A quantitative bio-economic farm model was developed to assess the cost-effectiveness of key GHG mitigation policy instruments to reduce emissions from crop and livestock production. Six policy instruments are examined and applied to farm cases in the European Union. The results show high abatement costs in mixed dairy and crop production when aiming for large GHG emission reductions and confirm that market-based policy instruments (GHG emission tax, GHG abatement subsidy, and cap-and-trade scheme) are the most cost-effective policy options. The results also show that policy instruments that target all GHG emissions from farms are more cost-effective than those that target only a subset of emissions or proxies of emissions, even when higher transaction costs of those policy instruments targeting all GHG emissions are accounted for.
Enhancing Climate Change Mitigation through Agriculture
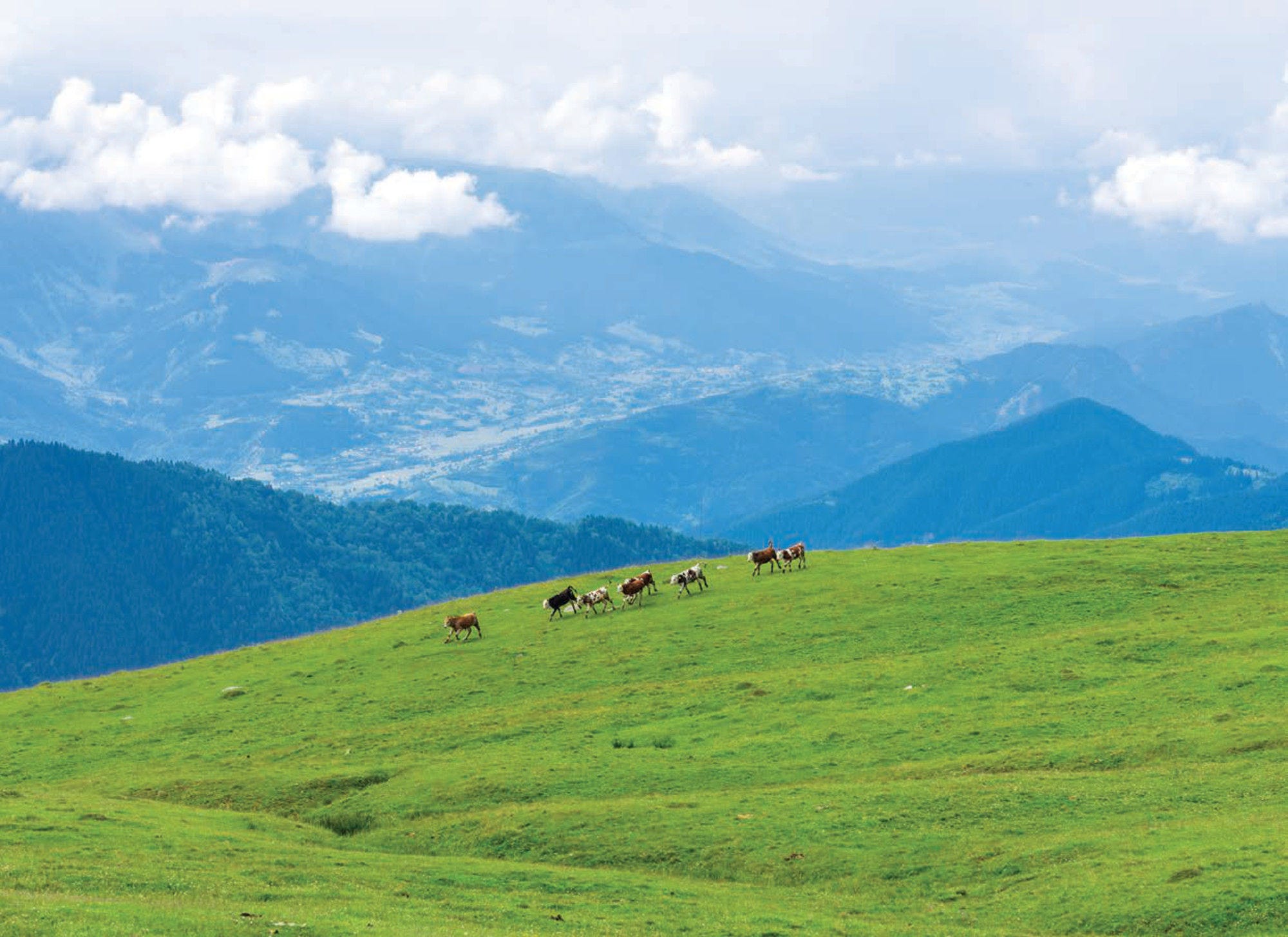
3. Farm-level analysis of mitigation policies for agriculture
Abstract
Introduction
Many greenhouse gas (GHG) mitigation options are readily available to the agriculture sector, including reducing nitrogen fertiliser use, adopting reduced or no tillage methods, conversion of arable land to grassland, and changing livestock diet (MacLeod et al., 2015[1]). Few of these options can be considered win-win, i.e. increasing farm profits while reducing GHG emissions, so their adoption often requires policy instruments or markets that incentivise and accelerate their uptake.
Most GHG mitigation studies in the agriculture sector focus on ranking the cost-effectiveness of technical mitigation options and deriving marginal abatement cost curves with various methodologies, including bottom-up cost engineering, micro-economic modelling with exogenous prices, and equilibrium models with endogenous prices (MacLeod et al., 2015[1]).
This chapter focuses on the question of mitigation policy design with the objective to assess the relative mitigation effectiveness and cost effectiveness of key GHG mitigation policy instruments to reduce emissions from crop and livestock production. It analyses six policy instruments: an emission constraint; an emission tax; an abatement subsidy; an input tax on nitrogen fertiliser; an input tax on ruminant heads; and emissions trading. These are applied to farm cases in the European Union. To do this, a model covering crop and livestock production activities was developed and applied to farms representing a diversity of situations at the regional-level.
A bio-economic framework for a mixed farming system made up of crop and dairy production was developed and implemented as a detailed bio-economic optimization model for arable-dairy farms with non-linear crop and milk yield functions, and a detailed accounting of GHG emissions parameterised to four regional production systems. In addition to adjusting the crop land allocation, herd size, feed mix, and mineral fertiliser and manure application levels, the model incorporates technological changes regarding manure storage (from non-covered to covered manure storage) and manure spreading (from broadcast spreading to injection) as GHG abatement options. Manure nitrogen excretion response to dietary changes is also modelled.
A bio-economic framework for dairy and crop production
Overview of the bio-economic framework
The bio-economic framework accounts for the interactions between decisions on livestock (milk production) and crop choices associated with on-farm fodder production, manure use as a source of nutrients, and competition for quasi-fixed resources such as land and labour between crop and dairy production. The model baseline depicts interrelated, profit-maximising choices of herd size, milk and crop yields, diet, fertilization, and land allocation between grass silage and crop production under current market and policy conditions. Under different greenhouse gas (GHG) mitigation policy instruments, the farmer adjusts decision variables to reach new profit-maximising levels.
For dairy production, the impact of diet composition on milk yield, manure excretion, and manure composition is modelled. Increased intake of concentrates increases milk yield per cow at decreasing rates up to a maximal yield level, while the intake of fodder decreases in parallel. Fodder sources include grass silage that is produced on-farm and grazing. The replacement of animals for the milk herd is based on heifers raised on the farm. The number of lactations is modelled as a function of the milk yield. All other revenues and costs are expressed per dairy cow.
Fodder and other crops compete for arable land. Their yields depend on the applied fertiliser. Mineral fertiliser and manure – reflecting plant available nutrients – are assumed to be perfect substitutes in the relevant simulation range. The marginal crop yield response decreases with increasing fertiliser application rates, up to a maximal crop yield. All activities compete for farm labour, which can alternatively be employed off-farm at a given reservation wage. As grass silage is not marketed, its costs reflect production costs, opportunity costs of labour, and land on the one hand, and the substitution value against feed concentrates on the other. Similarly, the value of manure reflects differences in application costs relative to mineral fertiliser and the content of plant-available nutrients.
Various decision variables affect GHG emissions. Different types of policy instruments can be modelled (emission constraint, emission tax, abatement subsidy, input and output taxes, and carbon trading).
A non-linear programming approach was used to simulate the optimal decision making of a farmer (Figure 3.1) under different endowments and technology, as well as different market and policy environments. The farmer manages three fixed endowments, indicated by the black-outlined boxes: grasslands, arable lands, and family labour. The latter can be used on- or off-farm. The interdependent and simultaneously determined decision variables in the comparative-static framework are the cowherd and acreages, crop and milk yields, mineral and organic fertilization levels, and the feed mix. The costs of mineral fertiliser and concentrates are explicitly included; other costs are summarised. Revenues stem from selling milk and arable crops. Costs for animal replacement and revenues from selling old cows are accounted for as well. Besides grassland and silage, the model includes the arable crops wheat, barley and rape. Input and output prices are considered exogenous.
Figure 3.1. Main interactions in bio-economic modelling framework
Crop yields are endogenously depicted by nitrogen dependent yield functions of either the Mitcherlich or quadratic functional form. The different crops including grass silage compete for arable land, while pastureland per cow is fixed. The model maximizes either profits or utility when production risks for crops are considered. Compared to other bio-economic models, this model differentiates itself by using non-linear crop and milk yield functions and by its endogenous use of IPCC Tier 3 emission accounting.1
While a vast body of literature analyses the interaction between yields, fertilization and climate-change-relevant emissions based on biophysical models (Britz and Leip, 2009[2]), application of non-linear yield functions in more complex farm-scale bio-economic models is still scarce. For example, Lengers et al. (2013[3]) use a purely linear model in their analysis, which includes similar abatement options as here, but does not consider yield response or non-linear substitution between concentrates and fodder, focusing on different GHG emission indicators. Similarly, De Cara et al. (2005[4]) use more aggregate linear single farm models in their European-wide analysis, however fixing crop and milk yields.
The following GHG emissions are accounted for:
methane emissions from enteric fermentation and from manure storage,
direct N2O emissions from manure storage
indirect N2O emissions from manure storage and spreading (NH3 emissions from manure storage and spreading cause indirect N2O emissions)
GHG emissions from cultivated land including nitrogen fertiliser use and autonomous soil emissions
emissions from cultivation practices, crop yield transportation and grain drying. Furthermore, soil carbon sequestration is taken into account when arable land is put under green set-aside.
Methane emissions from enteric fermentation per cow reflect milk yields and the digestibility of the cow’s diet. Methane and nitrous oxide emissions from manure storage depend on feeding and manure storage technologies (e.g. uncovered manure storage and manure storage with a floating cover). Feeding practices only impact emissions from uncovered manure storage, while a floating manure storage cover decreases emissions by about 30%, independent of concentrate feeding levels.
Annexes 3.A and 3.B detail all equations and parameters.
Data and model calibration
The model application draws on the Common Agricultural Policy Regional Impact (CAPRI) database.2, 3, 4 In order to derive stylised regional cases for this bio-economic model, data encompassing 23 European countries were taken from the CAPRI database for the year 2012. These data relate to, for example, regional crop acreage and dairy cow numbers, crop and milk yields, application of nitrogen and phosphorus in chemical fertiliser and manure, value of outputs and production inputs, various GHG emissions and total global warming potential of crop and milk production, ammonia emissions, and feed inputs.
To apply the model, four representative farms combining dairy and crop production were parameterised to illustrate the impact of productivity differences in both crop and milk production. The following data from the CAPRI database were used to calibrate these four representative farms: crop yields, milk yields, crop-specific production costs, milk-specific production costs, mineral and organic nitrogen application, dairy cow diet (the amount of feed cereals and protein) and output value for crops and milk. Based on these data, crop and milk yield functions for each farm were calibrated so that yield levels corresponded to input use given in the CAPRI database. In addition, production costs were calibrated based on the CAPRI database for each farm.
All four-farm cases were represented through a standard farm layout: a dairy and crop production farm with 60 hectares of arable land and up to 40 hectares of pastureland, reflecting the EU-15 average of about 60 dairy cows. Farm A represents a high milk and low crop yield situation;5 Farm B has both low milk and crop yields; Farm C represents a low milk and high crop yield situation; and Farm D features both high milk and crop yields. The yields for the four farms relate to four regions from the CAPRI database, and represent mean yields and production costs in a given region for both milk and crop production, and were selected to illustrate how productivity and profitability differences affect the mitigation and cost-effectiveness of GHG abatement policy instruments across regions.
Results
Baseline scenario
Table 3.1 presents the baseline situation for the four farms representing different conditions across four regions. The baseline assumes that all farms receive support payments of EUR 190/hectare under the first pillar of the Common Agricultural Policy (CAP). The following paragraphs briefly describe the situation of each farm type under the baseline scenario.
Under current market and policy conditions, Farm A receives EUR 19 000 per year as CAP support based on non-current production. With milk price of EUR 0.45/kg, the herd generates market revenues of around EUR 250 000 yearly. Dairy production is labour intensive, with more than 100 hours per dairy cow (including fodder production), such that the labour input totals 7 000 working hours per year. Significant costs are spent on concentrates, close to EUR 56 000, and nitrogen fertiliser, around EUR 30 000.
Profit before taxes and social security is around EUR 125 000, which suggests returns to labour of EUR 18/hour, potentially competitive to wages outside agriculture. Note that, by assumption, only 50% of fixed costs in dairy production are included in the medium-term calculation, with the remaining 50% considered sunk and not decision-dependent. It should be noted that in the real-world farm population, some farmers will have invested (or re-invested) recently in dairy operations and are likely to continue for several years despite relatively low returns to labour, while others will need to decide whether to re-invest in dairy production over the medium-term, or to continue crop production as well as work off-farm.
Table 3.1. Baseline scenario
Farm A High milk and low crop yields |
Farm B Low milk and low crop yields |
Farm C Low milk and high crop yields |
Farm D High milk and high crop yields |
|
---|---|---|---|---|
Herd size, dairy cows |
57 |
42 |
63 |
57 |
Land allocation, ha: CO:Si:P:Se:GSe1 |
18-42-40-0-0 |
31-29-32-0-8 |
37-23-21-0-19 |
40-20-40-0-0 |
Wheat yield, kg/ha |
4371 |
4866 |
6406 |
6760 |
Nitrogen fertiliser application, kg/ha |
151 |
159 |
181 |
184 |
Milk, kg/dairy cow/ year |
9098 |
8074 |
8070 |
9128 |
Concentrates, kg DM/dairy cow/day2 |
10.3 |
7.1 |
6.0 |
14.0 |
Silage, kg DM/dairy cow/day2 |
5.3 |
6.9 |
7.3 |
3.0 |
Total GHG emissions, kg CO2eq./year |
642 600 |
515 504 |
649 996 |
671 539 |
GHG emission shares: cultivation: fertiliser: soil: livestock |
7-22-13-58 |
11-26-15-48 |
9-22-10-58 |
11-22-13-55 |
GHG Emission intensity for wheat (kg CO2eq per value of output) |
4.3 |
3.9 |
3.1 |
2.9 |
GHG Emission intensity for milk (kg CO2eq per value of output) |
1.1 |
1.2 |
1.2 |
1.1 |
Profit, EUR/year |
125 606 |
99 234 |
159 140 |
146 159 |
1 Cereals and oilseeds (CO), Grass silage (Si), Pasture (P), Set-aside (Se) and Green set-aside (GSe).
2 DM refers to dry matter.
The total fixed costs amount to around EUR 800 per cow per year. Moving to a long-term perspective, and including the sunk part of these costs, profits would decrease by EUR 23 000 (50% of EUR 46 000 in total fixed costs). EUR 19 000 of support payments are decoupled and thus do not impact production decisions on the farm.6 If profit net of decoupled payment is decreased by the fixed costs, the total long-run decision-dependent profits of the farm amount to EUR 83 000, or to around EUR 12/hour. This illustrates that sunk costs of investment play a key role in farm adjustment and management response to different policy instruments.7
The majority of GHG emissions stems from enteric fermentation (representing 58% of total CO2eq emissions and 90% of livestock-related emissions), followed by nitrogen fertilisation (22% of total CO2eq emissions). These numbers are similar to findings in other studies for intensive dairy systems in the temperate zone. Due to the relatively low productivity of wheat production on Farm A, the greenhouse gas (GHG) emission intensity of wheat is high relative to the value of output, while GHG emission intensity is low for milk production owing to high milk yields.
Due to lower milk yields on Farm B, dairy cow feeding is less intensive than on Farm A, and thus requires higher grass silage areas per cow, while marginal returns per unit of silage produced are lower. Combined with moderate crop yields, this results in the smallest herd of the four farms as land is allocated to cash crops. The smaller dairy herd size results in low methane emissions from enteric fermentation and as a result the total GHG emissions are smaller than in Farm A. The emission intensity of milk production is however higher, as emissions linked to the energy maintenance needs of the herd are distributed over a smaller milk quantity. The GHG emission intensity for wheat is lower than on Farm A, reflecting slightly higher yields.
Although milk yields are relatively low on Farm C, higher crop yields (and in particular grass silage production) helps to push the optimal herd size of dairy cows to 63. High productivity grass silage production allows less land to be allocated to silage and more to wheat production. Although total CO2eq emissions are higher than on Farms A or B, the GHG emission intensity for wheat on Farm C is lower due to high wheat yields.
Farm D represents the case of both high milk and crop yields. This leads to high fertiliser intensity in wheat production and high concentrate feeding for dairy cows. Due to intensive production, total CO2eq emissions at the farm level are high, but the emission intensities for wheat and milk are relatively low.
GHG emission constraint: Abatement cost function and marginal abatement costs
Table 3.2 presents how the different farms respond to decreasing GHG emission ceilings. Calculations assume that farmers have no off-farm employment opportunities and face fixed investment costs of 50%.8 An enforced uniform 10% GHG emission reduction for each farm considerably affects input (reduced concentrate feeding and nitrogen fertiliser application) and land use (allocation of land from pasture and grass silage towards wheat and green set-aside). The latter reflects that most of the GHG savings stems from reduced methane emissions related to enteric fermentation, which decrease on average by about 15%, reflecting a reduction in herd size. This, in turn, decreases fodder needs and drives the land allocation from pasture and silage towards cereals and green set-aside. Note that except for Farm A, all farms increase concentrate intake per cow and thus milk yields. Farm A is a special case as its baseline shows the highest share of grass silage in land use of all farms because of the low productivity and profitability of wheat production relative to silage. Nitrogen fertiliser application per hectare is another way to respond to the emission ceiling, but its contribution to reducing GHG emissions is limited.
At higher GHG emissions reduction levels (20%-40%), farms adjust with the same mechanisms: they reduce the number of dairy cows and shift land allocation towards cereals and green set-aside, away from pasture and silage.
Table 3.3 shows the marginal abatement costs (MACs) of GHG emission reductions for the four farms. Marginal abatement costs represent the shadow price of emission constraint for each emission reduction level. The estimates made assume that farmers have no off-farm employment opportunity (thus zero opportunity cost of farm labour) in order to show the agricultural cost of adjustment on a given farm for each emission reduction level.9 These marginal abatement costs coincide with the literature. For an 8% GHG emission reduction, De Cara et al. (2005[4]) estimate an average marginal abatement cost of EUR 123/ton of CO2eq for the EU15, and Pérez Dominguez et al. (2003) an average of EUR 95/ton of CO2eq for the EU27 (with regional variation of EUR 30-230/ton of CO2eq). As Table 3.3 shows, the average marginal abatement cost is EUR 114/ton of CO2eq for 10% GHG emission reduction. For 8% GHG emission reduction, it would be EUR 107/ton of CO2eq. Note that off-farm employment opportunities are accounted for in the simulations presented in the other sections of this chapter.
The main GHG abatement technologies included in the model for livestock-related emissions are covered manure storage (so-called floating cover) to reduce GHG (especially methane) emissions from manure storage, and injection spreading of manure in the field parcels to reduce GHG (especially nitrous oxide) emissions from manure spreading. Table 3.4 presents the GHG reduction capacity and abatement costs related to these two technological options from dairy farming. Results are presented for Farm A relative to the baseline scenario (open manure storage and broadcast spreading of manure in the field parcels).
Table 3.2. Response of farmers to decreasing GHG emission ceilings
Percentage change from baseline under 10% to 40% emission reductions
Farms |
Baseline and GHG emissions reduction levels, % |
Profit, % change |
Milk production, % change |
Concentrate feeding, % change |
Nitrogen application for wheat, % change |
Cereals acreage, % change |
Share of green set-aside of total area, % |
Methane emissions, % change |
---|---|---|---|---|---|---|---|---|
Farm A |
Base |
125 606 |
519 303 |
10.3 |
151 |
18 |
0 |
363 015 |
(High milk |
10 |
-10.0 |
-15.9 |
-2.8 |
-2.6 |
+26.6 |
0 |
-15.8 |
and low |
20 |
-21.1 |
-32.8 |
-4.8 |
-4.1 |
+61.6 |
7.0 |
-39.7 |
crop yields) |
30 |
-32.8 |
-50.3 |
-6.6 |
-5.4 |
+100.1 |
15.0 |
-50.1 |
40 |
-45.2 |
-68.2 |
-8.4 |
-6.5 |
+144.6 |
22.0 |
-68.1 |
|
Farm B |
Base |
99 234 |
336 777 |
7.1 |
159 |
31 |
8 |
240 147 |
(Low milk |
10 |
-10.0 |
-18.3 |
+0.1 |
-0.3 |
+17.0 |
14.0 |
-18.3 |
and low |
20 |
-20.1 |
-36.6 |
+0.3 |
-0.6 |
+34.0 |
20.0 |
-36.7 |
crop yields) |
30 |
-30.3 |
-55.1 |
+0.4 |
-0.9 |
+51.2 |
26.0 |
-55.1 |
40 |
-40.5 |
-73.5 |
+0.5 |
-1.2 |
+68.6 |
32.0 |
-73.6 |
|
Farm C |
Base |
159 140 |
509 974 |
6.0 |
181 |
37 |
19 |
365 986 |
(Low milk |
10 |
-9.0 |
-14.1 |
+0.8 |
-8.0 |
+8.8 |
24.0 |
-15.5 |
and high |
20 |
-18.9 |
-30.0 |
+0.8 |
-8.3 |
+18.5 |
27.0 |
-31.8 |
crop yields) |
30 |
-29.0 |
-46.1 |
+0.8 |
-8.6 |
+28.4 |
30.0 |
-47.7 |
40 |
-39.2 |
-62.3 |
+0.9 |
-8.9 |
+38.3 |
33.0 |
-63.4 |
|
Farm D |
Base |
146 184 |
521 667 |
14.0 |
184 |
40 |
3 |
358 750 |
(High milk |
10 |
-8.7 |
-15.4 |
+0.2 |
-1.1 |
+7.5 |
10.0 |
-15.4 |
and high |
20 |
-17.7 |
-31.2 |
+0.2 |
-1.4 |
+15.2 |
16.0 |
-31.2 |
crop yields) |
30 |
-26.8 |
-47.1 |
+0.2 |
-1.7 |
+23.1 |
21.0 |
-47.1 |
40 |
-36.0 |
-63.0 |
+0.2 |
-2.1 |
+30.9 |
27.0 |
-63.0 |
Table 3.3. Marginal abatement costs (MACs) for farms
Reductions in tons of CO2eq, MAC in EUR per ton of CO2eq
10% |
20% |
30% |
40% |
|||||
---|---|---|---|---|---|---|---|---|
Reduction |
MAC |
Reduction |
MAC |
Reduction |
MAC |
Reduction |
MAC |
|
Farm A |
64.26 |
50.2 |
128.52 |
88.2 |
192.78 |
109.0 |
257.04 |
125.7 |
Farm B |
51.55 |
192.1 |
103.10 |
193.1 |
154.65 |
194.1 |
206.20 |
195.0 |
Farm C |
65.00 |
108.0 |
130.00 |
119.7 |
195.00 |
148.6 |
260.00 |
168.0 |
Farm D |
66.94 |
107.0 |
133.89 |
133.4 |
200.83 |
149.2 |
267.77 |
159.9 |
Table 3.4. GHG abatement technologies for Farm A
|
Manure storage with floating cover |
Manure injection spreading |
---|---|---|
Total GHG reduction, tons (%) |
3.65 (0.57%) |
7.96 (1.24%) |
Abatement cost, EUR per ton of CO2eq |
59 |
208 |
Reduction of total livestock emissions (%) |
0.9 |
1.2 |
The GHG reduction capacity appears relatively limited for both technologies as covered manure storage reduces total GHG emissions from the farm only by about 0.6% and livestock GHG emissions by 0.9%. Manure injection spreading has slightly higher GHG abatement capacity as it reduces both total GHG emissions and livestock GHG emissions by about 1.2%. The abatement cost per ton of CO2eq is lower for covered manure storage than for manure injection spreading, but the bottom line is that both technologies have relatively limited capacity for GHG abatement.
This chapter analyses only a limited set of technological abatement options. Other technological options, such as fat supplementation in ruminant diets to reduce enteric methane emissions or anaerobic digestion to reduce methane emissions from manure storage, exist and may be not only more effective but also more cost-effective. For example, MacLeod et al. (2015[1]) review the literature and reports, e.g. EUR 70/ton of CO2eq for fat supplementation diet (EU15) and EUR 77-214/ton of CO2eq for on-farm digesters (EU27). More significant dairy production system changes were analysed, for example, in a report published by the French Ministry of Agriculture in 2016,10 in which low-input, low-emission-intensive and economically viable dairy production systems were identified.
GHG emission tax, abatement subsidy, and cap-and-trade
Compared to command-and-control measures – such as enforcing specific abatement technologies by law – a tax, abatement subsidy, and cap-and-trade on GHG emissions give farmers the freedom to adjust to the tax, subsidy or permit price. Because of their cost-effectiveness, these tools should be considered as preferred policy options. However, they require monitoring of all GHG emissions on-farm including, for example, herd size, milk yields, fertiliser application levels of different crops, manure storage, and manure application techniques.11 Taking into account these potential implementation issues, this chapter considers other more practical but less cost-effective policy instruments.
The effect of three GHG emission tax and abatement subsidy rates are tested in the following analysis: a rate of EUR 9/ton of CO2eq, which corresponds to the European Emission Allowance price in January 2018; a rate of EUR 30/ton of CO2eq, which is a lower-end estimate of climate damage cost of CO2 emissions according to the OECD (2016[5]); and a rate of EUR 50/ton of CO2eq, which models indicate is the required value to limit the temperature increase to 1.5°C, in line with the more ambitious target of the Paris Agreement (Rogelj et al., 2015[6]).
Results from the simulations of the three emission tax rates (EUR 9, EUR 30, and EUR 50/ton of CO2eq) show that the mitigation effectiveness of the tax varies with the specificities of each farm, i.e. how dairy production responds to the tax level (Table 3.5).
Farm C keeps the herd unchanged up to a tax level of EUR 30/ton of CO2eq and pays the tax (EUR 19 401) rather than reduce the number of dairy cows. Only the intensity of fertiliser use is somewhat adjusted, leaving overall GHG emissions almost unchanged. A tax level of EUR 50/ton of CO2eq pushes the farmer to reduce somewhat dairy herd size, thus decreasing GHG emissions. The farm nevertheless pays a significant amount of tax (EUR 25 745). Farm B is characterised by both low milk and crop yields and has the lowest profit in the baseline, which implies low returns to labour and land. The lowest tax rate of EUR 9/ton of CO2eq renders milk production unprofitable and triggers the farm to switch to crop production only. In Farms A and D, EUR 30/ton of CO2eq is the critical tax rate where milk production is abandoned. Reduction of the number of dairy cows or switching the production line not only have a significant impact on GHG emissions, but on the profitability of production as seen from profits without tax payments. Moreover, the reduction of milk production or a switch to only crop production significantly reduces the labour input requirements. Depending on employment opportunities, the reduced labour input for farming creates the possibility to work part-time off-farm and to earn off-farm income in order to compensate for the decrease in farm income.
Table 3.5. Impact of three emission tax-rates on production, GHG emissions and profits
EUR 9, 30 and 50/ton of CO2
|
Dairy cows, number |
Nitrogen application for wheat, kg/ha |
Land allocation, CO:Si:P:Se:GSe1 |
GHG emissions, g CO2eq |
Profit, EUR |
Tax payments, EUR |
Profit without tax payments, EUR |
---|---|---|---|---|---|---|---|
Farm A (high milk, low crop yields) |
|||||||
Base |
57 |
151 |
18-42-40-0-0 |
642 600 |
125 606 |
0 |
125 606 |
9 |
52 |
148 |
21-39-40-0-0 |
602 795 |
112 629 |
5 425 |
118 054 |
30 |
0 |
141 |
60-0-0-0-40 |
228 979 |
32 902 |
6 869 |
39 771 |
50 |
0 |
136 |
60-0-0-0-40 |
226 363 |
28 348 |
11 318 |
39 667 |
Farm B (low milk, low crop yields) |
|||||||
Base |
42 |
159 |
31-29-32-0-8 |
515 504 |
99 234 |
0 |
99 234 |
9 |
0 |
156 |
60-0-0-0-40 |
235 648 |
42 169 |
2 121 |
44 290 |
30 |
0 |
150 |
60-0-0-0-40 |
232 721 |
37 251 |
6 982 |
44 233 |
50 |
0 |
144 |
60-0-0-0-40 |
230 105 |
32 623 |
11 505 |
44 128 |
Farm C (low milk, high crop yields) |
|||||||
Base |
63 |
181 |
37-23-21-0-19 |
649 996 |
159 140 |
0 |
159 140 |
9 |
63 |
178 |
37-23-20-0-20 |
648 939 |
153 295 |
5 840 |
159 135 |
30 |
63 |
171 |
37-23-19-0-21 |
646 698 |
139 691 |
19 401 |
159 092 |
50 |
43 |
166 |
44-16-13-0-27 |
514 899 |
102 116 |
25 745 |
127 861 |
Farm D (high milk, high crop yields) |
|||||||
Base |
57 |
184 |
40-20-40-0-0 |
671 539 |
146 159 |
0 |
146 159 |
9 |
43 |
182 |
45-15-26-0-14 |
559 610 |
119 996 |
5 036 |
125 033 |
30 |
0 |
175 |
60-0-0-0-40 |
244 510 |
54 439 |
7 335 |
61 774 |
50 |
0 |
170 |
60-0-0-0-40 |
241 894 |
49 575 |
12 095 |
61 670 |
Note: 1 Cereals and oilseeds (CO), Grass silage (Si), Pasture (P), Set-aside (Se) and Green set-aside (GSe).
A GHG abatement subsidy provides the same incentives for emission reduction as a tax, which also implies exactly the same adjustments and results. Input use, land allocation and dairy herd size are exactly as those presented for GHG emission tax in Table 3.5. The only difference is that an abatement subsidy increases farm profits while an emission tax reduces them. In the long run, however, their GHG mitigation impacts are not equivalent as they have totally different impacts on the entry-exit margin of production: the abatement subsidy induces entry to the sector while a tax induces exit from the sector. A tax and a subsidy are also naturally different from the viewpoint of net government revenue, as a tax increases net revenues whereas a subsidy decreases them. Furthermore, a subsidy violates the “beneficiary pays” principle and might be considered unfair if other agents in the economy are subject to environmental taxes or costly command-and-control measures.
A GHG abatement subsidy changes the income portfolio of the farms given that in addition to income from production there is also income from GHG abatement. Figure 3.2 illustrates the shares of production income (PI) and GHG abatement income (AI) at different abatement subsidy levels (EUR 9, EUR 30, and EUR 50/ton of CO2eq). This figure does not account for potential off-farm employment income and includes only the total income from production and GHG abatement. At the highest abatement subsidy level (EUR 50/ton of CO2eq), income from abatement represents between 24% and 34% of total income for Farms A, B, and D, while for Farm C it represents only 5%. Hence, income shares of production and GHG abatement depend on how farms respond to the abatement subsidy by adjusting dairy herd size, land use, and nitrogen application.
Figure 3.2. Shares of production and GHG abatement income under different abatement subsidy levels
Note: Production income (PI) and abatement income (AI).
A cap-and-trade scheme (emission trading) is often presented as a third cost-effective mitigation policy instrument as it provides the same incentives for emission reductions and implies exactly the same adjustments regarding input use, land allocation, and dairy herd size. Compared to a GHG abatement subsidy, emission trading has no impact on a government’s budget, as emission permits are distributed freely and are not auctioned, while net-buyers of permits in the agriculture sector finance GHG abatement rather than taxpayers, as is the case for an abatement subsidy.
This analysis tests a cap-and-trade scheme to illustrate the efficiency gains of market-based policy instruments in their ability to target GHG abatement to those farms that can mitigate GHGs at the least cost. In our example, a government caps the total GHG emissions and allocates a certain amount of tradable emissions permits to each farm free of charge (i.e. grandfathering). The original permit allocation is based on each farm’s baseline emissions minus 20%. Thus, emission reductions and permit allocations are equal in relative terms across the four farms under study.
The effects of this trading scheme are compared to those of the uniform 20% emission constraint to identify the efficiency gains of a cap-and-trade system (Table 3.6). The results show that the trading scheme generates an average efficiency gain of 17%; that is, the average cost of meeting the emission target is 17% lower with trading than with a uniform emission constraint. Large net-sellers (Farm A) reduce their compliance costs by 35%, and large net-buyers reduce them by 34%. Farms C and D, which have marginal abatement costs close to the equilibrium permit price (EUR 127.5/tons of CO2eq), gain only slightly (1%) from trading relative to a uniform emission restriction.
Table 3.6. Gains from GHG emission trading relative to uniform emission constraint
Farms |
Baseline emissions, tons of CO2eq |
Allocation of permits, tons of CO2eq |
Abatement cost without trading, EUR |
Permit sales (-)/ purchases (+) |
Net cost with trading, EUR |
Gains from trading, EUR |
---|---|---|---|---|---|---|
Farm A |
643 |
514 |
11 336 |
-101 |
7 350 |
3 985 |
Farm B |
516 |
412 |
19 909 |
103 |
13 145 |
6 763 |
Farm C |
650 |
520 |
15 560 |
-24 |
15 371 |
189 |
Farm D |
669 |
536 |
17 860 |
22 |
17 727 |
133 |
SUM |
2 478 |
1 982 |
64 665 |
0 |
53 593 |
11 071 |
Input taxes on ruminant heads and nitrogen fertiliser
Because detailed reporting on farm processes responsible for GHG emissions might be costly for both farmers and programme administrators, policies that would tax emission drivers (inputs) that can be more easily observed than emissions themselves are analysed. This more easily implemented tax comes at the cost of reduced economic efficiency as not all emissions are taxed and emissions linked to a driver such as herd size vary from farm to farm, such that the marginal emission cost carried by the farmer might be different from the (implicit) tax rate per CO2eq targeted.
Two input taxes on GHG emissions drivers were analysed: an input tax on nitrogen fertiliser and an input tax on ruminant heads.12 The same three levels of CO2eq tax rates (EUR 9, EUR 30, and EUR 50/ton of CO2eq) were used to analyse the second-best policy instruments, mapped into a tax per unit of fertiliser or cow.
The input tax on nitrogen fertiliser is based on CO2eq emissions from nitrogen fertiliser application. The tax rates applied to nitrogen fertiliser corresponding to the three levels of CO2eq taxes are: 3.1% (EUR 9/ton of CO2eq), 10.3% (EUR 30/ton of CO2eq), and 17.1% (EUR 50/ton of CO2eq). The input tax on ruminant heads targets GHG emissions from dairy herd, including enteric fermentation and manure management.
The input tax on nitrogen fertiliser has a relatively strong mitigation impact on Farm B only, where total GHG emissions reductions are 6%, 20%, and 33% under the respective tax rates (Table 3.7). This is due to the reduced herd size despite the fact that the fertiliser tax does not directly target dairy-production-related emissions. Here, the low concentrate and high grass silage use per dairy cow leads to a strong pass-through of the somewhat increased silage production costs on the profitability of milk production.
An input tax on ruminant heads directly targets GHG emissions from dairy production and has a strong mitigation impact in all other cases except Farm C, which has the highest MAC. Here, even an implicit tax rate of EUR 30/ton of CO2eq does not reduce the herd size. This is similar to the effect of a tax on emissions, except that at EUR 50/ton of CO2eq. the effectiveness of the tax on ruminant heads is only half that of a tax on emissions for Farm C. This is explained by the inability of the former to induce mitigation in crop activities.
Table 3.7. Detailed impact of a tax on nitrogen fertiliser and ruminant heads
EUR 9, EUR 30 and EUR 50/ton of CO2eq
|
Input tax on nitrogen fertiliser |
Input tax on ruminant heads |
||||
---|---|---|---|---|---|---|
|
Dairy cows, number |
Profit, EUR |
GHG emissions, kg CO2eq |
Dairy cows, number |
Profit, EUR |
GHG emissions, kg CO2eq |
Farm A (high milk, low crop yields) - Base |
57 |
125 606 |
642 600 |
57 |
125 606 |
642 600 |
9 EUR/ton of CO2eq |
57 |
124 675 |
640 268 |
52 |
113 387 |
602 795 |
30 EUR/ton of CO2eq |
57 |
122 223 |
632 899 |
0 |
34 419 |
228 979 |
50 EUR/ton of CO2eq |
53 |
117 318 |
612 485 |
0 |
34 419 |
228 979 |
Farm B (low milk, low crop yields) - Base |
42 |
99 234 |
515 504 |
42 |
99 234 |
515 504 |
9 EUR/ton of CO2eq |
37 |
92 511 |
483 579 |
0 |
42 624 |
235 648 |
30 EUR/ton of CO2eq |
27 |
77 377 |
411 497 |
0 |
42 624 |
235 648 |
50 EUR/ton of CO2eq |
17 |
63 725 |
346 317 |
0 |
42 624 |
235 648 |
Farm C (low milk, high crop yields) - Base |
63 |
159 140 |
649 996 |
63 |
159 140 |
649 996 |
9 EUR/ton of CO2eq |
63 |
158 143 |
649 295 |
63 |
153 905 |
649 311 |
30 EUR/ton of CO2eq |
63 |
155 862 |
647 750 |
63 |
141 720 |
647 804 |
50 EUR/ton of CO2eq |
63 |
153 749 |
646 385 |
54 |
119 095 |
585 950 |
Farm D (high milk, high crop yields) - Base |
57 |
146 159 |
671 539 |
57 |
146 159 |
671 539 |
9 EUR/ton of CO2eq |
57 |
145 184 |
668 314 |
46 |
125 162 |
584 081 |
30 EUR/ton of CO2eq |
57 |
142 911 |
665 509 |
0 |
55 957 |
244 510 |
50 EUR/ton of CO2eq |
57 |
140 826 |
662 963 |
0 |
55 957 |
244 510 |
In other cases, especially on Farms C and D, the number of dairy cows is not affected even with the highest tax rate of EUR 50/ton of CO2eq. As a result, GHG emissions reductions are relatively modest, ranging from less than 1% (Farms C and D) to 5% (Farm A), even under the highest tax rate.
Table 3.8 provides a summary of the impacts of the emissions- and input-based taxes and subsidies on GHG emissions and farm income with and without tax or subsidy payments for an equivalent of EUR 30/ton of CO2eq. Results show that the GHG emission tax and abatement subsidy have the same impact on GHG emissions and farm income without tax or subsidy payments. The only difference is found with farm income, which is lower with a GHG tax than with an abatement subsidy. An input tax on ruminant heads closely resembles the emissions-based instruments at EUR 30/ton of CO2eq emission price, since it leads to the same adjustments regarding dairy herd size, which is a key driver for changes in both emission reduction and farm income. The input tax on nitrogen fertiliser has relatively modest impacts on GHG emissions and income, except in the case of Farm B.
Table 3.8. Impacts of the emissions-based and the input-based policy instruments on GHG emissions and profit
|
GHG emissions, kg CO2eq |
Profit with tax or subsidy payment, EUR |
Profit without tax or subsidy payments, EUR |
---|---|---|---|
Farm A (high milk, low crop yields) - Base |
642 600 |
125 606 |
125 606 |
GHG emission tax |
-64% |
-74% |
-68% |
GHG abatement subsidy |
-64% |
-58% |
-68% |
Input tax on nitrogen fertiliser |
-2% |
-3% |
0% |
Input tax on ruminant heads |
-64% |
-73% |
-68% |
Farm B (low milk, low crop yields) - Base |
515 504 |
99 234 |
99 234 |
GHG emission tax |
-55% |
-62% |
-55% |
GHG abatement subsidy |
-55% |
-47% |
-55% |
Input tax on nitrogen fertiliser |
-20% |
-22% |
-19% |
Input tax on ruminant heads |
-54% |
-57% |
-55% |
Farm C (low milk, high crop yields) - Base |
649 996 |
159 140 |
159 140 |
GHG emission tax |
-1% |
-12% |
0% |
GHG abatement subsidy |
-1% |
0% |
0% |
Input tax on nitrogen fertiliser |
0% |
-2% |
0% |
Input tax on ruminant heads |
0% |
-11% |
0% |
Farm D (high milk, high crop yields) - Base |
671 539 |
146 159 |
146 159 |
GHG emission tax |
-64% |
-63% |
-58% |
GHG abatement subsidy |
-64% |
-50% |
-58% |
Input tax on nitrogen fertiliser |
-1% |
-2% |
0% |
Input tax on ruminant heads |
-64% |
-62% |
-58% |
Note: Impacts evaluated with a tax rate of EUR 30/ton of CO2eq.
Afforestation of agricultural land for carbon sequestration13
Carbon sequestration practices on agricultural lands may hold large potential and need to be considered in the overall mix of GHG mitigation options.
Compared with measures that reduce annual GHG emission flows, carbon sequestration measures face several policy design challenges: dynamics, additionality, permanence, and leakage. Carbon sequestration practices increase carbon storage with diminishing rate until they plateau at a new equilibrium, which may take 20-100 years. Policy needs to encourage sequestration practices that are additional (i.e. that would not have happened without a specific policy). Some sequestration practices, such as no-till and green set-aside, are relatively easily reversed, which would lead to a loss of the sequestration benefits. Because of potential impermanence, soil carbon sequestration practices may not have the same climate protection benefits as technological changes that permanently reduce GHG emissions. Finally, leakage occurs when a soil carbon sequestration project increases GHG emissions elsewhere due to production displacements.
Considering the potential of carbon sequestration practices on agricultural lands, Lal (2004[7]) estimates that agricultural soils can offset 15% of global GHG emissions. However, the global potential for carbon sequestration in agriculture, forestry, and land use sectors (AFOLU) remains uncertain. Recent estimates show that technical global carbon sequestration potential ranges between 2.6 and 4.8 Gt CO2 at carbon prices between USD 20 and USD 100/ton of CO2eq (Smith et al., 2015[8]).
This chapter presents a policy scenario supporting carbon sequestration through afforestation. Afforestation as a carbon sequestration practice is less easily reversible and its additionality is clearer than, for example, no-till adoption. Like many other soil carbon sequestration practices, afforestation provides ancillary environmental benefits, including improved water quality. This scenario introduces a subsidy for carbon sequestration (EUR 9, EUR 30, and EUR 50/ton of CO2eq) applied to GHG mitigation induced by the afforestation of agricultural land.14 Afforestation provides abatement benefits through above (trees crown) and below ground (roots) carbon sequestration. Kolari et al. (2004[9]) find that Scots pine (Pinus sylvestris) forests on mineral soils act as a sink with an average sequestration of 5 085 kg CO2/ha/year over an 80-year rotation period. The annual net-revenue over one rotation period (80 years) of afforested land is assumed to be EUR 47.8/hectare.
This afforestation subsidy does not induce much change. Afforestation of agricultural land is a profitable option to mitigate GHG emissions only in Farm A, and in this case afforestation only takes place at the highest subsidy level of EUR 50/ton of CO2eq. Other farms do not adopt afforestation as a mitigation option even at the highest subsidy level. Relative to the baseline for Farm A, under the afforestation subsidy, land allocation shifts from cereals and grass silage towards afforestation (20 hectares of land is afforested) and dairy herd size reduces from 57 to 55. The total emissions of Farm A decrease by 26% and this reduction is mainly due to afforestation through conversion of land from emissions source to sink. The decrease in the size of the dairy herd plays a small role only in decreasing emissions relative to the baseline.
In view of the poor GHG abatement performance of afforestation subsidies under carbon prices (EUR 9, EUR 30, and EUR 50/ton of CO2eq), it is not considered further as a mitigation option.
Mitigation policy instruments and the role of sunk investment costs
Sunk investment costs15 play a key role in farm adjustments and production response to different mitigation policy instruments, and can lead to substantially different reactions in the short and long run. This is especially the case in dairy farming, which is characterised by long-lasting investments in stables and milking parlours that account for a larger share of overall production cost. The following analysis tests the case of all fixed costs assumed as sunk (instead of the 50% assumed so far), simulating the short-run response. This simulates a situation where farms continue dairy operations as long as market revenues exceed the variable costs of milk production (including any GHG taxes).
As expected, the mitigation responses of all farms are drastically reduced when all fixed costs are assumed as sunk. Comparing results in Table 3.9 with corresponding results in Table 3.5 (GHG emission tax) and Table 3.7 (input tax on ruminants) shows the significant impact of fixed investment costs on adjustment possibilities of dairy farms under GHG mitigation policies. If investment costs for dairy operations are sunk and the opportunity costs of farm labour are low (the farmer has no opportunity to work off-farm), the GHG mitigation effectiveness of an emission tax or an input tax on ruminants is drastically lower in the short run than the medium-term adjustments shown in Tables 3.5 and 3.7. If farmers view all their investments in dairy production as sunk costs, they will keep the dairy herd and continue production even with high tax rates. As a result, reductions in GHG emissions are modest. In a real-world situation this would correspond to farmers who have invested recently and will continue to produce for several years rather than make adjustments. Farmers who will need to invest over the medium term would rather adjust their herd size as discussed above.
Table 3.9. Performance of GHG emission and input taxes on ruminant heads (EUR 30/ton of CO2eq) under the assumption that all dairy investments are sunk costs
|
Dairy cows, number |
Milk production, kg |
GHG emissions, kg CO2eq |
Profit, EUR |
Tax payments, EUR |
---|---|---|---|---|---|
Farm A (high milk, low crop yields) – Base |
58 |
517 843 |
639 431 |
148 572 |
0 |
GHG emission tax (EUR 30/ton of CO2eq) |
58 |
513 966 |
624 002 |
129 608 |
18 720 |
Input tax on ruminant heads (EUR 30/ton of CO2eq) |
58 |
513 966 |
624 002 |
132 137 |
16 191 |
Farm B (low milk, low crop yields) – Base |
63 |
499 669 |
642 970 |
149 270 |
0 |
GHG emission tax (EUR 30/ton of CO2eq) |
63 |
498 639 |
631 464 |
130 148 |
18 944 |
Input tax on ruminant heads (EUR 30/ton of CO2eq) |
63 |
498 639 |
631 464 |
132 677 |
16 415 |
Farm C (low milk, high crop yields) – Base |
62 |
510 752 |
661 683 |
169 232 |
0 |
GHG emission tax (EUR 30/ton of CO2eq) |
62 |
511 059 |
654 127 |
149 495 |
19 624 |
Input tax on ruminant heads (EUR 30/ton of CO2eq) |
62 |
510 643 |
655 373 |
151 764 |
17 359 |
Farm D (high milk, high crop yields) – Base |
57 |
520 322 |
667 369 |
169 087 |
0 |
GHG emission tax (EUR 30/ton of CO2eq) |
57 |
520 322 |
661 067 |
149 160 |
19 832 |
Input tax on ruminant heads (EUR 30/ton of CO2eq) |
57 |
520 322 |
662 472 |
151 505 |
17 493 |
Ancillary environmental costs and benefits of GHG mitigation policies
GHG mitigation policy instruments incentivise farmers to change input use, land allocation and production technologies. These adjustments may have significant ancillary environmental benefits or costs.
The indirect effects of mitigation efforts on nitrogen runoff (reflecting water quality impacts) are not straightforward and may vary according to the stringency of the mitigation policy applied (as seen for Farm A in Table 3.10). Results indicate that how this impacts water quality may depend on the chosen level of tax rate. For a GHG abatement subsidy, a GHG emission tax and an input tax on ruminants, the impact on water quality is first positive at the low tax rate of EUR 9/ton of CO2eq. The level of nitrogen runoff decreases by about 5% and counts as an environmental co-benefit of the GHG mitigation policy. This stems from the fact that at a low tax rate fertilization levels are adjusted, but the dairy herd is not reduced (by much), such that the grass silage area with lower nitrogen losses than wheat is more or less constant. At higher tax rates, however, the impact on water quality is negative (increased nitrogen runoff by about 11%). This is because higher tax rates reduce dairy herd size, and more land is allocated to cereals production and away from pasture and grass silage that have lower propensity for nutrient runoff. Moreover, the negative water quality impact is larger under a tax rate of EUR 30/ton of CO2eq than EUR 50/ton of CO2eq. For Farm A, under the three instruments (CO2 tax or subsidy, ruminant tax), the strongest adjustment in terms of dairy cow numbers and land allocation towards cereals takes place when the tax rate is EUR 30/ton of CO2eq. Under a tax rate of EUR 50/ton of CO2eq there is no additional change in dairy cow numbers or land allocation, but the tax induces reduced application of nitrogen fertiliser leading to lower nitrogen runoff, and thus a lower negative impact on water quality relative to a lower tax rate of EUR 30/ton of CO2eq. Potential ancillary environmental effects depend not only on a given type of GHG mitigation policy instrument, but also on its intensity. The input tax on nitrogen provides environmental co-benefits with all CO2eq tax rates.
Table 3.10. Impact of GHG mitigation instruments on nitrogen runoff for Farm A
Percentage change from base
Instrument |
EUR 9 /ton CO2eq |
EUR 30 /ton CO2eq |
EUR 50 /ton CO2eq |
---|---|---|---|
Baseline N runoff |
1351 |
1351 |
1351 |
GHG abatement subsidy |
-5.1% |
+11.1% |
+7.5% |
GHG emission tax |
-5.1% |
+11.1% |
+7.5% |
Input tax ruminants |
-5.1% |
+11.1% |
+7.5% |
Input tax fertiliser |
-2.0% |
-6.5% |
-5.1% |
Ranking alternative policy instruments by cost effectiveness
While the previous sections point to significant variations in cost-effectiveness across policy instruments, they also highlight that some are less dependent on GHG emission monitoring than others. Since the latter may have consequences on the cost of policy implementation, this section reviews the relative cost-effectiveness of GHG mitigation policy instruments with and without transaction costs. Accounting for transaction costs: improves comparison among and screening of alternative policy instruments; can help the design and implementation of effective policy instruments to achieve objectives; improves the evaluation of policy instruments; and helps track budgetary costs of policy instruments over their entire life cycle (McCann et al., 2005[10]).
The literature on transaction costs of GHG mitigation policy instruments in the context of European agriculture is scarce. De Cara et al. (2018[11]) analyse optimal coverage of GHG emission tax in the presence of monitoring, reporting, and verification costs in the context of European agriculture. To calculate the magnitude of these for GHG emission tax in their “medium” scenario, they use EUR 2.5/ton of CO2eq based on Ancev (2011[12]). Bakam et al. (2012[13]) assess the cost-effectiveness of a fertiliser tax, a GHG emission tax, and a permit-trading scheme based on data from Scottish agriculture. They assume zero transaction costs for the fertiliser tax, since the tax is included in the price of fertiliser. For medium-size farms, the transaction costs of the emissions tax are calculated to be 29% lower than those part of a permit-trading scheme (GBP 2 000 and GBP 2 825, respectively). Pérez Domínguez and Britz (2003[14]) analyse GHG emission trading for European agriculture and adopt a transaction cost (paid by permit buyers) of EUR 5/ton of CO2eq for trades within EU Member States and EUR 10/ton of CO2eq for trade between member states.
Based on the literature cited, the analysis undertaken here uses the following transaction cost estimates: EUR 3.5/tons of CO2eq for the GHG emission tax and GHG abatement subsidy; EUR 5.0/ton of CO2eq for GHG emission trading; and EUR 2.0/ton of CO2eq for ruminant tax.
As expected, when comparing cost effectiveness without considering transaction costs, all the emissions-based policy instruments continue to have highest level of cost effectiveness at 10% emissions reduction as they provide exactly the same marginal incentives (Table 3.11).16 As an input-based policy instrument, the tax on ruminant heads (which addresses only a subset of GHG emissions stemming from farms) induces slightly higher abatement costs, and thus is slightly less cost effective than the emissions-based policy instruments.
Including transaction costs improves slightly the relative performance of the tax on ruminant heads and worsens the relative performance of GHG emissions trading. The last row of Table 3.11 shows targeting gains to be about 30% for the GHG emission tax and GHG abatement subsidy relative to the input tax on ruminant heads. These targeting gains show that every euro spent on better monitoring, reporting and verification – which increases policy-related transaction costs – brings EUR 1.3 in improved cost-effectiveness. For GHG emission trading, the ratio of these targeting gains is less than one because of the relatively high transaction costs of this instrument in comparison to other emissions-based instruments, and because an input tax on ruminant heads is, as an input-based policy instrument, relatively cost-effective.
Table 3.11. Cost effectiveness of policy instruments with and without transaction costs (Farm A)
GHG abatement subsidy, EUR/ton of CO2eq |
GHG emission tax, EUR/ton of CO2eq |
Tax on ruminant heads, EUR/ton of CO2eq |
GHG emission trading, EUR/ton of CO2eq |
|
---|---|---|---|---|
Cost effectiveness |
50.2 |
50.2 |
53.6 |
50.2 |
Cost effectiveness with transaction costs |
53.7 |
53.7 |
55.6 |
55.2 |
Targeting gains ratio |
1.32 |
1.32 |
- |
0.15 |
Note: All instruments evaluated at 10% reduction of GHG emissions.
Discussion of results and caveats
Consistent with other studies of GHG mitigation in European agriculture, the results show rather high abatement costs in mixed dairy and crop production, at least when a high GHG emission reduction (20-40%) is targeted (Lengers, Britz and Holm-Müller, 2014[15]). The marginal abatement costs for the farms analysed in this study are in the range of results from other European studies. For an 8% GHG emission standard, De Cara et al. (2005[4]) estimate average EU15 marginal abatement costs of EUR 123/ton of CO2eq, and Pérez Domínguez et al (2003[16]) estimate average EU27 costs of EUR 95/ton of CO2eq (with regional variation of EUR 30-230/ton of CO2eq.). In this study, an 8% GHG emission reduction results in average marginal abatement costs of EUR 113/ton of CO2eq.
This study also confirms that the market-based instruments based on emissions (GHG emission tax, GHG abatement subsidy, and cap-and-trade scheme) are the most cost-effective options for GHG mitigation in agriculture. For example, they show that gains from emissions trading relative to uniform emission constraints average 17% for analysed farms. Pérez Domínguez and Britz (2003[14]) find similar gains (23%) from emissions trading relative to uniform emissions constraints for the EU27. De Cara et al. (2005[4]) compare GHG emissions tax with uniform emission constraints and show that for an 8% GHG abatement target, the cost saving ratio of GHG emission tax is 2.2. That is, meeting the abatement target is more than twice as expensive for the uniform emission constraint than for the emission tax.
Moreover, results confirm that it pays to target emissions broadly, as the emissions-based policy instruments (GHG emission tax and GHG abatement subsidy in particular) are more cost-effective, even when transaction costs related to monitoring, reporting, and verification are included. Targeting gains are about 30% for the GHG emission tax and GHG abatement subsidy relative to the input tax on ruminant heads. These targeting gains show that, for a GHG emission tax or a GHG abatement subsidy, every euro spent on better monitoring, reporting and verification, which increases policy-related transaction costs, brings EUR 1.3 through improved cost-effectiveness. Bakam et al. (2012[13]) get similar results for GHG emission trading over an input tax for GHG emission reduction targets that are over 29%. De Cara et al. (2018[11]) show that the social welfare of covering all farms or only the largest emitters of GHG emissions in European agriculture depends on the marginal social damage of GHG emissions and monitoring, reporting and verification costs. If the marginal damage of emissions is high (EUR 100/ton of CO2eq) and monitoring, reporting and verification costs per farm stay below EUR 1 220, then full coverage is welfare improving; that is, it pays to target all farms and not only the largest emitters.
These results are subject to two main caveats. First, data and the calibration of the bio-economic model focus on four regions in Europe drawing on the database of the CAPRI model. The four farms chosen represent regional differences in crop and milk yields, crop-specific costs, milk-specific costs, mineral and organic fertiliser use, and dairy cow feeding practices. However, calibration of a bio-economic model also requires a relatively large amount of detailed bio-physical data and considering that these data were not available for certain regions, the analysis aims to illustrate the performance of policy instruments under heterogeneous productivity and profitability of production. Thus, results cannot be considered representative of either Europe or OECD countries in general. A modification of the bio-economic model structure is ongoing to facilitate the representation of diverse agricultural production structures and technologies, environmental conditions, and policy contexts in different OECD and non-OECD countries
Secondly, as always in supply-side farm-level models, the prices of outputs and inputs are considered exogenous. The assumption of exogenous output prices causes a large decrease in output, especially in dairy production, under those policy instruments that reduce the profitability of production. Since demand for agricultural products is inelastic, market prices for these products would increase when product supply decreases, and this would at least partly offset the decrease in profitability due to mitigation policies, moderating production and income losses.
Conclusions
There is increasing policy interest in exploring means to reduce GHG emissions in agriculture. Studies have shown there is a diversity of technical measures that farms could undertake with varying cost-effectiveness, but the policy levers to encourage their uptake has not been studied as much. This chapter addresses this gap by assessing the relative effectiveness and cost effectiveness of key GHG mitigation policy instruments in reducing emissions from crop and livestock (focusing on dairy) production. It looks at six policy instruments: an emission constraint, emission tax, abatement subsidy, input tax on nitrogen fertiliser, input tax on ruminant heads, and emissions trading, and applies these to the European Union. To do this, a detailed quantitative bio-economic farm model covering both production activities was developed and applied to farms representing a diversity of regional-level situations in Europe, drawing on data from the CAPRI database. On the basis of these data, four representative farms from four EU countries were developed to illustrate how differential crop and milk productivity and production costs affect the GHG mitigation effectiveness and costs of policies.
Consistent with other studies about GHG mitigation in European agriculture, the results show rather high abatement costs in mixed dairy and crop production, at least when targeting large GHG emission reduction. Study results confirm that the market-based instruments based on all GHG emissions (GHG emission tax, GHG abatement subsidy, and cap-and-trade scheme) are the most cost-effective options for GHG mitigation in agriculture. Moreover, results show it pays to target GHG emissions broadly, since the policy instruments that target all GHG emissions from farms are more cost-effective than the instruments targeting only a subset of emissions or proxies of emissions (e.g. input tax on nitrogen fertiliser or input tax on ruminant heads). This is the case even when higher policy-related transaction costs (related to monitoring, reporting and verification of emissions) are accounted for, in particular for a GHG emission tax and a GHG abatement subsidy.
The results underline the importance of investment costs and the planning horizon when evaluating GHG abatement strategies and costs in crop and dairy production. Investment costs lead to substantially different reactions by farms in the short and long run. In the short run, investment costs are sunk and farms continue dairy operations as long as market revenues exceed variable costs of milk production (including any GHG tax payments or abatement subsidies). Dairy farming is labour intensive, and the impact of sunk investment costs is intensified if farm labour input has low or zero opportunity costs (that is, no off-farm employment opportunities). As a result, in the short run, reductions in GHG emissions are likely to be modest. Long-run calculations assume that farms face fixed costs of investment in dairy production. Under this assumption, the mitigation effectiveness of policy instruments increases. This, however, varies across farm situations. For example, in the case of a GHG emission tax, some farms start reducing the number of dairy cows with the lowest emission tax level of EUR 9/ton of CO2eq. Other farms start to adjust the size of their dairy operation only when the emission tax is EUR 50/ton of CO2eq. The latter farms find it more profitable to pay a large amount of tax rather than to reduce GHG emissions by decreasing the number of dairy cows.
Fixed investment costs are thus likely to slow the transition to lower-carbon agriculture. Transition will take longer where fixed investment costs are higher and investments recent. This may call for temporary policy packages to facilitate transition when needed, or at least there is the need for governments to avoid uncertainty in their long-term GHG mitigation objectives and policies so that farmers can make appropriate investment decisions.
The availability of off-farm sources of income plays an important role in facilitating the development of mitigation solutions, as it helps to reduce the economic cost for farmers. Economic policies and conditions that facilitate job mobility and flexibility are likely to favour more effective mitigation policies.
The livestock sector is likely to be the most affected by mitigation policies. This has three major implications: this is where research on cost-effective mitigation practices and technologies will be needed; transition policy packages are needed for this sector; and competitiveness issues, while not discussed in this chapter, will be more important for the agriculture sector.
The effects of GHG mitigation policies may indirectly affect other environmental dimensions, such as the impact on water quality of nitrogen and phosphorus runoff through changes in input use (application of chemical fertiliser and manure) and land use (land allocation between cereals and grasslands). The results presented in this chapter show that land use change driven by mitigation policies from grasslands (grass silage and pasture) to cereals and oilseeds could increase nutrient runoff. This calls for considering ancillary benefits and trade-offs with regard to other environmental dimensions in the design of GHG mitigation policies in order to improve policy coherence. This study shows, in particular, that effective policy instruments for water quality will be important when introducing climate policies in regions where livestock is a major activity.
Overall, the results confirm that it is difficult to significantly reduce livestock GHG emissions without reducing dairy herd size. However, the real effect of reduced herd size is difficult to judge in a supply-side model as the one here. If demand is inelastic, resulting price increases could trigger production elsewhere and simply shift GHG emissions (emissions leakage) from one region to another. The type of detailed, farm-level analysis presented in this chapter must therefore be complemented with a large-scale market-equilibrium framework analysis.
References
[12] Ancev, T. (2011), “Policy Considerations for Mandating Agriculture in a Greenhouse Gas Emissions Trading Scheme: Reply”, Applied Economic Perspectives and Policy, Vol. 33/4, pp. 668-672, http://dx.doi.org/10.1093/aepp/ppr025.
[22] Bäckman, S., S. Vermeulen and V. Taavitsainen (1997), “Long-term fertiliser field trials: Comparison of three mathematical response models”, Agricultural and Food Science in Finland, Vol. 6, pp. 151-160.
[13] Bakam, I., B. Balana and R. Matthews (2012), “Cost-effectiveness analysis of policy instruments for greenhouse gas emission mitigation in the agricultural sector”, Journal of Environmental Management, Vol. 112, pp. 33-44, http://dx.doi.org/10.1016/j.jenvman.2012.07.001.
[2] Britz, W. and A. Leip (2009), “Development of marginal emission factors for N losses from agricultural soils with the DNDC–CAPRI meta-model”, Agriculture, Ecosystems & Environment, Vol. 133/3-4, pp. 267-279, http://dx.doi.org/10.1016/j.agee.2009.04.026.
[11] De Cara, S., L. Henry and P. Jayet (2018), “Optimal coverage of an emission tax in the presence of monitoring, reporting, and verification costs”, Journal of Environmental Economics and Management, Vol. 89, pp. 71-93, http://dx.doi.org/10.1016/j.jeem.2018.03.001.
[4] De Cara, S., M. Houzé and P. Jayet (2005), “Methane and Nitrous Oxide Emissions from Agriculture in the EU: A Spatial Assessment of Sources and Abatement Costs”, Environmental and Resource Economics, Vol. 32, pp. 551-583.
[36] De Cara, S. and P. Jayet (2000), “Régulation de l’effet de serre d’origine agricole : puits de carbone et instruments de second rang”, Économie & prévision, Vol. 3, pp. 37-46, https://www.persee.fr/doc/ecop_0249-4744_2000_num_143_2_6003.
[34] Durandeau, S. et al. (2009), “Coupling biophysical and micro-economic models to assess the effect of mitigation measures on greenhouse gas emissions from agriculture”, Climatic Change, Vol. 98/1-2, pp. 51-73, http://dx.doi.org/10.1007/s10584-009-9653-8.
[30] Finlex (2014), 1250/2014 Valtioneuvoston asetus eräiden maa- ja puutarhataloudesta peräisin olevien päästöjen rajoittamisesta, https://www.finlex.fi/fi/laki/alkup/2014/20141250.
[21] Grönroos, J. (2015), “Maatalouden ammoniakkipäästöjen vähentämismahdollisuudet ja kustannukset”, Ympäristöministeriön raportteja, Vol. 26.
[35] Grosjean, G. et al. (2016), “Options to overcome the barriers to pricing European agricultural emissions”, Climate Policy, Vol. 18/2, pp. 151-169, http://dx.doi.org/10.1080/14693062.2016.1258630.
[17] Huhtanen, P., M. Rinne and J. Nousiainen (2008), “Evaluation of concentrate factors affecting silage intake of dairy cows: a development of the relative total diet intake index”, animal, Vol. 2/06, http://dx.doi.org/10.1017/s1751731108001924.
[32] IPCC (2006), 2006 IPCC Guidelines for National Greenhouse Gas Inventories, https://www.ipcc-nggip.iges.or.jp/support/Primer_2006GLs.pdf.
[9] Kolari, P. et al. (2004), “Carbon balance of different aged Scots pine forests in Southern Finland”, gLOBAL cHANGE BIOLOGY, Vol. 10, p. 110661119.
[7] Lal, R. (2004), “Soil Carbon Sequestration Impacts on Global Climate Change and Food Security”, Science, Vol. 304/5677, pp. 1623-1627, http://dx.doi.org/10.1126/science.1097396.
[37] Lankoski, J. et al. (2015), “Environmental Co-benefits and Stacking in Environmental Markets”, OECD Food, Agriculture and Fisheries Papers, No. 72, OECD Publishing, Paris, https://dx.doi.org/10.1787/5js6g5khdvhj-en.
[18] Lehtonen, H. (2001), “Principles, Structure and Application of Dynamic Regional Sector Model of Finnish Agriculture”, MTT Economic Research Publications, Vol. 98.
[3] Lengers, B., W. Britz and K. Holm-Muller (2013), “Comparison of GHG-Emission Indicators for Dairy Farms with Respect to Induced Abatement Costs, Accuracy, and Feasibility”, Applied Economic Perspectives and Policy, Vol. 35/3, pp. 451-475, http://dx.doi.org/10.1093/aepp/ppt013.
[15] Lengers, B., W. Britz and K. Holm-Müller (2014), “What Drives Marginal Abatement Costs of Greenhouse Gases on Dairy Farms? A Meta-modelling Approach”, Journal of Agricultural Economics, Vol. 65/3, pp. 579-599, http://dx.doi.org/10.1111/1477-9552.12057.
[1] MacLeod, M. et al. (2015), “Cost-Effectiveness of Greenhouse Gas Mitigation Measures for Agriculture: A Literature Review”, OECD Food, Agriculture and Fisheries Papers, No. 89, OECD Publishing, Paris, https://dx.doi.org/10.1787/5jrvvkq900vj-en.
[10] McCann, L. et al. (2005), “Transaction cost measurement for evaluating environmental policies”, Ecological Economics, Vol. 52/4, pp. 527-542, http://dx.doi.org/10.1016/j.ecolecon.2004.08.002.
[25] Myyrä, S. et al. (2005), “Land improvements under land: The case of pH and phosphate in Finland”, Land Economics, Vol. 81, pp. 557-569.
[19] Nennich, T. et al. (2005), “Prediction of Manure and Nutrient Excretion from Dairy Cattle”, Journal of Dairy Science, Vol. 88/10, pp. 3721-3733, http://dx.doi.org/10.3168/jds.s0022-0302(05)73058-7.
[5] OECD (2016), Effective Carbon Rates: Pricing CO2 through Taxes and Emissions Trading Systems, OECD Publishing, Paris, https://dx.doi.org/10.1787/9789264260115-en.
[33] Opio, C. et al. (2013), Greenhouse gas emissions from ruminant supply chains – A global life cycle, Food and Agriculture Organization of the United Nations (FAO), Rome, http://www.fao.org/3/i3461e/i3461e.pdf.
[27] OSF (2014), Official Statistics of Finland: Farm Structure Survey, Agricultural Census 2010, http://stat.luke.fi/e-lehti-kotielaimet/ (accessed on 20 September 2017).
[31] OSF (2010), Official Statistics of Finland: Producer Prices of Agricultural Products, http://stat.luke.fi/en/producer-prices-of-agricultural-products (accessed on 20 September 2017).
[29] Palva, R. (2015), Konetyön kustannukset ja tilastolliset urakointihinnat, https://docplayer.fi/16108419-Konetyon-kustannukset-ja-tilastolliset-urakointihinnat.html.
[16] Pérez Domínguez, I., W. Britz and C. Wieck (2003), “Modelling of passive environmental indicators for European agriculture: The role of marginal abatement costs”. Paper contributed to the 12th Annual Conference of the EAERE, Bilbao, Spain.
[14] Perez Dominguez, I. and W. Britz (2003), “Reduction of global warming emissions in European agriculture through a tradable permit system: An analysis with the regional agricultural model CAPRI”, Schriften der Gesellschaft für Wirtschafts- und Sozialwissenshaften des Landbaus e., Vol. 39, pp. 283-290.
[6] Rogelj, J. et al. (2015), “Energy system transformations for limiting end-of-century warming to below 1.5 °C.”, Nature Climate Change, Vol. 5, pp. 519-527, http://dx.doi.org/10.1038/nclimate2572.
[26] Rude, S. (ed.) (1991), Estimation of nitrogen leakage functions: Nitrogen leakage as a function of nitrogen applications for different crops on sand and clay soils, Institute of Agricultural Economics, Copenhagen.
[8] Smith, P. et al. (2015), “Biophysical and economic limits to negative CO2 emissions”, Nature Climate Change, Vol. 6/1, pp. 42-50, http://dx.doi.org/10.1038/nclimate2870.
[20] Statistic Finland (2016), Greenhouse gas emissions in Finland 1990–2014: National inventory report under the UNFCCC and the Kyoto protocol.
[28] Tuottopehtori (2017), “Tuottopehtori-hakemisto” (in Finnish), https://www.webwisu.fi/tuottopehtori/index.php?year=2014&locale=fi (accessed on 20 September 2017).
[24] Uusitalo, R. (2004), “Potential bioavailability of particular phosphorus in runoff from arable clay soils”, Agrifood Research Reports, Vol. 53/Doctoral Dissertation, MTT Agrifood Research Finland, Jokioinen, MTT Agrifood Research Finland.
[23] Uusitalo, R. and H. Jansson (2002), “Dissolved reactive phosphorus in runoff assessed by soil extraction with an acetate buffer”, Agricultural and Food Science, Vol. 11/4, pp. 343-353, http://dx.doi.org/10.23986/afsci.5734.
Annex 3.A. Key parametric equations of the empirical model
This annex provides a brief description of the key parametric equations of the model.
Total intake function of dairy cows, kg DM/animal/year
In the intake function v denotes concentrate feed intake, and silage intake is given be intake(v) – v. Intake function is from Huhtanen et al. (2008[17])
Milk production function, kg/animal/year
The quadratic milk production function is based on Lehtonen (2001[18]). By assumption each cow has 300 milking days and cows are dry the rest of the year. Milk production peaks roughly at 16-17 kg of the concentrate feeding.
Manure excretion to cowshed, m3/animal/year
To determine the manure excretion, one needs to define the following shares of animals in the farm. A notion of production animal refers to the steady-state process needed to maintain one lactating cow and is technically a composition of one lactating cow, 1/3 calf and 1/3 heifer. Thus,
where is the number of milking seasons before a dairy cow is slaughtered, and the ending D stands for dairy cows, H for heifers and C for calves. Using this notation, the manure excretion to cowshed and pasture, respectively, can be defined as follows (Nennich et al., 2005[19]):
Manure excretion to cowshed, m3/production animal/year
Manure excretion to pasture, m3/ production animal/year
Manure N content
To determine the manure N content, one needs to account for the share of manure N evaporated as NH3-N. This evaporation is affected by manure storage and spreading technologies and defined as , where and . Manure N content, kg N/m3 manure/year (ThetaN) and total N excretion in manure, kg N/animal/year (Nexcr), respectively, are based on Nennick et al. (2005[19]) and given by
Manure transport and application cost
Costs for manure spreading and transportation are determined based on the distance, amount of manure, spreading technology, gear capacity, and contractor charge as follows.
where , is m3/manure and is distance in km.
CH4 emissions from enteric fermentation
Methane emissions are calculated applying a procedure from GHG inventory calculations that follow IPCC’s recommendations. Calculation is based on the following set of equations, which also account for the possible abatement through fat supplementation. Equations are based on the inventory reporting of Statistic Finland (2016[20]). When estimating CH4 emissions from enteric fermentation, the diet’s gross energy digestibility is calculated only for dairy cows, and the same value is used for calves and heifers for simplification. The same set of equations are thus used for calculating the emissions for dairy cows, heifers and calves. Dry cows are not accounted for separately.
, where
(only for dairy cows)
(only for dairy cows)
, where is the share of digestible organic matter of the total organic matter as g/kg DM
GHG emissions from manure storage
Manure storage is a source of both methane and nitrous dioxide emissions. They are defined using the following equations.
CH4 emissions from storage, kg CH4/animal/year (based on Statistic Finland (2016[20])
where is storage without cover and is storage with floating cover
Direct N2O emissions from storage, kg N2O/animal/year (based on Statistic Finland (2016[20])
where is storage without cover and is storage with floating cover
GHG emissions from manure management
Manure storage and spreading cause NH3 emissions and based on those indirectly N2O emissions. Drawing on Statistic Finland (2016[20]) and Grönroos (2015[21]) they can be expressed using the following equations.
NH3 emissions from manure management, kg NH3-N/m3 manure/year
Indirect N2O emissions from manure managements, kg N2O/m3 manure/year
Emissions from fertiliser use, machinery and soil (kg CO2eq./ha) from cultivated land
The GHG emissions from cultivated land comprise autonomous soil emissions (soil N2O emissions due to fertilization are assumed to be included here, i.e. they are not accounted for separately) and emissions from cultivation practices, yield transportation to processing, crop drying and manufacturing mineral fertilisers.
where X is {s, c}={silage, barley}
Emissions from fertiliser use, machinery and soil (kg CO2eq./ha) from pasture land
The GHG emissions from pasture land are calculated based on Statistic Finland (2016[20]) with additional terms for autonomous soil emissions, cultivation practices and mineral fertiliser manufacture.
Crop yield response functions
The crop nitrogen response function for rape seed and silage (kg DM yield/ha) is given by quadratic response function
where a, b and c are parameters of a quadratic nitrogen response function. And for wheat and barley by Mitscherlich nitrogen response function
where and φ, σ and ρ are parameters of a Mitscherlich response function. The parameters of the quadratic crop yield functions are taken from Lehtonen (2001[18]) and those of the Mitscherlich yield function have been estimated by Bäckman et al. (1997[22]) on the basis of Finnish field experiments.
Nitrogen and phosphorus runoff functions
Both nitrogen and phosphorus runoff are included and in the case of phosphorus both dissolved reactive phosphorus (DRP) and particulate phosphorus (PP) runoff is estimated. Because in compound fertiliser (NPK) the three main nutrients are in fixed proportions, nitrogen fertiliser intensity determines also the amount of phosphorus used. Part of this phosphorus is taken up by the crop, while the rest accumulates and builds up soil P. Drawing on Finnish field experiment studies it is assumed that 1 kg increase in soil phosphorus reserve increases the soil P status (i.e. ammonium acetate-extractable P) by 0.01 mg/l soil. Uusitalo and Jansson (2002[23]) estimated the following linear equation between soil P and the concentration of dissolved phosphorus (DRP) in runoff: water soluble P in runoff (mg/l) = 0.021*soil_P (mg/l soil) – 0.015 (mg/l). The surface runoff of potentially bioavailable particulate phosphorus is approximated from the rate of soil loss and the concentration of potentially bioavailable phosphorus in eroded soil material as follows: potentially bioavailable particulate phosphorus PP (mg/kg eroded soil) = 250 * ln [soil_P (mg/l soil)]-150 (Uusitalo, 2004[24]). Thus, the parametric description of surface phosphorus runoff is given by
For particulate phosphorus PP runoff function, ζi is erosion rate (kg/ha), θ the amount of soil phosphorus (mg/l). Soil_P is fixed at 10.6 mg/l, which is the average for Finnish FADN farms situated in southern and south-western Finland (Myyrä et al., 2005[25]).
For DRP runoff function ψ is the amount of surface runoff (mm/ha). Pi is in both equations the phosphorus application rate (kg/ha). Runoff and erosion differ between different tillage methods (no-till versus conventional tillage) or land cover types (grasslands versus cereals) and technology specific factors, αi and βi, describe the distinctive characters of the different tillage methods and land cover types.
For nitrogen runoff following runoff function, estimated by Simmelsgaard (1991[26]), is employed
where
= nitrogen runoff at fertiliser intensity level li, kg/ha, = nitrogen runoff at average nitrogen application,
and
are constants and li = nitrogen fertilization in relation to the normal fertiliser intensity for the crop, 0.5 ≤ N ≤ 1.5. This runoff function represents nitrogen runoff generated by a nitrogen application rate of li per hectare, and the parameter reflects differences in tillage methods and land cover types.
Annex 3.B. List of parameter values
Parameter |
Symbol |
Value |
Reference |
---|---|---|---|
Market price, EUR/kg |
|
||
Milk |
pM |
0.4455 |
|
Concentrate, domestic |
pv |
0.183 |
|
Concentrate, soybean meal |
psoy |
0.3507 |
IndexMundi (2014) |
Mineral fertiliser, YaraMila Y2 |
pl |
0.45 |
Tuottopehtori (2014) |
Meat |
pmeat |
2.1 |
Tuottopehtori (2014) |
Calf (selling), EUR/animal |
pcalf |
115 |
Tuottopehtori (2014) |
Mineral fertiliser, YaraMila Y2 |
|||
N-content |
eN |
0.24 |
Tuottopehtori (2014) |
P-content |
eP |
0.04 |
Tuottopehtori (2014) |
Variable cost in barley production,EUR/kg |
hC |
0.056 |
Tuottopehtori (2011) |
Variable costs in silage production |
|||
EUR/kg yield |
h0 |
0.0918 |
Tuottopehtori (2014) |
Silage dry matter % |
dmpc |
25 |
|
Silage density, kg/m3 |
dens |
250 |
|
Loading capacity, m3 |
trcap |
20 |
|
Transport speed, km/h |
trsp |
15 |
|
Transport price, EUR/h |
trp |
63.1 |
|
Cost of floating storage cover, EUR/m2/year |
float |
2 |
|
Capacity of manure spreader, m3 |
spcap |
16 |
Palva (2015) |
Contractor charge for spreading,E UR/h |
|||
Broadcast spreading |
spp1 |
77.9 |
Palva (2015) |
Injection |
spp2 |
102.5 |
Palva (2015) |
Time for loading, h/m3 |
load |
0.004 |
|
Time for spreading, min/m3 |
|||
Broadcast spreading |
spread1 |
0.5 |
|
Injection |
spread2 |
1.5 |
|
Transport cost interrelated to spreading, EUR/m3/km |
ctran |
0.4 |
Palva (2015) |
Damage from GHG emissions, EUR/kg CO2eq. |
?G |
0.05 |
|
Animal body weight, kg |
|||
Dairy cow |
BWD |
600 |
|
Heifer |
BWH |
400 |
VTT (2000) |
Calf |
BWC |
150 |
VTT (2000) |
Number of milking seasons |
slaught |
3 |
|
Number of dairy cows |
H |
60 |
Chosen |
Share of animals per dairy cow |
|||
Dairy cow |
shareD |
1 |
|
Heifer |
shareH |
1/3 |
|
Calf |
shareC |
1/3 |
Parameter |
Symbol |
Value |
Reference |
---|---|---|---|
Total intake, kg DM/animal/day |
|
||
Heifer |
inH |
5 |
|
Calf |
inC |
10 |
|
Share of concentrates in H and C diet, % |
50 |
||
Manure excretion, m3/animal/year |
|||
Heifer |
wH |
8.5 |
|
Calf |
wC |
6.25 |
Finlex 1250/2014 |
Water in liquid manure, m3/animal/year |
h2o |
10 |
|
Manure density, kg/m3 |
kgm3 |
1000 |
|
Share of manure excreted on pasture |
wp |
0.15 |
|
Scaling factor to match Finnish statistics |
scale |
0.65 |
Chosen |
Parameter for manure excretion |
w0 |
9.4 |
|
Parameter for manure excretion |
w1 |
2.63 |
Nennich et al. (2005) |
Parameter for manure N content |
N1 |
84.1 |
Nennich et al. (2005) |
Parameter for manure N content |
N2 |
0.196 |
Nennich et al. (2005) |
Feed nutrition values |
|||
Concentrate (barley 54-62 kg/hl) |
v |
||
Dry matter, g/kg |
cka |
860 |
|
Organic matter, g/kg DM |
coa |
971 |
|
Organic matter digestibility |
coas |
0.82 |
|
Crude protein content of DM |
vcp |
0.126 |
|
P content of DM |
vp |
0.0041 |
|
Concentrate (soybean meal) |
v |
||
Dry matter, g/kg |
cka |
880 |
|
Organic matter, g/kg DM |
coa |
821 |
|
Organic matter digestibility |
coas |
0.88 |
|
Crude protein content of DM |
vcp |
0.520 |
|
P content of DM |
vp |
0.007 |
|
Silage feed (grass silage) |
s |
||
Dry matter, g/kg |
ska |
1000 |
|
Organic matter, g/kg DM |
soa |
911 |
|
Organic matter digestibility |
soas |
0.74 |
|
Crude protein content of DM |
scp |
0.161 |
|
P content of DM |
sp |
0.0031 |
Parameters for nitrogen response functions |
Symbol |
Value |
Reference |
---|---|---|---|
Quadratic response function for silage |
a |
1182.9 |
Bäckman et al. (1997) and Lehtonen (2001) |
b |
24.24 |
||
c |
-0.0394 |
||
Quadratic response function for rape seed |
a |
890.0 |
|
b |
9.95 |
||
c |
-0.0354 |
||
Mitscherlich response function for wheat |
φ |
4956 |
|
σ |
0.7624 |
||
ρ |
0.011 |
||
Mitscherlich response function for barley |
φ |
5218 |
|
σ |
0.8280 |
||
ρ |
0.017 |
Parameters for GHG emissions |
Symbol |
Value |
Reference |
---|---|---|---|
Conversion factors |
|
||
N2O to CO2eq. |
N2O |
298 |
|
CH4 to CO2eq. |
CH4 |
21 |
|
Enteric fermentation |
|||
Coefficients |
|||
Dairy cow |
CfiD |
0.379 |
|
Heifer |
CfiH |
0.322 |
Statistics Finland (2016) |
Calf |
CfiC |
0.322 |
Statistics Finland (2016) |
Pasture |
cap |
0.17 |
Statistics Finland (2016) |
Stall |
cao |
0.00 |
Statistics Finland (2016) |
Pregnancy (dairy cows) |
CpD |
0.10 |
Statistics Finland (2016) |
Growth |
|||
Dairy cow |
CoD |
0.00 |
Statistics Finland (2016) |
Heifer |
CoH |
0.80 |
Statistics Finland (2016) |
Calf |
CoC |
1.00 |
Statistics Finland (2016) |
Average weight gain, kg/day |
|||
Dairy cow |
WGD |
0.05 |
Statistics Finland (2016) |
Heifer |
WGH |
0.45 |
Statistics Finland (2016) |
Calf |
WGC |
0.90 |
Statistics Finland (2016) |
Pasture season, days |
|||
Dairy cow |
tpD |
125 |
|
Heifer |
tpH |
135 |
OSF (2010) |
Calf |
tpC |
115 |
OSF (2010) |
Milk fat content, % |
fat |
4.3 |
Statistics Finland (2016) |
CH4 conversion rate |
Ym |
0.065 |
Statistics Finland (2016) |
Manure storage |
|||
No cover |
|||
Emission factor N2O |
ef1 |
0 |
Statistics Finland (2016) |
Emission factor CH4 |
mcf1 |
0.17 |
Statistics Finland (2016) |
Manure N evaporated as NH3, % |
emstor1 |
10 |
Grönroos (2014) |
Floating cover |
|||
Emission factor N2O |
ef2 |
0.005 |
Statistics Finland (2016) |
Emission factor CH4 |
mcf2 |
0.10 |
Statistics Finland (2016) |
Manure N evaporated as NH3, % |
emstor2 |
6 |
|
Manure ash content |
ash |
0.08 |
|
Max. CH4 producing capacity, m3/kg vs |
chmax |
0.24 |
Statistics Finland (2016) |
Manure spreading |
|||
Broadcast spreading |
|||
Manure N evaporated as NH3, % |
emspread1 |
40 |
Grönroos (2015) |
Injection |
|||
Manure N evaporated as NH3, % |
emspread2 |
9 |
Grönroos (2015) |
Autonomous soil emissions, kg CO2eq./ha |
|||
Barley |
autoc |
1535 |
|
Silage |
autos |
426 |
|
Pasture |
autop |
1535 |
|
Cultivation practices, kg CO2eq./ha |
|||
Barley |
cultc |
362 |
|
Silage |
cults |
136.5 |
|
Pasture |
cultp |
362 |
|
N applied to pasture land, kg N/ha |
lp |
220 |
Parameter |
Symbol |
Value |
Reference |
---|---|---|---|
Other parameters |
|
||
Yield transport to processing, kg CO2eq./ha |
emtrans |
0.00696 |
|
Crop drying, kg CO2eq./kg yield |
emdry |
0.028 |
|
Mineral fertiliser manufacture, kg CO2eq./kg N |
emprod |
4.32 |
|
Soybean meal manufacture, kg CO2eq./kg |
emsoy |
5.35 |
|
Parameters for nutrient functions |
|||
Constant |
b0 |
-0.7 |
(Simmelsgaard, 1991[26]; Uusitalo and Jansson, 2002[23]; Uusitalo, 2004[24]) |
Constant |
b |
0.7 |
|
Average runoff from fertilisation |
ϖ |
15 |
|
Erosion |
ζ |
800 |
|
Surface runoff |
Ψ |
234 |
|
Soil phosphorus |
θ |
10.6 |
|
Phosphorus rate |
P |
0.143 |
|
Technology factor, PP |
α |
2.4 |
|
Technology factor, DRP |
β |
0.77 |
Notes
← 1. (Durandeau et al., 2009[34]) also adopt non-linear yield functions in their analysis of the first-best and the second-best taxation of GHG emissions from agriculture in northern France.
← 3. As an agricultural sector model, CAPRI combines a global partial equilibrium model for agri-food products (employing the Armington assumption to depict bi-lateral trade) with non-linear programming models for 280 NUTS2 regions, or about 3 000 farm group models, to detail agricultural production decisions in the European Union, EU candidate countries and Norway. Eurostat is the key data source for the European part of the model providing, for instance, crop and animal production statistics, land use statistics, market balances and Economic Accounts for Agriculture (EAA). The CAPRI database at national level integrates the EAA (valued output and input use) with, for instance, market balances, and trade and production statistics. The country data are subsequently used to derive a regionalised database at NUTS2 level that depicts the allocation of inputs across activities and regions, as well as acreages, herd sizes and yields. The regional data are subsequently further disaggregated to farm-type level, mainly based on farm structural data.
← 4. CAPRI is used in other GHG mitigation studies as well. For example, Pèrez Dominguez et al. (2003) derive marginal abatement costs of GHG emissions from CAPRI at regional level for analysing an EU-wide trading scheme of GHG emission permits for agriculture while explicitly including transaction costs in permit trading.
← 5. Terminology used referring to low and high milk and crop yields is relative to other farms modelled.
← 6. Farmers are assumed to be risk-neutral, in which case fully decoupled support does not affect farmer choices regarding input use and land use.
← 7. Their impact will be illustrated later using a sensitivity test, assuming that all fixed costs are sunk instead of only 50% of fixed costs of investment, reflecting a situation where the farm would continue dairy operation as long as market revenues exceed the variable costs of milk production (including taxes).
← 8. Other calculations assume that farmers have off-farm employment opportunities with wage-rate of EUR 13/hour. However, off-farm income is not reported in any of the result tables as it is important to show how farm income changes due to adjustments driven by policy instruments.
← 9. If farmers have off-farm employment opportunity and they use saved labour input from farming (e.g. due to reduction in dairy herd size) to work part-time off-farm to earn off-farm income, the forgone income (sum of farm income and off-farm income) at a given emission constraint level would not reflect fully the cost of adjustments in agriculture due to emission constraint.
← 10. Entitled “Les exploitations d’elevage herbivore economes en intrants (ou autonomes): quelles sont leurs caracteristiques? Comment accompagner leur developpement?”
← 11. (Grosjean et al., 2016[35]) discuss potential barriers to pricing agricultural GHG emissions in Europe. Since transaction costs also depend on the existing institutional frameworks and because agriculture sector is already regulated (such as the Nitrate directive) and subsidised through the Common Agriculture Policy (CAP), various monitoring, reporting, and verification tools are already in place, which would help to implement GHG emissions based policy instruments.
← 12. (De Cara and Jayet, 2000[36]) show that taxation of animals and their feed, the second-best policies for methane reduction, produces significant results in terms GHG emission reductions. However, their analysis finds subsidy for afforestation of set-aside land to be even more effective.
← 13. Carbon sequestration is dealt relatively briefly here as it will be analysed in the synthesis report of the whole project. Moreover, soil carbon sequestration and voluntary provision of carbon offsets to carbon credit markets have been analysed in detail in (Lankoski et al., 2015[37]).
← 14. In addition to afforestation of agricultural land there are other options, which have less impact on the amount of agricultural land, such as agroforestry or planting trees on field boundaries.
← 15. Sunk cost refers to a cost that has been incurred and cannot be recovered. Thus, it should not affect rational decision-making.
← 16. Marginal incentives and cost-effectiveness are same for these three instruments. They do differ in terms of distributional impacts (farm income and government net-revenues).