This chapter details the manner in which IT tools have been used to manage occupational safety and health inspections in construction sites in Lombardy as well as food safety inspections in the Campania region. Brief mention is also made to other OECD projects involving predictive systems for assessing water quality and self-certification tools. These projects illustrate the moving away from the systematic use of data in revenue-based regulatory areas to other domains where data has been historically difficult to manage. The chapter adds the following key observations: i) Machine Learning can help predict indicators of risk; ii) Artificial Intelligence systems can help improve enforcement performance through quantitative analysis of inspections, and; iii) conventional assumptions of the best predictor of non-compliance are being challenged through Artificial Intelligence systems.
Data-Driven, Information-Enabled Regulatory Delivery
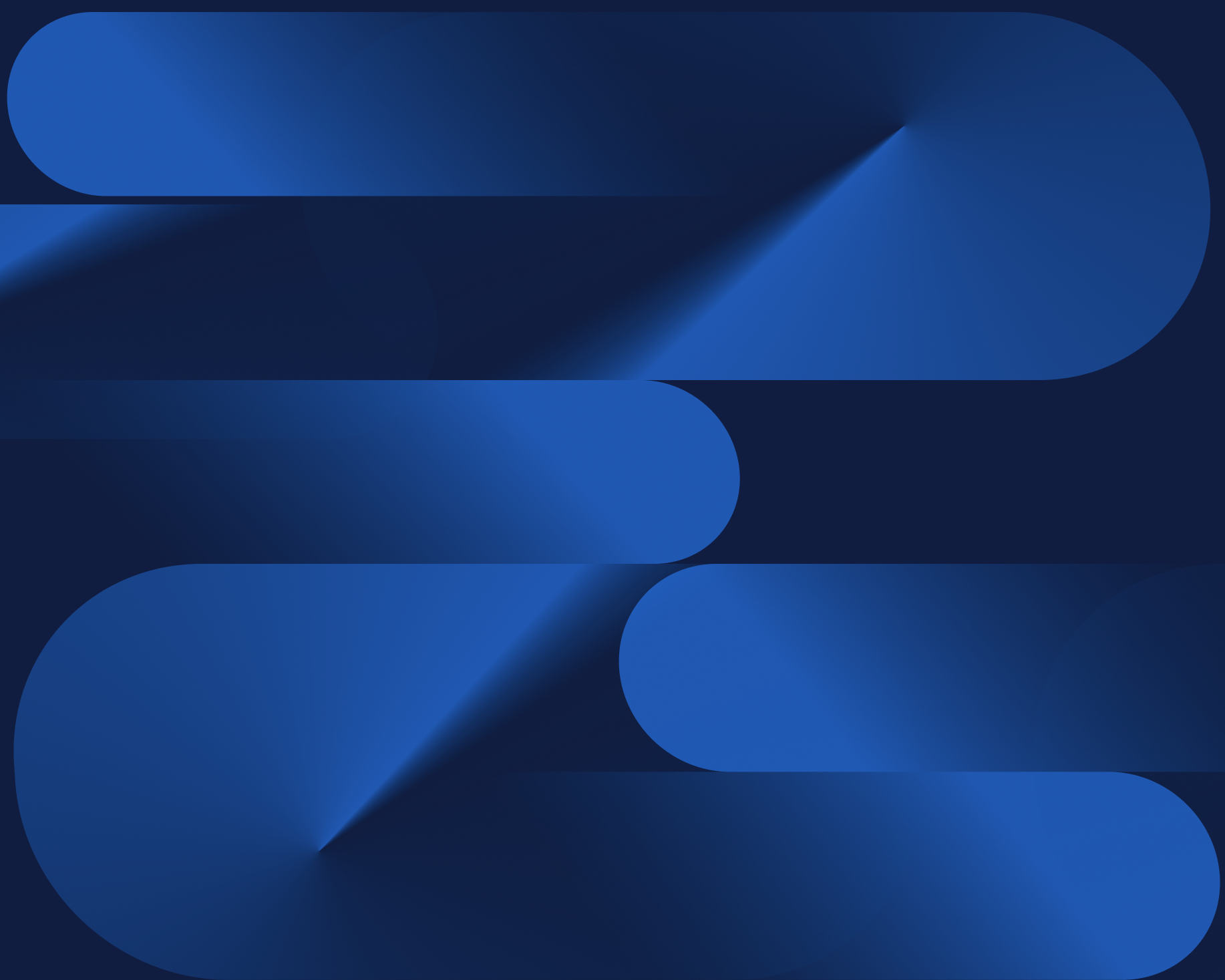
2. Applying machine learning techniques to inspections
Abstract
A key challenge in the implementation of risk-based approaches (and particularly for regulatory inspections and enforcement, for which risk is an essential instrument) is to adequately determine the factors that lead to increased risk and assess their relative importance. While revenue agencies had long started to systematically use data analysis techniques to identify risk indicators and their relative importance, this had until recently been difficult to replicate for non-revenue inspections. Data was either insufficiently digitalised, or too complex, or sometimes too narrow. Historical records were also insufficient, as new systems had been introduced too recently. In some cases, data systems with historical inspection records existed from earlier, but their scope was narrow and insufficient to identify risk indicators. Specific staff competences and capacity were also often missing. Despite these, an increasing understanding of risk-based regulation and of the importance of accurate risk-assessment (as opposed to reliance on “traditional” assessments of where priorities lay) have opened the way for a more systematic, data-driven approach. Despite remaining challenges in terms of assessing the “severity” dimension of risk, recent Machine Learning (ML) applications are very promising in terms of significantly improving the understanding of which characteristics of businesses and establishments are the best predictors of risk, and thus considerably improve the effectiveness of risk-based targeting.
While defining risk abstractly is relatively straightforward, developing robust methods to predict the level of risk of different businesses or establishments is far more difficult. Until recently, challenges in data availability and methods for analysis meant that defining risk criteria and their relative weights based on “data mining” or similar mathematical approaches was mostly reserved to tax and customs inspections, audit, infrastructure, health and social welfare programs (where the objects of regulation and control are inherently numerical, and computerisation was done earliest and in the most systematic way). In technical regulation, food safety, occupational safety, environmental protection etc., risk identification and weighting were done through a combination of scientific and technical findings, regulators’ experience and “trial-and-error”, but in a much less systematic and precise way.
The Mo.Ri.Ca. system for Management of OSH Inspections of Construction Sites in Lombardy
Lombardy regional OSH services use the Site Risk Monitoring System (Italian acronym Mo.Ri.Ca.) to conduct risk assessment for the planning of inspections of building sites. The system was developed as part of the National Prevention Plan for construction 2008-2010. Mo.Ri.Ca incorporates data from the regional enterprises database and data of the notification system for construction sites. Through a simple algorithm, it identifies the sites at greatest risk, based on a set of criteria and weights that were defined by the OSH services using the experience and knowledge of inspectors, fundamental physical safety elements, and assumptions about the impact on likelihood of compliance of different enterprise and site characteristics.
Given that construction is a particularly high-risk sector (as evidenced by the prevalence of occupational accidents, particularly fatal ones), and that supervision resources are inherently limited, selecting which construction sites to visit with the highest possible precision is particularly important. In particular, the criteria best predicting non-compliance, and their relative weight, need to be accurate. So far, these were based on a certain number of assumptions about the profile of construction operators, and thresholds for different risk categories were likewise set based on “common sense” discussions among inspectors – as is the case, globally, in most inspection services using risk-based approaches (which is already far superior to most services which use no risk-based planning whatsoever). At the same time, Mo.Ri.Ca. hosts results from past inspections since its start of operation, and allows to conduct several analyses, containing as it does detailed data on operators, sites (including geolocation), etc. The intent of the pilot was thus to analyse Mo.Ri.Ca’s. historical data using Machine Learning and determine the validity of the existing risk scorecard, test the predicting power of different criteria, and the appropriate thresholds.
GISA and other tools in Campania region
The Campania region has developed several IT tools aimed at supporting its enforcement system on food safety. Its initiatives have led the region to be one of the leading ones in Italy to develop good practices in technology meant to improve the regulatory delivery. The OECD, through the RAC Project, has had the opportunity to provide technical support to the most recent initiative – an online self-certification – , described at the end of this section.
The oldest tool developed in Campania is called GISA, System for the Cooperation and Management of Official Controls, created by the Region in 2007 with the support of the Higher Zoo prophylactic Institute for Southern Italy (IZSM) to support and facilitate ordinary and extraordinary activities related to food safety and public health veterinary services. New elements are constantly being implemented to allow more effective planning inspections and greater data collection and processing.
The system aims at facilitating inspections planning, preventing duplications, and promoting co-ordination between different information services and authorities involved in control activities. It involves different type of stakeholders. Local health authorities (ASL in Italian), which are inter alia in charge of food safety and occupational safety inspections, as well regulatory enforcement agencies involved in food safety controls different from ASL, municipal and private veterinary health and public hygiene services, universities, business associations, commons, etc., can consult and benefit from GISA and contribute to it by providing data needed for inspections.
As an integrated system, GISA has both operational and strategic functionalities that permit better inspection planning from a deontological point of view – i.e., greater health protection, and from a management perspective – i.e., using of resources. On the first aspect, GISA allows to identify different levels of risk, targeting Food Businesses Operators (FBOs) and accordingly selects proportional intervention measures. It collects, contains, and updates an FBOs census, and processes, in real time, data related to food safety inspections. Main information in this regard includes, FBOs’ geographical location, risk parameters used to plan inspections, checklists, and inspections results over time. Once controls are held, the system collects information from reports and checklists, and by applying ML techniques, monitors FBOs.
From the operational perspective, GISA contributes in improving enforcement performance by providing quantitative analysis of inspections. Given quantitative and qualitative data regarding public resources and inspections means, GISA processes inspectors’ performance and delivers information about optimal distribution of public resources. To define their use, the system considers geographical risk distribution and risk-based targeting of FBOs. Additional information relates to volume, types, and value of sanction. Given this information, and provided data-analysis engines, GISA can define an accurate risk-based rating of FBOs, a distribution of geographically critical areas to be inspected, an accurate geographic and risk-based distribution of public resources; and defines risk-based inspections frequency.
These functions are available to all inspectorates involved in the process, including the Police Forces. Each year the Region draws up a planning document, which is uploaded onto the system, indicating the inspection activities to be carried out during the following year. Inspections are subdivided by objectives considering high-risk targeted FBOs and geographical critical points. This way, the system automatically distributes the load of planned inspections among the various structures in charge and monitor objectives achievement.
Aside from GISA, the Campanian region partnered with the University of Naples Parthenope to develop MytiluSE, a system to predict the quality of waters to secure safety of mussels produced in the bay of Naples. The system works pre-emptively, enabling to know which days the harvesting of mussels would be unsafe. Rather than expending resources on ex post controls to find potential contamination, the system informs producers and guide inspectors’ work by mapping contamination sources and developing a reliable predictive model. This predictive approach for mussels avoids health hazards far more effectively than inspections because microbiological testing and sampling takes time, and results may come too late (leading to potential contaminations from other products harvested the same day) (OECD, 2021, forthcoming[1]).
In addition to these instruments, the Campanian region, with the technical support of the OECD, is implementing a self-certification tool. According to the Regulation EC 852/2004, FBOs are obliged to implement food safety systems, based on pre-requisite programmes and HACCP, and to perform periodical self-control of the appropriateness and effectiveness of such systems. To provide an audit tool for self-control, the electronic form of the checklist for dairy processing facilities was prepared and will be tested during 2021. It will be accessible on the official regional veterinary inspection website where FBOs will be able to fill it and submit it to the Veterinary inspectorate. By performing thorough periodical control, FBOs will be able to identify gaps in their systems and rectify them on time. During official control, inspectors will be able to compare the results of self-control to their own findings and understand better the level of compliance of FBOs as well as food safety culture in the facility. Repeated accordance of self-control results and that of official control will indicate good understanding or food safety requirements by businesses, the parameter used for determining the frequency of official inspections. Another benefit of this kind of self-control is in situations when physical inspection is not possible (as it is the case now due to Covid-19 restrictions). Information from filled self-check lists will help inspectors have an insight into the FBOs compliance to regulatory requirements.
Key findings
On ML applied to OHS, the analysis covered 5 of the 8 provincial subdivisions of the Lombard system. The data sources were i) the notification of the start of construction and ii) the history of controls on construction companies and “safety supervisors”, which are operators specifically entrusted within the Italian construction system with ensuring the safety of complex construction sites. The research looked at the ability, or lack thereof, between different characteristics of the construction operators (building companies and safety supervisors), including their historical inspection records, and of characteristics of the construction sites themselves (monetary value etc.) to predict non-compliance. In particular, the research aimed at testing whether the criteria currently included in the Mo.Ri.Ca. scorecard were good predictors of non-compliance (and in the expected direction of correlation) or not, and whether the thresholds currently used for different classes were adequate or not. For example, in the score-card, the risk indicator “value of construction works” is set with a different score for different ranges of value (less than 10K EUR, 10 to 50K, 50 to 100K, and above 100K), and the research would test not only whether construction value was a predictor, but also in which direction (higher likelihood of non-compliance for higher values, or the opposite?), and at which value level(s) could a significant difference be observed. Some aspects of work typology, which are used to determine the potential gravity of non-compliances, were not tested in this first phase of the research but will be investigated in a later stage looking at the impact side of risk.
Interestingly, the research found quite diverging results for different indicators. Some of those expected to predict non-compliance were in fact essentially neutral, or very weak predictors. Others were indeed correlated with non-compliance, but in the opposite direction of what the score-card model had expected. Others again were adequate predictors, but the thresholds chosen in the scorecard were inadequate (i.e., did not correspond to inflexion points in the non-compliance rate curve). Finally, some were good predictors and in the correct direction, but the research was able to suggest better ways to formulate and measure them. We provide a couple of examples below for illustration purposes.
The total value of the construction site was found to be a very weak predictor of non-compliance, with non‑compliance varying in a somewhat random way in function of the monetary value – and thus does not appear to be a very usable or useful indicator for risk-based targeting (see Figure 2.1)
Figure 2.1. Variation of the construction-value predictor

The number of sites supervised by one and the same safety supervisor, which was expected to be a predictor of non-compliance by the scorecard (the assumption being that supervising too many sites would lead to lack of attention to each), was in fact found to work in reverse. Having a safety supervisor in charge of only one or very few sites was found to be a very strong predictor of non-compliance (possibly this would correspond to people or companies with little experience or a bad reputation) – whereas above a certain threshold the curve is close to flat (i.e., the threshold effect is very strong, and there are just two categories).
Figure 2.2. Record of non-compliance

The previous record of compliance or non-compliance, on the other hand, proved to be a strong predictor in general – but different ways of formulating and measuring this track record yielded more-or-less strong results. Simply looking at the result of the last inspection was not the most meaningful indicator. Considering the average of non-compliance vs. compliance findings across all previous inspections of one company gave much better results, but with some “instability” in the overall trend (curve with two inflexion points, i.e., at one point the indicator was functioning “in reverse”).
Figure 2.3. Non-compliance trend

The best predictor was found to be the trend of (non-)compliance, i.e.
Figure 2.4. Non-compliance historical ratio

Reference
[1] OECD (2021, forthcoming), OECD Regulatory Policy Outlook 2021, OECD Publishing, Paris.