Conventional CBA for the most part continues to regard (intra-generational) distributional or equity concerns as having little or no place in making its recommendations about policy formulation or investment projects. Identifying this oversight is one thing, responding to it is more controversial especially where this involves weighting costs and benefits according to equity criteria. But this usefully might just involve simply identifying the costs and benefits of individuals and groups on the basis of differences in the characteristic of interest. Perhaps this sounds unambitious but given the starting position (where this seldom happens), more routine cataloguing of this type surely would be useful. Moreover, this could involve not only cataloguing how costs and benefits are distributed across people but also how particular environmental goods and bads (such as air quality, unwanted land uses and so on) are distributed. One catalyst almost certainly could be demand from policy makers. That is, the observation that too much practical CBA neglects distributional concerns may not just be a supply problem (a single-minded focus of cost-benefit practitioners on efficiency), it is also likely to be an issue about demand: perhaps, for example, policy makers perhaps have not required this information in the terms of reference guiding that practical work. Taking this further might involve weighting costs and benefits and scrutinising proposals on the basis of a distributional cost-benefit test. While a long-standing analytical option, there is no easy answer to the equally long-standing question about what value these weights should take. Nevertheless, exploring this question has led to some interesting empirical insights about inequality aversion generally and for specific goods and bads (such as health risks).
Cost-Benefit Analysis and the Environment
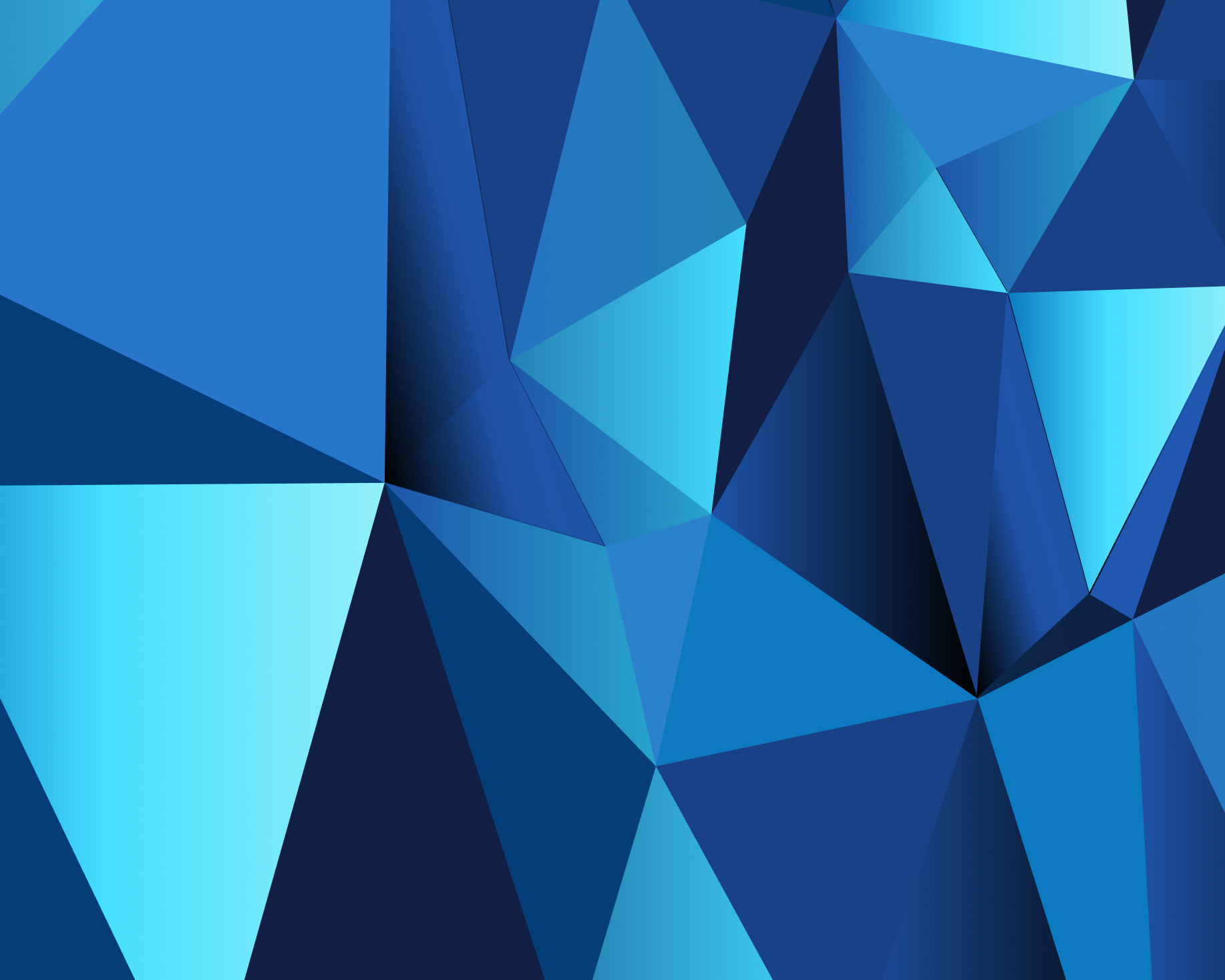
Chapter 11. Distribution and cost-benefit analysis
Abstract
11.1. Introduction
Practical cost-benefit analysis (CBA) is concerned (primarily) with efficiency in allocation of economic resources. That is, it recommends actions with benefits greater than costs, where these monetary net benefits reflect the willingness to pay (or accept) that affected people have for various impacts of the proposal. Of course, actual decisions will not solely be based on efficiency. For example, project or policy selection and design may give rise to questions about the “rightness” of a given action or the social desirability of a particular distribution of benefits and costs.
Economic appraisal is not silent on those issues. There exists long-standing and elaborate guidance on incorporating some of these distributional concerns in CBA. How far this advice is actually heeded is another matter, however. For example, in some OECD member states, including estimates of the distribution in CBA across transport investments, energy investments, new policy assessments as well as ex post assessments, is done compulsorily but in other members it is less frequent (see Chapter 16). This does not mean that distributional effects are ignored altogether where CBA is done. More likely these are dealt with in other ways, explicitly or otherwise.
A specific example is the economic appraisal that preceded the introduction of the London Congestion Charge (LCC). Under original proposals for the LCC, from February 2003, motorists would pay a uniform charge to enter the congestion charge zone around central London during (weekday) designated peak hours. Certain groups were to be exempted or to face a lower charge, depending on whether they were residents or worked in particular occupations. The charge revenues collected were to be reinvested in London’s bus services. Each of these provisions had a distributional rationale, but plausibly entailed some sacrifice in efficiency as, for example, those groups enjoying exemptions from the charge would still treat road-use as being “free” at the point of access.
Presumably London’s decision makers reasoned that this sacrifice was worth it if it allayed at least some of the public’s concerns about the distributional impacts of the LCC. However, the point here is that, insofar as CBA was concerned, these distributional considerations were (it appears) a “fait accompli’. In other words, the official CBA of this scheme carried out on behalf of the Greater London Authority (GLA) – the body responsible for administering the LCC – was conducted on an option which already had these distributional decisions built into it. One response might be to consider this the natural order of things. That is, distributional concerns were dealt with elsewhere in the impact assessment process, leaving CBA to deal with what is its core activity. However, if practical CBA were better able to reflect concerns about distribution then it too would have an obviously clearer contribution to such earlier deliberations about options.
This is the question considered in this chapter. That is, how distributional implications of projects and policies reasonably can be accommodated within environmental cost-benefit appraisals. This, in turn, reflects an assertion, not necessarily shared by all cost-benefit practitioners, for appraisals to be more sensitive to concerns about distributional justice or equity. (See also Chapters 8and 12 for a discussion of equity between generations in the context of CBA.) The emphasis is on how this might be done in practice. However, there is also a need for principles and theory to inform this pragmatic approach. And while the onus is on practitioners to advance this agenda, so too is there an onus on decision-makers to require this information; that is, it is about demand as well as supply.
11.2. Equity and CBA
Projects and policies with environmental impacts inevitably have distributional consequences. Indeed, for a great many environmental policies this is the point in that these interventions work by favouring (relative to the status quo) victims of pollution at the expense of polluters. Typically, the economic justification for these interventions is couched in terms of their efficiency (in the sense of giving rise to higher overall economic gains for society). However, this application of the polluter pays principle (e.g. OECD, 1975) owes as much to the perceived desirable distributional consequences of assigning property rights to the victims of pollution or natural capital damage.
Clearly, the relationship between polluter and victim is only one dimension of difference between people affected by project or policy proposals. Polluters might be households or economic producers (such as firms1), rich and poor, young and old, as well as vulnerable (in some other way) or otherwise. What is to be distributed between these people or institutions is also of considerable importance given that it can help to define differences in which are thought to be relevant. This could include well-being generally as well as its particular component parts, although it might instead refer to some other set of concepts such as “functionings” and “capabilities” (Kriström, 2005, Decancq et al., 2014).
In the context of economic appraisal, a starting point for this concern presumably is how the net benefits are distributed between those affected by a proposed action. A simple illustration is as follows. Assume there are just two individuals in society, this time denoted by R and P, affected by a project and that the net benefit to each individual (R and P) of some environmentally improving project is:
Individual R: +EUR 200
Individual P: -EUR 100
The total net benefit of the project is EUR 100; therefore, the project is worthwhile in the sense that it increases economic efficiency. These gains, however, are unevenly distributed. The question is whether this matters.
Much of practical CBA arguably takes the view that this outcome does not matter; that is, Kaldor-Hicks criterion can be invoked. As long as winners can potentially compensate the losers then all is well. That is, it makes sense to select proposals so as to maximise the size of economic pie that our society here can enjoy. If, for some reason, there were distributional concerns then policy makers could worry separately about how this pie is divided, using alternative redistributive policy instruments at their disposal. Perhaps, however, it is thought that – in the round – net losers of such a project will be net winners for efficient projects implemented elsewhere. In this case, worrying too much about one decision – in isolation – is a mistake. Alternatively, perhaps distribution is considered to be optimal (in terms of its implications for social welfare). In this case, there is nothing to be gained by shuffling economic resources from one person to another to achieve a better distribution (one that achieves more in terms of social welfare).
The case for arguing that such differences do matter might begin by considering the possible problems with these aforementioned perspectives. In practice, however, efficiency and equity might not be as straightforwardly separated as this standard approach implies. If, for example, compensation is not really feasible to sort out through other policy instruments then project selection (or design) arguably, and within reason, should not be ruled out as a way of addressing society’s distributional goals. Whether gains and losses ultimately do even out is another matter (Persky, 2001) – although presumably, an interim conclusion is that the case for believing in such a coincidence act is doubtful, while not impossible. If distribution is not optimal – e.g. maybe there are political or administrative obstacles to introducing the requisite measures – there is a further rationale for worrying about distributional impacts.
Even so, it is not altogether clear exactly to do to reflect distributional concern in an economic appraisal. While the above example is simplicity itself, the possible answers to this can get complicated very quickly. For example, what if individual P – the net loser – is poor relative to the net beneficiary of this project? In other words, the project worsens an already unequal distribution of income or wealth. Presumably, this might temper an otherwise positive interpretation of the net worth of the project. But what if also P is also a polluter and R is vulnerable and its gains reflect how he or she benefits from the environmental improvement. How might this alter again judgements about the proposal, and more importantly, what can a cost-benefit practitioner do about it?
11.3. Analysing the distributional impacts of projects within a cost-benefit framework
Various proposals exist about how to bring the distributional consequences of projects and policies within the ambit of cost-benefit appraisals. One suggestion, which is followed in this chapter, is to view these proposals as a hierarchy necessitating ever more explicit judgement, on the part of the cost-benefit analyst, about the social desirability of possible distributional outcomes. At the heart of any such judgement lies a standpoint or appeal to evidence about how society ought to distribute well-being, income, wealth or some more specific good such as environmental quality.
Kriström (2005) has shown how these proposals can be thought of as a hierarchy of options. These include: 1) identifying and cataloguing how project-related costs and benefits are distributed, in physical units but perhaps also in monetary terms; 2) calculating implicit distributional weights: e.g. if a project generates net aggregate losses but net gains are enjoyed by a group that society is particularly worried about, what weight would need to be assigned to these gains such that the project was deemed to have a positive social value?; and lastly, 3) re-calculating the project’s net benefit based on assigning explicit distributional weights to the benefits received and costs incurred by different societal groups.
Important to all these stages is the consideration of distributional consequences alongside (or within) standard appraisal procedures. In doing so, information can also be provided about the balance (or implied trade-off) between maximising the overall benefits of an intervention and directing interventions towards certain groups. Activities supported in the lower (initial) reaches of this hierarchy are less contentious in that these do not attempt to alter the main recommendation of a CBA. Rather what is done is augment that rule with further information, in effect, provided as an adjunct to a CBA. Towards the top of this hierarchy, however, the decision-rule itself is altered.
11.3.1. Identifying distributional impacts
CBA is frequently criticised as being pre-occupied with a project’s or policy’s “bottom-line” in the form of its net social benefits. Assuming, for the moment, that this criticism is justified, it is problematic for a variety of reasons, including that it abstracts from information that policy makers worry about: namely, how policy impacts are distributed. This disaggregated information might be valuable for a number of counts. There may be pragmatic reasons for knowing which groups win and which groups lose from implementing the project. Perhaps it is the case the project’s losers are in a position to affect the project’s success or failure (in the sense of net benefits being realised). But there are also analytical frameworks that are both relevant to a social decision and can be usefully illustrated using such information. This might be the established theory underpinning CBA, based on social welfare functions describing how (changes in) consumption translates in social well-being. But it may be a distinct tradition drawing on other disciplinary or policy perspectives as well perhaps taking as its inspiration the idea of “value plurality” discussed in Chapter 2.
For example, the environmental justice movement in the United States has argued that unwanted or hazardous land-uses (such as waste disposal and transfer facilities) are unfairly or inequitably distributed: i.e. located predominately in areas, which are relatively highly populated by low-income groups or particular ethnic groups. The environmental justice perspective has been broadened to a number of other environmental burdens both within and across countries (e.g. urban air pollution, lack of access to green space and vulnerability to climate change) (see, for example, Walker, 2012). This does have relevance for economic appraisal, based as it is on rationing economic resources to those projects and policies where willingness to pay is highest, whereas the contribution of environmental justice has been to emphasise how benefits and burdens are distributed amongst different socioeconomic or ethnic groups and whether the processes that have led to this distribution can be judged to be fair.
This suggests that, at a minimum, one useful element of a CBA would be the provision of detailed information about distributional impacts. Put this way, there is no requirement that the cost-benefit analyst makes a judgement about the empirical evidence as regards how to weight the impacts enjoyed or suffered by different groups. It requires only that these be documented to the extent that the data and other resources permit. How these distributional consequences might translate into an assessment of the social value of the project can be left to the political process. Of course, it would be naïve to assume that value judgements are wholly eliminated. For example, a decision must be made at some point, about which societal groups are to be described. However, as later sections will illustrate, there is less need for tricky judgements relative to other analytical options in the hierarchy of distributional CBA.
There may be practical difficulties in identifying “winners” and “losers” and their incomes and/or some other aspect of their relative position in society in sufficient detail. Of course, without this basic building block, the more ambitious analysis of distributional concerns (described below) cannot be contemplated either. This problem is likely to be a matter of degree and it is just as likely that many cost-benefit appraisals do not generate these data simply because they are not compelled to do so rather than because of the unfeasibility of the task. It is interesting that much of modern benefit assessment in the form of stated preference methods such as the contingent valuation method (Chapter 3) already may contain a wealth of data about the distribution of non-market impacts (Kriström, 2003). That is, these studies typically elicit a wealth of information about respondent’s demographic and socio-economic characteristics as well as detailed data about, for example, uses and experiences of an environmental good under consideration. Such data could provide valuable insights into how certain project impacts are distributed.
Although not necessarily incorporated within economic appraisals, empirical evidence exists about the way in which a growing number of environmental outcomes (but particularly, air quality) are distributed amongst different societal groups. Studies by Pearce et al. (2011) and Ribeiro et al. (2015) provide similar types of analysis for respectively New Zealand and Portugal. In a similar vein, a study by Defra (2006) for the UK, looked at how a range of air pollutants are distributed including PM10, NO2, SO2 and (ground-level) O3. A measure of multiple deprivation (including income, health and housing) was the object of interest, in that study, in terms of characterising the socioeconomic status of those affected by differential air quality.
A number of interesting findings and nuances emerge from the subsequent mapping of air quality levels and this deprivation index. Exposure to lower ambient air quality is influenced by proximity to urban areas and roads in particular. And, in general, the concerns of those who assert that worse environmental outcomes are associated with higher levels of deprivation appear to be confirmed, especially for PM10. However, in some cases, the resulting socioeconomic distributional outcome is one where those most exposed are those who are the least and the most disadvantaged (i.e. respectively those households in the bottom decile and the top decile of the deprivation index). In other words, geographical detail appears to be important with different areas of the country experiencing a somewhat different relationship between exposure to air pollution and deprivation. Presumably, this has implications for thinking through the distributional consequences of proposed policy interventions.
Day and Maddison (2015) suggest that cost-benefit practitioners could usefully respond to these environmental justice concerns. For example, they note the potential for using evidence about the way in which (air) pollution burdens are distributed by (household) income. These could be summarised in a Gini-coefficient, perhaps calculated for a policy proposal (and compared with the status quo). Examples of this work can be found in the water resource literature (via the so-called “Water Gini”; see, for example, Wang et al., 2012; Seekel et al., 2011). Clearly, however, there are multiple dimensions to inequality and, to be meaningful, such summaries of distribution also need to explicitly account for this (e.g. by age, vulnerability, and so on).
Another manifestation of distributional concern is in judgements about the affordability of policies for households. Practical examples here include the use of CBA in the implementation of environmental Directives amongst relatively new (and lower-income) entrants to the European Union (e.g. European Commission, 2008). This is often codified in (seemingly arbitrary) rules of thumb about when the proportion of income that households pay becomes unaffordable for a particular good (such as water supply or waste collection). Such concerns, however, may conflict with other considerations, such as the polluter pays principle or (where it is considered appropriate) full cost recovery for projects such as waste management. So while affordability is clearly a concern, there is a risk created if this is addressed by widening any gap between project revenues and project costs through setting tariffs too low.
Potential also exists, of course, for greater attention to the distributional impacts in other standard non-market valuation methods of the type discussed in earlier Chapters (see 3-7). Loomis (2011) illustrates this potential with reference to two empirical studies of non-market values from the United States. Specifically, the author discusses the results from a CV study of willingness to pay (WTP) to low flow alleviation in a river in Colorado in the United States from a distributional perspective. This leads to some interesting (and additional) insights. Benefits do not necessarily vary much with income levels of respondents. But while project selection is not being driven by a “bias” towards the disproportionately high WTP of those with high-income, on the cost side there is concern dependent, of course, on how the improvement is financed. Clearly this is important information for the implementing authority to know.
A further example that Loomis discusses is a hedonic price study in an area of Los Angeles again in the United States. Specifically, the policy measures looked at what are the effects on property values of actions that would decrease the risks of forest fires. This policy, it appears, is skewed towards benefiting the asset values of homes owned by higher income households as well as particular ethnic groups (i.e. predominately White and Hispanic neighbourhoods). Again the issue here is how to use this information on how benefits are distributed to frame policy discussion about how proposals should be financed. Perhaps the most important take-home message from both of these examples is that distributional insights could be garnered from the growing empirical record about environmental valuation. That this potential remains largely unrealised is a missed opportunity.
In a very different context, a relatively well-established finding is that particular groups appear to be vulnerable to the loss of ecosystem services. Specifically, a number of studies have highlighted the dependence (of at least some portion) of the rural poor in the developing world on services provided by nature. Ten Brink et al. (2011) term this the “GDP of the poor” although its antecedents can be traced to previous empirical studies of livelihoods including Jodha (1986) and Vedeld et al. (2004). These studies have been important in making plainer the contribution of ecosystems to the economic well-being of such communities, something which is typically only partially reflected in official statistics, if at all.
11.3.2. Implicit distributional weights
Should cost-benefit appraisals limit the analysis of distributional issues to carefully identifying and cataloguing how costs and benefits are distributed? Broadly speaking there are two further options. Both are premised on thinking about how distributional information might be used by policy makers but in a way that is comparable with the standard net benefit rule. This entails revising the CBA recommendation on the basis of the adjusted or distributionally weighted net benefit.
One long-standing way of being explicit about such distributional judgements in CBA is by writing the earlier simple two-person cost-benefit problem as follows: NB = aRNBR + aPNBP, where a weight (ai) is assigned to each of the parties” costs and benefits. An important feature of conventional CBA becomes apparent. It assumes that a1 = a2 = 1; that is, weights of unity are assigned to the net benefits of individuals regardless of who it is that receives a unit of benefit or suffers a unit of cost.
What do these weights represent? Essentially, ai, can be interpreted as providing a numerical description about the preferences of society regarding distributional outcomes. This might be based on an introspective reflection on how say changes in consumption translate into higher levels of well-being for individuals given how affluent they are prior to this change. It might be based on a (related or distinct) standpoint regarding equity for society. (See the Annex to this Chapter for a simple elaboration of this, within the cost-benefit framework.)
The distributional net benefit criterion is that a project should go ahead if the sum of distributional weighted net benefits is at least as great as zero. Not surprisingly, much of the controversy about distributional weighting surrounds debates about the relative merits of using projects in this way as well as the problems entailed in surmising what society’s distributional goals are. It is not difficult to imagine that there might exist some trepidation about launching into distributional analysis of this sort, given these difficulties and debates.
A convenient way around this controversy – at least as an interim stage – is to ask instead what set of weights would be required to “tip the balance” between recommending that the project go-ahead (i.e. positive total NB) or not go-ahead (i.e. negative total NB) (Gramlich, 1990; Kriström and Kanninen, 1993)? i.e.
. Hence,
this is an implicit distributional test, as it does not require that weights (ai) be imputed directly. Rather it asks, for aR = 1 and setting NB=0, how large would the implicit weight aP* need to be to affect the decision about the social worth of the project? For the simple example above, the answer is “2”: i.e.
.
Once this “tipping point” is known, what can be done with this information? Perhaps most importantly, it could be asked whether assigning this weight (or these weights) is justified, perhaps in the sense of whether or not it is commensurate with society’s preferences or what is known about political acceptability. The answer could depend, in this simple example, on the relative income difference between the two individuals as well as the distance that each is from recognised thresholds, such as poverty levels or average income. The catch is that this question arguably cannot be answered properly unless one has recourse to reliable and direct estimates of aP. Yet, the point of this implicit weighting approach is to allow the cost-benefit analyst to avoid these potentially deep waters. Nevertheless, implicit weights at least can be compared with the range of estimates in the literature, which is discussed in Section 11.3.3 below.
Gramlich (1990) notes a further use of the data previously discussed. Project selection or design is only one of many redistributive mechanisms available to governments. Moreover, critics of distributional CBA, such as Harberger, have argued that it must be asked whether these alternative measures are generally a less socially wasteful means of addressing distributional concerns. For example, this would certainly be true if, say, some fiscal mechanism could costlessly redistribute e.g. income. In such instances, it would always be desirable to shelve inefficient but equitable projects and address distributional disparities using this other redistribution mechanism. Needless to say, any redistributive scheme is inefficient to a greater or lesser extent.2 However, this emphasis on “extent” is important. One issue then is to compare, as a means of addressing distributional concerns, project selection or design with (practical) alternatives, such as direct ways of transferring incomes across individuals (perhaps via the tax system) or other public programmes, which explicitly focus on, say, raising low incomes.
Assuming that there is information about how inefficient various practical and alternative redistributive mechanisms are, then this sets an upper bound on how much inefficiency is permissible in choosing and designing projects on the basis on distributional criteria. Formally, this entails a comparison of the terms aP* and 1/(1-c). The coefficient, c, is an indicator of how inefficient alternative redistributive mechanisms are (i.e. what proportion of total resources is lost in the “act” of redistribution) and its value will lie between 0 and 1. In the example above, the project should go ahead as long as it is the case that c £ 0.5. In this way, distributional concerns are allowed to influence project choice subject to this being the most cost-effective means of addressing some distributional goal.
11.3.3. Explicit distributional weights
The previous broad analytical option departs from simply asking what values distributional weights would need to take. A rather more prescriptive approach would be to impute explicit weights, perhaps based on the findings of past studies. For example, one approach is based on a judgement about the importance of income to those who gain or lose from the project. The assumption of diminishing marginal utility of income implies that the utility value of a unit change in a poor individual’s income is greater than the utility value of the same unit change in income of a rich person. Other things being equal, this implies that a dollar or euro of benefit received by the latter receive less weight by the same change for the former reflecting this difference in its relative contribution to social welfare.
One possible weight, following this rationale, is:
where:
is average or mean income per capita; Yi is income of the ith individual (or group); and η is the elasticity of the marginal utility of income or society’s valuation of an increment to that individual’s income. (The derivation of this weight is illustrated in the Annex to this chapter.) Clearly, whereas data on the two former parameters are (in principle) easily measurable, it is information about η which is crucial. Intuitively, this elasticity is said to reflect society’s degree of inequality aversion. A logical starting point then for determining its likely magnitude is to ask the question as to how much inequality “society” is willing to tolerate?
In principle, e could range from 0 to ¥, although, fortunately for analysts, the literature as discussed below suggests that the plausible range is considerably narrower than this. Note that conventional or “unweighted” CBA is equivalent to assuming η = 0 (as this would result in ai = 1). At the other extreme, as the degree of inequality aversion becomes ever larger (η ® ¥), the cost-benefit test amounts to always “ruling-out” any project that adversely affects the very worse off. (Conversely, it will always “rule-in” a project that positively affects the very worse off.) And while the simplest assumption, in terms of ease of computation, is to set η = 1 (and thus compare each individual’s income relative to the mean) ultimately it must be asked whether or not this seems to imply stronger societal preferences towards income equality than observed evidence suggests.
Table 11.1 uses the earlier example in Section 11.2 to illustrate how the CBA recommendation for this distributional outcome alters depending on what value is taken by e. To this example, the assumption is added that the ratio of the income of our wealthier individual R to our poorer individual P is equal to 3: i.e. YR = 3YP (e.g. such that perhaps the former is EUR 90 000 and the latter is EUR 30 000). Note that, in general, the effect of assuming values of e which are greater than 0, is to shrink the positive net benefits of individual R and to boost the negative net benefits of individual P. The magnitude of e determines how large this relative adjustment is going to be. Thus, for η = 0.5 the project still has a small but positive NB. However, for η = 1, the sum of distributional weighted NB is negative. It is also apparent from the table that larger values of η very quickly result in relatively extreme weights to be placed on the losses suffered by the individual with income below the mean.
Table 11.1. Distributional weights and CBA – illustrative example
Degree of inequality aversion: |
Net benefits: Individual R |
Net benefits: Individual P |
Total net benefits |
---|---|---|---|
0 |
200 |
-100 |
100 |
0.5 |
163 |
-141 |
22 |
1 |
133 |
-200 |
-67 |
2 |
89 |
-400 |
-311 |
To reiterate, distributional weights reflect a judgement about the value to be placed on each dollar or euro received by or taken away from each individual or group. A variety of data might be sought in order to justify this judgement. Typically, it is argued that this judgement should be made on the basis on the revealed behaviour of (democratic and accountable) governments with regard to, say, redistributive policies. That is, by examining public policies where distributional issues are a predominant concern, something can be learned about the relative weights to be placed on the costs and benefits of different societal groups. A usual reference point is the income tax system where it is argued that the different marginal tax rates that people, with different incomes, face tells the analyst something useful about society’s preferences towards the social value of that income. A prominent variant of this notion is based on equal absolute sacrifice and argues that tax system operates by imposing an equal burden in terms of utility losses on all income classes relative to some utility function (Young, 1994; although see, for example, Gramlich, 1990, for a discussion of the problems of using information about marginal tax rates in this way).
Some empirical debate has centred specifically on the magnitude of e. Comprehensive reviews of this literature can be found in Pearce and Ulph (1999) and Cowell and Gardiner (1999). While the latter survey concludes that “a reasonable range seems to be 0.5 … to 4” (p. 33), Pearce and Ulph (1999) argue for a much narrower range in the region of 0.8. On this basis, Pearce (2003) argues that values of η in the range of 0.5 to 1.2 are defensible in the cost-benefit appraisal of climate change policy. Chapter 8 reviews more recent evidence which concludes that the value of η based on variety of analytical strategies and data might be higher. The point is there that this accommodates a range of possible values, and even for the simple example in Table 11.1 less clear implications of a CBA based upon this.
Estimating inequality aversion
Studies of people’s preferences for income inequality do appear to indicate that, on average, they prefer distributions which are more equal such that, for example, they find that less income overall is tolerable so long as it is more equally distributed. One implication of this is that policy or project interventions which result in negative net benefits – i.e. less overall consumption – could be preferred if that action results in a better distribution of the consumption that is left. This is the essence of distributional weighted CBA, with the magnitude of inequality aversion, for example, setting the parameters for what is an acceptable trade-off between equity and efficiency. Clearly, there is an interesting question of exactly how much net loss that people will tolerate: i.e. what are the weights that should be used. There is also an interesting question about what weights should apply for risks to non-income outcomes of policy or project interventions.
A study by Cropper et al. (2016), using a stated preference survey, does exactly this for health risks: specifically cancer (and other possibly serious) risks arising from exposure to air pollution. Respondents were asked to consider – and choose between – two scenarios each involving risks faced by two regions of equal population size (within a country) each facing distinct health risks. In one scenario, these health risks are (highly) unequal between these two regions. In another scenario, these risks were equally distributed.
This is described in Table 11.2. What the choice indicates is a respondent’s willingness to trade-off how the risk is distributed and the total risk. That is, option B shares the burden equally but entails a population risk of 14/1000 as opposed to option A where the overall risk is 10/1000. Asking multiple respondents to make a series of these choices allows preferences, and the magnitudes of trade-offs that people are prepared to make, to be teased out.
Table 11.2. Example choice card from Cropper et al. (2016)
Choice A |
Choice B |
|
---|---|---|
Region Y |
1/1 000 mortality risk |
7/1 000 mortality risk |
Region Z |
9/1 000 mortality risk |
7/1 000 mortality risk |
Preferred option? |
The results in Cropper et al. (2016) indicate that most respondents have a preference for greater equality in the way that health risks are distributed. That is, respondents appear to be prepared to tolerate a policy action that increases health risks so long as those risks are shared equally. Specifically, a key finding is that the average respondent is prepared to tolerate more than a 20% increase in total cancer cases that the population faces if everyone is confronted with the same (elevated) risk.
This actually understates the extent of this preference as it excludes those respondents for whom no equitable options were off the table. That is, for these respondents, no matter how high the overall cancer risk rate in the total population, they also chose an option which allocated this risk equally over options which had much lower (but unequally distributed) risks. As such they appear to have lexicographic or very strong preferences for health equality. In total, they accounted for around 30% of the sample. The result of including the preferences of this large group is to push the tolerable increase in total cancer cases up to 50% if these are equally distributed.
How do these trade-offs compare with those found in (analogous) studies of inequalities in income (or attitudes to risk more generally)? Cropper et al., include two further tests. One is a “leaky bucket experiment” whereby respondents are asked to choose between scenarios which involve (different sized) a USD 1 000 reduction in the incomes of the top 40% of income earners while simultaneously increasing the income of those in the bottom 40%. The size of the leak is indicated by how much less the latter is than the former: 0 < X £ 0. An option, for example, might involve reducing top earners’ income by USD 1 000 but raising the bottom earners’ incomes by USD 900 (in which case X = 100). Another option might only raise bottom earners’ incomes by USD 500 or USD 250 and so on. Again, respondents’ choices here can be used to infer preferences about income equality. These results appear to indicate that respondents are willing to sacrifice between 2 and 5% of average (mean) income to secure equality. As such, this study finds evidence that respondents seem to have stronger preferences for health equality than income equality.
Put another way, there is no reason to think that the weights to be used for changes in health risks are the same as those which might be used for changes in income more generally, at least as far as these U.S. respondents are concerned. Of course, determining appropriate weights is also a matter of social judgement rather than just the aggregation of these sort of individual responses. However, presumably such data will have a role in making that determination. Complications abound presumably, many projects involve health inequality and income inequality. For example, there seems to be some evidence that those particularly at risk from poor health as a result of exposure to air pollution are those with low socioeconomic status.
Dietz and Atkinson (2010) also use a stated preference study to look at the different distributional preferences of people towards policies which reduce air pollution. Respondents, from different areas of London in the United Kingdom, were asked to choose between options for a policy that differed in terms of conventional outcomes, such as its ambition i.e. how much London’s air quality would be reduced and its cost. In addition, some options included provisions for assisting those households with less income with the costs of paying for the policy (through taxation) and whether those who were most responsible for air pollution in London (e.g. motorists) should bear more of the burden of paying for policies which address this.
The results of that study suggest that respondents see a trade-off between distributional outcomes and costs: that is, on average, they are willing to pay more for policies which achieve a given improvement in air quality in a more equitable manner. For example, respondents were willing to pay GBP 153 more for a policy which led to a dramatic improvement in London’s air quality (relative to a policy leading to a modest improvement). If, however, this policy was targeted on those most responsible for the problem, these respondents would pay 64% more. If this policy additionally assisted those who were least able to pay, respondents were willing to pay 25% more.
The study by Dietz and Atkinson (2010) also surveyed a further sample of respondents about their distributional preferences for U.K. climate policy, thereby providing a test of how such preferences differ across environmental policy context. The “premium” on constructing a policy that is targeted on polluters is almost identical to that for the local air pollution context (65% compared with 63% above). However, respondents appear to care somewhat more for assisting those on lower incomes in the climate policy context (a “premium” of 43% compared with 25% as above).
11.4. Distributional CBA and climate change
A re-emergence of interest in distributional cost-benefit analysis (CBA) has been particularly prominent in the literature on the economics of climate change damage (see Chapter 14). This has involved a re-evaluation of the way in which the burdens of climate change damage are likely to be distributed between countries, which can be characterised as either rich or poor and vulnerable. As a result, equity weighting is now an established part of efforts to understand the social cost of carbon (SCC). Moreover, it appears that these procedures have influenced guidance on official (i.e. domestic government) positions on what the SCC should be for appraising climate change policy, although does not appear to be a universally held view.
As an illustration,
, where D is the value of global damage from climate change, Di is the damage suffered by country i and ai is the weight assigned to this damage in country i. The convention would be for these weights to be calculated as
, where YW and Yi is income (or consumption) per capita in the world and in country i respectively and η is a income (or consumption) inequality aversion parameter. Intuitively, if poorer countries suffer disproportionately from climate change damage, then this weighting should result in a higher social cost of carbon. The reality, however, is a little more complicated. A paper by Anthoff et al. (2009) explains why. One reason is that higher values of ε imply a higher discount rate: i.e.
, where r is the discount rate, r is the pure rate of time preference and g is the growth of per capita consumption. Not surprisingly, this technical issue has important practical ramifications.
Another important issue is highlighted by Anthoff and Tol (2010). Conventional weighting procedures are just one option. There are many others. At one extreme, a country may decide that people abroad have no standing. In terms of the implications for the SCC, this would mean that impacts elsewhere in other countries (i.e. climate change damage abroad) are not considered, when deciding on the SCC to use in appraising its climate policy. Of course, this is a rather extreme position but it provides at least a lower bound for further discussion. Once these impacts elsewhere are considered, there are a range of possible principles which could guide estimation of the SCC (some of which involve equity weighting).
From the perspective of a particular country, SCC can be expressed as follows:
The first term on the right hand side is the (discounted) value of damage, for the home country. The second term expresses concern for damage caused in other all countries. This latter term includes two weighting procedures. The first is ω and reflects whether or not any consideration at all is given to this damage occurring elsewhere. This can take a value of 0 or 1 (or somewhere in between, if consideration is a matter of extent). The second is an equity weight, which can take a value between (and including) 0 and 1.
Table 11.3 indicates a number of guiding principles (proposed by Anthoff and Tol) which the government within a country might use for estimating the SCC (and each will involve particular specifications of the above expression). The interpretation of each is indicated as well the implications for consideration of the well-being of people in other countries, whether (and what) equity weight to use, as well as the discount rate used to evaluate future damages in other countries. For values of a = 1, this means that damages abroad receive no greater (lesser) weight if a foreign country (f) is poorer (richer), in terms of income (or consumption) per capita (Y), than the home country (η).
Table 11.3. Distribution and the social cost of carbon – Principles and practice
SCC Principle |
Interpretation |
Weight attached to the well-being of citizens aboard |
Equity weight |
Discount rate |
---|---|---|---|---|
Co-operation |
A country behaves as a global decision-maker would: i.e. adopts the SCC that would maximise global welfare |
Yes: ω = 1 |
a = 1 |
|
Equity weighting |
Equity weighting that the global decision-maker would adopt on the basis on differences of income (or consumption) per capita between countries |
Yes: ω = 1 |
|
|
Sovereignty |
A country does not consider impacts elsewhere: damage to people abroad has “no standing” |
No: ω = 0 |
– |
– |
Altruism |
A country considers impacts elsewhere to the extent that its citizens care about those abroad |
Yes: depending on extent of altruism – i.e. 0 £ ω £ 1 |
|
|
Compensation |
A country considers it has a duty or requirement to compensate (nominally) damages it causes beyond its borders. Compensation refers to how this damage is valued by those in the victim countries |
Yes: ω = 1 |
a = 1 |
|
Good neighbour |
A country considers impacts elsewhere and cares about those abroad as it would do citizens in its domestic borders |
Yes: ω = 1 |
|
|
Source: Adapted from Anthoff and Tol (2011).
Table 11.3 also reports some of the findings for the United States in Anthoff and Tol (2010), assuming a rate of time preference of 1%. These are estimates of the dollar value of the social cost of tonne of carbon which a decision-maker in the United States might estimate, and use in CBA, as an analytical consequence of a particular distributional principle being adopted at the outset of an exercise to establish the SCC. The magnitudes of the results have important ramifications: that is, they provide an indication of the relative aggressiveness of emissions reduction. That is, higher values of SCC will imply more active climate mitigation policy in the home country.
Turning first to the initial column of results, for the case where an U.S. decision-maker simply adopts the SCC that maximises (unweighted) global well-being, this is equal to USD 16/tC. Equity weighting nearly doubles the magnitude of the SCC for the case where h = 1. Estimating SCC according to the sovereignty principle results in a value which is not much greater than zero (i.e. a few cents per tonne of carbon). Clearly being a “good neighbour” implies by far the highest SCC. It shares similarities with the altruism case with the exception of assuming that ω = 1 (rather than 0.1). Turning now to the sensitivity analysis for values of η equal to 0.5 and 1.5, perhaps paradoxically assuming higher (lower) levels of concern for inequality decreases (increases) the value of the equity weighted SCC in the case where η= 1. The main reason for this is that e also influences the discount rate (see Table 11.4).
Table 11.4. Estimates of the social cost of carbon for the United States
USD 1995, Time preference rate of 1%
Assumed value of |
|||
---|---|---|---|
1 |
0.5 |
1.5 |
|
Co-operation |
16 |
56 |
5 |
Equity weighting |
28 |
72 |
13 |
Sovereignty |
∼0 |
1 |
∼0 |
Altruism |
13 |
5 |
13 |
Compensation |
34 |
34 |
14 |
Good neighbour |
125 |
41 |
123 |
Source: Adapted from Anthoff and Tol (2010).
11.5. Concluding remarks
Conventional CBA for the most part continues to regard distributional or equity concerns as having little or no place in making recommendations about project selection and design. While this approach strikes some critics as an oddity, it would be a mistake to conclude that this downgrades the usefulness of CBA. Even if efficiency is only one piece of the puzzle in understanding the social worth of a project, it remains extremely important. Moreover, there are cogent reasons why cost-benefit analysts often take this approach to the appraisal of the costs and benefits of projects and policies. That is, it is not merely unmindful neglect (at least, not always). However, as has been noted in this chapter, each of the reasons supporting this assertion in favour of conventional CBA is contestable. This suggests greater scope for scrutinising the distributional consequences of projects within the cost-benefit framework.
Whatever the particular interpretation that is adopted, incorporating distributional concern implies initially identifying and then possibly weighting the costs and benefits of individuals and groups on the basis of differences in the characteristic of interest. A hierarchy (e.g. Kriström, 2005) is a useful way to understand the demands that a variety of proposals place on the cost-benefit analyst. First, there is the relatively straightforward but possibly arduous task of assembling organising raw (i.e. unadjusted) data on the distribution of project costs and benefits. Second, these data could then be used to ask what weight or distributional adjustment would need to placed on the net benefits (net costs) of a societal group of interest for a given project proposal to pass (fail) a distributional cost-benefit test. Third, explicit weights reflecting judgement about society’s preferences towards distributional concerns can be assigned and net benefits re-estimated on this basis.
A crucial question then is where should cost-benefit analysts locate themselves upon this hierarchy? Given that cost-benefit appraisals are sometimes criticised for ignoring distributional consequences altogether then the apparently simplest option of cataloguing how costs and benefits are distributed could offer valuable and additional insights. This suggests that, at a minimum, cost-benefit appraisals arguably should routinely provide these data. Whether more ambitious proposals should be adopted is a matter of deliberating about whether: (a) the gains in terms of being able to scrutinise the (weighted) net benefits of projects in the light of societal concerns about both efficiency and equity outweighs; (b) the losses arising from the need for informed guesswork in interpreting the empirical evidence with regards to the treatment of the latter.
On the one hand, empirical evidence about the “correct” magnitudes of distributional weights can be usefully employed in distributional CBA. On the other hand, even apparently small changes in assumptions about the size of distributional weights – indicated by the range of values in available empirical studies – can have significant implications for recommendations about a project’s social worth. This finding should not be a surprise for it primarily reflects the complexity involved in trying to disentangle society’s distributional preferences. As a practical matter, the danger is whether the most ambitious proposals for distributional CBA generate more heat than light.
Environmental CBA can play an important part here, notably through valuation practitioners paying greater attention to distributional concerns (e.g. of WTP or environmental impacts in physical terms). What might hasten that response? One catalyst almost certainly could be demand from policy makers. The suspicion must be that this is not only due to a supply problem (a manifestation of the singular emphasis of cost-benefit practitioners on efficiency), but it is also likely to be an issue about demand: i.e. policy makers have not required this information be provided in the terms of reference guiding that work. Addressing that element could have an important role to play.
References
Anthoff, D. and R. Tol (2010), “On international equity weights and national decision making on climate change”, Journal of Environmental Economics and Management, Vol. 60/1, pp. 14-20, http://dx.doi.org/10.1016/j.jeem.2010.04.002.
Anthoff, D., C. Hepburn and R. Tol (2009), “Equity weighting and the marginal damage costs of climate change”, Ecological Economics, Vol. 68/3, pp. 836-849, http://dx.doi.org/10.1016/j.ecolecon.2008.06.017.
Cowell, F. and K. Gardiner (1999), Welfare Weights. Report to the Office of Fair Trading, Office of Fair Trading, London.
Cropper, M., A. Krupnick and W. Raich (2016) “Preferences for Equality in Environmental Outcomes”, Resources for the Future, Washington, DC, www.rff.org/files/document/file/RFF-DP-16-36.pdf.
Day, B. and D. Maddison (2015), Improving Cost-Benefit Analysis Guidance, Report to the Natural Capital Committee (NCC), NCC, London, http://nebula.wsimg.com/ad8539d7216f5e6589bbe625c9750fff?Access KeyId=68F83A8E994328D64D3D&disposition=0&alloworigin=1.
Decancq, K., M. Fleurbaey and M. Schokkaert (2014), “Income, inequality and wellbeing”, CORE Discussion Paper No. 2014/18. University of Antwerp, https://cdn.uclouvain.be/public/Exports%20red dot/core/documents/coredp2014_18web.pdf.
Defra (2006), Air Quality and Social Deprivation in the UK: An environmental inequalities analysis, Final Report to Department of Environment, Food and Rural Affairs, Defra, London, https://uk-air.defra.gov.uk/assets/documents/reports/cat09/0701110944_AQinequalitiesFNL_AEAT_0506.pdf.
Dietz, S. and G. Atkinson (2010), “The equity-efficiency trade-off in environmental policy: Evidence from stated preferences”, Land Economics, Vol. 86/3, pp. 423-443, http://dx.doi.org/10.3368/le.86.3.423.
Gramlich, E.M. (1990), A Guide to Benefit-Cost Analysis, 2nd Edition, Prospect Heights, Waveland Press, Illinois.
Jodha, N.S. (1986), “Common Property Resources and the Rural Poor in Dry Regions of India”, Economic and Political Weekly, Vol. 21/27, pp. 1169-1181, www.jstor.org/stable/4375858.
Kriström, B. (2005), “Framework for assessing the distribution of financial effects of environmental policies”, in Serret, Y. and N. Johnstone (eds.) The Distribution of Benefits and Costs of Environmental Policies, Edward Elgar, Cheltenham, www.oecd.org/env/tools-evaluation/thedistributionaleffectsofenvironmental policy.htm.
Kanninen, B.J. and B. Kriström (1993), Welfare Benefit Estimation and Income Distribution, Beijer Discussion Paper Series No. 20, Beijer Institute of Ecological Economics, Stockholm.
Loomis, J. (2011), “Incorporating distributional issues into benefit-cost analysis: How, why and two empirical examples using non-market valuation”, Journal of Benefit-Cost Analysis, Vol. 2/1, Article 5, https://doi.org/10.2202/2152-2812.1044.
OECD (1975), The Polluter Pays Principle: Definition, Analysis and Implementation, Organisation for Economic Co-operation and Development, Paris, http://dx.doi.org/10.1787/9789264044845-en.
Pearce, D.W. (2003), “The social costs of carbon and its policy implications”, Oxford Review of Economic Policy, Vol. 16/3, pp. 362-384, https://doi.org/10.1093/oxrep/19.3.362.
Pearce, D.W. and D. Ulph (1999), “A social discount rate for the United Kingdom”, in Pearce, D.W. (ed.), Economics and Environment: Essays on Ecological Economics and Sustainable Development, Edward Elgar, Cheltenham.
Pearce, J. et al. (2011), “Environmental justice and health: A study of multiple deprivation and geographical inequalities in New Zealand”, Social Science and Medicine, Vol. 73, pp. 410-420, http://dx.doi.org/10.1016/j.socscimed.2011.05.039.
Persky, J. (2001), “Cost-benefit analysis and the classical creed”, Journal of Economic Perspectives, Vol. 15/4, pp. 199-210, http://dx.doi.org/10.1257/jep.15.4.199.
Riberio, A.I., M. de Pina and R. Mitchell (2015), “Development of a measure of multiple physical deprivation. After United Kingdom and New Zealand, Portugal”, European Journal of Public Health, Vol. 25/4, pp. 610-617, https://doi.org/10.1093/eurpub/cku242.
ten Brink, P. (ed.) (2011), The Economics of Ecosystems and Biodiversity in National and International Policy Making, Earthscan, London.
Vedeld, P. et al. (2004), “Counting on the environment: Forest incomes and the rural poor”, Environmental Economics Series No. 98, The World Bank, Washington, DC, http://documents.worldbank.org/curated/en/825651468778804896/Counting-on-the-environment-forest-incomes-and-the-rural-poor.
annex 11.A1. A marginal utility of income weighting procedure
Let utility be related to income, i.e. U = U(Y), such that the marginal utility of income function has a constant elasticity. The marginal utility of income function for individual i can then be written:
where –e is now the elasticity of the function. For the average income
we shall therefore have
and the relative weight for the ith individual would then be
Notes
← 1. Although these firms are in turn owned by households.
← 2. Explanations of why this is the case typically have used Arthur Okun’s analogy of the leaky bucket used to equalise the water volumes in two receptacles. Assuming that the distribution of water between the two receptacles is unequal in the first instance, the transfer, via a leaky bucket, inevitably leads to an overall loss of water in pursuit of the goal of a more equal distribution. This is the essence of society’s problem: how much efficiency should be traded-off for more equity? For example, in the case of taxation of incomes, the leaky bucket represents incentives affecting the work-leisure choice. That is, ever higher marginal tax rates discourage high-income earners from working more and thereby decreases, in some degree, the total amount of income that society has available to redistribute.