countries ICT was only a minor engine for MFP growth, with even those countries most intensive in ICT investment enjoying relatively modest benefits to MFP growth of between 0.1 and 0.3% annually compared with countries with lower ICT investment. Once again, the implication is that complementary investments, in intangibles among other drivers of MFP growth are particularly important.
Turning to the empirical evidence for Asian economies, Fukao et al. (2011) presented evidence on the growth contribution of ICT assets and resource allocation in Japan and Korea. The authors combined data from the EUKLEMS Database with ICT investment data for Japan and Korea based on Pyo, Jung and Cho (2007). The MFP growth rate in both the Japanese and Korean ICT-producing sectors was higher than in other sectors, including ICT-using sectors. Additionally, a much higher ICT investment/GDP ratio is found in Korea than in Japan, providing a potential explanation for the observed productivity differences. In fact, Japanese ICT capital accumulation was found to be slower than the United States and all major EU economies but Italy post-1995.
Intangible assets make up more than half of capital in some countries
R&D was discussed at length in the previous section, but it is in fact one of a number of intangible assets which contribute to productivity growth. Corrado, Hulten and Sichel (2005, 2009) identified three broad groups of business intangibles: computerised information, innovative property and economic competencies. Computerised information is largely composed of computer software, but also computerised databases used by businesses. Innovative property captures scientific and non-scientific R&D, mineral exploration, copyright and licensing costs, and other product development, design and research expenses. Finally, economic competencies include brand equity (e.g. spending on advertising, market research and developments of brands), firm specific human capital (e.g. spending on on-the-job training and education) and organisational structure (e.g. costs of organisational change and development). The European Investment Bank (2016) provides evidence on the relative scale of these three groups for a subset of European economies plus the United States. Their estimates for 2000-2013 show that in the EU141 investment in economic competencies (3.2% of GDP) and innovative property (2.6%) contributed most to intangible investment, while software (1.6%) played a smaller role. The same holds for the United States, where the overall role of intangible capital accumulation was found to be greater than the average EU economy.
The 2008 SNA enlarged the asset boundary by capitalising expenditures in weapons systems (tangible) and R&D (intangible), which were previously considered as intermediate consumption. As shown in Table 3.2, as well as R&D, several other intangible assets are identified and included in the asset boundary: mineral exploration and evaluation; computer software and databases; entertainment, artistic and literary originals; and other intellectual property products. Nevertheless, important intangible assets such as brand equity, organisational capital, and data remain at the moment outside of that asset boundary (OECD, 2021).
Depending on the purposes of the analysis, different capital assets can be grouped into different aggregate categories. For example, dwellings, other buildings and structures, machinery and equipment and weapons systems, and cultivated biological resources are often grouped to constitute the set of tangible assets, as opposed to intangible assets, also referred to in the 2008 SNA as intellectual property products (IPPs) (Table 3.2).
Table 3.2. Breakdown of fixed capital assets according to the 2008 SNA
|
2008 SNA code |
Produced fixed assets |
|
---|---|---|---|
Tangible assets |
N111 |
Dwellings |
Non-ICT assets |
N112 |
Other buildings and structures |
||
N11M |
Machinery and equipment and weapons systems |
||
N1131 |
Transport equipment |
||
N1132 |
ICT equipment |
||
N11321 |
Computer hardware |
ICT assets |
|
N11322 |
Telecommunications equipment |
||
N11O |
Other machinery and equipment and weapons systems |
Non-ICT assets |
|
N115 |
Cultivated biological resources |
||
Intangible assets |
N117 |
Intellectual property products |
|
N1171 |
Research and development |
||
N1172 |
Mineral exploration and evaluation |
||
N1173 |
Computer software and databases |
ICT assets |
|
N1174 |
Entertainment, artistic and literary originals |
Non-ICT assets |
|
N1179 |
Other intellectual property products |
Source: OECD Compendium of Productivity Indicators (2021), OECD elaboration based on the 2008 System of National Accounts.
Intangible assets make up an increasing part of economic capital, but many have not been or still are not included in the fixed asset boundary of national accounting standards. The average share of total investment over GDP fell in most countries over the past decade as compared with the period preceding the 2007-2009 financial crisis, which was driven by lower investment in tangible assets (OECD, 2021). However, investment in IPPs has performed much better. Indeed, even though there are differences across countries, investment in IPPs accounted for an increasing share of total investment in most economies over the past decade. For example, in Japan and Korea the share of total gross fixed capital formation (GFCF) made up of IPPs during 2010-2019 was greater than 2000-2007, growing from 20% to 23% and 15% to 20%, respectively. While substantial, this is only part of the picture, with investment in a more complete set of business intangible assets estimated at roughly equal to business investment in tangible assets (Corrado, Hulten and Sichel, 2005; Haskel and Westlake, 2017; Martin, 2019).
Measuring intangibles is far from simple
The exclusion of some intangibles, such as organisational capital, brand equity, and on-the-job training, from the production boundary of the SNA is not based on conceptual grounds but rather on the very practical difficulties involved in measuring them in a comparable and meaningful way across countries (OECD, 2016). Indeed, there are a number of measurement challenges associated with the estimation and in particular the valuation of intangible assets, with mismeasurement driving lower measured investment/GDP ratios, even if this has not been found to greatly affect its trend (Haskel and Westlake, 2017). For example, Martin (2019) outlined three main challenges in the measurement of business investment in brands: evidence is limited as to what fraction of branding constitutes investment; when it comes to in-house branding investment, it is difficult to identify which workers contributed; and once contributing workers have been identified there is limited evidence on what share of workers time is spent on long-lived (i.e. investment) branding activities. These types of challenges are common to most intangible assets that are developed on own-account (i.e. by a business for themselves), especially economic competencies like investments in training and organisational structure.
In the case of training, there is a clear conflict to treating it as investment due to the issue of ownership (Haskel and Westlake, 2017). The investment is made by the business in an individual employee, but from that point onwards, the employee essentially owns the asset with the benefits accruing to both the employee (through higher wages) and the business (through higher productivity). However, if the worker leaves the business they retain at least part of the benefits from that investment and may benefit from it elsewhere. It is for that reason that estimates of investment in training must make a clear distinction between general training (highly transferable) and firm-specific training which is more easily seen as owned by the firm. In this sense, while all on-the-job training is relevant and included as human capital accumulation, only the firm specific component (e.g. training on firm specific software) should be considered as an intangible asset under economic competencies. In practice, this is not a simple endeavor and requires detailed data, which do not always exist, that allows for the disentanglement of these components.
Data is a valuable resource in the information economy, but how can it be measured?
Over the past couple of decades, data – a new and distinct category of intangible – has become a social and economic resource, including for value creation, decision-making, innovation and production (Mitchell, Ker and Lesher, 2021). Data should be distinguished from the organisation of those data into computerised databases that unlike data are already considered as capital assets in the 2008 SNA.
The measurement of data is arguably even more difficult than the other categories of intangibles. The first challenge is defining what exactly it is that is being discussed. Data can refer to one of many things, including individual records of basic facts or observable phenomena (e.g. e-commerce transactions), wider datasets covering many individuals or countries, additional statistics and indicators derived from raw data, or even the business model of entire companies (e.g. weather forecasting, social media advertising, etc.). One definition, adopted by Mitchell, Ker and Lesher (2021), states that data is “information content that is produced by accessing observable phenomena and recording, organising and storing relevant information elements from these in a digital format, which can be accessed electronically for reference or processing”.
There are both conceptual and practical challenges to estimating the economic value of data. Conceptually, one might think that since data come in well-defined units, the value of data could be directly related to and distributed according to the volume of that data. However, two company records taking up the same quantity of storage space may be associated with vastly different values. On the same note, two identical databases could be used by different individuals or firms in different ways, providing very different economic or social value. These scenarios suggest that both content and context are important components in the valuation of data and that the volume approach is unlikely to yield reliable results (Mitchell, Ker and Lesher, 2021). How and what data were gathered, how they are stored, who can access them, under what terms and for what purpose, all have a bearing on the value associated with that asset.
The conceptual challenges to valuing data are interrelated with the various practical challenges. As most data are gathered by a business for their own purposes and are highly integrated with that business’ organisational capital, markets for data are relatively underdeveloped, limiting the prices that can be observed. Even if such prices were observed, the content and context of each transaction would likely yield a highly specific price for that transaction and not a generalisable price for similar data or data holdings more broadly. In addition, the diversity of content and context means that there is no universal standard for categorising data into types for statistical purposes. For this reason, indirect approaches to valuation are a potentially promising solution, often through expenditures on gathering, storing, maintaining, analysing and transferring data. However, even this is not exactly straightforward, with the ways expenditures are aggregated and published according to international standards making it difficult to distinguish expenditures and activities relate to data.
To overcome the various conceptual and practical challenges, there are a range of approaches to the valuation of data and data flows. For example, Ker and Mazzini (2020) consider four options directly linking data to economic value, including using business statistics to look at the revenues generated by firms creating explicit value from data, and comparing the growth in the value of data-driven firms with that of non-data-driven firms using stock market information. Li and Chi (2021) adopt a different approach, relating commercial estimates of global data flows to the organisational capital of big tech firms, with findings indicating that a five-fold increase in data flows is associated with a doubling of organisational capital (proxied by sales and general and administrative expenditures). However, while analytically useful, such methods are not necessarily aligned and integrated with the established frameworks used for compiling economic statistics such as the 2008 SNA (Mitchell, Ker and Lesher, 2021). In this context, there are a three major contenders for approaches to estimating the value of data: 1) market-based, using the market price of comparable products, 2) cost-based, using the cost of producing the information or know-how derived from the data, or 3) income-based, by estimating future cash flows that can be derived from the data. Due to limited markets for most types of valuable firm-specific data and given inconsistencies in valuation methods used by businesses to value the expected future income from an asset, the cost-based approach has so far proven to be the most conducive to the development of experimental estimates by countries. In short, this approach incorporates the value of the inputs used in production and the wage costs of production, as well as the return on capital for the use of any fixed asset in production.
Intangibles play a role in productivity growth
Like other fixed assets, intangible assets can be incorporated into the Solow–Jorgenson–Griliches sources-of-growth framework (Corrado, Hulten and Sichel, 2009). This framework allocates the growth rate of output to the share-weighted growth rates of inputs plus the residual. Therefore, in this approach intangible capital is treated symmetrically with tangible capital. As a result of casting a wider net for fixed capital assets, the share of labour in overall labour and capital costs is reduced and the share of capital is increased. This also has a bearing on estimates of MFP growth, measured by the residual.
Corrado, Hulten and Sichel (2009) apply this framework to data for the United States and present evidence on the role of intangibles in the growth accounting framework with and without the inclusion of intangibles for the periods 1973-1995 and 1995-2003. In terms of labour productivity, their results show that the inclusion of intangible investment in the real output increases the estimated growth rate of output per hour by 10-20% relative to the baseline case which completely ignores intangibles. Their results also highlight that between the first and the second period the role of intangible capital increased, reaching parity with tangible capital, and capital deepening played a larger role in accounting for labour productivity growth in that second period. This increasing role of capital deepening comes with an obvious consequence for estimates of MFP growth. The impact is greatest in the second period, during which the share of output growth attributed to growth in MFP falls from 1.4 percentage points to 1.1 percentage points when accounting for intangible capital. It is important to note that the majority of the contribution of intangibles comes from the non-traditional categories of intangibles they identified (i.e. those that at the time of that study were outside the fixed assets boundary), making their measurement important for productivity analysis.
Over the period 2000-2013, European Investment Bank (2016) indicate that including new intangibles raises the capital contribution and lowers MFP growth, as compared with the inclusion of only those intangibles included in the national accounts fixed asset boundary. The contribution of capital to GDP with intangibles capitalised in the EU14 was 0.7% per year (compared with 0.6% without) and the contribution of MFP growth fell from 0.4% to 0.3%. For the United States, the change was slightly greater, with the contribution of capital changing from 1% to 1.1% and the contribution of MFP growth changing from 0.9% to 0.7% per year.
Different intangible assets have different impacts on productivity in isolation or combination. Hintzmann et al. (2021) studied the productivity implications of the three intangible asset categories identified by Corrado, Hulten and Sichel (2005, 2009): computerised information, innovative property, and economic competencies. They found that for a set of 18 European countries between 1995 and 2017, all three categories of intangible assets contributed to productivity growth. Specifically, economic competencies together with innovative property were found to be the main drivers, with advertising and marketing, organisational capital, R&D investment, and design being most important. These results highlight the importance of complementarities between different intangible assets. Economic competencies were also singled out in Jona-Lasino et al. (2011), who found that economic competencies account for 9.7% of total labour productivity growth, compared to 4.8% for ICT and 3.5% for R&D.
At the firm level, the evidence also points to the importance of intangible assets, and complementarities between intangible assets, to productivity growth (Raknerud et al., 2020). Firms intensive in intangible assets are found to be an important driver of Norwegian productivity growth, but that increasing intensity alone is not the main driver. Again, complementarities between different types of intangible assets, such as human capital with R&D or ICT-capital, are key to yielding the greatest benefits. Results for German manufacturing and services firms paint a similar picture (Crass and Peters, 2014). Comparing the productivity effects of different intangible assets the evidence suggests a strong productivity effects of human and branding capital, with more mixed results for innovative capital and relatively weak results for the design & licenses and patents components of R&D, as well as organisational capital as a whole. Their results also identify several complementarities between different intangible assets.
Emerging evidence suggests that data and policies surrounding the use and movement of data affects firm productivity (Bakhshi et al., 2014; Ferrancane et al., 2018). Bakhshi et al. (2014) found that one standard deviation greater use of online data is associated with an 8% higher level of MFP and that firms in the top quartile of online data use are, other things being equal, 13% more productive than those in the bottom quartile. However, for the 500 UK firms studied, it was clear that accumulating data on its own has little or no effect on productivity, with greater data analysis and reporting of data insights being key to productivity gains. Ferrancane et al. (2018) examined how policies regulating the cross-border movement and domestic use of electronic data on the internet impact the productivity of firms in sectors relying on electronic data. They found that stricter data policies have a significant negative effect on the productivity performance of downstream firms in data-intensive sectors, with the strongest effects in countries with strong technology networks and for services sector firms.
Automation and AI promise growth, but are we measuring them properly?
While automation has been a process spanning the last 200 or more years, the tasks that have been automated have predominantly been routine lower-skilled tasks. Artificial Intelligence (AI) opens up a whole new set of less routine tasks which might be automated, such as driving cars or even making medical recommendations (Aghion, Jones and Jones, 2017).
In particular, AI could help to obviate the role of population growth in generating exponential economic growth as AI replaces people in the generation of new ideas. This is related with the concept of a “technological singularity” whereby a self-improving AI quickly outpaces human thought, leading to an “intelligence explosion” with infinite intelligence in finite time and accompanied by an explosion in economic growth. However, perhaps more relevant is the observation about how individual firms may influence, or be influenced by, the advance of AI, as this may actually reduce incentives for future innovation by facilitating rapid imitation and therefore reducing potential returns to innovation (Aghion, Jones and Jones, 2017).
The introduction of AI, which has already matched or surpassed human performance in at least certain domains, raises some measurement questions for productivity analysts: notably, where is this AI in our productivity statistics? While AI has been implemented and discussed at length over the past decade, productivity growth has declined and real income growth has stagnated in the United States. Brynjolfsson, Rock and Syverson (2017) provide a number of potential explanations for this observed “productivity paradox”. First, they consider the possibility of “false hope”, in that these technologies may not be as transformative as many expect and will not spur the type of economic growth experienced from the internal combustion engine or electrification. Another potential explanation is “mismeasurement”, whereby productivity benefits are already being enjoyed, but are just not accurately reflected in measures of output and productivity. However, there is a wealth of studies which present evidence that mismeasurement is not the primary explanation for the productivity slowdown (Cardarelli and Lusinyan, 2015; Byrne, Fernald, and Reinsdorf, 2016; Nakamura and Soloveichik, 2015; Syverson, 2017). A third possibility is “concentrated distribution”, meaning that the gains exist but are relatively narrowly distributed, partly owing to the fact that many profitable applications of AI, including targeting online advertisements and automating trading of financial instruments, have some zero-sum aspects. The final, and the one they judge potentially the most convincing argument, is one of “implementation lags”. Many of the most transformative applications of AI (including machine learning) have not yet been widely diffused and adopted, and like ICT and other technologies their full effects might not realise until they are accompanied by complementary innovations, skills and organisational adjustments. In addition, it is also possible that productivity gains from automation be quite small from small technological advances, for example where automation substitutes tasks in which labour was already relatively productive (Acemoglu and Restrepo, 2019).
More recent evidence suggests that there has been an upsurge in AI and robotics patenting activity in the latest years, implying that AI diffusion might have started to break through and exert some additional effect on individual firms and the economy as a whole. Damioli, Van Roy and Vertesy (2021) investigated the impact of AI on labour productivity using a panel of firms that filed at least one AI related patent, using a keyword-based approach and an AI-related dictionary to identify relevant patents. Their analysis shows that, once other patenting activities have been controlled for, AI patent applications are positively related to a company’s labour productivity and that this effect is particularly strong in the case of SMEs and services firms, suggesting the importance of an ability to quickly react and readjust to efficiently introduce AI applications into the production process.
One useful takeaway from this discussion is that the benefits of AI may not be automatic, but require effort and entrepreneurship to adjust and develop the required complements. This implies a role for governments to lower adjustment costs and put as many complements in place (e.g. through promoting innovation, technical education, improving organizational practices) as possible in order to reap the potential productivity gains from AI.
Human capital
Human capital is defined as the stock of knowledge, skills and other personal characteristics embodied in people that contributes to their productive capacity (OECD, 2022). The stock of human capital in an economy is a key input into the production process, with two principal avenues through which human capital is modelled in growth regressions (Engelbretcht, 2002). The first is the “Lucas approach” (i.e. the level effect), treating human capital as any other factor of production like labour and physical capital, with an accumulation of human capital increasing labour productivity for a given technology. The second is the “Nelson-Phelps approach” (i.e. the rate effect), affecting countries’ ability to innovate and to adapt and absorb new technologies.
There are a number of measurement challenges in accounting for human capital. Connected to the “Lucas approach”, it is important to measure labour input in terms of both the volume of hours worked and the skill level of the workers. Efforts to compute measures of composition-adjusted labour input have shown a clear and substantial impact of adding this skill dimension (Nomura and Akashi, 2017; Korhonen, 2020; ABS, 2021). Therefore, measurement efforts in this direction, including both at the national and international level, are encouraged. Furthermore, when thinking about the human capital as an engine for innovation and technological diffusion, as opposed to a factor input, the proxy used for human capital is consequential. Proxies used in the literature include literacy (Romer, 1989), average years of schooling (Benhibab and Spiegel, 2002; Coe et al., 2009; Maudos et al., 2003), educational attainment (Vandenbussche et al., 2006; Park, 2012), PISA scores (Égert, et al., 2022) and shares of different types of workers in firms (Andretta, Brunetti, and Rosso, 2021).
The empirical literature highlights that human capital plays a positive role in determining MFP at the aggregate level (Benhibab and Spiegel, 2002; Maudos et al., 2003; Vandenbussche et al., 2006; Park, 2012). Human capital has also been shown to play a complementary role alongside with other determinants of MFP, including trade openness (Miller and Upadhyay, 2000) and R&D (Coe et al., 2009), both highlighting the importance of a sufficiently qualified labour force in the adoption and diffusion of new technologies. These conclusions have been reinforced at the firm level by a number of studies using data identifying specific types of workers (Andretta, Brunetti, and Rosso, 2021; Criscuolo et al., 2021). Recent OECD work on the human side of productivity highlights clearly the importance of workforce composition in terms of skills, management and diversity (Criscuolo et al., 2021).
Some empirical work has addressed the connection between human capital and MFP in Asian economies, but this is generally either relatively old (Park, 2012) or relatively narrow (Lui and Bi, 2019). A review of recent literature on the intersection between human capital and productivity in Asian economies reveals that most studies address the relationship between human capital and labour productivity, not making the connection or exploring the role of the former as a driver of MFP (Vandenberg and Trinh, 2016; Dua and Garg, 2019). Further analysis and updated evidence on the links between human capital and MFP growth in these economies would be an asset.
This section covers the comprehensive measurement of labour input, the role of human capital in technological innovation and diffusion, and the importance of three components of workforce composition: skills, management and diversity.
Human capital can be measured in a number of ways
There have been a number of approaches to the measurement of human capital in the context of its impact on MFP growth. Romer (1989) outlined a theoretical framework for thinking about the role of human capital in a model of endogenous growth. He relied on level of literacy as the measure of human capital (measured as percentage of the population that can read and write), coming primarily from UNESCOs annual statistical yearbooks. He also referred to the utility of using higher-level measures such as the number of college graduates or the number of scientists and engineers. Another commonly used measure, at least in the earlier literature, is average years of schooling (Benhibab and Spiegel, 2002; Coe et al., 2009), or average years of schooling of the occupied population (Maudos et al., 2003), often using data from Barro and Lee (1993; 1996; 2001; 2013).
Human capital can also be measured by educational attainment (Vandenbussche et al., 2006; Park, 2012), which comes with some intuitive advantages. Measures of years of schooling may lack accuracy in terms of the knowledge and skills actually transferred to the student. For example, students may revisit grades/years of school or university, increasing their years of schooling, but not representing a higher level of educational attainment. Furthermore, there are likely to be differences across countries in the length of schooling (e.g. three versus four-year bachelor’s degrees) and differences in the split between general and vocational education. However, measures of educational attainment are not perfect, for example, failing to account for notable heterogeneity in the quality of a given level of schooling across countries – which may be better accounted for by internationally standardised tests such as the OECD's Programme for International Student Assessment (PISA) (Égert, et al., 2022). Such measures also fail to account for potential skills mismatches, with a high level of educational attainment or ability not necessarily being applied to a relevant occupation.
Further studies have accounted for such mismatches by taking a micro perspective, approaching the contributions of human capital by studying the impact of directors, white-collar workers and skilled or more experienced managers (e.g. Andretta, Brunetti, and Rosso, 2021). In a similar direction, OECD work on the human side of productivity uses cross-country micro-aggregated linked employer-employee data to better understand the importance of workforce composition in terms of skills, management and diversity (Criscuolo et al., 2021).
The way human capital is measured has been found to be important to the significance of results in some studies. For example, in an empirical study of productivity in the United Kingdom and selected European countries, human capital was found to be a significant driver of productivity developments when using a measure of human capital stock, but not when using a measure based on mean years of schooling (Kim et al., 2020).
It is important to account for both the volume and composition of labour input
Traditional measures of labour input, such as employment or hours worked, account only for the volume of labour. These measures treat the labour input of all workers equally, ignoring heterogeneity among workers with potentially vastly different skills and different contributions to output and productivity changes. Indeed, workers with different skills are not interchangeable and firms treat them as distinct inputs by paying different rates.
The need to account for not only the volume of hours worked, but also the skills and characteristics of the workforce was laid out in the Measuring Productivity OECD Manual (OECD, 2001), and subsequently in the System of National Accounts 2008 (2008 SNA). APO-OECD (2021) provides an outline of the literature that computes CALI measures, the estimation method, the measurement challenges and the results for a number of APO and OECD member economies.
Measuring labour input through only the volume of hours worked foregoes some of the explanatory power of one of the factor inputs, often resulting in the underestimation of the contribution of labour to real output growth and the overestimation of growth in multifactor productivity (MFP). To overcome this measurement challenge, many national statistics offices (NSOs) and international organisations produce estimates of composition adjusted labour input (CALI) or labour services, also commonly referred to as quality adjusted labour input (QALI) measures, accounting for both the volume and composition of labour. Such measures provide policy makers and analysts with a more accurate view of the sources of economic growth and more accurate measures of MFP.
While the Asia QALI Database, laid out in Nomura and Akashi (2017), is the most comprehensive attempt to estimate an adjusted labour input measure for Asian economies, it is not the only source for this type of data for these economies. For example, the Japan Industrial Productivity Database (JIP) also estimates a measure of CALI for Japan using data from both household surveys such as the population census, the Employment Status Survey, the labour force survey, and establishment surveys such as the Establishment and Enterprise Census, the Monthly Labour Survey, and the Basic Survey on Wage Structure, cross-classifying workers by education, age, sex and employment status (Fukao et al., 2007).
Measuring labour input purely through the volume of hours worked is a significant underestimate in the case of South Asia (Nomura and Akashi, 2017). Between 1970 and 2015 labour quality growth ranges from 0.7% per year for Bangladesh to 1.9% in Nepal. Changes in labour composition explained between 27% and 46% of labour input growth in the economies in question, implying that MFP growth had been previously overestimated, with downward revisions of 0.4 to 1.1 percentage points per year when accounting for changes in labour composition in addition to those in the volume of hours worked. The sources of change in labour composition vary by country, with the initial level of education and of female participation being important factors on the supply-side and changes to the industrial structure being an important demand-side component.
Such results are also found in developed economies. Since the 2008 economic downturn, labour productivity growth in the United Kingdom has been sustained largely by labour composition, with capital shallowing taking place and MFP growth experiencing a downwards level shift (Korhonen, 2020). This trend is likely driven by the shedding of lower-skilled labour during the downturn and its aftermath. In this case, without accounting explicitly for both the volume and composition of labour, estimates of MFP calculated as a residual would be distorted (i.e. measured MFP would decline less than actual MFP). Similarly, estimates presented by Australian Bureau of Statistics (ABS, 2021) indicate that composition adjusting hours worked reduces measured MFP, at least over the 2020-2021 period for the market sector (from 0.2% per year down to minus 0.2%).
Human capital acts as an engine for technological innovation and diffusion
Some of the benefits associated with improvements in the stock of human capital through education, training, experience and other avenues for the accumulation of skills and knowledge will be captured in estimates of labour services, i.e. through the inclusion of labour composition directly in the production function.
However, the level of human capital may also spill over directly into MFP growth, for example, through a greater propensity for innovation or greater capacity for technological adoption. In a seminal paper, Nelson and Phelps (1966) hypothesised that in a technologically advanced economy, production management requires adaptation to change, with more educated managers being able to introduce new production techniques more quickly and accelerate the process of technological diffusion. MFP reflects both innovation at the technological frontier and catching-up with the frontier, and human capital plays a positive role in both cases. From Nelson and Phelps’ (1966) perspective, the “straightforward insertion of some index of educational attainment in the production function may constitute a gross misspecification of the relation between education and the dynamics of production” because human capital contributes to MFP growth.
Benhibab and Spiegel (2002) generalised the Nelson-Phelps catch-up model of technology diffusion and found that human capital plays a positive role in the determination of MFP growth through its influence on the rate of catch-up. Using a panel dataset with about 80 countries between 1960 and 1995, they estimated that the minimum initial level of human capital required for catch-up in MFP growth with the United States was 1.78 years of schooling in 1960. The results showed that over the period of the analysis, 22 of the 27 countries falling below this threshold in 1960 did exhibit slower MFP growth over the period.
Using similar data sources, Maudos et al. (2003) confirmed that a higher level of human capital has both raised labour productivity and positively impacted the rate of technical change. On average, their findings indicate that a doubling in a countries’ initial human capital endowment would result in a 20% increase in MFP accumulated over the period. This has worked against productivity convergence, as richer countries, with generally higher human capital endowments, have experienced greater rates of technical change.
The aforementioned studies treat only total human capital, ignoring heterogeneity in the quality or type of schooling. This is especially concerning in the case of advanced economies closer to the technological frontier, where skilled labour is likely to be more important (for innovation) than other labour (for imitation/diffusion) for MFP growth. Vandenbussche et al. (2006) aimed to rectify this omission using a panel of OECD countries, including the distribution of the population across schooling attainment levels. Their results indicate that MFP growth is increasing with the fraction of adults with tertiary education and that these high-skilled workers are even more important for countries closer to the frontier.
The potential for productivity gains are much greater from improvements in the quality than quantity component of human capital. Égert et al. (2022) calculated a new macroeconomic measure of quality of human capital, the cohort-weighted average of past Programme for International Student Assessment (PISA) scores of the working age population. They compare this with the corresponding mean years of schooling, which represents the quantity of education. To close the quality gap between the median OECD country and the top three performers, they found that a sustained increase in PISA test scores of 5.1% would be required, resulting in an estimated 3.4-4.1% increase in MFP in the long run. On the other hand, they found that a sustained increase in mean years of schooling of 9.3% would be required to close the quantity gap, with a lower estimated increase in long run MFP of 1.8-2.2%. .
Human capital should also be considered not only for its direct impact on MFP growth, but in terms of its interactions and complementarity with other determinants of MFP. The impact of human capital has been found to move from negative to positive grounds when the country moves from low to higher levels of trade openness (Miller and Upadhyay, 2000). This suggests that investment in human capital is more worthwhile, in productivity terms, when that human capital can engage in the absorption and diffusion of new technologies from abroad, especially in the case of smaller economies. Similarly, some studies emphasised the role of human capital to fully reap the benefits that new technologies and innovation efforts have on MFP growth (Coe et al., 2009).
Focussing in on twelve high-growth Asian economies, Park (2012) found that human capital, proxied by educational attainment, was a significant source of MFP growth, with the contribution to MFP growth gradually rising in Hong Kong (China), Korea and Singapore in the most recent decade, but stagnating or weakening for other Asian economies. Lui and Bi (2019) analysed the heterogeneous and spatial effect of higher education on the regional MFP growth across provinces in China, finding that different levels of higher education have significant effects on MFP growth and that the spatial spillover effects play an important role (i.e. changes in level of education in one province affects MFP growth in neighbouring provinces). Specifically, the results point to strong positive effects of doctoral education, smaller positive effects of bachelor’s education and negative effects for technical schooling and master’s education.
Firm-level evidence reinforces the connection between skills and productivity
While the previous literature focused on the importance of human capital for productivity at the level of the whole economy, some of the latest literature has taken a granular approach using firm-level data. Using cross-country micro-aggregated linked employer-employee data for ten OECD countries from 2000, Criscuolo et al. (2021) determine that differences in skills, management and diversity account for around one third of the productivity gap between frontier firms (top 10% of the productivity distribution) and medium performers (40-60 percentile).
The skill composition of a firm’s workforce is an essential component of its human and organisational capital and a key driver of productivity performance. The use and diffusion of advanced technologies increasingly relies on high-skill workers, with routine tasks being largely absorbed by capital (i.e. computers, software, robots), leaving more room for non-routine creative tasks that require higher skills (Autor, 2014). In this direction, many countries have seen a polarisation of labour demand, with shifts away from occupations performing predominantly routine tasks, which are more readily automated or streamlined, and towards occupations engaged in non-routine cognitively intensive tasks (Autor, Levy and Murnane, 2003; Goos, Manning and Salomons, 2014; Acemoglu and Restrepo, 2019).
Using an occupation-based measure of skills, Criscuolo et al. (2021) found that more productive firms employ a more skill intensive workforce. High-skilled employees account for about a third of the workforce in high-productivity firms, about twice that of the least productive firms, indicating a significant role for high-skilled employees in high productivity performance. That being said, their results make clear that low and medium skilled employees remain indispensable, even to the most productive firms, where the vast majority of employees are low or medium skill. Furthermore, upskilling cannot solve all firms’ productivity challenges. Indeed, more productive firms also differ from less productive firms in that they tend to perform more complex tasks. Therefore, upskilling may require more comprehensive changes than simply employing more high-skill workers, requiring firms to change both what activities they engage in and how they carry out those activities, as well as who is doing them.
The balance of high, medium and low skilled workers in medium and high productivity firms varies markedly across sectors. On average high skilled employees appear to be most important in knowledge intensive services (ICT and professional services), while medium and low skilled employees are most important in less knowledge intensive services, or in manufacturing. In particular, frontier firms in different countries show different skill-use strategies. In some countries, frontier firms have relatively more of a high-skill focus than in other countries. For example, French frontier firms have been found to be more reliant on high skilled employees, whereas German frontier firms are relatively less focussed on high skilled employees, relying instead on a mixture of medium and high skilled employees (Criscuolo et al., 2021).
The high-skill intensity of firms at the productivity frontier has increased since 2000. Over the period, most countries for which the information was available saw an increase in the high-skill gap2 (by 0.3 percentage point per year) while the share of medium and low-skilled employees declined (by 0.2 and 0.1 percentage point per year, respectively) (Criscuolo et al., 2021). It is possible that this reflects the increasing use of advanced and often digital technologies since 2000, requiring or complementary to high-skilled employees. There has also been a broad-based improvement in educational attainment in most countries since 2000, which has started trickling through to the workforce as those students reach prime working age. Finally, the rising concentration of high skilled employees could indicate a shift for frontier firms towards off-shoring or domestic outsourcing of less profitable tasks, while focusing on the most profitable and generally more skill intensive tasks.
Approaching the frontier requires firms to utilise both general skills, measured by educational attainment for example, as well as more specific skills. Firms that are more productive stand out in terms of their use of specific skills, especially ICT skills and management and communications skills. Criscuolo et al. (2021) show large gaps in the use of specific skills along the productivity distribution, with those firms further from the frontier displaying systematically lower utilisation of these skills. More than general skills, specific skills tend to develop more through experience as employees learn-by-doing and through training throughout their career. This highlights the importance of policies that encourage continued professional development, through training and other means, as well as investment in the education system.
Further work from the OECD investigates the relationship between human capital and productivity for Italian firms (Andretta, Brunetti, and Rosso, 2021). This work investigates whether and how worker composition, ownership and management affect the productivity in a sample of Italian limited liability and partnership firms. It shows that higher shares of skilled workers within firms and more experienced managers are associated to higher productivity levels, and firms run by managers with higher education are more likely to introduce innovation. A higher share of directors was found to be correlated with higher level of MFP of around 42% for the whole sample, with the impact being significant only for services firms. A higher share of white-collar workers was found to be correlated with higher MFP, and was significant for both services and manufacturing firms.
Management plays a key role in firm productivity
There has been much discussion in the productivity literature on the importance of management, managerial quality and management practices in the explanation of large differences in productivity performance across firms within narrowly defined sectors. Bloom and Van Reenen (2007) made a first attempt at measuring management practices, drawing on survey data for seven hundred medium-sized firms in the United States, France, Germany, and the United Kingdom. Their rubric to score management practices included questions covering operations (e.g. what kind of lean manufacturing processes have you adopted?), monitoring (e.g. what key performance indicators do you use in performance tracking?), targets (e.g. what types of targets are set for your company?) and incentives (e.g. how does your bonus system work?). Their results indicate that a higher management score is positively and significantly correlated with the long-run component of MFP, with their management practice score also explaining between 10% and 23% of the interquartile range in productivity. This result is confirmed by more recent literature, such as Bloom et al. (2018), who using a larger sample of US manufacturing plants found that management practices account for around 20% of the cross-firm productivity spread, at least as large as that accounted for by either R&D or ICT.
The important role of management in firm productivity differences is also acknowledged in Criscuolo et al. (2021). Managers play an important role in a firm, making key operational decisions and acting as either enablers or bottlenecks to a firm achieving its goals. Their results show that firms at the productivity frontier employ a significantly higher share of managers compared with the average firm and with the laggards. While this holds across sectors, manager shares were found to be more uniform in manufacturing, with larger gaps between frontier firms and medium firms in services and even more so in knowledge intensive services. They found that if the average services firm closed the management share gap with the frontier they would stand to gain 3.5-4.5% in productivity terms, compared with around 1% in the manufacturing sector.
Productivity gains are found to vary substantially across countries. Firms in the United States were on average better managed than their European counterparts (Bloom and Van Reenen, 2007). Criscuolo et al. (2021) also found large variations in productivity gains across their sample of countries, showing almost no potential gain in Sweden and an 8% gain in France. These differences reflect either differences in the size of the gap between medium and frontier firms, and/or relative differences in the strength of the connection between manager share and productivity.
However, the largest differences existed between firms within countries, with a long tail of very badly managed firms. Their explanation for such differences in management practices, across countries and between firms are two-fold. First, low product-market competition may limit the incentives to adopt improved management strategies. Second, family-owned firms handing down management control via primogeniture, which on average leads to worse management practices. These two factors alone explained around half of the long tail of badly managed firms and between one half (France) and one third (United Kingdom) of the management gap between Europe and the United states. Later studies have since found evidence of two causal drivers of improved management practices in the United States (Bloom et al., 2018). Regulation of the business environment (as measured by the Right-to-Work laws) boosts management practices associated with incentives, while learning spillovers as measured by the arrival of large new entrants increases the management scores of incumbents.
Apart from the managerial share of a firm, the quality of those managers in terms of skills is also shown to be important. Criscuolo et al. (2021) indicated that the productivity gains from upskilling managers are up to three times larger than upskilling workers. This likely reflects the outsized role of managers in influencing firm efficiency and performance, for example, through the implementation of improved management practices. The potential productivity gains are also heterogeneous across sectors, with the greatest gains from upskilling managers accruing to less knowledge intensive services where the managerial and worker skill-gap to the frontier is larger.
A more diverse workforce is generally a more productive one
The literature also points to diversity as an essential human driver of productivity performance. A more diverse workforce can bring with it a more comprehensive perspective, a wider range of ideas, and better decision making, positioning such firms particularly well to exploit new business opportunities and improve productivity (Woolley et al., 2010; Parrotta, Pozzoli and Pytlikova, 2012). Criscuolo et al. (2021) focused on three dimensions of diversity for firm productivity: gender, cultural and age.
Firms that employ more gender diverse managers and workers were found to be more productive. The evidence suggests an inverted U-shape relationship between the share of women in a firm and firm productivity, peaking at a 40% share with a productivity premium of around 3% for managers and 2% for workers (Criscuolo et al., 2021). It is also worth noting that these measured productivity gains may be limited by the challenges women face in terms of career progression, especially when it comes to career breaks during and after pregnancy. As the playing field is further levelled with respect to parental leave, one might expect the losses in human capital from career breaks to be lessened and the productivity gains to gender diversity to be even greater. Furthermore, Criscuolo et al. (2021) identified an uneven distribution of productivity gains to gender diversity across sectors. While the gains for managers were similar (around 2.5%), gender diversity was associated with lower productivity gains in manufacturing and higher gains in services.
Firms that are more culturally diverse, as measured by the share of employees with a foreign cultural background (different country of birth or nationality), were also found to be more productive. Again, following an inverted U-shape, firms employing 5-10% of managers with a foreign cultural background were found to be around 7% more productive than those employing less than 5% (Criscuolo et al., 2021). This inverted U-shape means that firms employing very high shares of managers with a foreign cultural background are less productive than those employing an intermediate level, likely reflecting the trade-off between gaining a wider perspective and potential communications costs. The results indicated that the productivity premium associated with employing more culturally diverse workers is much smaller, possibly highlighting that the gains to diversity come principally through better decision making and information gathering. The productivity gains from diversity may also reflect greater integration of firms into the global economy. This argument works in both directions, as a more diverse workforce may help firms to integrate further into global value chains, while firms that are already well integrated may be more likely to hire a more diverse workforce.
Recent OECD work points to employee’s age diversity as another dimension that can impact firm productivity performance (OECD, 2020). This highlights strong productivity gains from employing older managers and from employing a CEO who is older relative to the rest of the workforce. This implies a crucial role for managerial experience, which builds on a set of skills that may take many years to develop. Firms were also found to benefit from employing a combination of different age groups, with younger employees performing better in the presence of a higher share of older employees and vice versa. These productivity gains likely stem from the ability to better leverage the knowledge of more experienced workers to improve the specific skills of younger workers, either passively or more directly through training or shadowing. In light of the widespread shift towards an ageing population, these findings confirm that this shift does not necessarily damage productivity performance and could in fact yield benefits for firms that embrace a more age diverse workforce. Similar results for Italy reinforce this finding, indicating that compared with middle-aged managers (40-59 years old), older managers (60+ years old) were associated with higher MFP levels, while younger managers were associated with lower MFP levels (Andretta, Brunetti and Rosso, 2021).
Public infrastructure
Public infrastructure commonly refers to a network of elements and systems that provide critical support to the functioning of our economies: “the basic systems that bridge distance and bring productive inputs together” (Cisneros, 2010). Regardless of whether they are owned and/or managed by the government sector or private actors, these elements and systems are essential to enable, sustain, or enhance economic activities and, more broadly, societal living conditions: hence the reference to “public” infrastructure or capital. As such, public infrastructure can act as a major catalyst for economic and productivity growth by facilitating an environment for enterprises to flourish and ensuring equal access to services to all citizens. Well maintained roads and efficient land and air freight networks are key to the logistics of firms and consumers, while the utilities, including broadband networks, are a necessity for firms’ functioning while facilitating market access to consumers.
These elements or systems are often subject to a market failure whereby these goods are not sufficiently provided by the private sector, as the latter does not consider the positive externalities that these investments bring to the economy as a whole (Fourie, 2006; IMF, 2015). In addition, their provision may may require large economies of scale, with the need to allow a monopolist to engage in the entirety of its production (Aschauer, 1989). For this reason, the government sector engages, participates or oversees the provision and maintenance of some forms of infrastructure, though engagement varies from one country to another.
A major issue is the absence of a universally accepted definition of public infrastructure. While this typically includes publicly-owned capital, the range of assets covered and their scope varies significantly across studies. Recent studies have broken down infrastructure into different categories, including basic or core economic infrastructure (roads, airports, electric utilities, etc.) and social infrastructure (such as schools or hospitals) (IMF, 2019). In addition, digitalisation is leading analysts to expand the concept further to consider the digital infrastructure, i.e. assets that enable the storage and exchange of data (Bennet et al., 2020). The lack of a common definition of public infrastructure complicates its measurement and undermines an accurate assessment of its impact on productivity. Nonetheless, national accounts can be used as a starting point to construct time series estimates of infrastructure and build a common sense view of its coverage.
Most studies found a positive long-term relationship between public infrastructure and productivity, with “core” infrastructure (i.e. transport infrastructure and utilities) typically playing a larger role than other types of infrastructure (e.g. educational and health care buildings) (Aschauer, 1989; Baltagi and Pinnoi, 1995; Shioji, 2001; Bom and Linghart, 2014). However, there is much less agreement on the magnitude of its impact, which largerly depends on model specifications choices and estimation techniques (Bom and Linghart, 2014). The most important concern is the direction of causality between public capital and productivity: while public capital may affect productivity, productivity can also shape the demand for and supply of public capital services, which is likely to cause an upward bias in the estimated returns to public capital if this reverse causality is not addressed (Romp and de Haan, 2007). Indeed, the use of estimation techniques to control for this reverse causality in more recent studies have proved to reduce the magnitude of effect of public capital on productivity as compared with early studies.
In addition to the estimation framework, quality considerations, non-linearities or threshold effects, network or spillover effects, financing and governance have proved to shape the impact of public infrastructure on productivity. The use of physical measures as opposed to monetary values of public infrastructure should be preferred as a way to capture quality characteristics. In addition, diminishing returns of investment in public infrastructure require accounting for the stock of public infrastructure in order to capture non-linearities or threshold effects in its impact on productivity. Further, local public capital projects may affect factor prices, production and demand within and outside of the project area pointing to the need to consider spillover effects across industries, regions, and countries.
In addition, poor governance has been identified is a major reason why infrastructure projects fail to meet their timeframe, budget, and service delivery objectives (OECD, 2017). Substantial benefits can be realised by better governance of public infrastructure (section covering Institutions). Economies with stronger governance at different stages of public investment management (planning, allocation, and implementation) appear to enjoy a positive output effect from public investment, while economies with weaker governance see output responses that are either not significant or even negative (Schwartz et al., 2020).
This section reviews empirical findings on the role of public infrastructure on productivity and provide some guidance on important aspects affecting this relationship, pointing readers to both technical and analytical considerations at the time of evaluating the results.
Measuring public infrastructure is still challenging
Defining and measuring public capital brings with it many challenges. First, the empirical definition of public infrastructure, also referred in many articles as public capital, differs across the literature. Second, there is support in the literature to account for both the stock of and new additions to (i.e. investment in) public infastructure.
There is no universally accepted definition nor a single measure of public infrastructure
There exists no commonly accepted definition and hence no single measure of public infrastructure. Indeed, infrastructure is not separately identified in national accounts or in any other internationally agreed set of statistics. One possible reason for this is that the boundaries of infrastructure are difficult to identify, vary across countries and can often be imprecise or subjective. While some studies analysing the linkages between public infrastructure and productivity limited themselves to account for transport infrastructure (Fernald, 1999; Deng, 2013; Melo et al., 2013), others included also water and sewer management systems (Égert et al., 2009) and other public buildings and structures (Baltagi and Pinnoi, 1995; Destefanis and Sena, 2005; Baldwin and Dixon, 2008).
In this context, several definitions have been put forward. Martin (2019) provided a first cross-country comparison of “economic infrastructure” across four major European countries. For this, he adopted a simple functional approach whereby he identified six types of physical capital assets that are essential to the provision of transport, energy, water, waste, communications, flood defence services. However, this definition excluded housing and social infrastructure (such as the education and health systems). Indeed, the IMF (2019) and van de Ven (2021) advocated to measure both “economic infrastructure” (also referred as “basic” or “core” infrastructure) and “social infrastructure”, where the latter includes education, health, public safety, culture and recreation related infrastructure. Bennett et al., (2020) extended further this definition to include a third category, i.e. digital infrastructure, although they recognised that its delineation and measurement is still challenging, both because much of it represents new and evolving technologies and because national statistics are not sufficiently granular to identify all assets of interest.
The lack of consensus on the definition of infrastructure leaves room for different compositions of public infrastructure indicators used in the literature, driving some inconsistency across the associated results. Indeed, some studies claimed that economic infrastructure is expected to have a stronger impact on economic growth and productivity than other components of public capital such as hospitals, education buildings and other public buildings (Melo et al., 2013; Bom and Ligthart, 2014). Increased granularity of the measures of infrastructure, and therefore more in-depth analysis of its impact on productivity, can be achieved using detailed gross fixed capital formation (GFCF) and net capital stock series available in countries’ national accounts (Baldwin and Dixon, 2008). While these are not uniformly available across countries, they may be a starting point towards a sense view of the state of infrastructure across different economies.
Accounting for net capital stocks
There is conceptual and empirical support in the literature to use measures of net capital stock and not only the inflow of investment into forming new infrastructure (Hurlin, 2006; Romp and de Haan, 2007; Deng, 2013; Candelon et al., 2013; Melo et al., 2013). Indeed, productive (capital) services are generated by the stock of infrastructure as a whole, this is both the existing stock as well as new investments (Grice, 2016, OECD, 2009). Besides, estimates of the output elasticity of investment in infrastructure may also differ across countries, industries and regions, as a result of differences in the existing stock of infrastructure. Indeed, when a minimum network of assets is available, the marginal productivity of infrastructure investment tend to be greater than the productivity of other investments (Égert et al., 2009; Candelon et al., 2013). For example, as transport networks develop and become larger, the marginal effect of new additions (i.e. investment) may become gradually smaller, that is, there may be scope for diminishing returns to transport investment (Melo et al., 2013).
It is important to note that the net capital stocks of infrastructure are typically estimated as the sum of past investments adjusted for the retirement of assets and depreciation. This brings with it the need to rely on long time series of investment volumes ideally broken down by detailed asset type, which may not be available for many economies and regions of interest (Grice, 2016; APO-OECD, 2021). In addition, there is a need to make assumptions about depreciation and retirement, i.e. the outflow due to the fact that wear and tear or obsolescence reduces the productive services the assets generate. These assumptions are far from trivial when dealing with infrastructure, as there is huge variation in the type of assets covered and therefore on their depreciation rates and retirement patterns. Recent analyses highlighted the need to regularly update and compare countries’ assumptions about depreciation and retirement, as these may have a significant impact on estimates of depreciation, and therefore on a range on net aggregates including net investment and net capital stocks (Bennet et al., 2020; Giandrea et al., 2021; Kornfeld and Fraumeni, 2022; Pionnier et al., forthcoming).
Another important issue when accounting for infrastructure is whether the selected indicator measures infrastructure in monetary terms or physical units (e.g. volumes measures of capital stock or more specific measures such as total length of paved roads, miles of highways per area) (Deng, 2013). Monetary values are easier to collect but may hide a huge heterogeneity in how resources are allocated or have been spent. For example, a new airport or a new high speed rail line may have similar monetary values but can produce very different effects on output and productivity (Melo et al., 2013). Indeed, the monetary cost of a given type of infrastructure may not be a good measure of its productive services (De la Fuente, 2010). On the other hand, physical units such as kilometres of roads are a more homogeneous measure and, more importantly, can better capture the quality of infrastructure.
Quantifying the productivity-infrastructure nexus: the empirical framework matters
Over the last three decades, a large number of studies analysed the role of public infrastructure on long-run economic growth and productivity. Most studies focused on the long-run impact of public infrastructure following the seminal and influential work of Aschauer (1989), which reported a large and significant impact of non-military public capital stock on MFP in the United States over the period 1949-1985. According to Aschauer (1989), the slowdown in MFP growth observed over the period 1970-1985 was associated with a decline in net capital stock of public non-military structures and equipment. Public buildings and structures accounted for about 93% of total public capital and “core infrastructure” (i.e. streets and highways, airports, electrical and gas facilities, mass transit, water systems, and sewers) had greater explanatory power for productivity than public buildings.
This approach relies on a theoretical specification of the production function and regresses aggregate private output on private sector inputs (hours worked and non-residential private fixed capital stock) and public capital stock. Further, rearranging the model specification, one could regress MFP on public capital. The coefficients associated to public capital in these regressions reflect the elasticity of aggregate private output and private sector MFP to public capital. According to Aschauer (1989)’s findings, an 1% increase in the stock of public capital would increase both output and MFP by almost 0.4%. Those results were supported by Munnell (1990).
More recent studies point to a positive impact of public infrastructure on output and productivity, which is lower than suggested in early studies. Gramlich (1994) reckoned that the previous studies were likely to be affected by model misspecification, spurious correlations (i.e. public infrastructure and productivity are associated but not causally related), and reverse causality (i.e. productivity and public capital may influence each other) (Duggal et al., 1999; Fourie, 2006; Romp and de Haan, 2007; Deng, 2013; Melo et al. 2013,). Indeed, subsequent studies noticed that these estimates were fragile, as minor specification changes could produce appreciable shifts in estimated elasticities (Evans and Karras, 1994). In a meta-analysis of 68 studies, Bom and Linghart (2014) found that the output elasticity of public capital could range from -1.7 for New Zealand (Kamps, 2006) to 2.04 for Australia (Otto and Boss, 1994). The characteristics of the study design, in particular, the specification of the models and the choice of control variables, can explain a large part of the heterogeneity of the results across the studies. Another potential factor is the publication bias, as different journals and authors may have a preference towards publishing more or less significant (depending on the subject) results.
Studies relying on the production function approach have one major drawback, which relates with the choice of a specific functional form for the production function and the assumption that labour and both private and government capital are paid according to their marginal productivities (Duggal et al., 1999). Some studies addressed this issue by relying on more flexible approaches that do not require the specification of production function, such a the use of a cost-function approach, i.e. based on the assumption that firms aim to minimise costs or maximise profits (Bonaglia et al., 2000; Demetriades and Mamuneas, 2000; Cohen and Morrison Paul, 2004). However, while these studies agree in that public capital reduces private costs and increase efficiency, there is still much heterogeneity in the results, in particular, in the magnitude of the elasticities (Romp and de Haan, 2007).
Another important problem when estimating the production function relates with the exogeneity assumption of public capital. This assumption rules out any reverse causation or endogeneity bias, i.e. that the causation may also run from output or productivity to public capital. Estimations techniques used in early studies like ordinary least squares (OLS) are the least able to correct for this endogeneity bias. Different empirical strategies have been implemented to tackle this unclear unidirectional relationship in more recent regression analyses. These are the estimation of a system of simultaneous equations (i.e. where two equations, one equation linking productivity to public capital and a second equation linking public capital, are estimated simultaneously), the use of instrumental variables, and/or Granger causality tests (Hurlin, 2006; Romp and de Haan, 2007; Égert et al., 2009; Melo et al., 2013).
Several factors shape the role of public infrastructure on productivity
Many studies have assessed the impact of public capital on output and MFP. However, they differ in the variable used to proxy public capital, model specification, and estimation techniques. Other factors such as the distinction of different types of public capital, quality considerations, non-linearities, network or spillover effects, governance and financing have proved to shape the impact of public infrastructure on productivity, regardless the technical considerations.
The economic benefits from the different types of public infrastructure can vary substantially across assets, with “core” infrastructure typically showing larger explanatory power for productivity growth than other types of infrastructure (Aschauer, 1989; Baltagi and Pinnoi, 1995; Shioji, 2001; Bom and Linghart, 2014). For example, Shioji (2001) found a significant and positive impact on economic growth in Japan and the United States only from “core” infrastructure, as opposed to “education” capital. However, some authors found different effects even across the constituents of “core” infrastructure. Baltagi and Pinnoi (1995) disaggregated the aggregate measure of infrastructure and showed that water and sewer capital consistently provide productive contributions to private productivity across states in the United States, as opposed to highways and streets which effect was found insignificant. Yeaple and Golub (2007) found a stronger impact on MFP growth from road networks in a sample of 18 countries, as opposed to power supply and telecommunications infrastructure. Similarly, Melo et al. (2013) found higher productivity effects for roads as compared with other transport infrastructure such as airports, railways and ports.
Recent studies highlighted the importance of accounting for the quality of public infrastructure (Fourie, 2006; Jiwattanakulpaisarn et al., 2012; Deng, 2013; Moyo, 2013; Bizimana et al., 2021). While the long-run accumulated effects of different types of highways on state output are quite small, expanding interstate highways seems to have a relatively larger effect compared with capacity improvements in other road categories (Jiwattanakulpaisarn et al., 2012). This reflects that the quality of highways (e.g. highway width, average speed) matters for growth performance (Deng, 2013). Similarly, Moyo (2013) found that lower quality in power supply infrastructure in Africa, measured as the number of hours per day without electricity, has a negative and significant impact on MFP growth.
Using physical measures, as opposed to monetary measures, of public infrastructure helps to better capture quality differences (Égert, 2009; Melo et al., 2013). Accounting for the quality of infrastructure allows for the alignment of public infrastructure with individuals and firms’ priorities as well as the mitigation of risks of early obsolescence or locking-in with unsustainable technologies (OECD, 2021). Both the quantity and the quality of electric power, transportation and telecommunications infrastructure have a robust impact on productivity (Bizimana et al., 2021). To account for the quality of infrastructure, they looked at the electricity provision service in 80 countries by measuring the megawatts of electricity transmitted and distributed to consumers in percent of total production; the quality of transportation infrastructure by measuring the share of paved roads of all roads; the quality of the communication infrastructure by measuring the international internet bandwidth per user, bit/s (rescaled to 0-1); the quality of health infrastructure by measuring the access to safely managed water proxied by percent of population using improved water supplies.
The magnitude of the impact of public infrastructure on productivity depends on the development stage of the infrastructure network. The effects of additional investment in public infrastructure on economic activity crucially depend on the available stock of public infrastructure (Fernald, 1999, Hurlin, 2006; Égert et al., 2009; Banister, 2012; Candelon et al., 2013, Deng, 2013, Melo et al., 2013). Fernald (1999) found that massive highway building in the United States triggered MFP growth during the 1950s and 1960s, offering a one-time boost as opposed to a permanent effect on MFP growth: the effects of road building on MFP growth were much smaller one the network was completed. Similarly, Roberts et al. (2012) suggested that the construction of the China’s expressway network caused a one-off level effect but not a permanent growth effect. Destefanis and Sena (2005) found that public infrastructure has a stronger impact in the South of Italy than in the rest of the country, reflecting that public infrastrcture in the South had not achieved yet their “saturation” level. Therefore, the accumulation of public infrastructure (i.e. stock) contributes to productivity growth only when the former is below certain “threshold” levels (Hurlin, 2006; Deng, 2013). .
Some studies explored the relationship between public capital and productivity at the regional level (Mas et al., 1996; Bonaglia et al., 2000; Stephan; 2000; Destefanis and Sena; 2005; Shanks and Barnes, 2008). Indeed, public infrastructure services may have an impact on the economic activity and productivity of the region in which they are located and, additionally, on other regions. These effects has been labeled spillover, spatial or network effects (Cohen, 2010; Deng, 2013; Melo et al., 2013; Elburz and Cubukcu, 2021) and can be positive or negative. Network improvements in the public infrastructure in neighboring regions might lead to a decrease in the production and transportation costs of intermediate and final products for a particular region, which might also translate into an increase in the demand for its goods and services, overall giving rise to positive spillover effects (Arbués et al., 2015). Negative output spillovers can emerge when mobile factors of production migrate to locations with better infrastructure stocks, so that infrastructure-rich locations experience productivity gains at the expense of the places from which factors of production migrated (Boarnet, 1998; Deng, 2013). However, the size of the spillovers will largely depend on the type of infrastructure: for example, some infrastructure may have a small positive impact on a local community but create significant externalities to the country (e.g. radar tower), while another type of infrastructure may only create local benefits, with little impact elsewhere (street light) (Fourie, 2006). This highlights the importance of considering production technologies and factor mobility to accurately measure how local public capital projects may affect factor prices, production and demand within and outside of the project area.
The impact of public infrastructure on productivity also depends on its financing: via taxes, borrowing, or public-private partnerships. Indeed, increases in public spending to maintain or improve public infrastructure may lead to an increase in interest rates or taxes that may curtail private sector spending, the so-called crowding-out effect (Gemmell, 2001). However, very few studies analysing the relationship between public infrastructure and productivity have accounted for the financing side (Romp and de Haan, 2007). Schwellnus and Arnold (2008) evaluated the differential effects of corporate taxes on firms characterised by different levels of profitability and supported the idea that corporate taxes negatively affect productivity at the firm level. Vartia (2008) showed that corporate and top personal income taxes reduce firms’ MFP growth, with a higher negative effect in firms with high corporate profitability since taxes constitute levies on corporate profits. When it comes to debt financing, Agénor and Yilmaz (2017) found that an increase in the share of investment in infrastructure has an ambiguous effect on long-run economic growth (i.e. crowding-out/negative or crowding-in/positive effect). On the one hand, it raises the debt–private capital ratio, which tends to lower economic growth; on the other, it raises the public–private capital ratio, which may lead to an increase in economic growth if the elasticity of output with respect to public capital is sufficiently high. They also showed that the overall impact depends on the stock of public capital, as non-linearities or threshold effects allow for a scenario with both high debt and high growth. Indeed, it is important to consider that countries where the initial level of public capital is low are likely to benefit the most from additional investment, as the latter is likely to have a high risk-adjusted rate of return in these economies (Mourougane et al., 2016).
The literature on the relationship between public infrastructure and productivity is more and more investigating the side-effects that could arise from public infrastructure, such as pollution and environmental damages and social welfare implications. Until recently, most studies have considered outputs (for example, GDP of MFP growth) as a reasonable proxy for outcomes but neglected that side-effects of changes in public capital may lower the benefits for productivity. Statistical efforts to account for output, capital and productivity measures adjusted for the depletion of natural resources and environmental damages (APO-OECD, 2021) must be implemented when assessing public infrastructure effects.
A final note relates with the governance of public infrastructure, i.e. the efficiency of institutions managing public infrastructure (section covering Institutions). Indeed, poor governance has been identified is a major reason why infrastructure projects fail to meet their timeframe, budget, and service delivery objectives (OECD, 2017). Substantial benefits can be realised by better governance of public infrastructure. Economies with stronger governance at different stages of public investment management (planning, allocation, and implementation) appear to enjoy a positive output effect from public investment, while economies with weaker governance see output responses that are either not significant or even negative (Schwartz et al., 2020).
Competition
In the traditional view, competition among businesses can deliver improvements in production efficiency, lower market prices and bring newer and better products to consumers, leading to both productivity gains and increases in consumer welfare. Firms compete to attract customers by offering lower prices, higher quality products or services, a larger variety of products, and/or more innovative goods and services (CMA, 2015). Efficient firms offering the products that the consumers want to buy at the appropriate price will thrive, while inefficient firms will be forced to leave the market or maybe adopt actions to attract customers and improve their productivity.
Digitalisation has changed the business models of firms entering the markets and questioned this vision of competition. Competition, measured by indicators of concentration, mark-ups, and profits, has been weakening in certain industries and economies, in particularly, in the United States and, to a lower extent, in Europe. Most empirical analyses differ in their interpretation and implications for productivity developments. While some authors claim that increased concentration, mark-ups and profits are indicative of greater efficiency and innovation (Autor et al., 2020; Hsieh and Rossi-Hansberg, 2019; Battiati et al., 2021; Bighelli et al., 2021), other studies argue that these trends point to growing market power, strategic increases in barriers to entry, a less dynamic environment, which leads to declining productivity (Gourio et al., 2016; Alon et al. 2018; Calligaris et al., 2018; Gutiérrez and Philippon, 2018; Calvino et al., 2020; Liu et al., 2022). Some authors rule out a relationship between competition measures and productivity (Grullon et al., 2019) and others claim that productivity will initially increase and later decline (Akcigit and Ates, 2021).
This section sheds light on whether and how competition impacts productivity. It reviews the variety of indicators that have been used in the literature to measure the degree of competition and analyses the mechanisms governing the competition-productivity dynamics. The section also investigates the role of regulations and competition policy on competition and productivity and the implications of the rise of “superstar firms” in recent years. While competition has been typically associated with productivity increases, further investigation is needed to understand whether recent increases in concentration and mark-ups can bring aggregate productivity gains. Monitoring a range of indicators of competition is key to better understand these trends.
Competition is a complex notion that is difficult to measure
Competition is not directly observable. As a result, numerous methods, which vary in their complexity and reliability, have been developed to measure the degree of competition. The indicators can be differentiated according to their capacity to capture the characteristics of markets, policy or performance indicators (Kegels and van der Linden, 2011; OECD, 2021).
Market concentration
Market concentration refers to the extent to which the distribution of the market across firms is limited to relatively few firms. Two of the most common measures of concentration are the concentration ratio (CR) (e.g. Haskel, 1991; Hsieh and Rossi-Hansberg, 2019; Tambe et al., 2021) and the Herfindahl-Hirschman index (HHI) (e.g. Grullon et al., 2019; Bighelli et al., 2021). The CR measures the overall market share of the firms with the largest market shares. The N-firm CR measures the market share of the top N firms in the market, so that the CR approaches to zero for an infinite number of equally market sized firms and equals one if large firms included in the calculation make up the entire market. The shares can be computed on total sales or total employment (Bajgar et al., 2019; Autor et al., 2020). By focusing only on the market share of the top N firms, however, the concentration ratio takes no account of the number and market share distribution of the remaining firms. For this reason, the Herfindahl-Hirschman index (HHI) is more appealing. This is calculated as the sum of the squares of the market share of each firm competing in the market. The HHI ranges from 1/N (in the case where all firms have the same market share) to 1 (in the case where one single firm supplies all the market). As such, it is more data intensive than the CR but captures the long-tail of firms with smaller market shares.
The CR and the HHI do not measure competition in a direct way but the structural market outcome (Battiati et al., 2021; OECD, 2021). Besides, these indicators require information on revenues or sales at the firm-level for a reasonable well-defined market, which can be particularly challenging, for example, if imports constitute a significant part of the market (Aghion et al., 2005). Covarrubias et al. (2020) showed that the traditional concentration ratio increased four times more than the import-adjusted concentration ratio, which takes into account share of domestic sales in the total of sales plus imports.
Policy measures of competition
The entry and exit conditions relate to the presence of barriers in the market. According to the theory of contestability of markets, firms behave competitively in the absence of entry and exit barriers. Some studies have looked at entry, exit and/or churning rates as well as changes in the size of the firms to analyse trends in competition (Alon et al., 2018; Calvino et al., 2020). Other studies measure entry barriers through the level or existence of sunk costs. Sunk costs reflect the (substantial) costs that a potential entrant must incur before it can enter, and which may deter the entry of efficient firms preventing quality improvements and price reductions. Most studies analysing the impact of sunk costs on productivity (Aw et al., 2002; Fariñas and Ruano, 2005), follow the work by Sutton (1991) and measure sunk costs as the share of advertising expenditure or capital assets to sales. Increasing investment in intangible assets and its associated sunk costs has brought back the attention to this approach (Haskel and Westlake, 2017).
Indicators of market regulation have also been largely used in the literature to analyse the impact of competition or regulatory reforms on productivity. A measure frequently used is the set of OECD Indicators of Product Market Regulation (PMR indicators). The OECD has been producing these indicators since 1988 in order to measure countries’ regulatory stance and to track progress in regulatory reforms. These indicators are grouped into two different sets. The first one includes economy-wide PMR indicators, which measure the regulatory barriers to firm entry and competition in a broad range of key policy areas, ranging from licensing and public procurement, to governance of state owned enterprises, price controls, evaluation of new and existing regulations, and foreign trade. The second set comprises the sector PMR indicators, which measure the regulatory barriers to firm entry and competition at the level of individual sectors, with a focus on network industries, professional services, and retail distribution.
As shown by the most recent PMR indicators, the stringency of the regulatory environment varies significantly across countries, even within the OECD (Figure 3.1.).
Figure 3.1. OECD Product Market Regulation, overall indicator, economy-wide values, 2018
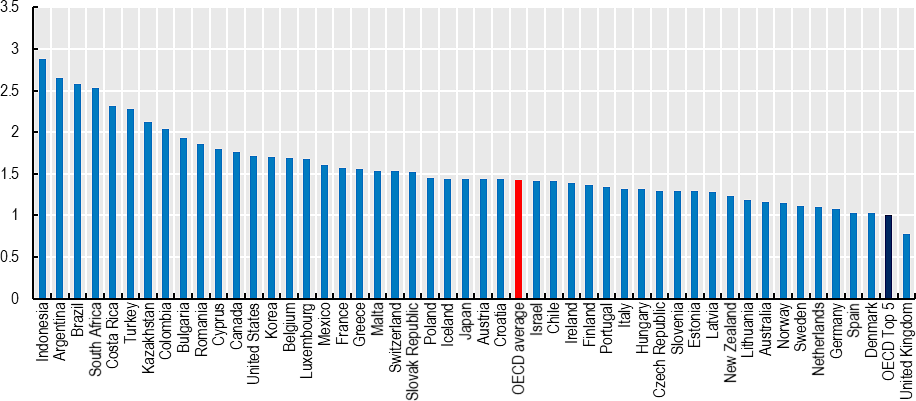
Note: for federal countries, where matters are regulated at state level, the values reflect the situation in one selected representative state. See as follows. Australia: New South Wales; Canada: Ontario; Indonesia: Special Capital Region of Jakarta (DKI Jakarta); Germany: Bavaria; Mexico: Districto Federal de Mexico; Switzerland: Canton of Zurich; United States: New York and Texas.
Source OECD PMR, extracted in May 2022, https://www.oecd.org/economy/reform/indicators-of-product-market-regulation/.
In addition, the OECD Services Trade Restrictiveness Index (STRI), which is released every year since 2014 for 48 countries, includes a sub-component on barriers to competition in 22 services sectors. Barriers to competition include information on anti-trust policy, government ownership of major firms and the extent to which government-owned enterprises enjoy privileges and are exempted from competition laws and regulations. Sector-specific pro-competitive regulation in network industries also falls under this category.. Similarly, the World Bank published for almost two decades the annual Doing Business rankings, which measured and ranked countries according to the regulations constraining and enhancing local businesses activity. However, these indicators have been discontinued and the World Bank is planning to replace it with a new approach to assessing the business and investment climate in economies worldwide: the Business Enabling Environment (BEE) Project.
All these indicators are de jure and translate what is written in the laws and regulations into a composite indicator. While they are likely to provide a fair picture of competition for developed countries, they may not reflect the reality in emerging-market or developing economies, where informality is widespread.
Performance indicators to measure competition
Performance indicators of competition aim to capture the outcome of firms’ behaviour through their mark-ups and profits. These indicators are de facto indicators and, if measured appropriately give a more accurate picture of the degree of competition.
The mark-up is commonly used as an indicator of market power, i.e. firm’s ability to set its prices above marginal costs. As competition increases, firms are forced to reduce their mark-up, the limit being where prices equal marginal costs (i.e. perfect competition). There exist three approaches to measure mark-ups (De Loecker et al., 2020):
The accounting approach defines the mark-up as the ratio of total revenues to total (variable) costs. This implies relying on particularly strong assumptions, including the equality between average and marginal costs, absence of economies of scale and the perfect substitution of factors of production.
The demand approach relies on an estimated demand curve, which requires data on prices and quantities for all products in a pre-specified market, and a particular model of competition.
The production approach uses information from firms’ financial statements and estimates a firm's production function. It allows then to estimate the output elasticity to variable inputs, and to derive the mark-up as the ratio between the elasticity of output to a variable input and the share of revenues the input is paid (Calligaris et al., 2018; De Loecker et al., 2020). Measures of mark-up resulting from this approach can be affected by discrepancies in accounting practices across firms, selection and simultaneity biases, and decisions related to the identification of variable costs. In addition, technology can change over time in a way that creates challenges for the mark-up measure resulting from this approach. For example, firms’ production can increasingly rely on the use of intangibles which costs are likely to be excluded from variable costs (Covarrubias et al., 2020).
Another perfomance indicator commonly used in the literature is a measure of rents or profit rate, which is constructed a measure of profits (output minus costs) normalised to a measure of output (e.g. output, value added, sales) – also known as the Lerner index.
Nickell (1996), Disney et al. (2003); Van Reenen (2011) and Covarrubias et al., (2020) used this indicator, among others, to measure the degree of competition and its impact on productivity performance.
Other approaches to measure competition
A number of alternative measures of competition have also been developed. Nickell (1996) and Disney et al. (2003) examine multiple measures of competition when analysing its impact on productivity, including the concentration ratio, market shares, import penetration3, and a measure of rents4. Nickell (1996) also included a measure of firms’ perception about competition, which is collected through specific questions in business surveys.
Kato (2009) computed an aggregated index of competition indicators using data from Indian manufacturing firms over the 1990s, consisting of a weighted sum of the HHI, the ratio of imports to domestic production for each sector and the firm’s market share in the sector, with the share of sales of the product on the firm’s total sales to account for the variety of products sold by certain firms. Similarly, Buccirossi et al. (2013) derive competition policy indicators at the aggregate level and for specific indicators on institutions, enforcement, antitrust and mergers competition policies for a sample of selected OECD countries. They combined information on institution and enforcement from competition authorities in each of the countries and the European Union that they collected through a tailored questionnaire, with information from the OECD PMR indicators and from the competition authorities’ websites and publications.
Other studies measure competition through the degree of the substitutability of the products, i.e. the higher the substitutability of products, the easier is for consumers to switch between producers. Syverson (2004) followed this approach and investigated the connection between competition and productivity in a case study of the ready-mixed concrete industry in the United States. Since ready-mixed concrete is a physically homogeneous product, the substitutability of products produced by different firms is determined by transport costs and the higher the spatial product substitution possibilities, the higher is competition. Similarly, Covarrubias et al. (2020) used the elasticity of substitution as a way to capture whether consumers have become more sensitive to price and quality changes, which in turn may reflect greater product market competition.
Digitalisation and new business models have altered the link between competition and productivity
Competition has been typically associated with productivity improvements
Competition is found to foster productivity, independently of the indicator of competition used, be it market share (Nickell, 1996; Kato, 2009), mark-up (Crouzet and Eberly, 2021) or more sophisticated measures such as market density (Syverson, 2004) or imported-adjusted concentration (Covarrubias et al., 2020).
The relation also holds when using a policy indicator to proxy competition. Pro-competitive regulations are likely to influence the incentives for new firms to enter a given market and low-productivity firms to exit, as well as for incumbents to engage in experimentation and investments, and the associated reallocation of resources. The opening up of markets and increased competitive pressures, from new domestic and/or foreign competitors, provide both opportunities and incentives for firms to upgrade their capital assets, adopt new technologies and innovate to reach, and possibly push out, frontier production techniques.
The study by Nicoletti and Scarpetta (2003) was pioneer in analysing the impact of market regulations, measured through the OECD PMR indicators, on productivity growth. Conway et al. (2006), Bourlès et al. (2013), Cette et al. (2016), Barseghyan (2008), Arnold et al. (2011), Buccirossi et al. (2013), Lanau and Topolova (2016), Cette et al. (2017), and Anderton et al. (2019) have provided further insights on the impact of the regulatory environment on productivity using the OECD PMR indicators. The World Bank Doing Business rankings have been also used to analyse the relevance of the regulatory environment on productivity growth (Barseghyan, 2008; Dall’ Olio et al., 2013). Other studies have analysed the impact of changes in the competitive environment on productivity by looking the impact of deregulations and liberalisations (Olley and Pakes, 1996; Pavcnik, 2002; Goldberg et al., 2010; Bustos, 2011; Eslava et al., 2013).
A similar diagnostic is found when looking at the sectoral or regional levels (Olley and Pakes, 1996; Schivardi and Viviano, 2010; Haskel and Sadun, 2011; Maican and Orth, 2015; Chesire et al., 2015). Pavcnik (2002) analysed the impact of Chile's large trade liberalisation (i.e. tariff reductions) during the 1970s on the productivity of domestic manufacturing plants. Liberalisation led to an increase in the productivity of the Chilean manufacturing sector, driven by both within-sector productivity increases in the import-competing sectors (those initially having some imports at the time of the liberalisation) and a reallocation of resources from less productive to more productive plants. Since then, many authors analysed the impact of changes in international trade regimes on productivity in other countries and industries, including, just to mention a few, Indonesian manufacturing plants (Amiti and Konings, 2007), Indian manufacturing firms (Goldberg et al., 2010), the Belgium’s textile sector (De Loecker, 2011), Australian manufacturing firms (Palangkaraya and Yong, 2011), and Colombia’s manufacturing establishments (Eslava et al., 2013). All these studies coincide in that trade liberalisation spur productivity via the within-effect and/or the between-effect. Bustos (2011) showed that, in addition, revenues’ increases can induce exporters to invest in new technologies.
Regulations that hinder competition can affect productivity not only in each regulated industry (or market) but also in other industries through intersectoral linkages (Bourlès et al., 2016; Cette et al., 2016; Lanau and Topolova, 2016; Cette et al., 2017). Lack of competitive pressures in a given industry (upstream), which provides intermediate inputs, can generate trickle-down productivity effects on other industries that use those inputs (downstream) by raising the costs, lowering the quality of their products or reducing the availability of intermediate inputs. Indeed, regulations that protect rents in upstream industries (providers of intermediate products) can reduce incentives to implement efficiency improvements in downstream industries (users of those intermediate products), since downstream industry firms will have to share the expected rents from such improvements with upstream industries. These considerations are particularly important, as upstream industries are typically services providers, for which the level of restrictiveness in international trade remains relatively high on average across countries despite recent signs of liberalisations (OECD, 2022).
Competition drives productivity through two key mechanisms (Syverson, 2011; Backus, 2020):
Market selection (also referred as allocative efficiency or between effect or selection effect), by moving the market share towards the more efficient (i.e. lower-cost and generally therefore lower-price) producers and forcing the exit of low-performers;
Efficiency increases (or within effect or treatment effect) inducing firms to take costly productivity-raising actions that they may not take otherwise. More competitive markets may give firms better incentives to monitor managers or invest in productivity enhancements, spur reorganisation of activities and renegotiate contracts, increasing their productivity. Lower monopoly power in firms may also reduce the ability of unions to extract high wages and low effort, hence increasing productivity (Haskel, 1991). Competition can also raise productivity through improvements in management practices (Kato, 2009; Van Reenen, 2011). Management should be seen partly as a transferable technology and competition fosters the adoption of better management practices through both selecting out the badly managed firms and giving incumbent firms stronger incentives to improve their management practices.
Market selection is found to be the main contributor of productivity gains. Gourio et al. (2016) estimated impulse responses to an increase in the number of startups and found that output and MFP are persistently affected by an increase in entry. Decker et al. (2018) found that impaired growth in allocative efficiency can account for the bulk of the productivity slowdown in the United States from the late 1990s to the mid-2000s. Disney et al. (2003) found that entry, exit and reallocation of market shares accounted for 80–90 per cent of MFP growth in establishments in the United Kingdom during the 1980s. According to Alon et al. (2018), declining firm entry and the subsequent aging of the United States’ business sector reduced aggregate labour productivity growth by roughly 0.1 percentage point per year, which equals to a cumulative drag of 3.1% over the entire period of their analysis. Indeed, conditional on surviving, new entrants registered cumulative productivity growth of roughly 20% in the first 5 years of operation but thereafter the productivity profile flattens dramatically, suggesting that fast gains in productivity of young firms are driven by the fact that inefficient entrants lose market share and exit quickly, rather than productivity growth within surviving firms.
There is evidence of a “within effect”, whereby firms facing stronger competition made substantial investments to raise their productivity performance. This can increase industry productivity (Holmes and Schmitz, 2010) or firm productivity. For example, after the entry of Wal-Mart, existing retailers in the United States made new investments in inventory control to better monitor potential stockouts (Matsa, 2011).
Initial conditions can alter the relation between competition and productivity
The economic environment and firm specificity can reinforce the impact of competition on productivity. A decline in the long-term interest rate can trigger a stronger investment response by market leaders relative to market followers, thereby leading to more concentrated markets, higher profits, and lower aggregate productivity growth (Liu et al., 2022). Similarly, firms with high sunk costs are subject to less market selection and may exhibit, on average, lower productivity than low sunk costs firms (Aw et al., 2002; Fariñas and Ruano, 2005).
The level of enforcement of regulations in place appears as an important determinant of the competition-productivity dynamics. Gutierrez and Philippon (2018) found that European markets have lower concentration, lower excess profits, and lower regulatory barriers to entry as compared with the United States, and suggest that this is related to the fact that European countries have delegated the enforcement of pro-competition policies to an EU supranational body, which acts with more independence than it does the American national counterpart. They found that stronger enforcement in Europe is associated with lower concentration, lower profitability and with faster MFP.
The links between the regulatory environment and productivity are also influenced by the level of development of the economy (Acemoglu et al. 2006; Aghion and Howitt, 2006) and the characteristics of both the industries and firms involved (Arnold et al. 2011). Indeed, the country-industry gap with the technological frontier has a positive and significant impact when analysing the link between competitive conditions and productivity enhancements (Nicoletti and Scarpetta, 2003, Conway et al., 2006; Buccirrosi et al., 2013). The larger the distance from the technological frontier, the larger the scope for imitation and adoption of new technologies, although this largely depends on the absorption capacity (e.g. human capital, available infrastructure) (Arnold et al., 2011).
In addition, the prevalence of small firms may hinder the ability for a country to benefit from pro-competitive reforms and liberalisations. The ability of small firms to benefit from knowledge transfers from abroad, economies of scale, and production-reallocation efficiencies may be more limited than for larger firms (Dall’Olio et al., 2013; Anderton et al., 2019).
Digitalisation and the emergence of new business models create challenges to competition, with still uncertain effects on productivity
Digitalisation and the emergence of new business models has questioned the traditional link between competition and productivity. Recent studies exploiting microdata for the United States have shown that recent increases in concentration, mark-ups and profits can be associated to the rise of “superstar firms”, a group of tech giant firms, such as Google, Amazon, Apple, Facebook, Uber and Airbnb, which business models heavily rely on the use of digital tools (Hsieh and Rossi-Hansberg, 2019; Autor et al., 2020; Ganapati, 2021). One possible explanation for the emergence of such “superstar firms” is the existence of an initial managerial and/or technological advantage (Van Reenen, 2018). Initial productivity differences across firms can have consequences on market shares when competition becomes more strenuous, turning leading (highly productive) firms into dominating firms. While the (initial) efficiency gains and innovations brought by these firms is hardly debated (Hsieh and Rossi-Hansberg, 2019; Autor et al., 2020; Covarrubias et al., 2020; Ganapati, 2021), the main question is whether and how they will be sustained over time, now that these firms face lower degrees of competition.
Increases in concentration and mark-ups have been also observed in Europe, though to a lower extent than in the United States (Bajgar et al., 2019) and with a less obvious link to digitalisation. Indeed, Bighelli et al. (2021) observed an increase in the HHI in Europe, driven by the reallocation of the economic activity towards concentrated industries and countries, in particular, to the German manufacturing sector. They argued that this reallocation process has been a strong driver of productivity growth in Europe in the past years. Similarly, Battiati et al. (2021) found a positive correlation between mark-ups and productivity at the country-industry level in selected EU countries, although they ruled out a correlation at the country-level as a whole.
Investments in intangible assets, often related to digital technologies, are likely to play a particularly important role in the emergence of very large firms because they allow economies of scale and network effects (Haskel and Westlake, 2017; Tambe et al., 2021). Indeed, investments in intangible assets like investment in skills training, new decision-making structures within the firm, management, and software customisation involve irrecoverable (sunk) costs that can be difficult to finance for small or less productive firms. Moreover, these firms often produce intangibles, which are easy to combine with other intangibles to create value, generate more spillovers than tangible assets, and can be easily used to scale up operations. A particular example is related to tacit knowledge and big proprietary data, which nowadays play a larger role in the production process (Akcigit and Ates, 2021; above discussion on data). For example, more data can help firms who own them to efficiently expand the customer base, which generates more data that help improve services and attract more customers. All these characteristics allow for large network effects, and leave space for leaders to entrench among themselves and later raise barriers to entry (Covarrubias et al., 2020).
These top firms may become increasingly specialised in a narrow set of industries that represent their primary line of business, while exiting other industries. The net effect is that there is essentially no change in concentration by the top firms in the economy as a whole. However, these firms may have become larger in their industries and unleashed productivity growth in these sectors (Hsieh and Rossi-Hansberg, 2019).
Another plausible story, still coherent with the above, can be that these firms initially gain large market shares by legitimately competing on the merits of their innovations or superior efficiency. Once they have gained a commanding position, however, they may use their market power to erect various barriers to entry to protect their positions. With increased consolidation of activity in their hands, these tech giants may potentially find it easier to defend their “turf”, substantially decreasing the chances for smaller and follower firms to learn from and catch up with them. This leads to the so-called “winner takes most” dynamics. A weakening enforcement of antitrust law, with the application becoming more lenient toward large firms, may contribute to this (Grullon et al., 2019).
Akcigit and Ates (2021) proposed a theoretical framework to explain such a dynamics. Their model shows how aggregate productivity growth in an economy adjusts to a decline in the intensity of knowledge diffusion across firms. At first, there is an increase in market concentration, as followers cannot learn anymore from the leaders (i.e. higher-productivity firms) and leaders have more incentives to compete among themselves for market leadership, leading to a “neck-and-neck” firms’ competition. This exerts a positive force on aggregate growth by stimulating innovation by “neck-and-neck” firms (“incentives effect”). However, as the sectoral composition of the economy shifts to sectors where more innovation (by “neck-and-neck” firms) took place and leading firms dominate the market, aggregate productivity growth would decline, as leading firms have no more incentive to innovate (“composition effect”). As a result, a decline in knowledge diffusion could generate a hump-shaped pattern in aggregate productivity growth over time. In this regard, OECD work pointed that the slowdown in the diffusion of knowledge across firms is among the major causes underlying increases in productivity dispersion across firms, in particular, between frontier firms and the rest (Andrews et al., 2016).
Against this background, competition authorities are leaning towards monitoring different indicators of competition simultaneously. Competition is becoming more difficult to measure and so its impact on productivity. Concentration, market shares, mark-ups, profits (rents), entries and exits, the stringency or changes in regulations, can tell only one piece of the story about the competition dynamics. Covarrubias et al. (2020) showed that the accession of China to the World Trade Organisation (in 2001) was consistent with an increase in exits and lower profit rates for firms in the United States, while their mark-ups if measured with the production approach (i.e. relying on the cost of variable inputs) appeared as rising. In their view, these measures of mark-up fail to capture the increase in intangibles-building costs incurred by firms in the United States as a follow-up of this event.
Another important outcome from the discussion relates with the need to distinguish between “good” and “bad” concentration (Covarrubias et al., 2020). Recent increases in concentration may be indicative of growing oligopolies which may exploit economies of scale, managerial or technical advantages or the returns from investment in substantial amounts of intangible capital. These firms build themselves on technical innovation or scale economies, contributing to productivity increases in their sectors (“good” concentration”). However, these firms may collude and find ways to raise barriers to entry, hence deterring competition (“bad” concentration). It is however difficult to make this distinction in practice, and it is therefore still premature to conclude that these increases in concentration can explain the sluggish aggregate productivity growth over the past years (Van Reenen, 2018).
One aspect is certain. A key characteristic of the “superstar firms” is that they operate globally and may therefore affect competition in many economies in parallel. This stresses the need for competition authorities in all countries to ensure that their policies keep up the pace with these developments, and to censor that current competition laws are well-defined, effectively enforced and timely reformed in order to reap up the benefits for all.
Globalisation
Globalisation generally encompasses international trade and investment in the form of foreign direct investment (FDI). It comprises the international integration of firms in global value chains (GVCs), which have become prevalent in recent years.
Globalisation has supported growth and development, but also profoundly altered the way firms operate and their business models. It can contribute to boost productivity through different channels (Madsen, 2007). Engagement in international trade and foreign direct investment allows firms to access new markets to sell their products but also buy inputs, allowing to maximize the efficiency of their production process and exploit economies of scale (Grossman and Helpman, 1991; Obstfeld and Rogoff, 1996). They are also the source of technology spillovers, through exposure to new production processes, materials, and management practices (Barro and Sala-i-Martin, 1995; Miller and Upadhay, 2000; Madsen, 2007; Dasgupta and Ratha, 2012). Effects may be amplified as engagement in international trade and foreign direct investments are often closely associated (Makoni, 2018; Sahoo, 2006; Zaman et al., 2018). There are potential downsides too. International trade can benefit primarily large firms, increasing the productivity gap. Multinational firms can also attract demand away from domestic firms in developing countries and/or import a high share of their intermediate goods (Herzer, 2017). Technology spillovers may be insignificant due to a low capacity for technology absorption (e.g. due to insufficient human capital), and/or spillovers that may not occur horizontally but vertically.
Looking forward, there are indications that the pace of globalisation is likely to stall if not revert, raising questions on its impact on productivity developments. Trade flows and integration in global value chains started to lose momentum even prior to the start of the COVID-19 pandemic. FDI flows grew by 4% in developing Asian economies in 2020, but the rise was driven by FDI inward flows to certain economies including China, Hong Kong (China), India and United Arab Emirates (UNCTAD, 2021). Many Asian economies that often benefit from FDI in the sectors of tourism and manufacturing were particularly hit due to lockdown measures, supply chain disruptions and economic uncertainty.
The economic literature finds a positive link between engagement in international trade and in global value chains and productivity. These results appear to be robust to the choice of the indicators, though the magnitude of the effect may vary. At the same time, there is a need to improve the quality of globalisation indicators. In particular, indicators of integration in global value chains need to be harmonised across the various initiatives that compute them, be timelier and account for firm heterogeneity.
The evidence on a relationship between FDI and productivity is mixed. The impact of FDI on economic growth and MFP depends on a number of factors including the economic environment, e.g. infrastructure in the host country, local labour market conditions, reliability of communications systems, limitations to capital flows as well as the overall macroeconomic and trade policy climate (Görg and Greenway, 2004), and characteristics of the analysis itself (e.g. cross-country study vs. country case study; use of macro vs. micro data).
This section discusses how engagement in international trade and investment have been captured in the economic literature, before discussing the link between globalisation and productivity.
Measuring engagement in international trade and investment has evolved over the years
Measuring engagement in international trade
Several measures of engagement in international trade have been used in the economic literature to investigate the link between international trade and productivity.
The first group are intensity measures, including standard (gross) flows of imports or exports (Balassa, 1982; Chen, 1999) and trade openness (i.e. sum of exports and imports as a share GDP) (Figure 3.2). A simpler measure relies on the export-to-GDP ratio (Miller and Upadhyay, 2000) or the import-to-GDP ratio (Liargovas and Skandalis, 2012).
Those measures present a number of limitations.
First, they measure do not account for differences in prices between economies and miss important information on their ability to trade. Alcalá and Ciccone (2004) proposed to measure trade openness correcting for the price effect by computing a “real” trade openness ratio, which uses as denominator the GDP expressed in purchasing power parities (PPPs).
Second, those measures may be lower for larger economies all other things equal, where size is measured in terms of population (Égert, 2016, 2017). While in Germany for example the openness of a Länder (state) is comparable to economies such as the Slovak Republic or the Czech Republic, Germany as a whole appears less open as the Länder trade intensively between them. One possible fix put forward by Égert (2016) would be to regress the traditional trade openness measure on country size (proxied by the total population), and use the residuals as a size-adjusted openness measure. An alternative would be to construct a trade openness adjusted for population, using GDP per capita as a denominator (Liargovas and Skandalis, 2012)
Third, those measures do not account for country-specificities. More sophisticated measures correct for outliers in trade data or reflect the geographical structure of trade (Liargovas and Skandalis 2012; Squalli and Wilson, 2009). Frankel (2000) developed a closeness index computed as one minus the ratio of imports plus exports over GDP, divided by two.
A second group of measures encompasses policy indicators. Sachs and Warner (1995) discussed the use of information on the implementation of import quotas or tariffs as proxies for trade openness. A country is considered to have higher trade openness if it maintains low tariffs and high quotas and does not have high black market exchange rate premium.
Since 2014, the OECD has been publishing the services trade restrictiveness index (STRI). It provides information on regulations that affect trade in services in 22 sectors across 48 countries, including all OECD countries and several emerging-market economies. The STRI covers limitations on market access and national treatment, as well as national regulatory and competition policies which apply to both national/resident and foreign/non-resident companies, and investment policies. The policy measures accounted for in the STRI database are organised under five policy areas: restrictions on foreign entry, restrictions on movement of people, other discriminatory measures (including discrimination of foreign services suppliers as far as taxes, subsidies and public procurement), barriers to competition and regulatory transparency.
Figure 3.2. Trade openness ratio
Exports plus imports as a share of GDP, percentage
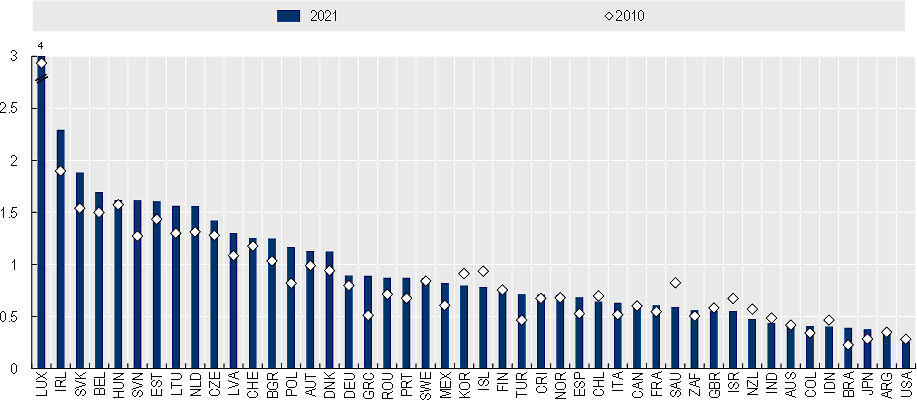
Source: OECD Quarterly National Accounts database, June 2022.
Measuring integration in global value chains
Conventional trade statistics which measure the value of “gross” trade flows (the factory-gate value of a good or service traded) failed to capture the complexity of globalisation, as goods and services today undergo transformations in many different countries, and can sometimes cross borders several times. Trade in value added (TiVA) addresses these limitations and consider the value added by each country in the production of goods and services that are consumed worldwide.
Measures of TIVA have been developed by international organisations and research institutions which have mapped out the global production network by integrating many countries’ Supply and Use Tables (SUTs – which show production linkages within countries) with trade statistics (which show exchanges between countries). Examples include the OECD’s Trade in Value-Added (TiVA) database, ADB’s Multi-Regional Input-Output Tables, Eurostat’s FIGARO and the Groningen University’s World Input-Output Tables. By its very nature, this approach is data intensive and requires a series of adjustments and estimates to set up comprehensive and consistent accounts for global trade and production.
A number of indicators of integration in GVCs are used in the economic literature, the most common are described in Box 3.1. Methodologies often vary across TiVA initiatives, for instance in the way re-exports should be accounted for, explaining possible inconsistencies across initiatives. In addition, indicators are often published with very long delays, reflecting the lack of timeliness of SUTs. Evidence also shows that these indicators fail to capture some forms of heterogeneity. While introducing more granularity and detailed sectoral information does not appear to matter significantly, heterogeneity stemming from different behaviours across firms is found to alter the magnitude of TiVA indicators significantly (OECD, 2022). According to an analysis on Finland, accounting for firm heterogeneity points to a much higher dependency on GVCs in Finland than suggested by the OECD TiVA (2018 version) (OECD and Statistics Finland, 2021). Lack of data availability prevents similar analysis for most countries.
Box 3.1. Most common TiVA indicators
Domestic value added content (or share) of gross exports by industry i to partner region p, represents the exported value added that has been generated anywhere in the domestic economy. This is an “intensity measure” and reflects how much value added, generated anywhere in the domestic economy, is embodied in total gross exports by industry.
The domestic value added content of gross exports can be split further into:
Direct domestic industry value added content, which measures the direct value added contribution made by industry i to the production of goods and services exported by industry i; and
Indirect domestic content, which corresponds to the value added originating from other, upstream, domestic industries (different from industry i) that are incorporated in the exports of industry i.
Foreign value added content (or share) of gross exports captures the value of imported intermediate goods and services that are embodied in a domestic industry’s exports. The value added can come from any foreign industry upstream in the production chain. This is an “intensity measure”, often referred to as “import content of exports” and considered as a measure of “backward linkages” in analyses of GVCs. It reflects how much value added, generated abroad, is embodied in total gross exports by industry.
Domestic services content (or share) of gross exports can be regarded as a sub-component of indirect domestic content of gross exports, but with intermediate inputs coming from upstream domestic services industries only. This indicator is often used to measure services content embodied in manufacturing exports, to capture the rising importance of services integration in manufacturing production and exports.
Measuring foreign direct investment
Foreign direct investment (FDI) is defined as a category of cross-border investment made by a resident in one economy (the direct investor or parent) with the objective of establishing a lasting interest in an enterprise (the direct investment enterprise or affiliate) that is resident in an economy other than that of the direct investor. FDI statistics are typically presented following either the asset/liability principle or the directional principle (inward/outward FDIs).
On an asset/liability basis, which is the approach recommended in the sixth edition of the Balance of Payments and International Investment Position Manual (BPM6), direct investment statistics are organised according to whether the investment relates to an asset or a liability for the country compiling the statistics. For example, a country’s assets include equity investments by parent companies resident in that country in their foreign affiliates because those investments are claims that they have on assets in foreign countries. Similarly, a country’s liabilities include foreign parents’ equity investments in affiliates resident in that country because those investments represent claims that foreigners have on assets in the reporting country.
Under the directional presentation, direct investment flows and positions are organised according to the direction of the investment for the reporting economy - either outward or inward. For a particular country, all flows and positions of direct investors resident in that economy are shown under outward investment and all flows and positions for direct investment enterprises resident in that economy are shown under inward investment. Moreover, FDI statistics can show FDI flows, which reflect trends in activities of transnational corporations, or FDI stocks, which help to analyse the importance of foreign companies in a host country and in the world more generally (Figure 3.3).
In empirical studies, inward and/or outward FDI flows, or a normalised or transformed measure of them, are typically used as a measure of FDI. Most common measures include per capita FDI inflows (Mayoshi et al., 2021), the ratio of a country’s inward and outward FDI flows to its gross capital formation (Zhu and Jeon, 2007). Other studies have favoured the use of FDI stock measures, such as stock of FDI as a share of GDP (Baltabaev, 2014), the real stock of FDI obtained by multiplying the FDI to GDP ratio by GDP in constant prices (Herzer, 2017).
Beugelsdijk et al. (2010) showed that FDI stocks can be a biased measure when the aim is to measure the multinational enterprises (MNE) affiliates activities, as many countries offering low corporate tax rates receive FDI that generate no actual productive activity and as such FDI stocks in such countries overestimate affiliate activity. In addition, FDI stocks do not include locally raised external funds, resulting in an underestimation of affiliate activity in such countries.
Figure 3.3. Foreign direct investment, total inward flows, 2021
As percentage of GDP
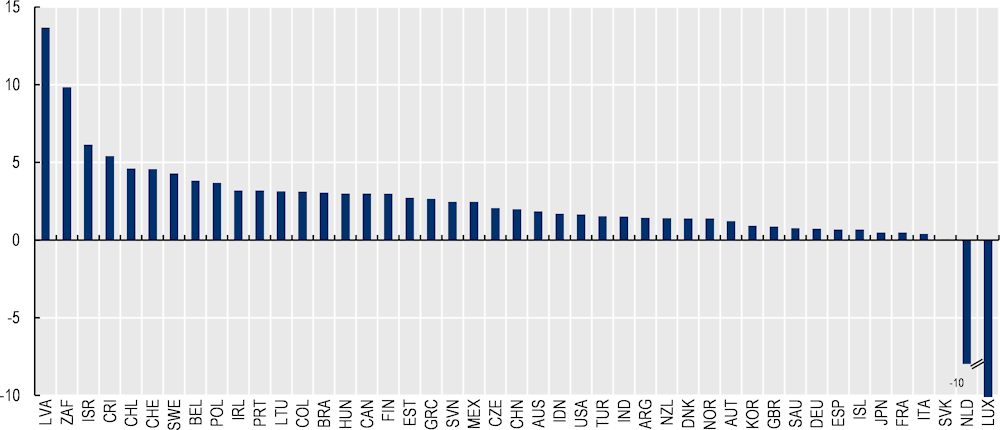
Source: OECD Foreign direct investment statistics, June 2022.
Globalisation is estimated to be a key driver of productivity
The relationship between trade openness and productivity growth varies depending on the stage of development and the sector considered
Greater trade openness is associated with higher MFP growth (Miller and Upadhyay, 2000) or with higher labour productivity (Alcalá and Ciccone, 2004), by enhancing technology adoption and diffusion (Égert, 2017).
The result appears to be robust to the choice of the trade openness measure, whether the latter is captured by a performance indicator (trade openness) or a policy indicator (barriers to trade). While many analyses rely on a standard trade openness measure, the link with productivity holds for size-adjusted trade openness on MFP (Égert, 2017). Turning to policy indicators, trade liberalisation, as measured by a decrease in barriers to trade, is estimated to boost firm performances and economic growth (Aghion and Howitt, 2008). While the use of the existing measures of trade to assess its effect on TFP has no impact on the sign and significance of the results, it changes the magnitude of the coefficient associated to the trade (Abizadeh and Pandey, 2009). The statistical significance of the effect is stronger for alternative measures such as the real trade openness developed by Alcalá and Ciccone (2004) (see above).
The relation also holds when correcting for endogeneity of trade openness and using an instrumental variable approach (Alcalá and Ciccone, 2004).
The effect of openness on multifactor productivity is found to vary with human capital as proxied by average years of schooling (Alcalá and Ciccone, 2004). More qualified workforce is associated with a stronger impact of the degree of openness on trade, underlining the importance of high skilled workforce to benefit from technology spillovers from trade.
Some variations across groups of countries are also observed, depending on their level of development. While size-adjusted trade openness appears associated with higher MFP, for a subgroup of most advanced OECD countries, R&D seems to have a higher impact than trade in less developed OECD economies (Égert, 2017). Reducing barriers to entry to foreign products and firms is found to have a more positive effect on the economic performance of firms in countries which are initially closer to the global technological frontier, exacerbating existing differences in productivity (Aghion et al., 2005 for the United Kingdom, Aghion et al., 2006 for India). In fact, trade liberalisation may have a negative effect on the performance of firms in countries which were initially far from the world technological frontier (i.e. laggard firms get discouraged from making innovations). Consequently, the positive effect of trade openness on MFP growth should be considered with caution as it highly depends on the level of development of the economies, their capacity to support strict trade laws, exchange rate fluctuations as well as many factors known for playing a role in external macroeconomic stability or relating to the quality of institutions.
At the sectoral level, trade openness is estimated to be associated with higher MFP growth in the services sector, but not in the agricultural and industrial sectors in a panel of 20 OECD economies between 1980 and 2000 (Abizadeh and Pandey, 2009). Restrictions to services trade are also found to be negatively to productivity growth in manufacturing (Beverelli et al., 2017).
This suggests that the positive effect of trade participation on aggregate MFP growth is driven by the effect of trade openness and productivity in the services sector.
Integration in global value chains is found to be a key source of productivity
A range of channels have been identified through which linking into GVCs can increase firm productivity. Firms can focus on specialised tasks, in which they enjoy core competencies, while offshoring tasks that they are less efficient in. Productivity gains in a GVC can occur through increased access to new, cheaper, and a greater diversity of input varieties and learning externalities as well as technology spillovers (Baldwin and Robert-Nicoud, 2014). Moreover, participating in a GVC exposes supplier firms to intense foreign competition, creating incentives for them to become more efficient and cost competitive (Chiarvesio et al., 2010). Further, GVC firms can benefit from cost complementarities from two-way trade, resulting in a higher productivity premium for two-way traders (Wagner, 2012).
Empirically the participation in GVCs is associated with productivity gains, and this better performance is found to cumulate over time (Baldwin and Yan, 2014; Formai and Vergara Cafarelli, 2016). In particular, the link between intermediate imports and productivity is found in a number of countries, including France (Bas and Strauss-Kahn, 2014), Chile (Kasahara and Lapham, 2013), Hungary (Halpern et al., 2015) and the United States (Bernard et al, 2007). Recently, the link between GVCs and productivity has also been demonstrated for emerging-market economies such as India (Banga, 2022) or Indonesia, Philippines, and Viet Nam (Urata and Baek, 2021)
The positive relationship between integration in global value chains and productivity growth can also be observed at the sectoral level (Kordalska et al. 2016; Urata and Baek, 2020)). An increase by 10 % in the level of GVC participation is found to associated with a long-term increase in labour productivity of 1.7 percentage points in 13 manufacturing sectors between 1995 and 2009 (Constantinescu et al., 2017). Using a sample of 54 countries and 20 industries over the period 1995-2011, Kummritz (2016) found that a 1 % increase in GVC participation (measured by the domestic value added embodied in foreign countries’ gross exports) is associated with an increase of 0.3% in labour productivity.
Productivity gains have been observed for industries that are highly integrated in GVCs, such as in high tech, research and development and capital goods industries but also others. Consistent with the learning by doing assumption and the hypothesis that imports provide a channel for technology diffusion, productivity growth was higher for GVC firms that trade intermediates with high-wage countries. Engaging in GVCs can also lead to productivity gains through trade with low-wage countries, where potential cost savings are the highest. Looking specifically at Indonesia, Philippines and Viet Nam, there is also evidence that a firm’s GVC participation improves productivity when they are engaged in both importing intermediate goods and exporting output, but that firm’s productivity does not increase when they are engaged in either importing intermediates only, or exporting output only (Urata and Baek, 2021).
Evidence on the link between FDI and productivity is mixed
Despite the potential benefits of FDI on MFP, no clear consensus has emerged in the economic literature regarding the causation of FDI (stock and/or flows) and MFP. While some studies conclude that there is a positive impact of FDI on TFP growth (Woo, 2009; Herzer, 2017), other empirical works suggest that foreign presence involve negative effects on domestic economic growth or productivity (Aitken and Harrison,1999; Azman-Saini et al., 2010a, 2010b, Barry et al., 2005; Heyman et al., 2007; Almeida, 2007; Pittiglio et al., 2015).
One key element explaining the lack of consensus is related to the empirical strategy, and the correction for endogeneity biases. Considering only the causation from FDI to growth, but not vice versa, might introduce a bias in the estimates as FDI may be influenced by higher economic or productivity growth rates (Baltabaev, 2014).
The heterogeneity of the results can also be explained by factors inherent to the scope of the studies, including:
Whether they use macroeconomic (Woo, 2009) or microeconomic data (Aitken and Harrison, 1999). Macroeconomic studies mostly conclude that there exists a positive relationship between FDI and MFP, while microeconomic studies conflict in their results, depending on the industries in which the impact on MFP is studied (Abizadeh and Pandey, 2009);
Whether cross-country analyses (Bitzer and Gorg, 2009) or country-case studies (Aitken and Harrison, 1999; Kinoshita, 2000) are considered. Cross-country studies are not all consistent, as some fail to find a significant effect of FDI on MFP (Herzer, 2012) while others provide evidence on a positive relationship (Bitzer and Gorg, 2009; Baltabev, 2014). Case-studies, which mostly focus on specific industries, such as Aitken and Harrison (1999), find a negative relationship between FDI and productivity, while Herzer (2017) finds a positive impact of FDI on productivity. The industries considered as well as the country as a whole matter for the conclusions.
Differences of scope or methodologies are not sufficient to explain the absence of clear results. A number of analysis also point to the lack of robustness in the relationship between FDI and productivity despite the use of a common approach. Baldwin et al. (2005) use industry-level data for 7 industries in the manufacturing sector and 9 countries over the period 1979-1991 and yield mixed results on the impact of FDI, measured as FDI-linked spillovers, on labour productivity growth of host countries. Herzer (2012) also find mixed results in a sample of developed economies. Looking at the sample of developed, developing and transition economies, Görg and Greenway (2004) failed to find robust evidence of FDI positive spillovers on productivity.
While the use of different FDI measures does not affect the significance nor the sign of the impact of FDI of MFP, it seems to affect the magnitude of the causal effect. However, differences in estimated effects of FDI on MFP are likely more related to differences in empirical strategies and in economic conditions across countries (e.g. developing economies vs. developed ones, the development of the financial system, macroeconomic stability).
The link between FDI and productivity is found to depend on the level of development of the economies. For example, differences in the rate of return to capital may appear and create asymmetries in productivity-enhancing investments in the presence of imperfect financial markets and limitations to capital flows across economies, especially from higher to lower-income countries (Acemoglu, 2008). Consequently, incentives for FDI may be reduced (Banerjee and Duflo, 2005; Chirinko and Mallick, 2007). By contrast, US multinationals contribute to MFP growth in developed countries, but not in developing countries (Xu, 2000).
The impact is also estimated to vary with the size of firms. There is some evidence in the case of industrial plants in Venezuela between 1976 and 1989, that small plants of less than 50 employees do benefit from foreign investment, while for large enterprises, the positive effects of foreign investment disappear when plant-specific differences are taken into account (Aitken and Harrison, 1999).
Financial development
The good functioning of the financial system has been proved to have a positive and significant impact on MFP growth (Beck et al., 2000; Benhabib and Spiegel, 2000; Andrews and Cingano, 2014; Midrigan and Xu, 2014; Adalet McGowan et al., 2017). Indeed, financial frictions, in the form for example of high cost insolvency regimes, may have a negative impact on productivity growth (Heil, 2017).
In addition, financial development has a “complementary” role to play, as they appear to multiply or constrain the impact of all other MFP driving factors, including competition and international trade, but also the returns from human capital, R&D investments and the adoption of digital technologies.
This section discusses the relationship between financial development and productivity. It provides an assessment of different indicators of financial development used in the empirical literature and describes the way in which financial development can affect productivity growth.
Financial development has been measured through a variety of metrics
The economic literature has measured the degree of financial development in an economy through a variety of indicators. In a seminal paper, King and Levine (1993) defined a first set of indicators of financial development, namely, the financial intermediation ratio or indicator of financial depth (the ratio of liquid liabilities to GDP), a measure of the relative importance of banks (the ratio of deposit money bank domestic assets to deposit money bank domestic assets plus central bank domestic assets), and the proportion of credit allocated to private enterprises (measured by the ratios of claims on the nonfinancial private sector to total domestic credit and to GDP).
Later on, many studies introduced other measures of financial development. The size of the stock market, bank loans to private enterprises as a ratio of GDP, the value of domestic equities traded on domestic exchanges relative to the market size and to GDP, and measures of international financial integration relying on capital asset pricing models and international arbitrage pricing theory, have been often used in the literature (Levine and Zervos, 1998). Other studies looked at the dependence of firms on external finance, using information on capital expenditures exceeding cash flows (Rajan and Zingales, 1998). Claims of financial institutions, other than banks, on the private sector as ratio of GDP, the ratio of bank overhead costs as a ratio of bank total assets (a measure of bank efficiency), bank net interest margins, the share of assets of the largest banks to total bank assets (i.e. bank concentration), have been also used to monitor the functioning of the financial system (Demirgüç-Kunt and Levine, 1999).
Financial development has an impact on productivity
In a seminal paper, King and Levine (1993) found a strong positive contemporaneous relationship between financial development and both economic growth and an allocative efficiency. This is consistent with the view that the good functioning of the financial system (i.e. financial development) can help foster economic growth through boosting the rate of capital accumulation and improving its allocation. However, this study did not address the direction of causality, i.e. whether the relationship occurs double-way, with financial development and economic growth influencing each other.
There is evidence that the causality runs from finance to economic growth. In an important study, Rajan and Zingales (1998) found that financial development raises value added growth in industries that are more dependent on external financing. They concluded that the positive effect of financial development on growth may work in part by facilitating the growth of new enterprises since they are more likely to need external funds than do incumbent firms, as well as by lowering financing costs which facilitates more investment.
Nonetheless, there is some evidence of a hump-shaped relationship between finance and economic performance. Some studies found that financing is an important contributor to economic development at low levels of financial development, but may exert a much lower effect at high levels of financial development (Manning, 2003; Law and Singh, 2014). There is indeed evidence that an expansion of financing in countries with low levels of financial development helps fuel higher growth rates, but the growth effect of more financial development shows diminishing returns (Cournède and Denk, 2015).
Many studies explored the relationship between financial development and productivity (Heil, 2017). Levine and Zervos (1998) found that both bank financing and stock market capitalisation (i.e. equity financing) are positively linked with productivity growth, suggesting a complementarity between these forms of financing. Beck et al. (2000) found that financial development is positively associated with MFP growth and that this finding is robust to changes in model specifications and different measures of financial development. They found that should financial development in Mexico (measured by the private credit to GDP ratio) had risen to their sample median level, Mexican annual MFP growth would have been 0.3% higher. Benhabib and Spiegel (2000) confirmed a positive and robust relationship between financial development (measured as the ratio of financial assets of the private sector to GDP) and MFP growth.
Recent studies underlined that financial development helps to increase productivity by contributing to business dynamism. More developed financial systems help to reduce the share of lower productivity firms (Andrews and Cingano, 2014; Midrigan and Xu, 2014). Similarly, financial frictions can create distortions in the allocation of capital influencing firms’ entry and exit decisions, which in turn affect MFP (Buera et al., 2011). Insolvency regimes which impose high costs on shutting down a business may slow the exit of low-productive firms increasing the number of “zombie firms”, weighing down on aggregate productivity (Caballero et al., 2008; Adalet McGowan et al., 2017). Expectations of costly bankruptcies can also discourage entrepreneurs’ experimentations with higher risks as well as investment with higher potential returns (Andrews et al., 2014).
Financial development can also exert some influence on other MFP drivers. R&D consists of long-run investments with expected positive returns often to be realised in the medium to long-term, features that often call for firms’ external financing (Brown et al., 2012). Similarly, financial institutions may facilitate or hinder human capital accumulation, affecting education decisions (Lochner and Monge-Naranjo, 2012).
Institutions
Institutions, also commonly referred as governance, design entities in charge and influencing the interaction among economic actors, playing an important role in shaping economic growth and productivity growth (North, 1990; Acemoglu and Robinson, 2008; Aghion and Howitt, 2009). For instance, property rights protection, investors’ protection, rule of law, economic freedom, the political regime, corruption, shape the business environment in an economy, stimulating or discouraging investments and influencing the allocation and/or re-allocation of resources.
Institutions act as an important driver of economic and productivity growth by affecting incentives of economic actors and imposing constraints on their actions (Acemoglu, 2008). They influence investments, the adoption of new technologies, and the organisation of production. The literature supports a positive association between, on the one hand, property rights protection, rule of law and economic freedom, and, on the other hand, productivity growth (Barro, 1996; Acemoglu et al., 2001; Lloyd and Lee, 2016; Kar et al., 2019; Alexandre et al., 2022). Recent studies have also shown a positive link between democracy and productivity (Acemoglu et al., 2019).
An important finding that is common to most studies analysing the role of institutions on productivity growth is the “complementary” nature of institutions, as they appear to multiply or constrain the impact of all other MFP driving factors, including competition and international trade, but also the returns from human capital, R&D investments and the adoption of digital technologies.
Nonetheless, the measurement of institutions has serious limitations, as these are typically composite indicators often based on perceptions, sometimes not comparable over time, and with potentially rough scoring systems (Glaeser, 2004; Kurtz and Schrank, 2007; Jellema and Roland, 2011; Ogilvie and Carus, 2014). This calls for caution when using them in empirical analysis with the aim to assess their impact on productivity. In addition, the relationship between productivity growth and institutions typically happens double-way. Analyses failing to account for this reverse causality are likely to cause an upward bias in the estimated returns institutions on MFP.
This section discusses the role of institutions on productivity growth. It first provides an assessment of different attempts to measure the quality of the institutional framework across different economies and over time. It then summarises the most important findings in the economic literature, pointing to those aspects of the institutional framework that appear to have the largest impact on productivity growth.
Measuring the quality of the institutional framework is far from simple
As pointed by Acemoglu et al. (2005), economic institutions affect the incentives of economic actors and determine the constraints on their actions, thereby affecting the distribution of resources. They influence investments, in human, physical and intangible capital, the adoption of new technologies, and the organisation of production. However, political institutions influence the allocation of de jure political power, while groups with greater economic power may possess the de facto political power. This implies that whichever group has more political power is likely to secure the set of economic institutions that it prefers. Therefore, political and economic institutions influence each other, and jointly, shape the economic outcomes.
The term institutions refers to a complex notion that is difficult to define and, hence, measure. In a seminal work, North (1990) defined institutions as “the rules of the game in a society or, more formally, the humanly devised constraints that shape human interaction”. In his view, these rules may be either formal (political constitutions, electoral rules) or informal (culture, social norms) (Aghion and Howitt, 2009). However, most studies exploring the relationship between institutions and economic and productivity growth have focused on the role of the formal rules prevailing in the society (de jure indicators). Institutions have been typically understood as deep-seated social arrangements, such as property rights, rule of law, legal traditions, democratic accountability of governments, and human rights (Easterly, 2005).
Early studies measured institutions and their relevance for long-run economic growth emphasising the role of the origins of countries’ legal codes (La Porta et al., 1998, 1999; Djankov et al., 2003; Glaeser et al., 2004) and colonial histories (Acemoglu et al., 2001; Acemoglu and Johnson, 2005). The legal origins view stresses the differences between the French civil law code and the English common law code, under the presumption that common law systems provide a more flexible environment for firms and entrepreneurs and that such systems facilitate financing and investment by inducing more efficient and speedy debt recovery processes. The colonial history view relies instead on the idea that when Europeans encountered in their colonies natural resources with lucrative international markets but did not find the land, climate, and disease environment suitable for large-scale settlement, only a few Europeans settled and created political institutions to extract those resources weighing down on the colonies’ long-run development. Instead, wherever Europeans found land, climate, and disease environments that were suitable for smaller-scale agriculture, they settled and shaped political institutions that fostered colonies’ development.
However, both the legal origins and the colonial histories approaches have been subject to several criticisms and re-assessments. For example, it was stated that the legal origins view does not explain why France, which initiated the civil law system, performs much better than its colonial transplants (Aghion and Howitt, 2009). Some studies argued that the main contribution of colonial settlers was to build physical and human capital and not necessarily to set up high quality institutions (Glaeser et al., 2004). Others found that even when the European settlement was small, adverse effects from the “extractive” institutions were more than offset by the other elements brought by Europeans, such as human capital, technology, and familiarity with global markets (Easterly and Levine, 2016). Some authors highlighted that the use of old historical variables leaves no room for changes in the institutional framework. However, they stressed the importance of property rights protection and helped to recognise that institutions may themselves be endogenously determined, leading researchers to account for potential reverse causality (i.e. institutions and development influence each other) when formulating their empirical strategy (Lloyd and Lee, 2016), typically using instrumental variables.
More recent studies have moved away from the use of de jure indicators and used composite indicators of institutional quality, which typically try either to capture firms’ and citizens’ perceptions on the functioning of institutions and/or to assess the risk of some sort of instability. The Worldwide Governance Indicators (WGIs) are the most widely used composite indicators of institutions’ quality and governance (Law et al., 2013; Égert, 2016; Kaasa, 2016; Issar et al., 2017; Karimi and Daiari, 2018; Aslam, 2020; Ngo and Nguyen, 2020; Alexandre et al., 2022; Ajide, 2022). The WGIs draw information on the quality of governance from four different types of data sources, namely, surveys of households and firms (e.g. Afrobarometer surveys, Gallup World Poll, Global Competitiveness Report survey), commercial business information (e.g. Economist Intelligence Unit, IHS Markit, Political Risk Services), data from non-governmental organisations (e.g. Global Integrity, Freedom House, Reporters Without Borders), and data from public sector organisations (e.g. country policy and institutional assessments from the World Bank and regional development banks) (Kauffman et al., 2010). The WGIs are divided into six broad dimensions of governance, which include:
Voice and accountability: the extent to which citizens are able to participate in selecting their government, as well as freedom of expression, freedom of association, and a free media.
Political stability and absence of violence/terrorism: the likelihood that the government will be destabilised by unconstitutional or violent means, including terrorism.
Government effectiveness: the quality of public services, the capacity of the civil service and its independence from political pressures, and the quality of policy formulation.
Regulatory quality: the ability of the government to provide sound policies and regulations that enable and promote private sector development.
Rule of law: the extent to which agents have confidence in and abide by the rules of society, including the quality of contract enforcement and property rights, the police, and the courts, as well as the likelihood of crime and violence.
Control of corruption: the extent to which public power is exercised for private gain, including both petty and grand forms of corruption, as well as “capture” of the state by elites and private interests.
The WGIs have been used later on for the computation of the European Quality of Government Index (EQGI) (Charron et al., 2014). The EQGI is a regional-level index calculated by taking the national level indices of governance from the WGIs and correcting these with survey data reflecting the experiences and perceptions of citizens at the regional level in European countries.
A similar set of indicators commonly used in the literature (Jong-A-Pin, 2009; Kim et al., 2018; Kar et al., 2019) are gathered under the International Country Risk Guide (ICRG), which presents monthly political, economic, financial and composite geopolitical risk ratings and forecasts produced by the risk rating agency Political Risk Services (PRS) Group (PRS, 2013). The ICRG offers a way of quantifying the probability of a range of geopolitical risks, from expropriation to social turmoil, capital repatriation, inflation and international liquidity risk, to terrorism. The ICRG ratings assess country’s political, economic and financial risk.
Political risk, aiming to assess a country’s political stability and compounding information from twelve components, namely, government stability, socioeconomic conditions, investment profile, external conflict, corruption, military in politics, religion in politics, law and order, ethnic tensions, democratic accountability, and bureaucracy quality.
Economic risk, with the goal to assess a country’s economic strengths and weaknesses, relying on information about country’s GDP per capita, real annual GDP growth, annual inflation rate, budget balance as percentage of GDP, and current account balance as percentage of GDP.
Financial risk, to assess a country's ability to finance its official, commercial, and trade debt obligations, using information on total foreign debt as percentage of GDP, debt service as percentage of exports of goods and services, current account balance as percentage of exports of goods and services, international liquidity as months of import cover and exchange rate stability.
Another widely use composite indicator is the Economic Freedom of the World index, which accounts for formal constraints on interactions among government, businesses and individuals (Bjørnskov and Foss; 2010; Doyle and Martinez-Zarzoso, 2011; Aisen and Veiga, 2013; Égert, 2016; Alexandre et al., 2022). The index measures the degree to which policies and institutions in a given country are supportive of economic freedom (Gwartney et al., 2021). The degree of economic freedom is measured by ranking countries on five areas: size of government, legal structure and property rights, access to sound money (inflation risk), freedom to trade internationally, and credit, labour and business regulations.
Other datasets such as the Polity Project datasets (Rodrik and Wacziarg, 2005; Aghion et al., 2007; Cavallo and Cavallo, 2010; Kim et al., 2018; Acemoglu et al., 2019), and the Freedom House Index (Aghion et al., 2007; Acemoglu et al., 2019) have focused on a particular characteristic of institutional frameworks: the prevalence of democracy. The Polity Project dataset examines concomitant qualities of democratic and autocratic authority through the “Polity Score” index and its sub-components. The conceptual framework for the “polity” (government) studies was derived from the analytic scheme formulated by Eckstein and Gurr (1975) to describe patterns of authority. The index ranges from -10 to 10 (where -10 is high-autocracy and 10 is high-democracy) and is constructed as the difference between sub-indexes for democracy and autocracy (Marshall, 2020). The Freedom House Index is a rating of perceptions on political rights and civil liberties, relying on separate scores and status for electoral process, political pluralism and participation, government transparency, corruption, freedom of expression and belief for media, academics and individuals, organisational rights and rule of law (Freedom House, 2022).
There exist many other metrics of institutional quality that, however, have been less frequently used in empirical analyses linking institutions to economic and productivity growth. These include the Varieties of Democracy (Akhremenko, et al., 2019; Berggren and Bjørnskov, 2022), which measures attributes of democracy (i.e. nature, causes, consequences) (V-Dem Dataset); the Index of Economic Freedom by the Heritage Foundation (Naanwaab and Yeboah, 2013; Uddin et al., 2019), the Database of Political Institutions (DPI) (Beck et al., 2001; Scartascini et al., 2021), which presents institutional and electoral results data; and “the regulation of entry” indicators produced by Djankov et al. (2002), including the number of procedures that firms must go through, the official time required to complete the process, and its official cost, which have later been included in the production of the World Bank Doing Business indicators (section covering Competition, Ciccone and Papaioannou, 2007).
All these composite “institutional variables” have some limitations. They do not always capture actual laws, rules and compliance procedures (Glaeser, 2004). They often rely on surveys and data sources that may be affected by perception and selection biases (Kurtz and Schrank, 2007). Certain components may be constructed on the basis of binary variables, so that they exclude gradations in between two opposite choices (Ogilvie and Carus, 2014). Other authors stressed that these are summary measures resulting from the weighting of several institution categories, which in turn are affected by subjective evaluation, noise and volatility (Jellema and Roland, 2011). These limitations also weigh down on the comparability of these indicators over time. In addition, the large number of metrics aiming to measure the very same phenomena have led researchers to consolidate indicators from different datasets producing new ones (Acemoglu et al., 2019) and to consider different datasets and indicators, each at a time, to assess the robustness of their results (Kim et al., 2018).
Institutions matter for productivity
The economic literature has widely recognised that a sound institutional framework is key to economic success of countries, regions and individual firms. The protection of property rights and the rule of law have been listed among the higher-order principles that ensure productive efficiency (Rodrik, 2005). Many empirical studies have found a positive association between the protection of property rights and the rule of law, on the one hand, and productivity growth (Barro, 1996; Acemoglu et al., 2001; Lloyd and Lee, 2016; Alexandre et al., 2022). Similarly, Kar et al., (2019) found a relationship between low-quality institutions traps (measured by the sub-components of the political risk within the ICRG, including bureaucratic quality, law and order and corruption) and low-income traps. Differences in the quality of the rule of law, government effectiveness, voice and accountability, corruption, regulatory quality across regions within a single country have also been found as one of the main drivers of MFP differentials across firms operating in different regions (Lasagni et al., 2015; Rodríguez-Pose and Ganau, 2022). These institutions have been found to be more important in fostering MFP for smaller, younger, less human-capital intensive firms and those operating in less technologically advanced industries in European regions, suggesting that well-designed and more effective regional government institutions may play a compensating role with respect to firms’ individual factors of weakness (Agostino et al., 2020).
In addition, a higher degree of economic freedom, in the form of a smaller regulatory burden, greater freedom to trade and invest, and smaller government intervention in the economy has been associated with greater economic growth (de Haan et al., 2006) and higher productivity growth rates (Aisen and Veiga, 2013; Alexandre et al., 2022). In this regard, market-creating and market-stabilising institutions have been found to be particularly important for lower-income countries, as they appear to favour the incentives to accumulate and innovate and build resilience towards macroeconomic shocks, reducing inflationary pressure and dissipating the risk of economic and financial crisis (Das and Quirk, 2016).
Many studies found a positive association between democracy and economic performance regardless the stage of development (Acemoglu et al., 2019). They suggest that democratic leaders are less likely to impose socially inefficient regulations or engage in rent-seeking activities and, hence, enhance firm productivity (Abeberese et al., 2021). These analyses also reveal that major democratic transitions have, if anything, a positive effect on economic growth in the short run and a decline in growth volatility (Rodrik and Wacziarg, 2005). In addition, in countries with democratic institutions, the negative effect of crises is mitigated or even eliminated, while in countries with autocratic institutions, the negative effect is exacerbated (Cavallo and Cavallo, 2010). Further, there seems to exist a virtuous circle, where accumulation of physical and democratic capital (this latter measured as the nation’s historical experience with democracy) reinforce each other, promoting economic development and the consolidation of democracy (Persson and Tabellini, 2009).
Similarly, corruption (Mauro, 1995; Rose-Ackerman, 1999; Venard, 2013; Azam and Emirullah, 2014) and political instability (Jong-A-Pin, 2009; Aisen and Veiga, 2013; Alexandre et al., 2022) have been found to be negatively associated with economic development and productivity. Corruption may lead to a poor allocation of resources and distorts the decision-making processes of officials, as these may be more likely to support investments associated with higher bribes than those associated with higher economic output (Venard, 2013). Political instability shortens the horizons of governments, disrupting long-term economic policies conducive to a better economic performance. Estimates for a sample of 170 countries from 1960 to 2004 suggest that an additional cabinet change per year (e.g. a new premier is named and/or 50% of cabinet posts are occupied by new ministers) reduces the average annual real GDP per capita growth rate by 2.4 percentage points (Aisen and Veiga, 2013).
Institutions may enhance or undermine the role of other productivity drivers
Most studies highlight that institutions act also indirectly on productivity growth, either by intensifying or undermining other drivers’ returns such as human capital, R&D, international trade and competition on productivity.
For instance, institutional factors have been found to drive the conditions for competition and entrepreneurship (Égert, 2016; Urbano et al., 2019). Procedures and costs to create a business, support mechanisms for new firm, property rights protection and the enforcements of contracts, tend to reduce the transaction costs enhancing market performance related to prices and distribution. Therefore, institutions can help the market work more efficiently by removing market imperfections and rigid administrative regulations (Djankov et al., 2002; Ciccone and Papaioannou, 2007; Urbano et al., 2019; Ajide et al., 2022).
Similarly, some studies showed that democracy is more likely to foster an environment with fewer restrictions on learning, travel, work, investment and communications, which in turn foster competition and facilitates the innovative and entrepreneurial process (Bhagwati, 2002). Democracy has been found to have higher impact on productivity growth in industries that are closer to the world technological frontier, likely reflecting the multiplier effects of the former on competition and innovation (Aghion et al, 2007).
Institutions can strengthen or weaken the relationship between human capital and growth. Democracy has been found to be more conducive to economic growth in countries with greater levels of secondary education (Acemoglu et al., 2019). Some authors found that institutions have only a second-order effect on economic performance, and the first-order effect comes instead from human capital as this shapes both the institutional and productive capacity of a society (Glaeser et al., 2004). Indeed, recent empirical findings showed that human capital alone, without improvements in institutional quality, cannot breed a significant growth generating process in Asian economies (Aslam, 2020). The returns of human capital on productivity growth may be reduced significantly in the presence of weak and dysfunctional institutions because of the increase in rent-seeking and socially unproductive activities (Uddin et al., 2020).
The quality of institutions can also trump the impact of international trade and FDI flows on economic performance. The positive of impact of international trade on productivity growth is estimated to be reduced once institutions are accounted for, suggesting that productivity gains attributed risk being overestimated is institutional quality is ignored (Rodrik et al., 2004; Doyle and Martínez-Zarzoso, 2011; Égert, 2016). In addition, the impact of international trade is found to increase when controlling for institutions in those countries with lowest institutional quality (Doyle and Martínez-Zarzoso, 2011).
Much of the benefits of better institutions transit through R&D investments. Indeed, a higher rule of law and better law enforcement appear to amplify the positive effect of R&D spending on MFP in OECD countries (Égert, 2016). At the same time, more costly and lengthy contract enforcement procedures offset some of the benefits of higher R&D spending.
References
OECD (2015), The Future of Productivity, OECD Publishing, Paris, https://doi.org/10.1787/9789264248533-en.
Research and development
Aghion, P. and Howitt, P. (1992), “A model of growth through creative destruction”, Econometrica, Vol. 60, No. 2 (March, 1992), pp. 323-351.
Ang, J.B. and Madsen, J.B. (2013), “International R&D Spillovers and Productivity Trends in the Asian Miracle Economies”, Economic Inquiry, Vol. 51, No. 2, April 2013, pp. 1523–1541.
APO/OECD (2021), Towards Improved and Comparable Productivity Statistics: A Set of Recommendations for Statistical Policy, OECD Publishing, Paris, https://doi.org/10.1787/1ae0ec74-en.
Asian Development Bank (2022), Value for money in public-private partnerships: An infrastructure governance approach, http://dx.doi.org/10.22617/TCS220103.
Aw, B.Y, Roberts, M. and Xu, Y. (2009), “R&D Investment, Exporting, and Productivity Dynamics”, NBER Working Paper 14670, National Bureau of Economic Research, Cambridge, Massachusetts.
Barro, R.J. and Sala-i-Martin, X. (2004), Economic Growth, Second Edition, The MIT Press.
Bloom, N., Jones, C.I., Van Reenen, J. and Webb, M. (2020), “Are Ideas Getting Harder to Find?”, American Economic Review, 110(4): 1104–1144, https://doi.org/10.1257/aer.20180338.
Cameron, G., Proudman, J. and Redding, S. (2005), “Technological convergence, R&D, trade and productivity growth”, European Economic Review 49, pp. 775 – 807.
Coe, D.T. and Helpman, E. (1995), “International R&D spillovers”, European Economic Review, 39(5): 859–887.
Coe, D.T., Helpman, E., and Hoffmaister, A.W. (2009), “International R&D spillovers and institutions”, European Economic Review 53(7): 723–741.
Diewert, W. E., and Huang, N. (2011), “Capitalizing R&D Expenditures”, Macroeconomic Dynamics, 15, 537–64.
Doraszelski, U. and Jaumandreu, J. (2013), “R&D and Productivity: Estimating Endogenous Productivity”, The Review of Economic Studies, October 2013, Vol. 80, No. 4 (285), pp. 1338-1383.
Elnasri, A. and Fox, K.J. (2015), “R&D, Innovation and Productivity: The Role of Public Support”, KDI Journal of Economic Policy, Vol. 37 No. 1, pp. 73-96.
European Investment Bank (2016), “Investment and Investment Finance in Europe: Financing Productivity Growth”, Economics Department, European Investment Bank.
Frantzen, D. (2000), “R&D, human capital and international technology spillovers: a cross‐country analysis”, Scandinavian Journal of Economics, 102(1): 57–75.
Frantzen, D. (2003), “The Causality between R&D and Productivity in Manufacturing: An international disaggregate panel data study”, International Review of Applied Economics, 17:2, 125-146, DOI: 10.1080/0269217032000064017.
Griffith, R., Redding, S. and Van Reenen, J. (2000), “Mapping the Two Faces of R&D: Productivity Growth in a Panel of OECD Industries”, Available at: http://dx.doi.org/10.2139/ssrn.229400.
Griliches, Z. (1973), Research expenditures and growth accounting, International Economic Association Series, in: B. R. Williams (ed.), Science and Technology in Economic Growth, chapter 3, pages 59-95, Palgrave Macmillan.
Griliches, Z. (1979), “Issues in assessing the contribution of research and development to productivity growth”, Bell Journal of Economics, 10 (1979), pp. 92-116.
Griliches, Z. (1980), New Developments in Productivity Measurement, Returns to Research and Development Expenditures in the Private Sector, pp. 419-462, http://www.nber.org/books/kend80-1.
Griliches, Z. and Lichtenberg, F. (1984), R&D and Productivity Growth at the Industry Level: Is There Still a Relationship?, R&D, Patents, and Productivity, University of Chicago Press.
Grossman, G.M and Helpman, E. (1991), Innovation and Growth in the Global Economy, The MIT Press.
Guellec, D. and Van Pottelsberghe De La Potterie, B. (2004) “From R&D to productivity growth: Do the institutional settings and the source of funds of R&D matter?”, Oxford Bulletin of Economic and Statistics, 66 (3) (2004), pp. 353-378.
Hall, B.H. (2020), “Innovation and productivity”, NBER Working Paper Series, Working Paper 17178, http://www.nber.org/papers/w17178.
Hammar, N. and Belarbi, Y. (2021), “R&D, innovation and productivity relationships: Evidence from threshold panel model”, International Journal of Innovation Studies, 5, pp. 113-126.
Herzer, D. (2022), “The impact of domestic and foreign R&D on TFP in developing countries”, World Development, 151 (2022), https://doi.org/10.1016/j.worlddev.2021.105754.
Krammer, S. M. S. (2010). International R&D spillovers in emerging markets: The impact of trade and foreign direct investment. Journal of International Trade and Economic Development, 19(4), 591–623.
Lu, W-C., Chen, J-R. and Wang, C-L. (2006), “Granger causality test on R&D spatial spillovers and productivity growth”, Applied Economics Letters, 13:13, 857-861, DOI: 10.1080/13504850500425360.
Madsen, J. B., Islam, M. R., and Ang, J. B. (2010). Catching up to the technology frontier: The dichotomy between innovation and imitation. Canadian Journal of Economics, 43(4), 1389–1411.
Martin, L. and Nguyen-Thi, T.U. (2015), “The relationship between innovation and productivity based on R&D and ICT use: An empirical analysis of firms in Luxembourg”, SciencesPo Les Presses, 2015/6 Vol. 66, pp. 1105-1130, https://www.cairn.info/revue-economique-2015-6-page-1105.htm.
Miyagawa, T. and Ishikawa, T. (2019), “On the decline of R&D efficiency”, RIETI Discussion Paper Series 19-E-052.
Rouvinen, P. (2002), “R&D – Productivity Dynamics: Causality, Lags, and ‘Dry Holes’”, Journal of Applied Economics, 5:1, pp. 123-156, DOI: 10.1080/15140326.2002.12040573.
Schreyer, P. and Zinni, M.B. (2021), “Productivity Measurement, R&D Assets, and Mark-Ups in OECD Countries”, Review of Income and Wealth, 67: 787-809, https://doi.org/10.1111/roiw.12492.
Su, Z., Wang, C. and Peng, M.W. (2021), “Intellectual property rights protection and total factor productivity”, International Business Review, https://doi.org/10.1016/j.ibusrev.2021.101956.
United Nations., European Commission., International Monetary Fund., Organisation for Economic Co-operation and Development., & World Bank. (2009), System of National Accounts 2008, New York: United Nations, https://unstats.un.org/unsd/nationalaccount/docs/sna2008.pdf.
Wieser, R. (2005), “Research and development productivity and spillovers: Empirical evidence at the firm level”, Journal of Economic Surveys, 19(4), pp. 587-621.
Digital technologies, ICT and other intangibles
Acemoglu, D. and Restrepo, P. (2019), “Automation and New Tasks: How Technology Displaces and Reinstates Labor”, Journal of Economic Perspectives, Volume 33, Number 2, Spring 2019, pp.3-30.
Aghion, P., Jones, B.F. and Jones, C.I. (2017), “Artificial intelligence and economic growth”, NBER Working Paper Series, Working Paper 23928, http://www.nber.org/papers/w23928.
Ahmad, N. and Koh, S. (2011), “Incorporating Estimates of Household Production of Non-Market Services into International Comparisons of Material Well-Being”, OECD Statistics Working Papers, No. 2011/07, OECD Publishing, Paris, https://doi.org/10.1787/5kg3h0jgk87g-en.
Ahmad, N., Ribarsky, J. and Reinsdorf, M. (2017), “Can potential mismeasurement of the digital economy explain the post-crisis slowdown in GDP and productivity growth?”, OECD Statistics Working Papers, No. 2017/09, OECD Publishing, Paris, https://doi.org/10.1787/a8e751b7-en.
Ahmad, N. and Schreyer, P. (2016), “Measuring GDP in a Digitalised Economy”, OECD Statistics Working Papers, No. 2016/07, OECD Publishing, Paris, https://doi.org/10.1787/5jlwqd81d09r-en.
APO (2022), Digital Readiness Assessment: Methodology & Framework, APO Publishing, Tokyo.
APO/OECD (2021), Towards Improved and Comparable Productivity Statistics: A Set of Recommendations for Statistical Policy, OECD Publishing, Paris, https://doi.org/10.1787/1ae0ec74-en.
Bakhshi, H., Bravo-Biosca, A. and Mateos-Garcia, J. (2014), “The analytical firm: Estimating the effect of data and online analytics on firm performance”, Nesta Working Paper 14/05, August 2014, www.nesta.org.uk/wp14-05.
Baumol, W.J. (1967), “Macroeconomics of Unbalanced Growth: The Anatomy of Urban Crisis,” American Economic Review (June 1967), 57, 415–426.
Bloom, N., Sadun, R. and Van Reenen, J. (2012), “Americans do I.T. better: US multinationals and the productivity miracle”, NBER Working Paper Series, Working Paper 13085, http://www.nber.org/papers/w13085.
Brynjolfsson, E., D. Rock and C. Syverson (2017), Artificial Intelligence and the Modern Productivity Paradox: A Clash of Expectations and Statistics, http://dx.doi.org/10.3386/w24001.
Byrne, D., Fernald, J. and Reinsdorf, M. (2016), “Does the United States have a productivity slowdown or a measurement problem?”, Brookings Papers on Economic Activity, Spring 2016, pp. 109-157, https://www.brookings.edu/wp-content/uploads/2016/03/byrnetextspring16bpea.pdf.
Cardarelli, R. and Lusinyan, L. (2015), “U.S. Total Factor Productivity Slowdown: Evidence from the U.S. States”, IMF Working Paper, WP/15/116.
Cette, G., Nevoux, S. and Py, L. (2020), “The impact of ICTs and digitalization on productivity and labor share: Evidence from French firms”, Banque de France WP #785, https://publications.banque-france.fr/en/impact-icts-and-digitalization-productivity-and-labor-share-evidence-french-firms.
Corrado, C., Haskel, J. and Jona-Lasinio, C. (2017), “Knowledge Spillovers, ICT and Productivity Growth”, Oxford Bulletin of Economics and Statistics, 79, 4.
Crass, D. and Peters, B. (2014), “Intangible Assets and Firm-Level Productivity”, ZEW - Centre for European Economic Research Discussion Paper No. 14-120, Available at SSRN: https://ssrn.com/abstract=2562302 or http://dx.doi.org/10.2139/ssrn.2562302.
Damioli, G., Van Roy, V. and Vertesy, D. (2021), “The impact of artificial intelligence on labor productivity”, Eurasian Business Review (2021) 11:1–25, https://doi.org/10.1007/s40821-020-00172-8.
Fernald J.G. (2014), “Productivity and Potential Output before, during and after the Great Recession”, NBER Macroeconomics Annual, Vol. 29.
Ferracane, M.F., Kren, J. and van der Marel, E. (2018), “Do Data Policy Restrictions Impact the Productivity Performance of Firms and Industries?”, European Center for International Political Economy (ECIPE).
Fukao, K., Miyagawa, T., Pyo, H.K. and Rhee, K.H. (2011), “Estimates of Total Factor Productivity, the Contribution of ICT, and Resource Reallocation Effects in Japan and Korea”, Global COE Hi-Stat Discussion Paper Series 177.
Gal, P., Nicoletti, G., Renault, T., Sorbe, S. and Timiliotis, C. (2019), “Digitalisation and productivity: In search of the holy grail – Firm-level empirical evidence from European countries”, OECD Economics Department Working Papers No. 1533, OECD Publishing, Paris.
Geppert, K. and Neumann, A. (2011), “Regional Patterns of Intangible Capital, Agglomeration Effects and Localised Spillovers in Germany”, SSRN eLibrary.
Haskel, J, and S Westlake (2017), Capitalism Without Capital The Rise of the Intangible Economy, Princeton, NJ: Princeton University Press.
Hawash, R. and Lang, G. (2020), “Does the digital gap matter? Estimating the impact of ICT on productivity in developing countries”, Eurasian Economic Review, 10, 189–209, https://doi.org/10.1007/s40822-019-00133-1.
Hintzmann, C., Lladós-Masllorens, J. and Ramos, R. (2021), “Intangible Assets and Labor Productivity Growth”, Economies 9: 82, https://doi.org/10.3390/economies9020082.
Jona-Lasinio, C., Iommi, M. and Manzocchi, S. (2011), “Intangible capital and Productivity Growth in European Countries”, Department of Economic and Business Sciences, LUISS Guido Carli.
Kaldor, N. (1961), “Capital Accumulation and Economic Growth”, in F.A. Lutz and D.C. Hague, eds., The Theory of Capital, St.Martins Press, pp. 177–222.
Mehmood, B. and Azim, P. (2014), “Total Factor Productivity, Demographic Traits and ICT: Empirical Analysis for Asia”, Informatica Economică, vol. 18, no. 1/2014.
Nakamura, L. and Soloveichik, R. (2015), “Capturing the Productivity Impact of the ‘Free’ Apps and Other Online Media”, Federal Reserve Bank of Philadelphia Working Paper: 15-25.
OECD (2004), The Economic Impact of ICT: Measurement, Evidence and Implications, OECD Publishing, Paris, https://doi.org/10.1787/9789264026780-en.
OECD (2018), Economic Outlook for Southeast Asia, China and India 2018: Fostering Growth through Digitalisation, OECD Publishing, Paris, https://doi.org/10.1787/9789264286184-en.
OECD (2019), Southeast Asia Going Digital: Connecting SMEs, OECD, Paris, www.oecd.org/going-digital/southeast-asia-connecting-SMEs.pdf.
OECD (2022), OECD Going Digital Toolkit, Theme 3: Productivity, https://goingdigital.oecd.org/theme/3.
Pyo H. K., Jung, S.Y. and Cho, J. S. (2007), “Estimates of Gross Fixed Capital Formation, Net Capital Stock and Capital Intensity in Korea: 11 Assets by 72 Sectors (1970-2005),” Journal of Korean Economic Analysis, vol. 13 No. 1, December 2007.
Raknerud, A., Rybalka, M. and von Brasch, T. (2020), “The impact of intangible assets on productivity growth in the Norwegian economy: Evidence from firm-level data”, GLOBALINTO, https://globalinto.eu/the-impact-of-intangible-assets-on-productivity-growth-in-the-norwegian-economy-evidence-from-firm-level-data-d5-1/.
Riley, R. and Robinson, C. (2011), “Agglomeration Effects and Localised Spillovers from Intangible Capital: An Analysis of UK City Regions”, INNODRIVE.
Syverson, C. (2017), “Challenges to Mismeasurement Explanations for the US Productivity Slowdown”, Journal of Economic Perspectives, 31(2): 165-86.
Timmer, M.P. and van Ark, B. (2005), “Does information and communication technology drive EU-US productivity growth differentials?”, Oxford Economic Papers 57, 693-716.
Venturini, F. (2015), “The modern drivers of productivity”, Research Policy, 44 (2015), 357-369, http://dx.doi.org/10.1016/j.respol.2014.10.011.
United Nations., European Commission., International Monetary Fund., Organisation for Economic Co-operation and Development., & World Bank. (2009). System of National Accounts 2008. New York: United Nations, https://unstats.un.org/unsd/nationalaccount/docs/sna2008.pdf.
Human capital
Acemoglu, D. and Restrepo, P. (2019), “Automation and New Tasks: How Technology Displaces and Reinstates Labor”, Journal of Economic Perspectives, Vol. 33/2, pp. 3-30, https://doi.org/10.1257/jep.33.2.3.
Andretta, C., Brunetti, I. and Rosso, A. (2021) “Productivity and Human Capital - The Italian case”, OECD Productivity Working Papers, 2021-25, OECD Publishing, Paris.
APO-OECD (2021), Towards Improved and Comparable Productivity Statistics: A Set of Recommendations for Statistical Policy, OECD Publishing, Paris, https://doi.org/10.1787/1ae0ec74-en.
Australian Bureau of Statistics, ABS (2021), “Estimates of Industry Multifactor Productivity”, available at: https://www.abs.gov.au/statistics/industry/industry-overview/estimates-industry-multifactor-productivity/latest-release.
Autor, D. (2014), “Polanyi’s Paradox and the Shapen of Employment Growth”, NBER Working Paper Series No. 20485, http://dx.doi.org/10.3386/w20485.
Autor, D., Levy, F. and Murnane, R. (2003), “The skill content of recent technological change: An empirical exploration”, The Quarterly Journal of Economics, Vol. 118/4, pp. 1279-1333, http://dx.doi.org/10.1162/003355303322552801.
Barro, R. and Lee, J.W. (1993), “International comparisons of educational attainment”, Journal of Monetary Economics 32:363–94.
Barro, R.J. and Lee, J.W. (1996), “International measures of schooling years and schooling quality”, American Economic Review, Papers Proc., 82 (2) (1996), pp. 218-223.
Barro, R.J. and Lee, J.W. (2001), “International data on educational attainment: updates and implications”, Oxford Economic Papers 53(3): 541–563.
Barro, R. and Lee, J.W. (2010), “A New Data Set of Educational Attainment in the World, 1950-2010”, NBER Working Paper No. 15902.
Barro, R. and Lee, J.W. (2013), “A New Data Set of Educational Attainment in the World, 1950-2010”, Journal of Development Economics, vol 104, pp.184-198, https://doi.org/10.1016/j.jdeveco.2012.10.001.
Benhabib, J. and Spiegel, M.M. (2002), “Human Capital and Technology Diffusion”, FRBSF Working Paper #2003-02.
Bloom, N. and Van Reenen, J. (2007), “Measuring and explaining management practice across firms and countries”, The Quarterly Journal of Economics, Vol. 122, Issue 4.
Bloom et al. (2018), “What drives differences in management practices?”, CEP Discussion Paper No. 1470, London School of Economics and Political Science, London.
Coe, D.T. and Helpman, E. (1995), “International R&D spillovers”, European Economic Review 39(5): 859–887.
Coe, D.T., Helpman, E., and Hoffmaister, A.W. (2009), “International R&D spillovers and institutions”, European Economic Review 53(7): 723–741.
Criscuolo, C., Gal, P., Leidecker, T. and Nicoletti, G. (2021), "The human side of productivity: Uncovering the role of skills and diversity for firm productivity", OECD Productivity Working Papers, No. 29, OECD Publishing, Paris, https://doi.org/10.1787/5f391ba9-en.
Dua, P. and Garg N.K. (2019), “Determinants of labour productivity: Comparison between developing and developed countries of Asia-Pacific”, Pac Econ Rev, 24: 686–704, https://doi.org/10.1111/1468-0106.12294
Égert, B., C. de la Maisonneuve and D. Turner (2022), "A new macroeconomic measure of human capital exploiting PISA and PIAAC: Linking education policies to productivity", OECD Economics Department Working Papers, No. 1709, OECD Publishing, Paris, https://doi.org/10.1787/a1046e2e-en.
Engelbrecht, H‐J. (2002), “Human capital and international knowledge spillovers in TFP growth of a sample of developing countries: an exploration of alternative approaches”, Applied Economics 34(7): 831–841.
Fukao, K., Hamagata, S., Inui, T., Ito, K., Kwon, H., Makino, T., Miyagawa, T., Nakanishi, Y. and Tokui, J. (2007), “Estimation Procedures and TFP Analysis of the JIP Database 2006”, RIETI Discussion Paper Series 07-E-003.
Goos, M., Manning, A. and Salomons, A. (2014), “Explaining job polarization: Routine-biased technological change and offshoring”, The American Economic Review, Vol. 104/8, pp. 2509-2526, http://dx.doi.org/10.1257/aer.104.8.2509.
Kim, E., Mourougane, A. and Baker, M. (2020), "What drives firm and sectoral productivity in the United Kingdom and in selected European countries?", OECD Economics Department Working Papers, No. 1630, OECD Publishing, Paris, https://doi.org/10.1787/36ceb723-en.
Korhonen, R. (2020), “Multi-factor productivity estimates, UK: July to September 2019”, Office for National Statistics (ONS), Available at: https://www.ons.gov.uk/economy/economicoutputandproductivity/productivitymeasures/articles/multifactorproductivityestimates/julytoseptember2019.
Lui, J. and Bi, C. (2019), “Effects of Higher Education Levels on Total Factor Productivity Growth”, Sustainability 2019, 11, 1790; doi:10.3390/su11061790.
Maudos, J., Pastor, J.M., and Serrano, L. (2003), “Human capital in OECD countries: technical change, efficiency and productivity”, International Review of Applied Economics 17(4): 419–435.
Miller, S.M. and Upadhyay, M.P. (2000), “The effects of openness, trade orientation, and human capital on total factor productivity”, Journal of Development Economics 63(2): 399–423.
Nelson, R.R. and Phelps, E.S. (1966), “Investment in humans, technological diffusion, and economic growth”, The American Economic Review 56(1/2): 69–75.
Nomura, K. and Akashi, N. (2017), “Measuring Quality-adjusted Labour Inputs in South Asia, 1970-2015”, KEO Discussion Paper No. 143. KEO: Tokyo.
OECD (2001), Measuring Productivity - OECD Manual: Measurement of Aggregate and Industry-level Productivity Growth, OECD Publishing, Paris, https://doi.org/10.1787/9789264194519-en.
OECD (2020), Promoting an Age-Inclusive Workforce: Living, Learning and Earning Longer, OECD Publishing, Paris, https://doi.org/10.1787/59752153-en.
OECD (2022), Productivity, human capital and educational policies, Available at: https://www.oecd.org/economy/human-capital/.
Park, J. (2012), “Total factor productivity growth for 12 Asian economies: the past and the future”, Japan and the World Economy 24(2): 114–127.
Parrotta, P., Pozzoli, D. and Pytlikova, M. (2012), “Does Labor Diversity Affect Firm Productivity?”, IZA Discussion Paper Series, No. 6973.
Romer, P.M. (1989), “Human capital and growth: theory and evidence”, NBER Working Paper No. 3173.
United Nations, European Commission, International Monetary Fund, Organisation for Economic Co-operation and Development, & World Bank. (2009), System of National Accounts 2008, New York: United Nations, https://unstats.un.org/unsd/nationalaccount/docs/sna2008.pdf.
Vandenberg, P., and Trinh, L.Q. (2016), “Small Firms, Human Capital, and Productivity in Asia”, ADBI Working Paper 582, Tokyo: Asian Development Bank Institute. Available: http://www.adb.org/publications/small-firms-human-capital-and-productivity-asia/.
Vandenbussche, J., Aghion, P. and Meghir, C. (2006), “Growth, distance to frontier and composition of human capital”, Journal of Economic Growth, 11: 97-127. DOI 10.1007/s10887-006-9002-y.
Woolley, A. et al. (2010), “Evidence for a Collective Intelligence Factor in the Performance of Human Groups”, Science, Vol. 330/6004, pp. 686-688, http://dx.doi.org/DOI:10.1126/science.1193147.
Public infrastructure
Agénor, P. and D. Yalmiz (2017) “The simple dynamics of public debt with productive capital goods”, Macroeconomic Dynamics, 21, 2017, 1059–1095. Printed in the United States of America.
Aghion, P. and Hémous, D. and Kharroubi, E. (2014), “Cyclical fiscal policy, credit constraints, and industry growth”, Journal of Monetary Economics vol. 62, p 41-58, https://doi.org/10.1016/j.jmoneco.2013.12.003
Aghion, P. and Angeletos, G-M. and Banerjee, A. and Manova, K. (2005), “Volatility and growth: Credit constraints and the composition of investment”, Journal of Monetary Economics vol. 57, p 246-265, https://doi.org/10.1016/j.jmoneco.2010.02.005
Arbués, P., J. Baños and M. Mayor (2015) “The Spatial productivity of transportation infrastructure”, Transportation Research Part A 75 (2015) 166–177.
Aschauer, D.A. (1989), “Is public expenditure productive?”, Journal of Monetary Economics vol. 23, p 177-200, https://doi.org/10.1016/0304-3932(89)90047-0
APO-OECD (2021), Towards Improved and Comparable Productivity Statistics: A Set of Recommendations for Statistical Policy, OECD Publishing, Paris, https://doi.org/10.1787/1ae0ec74-en.
Baldwin, J. and J. Dixon (2008), “Infrastructure Capital: What Is It? Where Is It? How Much of It Is There?”, The Canadian Productivity Review, Catalogue no. 15-206-X — No. 016.
Baltagi, B.H. and Pinnoi, N. (1995), “Public capital stock and state productivity growth: Further evidence from an error components model”, Empirical Economics vol. 20, p 351-359, https://link.springer.com/article/10.1007/BF01205444
Banister, D. (2012) “Transport and economic development: Reviewing the evidence”, Transport Reviews, 32(1), 1–2.
Bennett, J. and Kornfeld, R. and Sichel, D. and Wasshausen, D. (2020), “Measuring infrastructure in the Burea of Economic Analysis National Economic Accounts”, BEA working paper series WP 2020-12, https://www.bea.gov/system/files/papers/BEA-WP2020-12.pdf
Bizimana, O. and Jaramillo, L. and Thomas, S. and Yoo, J. (2021), “Scaling Up Quality Infrastructure Investment in South Asia”, IMF Working Paper WP/21/117, https://www.sipotra.it/wp-content/uploads/2021/05/Scaling-Up-Quality-Infrastructure-Investment-in-South-Asia.pdf
Boarnet, M. (1998) “Spillovers and the locational effects of public infrastructure”, Journal of Regional Science, Vol. 38, No. 3, 1998, ppp. 381-400.
Bom, P.R. and Ligthart, J.E. (2014): "What Have We Learned From Three Decades of Research on the Productivity of Public Capital?", Journal of Economic Surveys, 28(5), 889-916. http://hdl.handle.net/10.1111/joes.12037
Bonaglia, F., La Ferrara, E. and Marcellino, M. (2000), “Public Capital and Economic Performance: Evidence from Italy”, Giornale degli Economisti e Annali di Economia , Settembre 2000, Nuova Serie, Vol. 60 (Anno 113), No. 2 (Settembre 2000), pp. 221-244.
Candelon, B., G. Colletaz and C. Hurlin (2013), “Network Effects and Infrastructure Productivity in Developing Countries”, Oxford Bulletin of Economics and Statistics, 75, 6 (2013) 0305-9049.
Cisneros, Henry (2010), “America’s Essential Infrastructure: A Key to Competitiveness,” in The Handbook of Infrastructure Investing, ed. by Michael Underhill, (Hoboken, NJ: Wiley & Sons).
Cohen, J. P., and Morrison Paul, C. J. (2004) “Public infrastructure investment, inter-state spatial spillovers, and manufacturing costs”, Review of Economics and Statistics, 86(2), 551–560.
Cohen, J. (2010), “The broader effects of transportation infrastructure: Spatial econometrics and productivity approaches”, Transportation Research Part E 46 (2010) 317–326.
Elburz, Z., and Cubukcu, K.M (2021) “Spatial effects of transport infrastructure on regional growth: the case of Turkey” Spatial Information Research volume 29, pages19–30 (2021) https://doi.org/10.1007/s41324-020-00332-y
De la Fuente, A. (2010), “Infrastructures and Productivity: An Updated Survey”, Barcelona Economics Working Paper Series, Working Paper nº 475, June 2010.
Demetriades, P.O. and T.P. Mamuneas (2000), “Intertemporal Output and Employment Effects of Public Infrastructure Capital: Evidence from 12 OECD Economies”, Economic Journal 110, 687–712.
Deng, T. (2013), “Impacts of Transport Infrastructure on Productivity and Economic Growth: Recent Advances and Research Challenges”, ransport Reviews, 33:6, 686-699, https://doi.org/10.1080/01441647.2013.851745.
Destefanis, S. and V. Sena (2005), “Public capital and total factor productivity: New evidence from the Italian regions, 1970–98”, Regional Studies, 39:5, 603-617, https://doi.org/10.1080/00343400500151863.
Duggal, V.G., Saltzman, C. and L.R. Klein (1999), “Infrastructure and Productivity: A Nonlinear Approach”, Journal of Econometrics 92, 47–74.
Égert, B. and Koźluk, T. and Sutherland, D. (2009), “Infrastructure and Growth: Empirical Evidence”, OECD Economics Department Working Papers No. 685, OECD Publishing, Paris. https://doi.org/10.1787/18151973
Evans, P. and G. Karras (1994), "Are Government Activities Productive? Evidence from a Panel of U.S. States," The Review of Economics and Statistics, MIT Press, vol. 76(1), pages 1-11.
Fernald, J. (1999): "Roads to Prosperity? Assessing the Link between Public Capital and Productivity", American Economic Review, 89(3), 619-638. https://www.aeaweb.org/articles/pdf/doi/10.1257/aer.89.3.619
Fourie, J. (2006) “Economic Infrastructure: A Review of Definitions, Theory and Empirics”, South African Journal of Economics Vol. 74:3 September 2006.
Gemmell, N. (2001), "Fiscal Policy in a Growth Framework," WIDER Working Paper Series DP2001-84, World Institute for Development Economic Research (UNU-WIDER). https://www.wider.unu.edu/sites/default/files/dp2001-84.pdf
Giandrea, M., R. Kornfeld, P. Meyer and S. Powers (2021), “Alternative Capital Asset Depreciation Rates for U.S. Capital and Multifactor Productivity Measures”, BLS Working Papers, Working Paper 539.
Gramlich, E. (1994) “Infrastructure Investment: A Review Essay”. Journal of Economic Literature, 32, 1176-1196. https://www.jstor.org/stable/2728606
Grice, J. (2016), “National accounting for infrastructure”, Oxford Review of Economic Policy, Volume 32, Number 3, 2016, pp. 431–445.
Hurlin (2006) “Network effects of the Productivity of Infrastructure in Developing Countries”, World Bank Policy Research Working Paper 3808, January 2006.
IMF (2015), “Making public investment more investment”, IMF Policy paper series, https://doi.org/10.5089/9781498344630.007
IMF (2019), Estimating the stock of public capital for 170 countries, Methodological note for the Investment and Capital Stock Dataset, https://www.imf.org/external/np/fad/publicinvestment/pdf/csupdate_aug19.pdf
Jiwattanakulpaisarn, P., Noland, R. B., and Graham, D. J. (2012) “Marginal productivity of expanding highway capacity”, Journal of Transport Economics and Policy, 46(3), 333–347.
Kamps, C. (2006), “New estimates of government net capital stocks for 22 OECD countries 1960-2001” IMF Staff Papers Vol. 53, No. 1 (2006), pp. 120-150.
Kornfeld, R. and B. Fraumeni (2022) “How Should We Measure Infrastructure? The Case of Highways and Streets”, NBER Working Paper no. 30045, https://www.nber.org/papers/w30045.
Martin, J. (2019), “Experimental comparisons of infrastructure across Europe”, Office for National Statistics, https://www.ons.gov.uk/releases/developingnewstatisticsofinfrastructureaugust2018.
Mas, M. and Maudos, J. and Pérez, F. and Uriel, E.(1996): “Infrastructure and Productivity in the Spanish Regions”, Regional Studies, 30, 641-50, https://doi.org/10.1080/00343409612331349938
Melo, P., D. Grahanm and R. Brage-Ardao (2013), “The productivity of transport infrastructure investment: A meta-analysis of empirical evidence”, Regional Science and Urban Economics 43 (2013) 695–706.
Moyo, B. (2013) “Public infrastructure quality and manufacturing productivity in Africa: A firm level analysis”, Energy Policy 61 (2013), 1063–1070.
Mourougane, A., et al. (2016), "Can an Increase in Public Investment Sustainably Lift Economic Growth?", OECD Economics Department Working Papers, No. 1351, OECD Publishing, Paris, https://doi.org/10.1787/a25a7723-en.
Munnell, A.H. (1990), “Why has Productivity Declined? Productivity and Public Investment”, New England Economic Review, Federal Reserve Bank of Boston, 3-22.
OECD (2009), Measuring Capital - OECD Manual 2009: Second edition, OECD Publishing, Paris, https://doi.org/10.1787/9789264068476-en.
OECD (2017), Getting Infrastructure Right: A framework for better governance, OECD Publishing, Paris. http://dx.doi.org/10.1787/9789264272453-en
OECD (2021), OECD Implementation Handbook for Quality Infrastructure Investment: Supporting a Sustainable Recovery from the COVID-19 Crisis, https://www.oecd.org/finance/oecd-implementation-handbook-for-quality-infrastructure-investment.htm.
Otto,G. and Voss, G. (1994), “Public capital and private sector productivity”, Economic Record 70: 121–132.
Pionnier, P., B. Zinni and K. Baret (forthcoming), “Sensitivity analysis of capital and MFP measurement to asset depreciation patterns and initial capital stock estimates”, OECD Statistics Working Papers, forthcoming.
Roberts, M., Deichmann, U., Fingleton, B., and Shi, T. (2012) “Evaluating China’s road to prosperity: A new economic geography approach”, Regional Science and Urban Economics, 42(4), 580–594.
Romp, W. and J. de Haan (2007), “Public Capital and EconomicGrowth: A Critical Survey”, Perspektiven der Wirtschaftspolitik2007 8 (Special Issue): 6–52.
Schwartz, G., Fouad, M., Hansen, T. and G. Verdier (2020) “Well Spent: How Strong Infrastructure Governance Can End Waste in Public Investment”, Washington, DC: International Monetary Fund.
Schwellnus, C. and Arnold, J. (2008), “Do Corporate Taxes Reduce Productivity and Investment at the Firm Level? Cross-Country Evidence from the Amadeus Dataset”, CEPII Working Paper No 2008-19, http://www.cepii.fr/PDF_PUB/wp/2008/wp2008-19.pdf
Shanks, S., and Barnes, P. (2008). “Econometric modelling of infrastructure and Australia’s productivity”. Research Memorandum, Australia Productivity Commission, (08-01). https://www.pc.gov.au/research/supporting/econometric-modelling-infrastructure/economic-modelling-infrastructure.pdf
Shioji, E. (2001) “Public Capital and Economic Growth: A Convergence Approach”, Journal of Economic Growth, 6, 205-227.
Stephan, A. (2000), “Regional infrastructure policy and its impact on productivity: a comparison of Germany and France”, WZB Discussion Paper No. FS IV 01-02, https://www.econstor.eu/bitstream/10419/50972/1/332465667.pdf
van de Ven, P. (2021) “Defining Infrastructure”, OECD Working Party in National Accounts, SDD/CSSP/WPNA(2021)1/REV1, https://www.oecd.org/officialdocuments/publicdisplaydocumentpdf/?cote=SDD/CSSP/WPNA(2021)1/REV1&docLanguage=En.
Vartia, L. (2008), “How do Taxes Affect Investment and Productivity? An Industry-Level Analysis of OECD Countries”, OECD Economic Department Working Papers No. 656, OECD Publishing, Paris. https://doi.org/10.1787/230022721067
Yeaple, S. and S. Golub (2007) “International Productivity Differences, Infrastructure, and Comparative Advantage”, Review of International Economics, 15(2), 223–242, 2007.
Competition
Acemoglu, D., P. Aghion, and F. Zilibotti (2006). “Distance to frontier, selection and economic growth”, Journal of the European Economic Association, (4:1), pp. 37-74.
Aghion, P., N. Bloom, R. Blundell, R. Griffith and P. Howitt (2005), “Competition and Innovation: An Inverted-U Relationship”, The Quarterly Journal of Economics, Vol. 120, No. 2 (May, 2005), pp. 701-728.
Aghion, P. and Howitt, P. (2006). “Joseph Schumpeter lecture: Appropriate growth policy: A unifying framework”, Journal of the European Economic Association, (4:2-3), pp. 269-314.
Alon, T., D. Berger, R. Dent, B. Pugsley (2018), “Older and slower: The startup deficit’s lasting effects on aggregate productivity growth”, Journal of Monetary Economics 93 (2018) 68–85.
Akcigit, Ufuk, and Sina T. Ates. 2021. "Ten Facts on Declining Business Dynamism and Lessons from Endogenous Growth Theory." American Economic Journal: Macroeconomics, 13 (1): 257-98.
Amiti, M., and J. Konings (2007), "Trade Liberalization, Intermediate Inputs, and Productivity: Evidence from Indonesia", American Economic Review, 97 (5): 1611-1638.
Anderton, R., B Di Lupidio and B. Jarmulska (2019), “ Product market regulation, business churning and productivity: evidence from the European Union countries”, ECB Working Paper Series, No 2332.
Andrews, D., C. Criscuolo and P. Gal (2016), "The Best versus the Rest: The Global Productivity Slowdown, Divergence across Firms and the Role of Public Policy", OECD Productivity Working Papers, No. 5, OECD Publishing, Paris, https://doi.org/10.1787/63629cc9-en.
Arnold, J., G. Nicoletti and S. Scarpetta (2011), “Regulation, resource reallocation and productivity growth”, EIB papers, Volume16 N°1, 2011.
Autor, D., L. F. Katz, C. Patterson, and J.Van Reenen, “The Fall of the Labor Share and the Rise of Superstar Firms”, The Quarterly Journal of Economics, Volume 135, Issue 2, May 2020, Pages 645–709, https://doi.org/10.1093/qje/qjaa004.
Aw, B. Y., S. Chung and M. Roberts (2002), “Productivity, output and failure: a comparison of Taiwanese and Korean manufactures”. NBER Working Paper no. 8766, http://www.nber.org/papers/w8766.
Backus, M. (2020), “Why is productivity correlated with competition?”, Econometrica, 88(6), 2415-2444.
Bajgar, M., G. Berlingieri, S. Calligaris, C. Criscuolo, and J. Timmis (2019), "Industry Concentration in Europe and North America", OECD Productivity Working Papers, No. 18, OECD Publishing, Paris, https://doi.org/10.1787/2ff98246-en.
Barseghyan, L. (2008), “Entry costs and cross-country differences in productivity and output”, Journal of Economic Growth , June 2008, Vol. 13, No. 2 (June 2008), pp. 145- 167.
Battiati, C, C. Jona-Lasinio, E. Marvasi and S. Sopranzetti (2021), “Market power and productivity trends in the European economies: A macroeconomic perspective”, Working Paper 1/2021, LUISS University, January 18, 2021.
Bighelli, T., F. Di Mauro, M. Melitz, J. Marc and M. Mertens (2021), “European firm concentration and aggregate productivity”, IWH Discussion Papers, No. 5/2021, Leibniz-Institut für Wirtschaftsforschung Halle (IWH), Halle (Saale), http://nbn-resolving.de/urn:nbn:de:gbv:3:2-136056.
Bourlès, R., G. Cette, J. Lopez, J. Mairesse, and G. Nicoletti (2013), “Do Product Market Regulations in Upstream sectors Curb Productivity Growth? Panel Data Evidence for OECD Countries,” Review of Economics and Statistics, 95, 1750–68, December 2013.
Buccirossi, P., L. Ciari, T. Duso, G. Spagnolo, and C. Vitale (2013), “Competition Policy and Productivity Growth: an Empirical Assessment”, Review of Economics and Statistics, October 2013, 95(4): 1324–1336.
Bustos, P. (2011), “Trade Liberalization, Exports and Technology Upgrading: Evidence on the Impact of MERCOSUR on Argentinean Firms”, American Economic Review 101 (February 2011): 304–340.
Calligaris, S., C. Criscuolo and L. Marcolin (2018), "Mark-ups in the digital era", OECD Science, Technology and Industry Working Papers, No. 2018/10, OECD Publishing, Paris, https://doi.org/10.1787/4efe2d25-en.
Calvino, F., C. Criscuolo and R. Verlhac (2020), “Declining business dynamism: Structural and policy determinants”, OECD Science, Technology and Industry Policy Paper, Vol. 94, https://www.oecd-ilibrary.org/science-and-technology/declining-business-dynamism_77b92072-en.
Cette, G., J. López and J. Mairesse (2016), “Market Regulations, Prices, and Productivity”, American Economic Review: Papers & Proceedings 2016, 106(5): 104–108, http://dx.doi.org/10.1257/aer.p20161025.
Cette, G., J. López and J. Mairesse (2017), “Upstream Product Market Regulations, ICT, R&D and Productivity”, Review of Income and Wealth, Series 63, Supplement 1, February 2017, DOI: https://doi.org/10.1111/roiw.12252.
Cheshire, P., C. Hilber, and I. Kaplanis (2015), “Land use regulation and productivity—land matters: evidence from a UK supermarket chain”, Journal of Economic Geography, Volume 15, Issue 1, January 2015, Pages 43–73, https://doi.org/10.1093/jeg/lbu007
CMA, Competition & Markets Authority, “Productivity and Competition – A summary of the evidence”, CMA 45, July 2015.
Conway, P., D. De Rosa, G. Nicoletti and F. Steinar (2006), “Product Market Regulation and Productivity Convergence”, OECD Economic Studies No.43, 2006/2.
Covarrubias, M., G. Gutiérrez and T. Philippon (2020), “From Good to Bad Concentration? US Industries over the past 30 years”, NBER Macroeconomics Annual, 34(1), 1-46.
Crouzet, N. and J. Eberly (2021), “Intangibles, markups, and the measurement of productivity growth”, Journal of Monetary Economics 124S (2021) S92–S109.
Dall’Olio, A., M. Iootty, N. Kanehira and F. Saliola (2013), “Productivity Growth in Europe”, The World Bank, Policy Research Working Paper 6425.
De Loecker, J. (2011), “Product differentiation, multiproduct firms, and estimating the impact of trade liberalization on productivity”, Econometrica, 79(5), 1407-1451.
De Loecker, J., J. Eeckhout, and G. Unger, “The Rise of Market Power and the Macroeconomic Implications”, The Quarterly Journal of Economics, Volume 135, Issue 2, May 2020, Pages 561–644, https://doi.org/10.1093/qje/qjz041.
Decker, R. J. Haltiwanger, R. Jarmin and J. Miranda (2018), “Declining Dynamism, Allocative Efficiency, and the Productivity Slowdown”, American Economic Review: Papers & Proceedings 2017, 107(5): 322–326, https://www.aeaweb.org/articles?id=10.1257/aer.p20171020.
Disney, R., J. Haskel and Y. Heden (2003), “Restructuring and Productivity Growth in UK Manufacturing”, The Economic Journal, 113 (July), 666–694.
Eslava, M., J. Haltiwanger, A. Kugler and M. Kugler (2009), “Trade reforms and market selection: evidence from manufacturing firms in Colombia”, Review of Economic Dynamics 16 (2013) 135–158.
Fariñas, J. and S. Ruano (2005), “Firm productivity, heterogeneity, sunk costs and market selection”, International Journal of Industrial Organization 23 (2005) 505– 534.
Ganapati, S. (2021), “Growing Oligopolies, Prices, Output, and Productivity.” American Economic Journal: Microeconomics 2021, 13(3): 309–327, https://doi.org/10.1257/mic.20190029.
Gutiérrez G. and T. Philippon (2018), “How EU Markets Became More Competitive Than US Markets: A Study of Institutional Drift”, Working Paper no. 24700, NBER, Cambridge, MA.
Goldberg, P., A. Khandelwal, N. Pavcnik, and P. Topalova, Imported Intermediate Inputs and Domestic Product Growth: Evidence from India, The Quarterly Journal of Economics, Volume 125, Issue 4, November 2010, Pages 1727–1767, https://doi.org/10.1162/qjec.2010.125.4.1727.
Gourio, F., T. Messer and M. Siemer (2016), “Firm Entry and Macroeconomic Dynamics: A State-Level Analysis”, American Economic Review: Papers & Proceedings 2016, 106(5): 214–218 http://dx.doi.org/10.1257/aer.p20161052
Griffith, R. and R. Harisson (2004), "The link between product market reform and macro-economic performance," European Economy - Economic Papers 2008 - 2015 209, Directorate General Economic and Financial Affairs (DG ECFIN), European Commission.
Grullon, G., Y. Larkin, and R. Michaely (2019), “Are US Industries Becoming More Concentrated? ”, Review of Finance, 2019, pp. 697–743.
Haskel, J. (1991), “Imperfect competition, work practices and productivity growth”, Oxford Bulletin of Econmics and Statistics, 53, 3 (1991), 0305-9049.
Haskel, J., and R. Sadun (2012), “Regulation and UK retailing productivity: evidence from microdata”, Economica, 79(315), 425-448.
Haskel, J., and S.Westlake (2017), Capitalism without Capital: The Rise of the Intangible Economy, Princeton, NJ: Princeton University Press.
Holmes, T. and J. Schmitz (2010), “Competition and productivity: a review of evidence”, Annual Review of Economics 2, 619–642.
Hsieh, C. and E. Rossi-Hansberg (2019), “The Industrial Revolution in Services”, NBER Working Paper Series, Working Paper no. 25968, DOI 10.3386/w25968, Issue date June 2019.
Kato, A. (2009), “Product Market Competition and Productivity in the Indian Manufacturing Industry”, Journal of Development Studies, Vol. 45, No. 10, 1579–1593, November 2009.
Kegels, C. and J. van der Linden (2011), “Competitiveness, productivity, competition and structural reforms: the Belgian case”, Reflets et perspectives de la vie économique, 2011/3 Tome L | pages 27 à 51, https://www.cairn.info/revue-reflets-et-perspectives-de-la-vie-economique-2011-3-page-27.htm.
Lanau, S. and P. Topolova (2016), “The Impact of Product Market Reforms on Firm Productivity in Italy”, IMF Working Paper Series, WP/16/119.
Liu, E., A. Mian and A Sufi (2022), “Low interest rates, Market Power and Productivity Growth”, Econometrica, Vol. 90, No. 1 (January, 2022), 193–221.
Maican, F., and M. Orth (2015), “A dynamic analysis of entry regulations and productivity in retail trade”, International Journal of Industrial Organization, 40, 67-80.
Matsa, D. (2011), “Competition and Product Quality in the Supermarket Industry”, The Quarterly Journal of Economics Vol. 126, No. 3 (August 2011), pp. 1539-1591.
Nickell, S. (1996), “Competition and Corporate Performance,” Journal of Political Economy, 104, 724–46.
Nicoletti, G. and S. Scarpetta (2003), "Regulation, Productivity and Growth: OECD Evidence", OECD Economics Department Working Papers, No. 347, OECD Publishing, Paris, https://doi.org/10.1787/078677503357.
OECD (2021), Methodologies to measure market competition, OECD Competition Committee Issues Paper, https://oe.cd/mmmc
OECD (2022), “OECD Services Trade Restrictiveness Index: Policy Trends up to 2022”, https://issuu.com/oecd.publishing/docs/oecd_stri_policy_trends_up_to_2022.
Olley, S. and A. Pakes (1996), “The Dynamics of Productivity in the telecommunications equipment industry” Econometrica 64 (6), 1263–1298.
Palangkaraya, A., and J. Yong (2011), “Trade liberalisation, exit, and output and employment adjustments of Australian manufacturing establishments”, The World Economy, 34(1), 1-22.
Pavcnik, N. (2002), “Trade liberalization, exit, and productivity improvement: evidence from Chilean plants” Review of Economic Studies 69, 245–276.
Schivardi, F. and E. Viviano (2010), “Entry Barriers in Retail Trade”, The Economic Journal, Volume 121, Issue 551, 1 March 2011, Pages 145–170, https://doi.org/10.1111/j.1468-0297.2009.02348.x.
Sutton, J. (1991) Sunk Costs and Market Structure, The MIT Press.
Syverson, C. (2004) “Market Structure and Productivity: A Concrete Example”, Journal of Political Economy 112 (6): 1181–222.
Syverson, C. (2011) “What determines productivity?” Journal of Economic Literature 2011, 49:2, 326–365.
Tambe, P., D. Rock, L. Hitt and E. Brynjolfsson (2021), “Digital Capital and Superstar Firms”, Hutchins Center Working Paper no. 73, March 2021, https://www.brookings.edu/research/digital-capitaland-superstar-firms.
Van Reenen, J. (2011), “Does competition raise productivity through improving management quality?”, International Journal of Industrial Organization, 29 (2011) 306–316.
Van Reenen, J. (2018), “Increasing Differences Between Firms: Market Power and the Macro-Economy.” CEP Discussion Paper no. 1576, Centre for Economic Performance, London.
Globalisation
Abizadeh, S. and Pandey, M. (2009), “Trade openness, Structural and Total Factor Productivity”, International Economic Journal, p545-559, https://doi.org/10.1080/10168730903372273
Acemoglu, D. (2008), Introduction to modern economic growth. Princeton, NJ: Princeton University Press.
Aghion, P., Bloom, N., Blundell, R., Griffith, R. and Howitt, P. (2005), “Competition and Innovation: An Inverted-U Relationship”. The Quarterly Journal of Economics, Vol. 120, No. 2 (May, 2005), pp. 701-728, http://www.jstor.org/stable/25098750
Aghion, P., Burgess, R., Redding, S. and Zilibotti, F. (2006), “The Unequal Effects of Liberalization: Evidence from Dismantling the License Raj in India”. NBER Working Paper Series, Working Paper 12031, http://www.nber.org/papers/w12031.
Aghion, P. and Howitt, W.H. (2008), The Economics of Growth, https://mitpress.mit.edu/books/economics-growth.
Aitken, Brian J., and Harrison, A. E. (1999) , “Do domestic firms benefit from direct foreign investment? Evidence from Venezuela”, American Economic Review, vol 89, pp. 605–18
Alcalá, F. and Ciccone, A. (2004) “Trade and productivity”. The Quarterly Journal of Economics, 119, pp. 612-645, https://doi.org/10.1162/0033553041382139.
Almeida, R. (2007), “The labor market effects of foreign owned firms”. Journal of International Economics, 72(1), pp. 75–96.
Azman-Saini, W. N. W., A. Z. Baharumshah and S. H. Law (2010a), “Foreign Direct Investment, Economic Freedom and Economic Growth: International Evidence”, Economic Modelling, 27 (5), pp. 1079–89.
Azman-Saini, W. N. W., Law, S. H. and Ahmad, A. H. (2010b), “FDI and Economic Growth: New Evidence on the Role of Financial Markets”, Economics Letters, 107 (2), pp. 211–13.
Baldwin, R., Forslid, R. and Braconier, H. (2005). “Multinationals, Endogenous Growth, and Technological Spillovers: Theory and Evidence”. Review of International Economics. 13. 945-963.
Baldwin, R. and F. Robert-Nicoud (2014), “Trade-in-goods and Trade-in-tasks: An Integrating Framework”, Journal of International Economics, 92(1), 51–62. https://doi.org/10.1016/j.jinte co.2013.10.002
Baldwin, R. B. Yan (2014) “Global Value Chains and the Productivity of Canadian Manufacturing Firms”, Economic Analysis (EA) Research Paper Series, Catalogue no. 11F0027M — No. 090.
Baltabaev, B. Foreign (2014) “Direct Investment and Total Factor Productivity Growth: New Macro-Evidence”, The World Economy, vol 37(2), p 311-334, https://doi.org/10.1111/twec.12115
Banga, K. (2022), “Impact of Global Value Chains on Total Factor Productivity: The case of Indian Manufacturing”, Review of Development Economics, 00, 1–32. https://doi.org/10.1111/rode.12867
Barry, F., Görg, H. and Strobl, E. (2005), “Foreign direct investment and wages in domestic firms in ireland: Productivity spillovers versus labour market crowding out”. International Journal of the Economics of Business, pp. 67–84.
Banerjee, A. V. and Esther, D. (2005). Growth Theory through the Lens of Development Economics, Handbook of Economic Growth, in: Philippe Aghion and Steven Durlauf (ed.), Handbook of Economic Growth 1(1), chapter 7, 473-552.
Beugelsdijk, S., Hennart, JF., Slangen, A. et al. (2010) “Why and how FDI stocks are a biased measure of MNE affiliate activity”. Journal of International Business Studies 41, 1444–1459. https://doi.org/10.1057/jibs.2010.29.
Belassa, B. (1982), “Development Strategies in Semi-Industrial Countries”. Oxford: Oxford University Press.
Bernard, A. B., J. B. Jensen, S. J. Redding, and P. K. Schott (2007), “Firms in International Trade,” Journal of Economic Perspectives, 21(3), 105–130.
Beverelli C., M. Fiorini and B. Hoekman (2017), “Services Trade Policy and Manufacturing Productivity: The Role of Institutions”, Journal of International Economics, Volume 104, 2017, Pages 166-182, https://doi.org/10.1016/j.jinteco.2016.11.001.
Bitzer, J. and Gorg, H. (2009), “Foreign Direct Investment, Competition and Industry Performance”, The World Economy, 32 (2), pp. 221–33.
Central Bank of Sri Lanka (2014), Annual report, Central Bank of Sri Lanka, Colombo.
Chen, B.L. (1999). “Trade Openness and Economic Growth: Evidence in East Asia and Latin America”. Journal of Economic Integration, 14(2): 265–295.
Chiarvesio, M., Di Maria, E., & Micelli, S. (2010), “Global Value Chains and Open Networks: The case of Italian Industrial Districts”, European Planning Studies, 18(3), 333–350. https://doi.org/10.1080/09654 31090 3497637.
Chirinko, R.S. and Mallick, D. (2007), “The Fisher/Cobb-Douglas paradox, factor shares, and cointegration”. CESifo Working Paper, No. 1998, Center for Economic Studies and ifo Institute (CESifo), Munic.
Constantinescu, C. and Mattoo, A. and Ruta, M.. (2017). “Does vertical specialization increase productivity?” World Bank Policy Research Working Paper No. 7978, Washington, DC: World Bank.
Égert, B. (2017), “Regulation, institutions and productivity: New macroeconomic evidence from OECD countries”, OECD Economics Department Working Papers No. 1393, OECD Publishing, Paris. https://dx.doi.org/10.1787/579ceba4-en.
Égert, B. (2016), “Regulation, Institutions, and Productivity: New Macroeconomic Evidence from OECD Countries”, American Economic Review: Papers & Proceedings 2016, 106(5): 109-113, https://www.jstor.org/stable/43860997.
Frankel, J. A. (2000). “Assessing the efficiency gains from further liberalization”, KSG Faculty Paper No. RWP01-030. http://ssrn.com/abstract=284009.
Formai, S. and F Vergara Caffarelli (2016), “Quantifying the productivity effects of global sourcing”, Working paper Banca d’Italia No. 1075, July, en_tema_1075.pdf (bancaditalia.it).
Grossman, G.M. and Helpman, E. (1991) Innovation and Growth in the Global Economy (Cambridge, MA and London: MIT Press).
Herzer, D. (2012), “How Does Foreign Direct Investment Really Affect Developing Countries’ Growth”, Review of International Economics, 20 (2), pp. 396–414.
Herzer, D. (2017), “Foreign direct investment and total factor productivity in Bolivia”, Applied Economic Letters, vol 34, P 399-403, https://doi.org/10.1080/13504851.2016.1197359.
Heyman, F. and Sjöholm, F. and Tingvall, P.G. (2007), “Is there really a foreign ownership wage premium? evidence from matched employer–employee data”. Journal of International Economics, 73(2), pp. 355-376.
Kasahara, H., and B. Lapham (2013): “Productivity and the Decision to Import and Export: Theory and Evidence,” Journal of International Economics, 89(2), 297–316.
Kordalska, A., J. Wolszczak-Derlacz, and A. Parteka. (2016). “Global value chains and productivity gains: A cross-country analysis.” Collegium of Economic Analysis Annals, 41, pp. 11–28.
Kummritz, V. (2016). “Do global value chains cause industrial development?”, Centre for Trade and Economics Integration Working Paper No. 2016-01. Geneva: Centre for Trade and Economics Integration.
Liargovas, P.G. and Konstantinos, S. S. (2012), “Foreign Direct Investment and Trade Openness: The Case of Developing Economies”, Social Indicators Research vol 106, pp. 323-331, https://link.springer.com/article/10.1007/s11205-011-9806-9.
Madsen, J.B. (2007), “Technology spillover through trade and TFP convergence: 135 years of evidence for the OECD countries”, Journal of International Economics vol. 72, p 464–480, https://doi.org/10.1016/j.jinteco.2006.12.001.
Makoni, Patricia Lindelwa. (2018). “FDI and Trade Openness: The Case of Emerging African Economies”. Journal of Ac-counting and Management 8, pp. 141–52.
Mayoshi, M. and Mudiyanselage, R. and Epuran, G. and Tescas, B. (2021), “Causal Links between Trade Openness and Foreign Direct Investment in Romania”. Journal of Risk and Financial Management 14: 90. https://doi.org/10.3390/jrfm14030090.
Miller, S.M. and Upadhyay, M.P. (2000), “The effects of openness, trade orientation, and human capital on total factor productivity”, Journal of Development Economics Vol. 63, pp. 399–423, https://doi.org/10.1016/S0304-3878(00)00112-7.
Pittiglio, R. and Reganati, F. and Sica, E. (2015) Do multinational enterprises push up the wages of domestic firms in the italian manufacturing sector? The Manchester School, 83(3), pp. 346–378.
OECD and Statistics Finland (2021), Measuring Trade in Value Added for Finland, OECD Publishing, Paris.
OECD (2022), New approaches to measure TiVA indicators in the United Kingdom, OECD Publishing.
Obstfeld, M. & Rogoff, K. (1996) Foundations of International Macroeconomics (Cambridge and New York: Cambridge University Press).
Sahoo, P. (2006). “Foreign Direct Investment in South Asia: Policy, trends, Impact and Determinants”. ADB institute discussion Paper, No. 56. https://www.adb.org/sites/default/files/publication/156693/adbi-dp56.pdf.
Squalli, J., & Wilson, K. (2009), “Openness and access”. Applied Economics, 41(3), 363–379.
Urata, S., and Y. Baek. (2020). “Does participation in global value chains increase productivity? An analysis of trade in value added data.” Thailand and The World Economy, 38(1), pp. 1–28.
Urata, S. and Baek, Y. (2021), “Does GVC Participation Improve Firm Productivity? A study of Three Developing Asian Countries”, Asian Development Bank Institute, ADBI Working Paper Series No. 1245, https://www.adb.org/sites/default/files/publication/692486/adbi-wp1245.pdf.
Wagner J. (2012), “International Trade and Firm Performance: A Survey of Empirical Studies since 2006”, Review of World Economics, 148(2), 235–267.https://doi.org/10.1007/s1029 0-011-0116-8.
Woo,J. (2009), “Productivity growth and technological diffusion through foreign direct investment”, Economic Inquiry vol 47, pp. 226-248, https://doi.org/10.1111/j.1465-7295.2008.00166.x.
Xu, B. (2000), “Multinational Enterprises, Technology Diffusion, and Host Country Productivity Growth”, Journal of Development Economics, Vol. 62, p. 477-493.
Financial development
Adalet McGowan, M., D. Andrews, and V. Millot (2017) “The Walking Dead? Zombie Firms and Productivity Performance in OECD Countries”, OECD Economics Department Working Papers, No. 1372, OECD Publishing, Paris.
Andrews, D. and F. Cingano (2014), “Public Policy and Resource Allocation: Evidence from Firms in OECD Countries”, OECD Economics Department Working Papers, No. 996, OECD Publishing, Paris.
Andrews, D., C. Criscuolo, and C. Menon (2014), “Do Resources Flow to Patenting Firms? Cross-country Evidence from Firm Level Data”, OECD Economics Department Working Papers, No. 1127, OECD Publishing, Paris.
Beck, T., R. Levine and N. Loayza (2000), “Finance and the Sources of Growth” Journal of Financial Economics 58: 261-300.
Benhabib, J. and M. Spiegel (2000), “The Role of Financial Development in Growth and Investment”, Journal of Economic Growth 5: 341-360.
Brown, J., G. Martinsson, and B. Petersen (2012), “Do Financing Constraints Matter for R&D?” European Economic Review 56: 1512-1529.
Caballero, R., T. Hoshi and A. Kashyap (2008), “Zombie Lending and Depressed Restructuring in Japan”, American Economic Review 98: 1943-1977.
Cournède, B. and O. Denk (2015), “Finance and Economic Growth in OECD and G20 Countries”, OECD Economics Department Working Papers, No. 1223. OECD Publishing, Paris.
Demirgüç-Kunt, A. and R. Levine (1999) “Bank-based and Market-base financial systems. Cross-country comparisons”, World Bank Policy Research Woring Paper 2143.
Heil, M. (2017), "Finance and productivity: A literature review", OECD Economics Department Working Papers, No. 1374, OECD Publishing, Paris, https://doi.org/10.1787/41194fea-en.
King, R.G. and Levine, R. (1993) “Finance and growth: Schumpeter might be right”, The Quarterly Journal of Economics 108(3): 717–737.
Levine, R., and Zervos, S. (1998) “Stock markets, banks, and economic growth”, American economic review, 537-558.
Lochner, L. and A. Monge-Naranjo (2012), “Credit Constraints in Education.” Annual Review of Economics 4: 225-256.
Manning, M. (2003), “Finance Causes Growth: Can We Be So Sure?” Contributions to Macroeconomics 3: 1-22.
Midrigan, V. and D.Y. Xu (2014), “Finance and Misallocation: Evidence from Plant-Level Data.” American Economic Review 104: 422-458.
Rajan, R., and Zingales, L. (1998) “Financial dependence and growth”, American Economic Review, 88(3), 559-586.
Institutions
Abeberese, A., P. Barnwal, R. Chaurey and P. Mukherjee (2021) “Democracy and Firm Productivity: Evidence from Indonesia”, The Review of Economics and Statistics 2021; doi: https://doi.org/10.1162/rest_a_01077
Acemoglu, D. (2008) Introduction to Modern Economic Growth, Princeton, NJ: Princeton University Press.
Acemoglu, D., and S. Johnson (2005) “Unbundling Institutions”, Journal of Political Economy, 113, 949–995.
Acemoglu, D., J. A. Robinson, and S. Johnson. (2001) “The Colonial Origins of Comparative Development: An Empirical Investigation”, American Economic Review, 91, 1369–1401.
Acemoglu, D., and J. Robinson (2008) "Persistence of Power, Elites, and Institutions", American Economic Review, 98 (1): 267-93.
Acemoglu, D., S. Naidu, P. Restrepo, and J. Robinson (2019) “Democracy does cause growth”, Journal of Political Economy, 2019, vol. 127, no. 1.
Aghion, P. and Howitt, W.H. (2009) The Economics of Growth, The MIT Press, Cambridge, Massachusetts.
Aghion, P., A. Alesina and F. Trebbi (2007) “Democracy, Technology and Growth”, NBER Working Paper Series, Working Paper 13180, http://www.nber.org/papers/w13180.
Agostino, M., M. Di Tommaso, A. Nifo, L. Rubini and F. Trivieri (2020), “Institutional quality and firms’ productivity in European regions”, Regional Studies, Vol. 54, No. 9, 1275–1288.
Ajide, F. (2022) “Entrepreneurship and productivity in Africa: the role of institutions”, Journal of Sustainable Finance & Investment, 12:1, 147-168, DOI: 10.1080/20430795.2021.1939645.
Alexandre, F., P. Baçao and F. Veiga (2022) “The political economy of productivity growth”, European Journal of Political Economy, 2022.
Akhremenko, A., A. Petrov, and E. Yureskul (2019) “Institutions, Productivity Change, and Growth”, in Business Cycles in BRICS, edited by S. Smirnov, A. Ozyldirim and P. Picchetti, Springer.
Aslam (2020) “The hotly debate of human capital and economic growth: why institutions may matter?”, Quality & Quantity (2020) 54:1351–1362.
Azam, M. and C. Emirullah (2014) “The role of governance in economic development”, International Journal of Social Economics, Vol. 41 No. 12, 2014, pp. 1265-1278.
Bhagwati, J. (2002) “Democracy and Development: Cruel Dilemma or Symbiotic Relationship?”, Review of Development Economics, 6(2), 151–162, 2002.
Barro, R. (1996) “Determinants of Economic Growth: A cross-country empirical study”, NBER Working Paper Series, No. 5698.
Beck T., G. Clarke, A. Groff, P. Keefer and P. Walsh (2001) “New Tools in Comparative Political Economy: The Database of Political Institutions”, The World Bank Economic Review, Volume 15, Issue 1, June 2001, Pages 165–176, https://doi.org/10.1093/wber/15.1.165.
Berggren, N. and C. Bjørnskov (2022) “Academic freedom, institutions, and productivity”, Southern Economic Journal, 88, pp. 1313–1342.
Bjørnskov, C. and N. J. Foss (2010) “Do Economic Freedom and Entrepreneurship Impact Total Factor Productivity?” SMG Working Paper No. 8/2010, http://dx.doi.org/10.2139/ssrn.1683965
Brown, J., G. Martinsson, and B. Petersen (2012), “Do Financing Constraints Matter for R&D?” European Economic Review 56: 1512-1529.
Buera, F., J. Kaboski, and Y. Shin (2011), “Finance and Development: A Tale of Two Sectors”, American Economic Review 101: 1964-2002.
Cavallo A. and E. Cavallo (2010) “Are crises good for long-term growth? The role of political institutions”, Journal of Macroeconomics 32 (2010) 838–857.
Charron, N., L. Dijkstra and V. Lapuente (2014) “Regional Governance Matters: Quality of Government within European Union Member States”, Regional Studies, 48:1, 68-90, DOI: 10.1080/00343404.2013.770141.
Ciccone, A. and E. Pappaionau (2007) “Red tape and delayed entry”, Journal of the European Economic Association, April–May 2007 5(2–3):444–458.
Cournède, B. and O. Denk (2015), “Finance and Economic Growth in OECD and G20 Countries”, OECD Economics Department Working Papers, No. 1223. OECD Publishing, Paris.
Das, K. and T. Quirk (2016) “Which Institutions Promote Growth? Revisiting the Evidence”, Economic Papers, Vol. 35, No. 1, March 2016, 37–58.
de Haan, J., Lundstrom, S., Sturm, J. (2006) “Market-oriented institutions and policies and economic growth: A critical survey”, Journal of Economic Surveys, volume 20, pages 157–191.
Djankov, S., R. La Porta, F. Lopez-de-Silanes, and A. Shleifer (2003) “Courts”, Quarterly Journal of Economics, 118, 453–517.
Doyle E. and Inmaculada Martínez-Zarzoso (2011) “Productivity, Trade, and Institutional Quality: A Panel Analysis”, Southern Economic Journal 2011, 77(3), 726–752.
Easterly, W. (2005) “National policies and economic growth: a reappraisal”, in P. Aghion and S. Durlauf (eds.), Handbook of Economic Growth, Vol. 1 (pp. 1015–1059). Amsterdam: Elsevier.
Easterly, W. and R. Levine (2016) “The European origins of economic development”, Journal of Economic Growth (2016) 21:225–257.
Eckstein, H. and T. R. Gurr (1975), Patterns of Authority: A Structural Basis for Political Inquiry, A Wiley-Interscience publication, Comparative studies in behavioral science, ISSN 0190-1079.
Égert, B. (2016) “Regulation, Institutions, and Productivity: New Macroeconomic Evidence from OECD Countries”, American Economic Review: Papers & Proceedings 2016, 106(5): 109–113.
Freedom House (2022) “Freedom in the World 2022 Methodology”, https://freedomhouse.org/reports/freedom-world/freedom-world-research-methodology
Gwartney, J., Lawson, R., Hall, J., Murphy, R. (2021) “Economic Freedom Of The World: 2021 Annual Report”. Fraser Institute. Vancouver, BC, Dataset downloadable at: https://www.fraserinstitute.org/sites/default/files/economic-freedom-of-the-world-2021.pdf
Glaeser, E., R. La Porta, F. Lopez-de-Silanes, and A. Shleifer (2004) “Do Institutions Cause Growth?” Journal of Economic Growth, 9, 271–303.
Heil, M. (2017), "Finance and productivity: A literature review", OECD Economics Department Working Papers, No. 1374, OECD Publishing, Paris, https://doi.org/10.1787/41194fea-en.
Issar, A., J.J. Lim and S. Mohapatra (2017) “Institutional Quality and International Differences in Firm Productivity”, Indian Institute of Management, W.P. No. 2017-02-01, February 2017.
Jellema, J. and Roland, G. (2011) “Institutional clusters and economic performance”, Journal of Economic Behavior and Organization 79: 108–132.
Jong-A-Pin (2009) “On the measurement of political instability and its impact on economic growth”, European Journal of Political Economy 25 (2009) 15–29.
Kar, S., A. Roy and K. Sen (2019) “The double trap: Institutions and economic development”, Economic Modelling 76 (2019) 243–259.
Karimi, M.S., and Daiari, E.H. (2018) “Does Institutions Matter for Economic Development? Evidence for ASEAN Selected Countries”, Iranian Economic Review, 22(1), 1-20.
Kaasa, A. (2016) “Social Capital, Institutional Quality and Productivity: Evidence from European Regions”, Economics and Sociology, Vol. 9, No 4, pp. 11-26. DOI: 10.14254/2071-789X.2016/9-4/1.
Kaufmann, D., A. Kraay, and M. Mastruzzi (2010) “The Worldwide Governance Indicators - Methodology and Analytical Issues”, Policy Research Working Paper 5430, The World Bank, Development Research Group.
Kim, D. H., Y.C. Wu and S. H. Lin (2018) “Heterogeneity in the effects of government size and governance on economic growth”, Economic Modelling 68 (2018) 205–216.
Kurtz, M. and A. Schrank (2007) “Growth and governance: models, measures and mechanisms”, Journal of Politics, volume 69, issue 2, pages 538-554.
Lasagni, A., A. Nifo and G. Vecchione (2015) “Firm productivity and institutional quality: Evidence from Italian Industry”, Journal of Regional Science, Vol. 55, No. 5, 2015, pp. 774–800.
Law, S.H., Lim, T.C., and Ismail, N.W. (2013) “Institutions and economic development: A Granger causality analysis of panel data evidence”, Economic Systems, 37(4), 610-624.
Law, S. H. and N. Singh (2014) “Does Too Much Finance Harm Economic Growth?”, Journal of Banking and Finance 41: 36-44.
Lloyd, P. and L. Cassey (2016) “A review of the recent literature on the institutional economics analysis of the long-run performance of nations”, Journal of Economic Surveys (2018) Vol. 32, No. 1, pp. 1–22.
Marshall, M. (2020) “Political Regime Characteristics and Transitions, 1800-2018. Dataset Users’ Manual”, Polity Project POLITY5, Center for Systemic Peace, April 2020, http://www.systemicpeace.org/inscr/p5manualv2018.pdf
Mauro, P. (1995) “Corruption and growth”, Quarterly Journal of Economics, 110(3), 681-712.
Naanwaab, C. and O. A. Yeboah (2013) “Determinants of productivity in Africa: The role of economic freedom”, Journal of Development and Agricultural Economics, Vol. 5(10), pp. 390-402.
Ngo, M. and L. Nguyen (2020) “Economic Growth, Total Factor Productivity, and Institution Quality in Low-Middle Income Countries in Asia”, Journal of Asian Finance, Economics and Business Vol 7 No 7 (2020) 251 – 260.
North, D.C. (1990) Institutions, Institutional Change, and Economic Performance, Cambridge University Press.
Ogilvie, S. and Carus, A.W. (2014) “Institutions and economic growth in historical perspective”, in Handbook of Economic Growth, Vol. 2A. Amsterdam: Elsevier.
Persson, Torsten, and Guido Tabellini (2009) "Democratic Capital: The Nexus of Political and Economic Change", American Economic Journal: Macroeconomics, 1 (2): 88-126.
PRS (2013) “International Country Risk Guide – Methodology”, https://www.prsgroup.com/wp-content/uploads/2012/11/icrgmethodology.pdf
Rodríguez-Pose, A. and R. Ganau (2022) “Institutions and the productivity challenge for European regions”, Journal of Economic Geography (2022) 22, 1–25.
Rodrik, D. (2005) “Growth strategies”, in Handbook of Economic Growth, Volume 1A, Chapter 14, edited by Philippe Aghion and Steven N. Durlauf.
Rodrik, D., A. Subramanian and F. Trebbi (2004) “Institutions Rule: The Primacy of Institutions over Geography and Integration in Economic Development”, Journal of Economic Growth, June 2004, Vol. 9, No. 2, pp. 131-165.
Rodrik, D. and R. Wacziarg (2005) “Do Democratic Transitions Produce Bad Economic Outcomes?”, American Economic Review, Papers and Proceedings, Vol 95, No. 2.
Rose-Ackerman, S. (1999) “Corruption and government”, Causes, consequences, and reform. Cambridge, UK: Cambridge University Press.
Tavares, J., Wacziarg, R. (2001) “How democracy affects growth”, European Economic Review 45, 1341–1378.
Uddin, A., A. Ali and M. Masih (2020) “Institutions, human capital and economic growth in developing countries”, Studies in Economics and Finance, Vol. 38 No. 2, 2021, pp. 361-383.
Urbano, D., S. Aparicio and D. Audretsch (2019) “Twenty-five years of research on institutions, entrepreneurship, and economic growth: what has been learned?”, Small Business Economies, (2019) 53:21–49.
Venard, B. (2013) “Institutions, Corruption and Sustainable Development”, Economics Bulletin, Volume 33, Issue 4.
Notes
← 1. The EU14 included Austria, Belgium, Denmark, Finland, France, Germany, Greece, Ireland, Italy, the Netherlands, Portugal, Spain, Sweden and the United Kingdom.
← 2. Measured as the difference in the shares of high-skilled workers at the most productive firms relative to medium performers.
← 3. Import penetration is defined imports expressed as a fraction of imports minus exports plus sales.
← 4. Nickell (1996) defined rents as profits before tax plus depreciation plus interest payments minus the cost of capital multiplied by capital stock. Disney et al. (2003) defined the measure of rents as net output less material, capital and labour costs, expressed as a proportion of net output.