Margarita Kalamova
OECD
AI and the Future of Skills, Volume 2
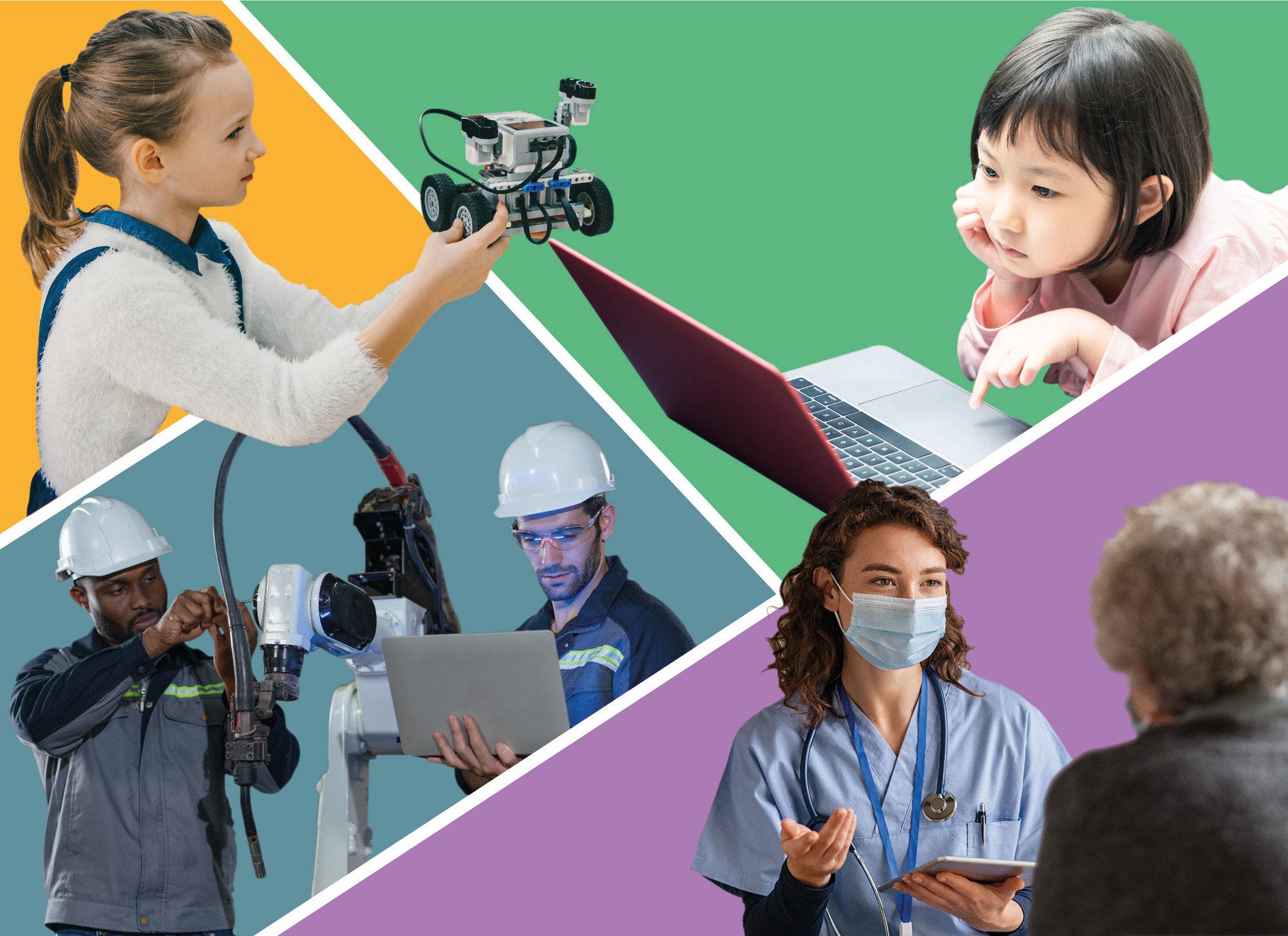
5. Assessing AI capabilities on occupational tests
Abstract
This chapter evaluates the capabilities of artificial intelligence (AI) in complex occupational tasks typical of real-world job settings. Using tasks from certification and licensing performance tests, the study aims to provide a more tangible assessment base than abstract constructs such as literacy and numeracy. Despite the clarity they offer, occupational tasks, given their complexity, pose methodological challenges in gathering expert judgements on AI’s proficiency. Two pilot studies, containing 13 tasks across six occupations, revealed AI’s aptitude in basic reasoning and language processing and limitations in nuanced and physically intricate activities. Expert feedback highlighted ambiguities in task descriptions and the difficulties of comparing AI and human skills. This chapter outlines the methodology, findings and implications of these assessments.
The AI Future of Skills (AIFS) project has extended the rating of artificial intelligence (AI) capabilities to complex occupational tasks taken from tests used to certify workers in different occupations. These tests present practical tasks that are typical in these occupations. As discussed in Chapter 4, this poses a clear advantage for gathering expert assessments on AI and robotics. Unlike assessments based on abstract constructs, such as general intelligence, broad content abilities (e.g. verbal, spatial, numerical abilities) or narrower abilities (e.g. perceptual speed, psychomotor abilities), occupational task evaluations provide meaningful insights into real-world scenarios and practical occupational behaviours. This offers a pragmatic and focused means to assess AI and robotics capabilities in specific occupational contexts.
The inherent complexity of these tasks means they differ from the questions in education tests used in the assessments discussed in Chapter 3. Occupational tasks require varied capabilities, often involving physical tasks, take place in real-world unstructured environments and are often unfamiliar to computer scientists. Consequently, the project had to develop different methods for collecting expert ratings of AI with such tasks.
The AIFS project carried out two exploratory studies on the use of performance tests of occupational tasks for assessing AI and robotics capabilities. The project selected 13 tasks from six occupations, which were presented in Chapter 4, for an exploratory assessment of AI and robotics performance on work tasks. The selected tasks represent some important elements of reasoning, language and sensory-motor capabilities, a diverse set of work contexts and different levels of complexity. This allows the project to test assessment methodologies in different set-ups. The two studies explored the use of two distinct online surveys, different response formats and different instructions for rating expected AI and robotics performance on the example occupational tasks.
The results of the exploratory studies showed that AI performs well in areas of basic language processing and reasoning, efficiently handling tasks like retrieving specific terminology and ensuring grammatical accuracy. However, challenges emerge when tasks demand depth and nuance, such as synthesising knowledge for product development or leading patient interactions. Complexities remain in physical dexterity, especially in intricate manual tasks and interaction with human body parts. Controlled environments amplify AI's capabilities, but unpredictable settings highlight its current limitations, underscoring the need for further advancements.
However, the results also revealed some methodological challenges in collecting expert judgements on AI capabilities with occupational tasks. The feedback from experts unveiled ambiguities in task descriptions, a lack of clarity regarding the assumptions and a need for more contextual information in the first study. The second study attempted to map AI capabilities against human job requirements, and while experts commended the initiative, they faced significant challenges in the rating process. A primary concern raised was the ambiguous categorisation of AI capabilities needed for tasks. Moreover, the measurement scale introduced in the survey further exacerbated the confusion. The survey’s structure also muddied the comparison between AI and humans, making it challenging for experts to assess AI’s proficiency in certain tasks.
This chapter will first describe the process of collecting expert judgement on performance tests of occupational tasks. It will then present and discuss the results of the two assessments. Finally, it will include some thoughts about the way forward.
Collecting expert judgement on performance tests of occupational tasks
The method for collecting expert judgement
Two different assessments within a spell of three months were carried out, each with a separate online survey. These were followed by a group discussion among computer scientists with the participation of two industrial-organisational psychologists. Each time, the participants took a week to complete the survey. During this period, they could access, re-access and modify their answers via an individualised link. In total, there were nine performance tests, containing 13 tasks, to rate.
A three-hour online group discussion took place a week after each of the online assessments. In each meeting, experts received detailed feedback on how the group rated AI and robotics abilities to take the various tests. Experts discussed the results, focusing on the performance tasks, on which there was some disagreement in the evaluation of AI and robotics performance. In addition, the experts provided feedback on the evaluation approach and described any difficulties in understanding and rating the survey questions.
In July 2022, the first exploratory study asked 12 experts to rate AI’s ability to carry out 13 occupational assignments. This aimed to collect first insights into the challenges that experts face in rating performance on the tasks and to develop corresponding solutions. The 13 occupational tasks covered diverse capabilities (e.g. reasoning, language and sensory-motor capabilities), occupations and working contexts. The materials describing the tasks varied in length and detail, which the project used to explore how different conditions for rating affect the robustness of the results.
In September 2022, a follow-up evaluation of the same tasks tested a new framing of the rating exercise. Experts rated potential AI performance with respect to several, pre-defined capabilities required for solving the task. The expectation was that linking occupational tasks to specific capability requirements would help experts abstract their evaluations from the concrete work context. They could thus focus more on general technological features needed for performing the task. A subsequent workshop with the experts elaborated the advantages and limitations of this approach.
Both exploratory studies followed a behavioural approach for collecting expert judgement. As described in Chapter 2, this approach relies on few experts who engage in in-depth discussions to arrive at a consensus judgement on a question. This aims to address questions in their complexity by considering different arguments and perspectives, and drawing on the best of these arguments to build a group judgement.
Developing the questionnaires
The first study contained 13 occupational tasks stemming from nine German and US performance tests for occupations.
For each occupational task, experts were first asked, “How confident are you that AI technology can carry out the task?”. The response options (“0% – No, AI cannot do it”; 25%; 50% – “Maybe”; 75%; “100% – Yes, AI can do it”; and “Don't know”) combined their confidence and rating of the capability of AI. Specifically, “0% – No, AI cannot do it” meant the expert was quite certain that AI cannot carry out the task, while 25% meant “AI probably cannot do it”. In answering this question, the study asked experts to have the final product/result in mind of that particular task, i.e. the assessment input/materials could be transformed to make them more “user friendly” for AI to carry out the task. The question aimed to understand whether AI can achieve the same results as humans independently of steps taken to achieve the results.
A second question asked: “Humans would typically execute a number of subtasks while carrying out the task. Which of the following subtasks do you think AI can carry out independently?”. This aimed to understand whether AI can take over certain work processes and complement humans at the workplace in that particular task.
Most experts provided detailed explanations of their responses to each of the two questions and each occupational task.
The survey gave experts detailed instructions that defined the parameters for evaluating the potential use of AI and robotics on the 13 occupational tasks. In making their judgement for each task, experts were asked to consider “current” computer techniques. These would be any available techniques addressed sufficiently in the literature whose capabilities and limitations can be roughly described. The intent was to include techniques whose capabilities have been demonstrated in research settings without worrying whether those techniques have been applied in any significant way. Experts could consider techniques that might need “reasonable advance preparation” to perform a particular occupational task. This advance preparation was to be considered applied research to prepare an existing technique with known capabilities for a new domain.
The follow-up study attempted to address some methodological issues encountered in the first study. Experts had pointed out that certain task descriptions lacked detail about the working context and boundary conditions for the tasks, requiring them to make speculative assumptions in their ratings. One task, for example, asked test takers to create a 3D solid model using computer-aided design software, without providing any information about the reference part (is it a cup, a car, etc.?) or how the task is to be carried out. To inform their judgements, some experts searched the Internet for explanations of work contexts. In particular, they sought work related to material sciences and engineering in technical occupations, and cosmetic and nursing procedures in the personal care industry.
To improve the task descriptions, some experts suggested the project should work with subject domain specialists and job analysts. The project would consider such collaboration in its explorations of task redesign, which is different stream of work from assessing AI and robotics capabilities. Instead, for the second study of occupational tasks, the project provided complementary videos and a revised job analysis of each task.
In the initial study, experts appeared to converge in their assessments regarding the capability needs of different tasks and the present proficiency of AI and robotics in these areas, which prompted the organisation of the subsequent study.
While the first survey had asked experts about their confidence that AI can carry out each specific occupational task, including a list of sub-steps, the second study asked them to rate the performance of AI on each task with regard to several categories of underlying capabilities. The categories of capabilities, 18 altogether, were borrowed from (McKinsey Global Institute, 2017[1]) (consult Annex Table 5.A.1 presenting the capability scales).
In making their judgement for each capability and each task, experts could choose between three performance levels defined for each capability. They could also rate the particular capability as not needed for AI and/or humans for carrying out the particular task. The OECD had selected the few capabilities (out of 18) considered most relevant for the execution of each particular occupational task. Finally, the experts could indicate any other essential capabilities for each occupational task which they considered missing from the list of capabilities pre-selected by the OECD.
The feedback was mixed, suggesting this approach might have felt forced or possibly that the scales the project used were not optimal. Experts acknowledged the project’s effort in outlining occupational tasks and capabilities but pinpointed challenges in capability ratings. The capability categories, derived from McKinsey’s framework, were deemed unclear and inconsistently structured. The measurement scales of the capabilities also faced scrutiny for their ambiguity, especially around the human‑level benchmark. Concerns arose regarding the questionnaire's design, especially its alignment between AI and human‑centred questions.
Evaluation of AI and robotics capabilities on tasks and subtasks
Average experts’ ratings of AI and robotics capabilities to carry out entire tasks
Figure 5.1 illustrates average measures of AI and robotics capabilities of carrying out the selected 13 occupational tasks. These measures are computed by taking the mean of the 12 experts’ responses to the question “How confident are you that AI technology can carry out the task?” for each of the 13 tasks. “Don’t know” responses were excluded from these calculations. The measures thus show experts’ average confidence that a certain task can be entirely automated by AI and/or robotics systems.
Figure 5.1. AI and robotics performance on entire task, by task format
The average confidence measures vary significantly – from 10-92% – reflecting the diversity of represented occupations, their varying capability requirements and formats of exam tasks. Two of the tasks are written exam questions of a knowledge-based nature, while the rest are performance-based practical tasks to assess various ability domains. Knowing that AI systems have super-human performance on information retrieval tasks, it is not surprising that ratings for the Dental Medical Assistant tasks are notably higher than for tasks that require precise dexterity (Cosmetologist or Specialist in Metal Technology) and/or advanced reasoning (Technical Product Designer Task 1). Further down, the chapter will look more closely into the breakdown of the 13 tasks and plausible conditions and constraints for automation.
Some task descriptions are more complex and detailed than others, describing multiple sub-steps and providing instructions, which may also affect expert ratings. Most tasks with shorter descriptions in Figure 5.1 are rated higher than tasks with lengthy descriptions. As one possible explanation, shorter descriptions convey false simplicity because the brief explanation of the task may miss key points. This might be the case with the Technical Product Designer Task 2, which contains no detail about the type of final product and instructions on what needs to be done, making it appear simpler to carry out than the thoroughly described Technical Product Designer Task 1.
Another possibility is that shorter descriptions happen to refer to “simpler” tasks. For instance, when rating two similar tasks in the cosmetology occupation, experts had only 19% confidence, on average, that an AI or robotics system can carry out the thoroughly described task (chemical waving). They had 35% confidence for the task with the short description (manicure). The higher rating for the task with a short description may reflect lower safety concerns and dexterity requirements, which indeed may make the task less demanding than the thoroughly described task involving a manipulation on a human head. It is difficult to draw conclusions about the potential bias in ratings arising from task descriptions. However, the project would need to carefully choose the right format and size of task descriptions for future assessments of occupational tasks.
Distribution of experts’ ratings of AI and robotics capabilities to carry out entire tasks
Figure 5.2 provides important insights into experts’ agreement on the various tasks. It shows the distribution of responses from 0% (“No, AI cannot do it”) to 100% (“Yes, AI can do it”), whereas 0% and 25% are counted as No-answers and 75% and 100% as Yes-answers. The figure includes the “Don’t know” answers as well. Following a simple majority rule – when seven or more experts provide the same No- or Yes-answer – a full consensus is reached on 9 of 13 tasks. Experts are confident about automating four tasks completely (those at the top of the figure). They are also confident that five other tasks (those at the bottom of the figure) are not fully feasible for AI and robotics systems yet. They disagree on the remaining four tasks: Technical Product Designer Task 2, Specialist in Metal Technology Task 2, Cosmetologist Task 2 and Office Management Assistant Task 1.1.
Figure 5.2. Distribution of expert ratings of AI and robotics performance on entire task
Ratings of AI and robotics capabilities to carry out entire tasks by expertise
Figure 5.3, Panel A shows the average ratings of each expert. These measures are computed by taking the mean of each expert’s 13 responses to the question “How confident are you that AI technology can carry out the task?”, one for each of the 13 tasks. “Don’t know” responses were excluded from these calculations. The measures show experts’ average confidence that AI and/or robotics systems can automate the selection of 13 diverse performance tasks. The results range from 30% for José Hernández‑Orallo up to 73% for Guillaume Avrin with the remaining ten experts having between 40-60% confidence about the bundle of 13 tasks.
Figure 5.3. Average AI and robotics performance, by expert and expertise
The 12 computer scientists come from different subfields of AI and robotics research. Four of the 12 experts – Guillaume Avrin, Tony Cohn, Elena Messina and Tadahiro Taniguchi – can be considered experts in robotics, while the remaining eight have a stronger expertise in disembodied AI. Although they all will most likely share the same knowledge on well-established techniques, each group may have specific expertise when it comes to new or less prominent approaches.
Figure 5.3, Panel B shows that the four robotics experts appear on average more confident about AI and robotics systems carrying out the bundle of 13 tasks than the other experts. However, due to the small number of observations (four robotics and eight other experts), these results need to be treated with caution. They do not necessarily mean that the robotics expertise is the driving factor. They may simply reflect differences across the whole group of experts, where a random selection of robotics experts happens to be rating the tasks more highly.
To further understand if robotics expertise was genuinely influencing the ratings, the project analysed average scores for various subtasks. These subtasks were divided into two broad categories: reasoning and language versus physical tasks that required dexterity, like those in robotic systems. To calculate the average scores for each subtask the project counted the number of “Yes”-responses to the question “Humans would typically execute a number of subtasks while carrying out the task. Which of the following subtasks do you think AI can carry out independently?” and then divided it by the total number of experts (12). Subsequently, the project calculated two simple means per task: one on the subtasks in the physical domain and another on the subtasks in reasoning and language, the results of which are presented in Figure 5.4.
Figure 5.4. AI and robotics performance in broad capability domains, by task and expertise
Figure 5.4 underscores the trend where robotics experts often rate tasks slightly higher, although there are exceptions. Physical tasks, especially those requiring dexterity, generally receive lower ratings compared to those centred around reasoning and language. This trend does not shift based on the presence or absence of robotics expertise, as shown in Panel C. However, when zooming into specific tasks, robotics experts exhibit confidence in AI's capacity to handle a large portion of the physical task within the areas of metal technology and product design. This might suggest that robotics experts are more optimistic in general. On the other hand, both roboticists and other experts display scepticism regarding AI's role in personal care tasks that involve comprehensive body movements, such as the Nursing professional role or the Cosmetology Task 1 (focusing on chemical waving). An exception here is the physical aspect of the cosmetology manicure task, which both groups believe AI can feasibly handle. The underlying reasons for these evaluations might revolve around safety considerations, the nature and quality of the target objects and other characteristics of the working environment.
What can and cannot AI systems do and under what conditions
AI's capabilities range from basic implementation to facing significant challenges, as demonstrated in Figure 5.5. By exploring distinct subtasks within the broad capability domains of reasoning and language and physical skills, a clearer picture emerges of where AI excels, where it performs moderately and where hurdles remain.
Figure 5.5. AI and robotics performance on subtasks, by complexity level and broad capability domain, mid-2022
In the domain of language and reasoning, AI represents varying degrees of proficiency. The computer scientists judged that AI could carry out basic subtasks, such as retrieving specific terminology. Examples include the task of a Dental Medical Assistant or using correct grammar and spelling in office documents. At the same time, more nuanced tasks such as “writing concisely and appropriately to addressee and purpose” and “documenting work steps” were judged as moderate challenges. Experts noted the greatest hurdles arise when AI was tasked with complex assignments such as synthesising knowledge into product design, communicating with patients or presenting novel ideas. This shows that while AI can process language, the depth and nuance of human reasoning remain a frontier.
The study took place in 2022 before the launch of ChatGPT, which appears to have meaningfully increased some AI language and reasoning capabilities. As highlighted by experts in follow-up meetings within the project, ChatGPT mimics language processing with more fluency and more contextual sensitivity than previous AI language systems. Moreover, its ability to simulate complex reasoning and human-like conversations signifies a marked improvement, bridging some of the subtasks experts initially identified as challenges in AI's mid-level mastery domain.1
In the physical and dexterity domain, AI's performance varies based on task specificity and complexity. Basic procedural tasks, such as selecting the right materials or maintaining cleanliness in a cosmetology setting, are within AI's grasp. However, AI's mastery starts to waiver as tasks evolve in complexity, such as performing manicure techniques or assembling technical parts. The highest challenges are observed in tasks that require intricate manual skills and precision. This could include, for instance, correctly placing rods in human hair in cosmetology, or deburring and building a model of a part in metal machining.
Descriptors of capability levels of complexity
The scope of AI's capabilities is diverse, and a single subtask might be seen as easy or challenging, depending on the unique requirements and characteristics of a given workplace. Figure 5.6 presents and categorises certain requirements and characteristics that may either promote or deter automation, as outlined by experts. On the more feasible end, there are tasks where AI can easily be deployed, characterised by rule-based, structured environments. On the more challenging end, tasks that demand meta-awareness, creativity or precise manual dexterity push the boundaries of current AI abilities. Between these extremes lies a spectrum of nuanced characteristics where AI can perform but also face obstacles and failures.
Figure 5.6. Expert descriptors of complexity levels of broad capability domains
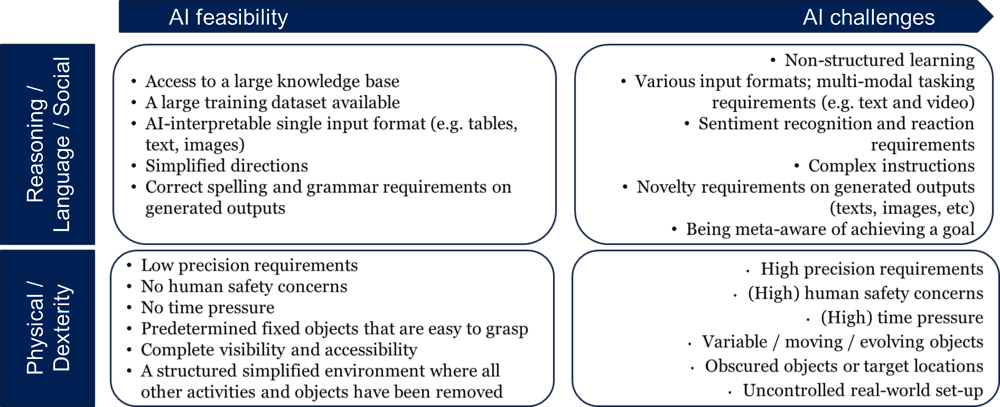
A recurring theme in the expert discussion was the differentiation between tasks in controlled environments, like factories, and those in more arbitrary settings, like homes. Controlled environments allow for a higher degree of automation and predictability, making certain tasks seemingly more achievable for robots. In contrast, arbitrary environments present challenges in terms of variability, requiring higher levels of adaptability and dexterity from robots. Notably, there is a consensus that tasks like picking objects in cluttered spaces, often referred to as the "picking challenge", remain hard despite advances in robotics.
While robots can be highly specialised for particular tasks, their flexibility in handling variations or changes in tasks is still a challenge. The experts distinguished between highly specific tasks (like welding in a car manufacturing facility) and those that require a broader range of skills (like creating a work of art through welding). It is also crucial to know how much a system or environment needs to be engineered for a robot to successfully complete a task.
An essential factor was the role of robots in interacting with human body parts. Some experts lacked familiarity with cutting-edge robotics control technologies. However, they raised concerns about the complexities of ensuring safety when robots interact with the human body, particularly with current technology limitations. Furthermore, the current robotics technology still struggles with tasks involving dexterous manipulation, particularly when it comes to flexible materials like human hair. By contrast, if there is a low precision requirement and tasks involve fixed objects that are easy to grasp, AI could perform.
Time, often a luxury in professional domains, becomes an adversary for AI in tasks under time pressure. In metal technology, while AI can potentially handle welding or manufacturing, the need for swift, real-time decisions and actions can hamper its efficiency as noted by experts. As discussed above, the stakes rise when humans are the focus in medical emergency, necessitating AI to respond quickly and safely – a proficiency that remains underdeveloped.
Despite advances in large language models such as ChatGPT, certain challenges in language and reasoning identified above remain according to experts. In follow-up meetings, experts mentioned these models still grapple with non-structured learning and multi-modal tasks, such as processing varied input formats simultaneously; sentiment recognition can be hit-or-miss, and the models’ ability to handle complex instructions or ensure novelty in output is inconsistent. Moreover, experts lack consensus about whether these models truly possess meta-awareness regarding broader goals.
Discussion of the first assessment
The varied nature of the occupational tasks provided a broad view of different types of AI and robotics strengths and limitations, ranging from straightforward information retrieval to more intricate, multi‑component activities.
Breakdown into subtasks
The experts greatly appreciated the task analysis and the breakdown of individual steps involved. This detailed segmentation of tasks into components provided clear insight into the challenges and requirements for AI and robotics. Many experts said this breakdown facilitated a more structured and nuanced understanding of the occupational tasks. While certain subtasks were deemed within the reach of AI, others remained elusive. This provided a more nuanced view on systems performance on a particular occupational task.
Experts raised the need for a more precise task analysis suited to AI and robotics. Some noted that human‑centred job analysis might not suffice for AI evaluation. They suggested a detailed breakdown to focus on specifics that AI would need to emulate rather than generic human attributes like dexterity or strength. This suggests a deeper collaboration between job analysis experts and AI professionals.
Unclear assumptions
Given the high-level nature of the tasks, experts often formed their own assumptions, leading to potential inconsistencies in their ratings. They frequently highlighted the contrast between general-purpose and specialised AI systems. For some tasks, a general-purpose system, even with its robust capabilities, might find itself handicapped without specific prior data or training. Conversely, a specialised system might be more efficient but economically unviable due to high costs, especially when compared to human labour. As some experts insightfully noted, the nature of the task and its surrounding uncertainties determine the system's efficacy. For instance, a robot might seamlessly operate in a stable industrial environment. However, it might falter in more uncertain terrains, like personal services, without certain controls or constraints.
Further complexity arises when tasks demand multifaceted AI competencies. Some tasks, especially those necessitating fine dexterity, might require a combination of specialised AI algorithms for different components of the task. A system could entail a myriad of AI algorithms, each catering to specific facets like sensory processing, actuation and high-level task planning. In many instances, the hardware limitations of robots overshadow the cognitive capabilities of AI. Thus, separating these evaluations could lead to clearer insights.
The discussions underscored the importance of defining not only the nature of the AI system but also the environment within which it operates. Assumptions regarding environmental uncertainties can significantly impact the system's effectiveness. Explicitly clarifying these assumptions can streamline expert evaluations, ensuring they are premised on a shared understanding of the task, the AI system and the environment.
Complexity and pipeline architecture
There was a consensus that certain tasks presented in the rating exercise were highly complex, requiring the combination of multiple components or steps. Experts noted the challenge of chaining tasks together, especially in terms of error propagation. In a pipeline architecture, errors at one stage can compound, leading to diminished overall performance. In tasks requiring multi-step object manipulation, for example, AI might handle individual steps efficiently. However, the accumulated uncertainty and error across multiple steps can compromise the outcome. Experts thought it might be valuable to explore and present tasks with alternative structures, such as parallel processing or hybrid models, to examine how AI and robotics perform under varied conditions.
Robotics considerations
When assessing tasks in the rating exercise, there is a notable distinction between the AI control mechanisms and the actual robotic capabilities. This distinction, though subtle, plays a pivotal role in the accurate evaluation of the feasibility and effectiveness of an AI-driven robotic system. The project did not provide any specific guidelines to experts on how to think about the level of robotics capabilities. Experts thus responded largely based on individual knowledge and understanding of contemporary robotics.
For many experts, the challenge arose not necessarily from the robotic capabilities side but more from a lack of clarity on the task requirements. As some experts were unfamiliar with tasks in areas such as metal technology and cosmetology, they admitted difficulty in matching up the task demands with existing robotic capabilities. This sentiment was shared even by those with expertise in robotics.
Another essential perspective brought forward was the importance of interpersonal interactions. For certain tasks, especially those in service sectors like cosmetology or nursing, technical performance is just one of several required dimensions. Experts highlighted interpersonal interaction as a critical part of these jobs. They underscored the need to consider the holistic requirements of an occupation and think beyond robotic capabilities.
Experts emphasised that while robotic systems capable of complex manipulations exist, they are not widely accessible. The difficulty of obtaining good robots for experimentation was noted as a significant barrier in many occupational contexts.
To provide a holistic picture, experts suggested that future exercises should consider including the current status of robotic hardware. Distinguishing between feasibility and limitations due to current hardware can be beneficial.
Lack of detail in some task descriptions
The feedback highlighted a desire for more context and detailed descriptions. Some experts felt the need to know more about the environment or specific task nuances. For instance, the “chemical waving” task did not consider hair types. Such information could significantly affect AI’s performance, as different hair types require varying product application times.
Experts noted that a more exhaustive breakdown of the tasks, considering various scenarios and nuances, might enable more precise ratings. Clarifying the specific environment, constraints and objectives would allow experts to rate capabilities based on a shared understanding in the future. Experts also suggested to enhance task descriptions with potential real-world variables. For instance, in tasks related to object manipulation, details about object weight, size and fragility can significantly influence the rating.
A significant feedback point was the need for visual aids or demonstrations to comprehend tasks better. For many, a brief video of an operator performing the task would provide a clearer perspective on the challenges and nuances. This idea extends to the suggestion that perhaps there could be an expert – a job analyst – on hand to answer questions or provide a brief overview.
Evaluation of AI and robotics capabilities on capability scales
As its primary aim, the second study explored diverse evaluation methods for the occupational tasks in response to feedback from the first survey. Experts rated potential AI performance with respect to several, pre-defined capabilities required for solving each task. The expectation was that linking occupational tasks to specific capability requirements would help experts abstract their evaluations from the concrete work context. In this way, they could focus more on general technological features needed for performing the task.
The study focused on the current state of AI technology and its ability to meet or surpass the complexities required of the tasks when carried out by humans. The first question of the new survey sought to measure the current capabilities of AI in relation to the task. In contrast, the second question aimed to understand the skillsets a human needs to perform the same task effectively. The underlying premise was to employ a scale for both AI and human capabilities, and then contrast these results. Ideally, an AI score above the human-required level on the scale would mean that AI could handle the task. However, the project recognised that AI might approach and solve the task differently, possibly without matching the exact complexity exhibited by humans. The survey, while looking at the capabilities of AI, also considered the potential for redesigning tasks, given the manner in which humans and AI tackle tasks may vary.
Of the initial 13 tasks, the project used only 9 for the second study; the other 4 were omitted due to irrelevance (written tasks) or inappropriateness for this more detailed exercise (tasks with limited descriptions).
Aggregate AI capability ratings
To evaluate AI capabilities based on the data from the second survey, the project determined three distinct aggregate indicators. The first measure considers all the essential capabilities for both entities for each task. It is calculated to represent the proportion of capabilities in which experts believe that AI's performance is equal to or surpasses the requirements set for human performance.
This evaluation was achieved by considering expert responses to two key questions in the survey. The first assessed the current capabilities of AI for specific occupational tasks (“In the context of this occupational task, what is the current AI capability in [particular capability]?”). The other determined the performance requirements for humans for those same tasks (“In the context of this occupational task and in your opinion, what are the requirements on humans in [particular capability]”). The calculations excluded “Don’t know” and 0 (“Capability not required for AI”) responses.
The study then compared each expert’s evaluation of AI capabilities with the corresponding evaluation of human requirements for each capability within each task. Whenever an expert judged AI's performance as superior, a score of 1 was assigned for that expert and capability within that task; otherwise, it was assigned 0. As a next step, the study calculates the percentage share of capabilities in a task that an expert considers equal or superior to the job requirements of the task. The aggregate measure is then constructed as the average of all experts’ means for a particular task.
While this method assumes that all capabilities are equally important, it suggests that certain tasks might be achievable if most of the capabilities are met. However, this might not always be true. This method is a simplistic way of consolidating the evaluations. The resulting metric ranged between 0 and 100%, aligning it with the 0%-100% confidence scale from the first study of occupational tasks (Figure 5.7, Panel A). Obviously, the two measures are not fully aligned. Some tasks show the same characteristics, while others move in another direction.
Figure 5.7. AI capability expert ratings and their comparison to the ratings of the first study
The second metric represents the average confidence of experts that AI’s performance is equal to or surpasses the requirements set for human performance in a particular capability domain across all tasks. It was calculated as the percentage share of expert scores of 1 (explained in the paragraph above) for each capability across all tasks. It thus aims to discern any logical distinction between lower-end and higher-end capabilities (Figure 5.7, Panel B).
Overall, experts are sceptical about AI performing at or superior to the job requirements in any of the broad capability domains. At the low end, there are factors like social and emotional sensing and reasoning. At the higher end, there are natural language understanding and recognition of known patterns in the reasoning/language domain and mobility in the physical abilities domain. However, the clarity of this perceived ordering at such a coarse level remains uncertain. In addition, the values for the four capabilities, Gross motor skills, Generation of novel patterns, Output articulation/display and Social and emotional reasoning, are based on ratings within the context of only one or two occupational tasks. Therefore, they should be considered with high caution.
Figure 5.7 does not include the capabilities that experts identified as missing in their response to the third question of the online survey “Are there any essential capabilities missing from the above list?”. Most experts generally did not dismiss the capabilities presented as being irrelevant to the task at hand.
AI capability ratings across task contexts
Figure 5.8 presents the analysis across various tasks using the study’s third aggregate metric, which was calculated as the percentage share of expert scores of 1 for a particular capability and task. Interestingly, experts gave relatively different ratings for different capabilities across most of the tasks. This suggests the context of individual tasks had the expected impact on the ratings. Using the Nursing Professional Task as an example, there is a significant discrepancy between what AI can currently achieve and the requirements on human performance. Referring back to the initial survey’s feedback, most experts believed AI was not adequately prepared to handle a large portion of the task. The comments particularly emphasised challenges related to the complexity inherent in NLP, the nuance of movements and the depth of sensory perception required. The Metal Technology Task 1 indicated similar challenges in the areas of sensory perception and dexterity as compared to the simpler Metal Technology Task 2. Meanwhile, the AI capability ratings did not indicate a vast divergence for certain aspects such as natural language and mobility.
Figure 5.8. AI capability expert ratings, by task
Discussion of the second study
Experts appreciated the project's initiative to compare AI capabilities and human job requirements. They considered it a valuable starting point for understanding the potential and limitation of AI in occupational contexts. However, they faced many challenges in rating the capabilities.
Ambiguous categories of capabilities
Most experts considered the capability categories, as presented in the survey, vaguely defined and confusing. The definition of "natural language generation" illustrates their point well: it contains “web crawl result” as an anchor task, which is better aligned with information retrieval (a separate category) than natural language generation. Additionally, criteria such as whether the output should be realistic, logical or follow a command are missing. Meanwhile, terms like "nuanced language output” are ambiguous. Experts were concerned also with the organisation of categories. For instance, the distinction between navigation and mobility seemed superfluous, while it seemed surprising to group emotional and social sensing together. More detailed definitions of the categories in the McKinsey framework might resolve these uncertainties.
The experts recommended the project develop an approach that uses clearer definitions for the different categories and provides tangible, real-world examples for each capability category. The categorisations and their subsequent groupings should be logical and intuitive to facilitate comprehension.
A confusing scale
The scale introduced in the survey was judged especially confusing. Experts noted the mention of quartiles did not correspond with the human-level benchmark, leading to uncertainty about what "human-level" genuinely meant. Moreover, the scale's metrics, including terms such as accuracy and complexity, were referenced without clear, defined thresholds, making it challenging to gauge the parameters. While certain categories did provide illustrative examples, like "picking up an egg", such examples were few. This scarcity left most categories without tangible references to calibrate the levels. Overall, experts found the labels arbitrary and problematic and felt confused about the proper use of the scales.
To foster greater clarity, experts highlighted the need to restructure the scales to include well-defined thresholds and distinctions between varying levels. They proposed introducing a nuanced scale, possibly leveraging a Likert statement. They also noted that most domains could likely benefit from at least five discernible capability levels, as the current state of AI often does not neatly fit into a single category. Especially when evaluating AI's dexterity, such as in manipulation skills – a domain where robots currently underperform – a more detailed scale becomes essential. Lastly, experts advised against using terms like "human" in labels and emphasised the importance of ensuring the scale's ends represent true opposites.
A questionnaire centred on AI versus humans
Some experts expressed concerns about the survey questionnaire not aligning its questions appropriately between those centred on machines and humans. The first question (“In the context of this occupational task, what is the current AI capability in [capability category] using the scale?”) sought to determine AI's current capability. However, the subsequent question tried to identify the level deemed necessary for humans to carry out the task (“In the context of this occupational task and in your opinion, what are the requirements on humans in [capability category]?”).
This differentiation raised concerns among some experts about the task assumptions. Did the survey envisioned a general-purpose humanoid robot designed to replicate human functions across various domains? Or did it envision specialised robotic systems tailored to specific tasks, such as adaptive devices to assist patients?
Furthermore, with respect to robotics operational independence, experts asked whether the robot would function autonomously after obtaining its occupational certification or serve as an assistant to humans. This was especially relevant in high-risk scenarios like heavy lifting or environments with extreme temperatures. Each perspective would change fundamentally the nature of the task.
Some experts also expressed confusion about whether they were rating an AI's overall ability in a specific capability domain or its competence in the context of a particular task. This dilemma was exacerbated when the task in question was relatively simple for humans but potentially complex for AI. The blending of these two perspectives in the instructions further complicated matters.
Experts agreed that merely determining if AI performs "better" than humans is not enough; they need to define what "better" means in terms of accuracy, speed or another metric. A main challenge they faced was comparing AI and human performance. They had to make assumptions during rating, which introduced variability in the responses. They expressed a strong need for clear guidelines when making comparisons, as different interpretations can significantly alter the results.
In turn, some experts disagreed with the response options to the first question, notably the “Capability not required for AI” option. They considered "capability not available in AI" as a more suitable response along the other three levels of AI capability (low, medium and high with descriptions). They also recommended to address the dynamics between AI and humans, ensuring the exercise captures the nuances of expectations placed on both sides. As a result of these ambiguities, many experts provided the “Don’t know” option to the first question.
Other experts noted that when social components were involved in the task, they raised their requirements on human performance. While the performance standard for simply completing a task might be similar for both AI and humans, expectations diverge when considering potential users or customers. They noted a general acceptance of certain limitations when it is known that AI performs a task, especially in social interactions or understanding. In contrast, for humans, the anticipation is considerably higher.
Useful videos
Experts found the videos accompanying the survey useful in understanding task complexities, particularly in areas unfamiliar to them. While these visuals conveyed the nuances and dexterity inherent in certain tasks effectively, the translation from instruction to action occasionally remained unclear. The videos underscored the challenges AI might face, yet some experts felt they mainly reinforced existing knowledge. While not deemed essential, the visual aids emphasised the intricacies of human roles and highlighted the challenges in adapting tasks for AI.
The feedback from experts has been mixed, indicating the new approach did not feel intuitive but also that the capability categories and scales the project used were not optimal.
The way forward
The two exploratory studies highlighted the inherent complexity of work tasks, which involve numerous individual capabilities. This complexity makes it difficult to provide ratings of AI’s capabilities in relation to the task. To do so, judgements are required for all the required capabilities individually, as well as their combination. As a result, the project has decided to explore alternative uses of the occupational tasks.
The exercise provided important insights about how to think of and define the capability domains and suggested developing anchor tasks to describe each level of capability. The project will consequently explore working with the O*NET system of occupational classification. This provides specific tasks as anchors to help understand better each level's capabilities as an alternative to the framework in this study that experts considered very general.
O*NET’s anchors serve as illustrative examples. This will make it easier for computer scientists and job analysts to agree upon the appropriate level for each task on the AI and human side, respectively. The O*NET system could provide clearer distinctions, especially in areas like natural language and fine motor skills. By presenting specific tasks for each capability level, it may be more intuitive and easier to comprehend than the broader categories in the current scale.
During the exercise, experts delved into the question of how AI can change the work context and suggested the use of occupational tasks to better anticipate how certain roles within the economy might evolve as new capabilities emerge. Experts highlighted the merit of exploring a human-AI collaborative approach where AI complements, rather than replaces (via automation) human efforts. Understanding these dynamics would be crucial for the goals of the project, ensuring that education, training and policy evolve hand-in-hand with technological advancements.
Experts provided useful advice on how to analyse task redesign. The project will draw on this advice in exploring the implications of evolving AI capabilities on education, work and everyday life. This exploratory work will consider the following points:
Rather than exclusively focusing on the current makeup of tasks, the design could contemplate the broader ecosystem within which these tasks exist. It is crucial to reflect upon how tasks can be reconceived, or entire systems revamped, to harness AI's strengths most effectively. Experts noted that while humanoid robots have allure, particularly from a human-computer interaction perspective, their development might not always be the most pragmatic or cost-efficient solution. In many scenarios, conceptualising the task or the system from scratch, with automation as a cornerstone, could yield higher efficiencies and superior user experiences. Expert reflections highlighted that such redesign decisions would be propelled by factors such as economic gains, consumer inclinations and technological breakthroughs.
Another significant observation stemmed from the potential disconnect between AI's capabilities and the specificities of the domain to which it is applied. While understanding the AI's capabilities is integral, having domain-specific knowledge is equally pivotal. To bridge this gap, some experts proposed a dyad approach. They felt a collaboration between an AI expert and a domain specialist could ensure a more holistic redesign of tasks that considered both AI's strengths and the intricacies of the domain.
References
[1] McKinsey Global Institute (2017), A Future that Works: Automation, Employment, and Productivity.
Annex 5.A. Categories of AI capabilities
Annex Table 5.A.1. Categories of AI capabilities
Each capability category is characterised by three performance levels ranging from 1 (basic) to 3 (human-like) performance (based on tech advancements and complexity)
AI capability |
1 |
2 |
3 |
Metric to define continuum |
---|---|---|---|---|
Natural language understanding |
Low language comprehension required (while still accurate with structure commands) |
Moderate language comprehension (medium accuracy of nuanced conversation) |
High language comprehension and accuracy, including nuanced human interaction and some quasi language |
Accuracy of comprehension Complexity of language/context integration |
Sensory perception |
Autonomously infers simple external perception (e.g., object detection, light status, temperature) using sensory data |
Autonomously infers more complex external perception using sensors (e.g., high resolution detail, videos) and simple integration using inference |
High human-like perception (including ability to infer and integrate holistic external perception) |
Accuracy of perception/complexity of scene Degree of integration across sensors |
Social and emotional sensing |
Basic social and emotional sensing (e.g., object detection, light status, temperature) using sensory data |
Comprehensive social and emotional sensing (e.g., voice, facial and gesture recognition-based social and emotional sensing) |
High human-like social and emotional sensing |
Quality of comprehension |
Recognising known patterns/category (supervised learning) |
Recognition of basic known patterns/categories (e.g., lookup functions in data modelling) |
Recognition of more complex known patterns/categories |
High human-like recognition of known patterns |
Complexity of pattern |
Generation of novel patterns/categories |
Simple/basic ability for pattern/category recognition |
More advanced capacity for recognition of new patterns/categories and unsupervised learning |
High human-like recognition of new patterns/categories, including development of novel hypotheses |
Complexity of pattern |
Logical reasoning/problem solving |
Capable of problem solving based on contextual information in limited knowledge domains with simple combinations of inputs |
Capable of problem solving in many contextual domains with moderately complex inputs. |
Capable of extensive contextual reasoning and handling multiple complex, possibly conflicting, inputs |
Complexity of context and inputs |
Optimisation and planning |
Simple optimisation (e.g., optimisation of linear constraints) |
More complex optimisation (e.g., product mix to maximize profitability, with constraint on demand and supply) |
High human-like optimisation based on judgement (e.g., staffing a working team based on team/individual goals) |
Degree of optimization (single vs. multi variate) |
Creativity |
Some similarity to existing ideas/concepts |
Low similarity to existing ideas/concepts |
No similarity to existing ideas/concepts |
Novelty/ originality and diversity of ideas |
Information retrieval |
Search across limited set of sources (e.g., ordering parts) |
Search across multiple set of diverse sources (e.g., advising students) |
Expansive search across comprehensive sources (e.g., writing research reports) |
Scale (breadth, depth, and degree of integration) of sources Speed of retrieval |
Coordination With multiple agents |
Limited group Collaboration; low level of interaction |
Regular group interaction requiring real-time collaboration |
Complex group interaction requiring high human‑like collaboration |
Complexity of coordination (i.e., number of interactions per decision) Speed/frequency of coordination |
Social and emotional reasoning |
Basic social and emotional reasoning |
More advanced social and emotional reasoning |
High human-like social and emotional reasoning |
Complexity of emotional inference |
Output articulation/ display |
Articulation of simple content (e.g., organising existing content) |
Articulation of moderately complex content |
High human-like articulation |
Complexity of message delivered. Variability in medium of message delivered |
Natural language generation |
System output with Basic written NLG (e.g., web crawl results) |
System output with advanced NLP (more complex structure) |
Nuanced, high human-like language output |
Complexity of message delivered. Note: includes use of quasi linguistics (idioms, common names, etc.) Accuracy of audience interpretation |
Emotional and social output |
Simple social and emotional discussions (e.g., conversations with no gestures) |
Advanced social and emotional discussions (e.g., conversations with gestures) |
Nuanced high human-like body language and emotional display |
Complexity of emotional communication Accuracy of audience interpretation |
Fine motor skills/dexterity |
Ability to handle and manipulate common simple objects (e.g., large solid objects) using sensory data |
Can handle and manipulate wide range of more complex and delicate objects (e.g., pickup egg) |
High human dexterity and coordination |
Precision, sensitivity, and dexterity of manipulation |
Gross motor skills |
Basic10/20motor skills |
More advanced multi-dimensional motor skills |
High human multi- dimensional motor skills |
Range and degree of motion Speed and strength of motion |
Navigation |
Use pre-defined algorithm for mapping and navigation |
Autonomous mapping and navigation in simple environment |
Autonomous mapping and navigation in complex environment |
Complexity of environment (while still maintaining accuracy) |
Mobility |
Mobility/locomotion in simple environment (e.g., limited obstacles/office space) |
Mobility/locomotion in more complex terrain of human scale environment (e.g., climbing stairs) |
High human mobility and locomotion |
Speed (gross motor) of mobility Scale of mobility vs.30) Complexity of environment/terrain |
Source: McKinsey Global Institute (2017[1]), A Future that Works: Automation, Employment, and Productivity.
Note
← 1. In the literature, there is uncertainty about the degree of generalisation reflected in the underlying language models that drive these AI systems and what that implies for the level of independent reasoning that the systems can carry out. In the context of this larger debate, the occupational tasks addressed in this chapter provide a special case. They occur in work settings where workers have been intentionally trained to carry out certain types of reasoning. Therefore, it makes sense to consider comparing those workers with AI systems that have been similarly trained on the reasoning required in that work setting.