Michail Moatsos
Utrecht University
How Was Life? Volume II
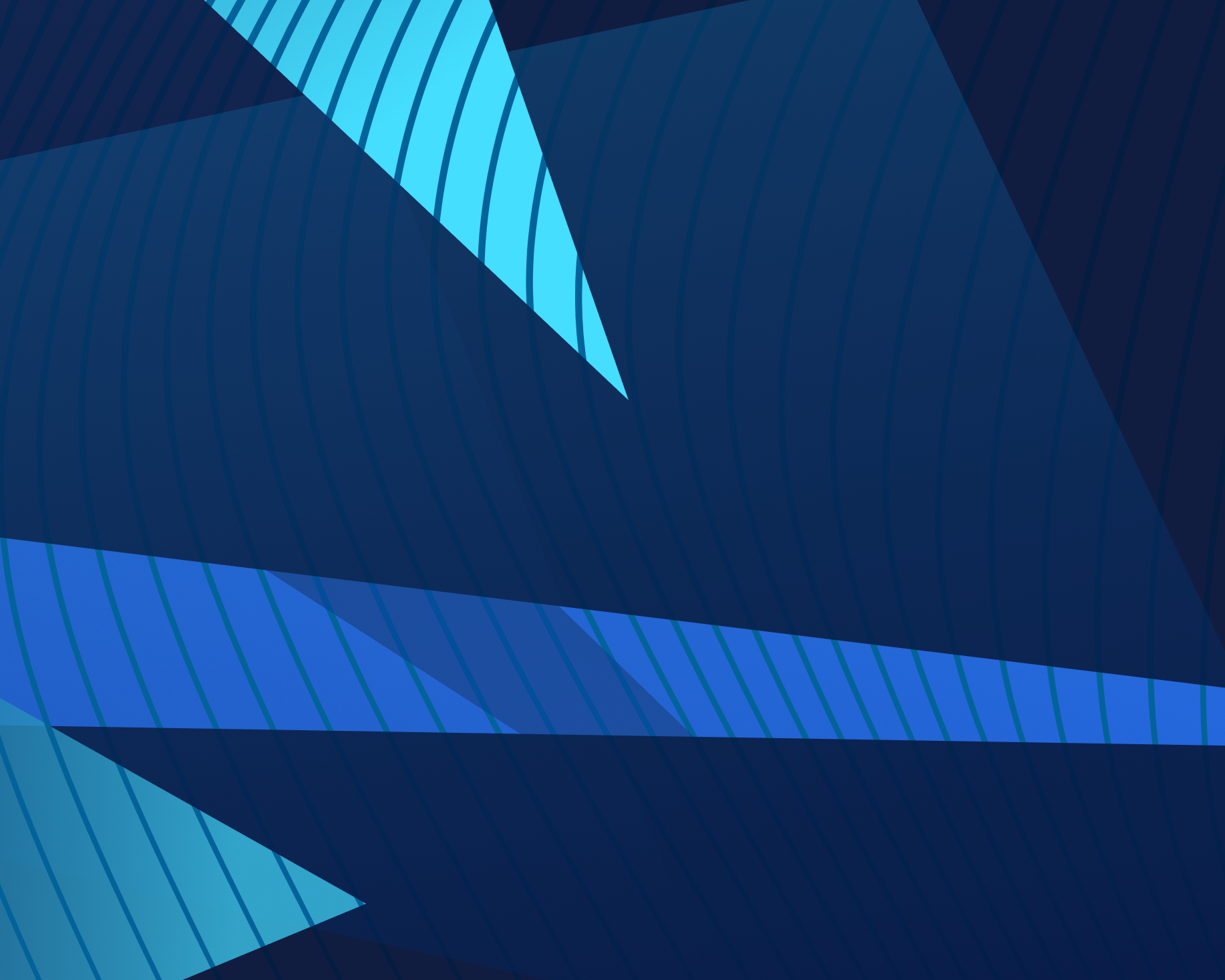
9. Global extreme poverty: Present and past since 1820
Abstract
This chapter relies on a global data set on basic commodity prices to provide first estimates of global extreme poverty in the long run using a “cost of basic needs” approach.1 For 135 years since 1820, more than half of the global population lived in conditions of extreme poverty. It took another 46 years to cut this rate in half, which only happened as recently as 2001. In the years that followed, the reduction of extreme poverty accelerated tremendously, and in 13 more years the global poverty rate was halved again. Compared to other available estimates, the world in the 19th century was less poor than we had thought, but poorer in the more recent period. Notably, the total number of people living in conditions of extreme poverty in 1820 stands at 757 million, which is almost identical with the count two centuries later in 2018, at 764 million.
Introduction
Economic growth has spread throughout the planet over the last two hundred years with unprecedented speed, leading to improvements in many well-being indicators, albeit at variable rates (van Zanden et al., 2014[1]). However, economic growth does not necessarily improve the well-being of all people within a country at the same rate, and some may miss the tide altogether. This can be the case when economic inequality within a country rises with, for example, low incomes stagnating and top incomes increasing.
This chapter focuses on the evolution of extreme poverty during the period 1820-2018.2 Only a handful of attempts have been made to estimate global extreme poverty in the short and medium run (Bhalla, 2002[2]; Bourguignon and Morrisson, 2002[3]; Sala-i-Martin, 2006[4]; Chen and Ravallion, 2010[5]; Ferreira et al., 2015[6]), and even fewer in the long run (Bourguignon and Morrisson, 2002[3]; Van Zanden et al., 2011[7]). All these approaches rely on a single value of the poverty line for all countries and for all years, an approach that many scholars find insufficient (Deaton, 2001[8]; Srinivasan, 2009[9]; Reddy and Pogge, 2010[10]; Atkinson, 2016[11]; Allen, 2017[12]).
The goal of this chapter is to provide global, regional and country estimates of a specific measure of extreme poverty for (almost) all present-day countries of the world as if they were sovereign in 1820. The first step in this exercise is to choose a definition of poverty. This chapter relies on the cost of basic needs (CBN) approach used by Allen (2017[12]). In this approach, poverty lines are calculated for every year and country separately, rather than using a single global line. The second step is to gather the necessary data to operationalise this approach, alongside imputation methods in cases where not all the necessary data are available. The third step is to devise a method for aggregating countries’ poverty estimates on a global scale to account for countries that lack some of the relevant data.
The estimates presented in this chapter show that between 1820 and 2018 the prevalence of extreme poverty across the globe fell from 76% to 10%, the lowest level ever achieved, according to our method. This reduction, however, is not distributed evenly throughout the period. It took 136 years from 1820 for our global poverty rate to fall under 50%, then another 45 years to cut this rate in half again by 2001. In the early 21st century, global poverty reduction accelerated, and in 13 years our global measure of extreme poverty was halved again by 2014. Whether this reduction has been sufficient to meet the 50% poverty reduction target set by the first Millennium Development Goal across the developing world between 1990 and 2015 depends decisively upon the treatment of data for pre-1995 China.3 According to the baseline calculations presented in this chapter, the MDG1 poverty reduction target has been met.
By operationalising Allen’s approach on a long-run global scale, this chapter moves beyond the dollar-a-day method towards an approach that provides a set of transparent poverty lines in every year and every country. Arguably, understanding what USD 1.9 at 2011 Purchasing Power Parity (PPP) rates (hereafter simply international dollars) would buy in each country and each year is far from obvious, while the cost of basic needs approach is easily understood by scholars and laymen alike. The cost of basic needs method was recommended by the World Bank Commission on Global Poverty, presided by the late Sir Tony Atkinson, as an alternative (or complementary) method in monitoring poverty for the needs of the United Nations Sustainable Development Goals (Atkinson, 2016[11]).
The following section describes the methodology in some detail. The two sections thereafter discuss the historical sources used and assess data quality, respectively. These are followed by a description of key trends in global extreme poverty since 1820, and of the correlation between these poverty estimates with GDP per capita. The last section conclude by sketching priorities for future research.
Description of the concepts used
All poverty measures require a yardstick (a poverty line) to distinguish those living in conditions of poverty from the rest of the population. The most well-known poverty line in global poverty measurement is the USD 1 a-day line adopted by the World Bank. This measure was originally expressed in 1985 prices and has been updated since then for each new round of PPP estimates by the International Comparison Programme (ICP). Its origin can be traced to Ravallion et al. (1991[13]), who gathered data on poverty lines in national currencies for 33 developing and developed countries. In order to make these national poverty lines comparable across countries, Ravallion converted them to a common currency based on PPP exchange rates provided by Summers and Heston (1988[14]). These estimates showed that the poverty line of the group of low-income countries considered clustered around a value of USD 1 a-day.
Several scholars have expressed concerns about the soundness of these global poverty estimates (Deaton, 2001[8]; Srinivasan, 2009[9]; Atkinson, 2016[11]; Reddy and Pogge, 2010[10]) or about their large margins of error (Atkinson, 2016[11]; Moatsos, 2018[15]). These criticisms mainly focus on the use of PPP exchange rates to estimate the equivalent income of every person on the planet in a single currency and, in particular, on the method used to derive the dollar-a-day poverty line. On the first point, the problem is that a single poverty line may not represent equivalent welfare in different countries and across time (Reddy and Pogge, 2010[10]; Subramanian, 2015[16]; Moatsos, 2017[17]). Reddy and Pogge (2010[10]) argued in favour of a “basic needs approach” in order to resolve the issues raised by the application of PPPs. In response to this criticism, Ravallion (2010[18]) argued that poor people may adapt their consumption habits following changes in market price, implying that the cost of a fixed consumption basket may exceed the cost actually paid for this basket by those living in poverty. Allen (2013[19]), while recognising this substitution effect, argued that this effect is overstated, and advocated the use of linear programming techniques (to estimate the least costly basket of goods) to account for this adaptation to price changes.
Estimates of extreme poverty at the global level are generally produced using data on the distribution of household income or consumption expenditure (often adjusted using non-survey information on mean income or consumption) and a single international poverty line denominated in PPP dollars (Bourguignon and Morrisson, 2002[3]; Bhalla, 2002[2]; Sala-i-Martin, 2006[4]; Chen and Ravallion, 2010[5]; Van Zanden et al., 2011[7]; Ferreira et al., 2015[6]). Recently, Allen, (2017[12]) estimated extreme poverty using linear programming techniques to identify the cost of a diet sufficient to provide a minimum nutritional content, to which additional expenses for housing, clothing and heating are then added. Allen tests four different setups for such minima: 1) a caloric minimum of 1 700 kcal per day; 2) a caloric-protein-fat minima (dubbed CPF) targeting 2 100 kcal, 50g of protein and 34g of fat; 3) a “basic diet” that adds half the quantities of additional nutrients recommended by the Indian daily allowances (RDA), namely iron, folate, thiamine, niacin and vitamins C and B12; and 4) a full-course model that adds 6 more vitamins and minerals to the basic diet. Allen concludes that the “basic diet” (option 3) is the more reasonable food standard, since “people eating a CPF diet suffer many nutritional deficiencies”, while the full-course model implies unreasonably high quantities of foodstuff to be consumed, and very high caloric intake.4
This chapter relies on a “basic diet” poverty line calculated separately for every country and year. However, as the data needed to apply linear programming to estimate a “basic diet” poverty line on a global scale and on the long run are not available,5 The second-best approach used in this chapter is based on the price data from the ILO October Inquiry (1924-2008).6 This approach applies linear programming on the ILO data to first calculate the CPF poverty line, which is then multiplied by the coefficient implied by Allen’s results to get a proxy of the “basic diet” poverty line.7 When price level data from the ILO are not available for a particular country and year, the chapter follows the general practice, common to the dollar-a-day approach, of using the national consumer price index (CPI) to extend over time these estimates of the basic diet poverty line.
The types of expenses considered by options 1) to 3) above refer only to the food expenses. To estimate the costs of the final “basic diet” poverty line, additional coefficients expressing the share of food in total household consumption (coefficients that change with the GDP per capita of each country) are estimated from Allen’s results in order to move from a “food” to a “full-line” approximating the expenses that people living in extreme poverty in each country and year have to incur.
Historical sources
Four main types of data are required to produce global estimates of extreme poverty with a cost of basic needs approach: 1) a set of prices for different consumption goods (supplemented by general price indices when needed); 2) a set of data on the distribution of consumption or income in each country; 3) a set of population data; and 4) a set of nutrients to be assigned to the various foodstuffs in the price database. The nutrient contents are taken from the US Department of Agriculture (USDA) website (2019[20]), while the population data are from the Maddison Project (Bolt and van Zanden, 2014[21]), complemented, whenever necessary, by data from the United Nations World Population Prospects (2019[22]). The sources for the remaining data are discussed below.
Prices from the ILO October inquiry
Since 1924, the International Labor Organization (ILO) has collected prices for basic foodstuffs and necessary consumables such as bread, rice and fat products in types typically purchased by working families. This data collection started with a small set of 16 capital cities surveyed every October, which grew to cover 130 countries by 1967, dropped to 79 countries in 1983 and then increased again to about 120 countries. The product listings included 15 items in the early years, which incrementally increased to cover 39 main products by 1984. The ILO stopped the collection of prices in 2008 due to a re-organisation of its activities.8
A long process of digitising these price data has been undertaken by de Zwart, van Leeuwen and van Leeuwen-Li (2014[23]) using manual entries and Optical Character Recognition (OCR) techniques; in addition, the estimates in this chapter rely on an independent digitisation using a manually assisted OCR approach undertaken by the author. When the two approaches provide different values, the data were investigated for possible errors; when the two approaches gave the same result, digitisations were assumed to be valid.
The October Inquiry commodity price dataset offers data points spanning a period of at least 80 years for 18 countries, and more than 50 years for 108 countries. These data were reported to the ILO by the national statistical agencies of each country, which were instructed to report prices that were relevant for working families. This makes this dataset particularly useful for the calculation of the costs of basic needs poverty lines. As with all historical data, the ILO dataset has its problems. For example, one needs to be careful with currencies before and after a currency redenomination takes place in a country.9 Another issue is that the October Inquiry dataset refers to prices in a single month, implying that, when inflation is high, these price data may not be representative of the full year. For high inflation years, e.g. in Brazil or Zimbabwe, the estimates in this chapter rely on changes in the average CPI to estimate the poverty line, rather than on the ILO price data. In addition, some ILO entries are clearly too low or too high, by a couple of orders of magnitude, relative to the price entries for the same products for that country in a nearby year; those prices were also removed from the final dataset.10
Moreover, prices for all products are not reported for all countries at all times. This means that one needs to impute these missing data using either the CPI index of that country or, when this is also not available, the evolution of similar prices in the ILO dataset.11 Such an imputation, however, may result in prices that are distant from those actually paid by working families. For example, the prices of the cheapest product providing a given number of calories may follow a different trajectory than the average CPI of a country, and after, say, 10 years one may have a price that is much higher or much lower than the unobserved one. This is an important limitation of our approach.
The same applies for years outside the 1924-2008 period, where the cost of the food component is extrapolated using available consumer price indices. This imputation rests on the assumption that, on average, the cost of the food component does not substantially diverge from the average CPI. This is a strong assumption, but is the same used by the dollar-a-day approach. More work to gather detailed price data will be required to extend the coverage of our price dataset to the full time period covered by this chapter.
Nevertheless, the use of detailed nominal prices brings the methodology of measuring extreme poverty one step closer to the experience of people living in poverty across the world. It removes one layer of assumptions, compared to other approaches that rely on one single poverty line expressed in PPPs, or that rely on a CPI index for moving the poverty line in time for about 20, 50 or 170 years (Bourguignon and Morrisson, 2002[3]; Bhalla, 2002[2]; Sala-i-Martin, 2006[4]; Chen and Ravallion, 2010[5]; Van Zanden et al., 2011[7]; Ferreira et al., 2015[6]).
Panel A in Figure 9.1 shows the number of CPF food components calculated from the complete ILO data, with a dark colour indicating non-imputed data for the food items selected by the linear programming. Panel B shows the same information expressed as percentages of the global population covered by those baskets. Direct estimates of poverty lines based on ILO data start only in 1924, covering around 14% of the global population at the time. For the period 1950-2008, the average population coverage is 78%. Using CPI extrapolation, the average population coverage for the period 1884-2018 is also 78%, while for the period before 1884 population coverage drops to 23%.
Figure 9.1. Country and population coverage of the poverty estimates used in this chapter
Note: Panel A shows the number of food baskets calculated in this chapter based on different methods: the original ILO data (in dark blue), the costs of food baskets imputed via CPI (blue), and those based on interpolations and regional extrapolations (in grey). In both panels, the part shown in white corresponds to counties/share of population not covered by the estimates. (See the section on imputations for details.) Panel B shows the share of the global population covered by these estimates. The spikes in population coverage are due to China and India (large spikes) or India (small spikes) in the 19th century, and China in the early 20th century.
Source: Clio-Infra, www.clio-infra.eu.
Consumption and income distributions
To compare with the global poverty estimates published by the World Bank, our calculations rely on the data of the distribution of consumption and income available via World Bank’s PovcalNet (2020[24]). While the combination of consumption and income data obviously reduces cross-country comparability in the PovcalNet dataset, the methodology used here does not try to correct for this in order to preserve comparability between these estimates and those produced by the World Bank. The distributional data in PovcalNet cover the post-1980 period.
Other sources of distributional information need to be used for the years not covered by PovcalNet. These sources typically provide values of the Gini index of the entire distribution, rather than the distribution itself.12 Thus, a method is required to convert this single statistic into an entire distribution, in order to estimate the share of the population with income or consumption below a poverty line. To do this, the log-normality assumption is used to convert a Gini index to a full distribution that follows a log-normal shape (Aitchison and Brown, 1957[25]; Van Zanden et al., 2011[7]).
In summary, for the most recent period, this chapter relies on the distributions provided by PovcalNet. For the period between 1950 and the year when PovcalNet coverage begins for a particular country, the chapter uses a variety of sources13 that, for many countries, rely on household income surveys typically conducted by national authorities.14 Preference is given to distributions of disposable income, followed by those distributions capturing gross (i.e. pre-tax) income, and finally distributions that do not specify the exact type of income they capture; in cases when several estimates of comparable quality are available, their average is used. The chapter also relies on databases providing synthetic Gini estimates15 to provide information on the evolution of the Gini index between observations from the aforementioned sources.16 For the period prior to 1950, inequality data are sourced from van Zanden et al. (2011[7]) and other studies, such as Bolt and Hillbo (2016[26]), Alfani and Tadei (2017[27]), Prados de la Escosura (2008[28]) and Kang (2001[29]).
Estimating unobserved household mean income
After having derived an estimate of the distribution of income or consumption in a country, one needs to “pin down” the dispersion of the distribution around a mean in all cases where this information is not available in the source used. Information about this mean can be drawn from either National Account statistics or from the same household survey that provides information about its distribution. Starting off from PovcalNet provides an advantage in this respect, as the values of the household survey mean are known and can be extrapolated back in time using consumption or GDP data.
Deaton (2001[8]) reports that, in the case of India, the ratio of mean household consumption measured by the System of National Accounts (SNA) and by the main survey on the distribution of household expenditures (the National Sample Survey) fell from almost unity in 1950 to about 50% by the late 1990s. Ferreira et al. (2015[6]) correct for this divergence between mean consumption growth from the surveys and from the National Accounts (by using 87% of the SNA consumption growth rate for all countries, 51% for India and 72% for China). The same approach is used here, while also taking account of the time effect noted by Deaton. To that end, the conversion factors of (Ferreira et al., 2015[6]) for 2000, linearly extrapolated to assume a value of 1 by 1950, are used; this implies that, prior to 1950, mean income from the survey is assumed to grow at the same pace as that from the SNA.
Imputations
To generate estimates of extreme poverty across the globe, imputations are needed for missing countries and years in order to avoid abrupt changes in coverage. In this chapter, imputations are based on the change in the average poverty rate of the countries in that region for which there are available data.17 This approach avoids the downward bias associated with the greater availability of data for rich countries in historical times.18 In addition, when enough data are available for a country at two distant points in time, the poverty rate between those two points is linearly interpolated – instead of regionally imputed. Such an approach is applied mostly to data during the 19th century.19
Data quality
Table 9.1 shows my assessment of the quality of poverty estimates for the eight world regions for a selection of years, in terms of credibility, accuracy and comparability. Among these dimensions, the degree of credibility is relatively high throughout the period, while the degree of accuracy is lower, especially when price data refer to non-market economies (China and the former Soviet Union among others); in these cases, no consumption goods may be available at these administrative prices, hence violating a basic assumption of the cost of basic needs approach.20 A similar problem arises when the average consumer price index is used instead of detailed product prices. This issue, however, is easier to treat, simply with more data collection in the future. With these caveats in mind, my assessment is that the degree of comparability is more or less sufficient, in spite of differences across countries in the underlying income distributions data.
Table 9.1. Quality of data on extreme poverty by world region and year
Year |
Western Europe |
Eastern Europe |
Western Offshoots |
Latin America and Caribbean |
Sub-Saharan Africa |
Middle East and North Africa |
East Asia |
South and Southeast Asia |
---|---|---|---|---|---|---|---|---|
1820 |
4 |
4 |
4 |
4 |
4 |
4 |
4 |
4 |
1870 |
4 |
4 |
4 |
4 |
4 |
4 |
4 |
4 |
1920 |
3 |
4 |
3 |
4 |
4 |
4 |
4 |
4 |
1950 |
2 |
4 |
2 |
2 |
2 |
2 |
4 |
2 |
1980 |
1 |
4 |
1 |
1 |
1 |
1 |
4 |
1 |
1990 |
1 |
4 |
1 |
1 |
1 |
1 |
4 |
1 |
2010 |
1 |
1 |
1 |
1 |
1 |
1 |
1 |
1 |
Note: 1. High quality: the product of an official statistical agency (national or international); 2. Medium quality: the product of economic-historical research using the same sources and methods as applied by official statistical agencies; 3. Moderate quality: economic historical research, but making use of indirect data and estimates; and 4. Low quality: estimates based on a range of proxy information. In case of multiple sources, the lowest quality source is given.
All the basic needs poverty lines estimated are shown in Figure 9.2. These poverty lines are based on linear programming to derive to the CPF line, to which relevant multipliers are applied for obtaining the basic diet poverty line and the non-food component. Poverty lines are estimated based on the ILO data,21 and extrapolated based on the CPI.22
When looking at our poverty lines throughout historical times, the United Kingdom has the highest poverty line in PPP terms at just below USD 5/day during the long 19th century (Figure 9.2). The poverty line for the United States is very close to USD 1.9/day until World War II, increasing thereafter.23 India has a poverty line that is very close to USD 1.9/day, for the entire period. Mexico begins with a poverty line slightly below USD 1.9/day, falling below USD 1/day in the early 1980s, but gradually returning to USD 1.9 by 2018. In the case of Russia, the poverty line shows a huge increase following the dissolution of the Soviet Union in 1991. The poverty line is very volatile in Turkey (starting at USD 2.7/day in 1924 and rising to USD 3.5 by 2018), while the lowest poverty line plotted here is Mozambique’s, at around USD 0.6/day.24
On average, these lines for extreme poverty hover around USD 2.5/day for almost the entire period, with only the years after 2010 showing a clear increase, reaching a maximum of USD 2.8/day in 2018.25 The population-weighted average poverty line has, however, a different trajectory. Starting from a level slightly below USD 1.9/day in the early 19th century, it is broadly constant from 1850 to the early 1940s, increasing thereafter to USD 2.8/day by 2018. It is clear that the values of these poverty lines vary considerably even for the most recent years, ranging in 2018 between USD 1 and USD 7 (Figure 9.1), which is in line with the findings of (Allen, 2017[12]; Hirvonen et al., 2019[30]).
Figure 9.2. Lines for extreme poverty, selected countries and years
Note: Grey reference line provides average of countries’ poverty lines.
Source: Clio-Infra, www.clio-infra.eu.
Main highlights on trends in extreme poverty
The figures in this section show the evolution of extreme poverty rates across the globe based on the cost of basic needs (CBN) method, alongside those based on other methods. Generally, three types of poverty estimates are shown. The main line, shown in dark blue, is the poverty rate based on the CBN methodology used in this chapter. The other lines correspond to the USD 1.9/day line (noted as DAD) and the USD 1.9/day poverty line applied to distribution data centred on GDP per capita as the mean value of the distribution (shown as GDP 1.9 prior to around 1979, i.e. before the PovcalNet data).26 Estimates of extreme poverty at the world level from (Bourguignon and Morrisson, 2002[3]) are also included in Figure 9.3.
Global extreme poverty
Both the CBN and the DAD approaches suggest a bleak picture of extreme poverty in 1820, at 76% and 79% respectively (Figure 9.3). (Bourguignon and Morrisson, 2002[3]), with less available data and using 1985 PPP exchange rates, estimate a higher value at 84%.
Figure 9.3. Extreme poverty around the world based on different estimates
Note: Share of people living in conditions of extreme poverty globally, based on different estimates. CBN stands for the Cost of Basic Needs threshold; DAD is the standard (survey-based) USD 1.9/day poverty line; GDP 1.9 is the USD 1.9/day poverty line adjusted using GDP per capita as the mean value of the distribution. The figure also shows the estimates reported in Bourguignon and Morrisson (2002).
Source: Clio-Infra, www.clio-infra.eu.
According to these measures, in 1820 roughly three-quarters of the world population, about 756 million people, could not afford a tiny space to live, food that would not induce malnutrition, and some minimum heating capacity. This total number of persons living in extreme poverty is almost identical to the estimate for 2018, which stands at 764 million people. Over the entire period between 1820 and 2018, the the number of people living in extreme poverty reached a peak of 2 billion in 1995.
By 2018, global extreme poverty dropped to 10%. Based on our methodology, the global poverty rate fell below 70% in 1873, and below 60% by 1897; after that, it takes much longer to drop below 50% by 1955, then much less time to drop below 40% by 1977. In another 20 years global extreme poverty dropped below 30% in 1997, while the 20% barrier was passed only 8 year later in 2005. The fastest drop in the entire period takes place between 1995 and 2000. During the World War years (I and II), extreme poverty rates show a small increase at the global level.
The global total count and the geographical distribution of those living in conditions of extreme poverty across the globe is shown in Figure 9.4. East Asia accounted for the largest share until 1962 but was overtaken by South and Southeast Asia thereafter. The upward trend in the global count of the population living in extreme poverty was interrupted decisively only in 1995, with a few other noticeable but transitory corrections in 1917, 1947 and 1976. At its peak in 1995, the total number of people living in extreme poverty was 2.7 times that of 1820. Both the overall increase in the total number of poor people by 1995 and the temporary decreases in the number following the two World Wars are clearly visible. The sharp increase in 1950 corresponds to the inclusion of several sub-Saharan African countries only from that year onward.
Figure 9.4. Geographical distribution of people living in extreme poverty across the globe, 1820-2018
Note: The horizontal line corresponds to the total population in extreme poverty in 1820.
Source: Clio-Infra, www.clio-infra.eu.
Table 9.2 provides an overview of extreme poverty rates across world regions in some benchmark years throughout the period. Based on these measures, Eastern Europe and the former Soviet Union achieved the largest reduction in extreme poverty, from 91% in 1820 to 2% in 2018. Sub-Saharan Africa, which started from the same level in 1820, stands at 37% in 2018. Western Europe in 1820 had a higher prevalence of extreme poverty (73%) than South and Southeast Asia (69%).
Table 9.2. Extreme poverty across world regions
Percentages
Year |
Western Europe |
Eastern Europe |
Western Offshoots |
Latin America and Caribbean |
Sub-Saharan Africa |
Middle East and North Africa |
East Asia |
South and Southeast Asia |
---|---|---|---|---|---|---|---|---|
1820 |
73 |
91 |
55 |
85 |
91 |
75 |
78 |
69 |
1850 |
65 |
84 |
28 |
84 |
95 |
71 |
80 |
69 |
1870 |
59 |
68 |
31 |
80 |
93 |
68 |
80 |
74 |
1900 |
41 |
43 |
9 |
74 |
91 |
59 |
76 |
66 |
1920 |
38 |
63 |
3 |
61 |
87 |
55 |
77 |
62 |
1950 |
21 |
19 |
1 |
45 |
70 |
33 |
82 |
67 |
1960 |
11 |
8 |
0 |
45 |
67 |
34 |
66 |
69 |
1970 |
2 |
2 |
0 |
42 |
62 |
26 |
64 |
64 |
1980 |
0 |
3 |
1 |
12 |
63 |
17 |
46 |
50 |
1990 |
0 |
1 |
1 |
17 |
58 |
7 |
37 |
47 |
2000 |
0 |
13 |
1 |
12 |
50 |
3 |
29 |
35 |
2010 |
1 |
3 |
1 |
8 |
41 |
1 |
17 |
23 |
2018 |
1 |
2 |
1 |
7 |
37 |
1 |
5 |
10 |
Table 9.3. Cost of basic needs extreme poverty rates for major countries
Percentages
Year |
GBR |
NLD |
FRA |
DEU |
ITA |
ESP |
SWE |
POL |
RUS |
AUS |
CAN |
USA |
MEX |
BRA |
ARG |
EGY |
TUR |
KEN |
NGA |
ZAF |
CHN |
JPN |
IND |
IDN |
THA |
---|---|---|---|---|---|---|---|---|---|---|---|---|---|---|---|---|---|---|---|---|---|---|---|---|---|
1820 |
80 |
75 |
77 |
66 |
75 |
83 |
86 |
64 |
98 |
87 |
74 |
52 |
86 |
91 |
63 |
60 |
90 |
92 |
92 |
86 |
76 |
95 |
65 |
96 |
82 |
1830 |
78 |
74 |
75 |
64 |
75 |
81 |
83 |
56 |
90 |
69 |
69 |
43 |
87 |
92 |
56 |
56 |
90 |
88 |
88 |
82 |
76 |
96 |
65 |
97 |
82 |
1840 |
78 |
67 |
72 |
63 |
74 |
78 |
82 |
58 |
90 |
45 |
58 |
34 |
87 |
92 |
48 |
52 |
89 |
93 |
93 |
86 |
77 |
97 |
65 |
97 |
82 |
1850 |
69 |
64 |
67 |
59 |
75 |
74 |
78 |
54 |
85 |
28 |
44 |
26 |
87 |
93 |
41 |
48 |
88 |
96 |
96 |
90 |
78 |
98 |
65 |
99 |
81 |
1860 |
68 |
65 |
63 |
55 |
75 |
67 |
72 |
36 |
70 |
15 |
41 |
28 |
89 |
90 |
45 |
44 |
88 |
89 |
89 |
83 |
78 |
97 |
67 |
98 |
81 |
1870 |
55 |
63 |
65 |
53 |
76 |
71 |
71 |
30 |
65 |
17 |
35 |
31 |
84 |
87 |
51 |
40 |
87 |
98 |
98 |
83 |
77 |
97 |
70 |
98 |
81 |
1880 |
48 |
56 |
61 |
45 |
77 |
52 |
68 |
17 |
49 |
7 |
35 |
18 |
77 |
89 |
43 |
36 |
86 |
70 |
70 |
54 |
76 |
96 |
68 |
97 |
75 |
1890 |
35 |
47 |
53 |
31 |
74 |
51 |
66 |
7 |
46 |
3 |
28 |
9 |
70 |
88 |
23 |
32 |
85 |
90 |
90 |
67 |
73 |
97 |
63 |
97 |
69 |
1900 |
32 |
47 |
51 |
25 |
72 |
53 |
55 |
3 |
31 |
5 |
19 |
9 |
66 |
95 |
21 |
27 |
84 |
100 |
100 |
77 |
74 |
92 |
61 |
95 |
68 |
1910 |
35 |
44 |
47 |
24 |
65 |
46 |
46 |
2 |
27 |
4 |
9 |
8 |
60 |
90 |
17 |
23 |
82 |
100 |
100 |
69 |
77 |
90 |
51 |
93 |
67 |
1920 |
33 |
36 |
45 |
32 |
45 |
51 |
34 |
1 |
70 |
4 |
10 |
2 |
56 |
76 |
18 |
21 |
92 |
100 |
100 |
70 |
76 |
83 |
57 |
90 |
69 |
1930 |
20 |
19 |
51 |
22 |
47 |
42 |
19 |
1 |
27 |
1 |
7 |
4 |
56 |
77 |
12 |
18 |
78 |
97 |
97 |
68 |
77 |
80 |
48 |
86 |
71 |
1940 |
6 |
12 |
49 |
14 |
40 |
42 |
19 |
0 |
11 |
0 |
2 |
1 |
50 |
89 |
6 |
16 |
66 |
100 |
100 |
52 |
79 |
64 |
53 |
85 |
68 |
1950 |
2 |
8 |
30 |
21 |
37 |
34 |
4 |
0 |
3 |
0 |
0 |
1 |
37 |
72 |
2 |
14 |
62 |
81 |
99 |
40 |
84 |
73 |
61 |
91 |
67 |
1960 |
4 |
5 |
16 |
5 |
20 |
20 |
2 |
3 |
0 |
0 |
0 |
0 |
30 |
73 |
1 |
14 |
51 |
74 |
94 |
38 |
73 |
23 |
66 |
90 |
60 |
1970 |
0 |
0 |
0 |
2 |
3 |
5 |
1 |
0 |
0 |
0 |
0 |
0 |
24 |
76 |
0 |
12 |
45 |
66 |
94 |
24 |
75 |
2 |
67 |
73 |
40 |
1980 |
0 |
0 |
0 |
0 |
0 |
0 |
0 |
0 |
0 |
0 |
0 |
1 |
6 |
11 |
2 |
1 |
32 |
62 |
95 |
15 |
53 |
0 |
49 |
40 |
32 |
1990 |
0 |
0 |
0 |
0 |
0 |
0 |
0 |
0 |
0 |
1 |
0 |
0 |
3 |
23 |
2 |
0 |
12 |
54 |
72 |
29 |
42 |
0 |
47 |
41 |
18 |
2000 |
0 |
0 |
0 |
0 |
0 |
0 |
0 |
1 |
18 |
1 |
1 |
1 |
5 |
12 |
9 |
0 |
8 |
65 |
71 |
28 |
33 |
0 |
32 |
42 |
10 |
2010 |
1 |
0 |
0 |
0 |
2 |
1 |
1 |
1 |
2 |
1 |
0 |
1 |
3 |
8 |
2 |
0 |
5 |
61 |
64 |
18 |
19 |
1 |
23 |
25 |
3 |
2018 |
1 |
0 |
0 |
0 |
3 |
2 |
1 |
0 |
2 |
2 |
1 |
1 |
2 |
7 |
0 |
0 |
2 |
61 |
62 |
21 |
5 |
1 |
9 |
11 |
1 |
Extreme poverty in world regions
Extreme poverty in Western Europe (Figure 9.5) fell continuously for the entire period until the late 1970s, when it is almost zero. This trend is interrupted twice by both World Wars, with the impact of World War II being much more pronounced. At 41%, the post-WWI peak is roughly 5 percentage points higher than the minimum achieved in 1913. The post-WWII peak, at 35%, is roughly 10 percentage points higher than the minimum in 1939.27 Since 2003, extreme poverty rates in Western Europe have experienced an upward creep, to a level slightly above 1% in 2015. The difference in extreme poverty between the CBN and DAD estimates for Western Europe is substantial, at around 15 percentage points for most of the pre-WWII period, possibly reflecting higher prices for more expensive services such as rents in the CBN estimates.
Figure 9.5. Extreme poverty in Western Europe based on different estimates
Note: CBN stands for the Cost of Basic Needs threshold; DAD is the standard (survey-based) USD 1.9/day poverty-line; GDP 1.9 is the USD 1.9/day poverty line adjusted using GDP per capita as the mean value of the distribution.
Source: Clio-Infra, www.clio-infra.eu.
The largest share of the total count of extreme poverty for the region in 1820 (101 million) comes from France (24 million), followed by the United Kingdom (17), Germany (16.5), Italy (15) and Spain (10). By 1900, Italy accounts for 24 million persons in extreme poverty, followed by France (20), Germany (13.7), the United Kingdom (13) and Spain (10). In 2018, Italy accounts for 1.7 million of the 4.3 million extreme poor in the region, followed by Spain (0.9), the United Kingdom (0.7), Germany (0.2) and Greece (0.2).
Figure 9.6, which describes the evolution of extreme poverty rates for Eastern Europe and the former Soviet Union, highlights three periods of abrupt changes: two after the World Wars and one after the dissolution of the Soviet Union. In 1820, this region had a poverty rate of 91%, practically the same rate as sub-Saharan Africa in the same year. A period of steady reduction starts in 1830, bringing it to 84% by 1850. In 1851 a strong – although not entirely uninterrupted – decline in poverty starts, which is faster than the one realised in Western Europe in this period, bringing the poverty rate to 38% by 1913. A remarkable increase in extreme poverty took place during WWI, which brings poverty to a high of 64% in 1921. A period of exceptional poverty decline starts from 1922, which brings extreme poverty to 1% in 1990, after a strong but temporary increase after World War II.28 Extreme poverty increases again after the dissolution of the Soviet Union, hovering at 10-15% until 2000, and then continuously declining to 2% by 2018.
Figure 9.6. Extreme poverty in Eastern Europe and the former Soviet Union based on different estimates
Note: CBN stands for the Cost of Basic Needs threshold; DAD is the standard (survey-based) USD 1.9/day poverty-line; GDP 1.9 is the USD 1.9/day poverty line adjusted using GDP per capita as the mean value of the distribution.
Source: Clio-Infra, www.clio-infra.eu.
In terms of country level contributions to the world total, Russia was the biggest contributor in 1820, with approximately 31 million, followed by Ukraine (11), Poland (6.7), Romania (6.4) and the Czech Republic (5). By 1990, Ukraine contributes 24 million (almost its entire population), Russia (22), Romania (5.5), the Czech Republic (5.2) and Bulgaria (2.8).29 In 1995, the peak year after the Soviet Union dissolution, Russia contributes 33.5, followed by Ukraine (8), Moldova (2), Armenia (1.2) and Poland (0.7).
Figure 9.7 shows the evolution of extreme poverty in South and Southeast Asia, a region that (population-wise) is dominated by India. Prior to the 1890s, data are very scarce and only available for a few countries in a few benchmark years; the linear interpolations for the best part of the 19th century are evident in the figure. In any case, the available data show a poverty rate of 69% in 1820, a level more or less unchanged until the 1890s. A volatile period between 1884 and the late 1920s brings extreme poverty to a minimum of 54%. The upward trend that follows takes the poverty rate back up to 71% in 1953, a higher level than the one recorded in the 1820s. Despite a temporary surge in 1998 to 46%, extreme poverty declined to a low of 10% in 2018.
In terms of absolute contributions, India accounts for 136.6 million people in global poverty counts in 1820, Indonesia contributes 17.3 million, followed by Thailand (3.8) and Nepal (3.2). By 1950, India contributes 217.9 million people to the global poverty counts, followed by Indonesia (75.1), Bangladesh (30.1), Pakistan (28.3) and Myanmar (17.4). In 2018, India contributes 126.9 million people, followed by Bangladesh (55.2), Indonesia (29), Pakistan (11) and the Philippines (6.4).
Figure 9.7. Extreme poverty in South and Southeast Asia based on different estimates
Note: CBN stands for the Cost of Basic Needs threshold; DAD is the standard (survey-based) USD 1.9/day poverty-line; GDP 1.9 is the USD 1.9/day poverty line adjusted using GDP per capita as the mean value of the distribution.
Source: Clio-Infra, www.clio-infra.eu.
Figure 9.8 shows the evolution of extreme poverty in East Asia.30 East Asia is the only region where extreme poverty reached its peak in the 20th century, at 83% in 1949. The poverty reduction during the post-WWII period stalled in 1958, with a local maximum of 69% in 1961, and then resumed its fall after 1969, to 37% in 1990. Since then, extreme poverty increased to a new peak of 59% in 1995, only marginally lower than the estimate for sub-Saharan Africa in the same year, before falling to an overall low of 5% in 2018.
Figure 9.8. Extreme poverty in East Asia based on different estimates
Note: CBN stands for the Cost of Basic Needs threshold; DAD is the standard (survey-based) USD 1.9/day poverty-line; GDP 1.9 is the USD 1.9/day poverty line adjusted using GDP per capita as the mean value of the distribution.
Source: Clio-Infra, www.clio-infra.eu.
As expected, China is by far the largest contributor in absolute terms throughout the period. In 1820, China contributes 288 million people to the global extreme poverty count, followed by Japan (29.4), Korea (9.2) and Mongolia (0.6). At the local high of poverty in 1995, China contributes 815.8 million people in global poverty counts, followed by Mongolia (1.4).31 In 2018, China contributes 77.1 million people, followed by Japan (1.4), Mongolia (0.5) and Korea (0.3).
Figure 9.9 shows the development of extreme poverty throughout the Middle East and North Africa region. For the best part of the period until 1970, both the DAD and CBN series are relatively close, and at times very close. Extreme poverty fell in the 19th century, from 75% in 1820 to 59% by 1900. The pace of reduction is slightly faster in the first half of the 20th century, to 18% by 1974. A sharp decrease follows in the 1980s, bringing extreme poverty to 7% in 1990, and to 1% in 2018.32
In terms of the countries’ contributions to the global count of extreme poverty, Turkey is the largest contributor in 1820 with approximately 9.1 million, followed by Sudan (5.2), Iran (4.5) and Algeria (1.2). By 1974, Turkey contributes 10.2 million, Sudan (10), Morocco (5.4) and Algeria (1.6). In 2018, Turkey is again the largest contributor, but at considerably lower levels, with 1.7 million people, followed by Yemen (0.8),33 Sudan (0.3) and Algeria (0.1).
Figure 9.9. Extreme poverty in the Middle East and North Africa based on different estimates
Note: CBN stands for the Cost of Basic Needs threshold; DAD is the standard (survey-based) USD 1.9/day poverty-line; GDP 1.9 is the USD 1.9/day poverty line adjusted using GDP per capita as the mean value of the distribution.
Source: Clio-Infra, www.clio-infra.eu.
Figure 9.10 shows the evolution of extreme poverty in the Western Offshoots region. This region has the lowest poverty rates throughout the period. Starting from a level of 55% in 1820, the decline in poverty rate comes to a halt in 1853, and resumes after the US Civil War until 1918, when it stands at 2%. Following the 1929 financial crisis, extreme poverty reaches a peak of 8% in 1933, more than double its level in 1924. During WWII, the Western Offshoots shows no sign of a poverty increase, and its rate in 1944 (at 1%) is the world’s lowest. In the years that follow, poverty rates are less than 0.5% until 1979. The period ends with a rise in extreme poverty to a rate above 1% after 2000. In terms of absolute contributions to global poverty, the United States contributes 5.2 million in 1820, followed by Canada (0.6) and Australia (0.3). By 2018, and despite the large reduction in poverty rates, the contributions are almost identical to those of 1820, with the United States contributing 4.9 million, followed by Canada (0.5) and Australia (0.4).
Figure 9.10. Extreme poverty in the Western Offshoots based on different estimates
Note: CBN stands for the Cost of Basic Needs threshold; DAD is the standard (survey-based) USD 1.9/day poverty-line; GDP 1.9 is the USD 1.9/day poverty line adjusted using GDP per capita as the mean value of the distribution.
Source: Clio-Infra, www.clio-infra.eu.
Figure 9.11 shows the evolution of extreme poverty for sub-Saharan Africa. This region featured the highest poverty rates throughout the entire period, with very few exceptions. In 1820, the regional poverty rate is 91%, which is still lower than the period’s highest rate of 98% in 1847. Substantial poverty reduction starts only after 1917 and brings extreme poverty to a local minimum of 64% by 1949.34 A slow improvement lasts until 1970, followed by slow increases until 1982. In the late 1980s, poverty rates fluctuate around 60% up until 1995, when a strong reduction is observed, bringing the rates to around 40%. By 2018 the poverty rate, at 37%, is close to its minimum reached in 2014.35
Figure 9.11. Extreme poverty in Sub-Saharan Africa based on different estimates
Note: CBN stands for the Cost of Basic Needs threshold; DAD is the standard (survey-based) USD 1.9/day poverty-line; GDP 1.9 is the USD 1.9/day poverty line adjusted using GDP per capita as the mean value of the distribution.
Source: Clio-Infra, www.clio-infra.eu.
In terms of countries’ contributions to the total count of extreme poverty, Ethiopia is the biggest contributor in 1820 with approximately 2.9 million, followed by Mozambique (1.9) and Madagascar (1.6).36 By 1950, when data for many more countries are available, Nigeria contributes 57 million, followed by Ethiopia (22.3), Kenya (9.2) and South Africa (7.7). In 2018, Nigeria is again the largest contributor, with 121.3 million people in extreme poverty, followed by Ethiopia (40.9), Kenya (31.2), Madagascar (14.1) and South Africa (12.3).
Figure 9.12 shows the development of extreme poverty for Latin America and the Caribbean. Starting from a level of 85% in 1820, the poverty rate fell to 45% in 1950, and to 7% by 2018. A strong decline from 42% in 1970 to 12% in 1980 was partially reversed in the following year, with the poverty rate doubling to 24% by 1984. Since then, a slow reduction brought extreme poverty to a minimum by 2018. In terms of countries’ contributions to the total population in extreme poverty, Mexico is the biggest contributor in 1820, with 5.7 million, followed by Brazil (4.1), Peru (1.2) and Colombia (1.1). By 1950, Brazil contributes 38.4 million, followed by Mexico (10.7), Colombia (4.8) and Peru (4.2). In 2018, Brazil is again the largest contributor with 15.6 million people in extreme poverty, followed by Haiti (7.9), Venezuela (6.7), Peru (3.4), Colombia (2.4) and Mexico (2).
Figure 9.12. Extreme poverty in Latin America and the Caribbean based on different estimates
Note: CBN stands for the Cost of Basic Needs threshold; DAD is the standard (survey-based) USD 1.9/day poverty-line; GDP 1.9 is the USD 1.9/day poverty line adjusted using GDP per capita as the mean value of the distribution.
Source: Clio-Infra, www.clio-infra.eu.
China
China requires special attention for two reasons: it was for a long period a non-market economy, and (because of its size) it has a disproportionate impact on global poverty counts. These two factors imply that uncertainty in Chinese estimates has large implications on a global scale. Figure 9.13 illustrates the magnitude of these concerns. It shows the trajectory of poverty rates based on the estimates discussed above, which result from averaging estimates based on two approaches: (a) taking price data at face value for the period 1990-95; and (b) attributing all CPI change to non-food items (explanation follows). Scenario (a) is the same approach used for all other estimates presented in this chapter, i.e. using nominal prices to estimate the CPF food poverty line, and then applying to them multipliers to obtain the Basic Diet and the non-Food poverty lines. The only difference is that the nominal prices used here are not those from the ILO data but from the Chinese Statistical Yearbooks.37 This estimate (the lower dashed blue line at the bottom of Figure 9.13) shows an almost zero extreme poverty rate in 1990, which is unrealistic. The alternative approach is based on the idea that, since food prices have a much higher volatility in this period than the CPI, all CPI changes should reflect changes in the food component of the index, and that prices for the non-food items of the poverty basket over the period 1990-94 are at the level attained in 1995. This alternative estimate (shown by the dashed blue line at the top of Figure 9.13) is close to 100% in 1990. Taking the average of two opposing scenarios that both seem unrealistic is far from resolving the problem, and the reader should consider the wide range of probable uncertainty as indicated in Figure 9.13, both for the 1990s and in the long run (implying possible values in the range of 60 to 95%).
Figure 9.13. Extreme poverty in China based on different estimates
Note: CBN stands for the Cost of Basic Needs threshold; DAD is the standard (survey-based) USD 1.9/day poverty-line; GDP 1.9 is the USD 1.9/day poverty line adjusted using GDP per capita as the mean value of the distribution. Low CBN estimates based on nominal prices from Chinese Statistical Yearbooks; the high CBN estimates line relies on food prices and keeps non-food prices fixed at 1995 levels.
Source: Clio-Infra, www.clio-infra.eu.
Correlation with GDP per capita
Figure 9.14 shows the correlation in each year between the CBN-based poverty rates and the estimates of GDP per capita shown in Chapter 2 of this book. Across the full period, the correlation is -0.59, implying that countries with higher GDP per capita also feature lower rates of extreme poverty, with the largest value of about -0.77 achieved in 1919, and the smallest one in 1831 at -0.3. In the post-World War II period, the largest correlation of about -0.63 is achieved in 1978, and the smallest at -0.48 in 2014. The decline in this correlation may be explained by the increase of the within-country income inequality observed in the period between those years, albeit at different rates between countries. At the same time, the drop in global poverty after 1995 is the largest observed, despite the low correlation with GDP per capita. This implies a “lost opportunity”, for even faster poverty reduction could have been achieved if measures had been taken to contain increasing within-country income inequality. It should be noted though that the confidence intervals around these correlation coefficients are very high, implying that other factors beyond GDP per capita played an important role. The confidence interval around the correlation coefficient stretches into positive terrain in the early 19th century, implying that in the early 19th century some countries with high extreme poverty featured high GDP per capita, and vice versa.
Figure 9.14. Correlation between rates of extreme poverty and GDP per capita
Note: Thick and thin lines depict correlation coefficient and 95 % confidence intervals respectively.
Source: Clio-Infra, www.clio-infra.eu.
Priorities for future research
This first attempt to estimate global extreme poverty using a cost of basic needs approach over the long run highlights a number of novel empirical patterns but also the many areas where more historical research is needed. In particular, the global character of our exercise, and the still sparse availability of relevant data, has required taking shortcuts on a number of empirical issues. Most importantly, to account for a richer diet and for other non-food costs, our estimates rely on Allen’s “multipliers” for 2011, instead of a direct calculation. Moving beyond this assumption will require the assembly of a broader global dataset on prices of all products and services relevant to people living in poverty. Such a dataset could then be used to provide direct estimates of poverty lines covering housing, education, health care and heating. Such a broader set of data on the prices of different consumption items would allow overcoming another limitation of the estimates presented in this chapter, i.e. the extensive use of CPI information.
An additional important consideration is the fixity across time of the welfare standard used here. The poverty line favoured by (Allen, 2017[12]) and used in this chapter is based on a requirement of 2 100 kcal of energy per person per day. Conversely, (FAO, 2001[31]; FAO, 2008[32]) provide measures of the so-called minimum dietary energy requirement as a function of the population’s age and gender. Applying the approach used in this chapter to these minimal dietary energy requirements would allow providing estimates of extreme poverty that take into account changes in population structure over long periods of time. The same approach could be applied to take into account the Physical Activity Level of those living in poverty (i.e. the physical intensity of work, home, leisure and life conditions), which plays an important role in shaping the nutrient targets to be considered.
At the same time, secondary indicators are needed to address the problem of price unrepresentativeness in non-market economies, in particular for China and the former Soviet Union. Finally, a priority for future historical research should be to produce metrics of the sensitivity of the poverty rate with respect to the poverty line.
References
[25] Aitchison, J. and J. Brown (1957), The Lognormal Distribution, The syndics of the Cambridge University Press, Cambridge.
[27] Alfani, G. and F. Tadei (2017), “Income inequality in colonial Africa: Building social tables for pre-Independence Central African Republic, Ivory Coast and Senegal”, African Economic History Working Paper Series, No. 33, https://www.aehnetwork.org/wp-con.
[12] Allen, R. (2017), “Absolute poverty: When necessity displaces desire”, American Economic Review, Vol. 107/12, pp. 3690-3721, https://doi.org/10.1257/aer.20161080.
[19] Allen, R. (2013), “Poverty lines in history, theory and current international practice”, Economics Series Working Papers, No. 685, Department of Economics, University of Oxford, https://ora.ox.ac.uk/objects/uuid:3c9df348-d545-43dc-87f2-ae53c840f1d2.
[11] Atkinson, A. (2016), Monitoring Global Poverty: Report of the Commission on Global Poverty, The World Bank, Washington, DC., https://openknowledge.worldbank.org/bitstream/handle/10986/25141/9781464809613.pdf.
[34] Atkinson, A. et al. (2017), The Chartbook of Economic Inequality, http://www.chartbookofeconomicinequality.com.
[41] Bank of Greece, Bulgarian National Bank, National Bank of Romania, Oesterreichische Nationalbank (2014), South-Eastern European Monetary and Economic Statistics from the Nineteenth Century to World, Athens, Sofia, Bucharest, Vienna, http://citeseerx.ist.psu.edu/viewdoc/download;jsessionid=4E26F2E53629D5ACC44E93115BE70B7A?doi=10.1.1.457.3832%26rep=rep1%26type=pdf.
[2] Bhalla, S. (2002), Imagine There’s No Country: Poverty, Inequality and Growth in the Era of Globalization, Peterson Institute for International Economics.
[26] Bolt, J. and E. Hillbo (2016), “Long-term trends in economic inequality: Lessons from colonial Botswana, 1921-74”, The Economic History Review, Vol. 69/4, pp. 1255-84, https://doi.org/10.1111/ehr.12326.
[21] Bolt, J. and J. van Zanden (2014), “The Maddison Project: Collaborative research on historical national accounts”, The Economic History Review, Vol. 67/3, pp. 627-51, https://doi.org/10.1111/1468-0289.12032.
[3] Bourguignon, F. and C. Morrisson (2002), “Inequality Among World Citizens: 1820-1992”, The American Economic Review, Vol. 92/4, pp. 727-44, https://www.jstor.org/stable/3083279.
[5] Chen, S. and M. Ravallion (2010), “The developing World is poorer than we thought, but no less successful in the fight against poverty”, The Quarterly Journal of Economics, Vol. 125/4, pp. 1577-1625, http://qje.oxfordjournals.org.proxy.library.uu.nl/content.
[40] Clio Infra (2015), Clio Infra Consumer Price Index dataset, https://clio-infra.eu/Indicators/Inflation.html (accessed on 12 August 2015).
[23] de Zwart, P., B. van Leeuwen and J. van Leeuwen-Li (2014), “Real wages since 1820”, in How Was Life?: Global Well-being since 1820, OECD Publishing, Paris, https://dx.doi.org/10.1787/9789264214262-8-en.
[8] Deaton, A. (2001), “Counting the World’s poor: Problems and possible solutions”, The World Bank Research Observer, Vol. 16/2, pp. 125-47, https://doi.org/10.1093/wbro/16.2.125.
[51] Eurostat (n.d.), Eurostat Statistical Dashboard, https://ec.europa.eu/eurostat/web/main/data/database.
[32] FAO (2008), Updating the Minimum Dietary Energy Requirements, Food and Agriculture Organization of the United Nations, Rome.
[31] FAO (2001), Human Energy Requirements, Food and Agriculture Organization of the United Nations, Rome.
[6] Ferreira, F. et al. (2015), “A global count of the extreme poor in 2012: Data issues, methodology and initial results”, Policy Research Working Paper, No. 7432, World Bank, Washington, http://hdl.handle.net/10986/22854.
[46] GINI (n.d.), Growing Inequalities’ Impacts (GINI), http://www.gini-research.org/articles/home.
[30] Hirvonen, K. et al. (2019), “Affordability of the EAT – Lancet reference diet: A global analysis”, The Lancet Global Health, Vol. 8/1, pp. 1-8, https://doi.org/10.1016/S2214-109X(19)30447-4.
[45] Institute for Fiscal Studies (n.d.), Living standards, poverty and inequality in the UK, https://www.ifs.org.uk/tools_and_resources/incomes_in_uk.
[33] Jordà, Ò., M. Schularick and A. Taylor (2016), Macrofinancial History and the New Business Cycle Facts, University of Chicago Press.
[29] Kang, S. (2001), Globalization and Income Inequality in Korea: An Overview, OECD Technical Meeting.
[44] LIS (n.d.), The Luxembourg Income Study Database (LIS), https://www.lisdatacenter.org/our-data/lis-database/.
[15] Moatsos, M. (2018), “The unbearable errorlessness of global poverty estimates”, The Economists’ Voice, Vol. 15/1, https://doi.org/10.1515/ev-2018-0004.
[17] Moatsos, M. (2017), “Global absolute poverty: Behind the veil of dollars”, The Journal of Globalization and Development, Vol. 7/2, https://doi.org/10.1515/jgd-2016-0033.
[35] Moatsos, M. (2017), Tracing global poverty paths, 1925-2010, Conference Proceedings of the 2017 Economic History Society Annual Conference.
[36] Moradi, A. and J. Baten (2005), “Inequality in Sub-Saharan Africa 1950-80: New estimates and new results”, World Development, Vol. 33/8, pp. 1233-65, http://www.eco.rug.nl/~jacobs/jjdownload/Workshop14May-Moradi%20Baten.pdf.
[42] OECD (n.d.), OECD Income Distribution Database (IDD): Gini, poverty, income, Methods and Concepts, https://www.oecd.org/social/income-distribution-database.htm (accessed on 11 February 2019).
[28] Prados de la Escosura, L. (2008), “Inequality, poverty and the Kuznets curve in Spain, 1850-2000”, The European Review of Economic History, Vol. 12/3, pp. 287-324, https://doi.org/10.1017/S1361491608002311.
[37] R Core Team (2018), R: A Language and Environment for Statistical Computing, R Foundation for Statistical Computing, Vienna, Austria, https://www.r-project.org/.
[18] Ravallion, M. (2010), “The debate on globalization, poverty, and inequality: Why measurement matters”, in Debates on the Measurement of Global Poverty, Oxford Scholarship Online, https://doi.org/10.1093/acprof.
[13] Ravallion, M. et al. (1991), Quantifying the Magnitude and a of Absolute Poverty in the Developing World in the Mid-1980s, Policy, Research, and External Affairs, The World Bank, Washington.
[10] Reddy, S. and T. Pogge (2010), “How not to count the poor”, Oxford University Press.
[4] Sala-i-Martin, X. (2006), “The World distribution of income: Falling poverty and... convergence, period”, The Quarterly Journal of Economics, Vol. 121/2, pp. 351-97, https://www.jstor.org/stable/25098796.
[38] Sandel, M. (2012), What Money Can’t Buy: The Moral Limits of Markets, Allen Lane, London.
[50] SEDLAC (n.d.), Socio-Economic Database for Latin America and the Caribbean, https://www.cedlas.econo.unlp.edu.ar/wp/en/estadisticas/sedlac/.
[39] Solt, F. (2016), “The Standardized World Income Inequality Database”, Social Science Quarterly, Vol. 97/5, pp. 1267-81.
[9] Srinivasan, T. (2009), “Irrelevance of the $1-a-day poverty line”, Initiative for Policy Dialogue Working Paper Series, http://policydialogue.org/files/publications/Ch5_Srinivasan.pdf.
[49] Statistics Canada (n.d.), Statistics Canada Data, https://www150.statcan.gc.ca/n1/en/type/data?MM=1.
[43] Stone Center on Socio-Economic Inequality (2019), All the Ginis (ALG) Dataset, https://stonecenter.gc.cuny.edu/research/all-the-ginis-alg-dataset-version-february-2019/.
[16] Subramanian, S. (2015), “Once more unto the breach”, Economic & Political Weekly, Vol. 45, pp. 35-40, https://www.epw.in/journal/2015/45/insight/once-more-unto-breach.html.
[14] Summers, R. and A. Heston (1988), “A new set of international comparisons of real product and price levels estimates for 130 countries, 1950-1985”, The Review of Income and Wealth, Vol. 34/1, pp. 1-25, https://doi.org/10.1111/j.1475-4991.1988.tb00558.x.
[24] The World Bank (2020), PovcalNet: An online analysis tool for global poverty monitoring, http://iresearch.worldbank.org/PovcalNet/home.aspx (accessed on 19 January 2020).
[22] United Nations (2019), World Population Prospects 2019, UN Department of Economic and Social Affairs, https://population.un.org/wpp/ (accessed on 12 September 2019).
[47] UNU-WIDER (2018), World Income Inequality Database - WIID, United Nations University, https://www.wider.unu.edu/database/wiid.
[48] US CENSUS Bureau (n.d.), US Census Data, https://data.census.gov/cedsci/.
[20] USDA (2019), FoodData Central, US Department of Agriculture, https://fdc.nal.usda.gov/ (accessed on 19 September 2019).
[7] Van Zanden, J. et al. (2011), “The changing shape of global inequality 1820-2000: Exploring a new dataset”, Center for Global Economic History (CGEH) Working Paper Series, No. 1, https://doi.org/10.1111/roiw.12014.
[1] van Zanden, J. et al. (eds.) (2014), How Was Life?: Global Well-being since 1820, OECD Publishing, Paris, https://dx.doi.org/10.1787/9789264214262-en.
Notes
← 1. Author’s affiliation: Department of History and Art History, Economic and Social History, Utrecht University. I wish to thank Marco Mira d’Ercole, Bram van Besouw, Tim van der Valk, Gareth Austin, Alex Kolev, Wouter Ryckbosch, Mark Sanders, Jutta Bolt, Aditi Dixit, Auke Rijpma and Jan Luiten van Zanden for their suggestions, comments and remarks. I particularly thank Bas van Leeuwen for providing price data on China, as well as for sharing digitisalised ILO price data (together with Pim de Zwart); Robert Allen for discussing his method and results; Leandro Prados de la Escosura for all his valuable comments and for sharing his newly updated long-run inequality estimates for Spain along with his invaluable remarks and comments; Guido Alfani for the inequality data on sub-Saharan Africa; Christoph Lakner and Prem Sangraula from the World Bank for sharing the CPI dataset used by the Bank for global poverty measurement; and Guus Wieman for his excellent research assistantship. I want to extend my gratitude to the participants at the 2017 Posthumus Conference, the 2017 Economic History Society Annual Conference, and the workshops organised by the editors of this volume in Utrecht and at the OECD in Paris. All analysis has been conducted with R open source statistical computing software (R Core Team, 2018[37]). All remaining errors are my own.
← 2. Throughout the chapter, poverty is qualified as “extreme” to distinguish it from the concept of “relative” income poverty used in most OECD publications. Relative income poverty relies on a threshold (based on a measure of household disposable income adjusted for household size) set at half of the median income of each country in each year.
← 3. For details on the treatment of pre-1995 China, see the discussion later in this chapter, where two scenarios are considered on how to treat the problem posed by non-market prices. All estimates for pre-1995 China presented throughout this chapter are based on the average of the two scenarios.
← 4. According to Allen’s calculations, the CPF poverty line for developing countries is USD 1.84/day, on average, which is very close to the 1.9 dollar-a-day poverty line used by the World Bank and United Nations to monitor global extreme poverty. Allen comments that this line is too low, as “people eating a CPF diet suffer many nutritional deficiencies” (Allen, 2017[12]).
← 5. Allen’s estimates are only for 2011, and he uses the 2011 ICP price data.
← 6. The data on prices from the October Inquiry of the ILO have been retrived from several ILO publications depending on the year: International Labour Review (1924-1935), Yearbook of Labour Statistics (1935-1964), Bulletin of Labour Statistics (1965-1991) and Statistics on Occupational Wages and Hours of Work and on Food Prices (1992-2009). All these publications are available at https://www.ilo.org/public/libdoc/ilo/P/.
← 7. Using Table 11 from Allen (2017[12]). This is done in two steps: 1) one multiplier is used to bring the cost of the food component of the CPF poverty line to the level of the basic diet; and 2) another multiplier is used to account for non-food items, based on the share of the food component in the total consumption basket of the basic diet, see Table 12 in Allen (2017[12]). In our approach, both multipliers are a function of GDP per capita (based on the estimates by Bolt and van Zanden (2014[21]). In the calculations of those multipliers, France is excluded, as the price for wheat flour used by Allen (at less than 0.5 euro per kg in 2011) appears very low relative to the ILO price data used here (around 1.5 euro for 2011). See the online Appendix for additional information on these multipliers. This approach is not sensitive to the between-country differences in heating requirements. For a discussion of this issue see Moatsos (2017[17]), Moatsos (2017[35]) and Allen (2017[12]).
← 8. The post-1984 price data were downloaded from the ILO’s website (https://www.ilo.org/public/libdoc/ilo/P/), while the 1924-1984 data were scanned from various publications at the ILO document repository. The new version of ILOSTAT does not contain the price data any more, while the document repository with all available price data in pdf format can be found mainly at https://www.ilo.org/public/libdoc/ilo/P/09615/.
← 9. Since price information in the ILO data are expressed using the currency denomination prevailing at the time (nominal prices), information on changes in currency denomination is required to denominate all prices in the currency used today. This was done using the dataset on the history of currencies provided by Global Financial Data, Global History of Currencies dataset; additional information on currency redenomination was used for the more recent period based on information available at the websites of national central banks, and collected on Wikipedia pages for local currencies.
← 10. For more details, see the Appendix available at https://doi.org/10.33540/129.
← 11. A variety of CPI sources were used, giving priority to the PovcalNet CPI data (http://iresearch.worldbank.org/PovcalNet/data.aspx), followed by other sources in order of preference: World Bank WDI data (https://datatopics.worldbank.org/world-development-indicators/), IMF CPI data (https://data.imf.org/?sk=4FFB52B2-3653-409A-B471-D47B46D904B5), Jordà, Schularick and Taylor (2016[33]), Sandel (2012[38]), Clio Infra Consumer Price Index dataset from the Clio Infra project website (Clio Infra, 2015[40]), Balkans CPI (historical CPI series from Balkan countries (Bank of Greece, Bulgarian National Bank, National Bank of Romania, Oesterreichische Nationalbank, 2014[41])) and the ILO general and food CPI index.
← 12. The Gini index is a widely used measure of inequality with 0 representing total equality – everyone having the same income – and 1 representing total inequality – one person having all the income while everyone else has zero.
← 13. Those sources are: (SEDLAC, n.d.[50]), STATCAN (Statistics Canada, n.d.[49]), US CENSUS data (US CENSUS Bureau, n.d.[48]), UNU-WIDER WIID (version 19 December 2018) (UNU-WIDER, 2018[47]), OECD Income Inequality Database (OECD, n.d.[42]), All The Ginis Dataset (Stone Center on Socio-Economic Inequality, 2019[43]), EUROSTAT (last update 31 January 2019) (Eurostat, n.d.[51]), GiniProject (version September 2013) (GINI, n.d.[46]), the Luxemburg Income Studies Database (LIS, n.d.[44]), UK Institute for Fiscal Studies (Living Standards, Inequality and Poverty Spreadsheet) (Institute for Fiscal Studies, n.d.[45]), and Chartbook of Economic Inequality in the May 2017 edition (Atkinson et al., 2017[34]).
← 14. As opposed to indirect estimates based on social tables or other methods (e.g. based on the relation between the coefficient of variation of height and income inequality (Moradi and Baten, 2005[36]; Van Zanden et al., 2011[7])). In addition to the scarce availability of data per se, the underlying methodologies and concepts used for carrying out a survey vary between countries and periods. Moreover, changes in the concepts and methodologies introduce structural breaks in the series for a given country.
← 15. Solt (2016[39]) and the Estimated Household Income Inequality Data Set (EHII) V2017 v.1 from the University of Texas.
← 16. The growth pattern implied by the evolution of the synthetic index is used to impute the Gini values between actual observations from other sources, with values that follow the same growth pattern as the synthetic index. When this is not possible, poverty rates are calculated using the available Gini indices at both ends; their weighted average is taken, with the estimates based on the closer Gini data point being linearly assigned a higher weight.
← 17. In this calculation, I used poverty estimates only from countries that are available in both years, and that are not a result of regional imputation or interpolation.
← 18. The World Bank takes a different route, assuming (regardless of the set of countries for which data are available) that countries without data have the same poverty rate as the rest of the region. This is a strong assumption that I relax here. The countries used for regional extrapolation in the 19th century going back to 1820 are: Japan for East Asia; South Africa (and Ghana up to 1870) for sub-Saharan Africa; Jordan, Lebanon, Egypt, Iran, Morocco, Tunisia, Syria and Turkey for the Middle East and North Africa; Denmark, Norway, Austria, Ireland, Italy, the Netherlands, France, the United Kingdom, Finland, Sweden and Portugal for Western Europe; the United States, Canada and Australia for the Western Offshoots; Argentina, Brazil, Chile, Colombia, Jamaica, Mexico, Peru, Uruguay and Venezuela for Latin America and the Caribbean; Indonesia, Sri Lanka, Myanmar, Malaysia, Nepal, the Philippines, Thailand and India (up to 1821) for South and Southeast Asia; and Poland for Eastern Europe and the former Soviet Union. More countries are used for imputations later in the 19th century and in the 20th and 21st centuries.
← 19. These linearly interpolated estimates are not used for the regional imputation described previously. Moreover, going back in time and when no other information on inequality is available, the last and closest available Gini index is used to pin down an estimation for the country-level poverty rate.
← 20. Once a cheap product is in shortage, a more expensive one should be used by the linear programming. Such information is lacking at the moment. In the case when the low-price products are indeed available and are purchased by those living in extreme poverty, those prices are used for calculating the cost of the basic needs poverty line.
← 21. Thus, they include interpolations and extrapolations of prices using CPI or the evolution of prices of similar products within the ILO dataset.
← 22. Both as a – generally increasing – function of real GDP per capita, see for more details the Appendix available at https://doi.org/10.33540/129.
← 23. During the last few years in the period, both the Swiss and the US poverty lines flatten out at a constant PPP level. This is because the CPF cost is fixed in PPP terms, and the applied multipliers are and remain at their maximum.
← 24. For these countries, the implications of using multipliers to estimate the non-food component and the richer basic diet (instead of using original data) become somehow evident. If the food component is very low, then the multiplier may underestimate the additional costs. Likewise, when the initial CPF food component is relatively high, then the multipliers most likely overestimate the additional costs.
← 25. A low value of USD 2.16/day is observed in 1820, due generally to different coverage than most of the other years in the 19th century. A similar reduction is observed in 1870 for the same reason.
← 26. In other words, the difference between GDP 1.9 and DAD is fully attributable to the effect of substituting GDP per capita with the estimated household mean income, and the difference between DAD and CBN lines is fully attributable to the effect of different poverty lines. More detailed estimates on a per country basis, and an exposition of some methodological details left out from the main text, can be found in the Appendix available at https://doi.org/10.33540/129.
← 27. In both World Wars, a methodological problem presents itself: if and how should the death toll of the wars be counted in the poverty statistics, especially due to the acceleration in poverty reduction in the period after the peak. The observation of a positive long-run impact of war on extreme poverty may well reflect a misuse of statistical information or a demonstration of wrong accounting.
← 28. As noted above, however, this period is particularly problematic due to the non-market nature of product prices; therefore caution is advised for the interpretation of these estimates.
← 29. By 1900, Poland has relatively low levels of extreme poverty at around 3%. This low value is partly attributable to the very low poverty line of just above USD 1/day in that period, and partly to its GDP level and GDP/HHS mean ratio.
← 30. Given the special subsection on China below, the focus of this subsection is mostly on other countries.
← 31. Japan contributes only a few thousand this year, and Korea none.
← 32. However, some relatively large countries are missing from this area: Libya, Iraq and Saudi Arabia. For the first two one would expect very high poverty rates.
← 33. This estimate is of particular concern, as the data seem not to be representative of the dire situation for the Yemen population due to the ongoing war in its territory.
← 34. Not many countries from this region have population data prior to 1950 in the sources used here (available pre-1950 population data cover: Angola, Ethiopia, Ghana, Madagascar, Mozambique, Mauritius, Somalia, Sudan and South Africa). Information from other sources could be added in later implementations of the approach used in this chapter to improve country coverage.
← 35. For the sub-Saharan region in particular there is one additional methodological consideration in terms of comparability with most other regions. The PovcalNet distributions do not use equivalence scales when converting the “per household” income/consumption to a “per individual” basis. With this approach, larger families, who are disproportionately present in the region, are relatively penalised, with a much lower income per capita than when equivalence scales are used (as done by the OECD for example).
← 36. Several countries in the region, including Nigeria, are not covered in this year by the population data.
← 37. This is done as a robustness check, but the same results obtain with ILO data as well. The advantage in keeping the Yearbook statistics is that they reduce the reliance on CPI conversions, as they offer complete coverage of basic items, and cover up to, and including, 2014.