Andrea Bassanini, Andrea Garnero and Agnès Puymoyen
OECD Employment Outlook 2024: The Net-Zero Transition and the Labour Market
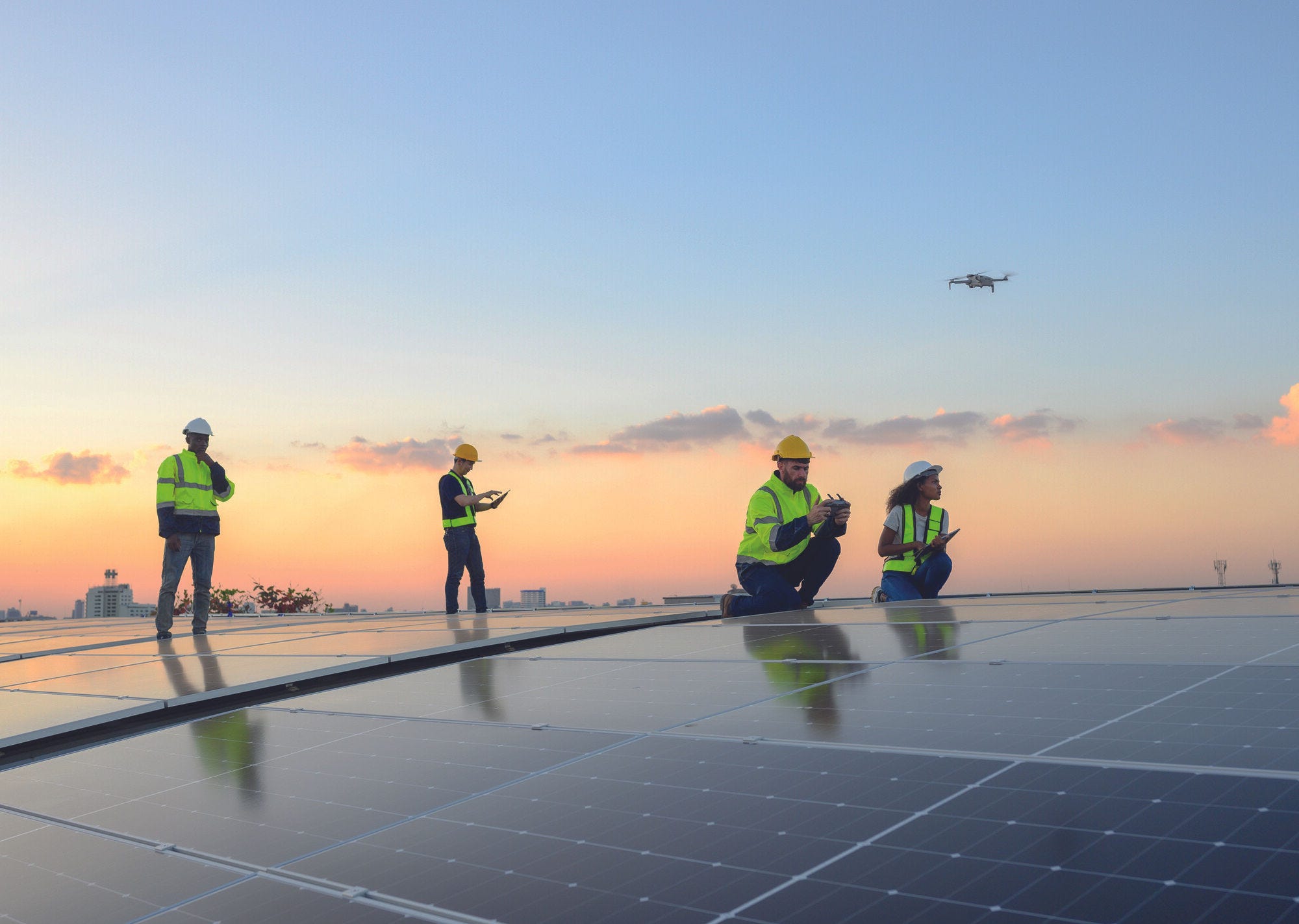
2. The jobs that will make the net-zero transition: Who holds them and are they good?
Abstract
The policies that OECD countries are adopting to achieve net-zero greenhouse gas emissions by 2050 will have a significant impact on labour markets: jobs in high-emission industries will be reduced, new opportunities will emerge in climate‑friendlier sectors, and many existing occupations will be transformed. Climate change itself will affect labour demand and working conditions, mainly through rising temperatures and more frequent extreme weather events. This chapter focuses on the jobs that are likely to thrive because of the net-zero transition and compares them with jobs concentrated in high-emission industries. In addition to looking at the characteristics of the workers who hold these jobs and where they are located, the chapter delves into the quality of these jobs by analysing their wages, labour market security and quality of the working environment.
In Brief
OECD countries are actively adopting ambitious climate change mitigation packages aimed at achieving net-zero greenhouse gas (GHG) emissions by 2050. The net effects of these policies on aggregate employment are expected to be modest given that employment in high-emission sectors represents only a small fraction of total employment and new opportunities will arise in climate‑friendlier occupations, and industries, including those producing intermediate goods and services for environmentally sustainable activities. Nevertheless, significant shifts are expected across industries, occupations, and regions.
The chapter first recalls that the absence of policy action as regards climate mitigation is, and will increasingly have, dramatic consequences for the labour market, largely concentrated on the most vulnerable groups. It shows that 13% of workers in European OECD countries and the United States suffer from significant heat discomfort, typically workers in outdoor occupations and workers in process and heavy industries, with potential negative effects on their health and productivity. Moreover, the regions where the proportion of workers exposed to high temperatures is already higher than average are also the regions projected to experience the largest temperature increases by 2050.
The chapter then focuses on the jobs that are likely to thrive in the context of the net-zero transition and compares them with the jobs most at risk of disruption. To this end, the chapter introduces the concept of “green-driven occupations”, which goes beyond the usual notion of “green jobs” to include also those jobs that do not directly contribute to emission reductions but are likely to be in demand because they provide goods and services required by green activities.
The key findings of the chapter are as follows:
Across the OECD, between 2015 and 2019, around 20% of workers are employed in green-driven occupations. Of these green-driven occupations:
46% are existing occupations whose skill set is being altered because of the green transition (referred to here as “green-enhanced skills occupations”).
40% are existing jobs that will be in demand because they provide goods and services required by green activities (referred to here as “green increased demand occupations”).
Only 14% are what can properly be described as “green new or emerging occupations”.
Conversely, over the same period, about 6% of OECD employment is in GHG-intensive occupations, i.e. occupations that are particularly concentrated in high-emission industries. Green-driven and GHG-intensive occupations are not mutually exclusive categories: about 10% of the green-driven occupations are, in fact, concentrated in high-emission industries, mostly in the group of “green-enhanced skills occupations”.
Both green-driven and GHG-intensive occupations are more widespread in rural than in urban areas. However, regions with higher incidence of GHG-intensive occupations are not necessarily the same as those with higher incidence of green-driven occupations. Without policy action, there is a concrete risk of widening regional labour market and economic inequalities.
Green-driven occupations are heterogeneous: green new and emerging occupations are typically high-skill jobs (i.e. managers, professionals and technicians) and employ highly educated workers in urban areas while the other green-driven occupations are on average more medium- and low-skill and employ more low-educated workers.
Green new and emerging occupations experienced the fastest growth in the past decade. Between 2011 and 2022, their share in total employment increased by 12.9% on average in European-OECD countries and the United States, while the share of all green-driven occupations in total employment increased by only 2%. The latter figure, however, rises to 5% once green-driven occupations concentrated in high-emission industries are excluded. In the same period, the share of GHG-intensive occupations declined by 18%.
Green-driven occupations tend to be characterised by higher wages and fewer temporary contracts than other occupations, although workers in these jobs are often exposed to larger unemployment risk. In addition, jobs that are not directly contributing to emission reductions but will likely be in demand because their products or services will be needed by green activities (i.e. green increased demand occupations) tend to be characterised by a higher incidence of job strain (insufficient resources to meet job demands).
The job-quality advantage of green-driven occupations, relative to other jobs, tends to be concentrated in high-skill occupations, suggesting that those workers who have the specific competences required by these expanding jobs have a competitive edge in the labour market and thrive compared to their peers. By contrast low-skill, green-driven occupations tend to command significantly lower wages and labour market security than other low-skill jobs, suggesting that, absent policy action, these occupations may be a relatively unattractive option for low-skilled workers.
Even though many workers in high-emission industries are paid relatively well (see Chapter 3), the occupations most concentrated in these industries (GHG-intensive occupations) are, on average, characterised by a higher incidence of low pay as well as a lower quality of the working environment than the average job. In contrast, they do not display, so far, larger unemployment risk than other occupations. This is consistent with the fact that workers in GHG-intensive jobs are often relatively stable jobs (until they are suppressed because of industry downsizing – see Chapter 3). However, workers in GHG-intensive occupations are likely to have fewer options outside the downsizing GHG-intensive industries, which may put additional pressure on their bargaining power, resulting in lower-than-average wages.
The characteristics of the workers employed in declining GHG-intensive occupations are remarkably similar to those of the workers more exposed to high temperatures. This suggests that while climate change mitigation policies may be costly for some groups of workers, there are also costs to inaction for these groups.
Overall, these results suggest that the net-zero transition is creating and will continue to create good-quality jobs. However, these tend to be concentrated in high-skill occupations and represent an opportunity mainly for highly educated, urban workers who have the competences required for these jobs. In contrast, for low-skilled workers, green-driven occupations may not be a sufficiently attractive alternative to jobs in the rest of the economy (including high-emission industries). This points to the importance of measures to improve the quality of green-driven jobs as well as the critical role of public policies in facilitating and accompanying the transition, such as training policies (Chapter 4), active labour market policies, social dialogue and collective bargaining (Chapter 3).
Going forward, to be able to properly monitor and analyse the effects on the labour market of climate change mitigation policies, more timely and granular data will be necessary. In particular, OECD countries should consider collecting and releasing survey and administrative data at a higher degree of disaggregation, run ad hoc surveys collecting workers’ use of green technologies and practices and develop country-specific inventories of green tasks by occupation – such as O*NET for the United States.
Introduction
The urgency of tackling climate change has reached a critical juncture and it represents one of the greatest challenges ahead in all sectors of the economy. The latest report from the Intergovernmental Panel on Climate Change (Arias et al., 2023[1]) leaves no room for doubt: human-driven climate change is advancing rapidly and demands immediate, collective action to swiftly reduce global emissions towards net zero, as well as to confront the escalating impacts of climate‑related disruptions.
This will have direct effects on OECD labour markets. Most analyses so far have focused on the effect of the green transition on the quantity of jobs, i.e. whether it will create more or fewer jobs than those that it will destroy. While the precise estimates from macro-models differ, there is a broad agreement that the net effect of the net-zero transition on aggregate employment will be modest – see Box 2.1. But such a question risks concealing the very significant impact that the transition will have in reshaping the labour market: some jobs will disappear, and new opportunities will arise leading to a major reallocation within and across sectors and regions. Moreover, many existing professions will be transformed and redefined as day-to-day tasks and work methods become greener. Finally, climate change itself will impact labour demand and working conditions, primarily through an increase in temperatures and in the frequency of extreme weather events.
While climate change mitigation policies may entail significant economic costs, especially for some groups of workers, absence of climate action indeed implies major costs and may lead to increasing inequalities (OECD, 2021[2]): in a recent paper, Bilal and Känzig (2024[3]) estimate that world GDP per capita would be 37% higher today had no warming occurred since 19601 and these high costs of global warming are concentrated among vulnerable groups. For example, the increasing frequency of heatwaves due to climate change poses a significant threat to individuals employed in outdoor settings or those already regularly exposed to elevated temperatures, often comprising middle‑ and low-income earners – see Box 2.2. Furthermore, some studies have emphasised the adverse effects of outdoor air pollution on indoor workers with lower skill levels (Chang et al., 2016[4]; Adhvaryu, Kala and Nyshadham, 2019[5]; Chang et al., 2016[6]). In addition, the influence of climate change on the productivity of vital sectors for rural economies, such as agriculture and fisheries, could also worsen the urban-rural disparity.
Policy makers, therefore, face a double challenge: they must design policies to facilitate and manage labour reallocation induced by climate change mitigation policies but also enhance the capacity of workers, companies and communities to adapt to increasing temperatures and the escalating severity of extreme weather events (Keese and Marcolin, 2023[7]).
The exact extent of these challenges remains uncertain and hinges on the implementation of policy commitments and goals, as well as the widespread adoption of existing environmentally friendlier technologies and the development of new ones (e.g. carbon capture, utilisation and storage – CCUS hereafter) across various sectors and enterprises. But there is no doubt that climate change and climate change mitigation policies will have a significant impact on OECD labour markets, well beyond their net effects on aggregate employment. Moreover, the transition to net-zero comes at a time when OECD labour markets are experiencing other transitions. Technological advances, including in the area of generative artificial intelligence, the reorganisation of global value chains, and rapid demographic ageing are all affecting households and putting many workers under pressure. But the net-zero transition is unique in its largely policy-driven nature. Policy makers therefore have a heightened responsibility to ensure a just transition that leaves no one behind.
Box 2.1. The effects on aggregate employment of the net-zero transition
The net-zero transition will entail structural macroeconomic and sectoral transformations in production processes and technologies, demand patterns, international trade and competitiveness. One particular concern is what will happen to employment as the transition will imply a reduction of fossil fuel use and related economic activities. Will the employment created in the sectors that will be instrumental to change the energy mix and reduce emissions be enough to compensate for the employment losses in high-emission sectors? The answer depends on a range of factors. Some of these are known or can be anticipated such as the size of the downsizing sectors compared with the sectors that are likely to grow and demographic trends. Other factors are difficult to fully anticipate in the long run, such as technological developments and how existing companies will adapt to changing regulations and demand patterns.
There is a wide range of estimates of the net employment effects based on macroeconomic, general-equilibrium modelling. Under a number of simplifying assumptions (typically, the modelling of the functioning of the labour market tends to be rather simplistic), these models simulate the impact of climate mitigation policies, such as an increase in the carbon tax – see also Chapter 5.
At the OECD, the ENV-Linkages model projects economic activities and emissions several decades into the future to shed light on the medium- and long-term impacts of environmental policies. Using this model, Chateau et al. (2018[8]) simulate the effect of a carbon tax of USD 50 per ton of CO2 in all regions of the world and find that, despite large impacts on specific sectors, the aggregate effect of decarbonisation policies on employment is generally small and positive, but turns negative when the carbon tax considered exceeds USD 100 per tonne of CO2 and when the carbon tax revenues are not used to lower labour tax rates. More recently, Borgonovi et al. (2023[9]) used ENV-Linkages to estimate the effects of the EU Fit for 55 package, an ambitious set of measures adopted by the European Union to achieve a 55% reduction in EU greenhouse gas emissions by 2030 compared with 1990 (corresponding to a carbon tax of USD 202 per tonne of CO2 in the model). They find that employment growth is projected to be somewhat lower than in a scenario without the Fit for 55 package (1.3% employment growth between 2019 and 2030 in the Fit for 55 scenario against 3% in the baseline scenario).
The likely impact of the Fit for 55 package has also been simulated with other models. According to the impact assessment of the package by the European Commission (2020[10]), using the applied general equilibrium model JRC-GEM-E3, the Fit for 55 package would have a small negative effect on aggregate employment by 2030 (‑0.26%) compared to the baseline (the existing 2030 climate and energy legislative framework) but if carbon revenues are used instead to reduce labour taxation, the impact may be slightly positive (0.06%). These employment impacts differ across occupations, skill groups, and policy design options – carbon pricing vs. regulatory measures (Weitzel et al., 2023[11]). Using the GEM-E3‑FIT model, Eurofound (2023[12]) also finds modest net gains by 2030. Other macro models are somewhat more optimistic: E3ME, a macroeconomic model used to simulate and assess the medium to long-term effects of environmental and economic policies for Europe, projects no change in employment under the assumption of a lump sum transfer of carbon revenues to households. If carbon revenues are recycled to support energy efficiency investment and reduce VAT, the boost to consumption and GDP would generate an increase in employment of up to 0.2% relative to baseline. E‑QUEST, a micro-founded multi-region model with energy sectors, estimates an increase of 0.45% if carbon revenues are recycled to reduce labour taxation for low-skilled workers (European Commission, 2020[10]).
Estimates are available also at the country level. For instance, Fontaine et al. (2023[13]) estimate the impact on employment in France of a EUR 100 carbon tax using a simulation model (ThreeMe2) specially developed to analyse the medium- and long-term consequences of energy and environmental policies at the national level. They find a negative effect (‑0.6%) by 2030 that becomes positive (+0.3%) if carbon revenues redistributed in full to households (in the form of a reduction in income tax) and companies (via a reduction in social security contributions). In the United States, Finkelstein, Shapiro and Metcalf (2023[14]) estimate the impact of a 35% reduction in carbon emissions (a target broadly consistent with the country’s Paris Agreement commitment) and find that, under a scheme where carbon-tax revenue is transferred to households through a lump sum, it can generate mild positive long-run effects on employment, thanks to a boost in consumption and output and an increase in the use of green technologies.
A full review of all studies is beyond the scope of this chapter, but it is fair to conclude that the available estimates based on macro models suggest that the net aggregate effects of the transition to net zero are likely to be modest. Differences in the results can be explained by the type of models used, the climate mitigation policy mix simulated, the stringency of climate action in the rest of the world, and the accompanying measures put in place. On the one hand, models finding slightly negative results tend to assume that carbon revenues are not recycled via lower labour taxes and that international co‑ordination in climate mitigation policies leads to a general slowdown of economic growth (even if ensuring a level playing field between companies across the world). Studies finding slightly positive effects, on the other hand, usually assume some degree of revenue recycling that stimulates the labour market. A consistent outcome across models, however, is the finding that the transition to net zero will shift jobs away from fossil fuel sectors towards other economic activities that play an important role in decarbonising the economy, such as renewable electricity generation and construction sector activities that enhance the energy efficiency of buildings, as well as that, if policy commitments are held, these shifts will already become sizeable within a short time horizon (e.g. by 2030).
This chapter focuses on the jobs that are likely to benefit from the net-zero transition and compares them with the jobs that are the most at risk of downsizing. Most analyses in the literature have centred on “green jobs” viewed as jobs which directly contribute to reducing emissions. However, there is no agreement on what a green job is. Moreover, to have the full picture, it is also necessary to identify the jobs at the greatest risk of becoming obsolete because of the net-zero transition (the “high-emission jobs”) and the approaches used to define these jobs also differ. Finally, the net-zero transition will have a much larger impact than just on the narrow groups of green and high-emission jobs: other jobs that are neither high-emission nor actively contributing to reduce emissions but that are in demand because of green activities will be impacted. To be able to consider the full extent of the challenge, this chapter takes a broader definition than most previous studies and looks at all green-driven jobs, i.e. all those jobs that are likely to be positively affected by the net-zero transition, even those that are not green as such.
Beyond providing a ballpark estimate of the jobs likely to benefit from the net-zero transition and of those most at risk and the characteristics of the workers holding these jobs, this chapter delves into the quality of these jobs. A successful transition towards a low-carbon economy not only will induce greater demand for green-driven occupations but also requires greater workers’ willingness to search for, and pick up, job offers in these occupations. Jobs in (downsizing) high-emission industries are typically regarded as relatively good jobs, paying comparatively well and with a prevalence of open-ended, full-time employment contracts. This is less clear for the jobs that will emerge from the net-zero transition. In fact, a key policy issue, which has already led to some industrial disputes and growing concerns among policy makers and social partners, is that downsizing of traditional jobs in high-emission sectors and development of green-driven jobs may result in a worsening of job quality. This chapter will show that some, but only some, of these concerns may be warranted by looking at the quality of green-driven and other jobs.2
The remainder of this chapter is structured as follows: Section 2.1 starts by providing an overview of the measurement approaches adopted so far to measure green and high-emission jobs and describes the approach adopted in this chapter. Section 2.2 presents a panorama of green-driven and high-emission jobs, focusing especially on the characteristics of the workers currently holding these jobs and of the areas where they are located. Section 2.3 provides a detailed analysis of job quality in green-driven occupations, focusing on the three dimensions of the OECD Job Quality framework, namely wages, labour market security and the quality of the working environment (OECD, 2014[15]). Section 2.4 concludes by discussing the data and methodological improvement needed to sharpen our understanding of the impact of the net-zero transition on the labour market.
Box 2.2. Increasing temperatures will also have labour market costs
While this edition of the Employment Outlook essentially focuses on the consequences of climate change mitigation policies, OECD labour markets will also be affected by the impacts of climate change itself. In particular, all workers will experience the effects of global warming, but to varying degrees depending on their professional situation and their exposure as well as vulnerability to climate risks.
Climate change is not only gradually increasing temperatures globally but is also increasing the intensity and duration of heatwaves. Heat-stress can lead to fatigue or exhaustion as well as cardio-vascular and respiratory health problems. Heat exposure impairs workers’ capacities with a negative effect on productivity, both in complex activities with a high cognitive content or relatively simple, routine activities requiring particular attention and vigilance (Benhamou and Flamand, 2023[16]). Heat has been shown to lower productivity (Day et al., 2019[17]; ILO, 2019[18]), increase absenteeism (Somanathan et al., 2021[19]), heighten the risk of work-related accidents (Fatima et al., 2021[20]; Park, Pankratz and Behrer, 2021[21])1 as well as the functioning of machinery and infrastructure (Benhamou and Flamand, 2023[16]).
There is limited comprehensive evidence on the share of workers exposed to the risks related to extreme heat events as this requires detailed knowledge on their specific tasks, working conditions and their place of work. Some of this information can be retrieved using working condition surveys to have a ballpark estimate of the workers currently exposed to high temperatures. Building on the analysis for France by Benhamou and Flamand (2023[16]), Figure 2.1, Panel A shows that in European OECD countries and the United States, in 2015,2 after accounting for the different timing of the interviews,3 13% of workers were exposed to high temperatures at least half of their working time, with peaks of 26% in Türkiye, 25% in Spain, and 22% in Greece. On average, an additional 10% of workers were exposed to high temperatures at least one‑quarter of their working time.
The sectoral and occupational characteristics contribute to explain much of the variation across countries: everywhere, workers in outdoor occupations (e.g. street vendors, construction workers, farmers and fishers) are particularly affected by heat (as well as cold), as are workers in process and heavy industries. Given the type of occupations and industries concerned, the highest share of workers exposed to high temperatures can be found in rural areas and more among low educated and middle‑skilled workers (i.e. sales, skilled agricultural, craft and plant workers) – see Figure 2.1, Panel B. Given their occupational concentration, men tend be more exposed to high temperatures than women, while older workers tend to be slightly less exposed than younger ones – a more in-depth discussion of the results in this box is available in OECD (forthcoming[22]).
Beyond the occupational characteristics and the working environment (typically the presence of air conditioning), the geographic distribution of workers also plays a role: worryingly, regions where workers already report heat-related discomfort, typically many southern regions of Europe, are also those that are predicted to experience higher heat stress in the coming decades as per the projections by Casanueva et al. (2020[23]) and García-León et al. (2021[24]) – see Figure 2.2. However, in other countries and regions where heatwaves are a more recent phenomenon, the effects of heat stress may be just as challenging, if not more so, as buildings and work organisation may be less prepared.
Figure 2.1. One in seven workers report heat-related discomfort in Europe and the United States
Percentage of employment who declare to be exposed to high temperature at least half of their working time, 2015

Note: Those who declare to be exposed to high temperature which make them perspire even when not working at least half of their working time are classified as at-risk. The shares are the predictions in May, obtained by running a regression of the exposure to heat at country level on the share of respondents by month of interview. For Panel A, the average is unweighted. For Panel B, averages are unweighted and include Austria, Belgium, Czechia, Denmark, Estonia, Finland, France, Germany, Greece, Hungary, Ireland, Italy, Latvia, Lithuania, Luxembourg, the Netherlands, Norway, Poland, Portugal, the Slovak Republic, Slovenia, Spain, Sweden, Switzerland, the United Kingdom and the United States. Youth are those aged from 15 to 24, prime from 25 to 54, and senior from 55 and above. For urbanisation, the United States is not included.
Source: Secretariat’s calculations based on data from European Working Conditions Survey (Eurofound) and American Working Conditions Survey (RAND Corporation).
Occupational safety and health (OSH) regulations in OECD countries already cover aspects related to temperature, heat stress and extreme weather at work. However, some countries, often with the direct involvement of social partners, have adapted the legislation to the specific challenges related to climate change (e.g. Lithuania) or developed specific OSH programmes and tools (e.g. Germany, Lithuania, Slovenia and Spain). In some countries (e.g. Belgium, Italy, Spain) companies can, under some conditions, use the job retention scheme in case of exceptionally high temperatures. Practical guidance on how to manage risks has been developed by occupational safety and health agencies in several countries (Safe Work Australia, the Canadian CCOSH, EU OSHA, UK HSE and US NIOSH). Specific initiatives have also been taken in some of the most exposed sectors (e.g. agriculture in Lithuania or construction in Austria, Germany and Lithuania), including the development of new tools (e.g. a warning system in Slovenia accessible to employers and employees with site‑specific predictions) – for more information on the policies in place see the review by the European Commission (2023[25]).
Figure 2.2. Regions with higher proportions of workers reporting heat-related discomfort are also projected to face higher heat stress in 2050
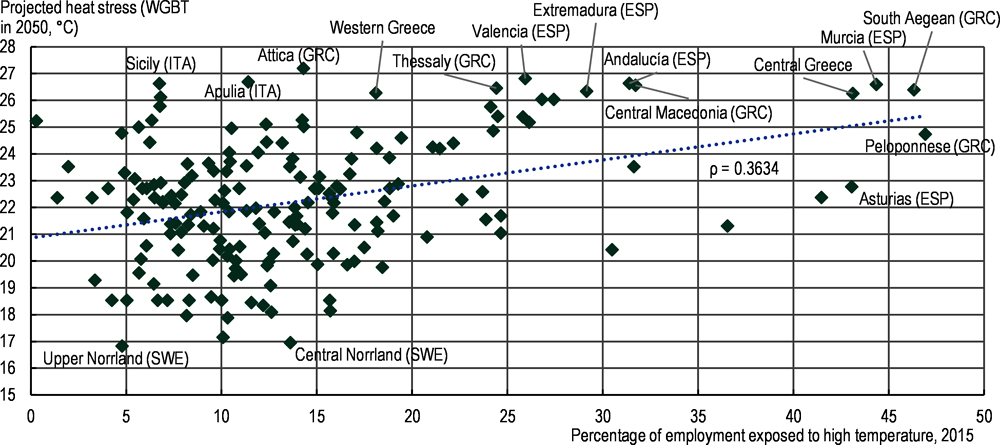
Note: The X-axis refers to those who work under high temperature at least half of their working time. The shares of workers exposed to high temperatures are the predictions in May, obtained by running a regression of the exposure to heat at country level on the share of respondents by month of interview. Projected heat stress in 2050 is measured using the Wet-bulb Globe Temperature (WBGT) which factors in temperature, dew point temperature, wind speed, and solar radiation to assess heat stress experienced by individuals working outdoors (thus it should not be interpreted in the same way as standard temperatures). The 2050 projection is calculated based on daytime working hours during the summer with the KNMI regional climate model (RACMO), which uses a high-mission scenario of the Representative Concentration Pathway 8.5. The OECD large (TL2) regions of Austria, Belgium, Czechia, Denmark, Estonia, Finland, France, Germany, Greece, Hungary, Ireland, Italy, Latvia, Lithuania, Luxembourg, the Netherlands, Norway, Poland, Portugal, the Slovak Republic, Slovenia, Spain, Sweden and Switzerland are included. The TL2 regions for which the number of observations is fewer than the bottom 10th percentile (i.e. 30 observations) are removed. For France, the 2010 data are used. The correlation is statistically significant at a 95% confidence level.
Source: Secretariat’s calculations based on data from the European Working Conditions Survey (Eurofound); García-León et al. (2021), “Current and projected regional economic impacts of heatwaves in Europe”, https://doi.org/10.1038/s41467-021-26050-z; and Casanueva et al. (2020), “Escalating environmental summer heat exposure – a future threat for the European workforce”, https://doi.org/10.1007/s10113-020-01625-6.
Note: Satoshi Araki contributed to this box. More detailed results can be found in OECD (forthcoming[22]).
1. Park, Pankratz and Behrer (2021[21]) estimate that each day with temperature above 40 degrees Celsius increases the risk of workplace accidents by more than 10% with respect to a day with normal temperatures.
2. Information on exposure to high temperatures is not available in the 2021 wave of the European Working Conditions Survey. In the 2015 wave high temperatures are defined as temperatures which make the respondent perspire even when not working.
3. The information on exposure to high temperatures is self-reported by workers. The cross-country comparison may, therefore, be driven by some degree of cross-country differences in how heat is perceived. Country differences may also reflect differences in the degrees of adaptation to heat, so that it may be more difficult to cope with high temperatures in certain countries than others (Heutel, Miller and Molitor, 2021[26]). Moreover, the timing of the interviews differs across countries and may influence the reported exposure to heat. To correct for the differences in the timing of interview, the estimates presented in this box are the predictions of the exposure to heat in May, obtained by running a regression of the exposure to heat at country level on the share of respondents by month of interview.
2.1. Overview of measurement approaches
2.1.1. What is a “green job”?
Despite some standardisation efforts at international level3 there is still no universally accepted definition of green job in the literature (OECD, 2023[27]; Cedefop, 2019[28]), but the range of used approaches can broadly be labelled as “top-down” and “bottom-up” or a mixture of both (Valero et al., 2021[29]):
Top-down approaches consider as green all jobs within specific sectors, establishment or activities that contribute substantially to preserving or restoring environmental quality, maintain low emissions or reduce them, and minimise waste creation and pollution. This may be operationalised through a process-based approach, which incorporates direct and indirect emissions and therefore considers as green all production processes and service delivery using more resource‑efficient or environmentally friendly technologies, as defined with respect to a defined benchmark (Bontadini and Vona, 2023[30]; Verdolini and Vona, 2022[31]).4 More common, however, is a top-down, output-based approach, in which environmental activities are restricted to the production and delivery of environment-related goods and services. For example, the United Nations System of Environmental Economic Accounting (SEEA) defines “economic activities related to the environment” as those associated with environmental protection and resource management5 as well as the production of goods and services strictly connected with these activities (United Nations, 2012[32]). Consistent with this definition, Eurostat collects statistics on the environmental goods and service sector – based on national account data and described as the economic sector that generates environmental products, i.e. goods and services produced for environmental protection or resource management – and estimate its overall employment, referring to all workers employed in the sector (European Commission, 2016[33]; Vandeplas et al., 2022[34]). A similar approach is followed by the UK Office of National Statistics.6 An alternative top-down approach is to consider as “green jobs” all jobs in expanding sectors as identified using macroeconomic models simulating the impact of green policies – see Box 2.1 and Borgonovi et al. (2023[9]). No matter the method and precise definitions, all jobs in, for example, the renewable energy power generation sector are green according to this class of approaches, including administrative and support jobs.7
Bottom-up approaches start, by contrast, from the characteristics of jobs and occupations independently of the sector of the economy where they operate in. Researchers have applied this approach in different ways, for example by computing the share of green tasks that are specific to a subset of jobs and considering as green only jobs with a reasonably high green-task8 intensity – e.g. Peters (2013[35]), Elliott et al. (2021[36]), Office of National Statistics (2022[37]) and OECD (2023[27]), which all start from the list of green tasks by occupation as published and regularly updated by O*NET until 20199 (see Box 2.3)– or by examining the presence of “green” keywords (such as, for example, “solar”, “photovoltaic” or “wind”) in online job ads – e.g. Saussay et al. (2022[38]), Curtis and Marinescu (2023[39]) and Curtis, O’Kane and Park (2023[40]). In this approach, a wind turbine service technician is considered a green-job worker even if working for a company mainly active in fossil fuel electric power generation, while a security guard for a company in renewable energy power generation will not be considered green. This approach may also end up in a non-binary measure of greenness, for example based on the intensity of green tasks of each examined job – e.g. Vona et al. (2018[41]), Vona, Marin and Consoli (2018[42]) and Scholl, Turban and Gal (2023[43]).
Many of the attempts to measure green jobs are in practice a mixture of top-down and bottom-up approaches. For example, the, now discontinued, US Green Jobs Initiative of the Bureau of Labor Statistics employed an extensive definition of green jobs encompassing both green output production (“jobs in businesses that produce goods or provide services that benefit the environment or conserve natural resources”) and jobs involved in greening production processes of neutral or polluting establishments (“jobs in which workers’ duties involve making their establishment’s production processes more environmentally friendly or use fewer natural resources”) – see BLS (2013[44]). The O*NET Greening of the World of Work Project (Dierdorff et al., 2009[45]; 2011[46]) considers both the sectors and activities “related to reducing the use of fossil fuels, decreasing pollution and greenhouse gas emissions, increasing the efficiency of energy usage, recycling materials, and developing and adopting renewable sources of energy” (Dierdorff et al., 2009, p. 3[45]),10 and the occupations for which these activities and technologies are likely to increase demand, shape work and workers requirements, or generate new, unique work and worker requirements.
Box 2.3. The O*NET database
The O*NET database was created in 1998 by the U.S. Department of Labor, building on its predecessor the Dictionary of Occupational Titles (DOT), and is updated on a regular basis. O*NET contains a wealth of information on occupations, including tasks characterising work and skill and ability requirements in each of the about 1 000 occupations (Handel, 2016[47]).1 Most of this information is collected, and updated regularly, from job incumbents and occupations experts through surveys. More information on the O*NET database, and current and archive versions of the dataset can be found online.2
As O*NET is developed by the National Center for O*NET Development under the supervision of the Employment and Training Administration in the United States, it is geared towards the content of jobs in the labour market in the United States. Despite this, O*NET has been regularly used for the analysis of countries other than the United States. The assumption that occupational information from one country can be generalised to other countries has been tested and largely holds. For example, Handel (2012[48]) finds that occupational titles refer to very similar activities and skill demands across different countries. As a result, the O*NET information on tasks, skills and abilities has been used extensively in labour market research in high-income countries – see e.g. Deming (2017[49]); Brynjolfsson, Mitchell and Rock (2018[50]); Dingel and Neiman (2020[51]); and Webb (2020[52]) as well as many studies concerning “green jobs”, including e.g. Peters (2013[35]), Elliott and Lindley (2017[53]); Vona et al. (2018[41]); Vona, Marin and Consoli (2018[42]); Bowen, Kuralbayeva and Tipoe (2018[54]); and Popp et al. (2021[55]). More caution, however, may be needed about the use of O*NET to describe skills and tasks of occupations in low-income countries, as these could differ significantly in terms of technology and regulatory context compared to the United States – see also Vona (2021[56]).
Note: Julie Lassebie contributed to this box.
1. See O*NET Taxonomy at: www.onetcenter.org/taxonomy.html#latest.
One of the reasons for the lack of consensus on the definition of “green job” comes from the fact that the concept is used to try to shed light on very different policy questions. On the one hand, the concept of green job may be used to measure the contribution of the labour market to the transition towards a low-carbon economy (or more generally a low-pollution or “green” economy), as a sort of thermometer of the extent of the transition – e.g. Elliott and Lindley (2017[53]), Georgeson and Maslin (2019[57]). Relatedly, it can be used to identify and characterise jobs in the sectors directly benefitting from climate mitigation policies, including incentives to “clean energy” or “green” products and technologies, or to ask how easily workers move into low-emission activities – e.g. Bluedorn et al. (2022[58]), US White House (2023[59]) and European Commission (2023[60]) – see also Chapter 3. Consistently, in these cases, the definition of “green job” has tended to exclude those support jobs that are not directly involved in the production of green goods or the provision of green services or green processes but whose demand is likely to expand by backward linkages because of the implementation of climate change mitigation policies.11 The ILO further requires green jobs to be “decent” jobs, adding a requirement related to fundamental principles and rights at work, working conditions including wages, and access to social protection to the green dimension, thereby using the concept of green jobs as a “double thermometer” of the transition towards low-emission and decent employment (ILO, 2016[61]; van der Ree, 2019[62]).12
On the other hand, the term “green job” is also used to identify the type of jobs that will likely expand because of the policy-induced transition towards a low-carbon economy (or more generally a low-pollution, or green economy). This approach implies considering both the jobs that are directly involved in green or low-carbon activities, as defined above, and those that are linked to green or low-carbon activities by backward linkages or support activities, and therefore without necessarily involving green tasks. Jobs in expanding sectors and occupations are often identified using macroeconomic models – e.g. Vandeplas et al. (2022[34]); Eurofound (2023[12]); and Borgonovi et al. (2023[9]) – or specific sectoral or occupational analyses – e.g. Dierdorff et al. (2009[45]; 2011[46]); Asikainen et al. (2021[63]); Popp et al. (2021[55]); and Causa, Nguyen and Soldani (2024[64]).
Relatedly, policy makers often ask whether workers hold the skills required by the transition. In order to answer this question, researchers have examined the skills required for the jobs directly involved in the green/low-carbon activities (Consoli et al., 2016[65]; Vona et al., 2018[41]; Tyros, Andrews and de Serres, 2023[66]). Yet, answering this question may require focusing on all types of jobs that will be in demand, insofar as also a lack of adequate skills among the suppliers of intermediate inputs for green products and services may undermine the transition – see Borgonovi et al. (2023[9]) and Chapter 4.
Resulting estimates of the incidence of green jobs in the economy vary greatly, depending on the adopted definition. Top-down, output-based approaches tend to yield low estimates of the shares of green jobs in OECD economies of the order of 2% to 4% – see e.g. Elliott and Lindley (2017[53]), Georgeson and Maslin (2019[57]) and Bluedorn et al. (2022[58]). The same is true for bottom-up approaches relying on “green” keywords or computing continuous measures of task-intensity. For example, Saussay et al. (2022[38]) estimate that less than 1.5% of online job postings in the United States advertised between 2010 and 2019 concern low-carbon, and thus green jobs. Vona, Marin and Consoli (2018[42]) estimate the share of green tasks over total tasks for the same country to be about 3%. Yet, the UK Office of National Statistics, also taking into account the time spent on each task, estimates that around 7% to 8% of hours worked in the United Kingdom were spent on green tasks in 2019 (Office of National Statistics, 2022[37]). At the opposite side of the spectrum, bottom-up, binary approaches relying on occupational characteristics and including jobs that are indirectly green (that is, that will likely be in demand because of the transition without necessarily entailing green tasks) tend to yield higher estimates of the order of 20% – see e.g. Bowen, Kuralbayeva and Tipoe (2018[54]) and Valero et al. (2021[29]).13 Binary approaches excluding jobs that are indirectly green are somewhat in between these two extremes: OECD (2023[27]), for example, setting a minimum task-intensity threshold at 10%, finds 13% of green jobs in the United States in 2021 while, using a similar approach, Causa, Nguyen and Soldani (2024[64]) find 8% of green jobs in European countries.
2.1.2. The concept of “green-driven jobs” adopted in this publication
This edition of the OECD Employment Outlook focuses on the effect of climate change mitigation policies on employment and incomes, rather than investigating the symmetric issue of the contribution of the labour market to the green transition as an enabling factor. Therefore, this chapter takes a broader operational definition than many previous studies in the literature, including at the OECD (see Section 2.1.1), and considers all jobs that are likely to be affected by the net-zero transition and not just those that may be considered green as such.14
Ideally, to identify such broad group of jobs, one would like to use a general equilibrium model to classify expanding and contracting industries and jobs following the introduction of mitigation policies – that is using a top-down approach as defined in Section 2.1.1. However, while many existing macro models allow to identify precisely industries characterised by high levels of greenhouse gas (GHG) emissions, which are expected to downsize, and those occupations that are concentrated in these industries (see Box 2.4 and Chapter 3), the published results of these models are not sufficiently detailed about expanding industries – most of which are bundled together into generic low-emission industries (Borgonovi et al., 2023[9]; Eurofound, 2023[12]; Fragkiadakis, 2022[67]). Considering all jobs concentrated in this large low-emission sector would result in a too large list of positively impacted jobs.15
For these reasons, following most of the studies in the literature which try to identify those occupations that are likely to benefit from the net-zero transition, this chapter makes use of the O*NET Greening of the World of Work Project that, as discussed in Section 2.1.1, identifies a set of occupations that are likely to expand and/or being transformed by the transition. More precisely, the O*NET project distinguishes three groups of occupations of this type and a residual category:
Green new and emerging occupations: new occupations (entirely novel or “spinoffs” from an existing occupation) with unique tasks and worker requirements (e.g. Biomass Plant Engineers; Carbon Trading Analysts; Solar Photovoltaic Installers).
Green-enhanced skills occupations: existing occupations whose tasks, skills, knowledge, and external elements, such as credentials, tend to be altered because of the net-zero transition (e.g. Arbitrators, Mediators, and Conciliators; Architects; Automotive Specialty Technicians; Farmers and Ranchers). Note, however, that even if the net-zero transition alters the characteristics of these jobs, in non-green sectors of the economy (e.g. certain GHG-intensive industries – such as chemicals, fossil fuel power generation) these occupations may still be associated with the old (non-green) list of tasks, skills, knowledge and credentials and their demand may therefore not necessarily grow in the short term.16
Green increased demand occupations: existing occupations in increased demand due to the net-zero transition but with no significant changes in tasks or worker requirements. Some occupations in this group can be considered as directly contributing to low emissions and clearly involve green tasks (e.g. Environmental Scientists and Specialists; Forest and Conservation Workers) but most are not and should rather be seen as in support of green economic activities (e.g. Construction Workers; Drivers; Chemists and Materials Scientists).
Other occupations: all other occupations, including jobs associated with high-emission activities (e.g. Gas Compressor and Gas Pumping Station Operators) and low-emission, low-pollution jobs that are not directly or indirectly related to the reduction of the use of fossil fuels, pollution and greenhouse gas emissions, or the increase in the efficiency of energy usage, or material recycling, or the development of renewable sources of energy (e.g. Actuaries; Medical Appliance Technicians).
Box 2.4. Greenhouse gas-intensive industries and occupations
Following the literature (see Chapter 3), this edition of the Employment Outlook defines high-emission industries based on greenhouse gas (GHG) emissions, using 2‑digit ISIC rev.4 industry data for EU‑27 countries as well as the United Kingdom, Norway, Iceland and Switzerland over the period 2009‑20. For all countries (including countries for which data are not available), an industry is labelled “high-emission” if it ranks in the upper two deciles of emissions per unit of value added in at least 10 out of the countries for which data are available. These industries represent at least 70% of the GHG emissions in each OECD country for which data are available. This country invariant group of industries closely corresponds to the group of downsizing industries in the simulation of the effects of the European Union’s Fit for 55 (FF55) package through the OECD ENV-Linkages model (Borgonovi et al., 2023[9]). To improve consistency with the ENV-Linkages model, the Sewerage and Waste Collection industry is however omitted from the final list of high emission industries, as it is not projected to downsize – see Chapter 3.1
Occupations that are intensive in GHG emissions are defined as those occupations that are particularly concentrated in GHG-intensive industries. To obtain a list of GHG-intensive occupations that is comparable with green-driven occupations, without the comparisons being blurred by aggregation, these occupations are initially defined in the US Standard Occupational Classification (SOC) system in the following way. First, the list of GHG-intensive industries is mapped into the North American Industry Classification System (NAICS) at the 6‑digit level, adapting the Dingel and Neiman’s method (Dingel and Neiman, 2020[51]) – see Box 2.5 and Annex 2.A for details.2 Second, data on GHG-intensive industries are aggregated into 4‑digit NAICS industries (keeping 6‑digit for energy power generation industries) and, at this level of aggregation, the group of GHG-intensive industries is labelled GHG-intensive sector.3 Third, using 2019 US Occupation Employment and Wage Statistics on the number of employees by industry by 6‑digit SOC occupation, a GHG-intensive occupation is defined as an occupation that is highly concentrated in the GHG-intensive sector – operationalised as an occupation for which its share of employees in the GHG-intensive sector is at least 7 times larger than the share of the GHG-intensive sector in aggregate dependent employment, as in Vona et al. (2018[41]). Fourth, for countries other than the United States, SOC-based GHG-intensive occupations are then mapped into other occupational classifications using the same methodology as for green-driven occupations – see Box 2.5 and Annex 2.A.
1. One remaining difference with the FF55 simulation of the ENV-Linkages model is that the agricultural sector is kept in the high-emission category despite not being predicted to downsize in the FF55 simulation. Even if the EU FF55 package does not include significant action in agriculture, it can be argued that, because of the high GHG emissions of this sector, mitigation policies will have to target some of its subsectors in the future to meet the goal of zero net emissions by 2050. See for example the COP28 Declaration on Food and Agriculture, signed by 159 countries, including most OECD countries (www.cop28.com/en/food-and-agriculture).
2. For this step, 2015‑19 employment data from European Labour Force Surveys (EULFS), for ISIC, and 2019 employment data from US Quarterly Census Employment and Wage (QCEW) Statistics, for NAICS, are used.
3. To improve consistency with the ENV-Linkages model (and following common sense), the (tiny but rapidly expanding) sector of renewable energy power generation is excluded from the list of GHG-intensive industries at this step (this is possible at the 6‑digit level of the NAICS classification, but it would not be possible in the ISIC classification).
Although the impact of the net-zero transition is likely to be heterogeneous, all the first three groups listed above include occupations that are likely to be positively impacted by climate change mitigation policies. Using the latest version of the O*NET database (2019),17 these occupations will therefore be labelled “green-driven occupations” hereafter, to underline the fact that a few of them are not directly contributing to low emissions but, despite this, are still expected to be in demand because of backward linkages.
The approach adopted here, therefore, departs significantly from the task-based approach to green jobs used in most of the literature based on O*NET data. This is done for two reasons. On the one hand, as mentioned above, occupations with expanding demand because of the net-zero transition may have no or limited green-specific tasks. On the other hand, O*NET classifies into green and non-green only the tasks of green new and emerging occupations and green-enhanced skill occupations. Yet, simple inspections of the list of task descriptions (task statements in O*NET terminology) of green increased demand occupations, suggests that a few of them are clearly intensive in green tasks but, nonetheless, are de facto arbitrarily excluded by the studies in this literature because O*NET does not provide the green/non-green task classification for this group. For example, at least half of the core tasks of Forest and Conservation Technicians – a green increased demand occupation – are related to forest preservation and therefore maintenance of the forest carbon absorption potential, with an unambiguous, direct positive impact on green objectives. For all these reasons, green-task intensity does not appear ex ante a good predictor of the potential impact of mitigation policies on the growth of occupations. A binary approach – that is classifying occupations into green-driven and other occupations, although also distinguishing the three O*NET categories mentioned above – is therefore preferred here to a cardinal approach.
The grouping of green-driven occupations used for this chapter is defined by O*NET for the US Standard Occupational Classification (SOC) system at a very detailed level (8 digits – about 1 000 occupations). All other OECD countries, however, collect employment data using different classifications (notably the International Standard Classification of Occupations – ISCO), usually at a more aggregate level (4‑digit – about 400 occupations – in the case of ISCO). As existing crosswalks between classifications are usually such that each occupation in one classification corresponds to many occupations in another classification and vice versa – a situation often referred to as “many-to-many crosswalk” – this has often resulted in disparate estimates of the amount and characteristics of green-driven occupations outside North America – see Vona (2021[56]) and Section 2.1.1 above. Following previous OECD work (Basso et al., 2020[68]; Scholl, Turban and Gal, 2023[43]; Tyros, Andrews and de Serres, 2023[66]; Causa et al., 2024[69]; Causa, Nguyen and Soldani, 2024[64]), this chapter makes therefore use of the methodology developed by Dingel and Neiman (2020[51]), which allows improving the precision of available crosswalks and obtain unbiased estimates of the proportion of green-driven and other jobs in countries that do not use the US SOC classification system (see Box 2.5).18
Box 2.5. Cross-walking occupational classifications
Existing crosswalks between the different occupational classifications used in different OECD countries are typically many-to-many, meaning that each occupation in one classification corresponds to many occupations in another classification, and the correspondence between two couples of occupations is, therefore, only partial. Following previous OECD work, this chapter adapts and applies the methodology originally developed by Dingel and Neiman (2020[51]) for indicators of occupational characteristics that are shared by all jobs in the same occupation as defined in the standard occupational classification of one country (for example the proportion of workers in green-driven jobs, which takes the same value – 0 or 100% – within each 8‑digit SOC occupation). This methodology provides a weight to each combination of one occupation in the origin classification (origin occupation hereafter) and country (e.g. US SOC in the United States) and another in the target classification (target occupation) and country (e.g. ISCO at the 4‑digit level and Germany) that partially match each other. The weight is a function of the employment share of the origin and target occupations in their respective countries. The average value of each indicator (that is, continuing the example, the proportion of workers in green-driven jobs) for each target occupation and country is then obtained as a weighted average across all combinations that include the target occupation1 – see Annex 2.A for details.
One of the advantages of the procedure described above is that, because the employment-weighted average of group averages is the global average, there is no systematic aggregation bias as regards the country averages of each indicator that is invariant within each origin occupation. As a consequence, computing the incidence of green-driven occupations directly on 8‑digit SOC data or as a weighted-average of the estimated incidence in each target occupation (for example 4‑digit ISCO occupations) would yield the same result – see Annex 2.A for the proof. This is a crucial validation step of the procedure: as argued by Vona (2021[56]), a sensible validation test of the implementation of any crosswalk indeed requires that, for the same country, aggregate indicators constructed from crosswalked and original data are approximately the same.
Obviously, a key assumption and limitation of this approach is that the way occupations will be affected by the net-zero transition is similar across countries. This is a crude assumption, and it is possible to find counter-examples of occupations for which it is unlikely to hold.2 Concerning average statistics, this assumption, however, is probably less problematic in the case of binary indicators (such as green-driven or other occupations) than in the case of continuous measures of green-task intensity, as the intensity in green tasks is likely to differ according to differences in technology adoption across countries (Biagi, Vona and Bitat, 2021[56]).
The data at the level of the target occupation can then be matched with other datasets for the target country (e.g. labour force surveys) to estimate the average of the chosen indicator among groups with different characteristics – for example, the frequency of green-driven occupations among women. This will be obtained as a weighted average of the indicators for the target occupation where group-specific employment by occupation will be used as weight – that is, in the same example as above, a weighted average of the proportion of green-driven jobs by occupation, with female employment by occupation being used as weight.
1. This method does not necessarily yield a final binary indicator in each target occupation of the target country even when the origin indicator is binary. For example, the proportion of green-driven occupations is either 0% or 100% in the origin occupations and country. Yet, in the target occupation and country, most target occupations will have a proportion of green-driven jobs comprised between these two values.
2. For example, while Heavy and Tractor-Trailer Truck Drivers is considered a green increased demand occupation in the United States, which do not have a large network of electrified railways, this is unlikely to be correct for countries like Switzerland or Belgium, where the network of electrified railways is extensive.
2.2. Green-driven jobs: How many, where, who?
This section analyses the distribution of green-driven jobs across the OECD in comparison with GHG-intensive occupations, exploring their incidence across countries, regions and sectors, the evolution over the last decade and the characteristics of the workers holding these jobs. This analysis provides a snapshot of the distribution of green-driven and GHG-intensive occupations between 2015‑19 (to avoid the very strong labour market fluctuations generated by the COVID‑19 pandemic and the recovery that followed), not a projection of how many of these jobs there will be in the future and what will be their characteristics. The goal of this analysis is rather to identify which countries, regions or groups of workers are over-represented in green-driven occupations and, therefore, likely better placed to seize the opportunities that will be created by the green transition, or, conversely, are over-represented in GHG-intensive occupations and, hence, more at risk in the net-zero transition.
2.2.1. How many and where?
Across the OECD, between 2015 and 2019, around 20% of workers are employed in green-driven occupations (Figure 2.3, Panel A), ranging from about 15% in Greece to 25% in Estonia. On average, among these workers, about 46% are employed in green-enhanced skills occupations, i.e. existing occupations whose skill set is being altered because of the net-zero transition, and 40% in green increased demand occupations, i.e. existing occupations in increased demand due to the green transition but with no significant changes in tasks or worker requirements (Figure 2.3, Panel B). Only 14% are employed in green new and emerging occupations. The very large majority of workers employed in green-driven occupations are, therefore, employed in jobs that are not new. In fact, about two in five of these jobs are not even experiencing major changes in their work requirements.19
Occupations concentrated in high-emission industries employ around 6% of workers, ranging from almost 4% in Luxembourg to more than 10% in Poland (Figure 2.3, Panel A). The share of workers employed in GHG-intensive occupations is therefore significantly lower than the share of workers employed in green-driven occupations. However, it is higher than the share of workers employed in the new and emerging occupations (2.9%).20
Figure 2.3. One out of five workers is employed in green-driven occupations, but only few in new and emerging occupations
Percentages, average 2015‑19
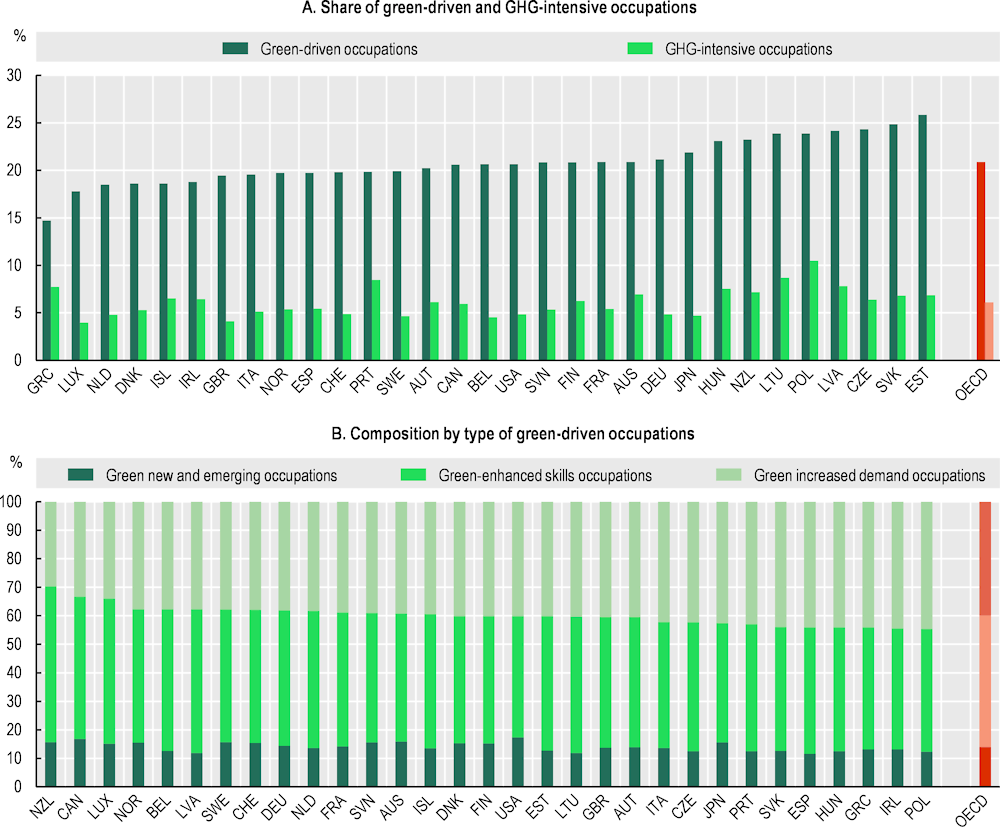
Note: Data refer to the average for 2015‑19, except for Canada: 2017‑19 and New Zealand: 2018. OECD: unweighted average of countries shown.
Source: Secretariat’s estimates based on version 24.1 of the O*NET database and the following country-specific sources: Australian Labour Force Survey; Canadian Labour Force Survey; Japanese Labour Force Survey; New Zealand: Household Labour Force Survey; United States: Current Population Survey; All other countries: EU Labour Force Survey.
It is important to recall that green-driven and GHG-intensive occupations are not mutually exclusive – see Section 2.1.2 above. There are indeed 2.3% of workers, on average, who are employed in occupations that are both green-driven and GHG-intensive (Annex Figure 2.C.1). These “mixed” occupations can be found, especially,21 in the group of green-enhanced skills occupations that are indeed occupations where skills and work requirements are changing because of the transition to net zero but may still be concentrated in high-emission sectors.
Between 2011 and 2022, the share of green-driven occupations in total employment has increased by 2% on average in European-OECD countries and the United States (Figure 2.4). This aggregate result masks diverging trends among green-driven occupations: in particular, Figure 2.4 shows that the incidence of green new and emerging occupations has increased by 12.9% over the same period22 while green increased demand occupations and green-enhanced skills occupations also increased but at a significantly slower rate. However, if those occupations that are both green-driven and GHG-intensive are excluded, the growth of the remaining, green-driven occupations (labelled in the chart as “green residual”) is more than twice as large (5%). This is because occupations concentrated in high-emission sectors, including those occupations that are both green-driven and GHG-intensive,23 experienced a ‑18% decline over the same period.24
Figure 2.4 suggests that the shares of green-driven and GHG-intensive occupations are positively correlated across countries,25 which is, to some extent, linked to countries’ industrial composition. Not the entire economy is equally affected by the net-zero transition. While green-driven occupations can be found in all sectors, Figure 2.5 shows that, compared to the distribution of employment across sectors, green-driven occupations are more likely to be found in manufacturing, utilities and mining, construction and transports. GHG-intensive occupations, partly as a result of the definition itself (see Box 2.4 above), are even more concentrated in these macro-sectors and agriculture (where high emission industries are). Other services, which represent more than two‑thirds of total employment, are composed mostly by occupations that are neither green-driven nor GHG-intensive.
Figure 2.4. Green new and emerging occupations are growing fast
Percentage change in the share of green-driven and GHG-intensive occupations in total employment
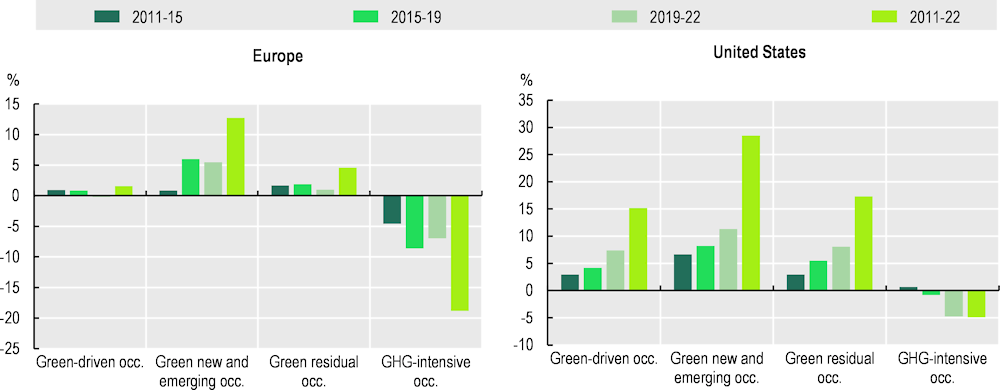
Note: Europe: unweighted average of Austria, Czechia, Estonia, Finland, France, Hungary, Lithuania, Luxembourg, the Netherlands, Norway, Poland, the Slovak Republic, Slovenia, Sweden and Switzerland. Green residual occupations are green-driven occupations excluding those occupations that can be both green-driven and GHG-intensive.
Source: Secretariat’s estimates based on version 24.1 of the O*NET database and the following country-specific sources: United States: Current Population Survey; All other countries: EU Labour Force Survey.
Figure 2.5. Green-driven occupations are more likely to be found in manufacturing, construction and transports
Percentages of employment by sector, average 2015‑19
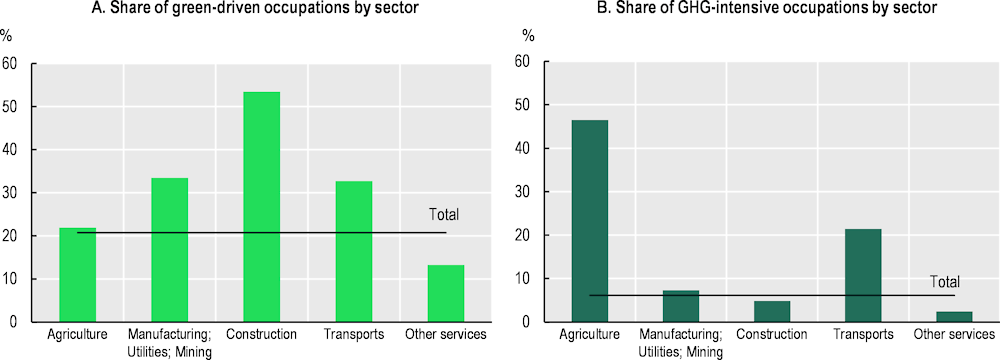
Note: Data refer to the unweighted average for 2015‑19 of Austria, Belgium, Czechia, Denmark, Estonia, Finland, France, Germany, Greece, Hungary, Iceland, Ireland, Italy, Latvia, Lithuania, Luxembourg, the Netherlands, Norway, Poland, Portugal, Slovak Republic, Slovenia, Spain, Sweden, Switzerland, the United Kingdom and the United States.
Source: Secretariat’s estimates based on version 24.1 of the O*NET database and the following country-specific sources: United States: Current Population Survey; All other countries: EU Labour Force Survey.
Given these strong sectoral patterns, it is not surprising, that in countries where the share of service sectors is high, e.g. Luxembourg, both the shares of green-driven and GHG-intensive occupations are lower than in countries where agriculture and manufacturing play a larger role, such as several central and eastern European countries. Nevertheless, Figure 2.3 shows that the gap between the shares of workers employed in green-driven and GHG-intensive occupations varies across countries, suggesting that, absent policy action, the transition to net-zero may be relatively more challenging for those countries where the gap is low such as Greece where there are less than two green-driven occupations for each GHG-intensive one than in those countries where the gap is large, as for instance in the United Kingdom, where there are almost five green-driven occupations for each GHG-intensive one.
The positive correlation between the shares of green-driven and GHG-intensive occupations that can be found across countries does not hold when descending at subnational level. Previous OECD work (2023[27]; 2023[70]) has already shown that there is substantial heterogeneity across regions in OECD countries with some having already benefitted from the net-zero transition while others having a high share of high-emission industries that are particularly at risk. Figure 2.6 shows that in all OECD countries occupations concentrated in high-emission sectors are more prevalent in rural areas26 (or non-metropolitan areas in the United States), where agriculture, mining and manufacturing tend to be concentrated. However, the data also show that, with the exception of Greece and Luxembourg, green-driven occupations are also more prevalent in rural areas.27 Yet, the areas where green-driven occupations are located tend not to be the same where occupations concentrated in high-emission sectors can be found, as already documented in Vona, Marin and Consoli (2018[42]) and Lim, Aklin and Frank (2023[71]) for the United States. In fact, the cross-region correlation between the shares of GHG-intensive and green-driven occupations is weak (0.21) and, when those occupations that are both green-driven and GHG-intensive are excluded, it becomes close to zero (Figure 2.7). OECD (2023[27]; 2023[70]) show that differences across regions are related to their degree of innovation, industrial composition, and the education of the workforce. Moreover, not all types of green-driven occupations are more prevalent in rural areas: new and emerging occupations which employ, on average, more high skilled and high educated workers, pay better wages and have higher job quality (see Sections 2.2.2 and 2.3) are, in fact, concentrated in urban areas. Without an effective policy strategy to mitigate the disadvantage of those regions most specialised in GHG-intensive activities and least specialised in green-driven occupations (see Box 2.6), there is a significant risk that the net-zero transition exacerbates regional disparities and endangers social cohesion.
Figure 2.6. Both green-driven and GHG-intensive occupations are more widespread in rural than in urban areas
Share of green-driven and GHG-intensive occupations by rural/urban area, average 2015‑19

Note: Countries are ranked by decreasing gap of the share in rural areas compared to total. For European countries, the degree of urbanisation is defined on the share of local population living in urban clusters and in urban centres. It classifies areas into three types: thinly populated area (rural area); intermediate density area (towns and suburbs/small urban area), and densely populated area (cities/large urban area). In the United States, rural and urban areas are defined as non-metropolitan and metropolitan areas, respectively.
Source: Secretariat’s estimates based on version 24.1 of the O*NET database and the following country-specific sources: United States: Current Population Survey; All other countries: EU Labour Force Survey.
Figure 2.7 shows the share of green-driven occupations net of GHG-intensive occupations (the so-called “residual green”) and GHG-intensive occupations at regional/state level in deviation in percentage points of the national average. This allows to identify in which regions jobs are relatively more at risk because of the net-zero transition, and which are relatively better placed to reap its benefits. In the top-left quadrant there are those regions/states where the deviation from the country average of GHG-intensity is significantly greater than that of green-driven intensity, i.e. regions/states that, as things stand, compared to the national average are more at risk of being left behind in the transition to net zero. In the bottom-right quadrant are those regions/states where the deviation from the country average of the share of GHG-intensive occupations is significantly lower than that of green-driven occupations, i.e. regions/states that, as things stand, are better placed to benefit from the transition to net zero compared to the national average. Figure 2.7 suggests that issues of geographic inequality may be particularly relevant in Poland which has regions both in the top-left and bottom-right quadrant, i.e. both regions well positioned to benefit from the net-zero transition as well as regions facing stronger challenges. To a more limited extent, similar geographical distribution issues emerge for Greece, Portugal and the United States.
Figure 2.7. Regions with higher incidence of GHG-intensive occupations are not necessarily the same as those with higher incidence of green-driven occupations
Share of green-driven occupations net of GHG-intensive occupations and GHG-intensive occupations at regional/state level in deviation of the national average, percentage points, average 2015‑19
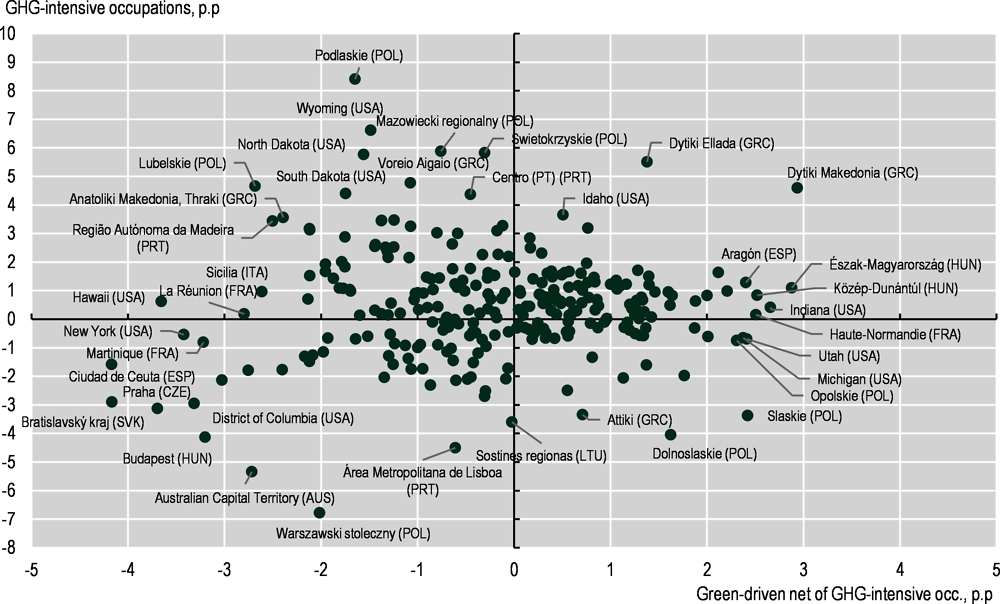
Reading: The Podlaskie Voivodeship in Poland or the state of Wyoming in the United States in the top-left quadrant have a share of GHG-intensive occupations compared to the national average significantly higher than that of green-driven occupations and, therefore, as things stand, are areas more at risk of being left behind in the transition to net zero compared to the national average. On the opposite, the Lower Silesian Voivodeship in Poland (Dolnośląskie) or Attica (Attiki) in Greece in the bottom-right quadrant have a share of GHG-intensive occupations significantly lower than that of green-driven occupations and, therefore, as things stand, are better placed to benefit from the transition to net zero compared to the national average.
Source: Secretariat’s estimates based on version 24.1 of the O*NET database and the following country-specific sources: Australian Labour Force Survey; Canadian Labour Force Survey; United States: Current Population Survey; All other countries: EU Labour Force Survey.
Box 2.6. Place‑based policies for a just transition
GHG-intensive industries and occupations are typically concentrated in specific regions. These are often different from the areas where green-driven occupations are more widespread and where the skills required by the net-zero transition are more common among the workforce – see Figure 2.7, Chapter 4 and OECD (2023[27]). Moreover, a successful transition to a net-zero emission economy requires new forms of production and, in some cases, new energy carriers and raw materials. These in turn require major transformations, with significant new climate‑neutral investment and infrastructure, while abandoning investment in production assets that are inconsistent with reaching climate neutrality. Regions differ in their conditions to access such infrastructure, for example because of their remoteness and/or because of economies of scale and agglomeration. For example, hydrogen is best transported via pipelines (and so is unabated carbon dioxide, when channelled to storage sites). This implies that concentrated production sites, such as chemical clusters in Belgium and Germany, will likely be better served by (new or reconditioned) pipelines and will face lower costs than dispersed sites. Moreover, the locations of existing pipelines may be quite different from those needed by expanding low-emission production (OECD, 2023[70]). In addition, the regions hosting industries with the biggest challenges to reach climate neutrality are often socio-economically relatively weak.1
To be effective, policies to facilitate the needed transformations, and support workers, need to take into account the fact that these regions as well as their workers and firms in key industries need support to make these transformations happen. This will help avoid further widening of regional economic inequalities and prevent a “geography of discontent” (Rodríguez-Pose, Dijkstra and Poelman, 2023[72]), risking a major backlash to climate action. In addition, the spatial distribution of these activities may change based on new patterns of comparative advantage of different regions. This is why many countries are focussing on placed-based policies to ensure a just transition. As Neumark and Simpson (2015, p. 1198[73]) put it, place‑based policies can be defined as interventions of a government “to enhance the economic performance of an area within its jurisdiction, typically in the form of more job opportunities” – see also OECD (2018[74]). Place‑based policies are particularly suitable for interventions in the context of a just transition to reach climate neutrality, as they can harness local stakeholders around the joint use of new climate neutral infrastructure. Two complementary approaches can roughly be distinguished in the context of climate‑change mitigation:
Targeting green subsidies and spending to enhance the transition towards green production to areas that are most vulnerable to the effects of the transformations to reach climate neutrality, for example because they are specialised in GHG-intensive industries.
Targeting compensatory interventions to the most negatively affected regions and people, but without orienting them specifically towards green activities, while keeping climate‑change mitigation policies mostly space‑blind.2 In this case place‑based policy interventions will aim at exploiting the relative comparative advantage of people and regions and will be geared to the most feasible transitions towards high-growing activities, which may be promising independently from climate‑change mitigation policies.
The first approach is at the heart of the US Inflation Reduction Act (IRA) of 2022.3 The IRA includes a combination of grants, loans, tax provisions and other incentives to accelerate the deployment of clean energy, vehicles, buildings, and manufacturing. In total, around USD 370 billion will be disbursed for measures dedicated to improving energy security and accelerating clean energy transitions, with much of this amount being targeted to communities that have been identified as negatively affected by the phasing out of emission-intensive technologies and productions.4 In practice this implies stimulating a transition of the most affected regions from being specialised in GHG-intensive activities to being specialised in green activities.
The European Union’s strategy, articulated around the European Green Deal5 and a number of cohesion-fostering instruments,6 can be seen as a mix of the two approaches. On the one hand, about one‑third of the European Regional Development Fund and the EU Cohesion Fund are specifically devoted to actions aiming to achieve climate neutrality by 2050. On the other hand, the Just Transition Mechanism provides targeted support to help mobilise around EUR 55 billion over the period 2021‑27 to alleviate the socio‑economic impact of the transition in the most affected regions, but includes, in its scope, all types of projects that do no significant harm to the environmental objectives.7
Whatever the approach taken, labour market policy interventions to accompany and support workers and communities along the net-zero transition need to incorporate the local dimension to be effective, for example by steering transitions towards industries and occupations where local labour shortages already exist or that are expected to emerge because of place‑based investment and infrastructure programmes. Locally-based skill anticipation exercises may play a key role in this respect – see e.g. OECD (2023[27]).
In some cases, however, the economy of regions, which are mostly affected by the downsizing of GHG-intensive industries, may have a limited capacity of supplying enough good quality jobs in the short term. In those cases, complementary geographical mobility policies might be needed. This would require an integrated approach overcoming all barriers to mobility (including information provision, job-search assistance, housing assistance, childcare support, etc…), since it has been shown that financial incentives to mobility alone may be insufficient and result in bad-quality and unstable jobs in the destination location – see e.g. Caliendo, Künn and Mahlstedt (2023[75]). For example, in Sweden, the EU-financed “Relocate and React EU Relocate” project, concluded in 2023, organised a network of public employment services in cities and regions with high unemployment, which informed, recruited, and supported unemployed adults who were interested in moving to the area of the Northern city of Skellefteå, which was facing significant labour shortage due to significant development of green activities – see Chapter 4. However, in most countries, policies for geographical mobility remain unrelated to their green strategy. According to the answers to the OECD policy questionnaire on labour and social policies for the net-zero transition8, only seven out of the 35 countries which have provided a response, have programmes to support relocating workers’ housing needs, and they are not specifically linked to the transition to net zero.
1. For example, in the EU their GDP per capita and wages are more than 30% lower than national averages. Their firms in GHG-intensive industries may be less able to invest in needed new technologies, while workers are often unskilled. In some of these regions, many young workers are on temporary contracts, making access to needed training more difficult.
2. Space‑blind policies can be defined as policies that are not targeted to the specifics of any particular place (World Bank, 2008[76]).
5. See https://commission.europa.eu/strategy-and-policy/priorities-2019-2024/european-green-deal_en.
8. A policy questionnaire on the labour and social policies that OECD member countries have in place or are developing to accompany the net-zero transition was distributed at the end of 2023. Some of the responses are reported in this Employment Outlook, while the full overview will be made available in a forthcoming policy brief.
2.2.2. Who?
How do workers employed in green-driven occupations compare to those employed in occupations concentrated in high-emission sectors? Who are the workers likely better placed to seize the opportunities of the net-zero transition? And, conversely, who are those more at risk because employed in occupations concentrated in high-emission sectors? Figure 2.8 summarises the main characteristics while Annex 2.C provides country-specific results. The findings that emerge are the following:
On average, men are more likely to hold green-driven occupations. At the same time, men are also more likely to be employed in occupations concentrated in high-emission sectors. This is in line with past analyses and reflects the fact that most green-driven and GHG-intensive occupations can be found in agriculture, manufacturing, mining and utilities and transports while women are predominantly employed in other service sectors. Remarkably, all OECD countries display such stark gender gap (Annex Figure 2.C.2).28 This suggests that men stand to be both those more exposed to the risk of job loss in high-emission industries (see Chapter 3) but also better placed to reap the benefits of the net-zero transition. On the opposite, the concentration of women in services puts them less at risk of transition-induced job losses29 but also raises concerns about their ability to benefit from the job opportunities that will open. In particular, the current underrepresentation of females in science, technology, engineering and mathematics (STEM) educational fields and enduring gender stereotypes raise concerns about women’s capacity to benefit from the growing employment opportunities in the best paying expanding sectors (OECD, 2021[2]).
Differences across age groups are very small (Figure 2.8), but, on average, young workers (between 15 and 34 years old) tend to be slightly less likely to be employed in both green-driven and GHG-intensive occupations. This pattern holds in the large majority of OECD countries (Annex Figure 2.C.3) and across types of green-driven jobs with the only exception of green increased demand occupations where young workers are slightly more likely to be employed than prime‑age and older workers (Annex Figure 2.C.4). In contrast, prime‑age workers (between 35 and 54 years old) are slightly more likely to be employed in a green-driven occupation while older workers (older than 54) are more likely to be employed in occupations concentrated in high-emission sectors. While on the one hand this suggests that the reallocation challenge may be more significant for workers who, on average, already experience practical and cultural difficulties in reskilling and finding a new occupation, these results also suggest that part of the reallocation process may be managed by natural attrition of older workers retiring at the normal age.
In terms of occupational categories, Figure 2.8 shows that green-driven occupations are not more likely to be found among occupations in the top of the pay and skill distribution (high-skill occupations hereafter, including managers, professionals and technicians and associate professionals) but rather among occupations in the middle of the pay and skill distribution (medium-skill occupations hereafter, including clerical support workers, craft and related trades workers, plant and machine operators, and assemblers). On the opposite, the share of green-driven occupations among occupations at the bottom of the pay and skill distribution (low-skill occupations hereafter: service and sales workers and elementary occupations) is much lower than the average. These results also hold for occupations concentrated in high-emission sectors which are more prevalent among occupations in the middle of the pay and skill distribution than those at the top and the bottom. The patterns are very similar across countries (Annex Figure 2.C.5). These results differ from those of comparable analyses that had a narrow focus on a subset of green-driven jobs: in fact, the occupational pattern varies significantly across types of green-driven occupations. Annex Figure 2.C.6 shows that in all countries, new and emerging occupations are much more frequent among high-skill occupations, while it is the green-enhanced skills occupations and increased demand ones which are more frequent among medium-skill occupations.
Finally, a different picture than for occupational categories emerges when looking at education: workers with low levels of education are more likely to be in both green-driven and GHG-intensive occupations relative to workers with middle and high levels of education (Figure 2.8) with remarkably similar patterns across countries (Annex Figure 2.C.7). This aggregate result is again driven by the groups of green-enhanced skills occupations and increased demand ones while new and emerging occupations, with the exception of the Slovak Republic, are more prevalent among high-educated workers – see Annex Figure 2.C.8.
Figure 2.8. Workers in green-driven and GHG-intensive occupations tend to have similar characteristics
Gap in percentage points between the share of green-driven (respectively GHG-intensive occupations) in each characteristic and the share in the whole economy

Note: The figure reports gaps in percentage points between the share of green-driven (Panel A) and GHG-intensive (Panel B) occupations by characteristics and the average share. Data refer to the average for 2015‑19, except for Canada: 2017‑19. In terms of age, youth are 15‑34 years old, middle 35‑54 years old, older 55+ year old. In terms of occupation, low-skill refers to Service and Sales Workers and Elementary Occupations (ISCO‑08 5 and 9, medium-skill to Clerical Support Workers, Craft and Related Trades Workers and Plant and Machine Operators, and Assemblers (ISCO‑08 4, 7 and 8), high-skill to Managers, Professionals and Technicians and Associate Professionals (ISCO‑08 1, 2 and 3). Low education refers to less than upper secondary, medium education to upper secondary, high education to more than upper secondary. Unweighted average of Australia, Austria, Belgium, Canada, Czechia, Denmark, Estonia, Finland, France, Germany, Greece, Hungary, Iceland, Ireland, Italy, Japan, Latvia, Lithuania, Luxembourg, the Netherlands, Norway, Poland, Portugal, Slovak Republic, Slovenia, Spain, Sweden, Switzerland, the United Kingdom and the United States.
Reading: The share of women employed in green-driven occupations is 9 percentage points lower than the share of women employed on average.
Source: Secretariat’s estimates based on version 24.1 of the O*NET database and the following country-specific sources: Australian Labour Force Survey (gender, age); Canadian Labour Force Survey (gender, age, education); Japanese Labour Force Survey (gender, age); United States: Current Population Survey; All other countries: EU Labour Force Survey.
In conclusion, the implementation of ambitious measures to combat climate change will result in decreased employment levels within GHG-intensive industries to the benefit of renewable energy generation as well as a larger number of industries that will be instrumental to make the transition happen, such as certain segments in construction and transport. This reallocation may also give rise to disparities across workers and regions. The analysis shown in this section suggests that, even if the reallocation process will go well beyond the two relatively small groups of GHG-intensive and green-driven occupations, these two groups have some similarities in common in terms of the characteristics of the workers who hold them that could help to make the transition manageable. And yet, the risk of widening inequalities across demographic groups and geographical areas is real – see Botta (2019[77]); OECD (2021[2]); and Chapters 3 and 4. In particular, the analysis has shown that green-driven occupations are a heterogenous group of jobs: fast-growing green new and emerging occupations are typically high-skill and employ highly educated workers in urban areas while the much larger but less dynamic groups of green-enhanced skills occupations and green increased demand ones are on average relatively more medium- and low-skill and employ many more low-educated workers.
Interestingly, the characteristics of the workers employed in declining GHG-intensive occupations are remarkably similar to those of the workers more exposed to high temperatures, as shown in Box 2.2. This suggests that while climate change mitigation policies may have costs for some groups of workers, there are also labour market costs of inaction for these same groups.
2.3. Green-driven jobs: Are they good?
How attractive are green-driven jobs? A successful transition towards a low-carbon economy not only will induce greater demand for green-driven occupations but also requires greater workers’ willingness to search for, and pick up, job offers in these occupations. As recent evidence on labour shortages has demonstrated, the attractiveness of a job depends not only on its wage but also on other aspects of job quality (OECD, 2022[78]; OECD, 2023[79]). Workers indeed consider wages and working conditions together when evaluating jobs and job offers, and are ready to trade off part of their wage for terms and conditions of employment that they consider to be better – see e.g. Mas and Pallais (2017[80]); Taber and Vejlin (2020[81]); Albanese and Gallo (2020[82]); and Bassanini et al. (forthcoming[83]). Jobs in (shrinking) GHG-intensive industries are typically regarded as relatively good jobs, paying comparatively well (see also Chapter 3) and with a prevalence of permanent, full-time jobs. Overall, a key policy concern is that developing green-driven jobs may imply worsening job quality (Verdolini and Vona, 2022[31]).
This section, therefore, investigates the quality of green-driven occupations following the structure of the OECD job quality framework, which focuses on earnings, labour market security and the quality of the working environment (see Chapter 1).30 Obviously, increasing demand in green-driven occupations will likely improve job quality in these occupations as companies will have to compete for workers. Nevertheless, current job quality is likely to be a good predictor of current job attractiveness and future job quality. However, to the extent that data on green-driven occupations for most countries are constructed using a complex crosswalk between the US SOC classification and the occupational classifications adopted in other countries (see Box 2.5), average differences in labour market performance variables (such as wages) between green-driven and other occupations are likely to suffer from a significant aggregation bias (see Box 2.7). To minimise bias issues, in this chapter job quality variables are transformed into discrete categories (for example high- and low-wage earners) and the share of green-driven occupations within these categories is estimated. Qualitative implications for job quality gaps between green-driven and other occupations can then be easily derived. For example, a relatively high share of green-driven occupations among high-wage workers (and a low share within low-wage workers) would be indicative of a positive average wage gap between green-driven and other occupations.
Box 2.7. Why are data on job quality presented in this chapter as frequencies of green-driven occupations among good- or bad-quality jobs?
All countries included in the analysis of this chapter, except the United States, do not use the US SOC occupational system. In these countries, therefore, the cross-walking procedure developed by Dingel and Neiman (2020[51]) is adapted and applied to obtain estimated shares of green-driven (resp. other) occupations within aggregate cells defined based on the occupational classification of each country – see Box 2.5, and Annex 2.A for details. For any given cell, these estimates could be seen as an estimate of the probability that each individual’s job in that cell is a green-driven (resp. other) occupation. The data can then be matched with individual-level data reporting the cell each individual belongs to.
Once data are matched, it may seem natural to use the estimated shares of green-driven (resp. other) occupations in each cell as weights to estimate the average level of those variables that vary at the individual level but are not an invariant characteristic of the occupation. For example, the average wage of green-driven (resp. other) occupations could be obtained by aggregating cell-level average wages using, for each cell, the product of the employment share of the cell in total employment multiplied by the estimated proportion of green-driven (resp. other) jobs in that cell as weight. The average wage gap can then be obtained as the difference between the two average variables.
As shown in Annex 2.B, however, this procedure would underestimate the average wage gap between green-driven and other occupations, with the error being larger the larger the employment share of cells with the incidence of green-driven jobs close to 50%. The same applies to the gap in any variable which vary within occupations. For this reason, in this chapter, the analysis of differences across individual job quality indicators, such as wages, is performed by building a small number of discrete categories in the population (when these do not already exist in the data) and estimating differences in the incidence of green-driven and other occupations within these categories. For example, in the case of wages, three categories (low, middle and high wage) are identified, with the high-wage (resp. low-wage) category being defined as gross hourly wage larger than, or equal to, one‑and-a-half times (resp. smaller than two‑thirds of) the median wage in the country. Then, the proportion of green-driven and other occupations in each category are computed. A larger (resp. lower) incidence of green-driven jobs in the high-wage (resp. low-wage) category than in the whole economy would then be cautiously interpreted as evidence of a positive wage gap1 – and the larger (resp. lower) the share of green-driven jobs in the high-wage (resp. low-wage) category, the larger the wage gap.2 It can be shown that, mathematically, the risk of aggregation bias is limited, in this case, and empirical tests on US data suggests that such a bias is, at worst, small – see Annex 2.B.
1. A reading note is added in all charts to facilitate the interpretation of the results.
2. Rigorously speaking, a regularity condition (a weak monotonicity of the wage gap as a function of the wage categories used in the analysis) is necessary for this interpretation to hold.
2.3.1. Wages
Green-driven occupations tend to pay higher than average hourly wages. On average among the OECD countries for which data are available, the incidence of green-driven occupations is indeed larger among high-wage employees31 (22%) than among low-wage employees (18.8%) – Figure 2.9, Panel A. In between these values, the incidence of green-driven occupations among middle‑wage employees (20.6% on average) is close to the incidence in the overall economy. The gap between high-wage and low-wage workers remains robust to controlling for worker characteristics such as age, gender and education (Figure 2.9, Panel B).32 On the opposite, GHG-intensive occupations are more frequent among low and middle‑wage employees than among high-wage employees – see also Annex Figure 2.C.9. Nevertheless, the available evidence suggests that the negative wage gap between GHG-intensive and other occupations is relatively more important for men than for women, while there are no significant gender differences as regards wage gaps between green-driven and other occupations – see Annex Figure 2.C.10. This suggests that the transition to net-zero emissions may lead to a larger reduction of low-paid jobs for men than for women and, mechanically, a slowdown in the aggregate wage convergence across genders (even if the job-specific pace of convergence is unaffected).33
The aggregate figures concerning green-driven occupations, however, conceal significant cross-country heterogeneity. The incidence of these occupations is larger among high-wage employees than among low- or middle‑wage employees in 16 out of the 26 OECD countries for which estimates are possible (Figure 2.9, Panel A). The largest difference between high-wage and low-wage employees, pointing to the largest wage gap between green-driven and other occupations, is found in Nordic and English-speaking countries – ranging between 7 percentage points in Finland and 16 percentage points in Norway, where over 30% of high-wage employees work in green-driven occupations.34 In other six countries, the largest incidence of green-driven occupations is found among middle‑wage employees. However, in Hungary, Italy, the Netherlands and Slovenia, green-driven occupations are more frequent among low-wage employees, suggesting a negative wage gap between green-driven and other occupations in these countries. By contrast, in all countries except Norway and Greece, the evidence presented in Annex Figure 2.C.9 suggests a negative wage premium for GHG-intensive occupations.35
Figure 2.9. Jobs in green-driven occupations are on average well paid
Percentage incidence by hourly wage category, 2018

Note: Panel A reports the percentage of green-driven occupations in wage and salary employment, by country and hourly wage category. Panel B reports the point estimate (and 95% confidence intervals) of the percentage difference in the incidence of each type of occupation between high and low-wage employees. High (resp. low) wage is defined as hourly wage above one‑and-a-half times (resp. below two‑thirds of) the median wage. “Total” refers to the share of green-driven occupations among all employees. Agriculture is excluded except in Australia, Canada and the United States in Panel A. Data for Belgium, Denmark, France, Greece, Iceland, Italy, Luxembourg, Portugal, Sweden and the United Kingdom do not include firms with less than 10 employees. Data for Canada and the United States refer to 2019. OECD: unweighted average of countries shown. Australia is not included in Panel B. GHG: greenhouse gases. In Panel A, countries are ranked by the difference in the incidence of green-driven occupations between high and low wage workers. Estimates of percentage effects in Panel B are obtained from a linear regression with the inverse hyperbolic sine of the share of each type of occupation as dependent variable and including high and middle‑wage dummies, educational attainment (3 classes), gender, age (3 classes), and country dummies as explanatory variables and standard errors clustered on the dimensions of variability of the dependent variable. The reported point estimates and confidence intervals refer to the estimated coefficient of the high wage dummy and are expressed in percentage of the untransformed dependent variable.
Reading: Panel A: In Norway, 30.6% (resp. 19.7% and 14.6%) of high-wage (resp. middle‑wage and low-wage) employees have a job in a green-driven occupation. Panel B: Controlling for demographic characteristics, the percentage share of green-driven occupations is, on average, 58% higher among high-wage employees than among low-wage employees. A larger incidence of a given type of occupation among high-wage workers than among middle or low-wage workers is indicative of a positive wage gap between that occupation and the others.
Source: Secretariat’s estimates based on version 24.1 of the O*NET database and the following country-specific sources: Australia: Table Builder of the Australian Bureau of Statistics (Labour Force: Characteristics of Employment); Canada: Canadian Labour Force Survey; United States: Current Population Survey; All other countries: EU Structure of Earnings Surveys.
The negative wage premium for GHG-intensive occupations may seem surprising given that jobs in GHG-intensive industries36 are typically regarded as relatively well-paid jobs, a stylised fact that is confirmed in the data (see Chapter 3). However, as shown in Chapter 3, the typical worker in GHG-intensive occupations has much lower education and hold a lower-skill job than the average worker in these industries. Moreover, by definition, workers in GHG-intensive occupations have fewer options outside downsizing GHG-intensive industries, which may put additional pressure on their bargaining power, while this argument does not apply to workers in these industries that have a job which is frequent also in other industries and can therefore more easily change sector of activity.
Given the composition of the three types of green-driven jobs discussed in the previous section, it is not surprising that, in all countries, green new and emerging occupations are much more frequent among high-wage employees: the incidence of these occupations is, on average, four times larger (or 3.9 percentage points larger) among high-wage than among low-wage employees (see Annex Figure 2.C.11). By contrast, the opposite holds for green increased demand occupations: in all countries, these occupations are more frequent among low- or middle‑wage employees than among their higher paid counterparts – the incidence of these occupations among low-wage employees is 40% larger (or 2.8 percentage points larger) than among high-wage workers.37 Green-enhanced skills occupations are somewhat in between these two opposites, as they are more frequent among high-wage employees than among other employees in 16 out of the 26 countries with available data.38
Part of the differences between high- and low-wage employees in the incidence of different types of green-driven occupations is due to workers sorting – for example, high-educated workers, which are usually better paid, are more frequent among new and emerging green occupations – see Section 2.2.2. In fact, controlling for demographic characteristics wipes out the negative effect concerning green increased demand jobs and reduces the positive effect for new and emerging occupations, which nonetheless remain very large (Figure 2.9, Panel B). Yet, the estimated average difference between high and low-wage employees is larger for green-enhanced skills occupations, suggesting worker sorting is far from fully explaining wage differentials.39
Another explanatory factor is that, in all countries, green new and emerging occupations are mainly high-skill occupations,40 that is belong to a class of occupations that typically pay higher salaries, while the other two types of green-driven jobs are rather concentrated in middle‑ and low-skill occupations, which typically pay lower wages – see Section 2.2.2. Controlling also for main job characteristics (sectors and 1‑digit ISCO occupations), the difference between low-wage and high-wage employees concerning the incidence of green-driven jobs, no matter their type, becomes smaller (Figure 2.10),41 showing that the results of Figure 2.9 are mainly due to this composition effect.42
Further analysis, however, suggests that all types of high-skill, green-driven occupations, with the exception of green-enhanced skills occupations, are better paid than other high-skill occupations (Figure 2.10). Controlling for demographic and job characteristics, the shares of green new and emerging and green increased demand occupations are estimated to be, respectively, 46% and 43% higher among high-skill, high-wage workers than among high-skill, low-wage workers.43 A similar pattern emerges for medium-skill occupations. These results likely reflect the specific scientific and technical competences that are still in scarce supply in the labour market but are required by these emerging occupations (see also Chapter 4). At the same time, all low-skill, green-driven occupations are less frequent among high-wage employees (and more frequent among low-wage employees) than other low-skill occupations – although results are statistically insignificant for new and emerging occupations – suggesting that low-skilled, green-driven occupations pay on average lower wages than other low-skill jobs.44 The latter finding may result from the fact that the skill requirements of these jobs are not different from other jobs (and, in particular, in declining GHG-intensive occupations), so that labour supply may well exceed labour demand in these labour market segments. Lower wages in low-skill jobs may also result from lower workers’ bargaining power, for example because green-driven occupations may have a lower level of unionisation (see Box 2.8 for evidence on the United States) and low-skill workers tend to have low bargaining power when not protected by collective representation – see e.g. Cahuc, Postel-Vinay and Robin (2006[84]) and Caldwell and Danieli (forthcoming[85]).
Figure 2.10. Green-driven occupations are often better paid than other high-skill occupations
Estimated percentage difference in the share of green-driven occupations between high and low-wage workers, by type of occupation and controlling for main demographic and job characteristics, 2015‑19
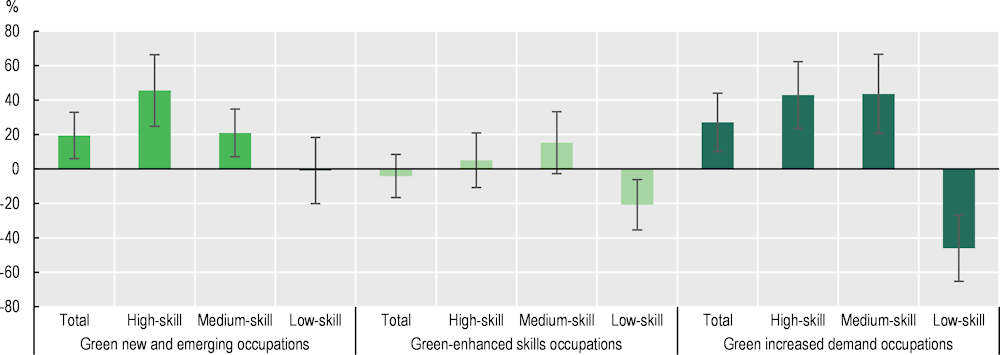
Note: The chart reports the point estimate (and 95% confidence intervals) of the difference in the incidence of each type of green-driven occupation between high and low-wage employees. High (resp. low) wage is defined as hourly wage above one‑and-a-half times (resp. below two‑thirds of) the median wage. Estimates are obtained from a linear regression with the inverse hyperbolic sine of the share of each type of occupation within all, high, medium or low-skilled occupations as dependent variable and including high and middle‑wage dummies, the share of 1‑digit ISCO occupations, educational attainment (3 classes), gender, age (3 classes), sectors (5 categories), and country dummies as explanatory variables. Except for the case of all occupations, regressions are estimated separately for high, medium, and low-skill occupations. “Total” indicates the full sample with all occupations. Standard errors are clustered on the dimensions of variability of the dependent variable. The reported point estimates and confidence intervals refer to the estimated coefficient of the high wage dummy and are expressed in percentage of the untransformed dependent variable. The sample excludes Agriculture and includes Belgium, Czechia, Denmark, Estonia, Finland, France, Germany, Greece, Hungary, Iceland, Italy, Latvia, Lithuania, Luxembourg, the Netherlands, Norway, Poland, Portugal, the Slovak Republic, Slovenia, Spain, Sweden, the United Kingdom, the United States. It does not include Agriculture and firms with less than 10 employees in Belgium, Denmark, France, Greece, Iceland, Italy, Luxembourg, Portugal, Sweden and the United Kingdom. Data for the United States refer to 2019.
Reading: Controlling for demographic and job characteristics and restricting the sample to high-skill occupations, the percentage share of high-skill, green new and emerging occupations is, on average, 46% higher among high-wage employees than among low-wage employees. A larger incidence of a given type of green-driven occupation among high-wage workers than among middle or low-wage workers is indicative of a positive wage gap between that occupation and the others included in the regression.
Source: Secretariat’s estimates based on version 24.1 of the O*NET database and the Current Population Survey, for the United States and the EU Structure of Earnings Surveys, for all other countries.
Overall, the good news is that, on average, green-driven jobs tend to be better paid (or at least, not less paid) than other jobs. Moreover, for many types of high-skill and medium-skill, green-driven occupations, including support jobs, there is a significant wage premium, which makes them not only attractive but likely reachable for similar level of competences (see Chapter 4). The bad news is that this does not hold for low-skill, green-driven occupations: jobs in these occupations are usually less paid than other low-skill jobs. These results point to the importance not only of upskilling policies (Chapter 4) but also of other labour market policies, including, potentially, certain forms of wage insurance (Chapter 3), to facilitate and accompany the transition.
Box 2.8. Are green jobs union jobs?
In some countries, trade unions are concerned that the reallocation of workers from emission-intensive activities to low emission ones may also imply a shift away from jobs that are more likely to be unionised to new jobs that are less likely to be unionised and therefore may offer worse working conditions. This was one of the issues at the centre of the long strike by the United Auto Workers (UAW) in the United States in the fall of 2023: UAW wanted guarantees that existing collective agreements will apply also in the new battery plants that will be built in the coming years to ensure that workers working in factories dedicated to internal combustion engines will be able to move to new plants while maintaining the same structure of wages and benefits.1 But even in countries where trade union membership and collective bargaining coverage is high, the transition to net zero may lead to a shift away from the most organised sectors. In Sweden, for instance, the electric carmaker Tesla experienced its first strike around the world in November 2023 over its “no‑union policy” and its refusal to negotiate a collective agreement for its repair and service shops across Sweden.2
In OECD countries where collective agreements are negotiated predominantly at sectoral level, the risk of a shift away from existing agreements is more limited since in some countries collective agreements are, under certain criteria, extended to all companies and/or employers’ organisations also have an interest in having all companies applying the provisions set in collective agreements to ensure a level-playing field among them. In contrast, in countries where collective agreements are negotiated exclusively at the firm level, ensuring that the provisions set in the collective agreement continue to apply to new companies and plants may be more challenging. This is especially the case in the United States, where a hard-fought plant-by-plant vote is often required to establish a union and negotiate a collective agreement.
Figure 2.11 shows that, at least in the United States, these concerns are warranted: workers employed in occupations concentrated in high-emission sectors are more frequent among those covered by a collective agreement than among other workers (in other words, GHG-intensive occupations are more likely to be covered by a collective agreement) while workers in green-driven occupations, in particular those in new and emerging occupations and in green-enhanced skills ones, are less likely to be found among those with a collective agreement. While unionisation patterns for the United States may not be representative of OECD countries, a similar analysis for the European Union by Zwysen (2024[86]) shows that, despite very different institutional settings, the coverage rate by collective agreements is also substantially higher in GHG-intensive occupations than among other occupations; therefore, without an increase in bargaining coverage among the latter, it is set to decline as GHG-intensive industries shrink.
To address, at least in part, unions’ concerns that the shift to net-zero emissions may imply a reduction in wages and benefits for the workers directly involved, the United States Inflation Reduction Act, a federal law aimed at promoting clean energy (among other objectives), includes specific provisions to favour companies that pay wages in line with those set in collective agreements.
Figure 2.11. In the United States, workers in green-driven occupations are less likely to be covered by a collective agreement
Marginal effect of being covered by a collective agreement on the share of green-driven occupations, controlling for main individual characteristics, 2015‑19

Note: The chart reports the point estimate (and 95% confidence intervals) of the percentage difference in the incidence of each type of green-driven occupation between employees covered by a collective agreement and those not covered. Estimates are obtained from a linear regression with the inverse hyperbolic sine of the share of each type of green-driven occupation as dependent variable and including a collective agreement dummy, educational attainment (3 classes), gender, age (3 classes), time dummies as explanatory variables. Standard errors are clustered on the dimensions of variability of the dependent variable. The reported point estimates and confidence intervals refer to the estimated coefficient of the fixed-term contract dummy and are expressed in percentage of the untransformed dependent variable.
Reading: Controlling for demographic characteristics, the percentage share of green-driven occupations is 25.8% lower among workers covered by a collective agreement than among those not covered while the percentage share of GHG-intensive occupations is 59.6% higher among workers covered by a collective agreement than among those not covered.
Source: Secretariat’s estimates based on the Current Population Survey, U.S Census Bureau.
2.3.2. Labour market security
A second dimension of job quality is labour market security, which can be measured as the combination of the risk of job loss and the overall individual cost of the subsequent unemployment spell in terms of foregone wage (Nickell, Jones and Quintini, 2002[87]). The individual risk of unemployment could therefore be considered a synthetic measure of labour market insecurity (see Chapter 1).45 For unemployed workers who had a previous work experience, European and US labour force surveys report information on the last occupation before unemployment. It is therefore possible to estimate the percentage of unemployed workers whose last job was in a green-driven occupation and compare it with the percentage of green-driven occupations among employed workers.46 A higher figure for the unemployed would point to a greater risk of protracted unemployment spells, and lower labour market security, in the case of green-driven jobs. In fact, this is what emerges from the data: on average among the countries for which data are available, green-driven occupations represent 23% of the last-job occupations among the unemployed, while they represent only 21.1% among the employed – Figure 2.12, Panel A.
Green-driven jobs are on average more insecure than other jobs in most OECD countries: in only five of them (Denmark, Finland, Sweden, Switzerland and the United Kingdom), the evidence suggests that green-driven occupations are less frequent among the last jobs of the unemployed than among the employed. These results also appear remarkably stable to controlling for individual characteristics – Figure 2.12, Panel B. By contrast, GHG-intensive occupations are only marginally more frequent among the unemployed than among the employed (3% more frequent once observable demographic characteristics are controlled for), and this difference is statistically insignificant. This is consistent with the fact that these jobs are relatively stable jobs until the job is suppressed due to restructuring events (see Chapter 3). For this reason, once the transition accelerates, one could expect these jobs’ labour market security will deteriorate.
The labour market security gap between green-driven and other occupations is, nonetheless, entirely driven by the greater insecurity of green increased demand occupations: the share of these occupations is 13% higher among the unemployed workers’ last jobs than among the employed – Figure 2.12, Panel B.47 By contrast, the share of green new and emerging occupations and green-enhanced skills occupations is either insignificantly different between employed and unemployed workers or lower for the latter, pointing to equal or lower labour market insecurity for these two groups of occupations.
As in the case of wages, however, further analysis suggests that the differences among the three types of green-driven occupations are mainly due to the fact that new and emerging occupations are concentrated in high-skill occupations with greater labour market security, while the opposite is true for green-enhanced skills and, especially, increased demand occupations. Once both observable demographic and job characteristics are controlled for, all green-driven occupations appear slightly more insecure than other occupations. Controlling for sector and 1‑digit ISCO occupations, new and emerging, green-enhanced skills, and green increased demand occupations are estimated to be, respectively, 2.7%, 0.2%, and 10% more frequent among the unemployed than among the employed (Figure 2.13).48
Except in the case of green-enhanced skills occupation, however, labour market insecurity levels are mainly due to low-skill occupations: for these green-driven jobs, labour market security appears indeed lower in low-skill, green increased demand occupations than in other low-skill occupations, while there are no significant differences within high-skill occupations – see Figure 2.13.49 By contrast, the opposite occurs for green-enhanced skills occupations.50
Figure 2.12. Labour market security is lower in green-driven jobs in many OECD countries
Percentage incidence of green-driven occupations by labour market status, 2015‑19
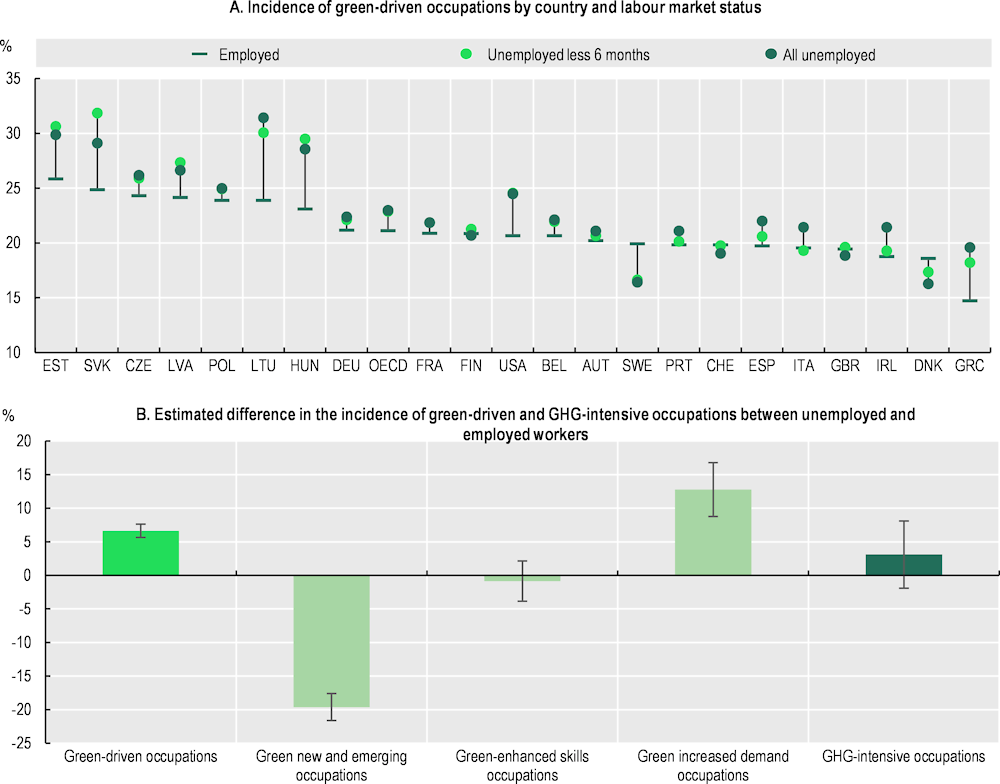
Note: Panel A reports the average percentage of green-driven occupations, by country and labour market status. Panel B reports the point estimate (and 95% confidence intervals) of the percentage difference in the average incidence of each type of occupation between unemployed and employed workers. Unemployed workers are classified according to their last occupation. Unemployed less 6 months refers to unemployed workers with an unemployment spell shorter than 6 months. Unemployed workers with an unemployment spell equal to or longer than 7 years are excluded in all countries except in the United States. Agriculture is not included. OECD: unweighted average of countries shown. In Panel A, countries are ranked by the difference in the incidence of green-driven occupations between unemployed and employed workers. Estimates in Panel B are obtained from a linear regression with the inverse hyperbolic sine of the share of each type of occupation as dependent variable and including an unemployment dummy, educational attainment (3 classes), gender, age (3 classes), year and country dummies as explanatory variables and standard errors clustered on the dimensions of variability of the dependent variable. The reported point estimates and confidence intervals refer to the estimated coefficient of the unemployment dummy and are expressed in percentage of the untransformed dependent variable.
Reading: Panel A: In Estonia, 25.8% of the employed (resp. 29.9% of the unemployed and 30.6% of those unemployed by less than 6 months) have a job (resp. were employed before entering unemployment) in a green-driven occupation. Panel B: Controlling for demographic characteristics, the percentage share of green-driven occupations is, on average, 6.6% lower among the employed than among the unemployed (where data for the unemployed refer to the occupation in the last held job). A lower incidence of a given type of occupations among the employed than among the unemployed is indicative of greater labour market insecurity for that type of occupations.
Source: Secretariat’s estimates based on version 24.1 of the O*NET database and the following country-specific sources: United States: Current Population Survey; All other countries: EU Labour Force Surveys.
Figure 2.13. Many low-skilled, green-driven occupations have worse labour market security than other low-skill occupations
Marginal effect of labour market insecurity indicators on the share of green-driven occupations, by type of occupation and controlling for main individual and job (or last job) characteristics, 2015‑19
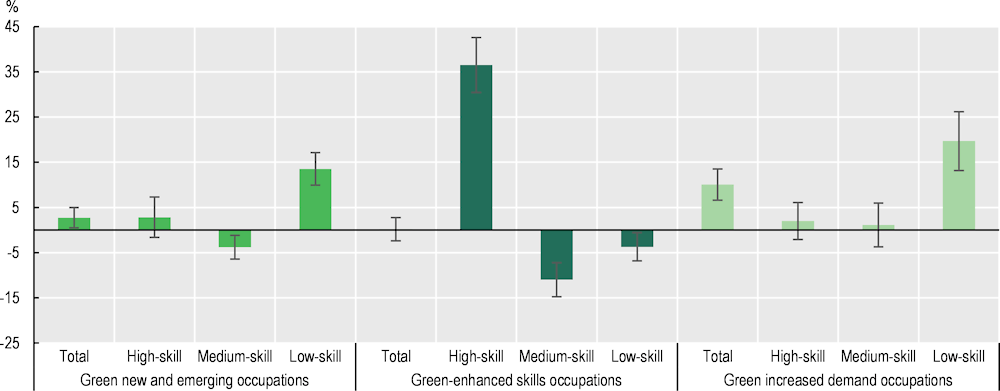
Note: The chart reports the point estimate (and 95% confidence intervals) of the percentage difference in the incidence of each type of green-driven occupation between unemployed and employed workers. Unemployed workers are classified according to their last occupation. Estimates are obtained from a linear regression with the inverse hyperbolic sine of the share of each type of green-driven occupation within all, high, medium or low-skilled occupations as dependent variable and including an unemployment dummy, the share of 1‑digit ISCO occupations, educational attainment (3 classes), gender, age (3 classes), sectors (5 categories), time and country dummies as explanatory variables. Except for the case of all occupations, regressions are estimated separately for high, medium, and low-skill occupations. “Total” indicates the full sample with all occupations. Standard errors are clustered on the dimensions of variability of the dependent variable. The reported point estimates and confidence intervals refer to the estimated coefficient of the unemployment dummy and are expressed in percentage of the untransformed dependent variable. The sample excludes Agriculture and includes Austria, Belgium, Czechia, Denmark, Estonia, Finland, France, Germany, Greece, Hungary, Ireland, Italy, Latvia, Lithuania, Poland, Portugal, the Slovak Republic, Spain, Sweden, Switzerland, the United Kingdom and the United States. Unemployed workers with an unemployment spell equal to or longer than 7 years are excluded in all countries except in the United States.
Reading: Controlling for demographic characteristics and job characteristics and restricting the sample to high-skill occupations, the percentage share of high-skill, green new and emerging occupations is, on average, 2.8% lower among the employed than among the unemployed (where data for the unemployed refer to the occupation in the last held job), although the difference is not statistically significant. A lower incidence of a given type of occupation among the employed than among the unemployed is indicative of lower labour market security for that type of occupation than for the other occupations included in the regression.
Source: Secretariat’s estimates based on version 24.1 of the O*NET database and the Current Population Survey, for the United States and the EU Labour Force Surveys, for all other countries.
One factor that may explain the divergent patterns of labour market security among different types of green-driven occupations is the different incidence of fixed-term contracts. The evidence indeed suggests that, on average, fixed-term contracts are often associated with lower labour market security, and in particular a higher risk of job loss – see e.g. OECD (2014[15]).51 Consistent with this hypothesis, the labour market insecurity pattern concerning low-skill occupations presented in Figure 2.13 is mirrored by similar results as regards the type of contract. Within low-skill occupations, fixed-term contracts are, on average, more frequent among green increased demand and new and emerging occupations (by 24% and 26%, respectively), while the opposite is true for green-enhanced skills occupations (see Figure 2.14). By contrast, the patterns depicted in Figure 2.13 and Figure 2.14 diverge as regards high-skill occupations. Whatever their type, high-skill, green-driven occupations are systematically less frequent among employees with fixed-term contracts but no less frequent among the unemployed than other high-skill occupations, suggesting that contract type is not the main driver of labour market security in these high-skill occupations. In other words, these results suggest that the typical worker in high-skill, green-driven occupations is more likely to hold an open-ended contract than workers in other high-skill occupations, and nevertheless has often no better labour market security.52
Figure 2.14. Among high-skill jobs, green-driven occupations have fewer fixed-term contracts
Marginal effect of the fixed-term contract indicator on the share of green-driven occupations, by type of occupation and controlling for main individual and job (or last job) characteristics, 2015‑19
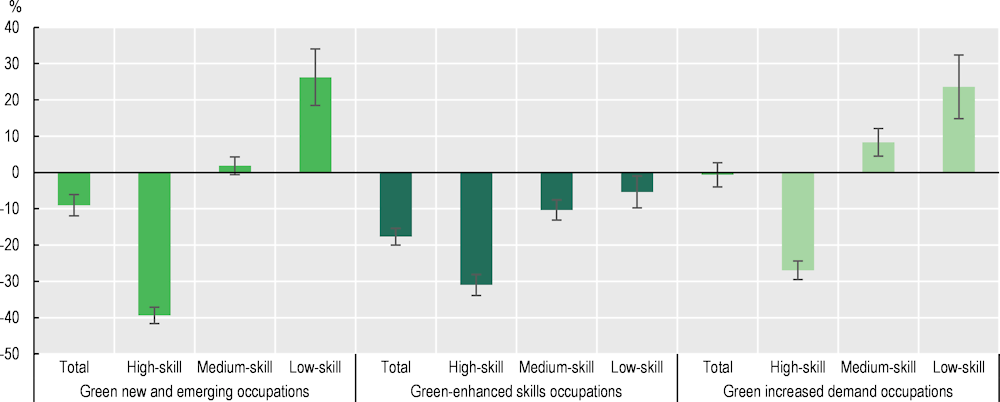
Note: The chart reports the point estimate (and 95% confidence intervals) of the percentage difference in the incidence of each type of green-driven occupation between employees on fixed-term and open-ended contracts. Estimates are obtained from a linear regression with the inverse hyperbolic sine of the share of each type of green-driven occupation within all, high, medium or low-skilled occupations as dependent variable and including a fixed-term contract dummy, the share of 1‑digit ISCO occupations, educational attainment (3 classes), gender, age (3 classes), sectors (5 categories), time and country dummies as explanatory variables. Except for the case of all occupations, regressions are estimated separately for high, medium, and low-skill occupations. “Total” indicates the full sample with all occupations. Standard errors are clustered on the dimensions of variability of the dependent variable. The reported point estimates and confidence intervals refer to the estimated coefficient of the fixed-term contract dummy and are expressed in percentage of the untransformed dependent variable. The sample excludes Agriculture and includes Austria, Belgium, Czechia, Denmark, Estonia, Finland, France, Germany, Greece, Hungary, Iceland, Ireland, Italy, Latvia, Lithuania, Luxembourg, the Netherlands, Norway, Poland, Portugal, the Slovak Republic, Slovenia, Spain, Sweden, Switzerland and the United Kingdom.
Reading: Controlling for demographic characteristics and restricting the sample to high-skill occupations, the percentage share of high-skill, green new and emerging occupations is, on average, 39% higher among employees on open-ended than fixed-term contract. A higher incidence of a given type of occupation among employees on open-ended contract is indicative that the share of fixed-term contracts within that type of occupation is lower than in the other occupations included in the regression.
Source: Secretariat’s estimates based on version 24.1 of the O*NET database and the Current Population Survey, for the United States and the EU Labour Force Surveys, for all other countries.
One possible explanation of these results is that high-skill workers in green-driven occupations tend to have high-level positions in innovative startups or activities characterised by high average growth but also high failure rates and shakeouts, and therefore a relatively high risk of job destruction. Wage premia, however, likely compensate for the lack of labour market security. Moreover, high-skill, green-enhanced skills occupations are likely characterised by high rates of restructuring due to changing competences and work requirements – see Section 2.1.2. Workers unable to adapt to the new requirements may therefore find it difficult to maintain their employability.53 At the same time, some other (not green-driven) high-skill occupations, such as health professionals or software developers, have a relatively high share of fixed-term contracts but still enjoy high labour market security (low unemployment rates) since they face strong labour demand. By contrast, the situation of workers in low-skill occupations is likely different: like their negative wage gap (see Section 2.3.1), their higher unemployment risk and incidence of fixed-term contracts probably reflect the weakness of their position in the labour market.
2.3.3. Quality of the working environment
The third dimension of job quality is the quality of the working environment which captures non-economic aspects of jobs including the nature and content of the work performed, working-time arrangements and workplace relationships. In the OECD Job Quality framework, the quality of the working environment is measured by the incidence of job strain, which results from insufficient resources in the workplace (e.g. work autonomy, social support at work or learning opportunities) to meet job demands (e.g. work intensity or physical health risk factors) – see Chapter 1 for a more in-depth discussion.
Measuring the quality of the working environment requires detailed information on working conditions that are typically available only in ad hoc surveys at international or national levels that are run at irregular intervals. Moreover, linking these surveys to the O*NET taxonomy of green occupational categories requires information on occupations at very detailed level, which is an additional stringent requirement that often does not hold in ad hoc surveys. As a result, only information for 2021 for European countries54 and 2015 for the United States is available in this section.55 Moreover, the sample size of these surveys is relatively small and, therefore, only aggregate results are displayed in the main text.
Keeping these limitations in mind, available data provide useful insights. Figure 2.15 shows that, on average, green-driven jobs are characterised by similar levels of job strain as other jobs (more precisely, the figure shows that the share of green-driven occupations is similar among strained and non-strained workers), i.e. the quality of the working environment is neither better nor worse than elsewhere. As for the other components of the OECD job quality framework (see Sections 2.3.1 and 2.3.2), averages hide significant cross-country heterogeneity (Annex Figure 2.C.12): in countries such as Czechia, Greece or Portugal, job strain is higher in green-driven occupations than in other jobs, while in Belgium, Italy and Sweden, job strain is lower among green-driven occupations than in other jobs.
Figure 2.15 shows that there is also a substantial heterogeneity across the three components of green-driven occupations, as already highlighted by Eurofound (2022[88]): new and emerging and green-enhanced skills occupations are significantly less strained while green increased demand occupations are significantly more strained. Differently from the two other dimensions of job quality, the differences among the three types of green-driven occupations are not due to the fact that new and emerging occupations are concentrated in high-skill occupations: even when controlling for the main job characteristics, the results for the three green-driven occupations remain qualitatively unchanged, suggesting that the differences in job strain are a more structural feature of these groups than the differences in wages and labour market security (Annex Figure 2.C.13).
Figure 2.15. The incidence of job strain is lower in new and emerging and green-enhanced skills green occupations
Marginal effect of the job strain indicator on the share of green-driven occupations, controlling for main individual characteristics, 2021
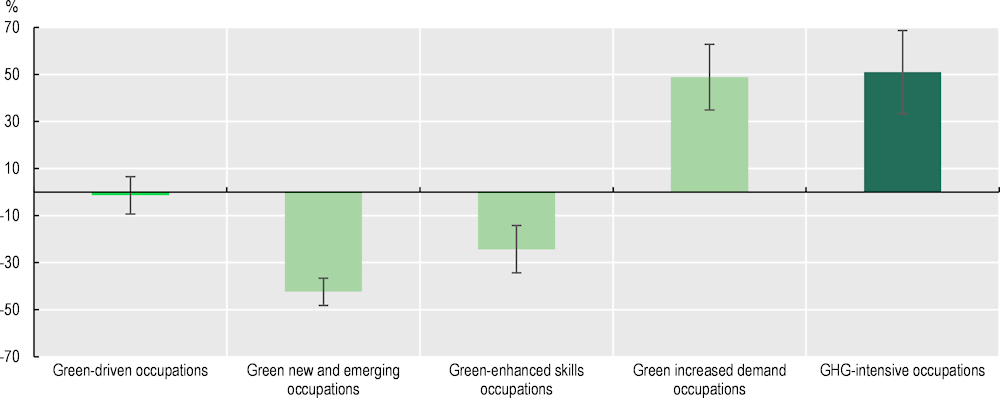
Note: The chart reports the point estimate (and 95% confidence intervals) of the percentage difference in the average incidence of each type of occupation between job strained and not-strained workers. Agriculture is not included. Estimates are obtained from a linear regression with the inverse hyperbolic sine of the share of each type of occupation as dependent variable and including a job strain dummy, educational attainment (3 classes), gender, age (3 classes) and country dummies as explanatory variables and standard errors clustered on the dimensions of variability of the dependent variable. The reported point estimates and confidence intervals refer to the estimated coefficient of the job strain dummy and are expressed in percentage of the untransformed dependent variable. Unweighted average of Austria, Belgium, Czechia, Denmark, Estonia, Finland, France, Germany, Greece, Hungary, Ireland, Italy, Latvia, Lithuania, Luxembourg, the Netherlands, Norway, Poland, Portugal, Slovak Republic, Slovenia, Spain, Sweden, Switzerland, the United Kingdom and the United States.
Reading: Controlling for demographic characteristics, the percentage share of green new and emerging occupations is, on average, 42% lower among strained employees, i.e. employees whose number of job demands exceeds the number of job resources, than among non-strained employees.
Source: Secretariat’s estimates based on version 24.1 of the O*NET database and the European Working Conditions Telephone Survey 2021 and the American Working Conditions Survey 2015.
Occupations concentrated in high-emission sectors, on the opposite, are characterised by higher levels of job strain than other jobs (again, more precisely, Figure 2.15 shows that the share of GHG-intensive occupations is higher among job strained than non-strained workers), i.e. the quality of the working environment is worse than elsewhere. Except for Slovenia, in all countries job strain is higher in GHG-intensive occupations than in other jobs (Annex Figure 2.C.12).
In conclusion, occupations concentrated in high-emission sectors have a lower quality of the working environment than other occupations. This may push some workers to look for better alternatives elsewhere. Therefore, as mentioned above when discussing the age profile of the workers employed in GHG-intensive sectors (see Section 2.2.2), part of the reallocation challenge may be dealt by some natural attrition. In contrast, the picture for green-driven occupations is more mixed: new and emerging occupations and green-enhanced skills occupations clearly display a better quality of the working environment while the group of green increased demand occupations appears less attractive in terms of working environment than other jobs, raising further questions on its ability to attract the workers that will be needed for the transition.
2.4. Concluding remarks
This edition of the Employment Outlook focuses on the effects of the transition to net-zero carbon emission on jobs and incomes. While there is broad agreement that the net effect of the transition on aggregate employment will be modest, the involved industry, occupational and regional reallocation of jobs will be significant. This chapter focuses on the jobs that are likely to benefit from the net-zero transition and examines their distribution and attractiveness. To do so, it introduces the concept of green-driven occupations, which extends beyond the more usual notion of “green jobs” and also includes other jobs that do not directly contribute to reducing emissions, but which will be in demand because of backward linkages.
The chapter shows that around 20% of the workforce is engaged in green-driven occupations across OECD countries, but less than one in six of these occupations are new and emerging ones. The rest are existing jobs in which skill requirements are changing because of the net-zero transition and jobs that will be in demand because they provide goods and services required by green activities. In contrast, about 6% of employment is in greenhouse gas (GHG)-intensive occupations. Both green-driven and GHG-intensive occupations tend to be concentrated in rural areas, but in different regions, indicating a concrete risk of widening geographical disparities during the transition to net-zero emissions.
This chapter has a specific focus on the quality of green-driven occupations, using the OECD Job Quality framework. The results show that green-driven jobs tend to pay higher wages and have fewer temporary contracts but enjoy less labour market security. Jobs that will likely be in demand because they provide support and intermediate goods and services to green activities (green increased demand occupations) also have worse quality of working conditions.
The sector of activity and skill-level of the occupation, however, are important determinants of these results: the difference between green-driven and other jobs is smaller when observable job characteristics are taken into account, except for the quality of the working environment (i.e. the non-economic aspects of jobs including the nature and content of the work performed, working-time arrangements and workplace relationships), which is unaffected. Moreover, the job quality advantage of green-driven occupations, including support jobs, tends to be concentrated in high-skill occupations, suggesting that workers endowed with the specific skills required by these jobs have a competitive edge in the labour market and are well positioned to reap the benefits of the transition. In contrast, low-skill, green-driven occupations tend to command significantly lower wages and labour market security, which suggests that green-driven occupations may appear less attractive than other jobs or unreachable for low-skilled workers without significant skill upgrading. The rising demand for green-driven occupations will most likely contribute to enhance job quality, because of increased competition among companies for workers with suitable competences. However, current job quality is an indicator of current job appeal and a good predictor of future job quality. Labour market policies will therefore be key in ensuring that the transition to net-zero emissions is a just transition, with equally shared benefits and costs. Measures to improve the job quality of green-driven jobs (e.g. conditionality of green subsidies on working conditions) as well as skill policies, active labour market policies, social dialogue and collective bargaining may have an important role to play in this process and will be discussed in detail in the next two chapters.
To monitor the labour market side of the transition to net-zero emissions, however, better quality data will be crucial. As the discussion in Section 2.1 shows, in most countries existing data are not sufficiently granular, which results inevitably in lack of precision and a high degree of uncertainty. To allow timely and effective monitoring and assessment of the effects of the transition on the labour market, most countries would need to re‑consider the level of disaggregation of the labour market statistics they collect and make available for research, such as labour force surveys and register data. Existing international occupational and industry classifications such as ISCO and ISIC prove ill-suited to precisely identify workers in expanding green jobs. Therefore, it would be desirable to have labour force surveys and other labour market data at a more disaggregate level for specific occupations and sectors. For instance, occupations and sectors related to renewable energy use could be separated from those related to fossil fuels.56 As the work of similar workers may be more or less green, depending on how tasks are performed and the overall activity of their plant, even this level of disaggregation may prove insufficient. Ad-hoc employer-employee surveys collecting workers’ use of green technologies and practices – such as the 2012 US Green Technologies and Practices (GTP) survey (BLS, 2013[44]) – or country-specific inventories of green tasks by occupation – such as O*NET in the United States – are therefore likely to be needed as a complement. In addition, it would be important to envisage the possibility of ex-post matching of these surveys with comprehensive and regularly updated linked employer-employee data, which could then be used for monitoring and analysis.
References
[5] Adhvaryu, A., N. Kala and A. Nyshadham (2019), Management and Shocks to Worker Productivity, National Bureau of Economic Research, Cambridge, MA, https://doi.org/10.3386/w25865.
[82] Albanese, A. and G. Gallo (2020), “Buy flexible, pay more: The role of temporary contracts on wage inequality”, Labour Economics, Vol. 64, p. 101814, https://doi.org/10.1016/j.labeco.2020.101814.
[89] Aragón, F., J. Rud and G. Toews (2018), “Resource shocks, employment, and gender: Evidence from the collapse of the UK coal industry”, Labour Economics, Vol. 52, pp. 54-67, https://doi.org/10.1016/j.labeco.2018.03.007.
[1] Arias, P. et al. (eds.) (2023), IPCC, 2023: Climate Change 2023: Synthesis Report. Contribution of Working Groups I, II and III to the Sixth Assessment Report of the Intergovernmental Panel on Climate Change [Core Writing Team, H. Lee and J. Romero (eds.)]. IPCC, Geneva, Switzerland., Intergovernmental Panel on Climate Change (IPCC), https://doi.org/10.59327/ipcc/ar6-9789291691647.
[63] Asikainen, T. et al. (2021), The future of jobs is green, Publications Office of the European Union, https://doi.org/10.2760/218792.
[83] Bassanini, A. et al. (forthcoming), “Labor Market Concentration, Wages and Job Security in Europe”, Journal of Human Resources.
[68] Basso, G. et al. (2020), “The new hazardous jobs and worker reallocation”, OECD Social, Employment and Migration Working Papers, No. 247, OECD Publishing, Paris, https://doi.org/10.1787/400cf397-en.
[94] Bellemare, M. and C. Wichman (2019), “Elasticities and the Inverse Hyperbolic Sine Transformation”, Oxford Bulletin of Economics and Statistics, Vol. 82/1, pp. 50-61, https://doi.org/10.1111/obes.12325.
[16] Benhamou, S. and J. Flamand (2023), Le travail à l’épreuve du changement climatique, France Strategie, Paris.
[56] Biagi, F., F. Vona and A. Bitat (2021), Labour Markets and the Green Transition: a practitioner’s guide to the task-based approach, Publications Office of the European Union, https://doi.org/10.2760/65924.
[3] Bilal, A. and D. Känzig (2024), “The Macroeconomic Impact of Climate Change: Global vs. Local Temperature”, NBER Working Papers, No. 32450, National Bureau of Economic Research, Cambridge, MA, https://doi.org/10.3386/w32450.
[44] BLS (2013), Measuring Green Jobs, https://www.bls.gov/green/#definition.%20 (accessed on 11 December 2023).
[58] Bluedorn, J. et al. (2022), Transitioning to a Greener Labor Market: Cross-Country Evidence from Microdata.
[30] Bontadini, F. and F. Vona (2023), “Anatomy of Green Specialisation: Evidence from EU Production Data, 1995–2015”, Environmental and Resource Economics, Vol. 85/3-4, pp. 707-740, https://doi.org/10.1007/s10640-023-00781-7.
[9] Borgonovi, F. et al. (2023), “The effects of the EU Fit for 55 package on labour markets and the demand for skills”, OECD Social, Employment and Migration Working Papers, No. 297, OECD Publishing, Paris, https://doi.org/10.1787/6c16baac-en.
[77] Botta, E. (2019), “A review of “Transition Management” strategies: Lessons for advancing the green low-carbon transition”, OECD Green Growth Papers, No. 2019/04, OECD Publishing, Paris, https://doi.org/10.1787/4617a02b-en.
[90] Bowen, A. and B. Hancke (2019), The Social Dimensions of ‘Greening the Economy’, Publications Office of the European Union.
[54] Bowen, A., K. Kuralbayeva and E. Tipoe (2018), “Characterising green employment: The impacts of ‘greening’ on workforce composition”, Energy Economics, Vol. 72, pp. 263-275, https://doi.org/10.1016/j.eneco.2018.03.015.
[50] Brynjolfsson, E., T. Mitchell and D. Rock (2018), “What Can Machines Learn and What Does It Mean for Occupations and the Economy?”, AEA Papers and Proceedings, Vol. 108, pp. 43-47, https://doi.org/10.1257/pandp.20181019.
[84] Cahuc, P., F. Postel-Vinay and J. Robin (2006), “Wage Bargaining with On-the-Job Search: Theory and Evidence”, Econometrica, Vol. 74/2, pp. 323-364, https://doi.org/10.1111/j.1468-0262.2006.00665.x.
[85] Caldwell, S. and O. Danieli (forthcoming), “Outside Options in the Labor Market”, Review of Economic Studies, https://www.restud.com/outside-options-in-the-labor-market/ (accessed on 23 January 2024).
[75] Caliendo, M., S. Künn and R. Mahlstedt (2023), “The Intended and Unintended Effects of Promoting Labor Market Mobility”, Review of Economics and Statistics, pp. 1-52, https://doi.org/10.1162/rest_a_01397.
[23] Casanueva, A. et al. (2020), “Escalating environmental summer heat exposure — a future threat for the European workforce”, Regional Environmental Change, Vol. 20/40, https://doi.org/10.1007/s10113-020-01625-6.
[64] Causa, O., M. Nguyen and E. Soldani (2024), “Lost in the green transition? Measurement and stylized facts”, OECD Economics Department Working Papers, No. 1796, OECD Publishing, Paris, https://doi.org/10.1787/dce1d5fe-en.
[69] Causa, O. et al. (2024), “Labour markets transitions in the greening economy: Structural drivers and the role of policies”, OECD Economics Department Working Papers, No. 1803, OECD Publishing, Paris, https://doi.org/10.1787/d8007e8f-en.
[28] Cedefop (2019), Skills for green jobs: 2018 update: European synthesis report, Publications Office of the European Union, Luxembourg.
[4] Chang, T. et al. (2016), “Particulate Pollution and the Productivity of Pear Packers”, American Economic Journal: Economic Policy, Vol. 8/3, pp. 141-169, https://doi.org/10.1257/pol.20150085.
[6] Chang, T. et al. (2016), The Effect of Pollution on Worker Productivity: Evidence from Call-Center Workers in China, National Bureau of Economic Research, Cambridge, MA, https://doi.org/10.3386/w22328.
[8] Chateau, J., R. Bibas and E. Lanzi (2018), “Impacts of Green Growth Policies on Labour Markets and Wage Income Distribution: A General Equilibrium Application to Climate and Energy Policies”, OECD Environment Working Papers, No. 137, OECD Publishing, Paris, https://doi.org/10.1787/ea3696f4-en.
[65] Consoli, D. et al. (2016), “Do green jobs differ from non-green jobs in terms of skills and human capital?”, Research Policy, Vol. 45/5, pp. 1046-1060, https://doi.org/10.1016/j.respol.2016.02.007.
[39] Curtis, E. and I. Marinescu (2023), “Green Energy Jobs in the United States: What Are They, and Where Are They?”, Environmental and Energy Policy and the Economy, Vol. 4, pp. 202-237, https://doi.org/10.1086/722677.
[40] Curtis, E., L. O’Kane and R. Park (2023), Workers and the Green-Energy Transition: Evidence from 300 Million Job Transitions, National Bureau of Economic Research, Cambridge, MA, https://doi.org/10.3386/w31539.
[17] Day, E. et al. (2019), “Upholding labour productivity under climate change: an assessment of adaptation options”, Climate Policy, Vol. 19/3, pp. 367–385, https://doi.org/10.1080/14693062.2018.1517640.
[49] Deming, D. (2017), “The Growing Importance of Social Skills in the Labor Market*”, The Quarterly Journal of Economics, Vol. 132/4, pp. 1593-1640, https://doi.org/10.1093/qje/qjx022.
[45] Dierdorff, E. et al. (2009), Greening of the World of Work: Implications for O*NET®-SOC and New and Emerging Occupations, U.S. Department of Labor Employment and Training Administration Office of Workforce Investment Division of Workforce System Support Washington, DC.
[46] Dierdorff, E. et al. (2011), Greening of the World of Work: Revisiting Occupational Consequences.
[51] Dingel, J. and B. Neiman (2020), “How many jobs can be done at home?”, Journal of Public Economics, Vol. 189, p. 104235, https://doi.org/10.1016/j.jpubeco.2020.104235.
[36] Elliott, R. et al. (2021), “Eco-Innovation and Employment: A Task-Based Analysis”, IZA Discussion Papers, No. 14028.
[53] Elliott, R. and J. Lindley (2017), “Environmental Jobs and Growth in the United States”, Ecological Economics, Vol. 132, pp. 232-244, https://doi.org/10.1016/j.ecolecon.2016.09.030.
[12] Eurofound (2023), Fit for 55 climate package: Impact on EU employment by 2030, Publications Office of the European Union, Luxembourg.
[88] Eurofound (2022), Working conditions in the time of COVID-19: Implications for the future, Publications Office of the European Union, Luxembourg.
[60] European Commission (2023), “The Green Deal Inustrial Plan”, https://commission.europa.eu/document/41514677-9598-4d89-a572-abe21cb037f4_en.
[25] European Commission (2023), Thematic Review 2023: Fair Transition Towards Climate Neutrality, Publications Office of the European Union, Luxembourg.
[10] European Commission (2020), Impact Assessment. Investing in a climate-neutral future for the benefit of our people., European Commission, Brussels.
[33] European Commission (2016), Environmental goods and services sector accounts – Practical guide – 2016 edition, Publications Office of the European Union, https://doi.org/10.2785/688181.
[96] Eurostat (2020), “Classification of Environmental Protection Activities and Expenditure (CEPA) and Classification of Resource Management Activities (CReMA) - Explanatory Notes”, European environmental economic accounts Technical Note, No. EEEA/2020/03, https://ec.europa.eu/eurostat/documents/1798247/12177560/CEPA+and+CReMA+explanatory+notes+-+technical+note.pdf/b3517fb9-1cb3-7cd9-85bd-4e3a3807e28a?t=1609863934103 (accessed on 4 December 2023).
[20] Fatima, S. et al. (2021), “Extreme heat and occupational injuries in different climate zones: A systematic review and meta-analysis of epidemiological evidence”, Environment International, Vol. 148, p. 106384, https://doi.org/10.1016/j.envint.2021.106384.
[14] Finkelstein Shapiro, A. and G. Metcalf (2023), “The macroeconomic effects of a carbon tax to meet the U.S. Paris agreement target: The role of firm creation and technology adoption”, Journal of Public Economics, Vol. 218, p. 104800, https://doi.org/10.1016/j.jpubeco.2022.104800.
[13] Fontaine, F. et al. (2023), Transition énergétique: faut-il craindre pour l’emploi ?, Conseil d’Analyse Économique, Paris.
[67] Fragkiadakis, D. (2022), Provision of detailed employment forecasts by occupation and sector for the EU based on policies consistent with achieving the EU’s carbon neutrality goal, Final Report for Eurofound, E3 Modelling.
[92] France Stratégie (2023), Les incidences économiques de l’action pour le climat: Marché du Travail, https://www.strategie.gouv.fr/sites/strategie.gouv.fr/files/atoms/files/fs-2023-les_incidences_economiques_de_laction_pour_le_climat-thematique-marche_du_travail.pdf (accessed on 11 December 2023).
[91] France Stratégie and Dares (2022), Les Métiers en 2030, France Stratégie and Dares, https://www.strategie.gouv.fr/sites/strategie.gouv.fr/files/atoms/files/fs-2022-pmq-rapport-mars_4.pdf (accessed on 14 December 2023).
[24] García-León, D. et al. (2021), “Current and projected regional economic impacts of heatwaves in Europe”, Nature Communications, Vol. 12/1, https://doi.org/10.1038/s41467-021-26050-z.
[57] Georgeson, L. and M. Maslin (2019), “Estimating the scale of the US green economy within the global context”, Palgrave Communications, Vol. 5/1, https://doi.org/10.1057/s41599-019-0329-3.
[95] Halvorsen, R. and R. Palmquist (1980), “The Interpretation of Dummy Variables in Semilogarithmic Equations”, The American Economic Review, Vol. 70/3, pp. 474-475, http://www.jstor.org/stable/1805237.
[47] Handel, M. (2016), “The O*NET content model: strengths and limitations”, Journal for Labour Market Research, Vol. 49/2, pp. 157-176, https://doi.org/10.1007/s12651-016-0199-8.
[48] Handel, M. (2012), “Trends in Job Skill Demands in OECD Countries”, OECD Social, Employment and Migration Working Papers, No. 143, OECD Publishing, Paris, https://doi.org/10.1787/5k8zk8pcq6td-en.
[26] Heutel, G., N. Miller and D. Molitor (2021), “Adaptation and the Mortality Effects of Temperature Across U.S. Climate Regions”, The Review of Economics and Statistics, Vol. 103/4, pp. 740–753, https://doi.org/10.1162/rest_a_00936.
[18] ILO (2019), Working on a warmer planet. The impact of heat stress on labour productivity and decent work., International Labour Office, Geneva.
[61] ILO (2016), What is a green job?, https://www.ilo.org/global/topics/green-jobs/news/WCMS_220248/lang--en/index.htm.
[93] ILO (2013), Guidelines concerning a statistical definition of employment in the environmental sector, 19th International Conference of Labour Statisticians, Geneva.
[7] Keese, M. and L. Marcolin (2023), “Labour and social policies for the green transition: A conceptual framework”, OECD Social, Employment and Migration Working Papers, No. 295, OECD Publishing, Paris, https://doi.org/10.1787/028ffbeb-en.
[71] Lim, J., M. Aklin and M. Frank (2023), “Location is a major barrier for transferring US fossil fuel employment to green jobs”, Nature Communications, Vol. 14/1, https://doi.org/10.1038/s41467-023-41133-9.
[80] Mas, A. and A. Pallais (2017), “Valuing Alternative Work Arrangements”, American Economic Review, Vol. 107/12, pp. 3722-3759, https://doi.org/10.1257/aer.20161500.
[73] Neumark, D. and H. Simpson (2015), “Place-Based Policies”, in Handbook of Regional and Urban Economics, Elsevier, https://doi.org/10.1016/b978-0-444-59531-7.00018-1.
[87] Nickell, S., P. Jones and G. Quintini (2002), “A Picture of Job Insecurity Facing British Men”, The Economic Journal, Vol. 112/476, pp. 1-27, https://doi.org/10.1111/1468-0297.0j671.
[27] OECD (2023), Job Creation and Local Economic Development 2023: Bridging the Great Green Divide, OECD Publishing, Paris, https://doi.org/10.1787/21db61c1-en.
[79] OECD (2023), OECD Employment Outlook 2023: Artificial Intelligence and the Labour Market, OECD Publishing, Paris, https://doi.org/10.1787/08785bba-en.
[70] OECD (2023), Regional Industrial Transitions to Climate Neutrality, OECD Regional Development Studies, OECD Publishing, Paris, https://doi.org/10.1787/35247cc7-en.
[78] OECD (2022), OECD Employment Outlook 2022: Building Back More Inclusive Labour Markets, OECD Publishing, Paris, https://doi.org/10.1787/1bb305a6-en.
[2] OECD (2021), “The inequalities-environment nexus: Towards a people-centred green transition”, OECD Green Growth Papers, No. 2021/01, OECD Publishing, Paris, https://doi.org/10.1787/ca9d8479-en.
[74] OECD (2018), Rethinking Regional Development Policy-making, OECD Multi-level Governance Studies, OECD Publishing, Paris, https://doi.org/10.1787/9789264293014-en.
[15] OECD (2014), OECD Employment Outlook 2014, OECD Publishing, Paris, https://doi.org/10.1787/empl_outlook-2014-en.
[22] OECD (forthcoming), Feeling the Heat: Working under increasing temperatures, OECD Publishing, Paris.
[37] Office of National Statistics (2022), Research into “green jobs”: time spent doing green tasks, UK: 1997 to 2019, https://www.ons.gov.uk/economy/environmentalaccounts/articles/researchintogreenjobstimespentdoinggreentasksuk/1997to2019 (accessed on 13 December 2023).
[21] Park, R., N. Pankratz and A. Behrer (2021), “Temperature, Workplace Safety, and Labor Market Inequality”, IZA Discussion Papers, No. 14560, IZA, Bonn, https://docs.iza.org/dp14560.pdf.
[35] Peters, D. (2013), “Understanding Green Occupations from a Task‐Based Approach”, Applied Economic Perspectives and Policy, Vol. 36/2, pp. 238-264, https://doi.org/10.1093/aepp/ppt026.
[55] Popp, D. et al. (2021), “The Employment Impact of a Green Fiscal Push: Evidence from the American Recovery and Reinvestment Act”, Brookings Papers on Economic Activity, Vol. Fall, pp. 1-49.
[97] Rodrigues, J. et al. (2018), “Uncertainty of Consumption-Based Carbon Accounts”, Environmental Science and Technology, Vol. 52/13, pp. 7577-7586, https://doi.org/10.1021/acs.est.8b00632.
[72] Rodríguez-Pose, A., L. Dijkstra and H. Poelman (2023), The geography of EU discontent and the regional development trap, Publications Office of the European Union, https://ec.europa.eu/regional_policy/sources/reports/Geography_of_Discontent_and_development_trap_forlay-out.pdf.
[38] Saussay, A. et al. (2022), Who’s fit for the low-carbon transition? Emerging skills and wage gaps in job ad data, https://www.lse.ac.uk/granthaminstitute/publication/whos-fit-for-the-low-carbon-transition-emerging-skills-and-wage-gaps-in-job-ad-data/ (accessed on 15 September 2023).
[43] Scholl, N., S. Turban and P. Gal (2023), “The green side of productivity: An international classification of green and brown occupations”, OECD Productivity Working Papers, No. 33, OECD Publishing, Paris, https://doi.org/10.1787/a363530f-en.
[19] Somanathan, E. et al. (2021), “The Impact of Temperature on Productivity and Labor Supply: Evidence from Indian Manufacturing”, Journal of Political Economy, Vol. 129/6, pp. 1797-1827, https://doi.org/10.1086/713733.
[81] Taber, C. and R. Vejlin (2020), “Estimation of a Roy/Search/Compensating Differential Model of the Labor Market”, Econometrica, Vol. 88/3, pp. 1031-1069, https://doi.org/10.3982/ecta14441.
[66] Tyros, S., D. Andrews and A. de Serres (2023), “Doing green things: skills, reallocation, and the green transition”, OECD Economics Department Working Papers, No. 1763, OECD Publishing, Paris, https://doi.org/10.1787/286a5007-en.
[32] United Nations (2012), System of Environmental-Economic Accounting 2012 Central Framework, United Nations, New York City.
[59] US White House (2023), “Building a clean energy economy: A guidebook to the inflation reduction act’s investments in clean energy and climate action”, https://www.whitehouse.gov/wp-content/uploads/2022/12/Inflation-Reduction-Act-Guidebook.pdf.
[29] Valero, A. et al. (2021), Are ’green’ jobs good jobs?, https://cep.lse.ac.uk/_new/publications/abstract.asp?index=8581.
[62] van der Ree, K. (2019), “Promoting Green Jobs: Decent Work in the Transition to Low-Carbon, Green Economies, Fomento de los empleos verdes: trabajo decente en la transición hacia economías verdes y bajas en carbono”, International Development Policy 11, pp. 248-271, https://doi.org/10.4000/poldev.3107.
[34] Vandeplas, A. et al. (2022), The possible implications of the green transition for the EU labour market, European Commission, https://commission.europa.eu/publications/economic-and-financial-affairs-publications_en.
[31] Verdolini, E. and F. Vona (2022), “Lavoro e transizione energetica”, in CNEL (ed.), XXIV Rapporto Mercato del Lavoro e Contrattazione Collectiva 2020, CNEL, https://air.unimi.it/bitstream/2434/952399/2/3_CNEL_Rapporto-mercato-del-lavoro-2022.pdf (accessed on 18 January 2024).
[42] Vona, F., G. Marin and D. Consoli (2018), “Measures, drivers and effects of green employment: evidence from US local labor markets, 2006–2014”, Journal of Economic Geography, Vol. 19/5, pp. 1021-1048, https://doi.org/10.1093/jeg/lby038.
[41] Vona, F. et al. (2018), “Environmental Regulation and Green Skills: An Empirical Exploration”, Journal of the Association of Environmental and Resource Economists, Vol. 5/4, pp. 713-753, https://doi.org/10.1086/698859.
[52] Webb, M. (2020), The Impact of Artificial Intelligence on the Labor Market, https://www.michaelwebb.co/webb_ai.pdf.
[11] Weitzel, M. et al. (2023), “A comprehensive socio-economic assessment of EU climate policy pathways”, Ecological Economics, Vol. 204, p. 107660, https://doi.org/10.1016/j.ecolecon.2022.107660.
[76] World Bank (2008), World Development Report 2009, The World Bank, https://doi.org/10.1596/978-0-8213-7607-2.
[86] Zwysen, W. (2024), Green transition and job quality: risks for worker representation, European Trade Union Institute Technical Brief, Brussels.
Annex 2.A. Crosswalking occupational classifications using Dingel and Neiman’s method
Existing crosswalks between the different occupational classifications used in different OECD countries are typically many-to-many, meaning that each occupation in one classification corresponds to many occupations in another classification and vice versa. Therefore, correspondence between two occupations is often only partial. This chapter adapts and applies the methodology originally developed by Dingel and Neiman (2020[51]) for indicators of occupational characteristics that are shared by all jobs in the same occupation as defined in the standard occupational classification of one country (for example the proportion of workers in green-driven jobs, which takes the same value – 0 or 100% – within each 8‑digit SOC occupation). The method allows to estimate reliably the average of such indicators for occupations defined in a different classification system used in another country. Dingel and Neiman (2020[51]) originally developed the method to translate any occupation-specific indicator available in the US SOC system into the ISCO system by constructing a weighted crosswalk, but the method can be applied more generally.
An example using occupations in the US SOC system as origin occupations, the United States as origin country and the ISCO system as target occupations would simplify the exposition of the method. For each target country using the ISCO system (e.g. one European country), the method is performed in four steps.
1. ISCO-SOC cells are determined based on a standard many-to-many crosswalk. These cells will be called connection cells hereafter.
2. For each target country, the US employment share of each ISCO-SOC connection cell ic is determined by using the following formula:
where i (resp. c) indexes ISCO (resp. US SOC) categories, indicates the share of the US SOC occupation c in US employment, indicates the employment share of ISCO occupation i in the target country and indicates all ISCO occupations (indexed by k) that match with the SOC occupation c. In other words, the US employment share is “distributed” across occupations proportionally to their employment shares in the target country.
3. The same value of the indicator defined at SOC level (e.g. indicator of being green-driven or not) is assigned to all ISCO-SOC connection cells that correspond to the same SOC category.
4. For each indicator of interest (e.g. the proportion of green-driven jobs), its value for each ISCO occupation is obtained as a weighted average of the indicator in each ISCO-SOC connection cell, where are used as weight, that is:
where X stands for the chosen indicator and and indicates all SOC occupations (indexed by j or c) that match with ISCO occupation i. In other words, can be seen as a (country-specific) crosswalk weight.
This method delivers a country-specific weighted crosswalk. The country-specific national average of the indicator X will be estimated by aggregating over ISCO occupations:
where X stands for the chosen indicator.
It is important to note that, because the employment-weighted average of group averages is the global average, in the special case in which in the equation above and the indicator is constant within all jobs of each SOC occupation category (e.g. the proportion of employees in green-driven jobs), the economy-wide average of the indicator is the same no matter if computed on original or converted data.57 In other words, when US data are converted from SOC into ISCO, since , it follows that:
This implies that the share of employment in one type of jobs (e.g. green-driven jobs) computed in the SOC system for the United States is comparable to the share of employment of that type of job in other countries, when computed with the method described here.58
This method does not necessarily yield a final binary indicator in each target occupation of the target country even when the origin indicator is binary. For example, the proportion of green-driven occupations is either 0% or 100% in the origin occupations and country by construction. Yet, in the target country, most target occupations will have an estimated proportion of green-driven jobs comprised between these two values.
The data at the level of the target occupations can then be matched with other datasets for the target country (e.g. labour force surveys) to estimate the average of the chosen indicator among groups with different characteristics – for example, the estimated frequency of green-driven occupations among women. This will be obtained as a weighted average of the indicators for the target occupations where group-specific employment by occupation will be used as weight – that is, in the same example as above, a weighted average of the proportion of green-driven jobs by occupation, with female employment by occupation being used as weight.
The original method was applied by Dingel and Neiman to 6‑digit SOC categories and 2‑digit ISCO categories.59 However, nothing prevents to better exploit the available information in labour force surveys and construct weighted crosswalks that are specific for countries and other dimensions that are likely to be key determinants of the distribution of occupations (such as gender and industry). In this chapter, to compute the share of green-driven and GHG-intensive occupations, the method is alternatively applied to 8‑digit SOC and 4 or 3‑digit ISCO occupations, usually coupled with other dimensions, such as gender and 1‑digit ISIC industries. To do so, a new many-to-many crosswalk developed by the European Commission (The ESCO Secretariat) and the US Department of Labor (The O*NET Network, under the auspices of the Employment and Training Administration) is employed.60
Nothing prevents the application of this method to other crosswalks. In this chapter it is used to match the US SOC system with occupational classifications used in other countries (e.g. CAN SOC and ANZSCO). It is also used to match 2‑digit ISIC industries and 6‑digit NAICS industries.
Annex 2.B. Downward aggregation bias for gaps in job quality variables
Wage gap (or gaps of individual variables): Large attenuation bias
A natural way to compute gaps in individual variables between green-driven and other occupations where individual data are aggregated by cells (including both green-driven and other occupations in each cell) would be to compute them as percentage differences of computed averages of each occupation, where the latter are weighted average of cell-level averages, and the weights are the estimated number of individuals of each group. However, if individual data vary within cells, this leads to an attenuation bias.
Let us consider the case of individual wages as a practical example and assume that the true wage gap is positive and, for simplicity, constant across occupations. The wage gap can then be written as:
where w stands for average wages (overall average wages when no superscript is indicated), c for the SOC occupation, g stands for green-driven jobs (with G being total employment in green-driven jobs), n for not-green-driven jobs (with N being total employment in these other jobs), s is the share of green-driven job (in the cell c where the subscript c is indicated) and is employment in the SOC cell. Note that in the SOC system is either 0 or 1, so that when and when , which implies and
Let us use ISCO as target occupational classification to make things concrete – see Annex 2.A. Applying the same formulas in ISCO generates a downward bias in every time the estimated share of green-driven jobs in one ISCO occupation is different from 0 or 1. This is because (with i denoting the ISCO occupation):
The term on the right-hand side of the rightmost equality represents the natural way to guess-estimate the average wage of green-driven occupations from the ISCO-aggregated data (since is unobservable). The last equality comes from the fact that:
In the same way it can be shown that:
This implies that the estimated numerator of the wage gap from ISCO-aggregated data is biased towards zero. Note that the bias is larger, the larger the amount of employment in cells such that is close to its maximum, that is close to This occurs no matter the relationship between wages and the precision of ISCO-aggregated shares in each cell. In other words, for this method to yield an accurate estimate of the wage gap, most ISCO-aggregated shares should be close to 0 or 1.
In principle, the same issue emerges with discrete variables, if data needs to be aggregated into shares by cells but original data vary across individuals within the same cell. In that case the bias is larger, the larger the share in employment of cells where the proportion of green-driven jobs is close to one half.
Proportion of green-driven jobs by category: No systematic bias
When one computes the proportion of green-driven jobs within a wage interval or a specific category (e.g. educational attainment), the aggregation problem discussed above is less important. Consider the proportion P of green-driven jobs by a given category S:
where j indexes the individuals in category S, is the true share of green-driven jobs corresponding to the SOC occupation of the individual (is therefore equal to 0 or 1), while represents the employment weight of the individual j. Suppose now that P is approximated by:
where denotes the sum of individual weights for each ISCO category i and category S, while indicates the share of green-driven jobs in category i. For each individual j in category S, the measurement error would be either or As a consequence, for each ISCO category the average error would be , where stands for the true proportion of green-driven jobs within the category S in ISCO cell i. For this to generate large, systematic bias, should be much larger or smaller than on average over all ISCO categories. In other words, the average precision of ISCO-aggregated shares in each cell should be very weak. This is a much stronger (and less likely) condition than the one holding for gaps. An analysis performed by the Secretariat using CPS data by high/medium/low-wage categories and ISCO occupations instead of SOC occupations, indeed yields measurement errors of 15% or lower,61 while the error on the wage gap, directly computed as a weighted average of cell-level average wages, is of the order of 70%.
Annex 2.C. Additional figures
Annex Figure 2.C.1. One in eight green-driven occupations is concentrated in GHG-intensive industries
Percentage of total employment, average 2015‑19

Note: Data refer to the average for 2015‑19, except for Canada: 2017‑19 and New Zealand: 2018. OECD: Unweighted average of countries shown. GHG: greenhouse gases.
Source: Secretariat’s estimates based on version 24.1 of the O*NET database and the following country-specific sources: Australian Labour Force Survey; Canadian Labour Force Survey; Japanese Labour Force Survey; United States: Current Population Survey; All other countries: EU Labour Force Survey.
Annex Figure 2.C.2. Men are more likely to be employed in green-driven and GHG-intensive occupations
Percentage of total employment, average 2015‑19
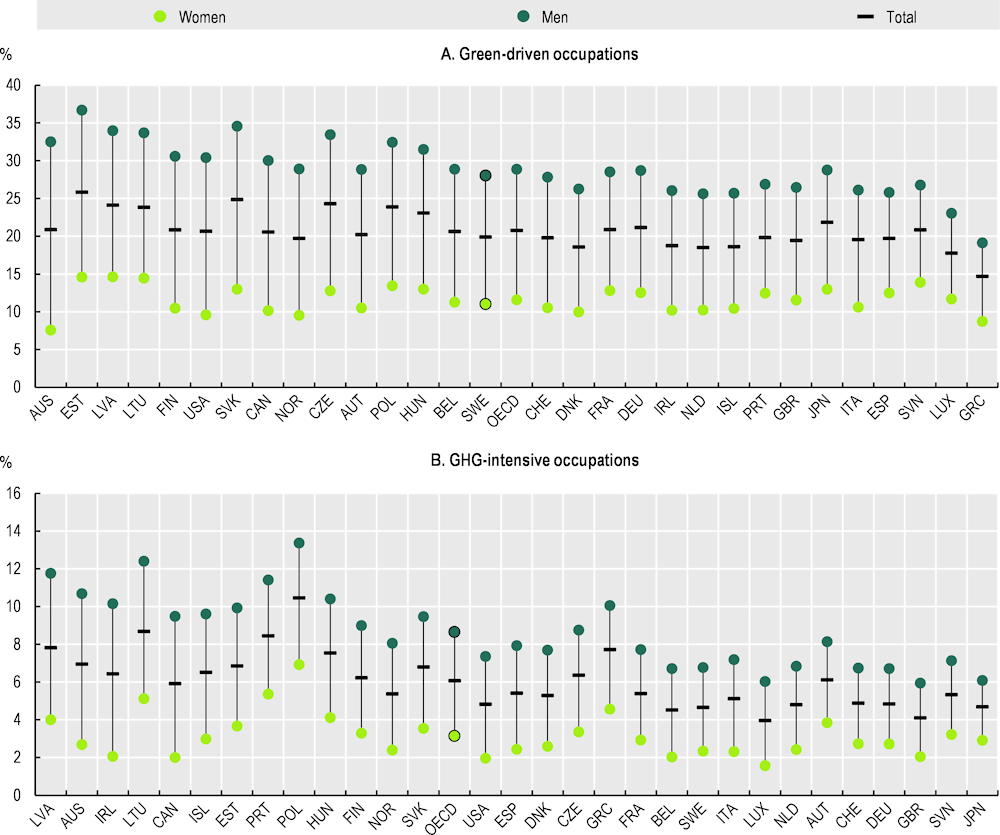
Note: Data refer to the average for 2015‑19, except for Canada: 2017‑19 and New Zealand: 2018. Countries are ranked by decreasing gap of the share for men compared to the total. OECD: Unweighted average of countries shown. GHG: greenhouse gases.
Source: Secretariat’s estimates based on version 24.1 of the O*NET database and the following country-specific sources: Australian Labour Force Survey; Canadian Labour Force Survey; Japanese Labour Force Survey; United States: Current Population Survey; All other countries: EU Labour Force Survey.
Annex Figure 2.C.3. Older workers are more likely to be found in GHG-intensive occupations
Percentage of total employment, average 2015‑19
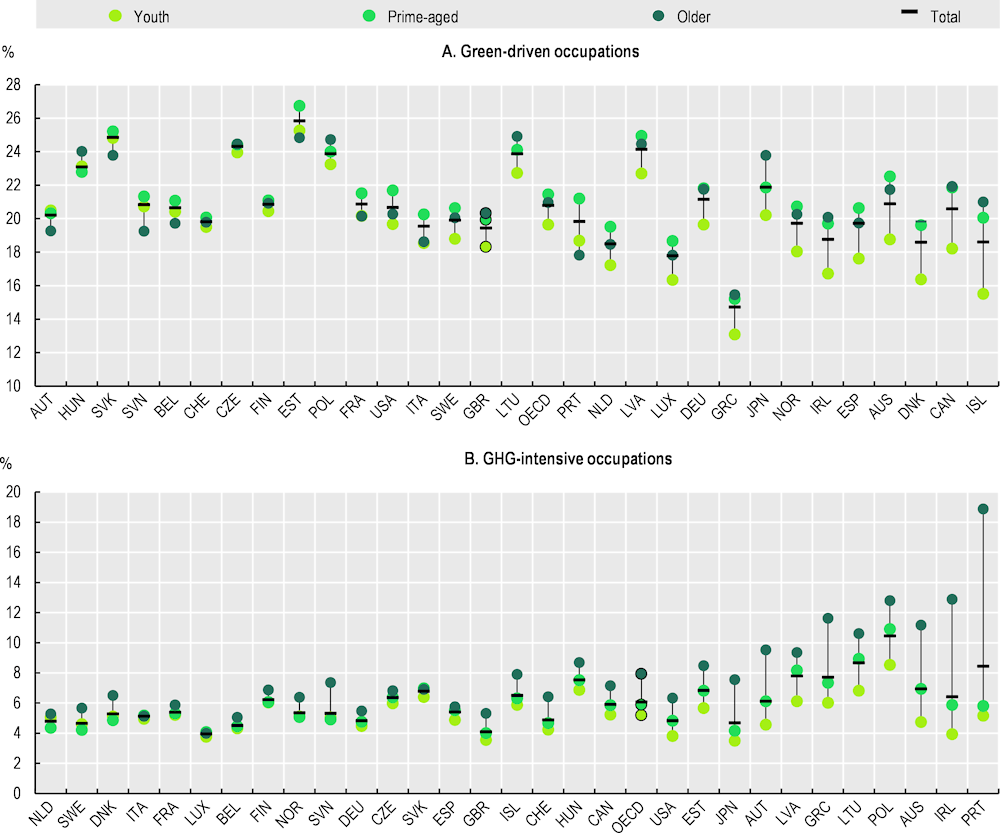
Note: Youth refer to those aged 15 to 34, prime‑aged to those aged 35 to 54, older to those aged 55 and more. Countries are ranked by decreasing gap of the share for youth compared to the total. Data refer to the average for 2015‑19, except for Canada: 2017‑19 and New Zealand: 2018. OECD: Unweighted average of countries shown. GHG: greenhouse gases.
Source: Secretariat’s estimates based on version 24.1 of the O*NET database and the following country-specific sources: Australian Labour Force Survey; Canadian Labour Force survey; Japanese Labour Force Survey; United States: Current Population Survey; All other countries: EU Labour Force Survey.
Annex Figure 2.C.4. There is limited variation in the age composition of different types of green-driven jobs
Percentage of total employment, average 2015‑19
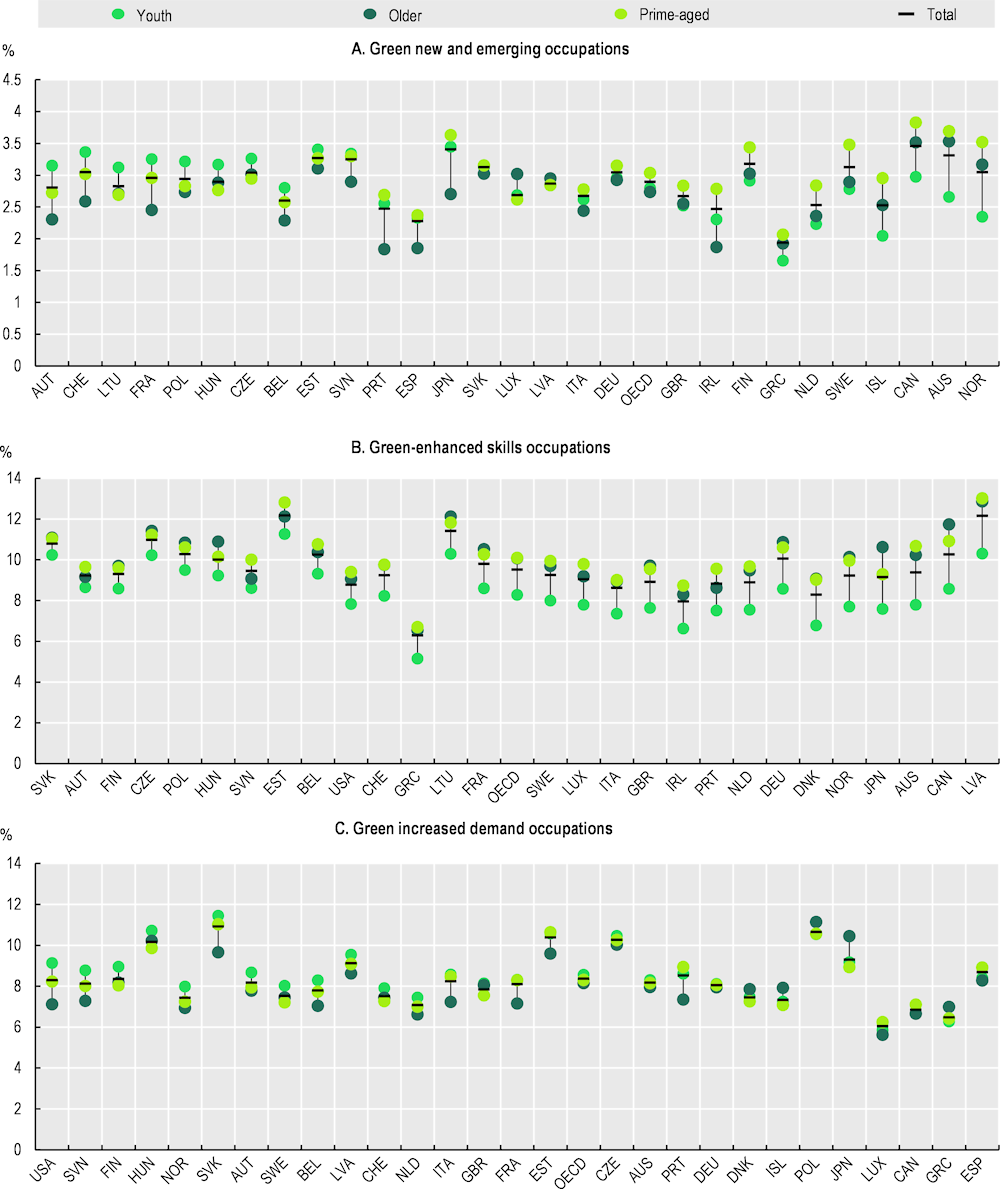
Note: Countries are ranked by decreasing gap of the share for youth compared to the. Data refer to the average for 2015‑19. OECD: Unweighted average of countries shown.
Source: Secretariat’s estimates based on version 24.1 of the O*NET database and the following country-specific sources: Australian Labour Force Survey; Canadian Labour Force Survey; Japanese Labour Force Survey; United States: Current Population Survey; All other countries: EU Labour Force Survey.
Annex Figure 2.C.5. Green-driven and GHG-intensive occupations are typically medium-skill ones
Percentage of total employment, average 2015‑19
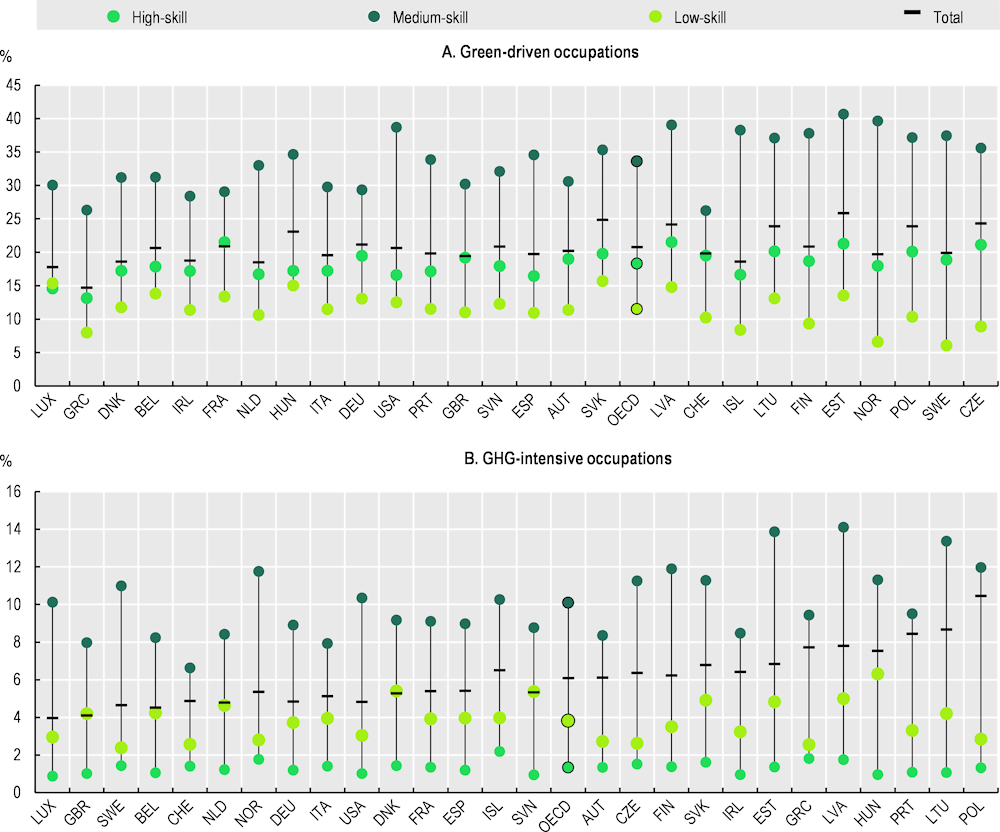
Note: Countries are ranked by decreasing gap of the share for low-skilled compared to the total in Panel A, and by decreasing gap of the share for high-skilled compared to the total in Panel B. Low-skill refer to Service and Sales Workers and Elementary Occupations (ISCO‑08 5 and 9, medium skill to Clerical Support Workers, Craft and Related Trades Workers and Plant and Machine Operators, and Assemblers (ISCO‑08 4, 7 and 8), high-skill to Managers, Professionals and Technicians and Associate Professionals (ISCO‑08 1, 2 and 3). Data refer to the average for 2015‑19. OECD: Unweighted average of countries shown. GHG: greenhouse gases.
Source: Secretariat’s estimates based on version 24.1 of the O*NET database and the following country-specific sources: United States: Current Population Survey; All other countries: EU Labour Force Survey.
Annex Figure 2.C.6. The occupational composition of different types of green-driven jobs differs significantly
Percentages, average 2015‑19
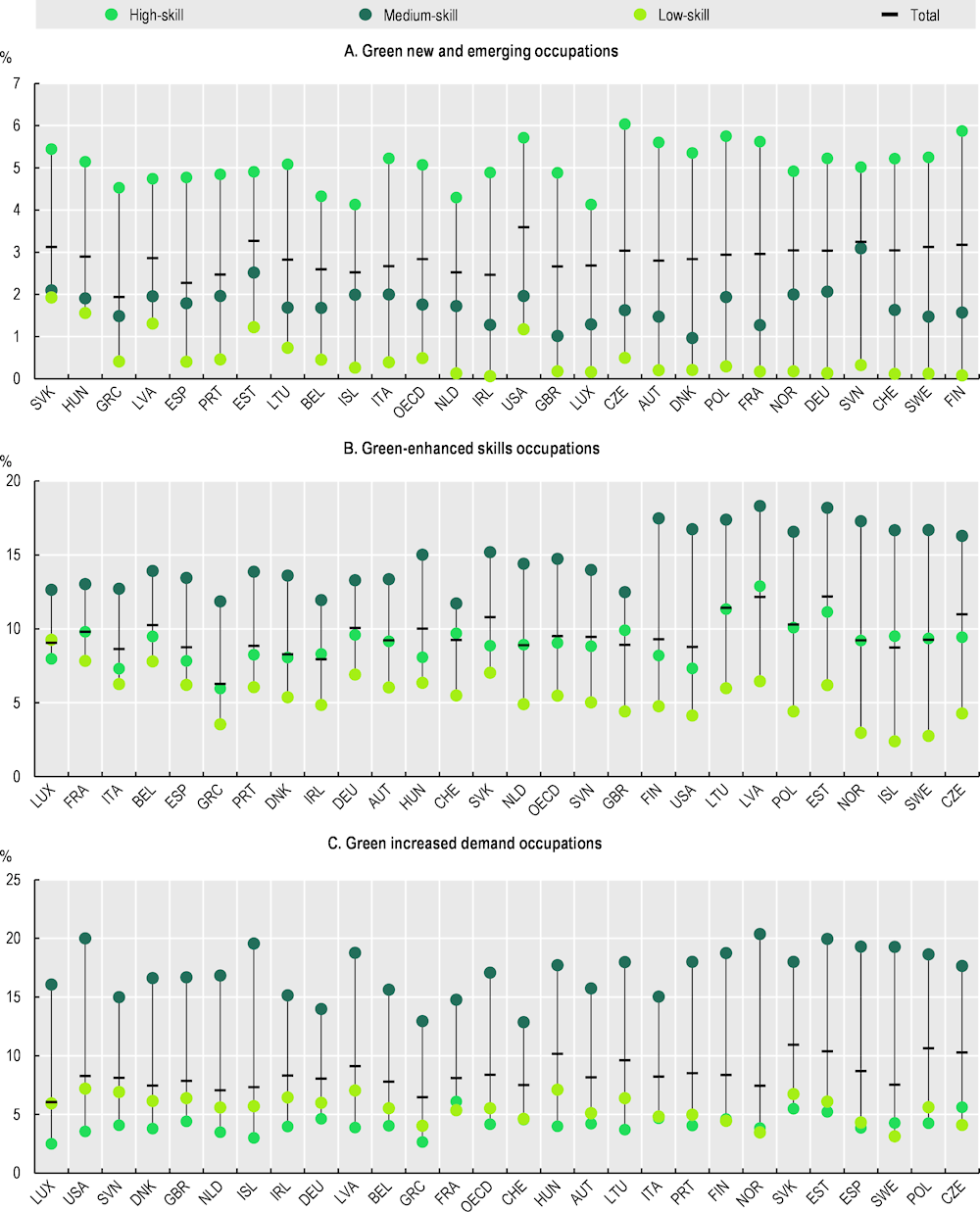
Note: Data refer to the average for 2015‑19. Low-skill refer to Service and Sales Workers and Elementary Occupations (ISCO‑08 5 and 9, medium skill to Clerical Support Workers, Craft and Related Trades Workers and Plant and Machine Operators, and Assemblers (ISCO‑08 4, 7 and 8), high-skill to Managers, Professionals and Technicians and Associate Professionals (ISCO‑08 1, 2 and 3). OECD: Unweighted average of countries shown.
Source: Source Secretariat’s estimates based on version 24.1 of the O*NET database and the following country-specific sources: United States: Current Population Survey; All other countries: EU Labour Force Survey.
Annex Figure 2.C.7. GHG-intensive occupations are more likely low educated while green-driven occupations are more heterogeneous
Percentages, average 2015‑19
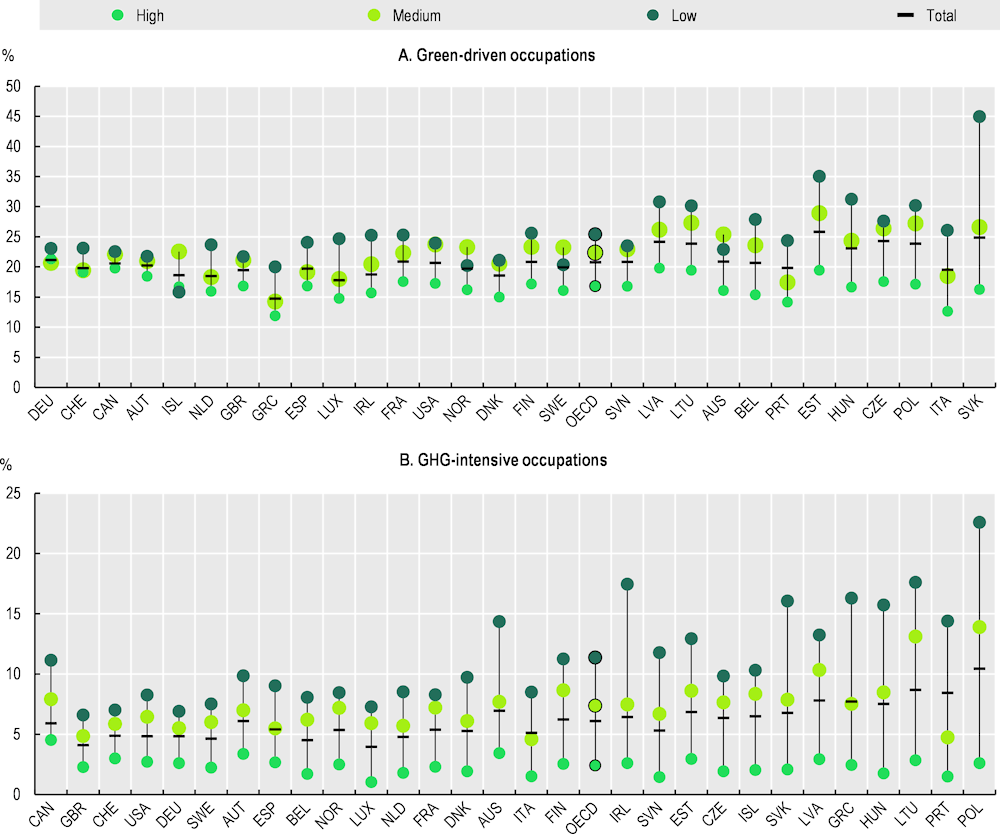
Note: Countries are ranked by decreasing gap of the share for high education compared to the total. Data refer to the average for 2015‑19. Low education refers to less than upper secondary, medium education to upper secondary, high education to more than upper secondary. OECD: Unweighted average of countries shown. GHG: greenhouse gases.
Source: Secretariat’s estimates based on version 24.1 of the O*NET database and the following country-specific sources: United States: Current Population Survey; All other countries: EU Labour Force Survey.
Annex Figure 2.C.8. The educational composition of different types of green-driven jobs differs significantly
Percentages, average 2015‑19
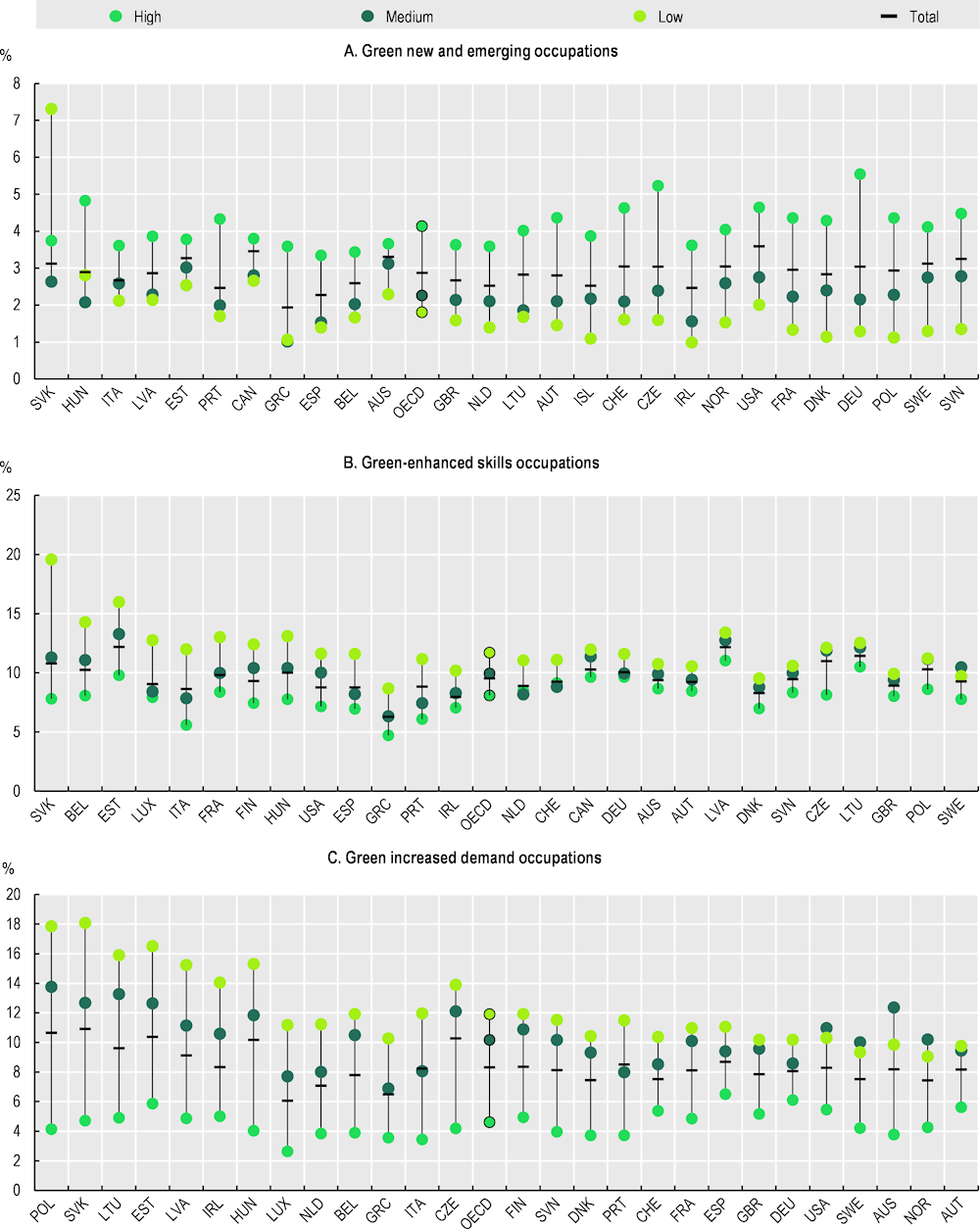
Note: Countries are ranked by decreasing gap of the share for high education compared to the total. Data refer to the average for 2015‑19. Low education refers to less than upper secondary, medium education to upper secondary, high education to more than upper secondary. OECD: Unweighted average of countries shown.
Source: Secretariat’s estimates based on version 24.1 of the O*NET database and the following country-specific sources: United States: Current Population Survey; All other countries: EU Labour Force Survey.
Annex Figure 2.C.9. Employees in GHG-intensive occupations typically earn low or middle wages
Percentage shares of GHG-intensive occupations, by wage category and country, 2018
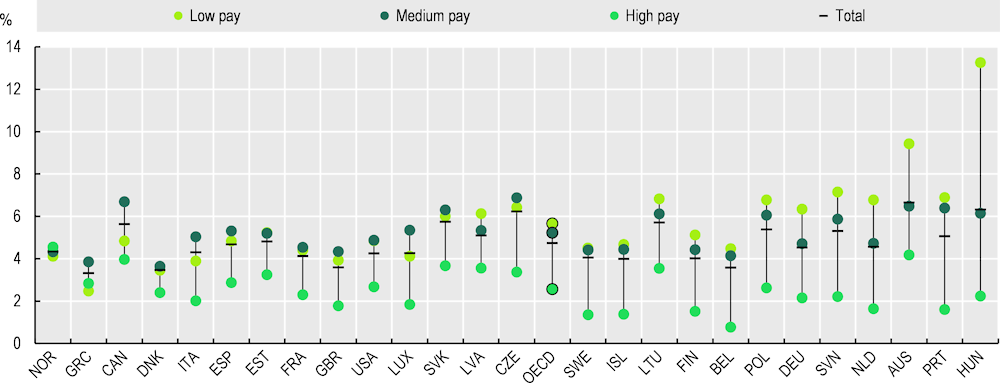
Note: The figure reports the percentage of GHG-intensive occupations in wage and salary employment, by country and hourly wage category. High (resp. low) wage is defined as hourly wage above one‑and-a-half times (resp. below two‑thirds of) the median wage. Agriculture is excluded except in Australia, Canada and the United States. Data for Belgium, Denmark, France, Greece, Iceland, Italy, Luxembourg, Portugal, Sweden and the United Kingdom do not include firms with less than 10 employees. Data for Canada and the United States refer to 2019. OECD: Unweighted average of countries shown. GHG: greenhouse gases. Countries are ranked by the difference in the incidence of GHG-intensive occupations between high and low wage workers.
Reading: In Hungary, 13.3% (resp. 6.2% and 2.2%) of low-wage (resp. middle‑wage and high-wage) employees have a job in a GHG-intensive occupation. A larger incidence of a given type of occupation among high-wage workers than among middle or low-wage workers is indicative of a positive wage gap between that occupation and the others.
Source: Secretariat’s estimates based on version 24.1 of the O*NET database and the following country-specific sources: Australia: Table Builder of the Australian Bureau of Statistics (Labour Force: Characteristics of Employment); Canadian Labour Force Survey; United States: Current Population Survey; All other countries: EU Structure of Earnings Surveys.
Annex Figure 2.C.10. The wage gap in GHG-intensive occupations is less negative for women
Percentage difference between the share of green-driven and GHG-intensive occupations among high and low-wage workers, 2018
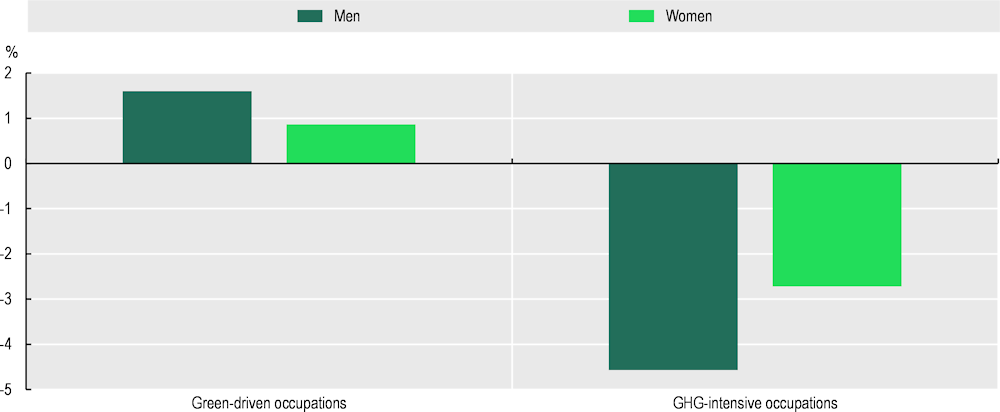
Note: The figure reports the cross-country unweighted average difference in the percentage of green-driven and GHG-intensive occupations in wage and salary employment between high and low wage workers, by gender. High (resp. low) wage is defined as hourly wage above one‑and-a-half times (resp. below two‑thirds of) the median wage. Included countries are Australia, Belgium, Canada, Czechia, Denmark, Estonia, Finland, France, Germany, Greece, Hungary, Iceland, Italy, Latvia, Lithuania, Luxembourg, the Netherlands, Norway, Poland, Portugal, the Slovak Republic, Slovenia, Spain, Sweden, the United Kingdom, the United States. Agriculture is excluded except in Australia, Canada and the United States. Data for Belgium, Denmark, France, Greece, Iceland, Italy, Luxembourg, Portugal, Sweden and the United Kingdom do not include firms with less than 10 employees. Data for Canada and the United States refer to 2019. GHG: greenhouse gases. Countries are ranked by the difference in the incidence of GHG-intensive occupations between high and low wage workers.
Reading: On average, the share of green-driven occupation is 1.6% higher among high-wage men than among low-wage men. A larger incidence of a given type of occupation among high-wage workers than among middle or low-wage workers is indicative of a positive wage gap between that occupation and the others.
Source: Secretariat’s estimates based on version 24.1 of the O*NET database and the following country-specific sources: Australia: Table Builder of the Australian Bureau of Statistics (Labour Force: Characteristics of Employment); Canadian Labour Force Survey; United States: Current Population Survey; All other countries: EU Structure of Earnings Surveys.
Annex Figure 2.C.11. Employees in green new and emerging occupations are mostly high wage workers
Percentage shares of green-driven occupations by country and wage category, 2018
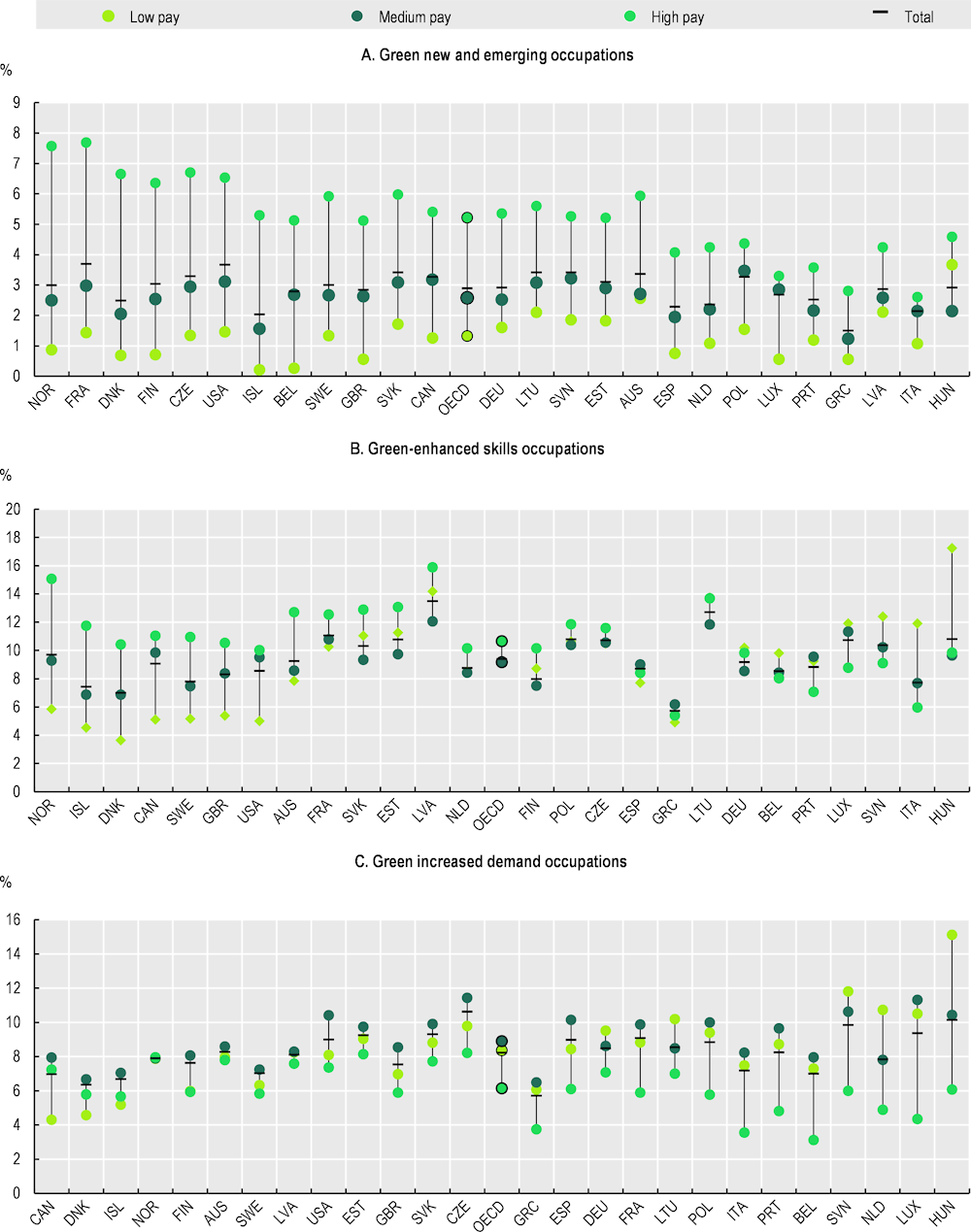
Note: The figure reports the percentage of each type of green-driven occupations in wage and salary employment, by country and hourly wage category. High (resp. low) wage is defined as hourly wage above one‑and-a-half times (resp. below two‑thirds of) the median wage. Agriculture is excluded except in Australia, Canada and the United States. Data for Belgium, Denmark, France, Greece, Iceland, Italy, Luxembourg, Portugal, Sweden and the United Kingdom do not include firms with less than 10 employees. Data for Canada and the United States refer to 2019. OECD: Unweighted average of countries shown. GHG: greenhouse gases. Countries are ranked by the difference in the incidence of each type of occupations between high and low wage workers.
Reading: In Norway, 7.6% (resp. 2.5% and 0.9%) of high-wage (resp. middle‑wage and low-wage) employees have a job in a green new and emerging occupation. A larger incidence of a given type of occupation among high-wage workers than among middle or low-wage workers is indicative of a positive wage gap between that occupation and the others.
Source: Secretariat’s estimates based on version 24.1 of the O*NET database and the following country-specific sources: Australia: Table Builder of the Australian Bureau of Statistics (Labour Force: Characteristics of Employment); Canadian Labour Force Survey; United States: Current Population Survey; All other countries: EU Structure of Earnings Surveys.
Annex Figure 2.C.12. Gap in the share of green-driven or GHG-intensive occupations in non-strained jobs compared with strained jobs
Percentage points, 2021 for EU-OECD countries and 2015 for the United States
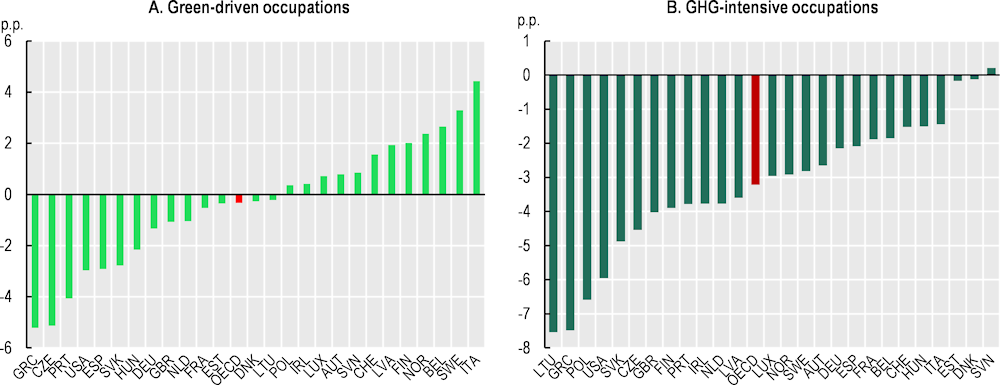
Note: The chart reports the difference in percentage points between the share of green-driven occupations in non-strained jobs compared with the share of green-driven occupations in strained jobs (Panel A) and between the share of GHG-intensive occupations in non-strained jobs compared with the share of GHG-intensive occupations in strained jobs (Panel B). OECD: Unweighted average of countries shown. GHG: greenhouse gases.
Reading: In Panel A, in Greece, the share of green-driven occupations in non-strained jobs is 5.5 percentage points lower than in strained jobs, which means that workers in green-driven occupations are more strained than the others. In Italy, on the opposite, the share of green-driven occupations in non-strained jobs is 4.4 percentage points higher than in strained jobs, which means that workers in green-driven occupations are less strained than the others.
Source: Secretariat’s estimates based on version 24.1 of the O*NET database and Secretariat’s estimates based on the European Working Conditions Telephone Survey 2021 and the American Working Conditions Survey 2015.
Annex Figure 2.C.13. The incidence of job strain is lower in new and emerging and green-enhanced skills green occupations even when controlling for the job characteristics
Marginal effect of the job strain indicator on the share of green-driven occupations, controlling for main individual and job characteristics, 2021 for EU-OECD countries and 2015 for the United States
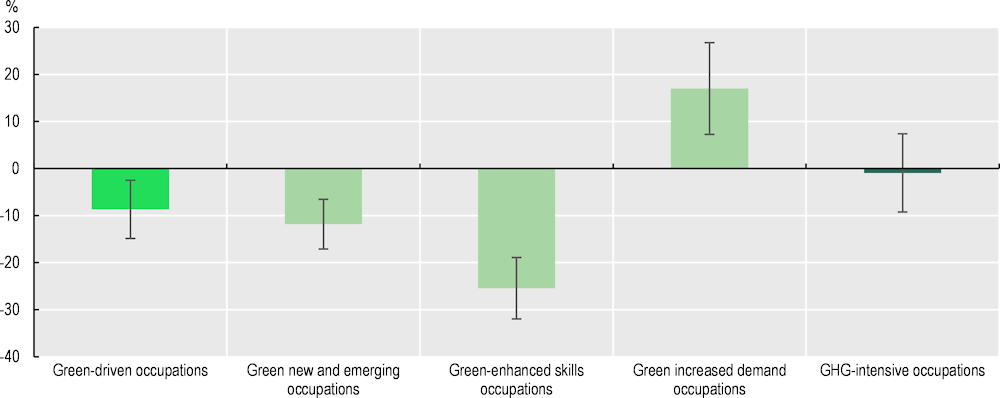
Note: The chart reports the point estimate (and 95% confidence intervals) of the percentage difference in the incidence of each type of green-driven occupation between job strained and not strained workers. Estimates are obtained from a linear regression with the inverse hyperbolic sine of the share of each type of green-driven occupation within all, high, medium or low-skilled occupations as dependent variable and including a job strain dummy, the share of 1‑digit ISCO occupations, educational attainment (3 classes), gender, age (3 classes), sectors (5 categories), and country dummies as explanatory variables. Standard errors are clustered on the dimensions of variability of the dependent variable. The reported point estimates and confidence intervals refer to the estimated coefficient of the job strain dummy and are expressed in percentage of the untransformed dependent variable. The sample excludes Agriculture.
Reading: Controlling for demographic and job characteristics, the percentage share of green new and emerging occupations is, on average, 12% lower among strained employees, i.e. employees whose number of job demands exceeds the number of job resources.
Source: Secretariat’s estimates based on version 24.1 of the O*NET database and the European Working Conditions Telephone Survey 2021 and the American Working Conditions Survey 2015.
Notes
← 1. In addition, estimated costs would be even larger if a wider definition of well-being were considered: for example, Heutel, Miller and Molitor (2021[26]) estimate that, with inaction against climate change, elderly mortality in a country like the United States would increase by more than 2% by the end of this century.
← 2. Individual trajectories out of high-emission jobs will be studied in Chapter 3.
← 3. In 2012, the United Nations Statistical Commission (United Nations, 2012[32]) adopted the Central Framework of the System for Integrated Environmental Economic Accounting (SEEA). This framework allows for the estimation of employment in what is referred to as the environmental goods and services sector (EGSS). Building on the SEAA Central Framework, the ILO (ILO, 2013[93]) proposed a definition on green jobs which was adopted at the 19th Conference of Labour Statisticians Guidelines (ICLS), which differentiates between employment in the environmental sector (employment in environmental processes and employment in the production of environmental outputs) and green jobs, which includes these categories but must also fulfil the criteria for decent work. Conceptual and practical difficulties in its operationalisation have limited the adoption of this definition in the literature.
← 4. This approach typically uses direct and indirect greenhouse gas emissions generated in producing a good or service, and takes activities with the lowest emissions as green. However, although this approach is attractive, data limitations makes it difficult to use it systematically, in particular to identify green jobs (Rodrigues et al., 2018[97]; Bontadini and Vona, 2023[30]).
← 5. These are defined as: i) those activities whose primary purpose is the prevention, reduction and elimination of pollution and other forms of degradation of the environment; and ii) those activities whose primary purpose is the preservation and maintenance of the stock of natural resources and hence safeguarding against depletion – see e.g. Keese and Marcolin (2023[7]).
← 6. See www.ons.gov.uk/economy/environmentalaccounts/methodologies/thechallengesofdefiningagreenjob.
← 7. This implies that a security guard working for a renewable energy power generation plant would be considered green, while a wind turbine service technician working for a plant mainly active in fossil fuel electric power generation would not be considered green.
← 8. Green tasks can be defined as typical activities associated with a particular occupation which have a direct positive impact on green objectives and are directly associated with green activities, such as: input reduction, efficiency, and use of renewables; waste reduction, reuse, and mitigation; pollution and greenhouse gas reduction, prevention, and mitigation; natural resource conservation and reclamation; and environmental advocacy and analysis (Peters, 2013[35]). A more extensive definition could consider any task that is executed using resource‑efficient or environmentally friendly technologies (Biagi, Vona and Bitat, 2021[56]).
← 9. Most, if not all, of the studies that look at the intensity of jobs in terms of green tasks start from the list of green tasks by occupation as published by O*NET.
← 10. Renewable energy generation; green transportation (activities related to increasing efficiency and reducing pollution in transportation); energy efficiency; green construction (construction of new green building, retrofitting and installation of other green technologies in buildings); energy trading; energy storage; CCUS; energy-related research, design and consulting services; environment protection; natural or high-efficiency agriculture and forestry; manufacturing of green technology and energy-efficient manufacturing processes; governmental and regulatory administrations associated with conservation and pollution prevention, regulation enforcement, and policy analysis and advocacy.
← 11. For example, most type of construction workers – e.g. cement masons and concrete finishers – will likely be in demand because of the need of new infrastructure, even if their job is not directly involved in any green or low-carbon activity as described above – see e.g. Dierdorff et al. (2011[46]).
← 12. ILO also adds climate adaptation activities in the list of green activities.
← 13. A few studies for Europe tend to obtain much larger estimates of the order of 40% – e.g. Bowen and Hancké (2019[90]) and Eurofound (2022[88]). However, as shown by Valero et al. (2021[29]), these large estimates are essentially due to the complexity of translating information from one occupational classification (US SOC) to another (ISCO) and the questionable choice of assigning a “green” label to each ISCO occupation that matches at least one US SOC occupation. More generally, as argued by Vona (2021[56]), a much larger size of green employment in other countries than in the United States is a red flag for unsuccessful crosswalking across occupational classifications – see also Annex 2.A.
← 14. It is important to underline here that this definition is purely operational, as it serves the purpose of characterising those jobs that are likely positively impacted by the net-zero transition, and should not be seen as a normative definition of desirable jobs.
← 15. France Stratégie and Dares (2022[91]) made a growth forecast exercise by occupation with results published at a relatively disaggregate level (83 occupations). This exercise includes published forecasts for a low-carbon scenario, which has been used by France Stratégie (2023[92]) to identify occupations that are likely to be boosted by the net-zero transition. However, the classification used in these publications (FAP87) does not have an obvious crosswalk into ISCO (or other commonly used occupational classifications) and remains relatively aggregate with respect to the classifications more frequently used in this chapter. For these reasons, these data have not been exploited in this chapter.
← 16. More precisely, it is likely that, in each occupation of this group, new jobs with altered, and greener, tasks (requiring different skills and credentials) will expand and old jobs with old types of tasks and skill requirements will downsize.
← 17. The 2019 version of the O*NET database used here to define these occupations is the latest version updating jobs and tasks associated with green economy activities (O*NET database version 24.1 – www.onetcenter.org/dictionary/24.1/excel/).
← 18. In contrast with previous OECD work, however, for countries using the ISCO classification, this chapter exploits a new crosswalk developed at a very disaggregate level as a joint effort by the European Commission and the US Department of Labor – European Commission, Directorate General for Employment, Social Affairs and Inclusion, through the ESCO Secretariat, and the US Department of Labor, Employment and Training Administration, through the O*NET Network. This crosswalk has been developed between 8‑digit SOC occupations and 3 008 ESCO categories which represents a further disaggregation of ISCO occupations at the 4‑digit level. The crosswalk is available at https://esco.ec.europa.eu/en/about-esco/data-science-and-esco/crosswalk-between-esco-and-onet and www.onetcenter.org/crosswalks/esco/ESCO_to_ONET-SOC.xlsx. Moreover, this chapter extends the country coverage by applying other crosswalks between the US SOC classification and the Canadian National Occupation Classification (NOC) and Australian and New Zealand Standard Classification of Occupations (ANZSCO). See https://github.com/thedaisTMU/NOC_ONet_Crosswalk and https://labourmarketinsights.gov.au/media/pmukn3qt/ anzsco-2013-to-onet-soc-2019.xlsx.
← 19. The aggregate estimates of the workers employed in green-driven occupations shown in Figure 2.3 are higher than most of those that have been found in previous analyses on “green jobs” – see Section 2.1.1 above – including some of the OECD studies (Causa et al., 2024[69]; Causa, Nguyen and Soldani, 2024[64]), essentially because the concept used is more general as this analysis covers all those occupations that are likely to benefit from the transition to net zero. The estimates of GHG-intensive occupations, on the opposite, are broadly aligned with previous work and the small differences can be explained by the selection of high-emission industries and by the different crosswalks across different occupational classifications that have been used.
← 20. Green new and emerging occupations will be shown below to be the fastest growing group of green-driven occupations.
← 21. Two-thirds of the employment in these “mixed” occupations is on average in green-enhanced skills occupations, one‑third in green increased demand occupations and less than 0.2% in new and emerging occupations.
← 22. Yet, as discussed above, new and emerging occupations weigh little in the aggregate stock of green-driven occupations.
← 23. For this latter group, the employment dynamics is likely to result from a, possibly temporary, composition effect, as the (larger) segment with old requirements in declining industries tends to downsize while jobs with new requirements in the (smaller) environment-friendlier sector tend to expand. Once the transition reaches an advanced stage, it is likely that employment growth in these occupations will start again.
← 24. If occupations concentrated in agriculture are excluded, the average rate of decline is halved but remains significant.
← 25. There is a correlation coefficient of 0.41, with zero being no correlation and 1 perfect positive correlation, between green-driven and GHG-intensive occupations, which decreases to 0.29 if those occupations that can be both green-driven and GHG-intensive are excluded.
← 26. Eurostat defines rural an area where more than 50% of its population lives in rural grid cells, i.e. not urban centres or urban clusters.
← 27. However, the rural-urban difference is larger in the case of GHG-intensive occupations than in the case of green-driven occupations, suggesting that GHG-intensive occupations are much more concentrated in rural areas than green-driven jobs.
← 28. In Australia, where the gap is the largest, the share of green-driven occupations among men is 11.6 percentage points higher than the national average while the share of green-driven occupations among women is 13 percentage points lower than the national average. In Greece, where the gap is the smallest, the share of green-driven occupations among men is 4.4 percentage points higher than the national average while the share of green-driven occupations among women is 6 percentage points lower than the national average.
← 29. As noted in OECD (2021[2]), women, even if not directly impacted, may still suffer from second-round effects of plant closures. In the United Kingdom, Aragon et al. (2018[89]) show that female employment was hit a generation after the closure of the mines. The authors of the study attribute the time lag to the possibility that former miners may have rejected certain jobs perceived as “women’s work”, while the subsequent generation displayed greater willingness to pursue such opportunities.
← 30. This section follows the three dimensions of the OECD Job Quality framework but the operationalisation differs slightly to make it applicable to the context and data availability. In particular, while the measure of earnings quality captures both average hourly wages and their distribution at national level, this chapter, which looks at individual workers, only considers average hourly wages.
← 31. High-wage (resp. low-wage) employees are defined as those with gross hourly wage larger than, or equal to, one‑and-a-half times (resp. smaller than two‑thirds of) the median wage in the country. Middle‑wage employees are the residual category including the median.
← 32. As shown in Figure 2.9, Panel B, the unconditional average statistic presented in Figure 2.9, Panel A is within the 95% confidence interval of the cross-country estimate obtained through regression methods, controlling for individual characteristics. Regression estimates presented in the rest of this chapter are obtained by fitting linear regression models in which the dependent variables have been multiplied by 20 and transformed using an inverse hyperbolic sine transformation. The pre‑multiplication by 20 is done to ensure that sample means are greater than 10 for all the dependent variables, as required for estimate reliability (Bellemare and Wichman, 2019[94]). Percentage effects are retrieved by applying the standard logarithmic approximation (Halvorsen and Palmquist, 1980[95])
← 33. Given the employment size of GHG-intensive occupations, the effect on the aggregate gender wage gap would be minimal, however.
← 34. The results for Norway are not due to offshore extractive activities. When the corresponding sector is excluded altogether from the sample, results remain unchanged.
← 35. Interestingly, in 12 out of 26 countries, the highest share of GHG-intensive occupations is among middle‑wage employees.
← 36. See Box 2.4 for a detailed explanation of the difference between workers in GHG-intensive industries and workers in GHG-intensive occupations.
← 38. Despite these differences, cross-country correlations between wage premia (penalties) by type of green-driven occupations are high (always larger than 0.6), and country rankings are relatively stable – the lowest spearman rank correlation coefficient (0.6) is found between new and emerging green and green-enhanced skills occupations, but it remains largely significant at standard levels. In other words, in a country, in which one type of green-driven occupation commands higher salaries, salaries will also be higher in all other types of green-driven occupations.
← 39. Results are qualitatively similar if occupations that are green-driven but are concentrated in GHG-intensive occupations are excluded from green-driven jobs of different types.
← 40. See Annex Figure 2.C.6. Typical examples of new and emerging occupations with a significant employment share are for example Water/wastewater engineers and Solar sales representatives and assessors, all high-skill occupations.
← 41. Except for green increased demand occupations, where the effect becomes slightly larger, while remaining contained.
← 42. Results even change sign in certain cases: estimated differences are 19%, ‑4%, and 27% for, respectively, new and emerging, green-enhanced skills, and green increased demand occupations, respectively.
← 43. These results are obtained by splitting samples into high, medium and low-skilled occupations, and fitting the regression models on the split samples.
← 44. Somewhat similar results are found for GHG-intensive occupations that, within high-skill occupations, are more frequent among high-wage employees than among low-wage employees (with an estimated difference of 25%).
← 45. In principle, this risk should be compounded with the effective replacement rate of unemployment benefits (see Chapter 1). However, an estimate of this rate is not available at the individual level in the microdata used for this chapter.
← 46. The underlying assumption, which these estimates rely on, is that all unemployed who worked before lost their previous job. In appreciating these results, it is important to keep in mind that, in dynamic labour markets, workers may quit their job to search for better job opportunities while unemployed. To the extent that certain subsegments of the labour market of green-driven occupations are highly dynamic, the statistics presented in Figure 2.12 may therefore overestimate the level of job insecurity of these jobs. Nevertheless, the fact that green-driven occupations are no more frequent among short unemployment spells (less than 6 months) than among all others, suggest that the risk of overestimation is extremely limited.
← 47. Controlling for demographic characteristics.
← 48. Although insignificantly so in the case of green-enhanced skills occupation.
← 49. More precisely, low-skill green new and emerging and green increased demand occupation are estimated to be, respectively, 12.7% and 18% more frequent among the unemployed than other low-skill jobs.
← 50. These occupations are 31% more frequent among the unemployed, when high-skill, and 11% less frequent, when medium or low-skill.
← 51. While this holds on average, there are nevertheless specific jobs and cases in which temporary contracts are associated to stable, good-quality jobs – see e.g. OECD (2014[15]) and below for a discussion.
← 52. And even worse labour market security in the case of green-enhanced skills occupations.
← 53. Unfortunately, available data do not allow testing these hypotheses.
← 54. For European countries, the 2015 wave of the European Working Condition Survey could also have been used. However, the difference in survey mode (until 2015, workers were interviewed face to face while in 2021, due to COVID‑19, the survey was undertaken via telephone) and the changes to the wording and response options for some of the questions of interest advise against pooling together the 2015 and the 2021 waves.
← 55. The European Working Condition Survey and the American Working Conditions Survey are broadly comparable but the differences in the wording and response options suggest some caution in comparing the results between the two surveys.
← 56. In the case of ISCO occupations, this could be accomplished by using more detailed ESCO (European Skills, Competences and Occupations) categories for specific occupations, for example by collecting up to the 6‑digit ESCO category for certain occupations such as “power production plant operator” (ISCO/ESCO 3131). Note that up to the 4th digit, ISCO and ESCO are the same classification. In the case of ISIC industries, CReMA categories could be used to further split detailed ISIC industries. The Classification of Resource Management Activities, abbreviated as CReMA, was developed by Eurostat and classifies activities, products, expenditure and other transactions that aim to preserve and enhance the stock of natural resources (Eurostat, 2020[96]). For example, “Production of energy from renewable resources” (CReMA 13A) could be separated from the rest of “Electric power generation, transmission and distribution” (ISIC 3510).
← 57. By contrast, this property does not hold if the whole occupation i that matches with both green occupation c and non-green occupations c’ is considered green, as done in some of the previous works in the literature – see Section 2.1.
← 58. In other words, for any indicator that is a proportion with total employment at the denominator, the bias issue discussed in Annex 2.B does not emerge.
← 59. That work and most of previous OECD work – e.g. OECD (2023[27]) – has applied it starting from an old BLS many‑to-many crosswalk between 6‑digit SOC categories and 3‑digit ISCO categories, – see www.bls.gov/soc/ISCO_SOC_Crosswalk.xls.
← 60. https://esco.ec.europa.eu/en/about-esco/data-science-and-esco/crosswalk-between-esco-and-onet and www.onetcenter.org/crosswalks/esco/ESCO_to_ONET-SOC.xlsx.
← 61. No bias is found for educational attainment categories.