As the world goes more digital in the manner in which it functions, regulatory delivery also needs to be digitally enabled to better its effectiveness and efficiency. This chapter sets out the need for infusing data-driven systems towards conventional risk-based approaches. The first part explains risk, its various components and the inherent limitations in the current system. The second part introduces real-time applications of digital tools such as predictive Artificial Intelligence and Machine Learning in three OECD projects in progress in Italy.
Data-Driven, Information-Enabled Regulatory Delivery
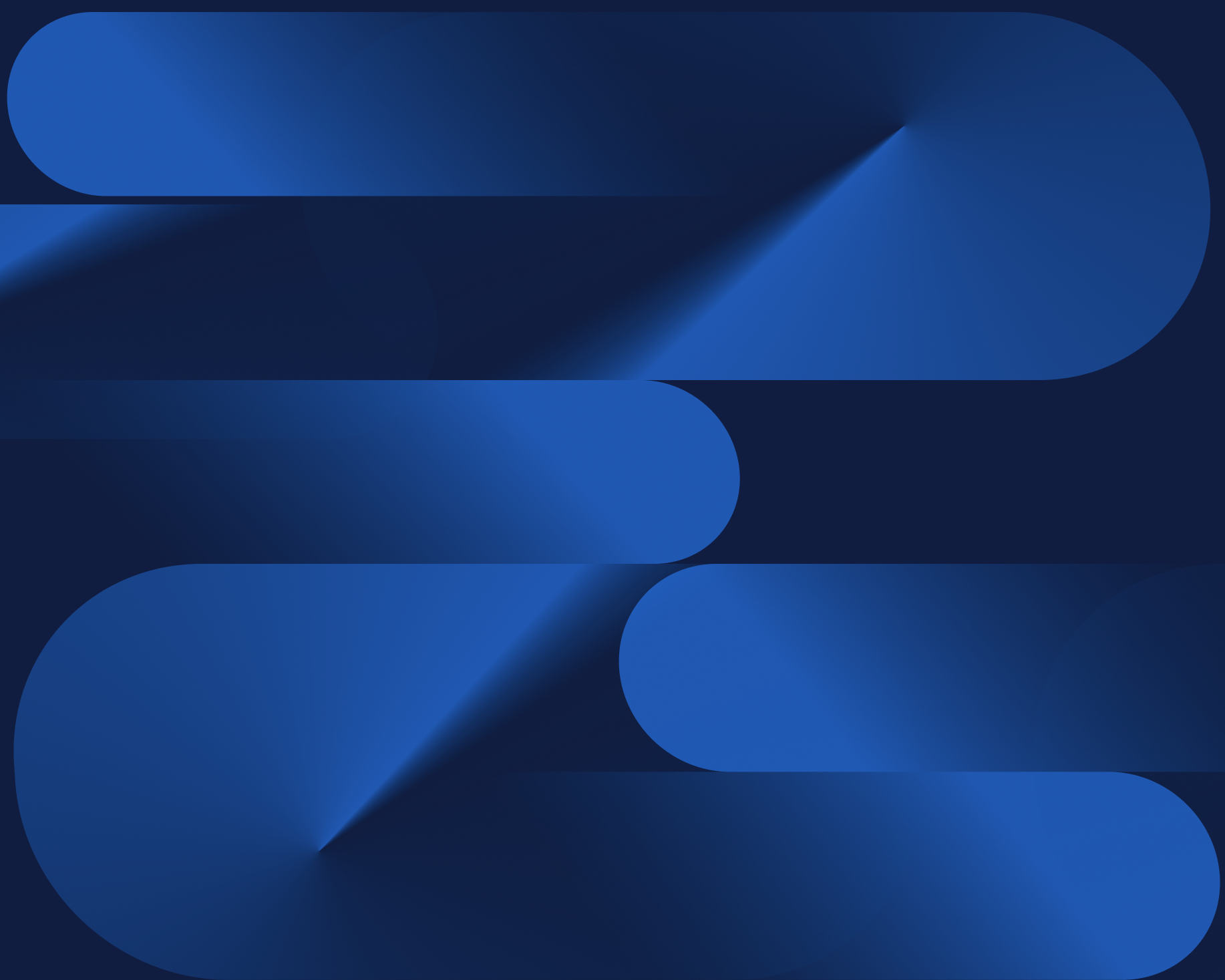
1. Data driven approaches towards risk-based regulatory delivery
Abstract
Regulatory resources are inherently limited, compared to the scope of economic activities they are set to supervise. Economic growth and technology-driven transformations, combined with budget constraints, as well as significantly higher public expectations of regulatory protection from various risks (Blanc, 2015[1]), have made this mismatch increasingly important. Regulatory “delivery” (supervision, inspections, enforcement) is thus faced with major resources and effectiveness challenges – but it also can represent an important burden for economic actors (and, sometimes, create real barriers to growth) (Blanc, 2018[2]). Making it more efficient is thus a key priority, and risk-based approaches to targeting of inspections, as well as risk-proportional enforcement, have long been put forward as critical for such improvements (OECD, 2014[3]). Full effective implementation of risk-based inspections has at times been limited by a number of factors, including challenges in data availability and management, both to identify risk factors and assess risks of specific operators and establishments (OECD, 2018[4]). Technological improvements and increased use of data management tools in regulatory services present, however, considerable opportunities for improved implementation of risk-based regulatory delivery (OECD, 2015[5]). A Data Driven Public Sector (DDPS) achieved through data governance can help policy makers become more efficient and transparent. Alongside this, the use of data by public authorities, to plan, deliver and evaluate policy activities, should add to public value while at the same time, help foster institutional trust through privacy and transparency, in the minds of the public (OECD, 2019[6]). In the recent past, risk-based regulation of infrastructure projects using data analytics, have helped in identifying a data value chain and its common components for improving data analytics. These include effective institutional and data governance, data integrity and project planning (OECD, 2019[7]). This note presents several cases that illustrate the development of such initiatives and which could also provide inspiration for new ones.
Risk-based regulation (OECD, 2010[8]), including risk-based regulatory delivery, involves understanding the specifics of each risk (risk analysis) allows to better determine the most appropriate tools, assessing the level of risk (risk assessment) allows to select the most appropriate intensity of regulatory response (stringency of requirements, and delivery processes and resources). Studying risk, its probability and scope, allows to better formulate what it is that a given regulation is trying to address (reducing or managing a risk). Risk management is important to ensure the optimal functioning of the public authority and safeguarding of its integrity objectives and preventing harms (OECD, 2020[9]).1 Understanding risk, its causes and characteristics, helps to better design the contents and mechanisms of the regulation and to target enforcement and implementation efforts more efficiently (on the areas, sectors, businesses etc. that pose the highest risk). It is defined as the combination of the likelihood and potential magnitude and severity of harm. This can also be expressed as the combination of the likelihood and degree of hazard.
Thus, risk combines a) probability, b) scope of the harm (number of people affected etc.) and (c) degree of harm (type of damage). Harm is any form of damage done to people (their life, health, property etc.), the environment (natural and human), or other public interests (e.g., tax fraud harms state revenue). Not all types of harm are of the same nature. Some harms are irreversible (e.g., death), whereas others (e.g., financial) can be corrected once identified. Unpredictability and uncertainty are distinct from risk and from estimations of probability of harm. They are inherent limitations in the process of risk assessment and thus likewise limitations of risk-based regulation, that should be acknowledged as such. Generally, risk criteria in most regulatory domains have typically been defined based on expert assessment (Blanc, 2012[10]) – with only revenue services having sufficient data to determine them based on quantitative analysis (Khwaja, Awasthi and Loeprick, 2011[11]). Collection and management of up-to-date and adequate information on supervised businesses was also an issue, even though good practices have been known for some time (Wille, 2013[12]).
Improvements in information technology (processing power and costs, Machine Learning methods, etc.), combined with the increasing number of regulatory services using digital information systems to record inspection results (thus providing crucial historical data), have combined to change the context, and create new opportunities to improve regulatory delivery (Mangalam, 2020[13]). From a regulatory perspective, data analytics is also proving to be increasingly cross-functional. It has both vertical and horizontal applications with the same data being used to pursue different regulatory objectives (OECD, 2019[7]). Data analytics planning to effectively assess risk objectives- including identification of data needs and sources, selection and understanding of data and the design and analysis of data itself have become far more streamlined. Building on previous work assessing existing practices and documenting improvements and reforms (OECD, 2020[14]), the OECD Secretariat has been involved in several initiatives to further research and support the use of information technology to make inspection systems more genuinely risk-based. Most of this work has taken place in Italy, at the regional level, as part of a project requested by the Public Service Department of the Italian Government, and funded by the European Commission – DG REFORM. This note presents the main findings and lessons from this work.
The project, called “Rating Audit Control” (RAC), aims at making regulatory inspections more targeted and risk-based, and works primarily at the regional level, where most technical and safety inspections are managed in Italy, with an important information technology and data analysis component. The activities support different regions and regulatory services in improving inspection planning by making better use of existing data and systems and, where possible, improving these systems in terms of risk integration. The project works in four regions – Campania, Lombardy, Trentino, and Friuli-Venezia-Giulia. However, bulk of the IT and data-related work has so far been conducted in the former three. This note presents the main activities conducted in these three regions- the application of Machine Learning techniques to improve the risk criteria used to target occupational safety inspections on Lombardy’s construction sites (through the Site Risk Monitoring System, Mo.Ri.Ca.); the development of a risk-assessment “engine” for the Autonomous Province of Trento’s Single Register of Provincial Controls on Businesses (RUCP); and work to improve the risk-assessment function of Campania’s Integrated System for the Cooperation and Management of Official Controls (GISA), used for food safety inspections in the region.
Autonomous Province of Trento: the project has been supporting the further development of the new inspections’ management system, called Single Register of Provincial Controls on Businesses (RUCP), which has been under development for several years, but still covers only a limited number of inspection services. The focus has been on developing a “RAC Engine”, relying on a set of algorithms (each specific to a given type of inspection) to allow the RUCP to produce a risk-based rating for each business or establishment, enabling inspectorates to select inspection targets based on their risk level.
Lombardy: the assessment of existing data and information systems in Occupational Safety and Health (OSH) and food safety services revealed significant opportunities to apply Machine Learning techniques to improve risk prediction and thus inspections targeting. The initial focus was on OSH services, which use the Site Risk Monitoring System (Mo.Ri.Ca. in Italian) to conduct risk assessment for the planning of inspections of building sites. The work is gradually being expanded to food safety (assessment of risk in sites producing food of animal origin).
Campania: the region has been a kind of “trailblazer” within Italy with the development of the Integrated System for the Cooperation and Management of Official Controls (GISA, in Italian), which has been in place for nearly 10 years and support co-ordination, risk-based planning and management of records for food safety inspections in the region. The project has worked to support the further development of risk criteria to improve the efficiency and effectiveness of targeting, and Machine Learning work on historical data is planned for the next phase.
References
[2] Blanc, F. (2018), From Chasing Violations to Managing Risks, E. Elgar.
[1] Blanc, F. (2015), Understanding and addressing the Risk Regulation Reflex - Lessons from international experience in dealing with risk, responsibility, and regulation, https://www.government.nl/binaries/government/documents/reports/2015/01/21/understanding-and-addressing-the-risk-regulation-reflex/understanding-and-addressing-the-risk-regulation-reflex-v2.pdf.
[10] Blanc, F. (2012), Inspections Reforms: Why, How and with What Results?, OECD.
[11] Khwaja, M., R. Awasthi and J. Loeprick (eds.) (2011), Risk-Based Tax Audits, The World Bank, http://dx.doi.org/10.1596/978-0-8213-8754-2.
[13] Mangalam, S. (2020), Use of New Technologies in Regulatory Delivery, https://www.enterprise-development.org/wp-content/uploads/DCED-BEWG-Use-of-New-Technologies-in-Regulatory-Delivery.pdf.
[9] OECD (2020), OECD Public Integrity Handbook, OECD Publishing, Paris, https://dx.doi.org/10.1787/ac8ed8e8-en.
[14] OECD (2020), Regulatory Enforcement and Inspections in the Environmental Sector of Peru, OECD Publishing, Paris, https://dx.doi.org/10.1787/54253639-en.
[7] OECD (2019), Analytics for Integrity. Data-Driven Approaches for Enhancing Corruption and Fraud Risk Assessments, https://www.oecd.org/gov/ethics/analytics-for-integrity.pdf.
[6] OECD (2019), The Path to Becoming a Data-Driven Public Sector, OECD Digital Government Studies, OECD Publishing, Paris, https://dx.doi.org/10.1787/059814a7-en.
[4] OECD (2018), OECD Regulatory Enforcement and Inspections Toolkit, OECD Publishing, Paris, https://dx.doi.org/10.1787/9789264303959-en.
[5] OECD (2015), Regulatory Policy in Lithuania: Focusing on the Delivery Side, OECD Reviews of Regulatory Reform, OECD Publishing, Paris, https://dx.doi.org/10.1787/9789264239340-en.
[3] OECD (2014), Regulatory Enforcement and Inspections, OECD Best Practice Principles for Regulatory Policy, OECD Publishing, Paris, https://dx.doi.org/10.1787/9789264208117-en.
[8] OECD (2010), Risk and Regulatory Policy: Improving the Governance of Risk, OECD Reviews of Regulatory Reform, OECD Publishing, Paris, https://dx.doi.org/10.1787/9789264082939-en.
[12] Wille, J. (2013), Implementing a Shared Inspection Management System - Insights from recent international experience, http://documents1.worldbank.org/curated/en/190851468152706158/pdf/907550BRI0Box30ment0System0apr02013.pdf.
Note
← 1. See specifically Chapter 10, https://www.oecd-ilibrary.org/sites/ebbed075-en/index.html?itemId=/content/component/ebbed075-en.