This chapter offers an overview of changes in human skills and computer capabilities in the domains of literacy and numeracy over time. It first analyses changes in the skill levels of adults aged 16 to 65, working adults and students aged 15 using data from the Programme for International Student Assessment (PISA), the Survey of Adult Skills (PIAAC), the International Adult Literacy Survey (IALS) and the Adult Literacy and Life Skills Survey (ALL). The chapter then describes recent trends in the fields of natural language processing and mathematical reasoning of artificial intelligence (AI). These technological developments are relevant for the potential performance of AI on the PIAAC test. By showing that technological progress develops much faster than human skills in key skill domains, the chapter highlights the need for periodically and systematically monitoring the evolution of AI capabilities and comparing them to human skills.
Is Education Losing the Race with Technology?
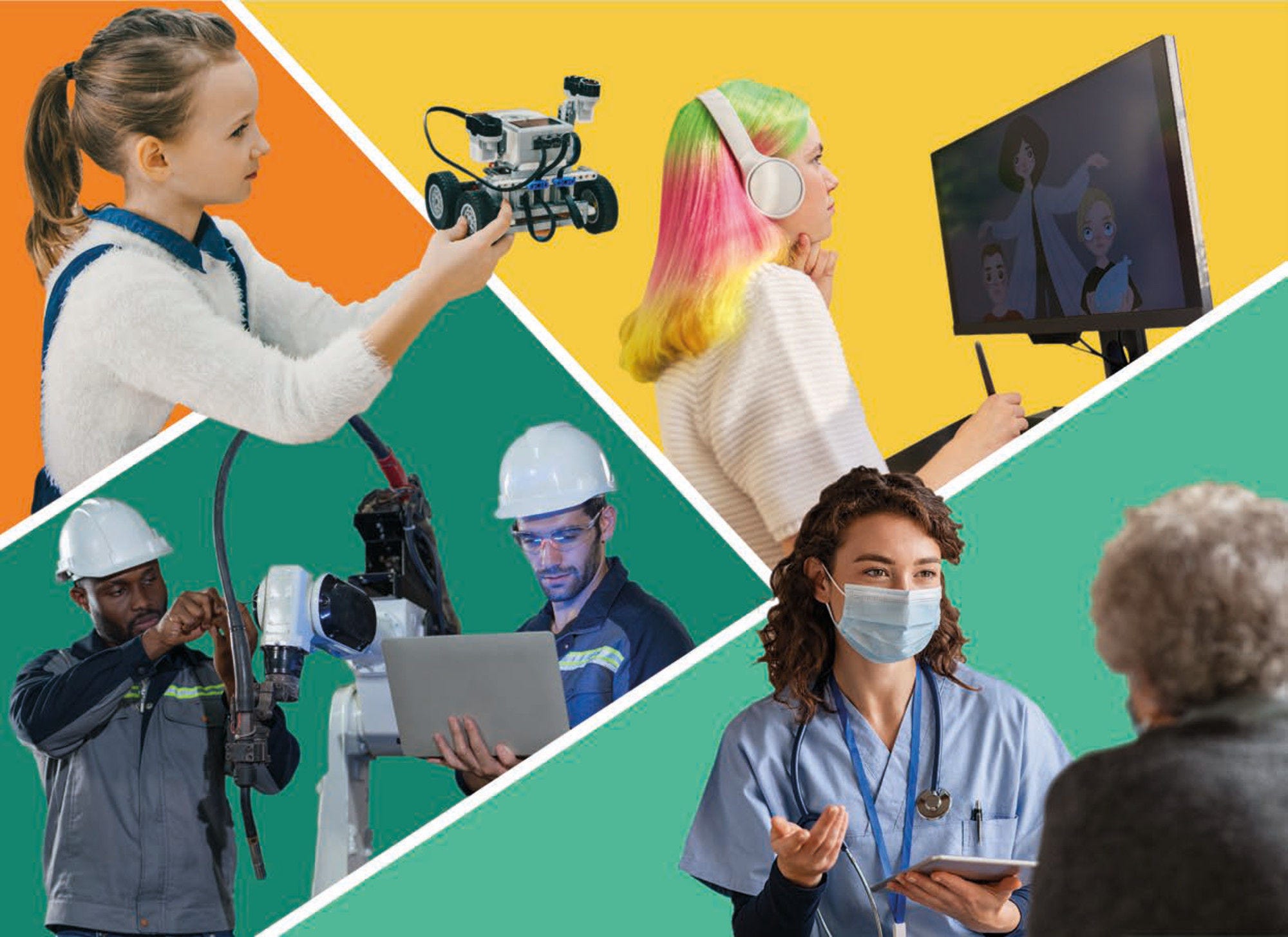
2. Evolution of human skills versus AI capabilities
Abstract
The skill level of a population is key to a country’s capacity for innovation, growth and competitiveness. Therefore, countries have large incentives to raise the supply of skills and optimise the available stock of skills. They use different policies to achieve this. Governments invest in education and try to improve the labour-market relevance of training programmes to develop the “right” skills in the future workforce – the skills that are needed by the economy and that help individuals thrive. Other policies aim at up-skilling and re-skilling the workforce, for example, by encouraging employers to offer more learning opportunities at the workplace; by strengthening lifelong learning; by activating the unemployed; or by training migrants to help them enter the labour market. However, all these policy efforts take time to contribute effectively to the formation of skills in the workforce.
By contrast, technological progress is moving fast and machines can reproduce more and more of the skills of human workers. Advances in big data, computational power, storage capacity and algorithmic techniques have driven big improvements especially in artificial intelligence (AI) and robotics capabilities over the past decade. AI is now faster, less biased and more accurate on a variety of tasks compared to humans. Language processing technology, for example, already exceeds human-level performance in speech recognition and in translation in a restricted domain. It has also made considerable progress on linguistic challenges that require logical reasoning or commonsense knowledge. In the field of vision, AI has surpassed humans in object detection, face recognition and many medical diagnostics tasks based on images. In robotics, systems are still constrained in unstructured environments. However, they have become more agile, mainly due to advances in machine learning and increased availability of sophisticated sensor systems (Zhang et al., 2022[1]).
This chapter provides background information on how human skills and computer capabilities with regard to numeracy and literacy evolve over time. By showing how much more rapidly the latter progress, the chapter highlights the need for periodically and systematically monitoring the evolution of AI capabilities and comparing them to human skills.
This chapter first analyses the skill level of adults aged 16 to 65 in the domains of literacy and numeracy and shows how it changes over time. The analysis draws on the Survey of Adult Skills (PIAAC), as well as on comparable data from two earlier skills assessments – the International Adult Literacy Survey (IALS) carried out in 1994-98 and the Adult Literacy and Life Skills Survey (ALL) carried out in 2003-07. Additional analyses focus on the reading and mathematical skills of students using data of the Programme for International Student Assessment (PISA) from 2000 to 2018. The chapter then provides an overview of recent technological developments in the fields of natural language processing (NLP) and quantitative reasoning of AI.
Changes in skills supply
Long-term developments in human skills are hard to assess. Economic studies have traditionally used average years of schooling and qualifications and diplomas attained as proxies for the supply of skills. From this perspective, skills supply should have increased across the OECD over the last decade because all member countries had an increase of the share of the adult population holding a tertiary degree (OECD, 2023[2]).
However, formal educational qualifications do not always fully capture the actual skills of individuals. For example, they do not account for skills and knowledge acquired after formal education. Nor do they capture loss of skills due to inactivity or ageing (OECD, 2012[3]). Skills assessments like PIAAC, by contrast, offer a direct measure of skills, although they are necessarily limited to a narrow set of skills.
In the following, the levels of literacy and numeracy skills of adults are presented using results from PIAAC. So far, data from only one survey wave are available. These data are combined with comparable data from IALS to address changes in literacy skills over time. Nineteen countries or economies participated in both PIAAC and IALS, with results 13-18 years apart, depending on the country.1 Changes in numeracy skills are analysed by comparing PIAAC results with those from ALL conducted in 2003 and then again between 2006 and 2008. This comparison is possible for only seven countries and has a shorter time frame of five to nine years.2
Changes in literacy skills
The comparability of the literacy data from PIAAC and IALS is limited because of changes in the assessment instruments between the two surveys. The literacy domain in PIAAC incorporates material assessed in two separate domains of prose and document literacy in IALS (OECD, 2016[4]). However, the data from IALS were re-analysed to create scores for a comparable joint literacy domain (OECD, 2013[5]). Over half of the literacy items used in PIAAC had also been used in IALS, and these linking items provided the basis for constructing comparable scales for the two surveys.
Skills are assessed on a 500-point scale, which is used to describe both the difficulty of individual test questions and the proficiency of individual adults who took the survey. For ease in understanding, the continuous scale is often described using six difficulty/proficiency levels – from below Level 1 to Level 5. Literacy questions at the lower difficulty levels (Level 1 and below) use short texts of a few sentences and ask about information that can be clearly identified in the text from the words used in the question. At the higher levels, the texts are longer and the questions may require interpreting or synthesising, as well as avoiding misleading information that may superficially appear to provide the answer. Individuals at each proficiency level can successfully complete two-thirds of the questions at that level. They also have higher chances of completing less difficult questions and lower chances of answering more difficult ones.
Figure 2.1. Literacy proficiency levels of 15-65 year-olds, IALS and PIAAC
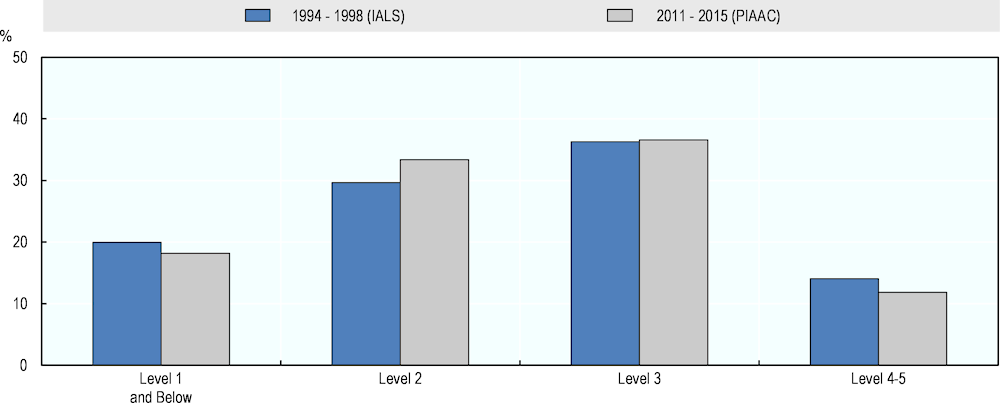
Source: Adapted from Elliott, S. (2017[6]), Computers and the Future of Skill Demand, Figure 2.1, https://doi.org/10.1787/9789264284395-en.
Figure 2.1 shows the literacy proficiency results of adults aged 16 to 65, averaged across the 19 OECD countries and economies that participated in both IALS and PIAAC. Because of relatively small numbers of adults at the top and bottom of the scale, respondents with proficiency scores at Level 1 and below Level 1 are combined in a single category, as are those at Levels 4 and 5. In PIAAC, over two-thirds of adults have literacy proficiency at Levels 2 or 3. Over the gap of 13-18 years between IALS and PIAAC, skill levels in the adult population have shifted marginally, on average. The share of adults at Level 2 increased by four percentage points, while the shares in the bottom and top categories decreased by two percentage points each.
Figure 2.2. Literacy proficiency levels of working population, IALS and PIAAC
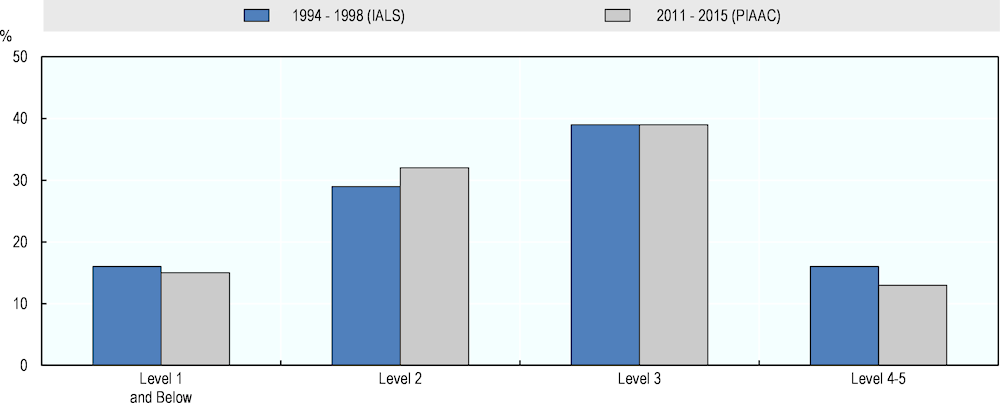
Source: Adapted from Elliott, S. (2017[6]), Computers and the Future of Skill Demand, Figure 2.2, https://doi.org/10.1787/9789264284395-en.
For comparison, Figure 2.2 shows the literacy proficiency of working adults only. The working population has similar skill levels as the full adult population. Nearly three-quarters (71%) of the employed have literacy skills at Levels 2 and 3 and 13% are proficient at the highest levels. Comparison with the IALS results shows that literacy skills of the working population have slightly decreased over time. The share of individuals at Levels 4 and 5 decreased by three percentage points, while the share of Level 2 individuals increased by four percentage points. A look at the individual countries reveals this pattern of decreasing literacy skills is more strongly pronounced in Canada, Denmark, Germany, Norway, Sweden and the United States (see Table A2.2 in Annex 2.A). Only Australia, Poland and Slovenia have shifted the distribution of literacy skills towards higher skill levels.
That literacy skills of adults do not improve over time in most countries, despite increases in educational attainment, is related to changes in the composition of the adult population (Paccagnella, 2016[7]). In the period between both surveys, all countries experienced increases in the average age of the population. In addition, in all countries, immigration has led to a higher proportion of foreign-born adults in the population. Both trends are linked to lower levels of literacy skills and counterbalance the literacy gains made by increased educational attainment.
The skills supply of a country depends not only on the skill level of the active population, but also on how well a country develops the skills of youth cohorts in preparing them to enter the workforce. PISA provides results on the knowledge and skills of young people in reading, mathematics and science. The assessment has taken place every three years since 2000, thus enabling the observation of long-term trends in students’ skills. Each round focuses on one of the three subjects and provides basic results for the other two. The first full assessment of a subject sets the starting point for future trend comparisons in this subject. Since the very first round had reading as a major domain, trends in reading performance of students can be observed since 2000.
Figure 2.3. Long-term trends in average reading proficiency of 15-year-olds
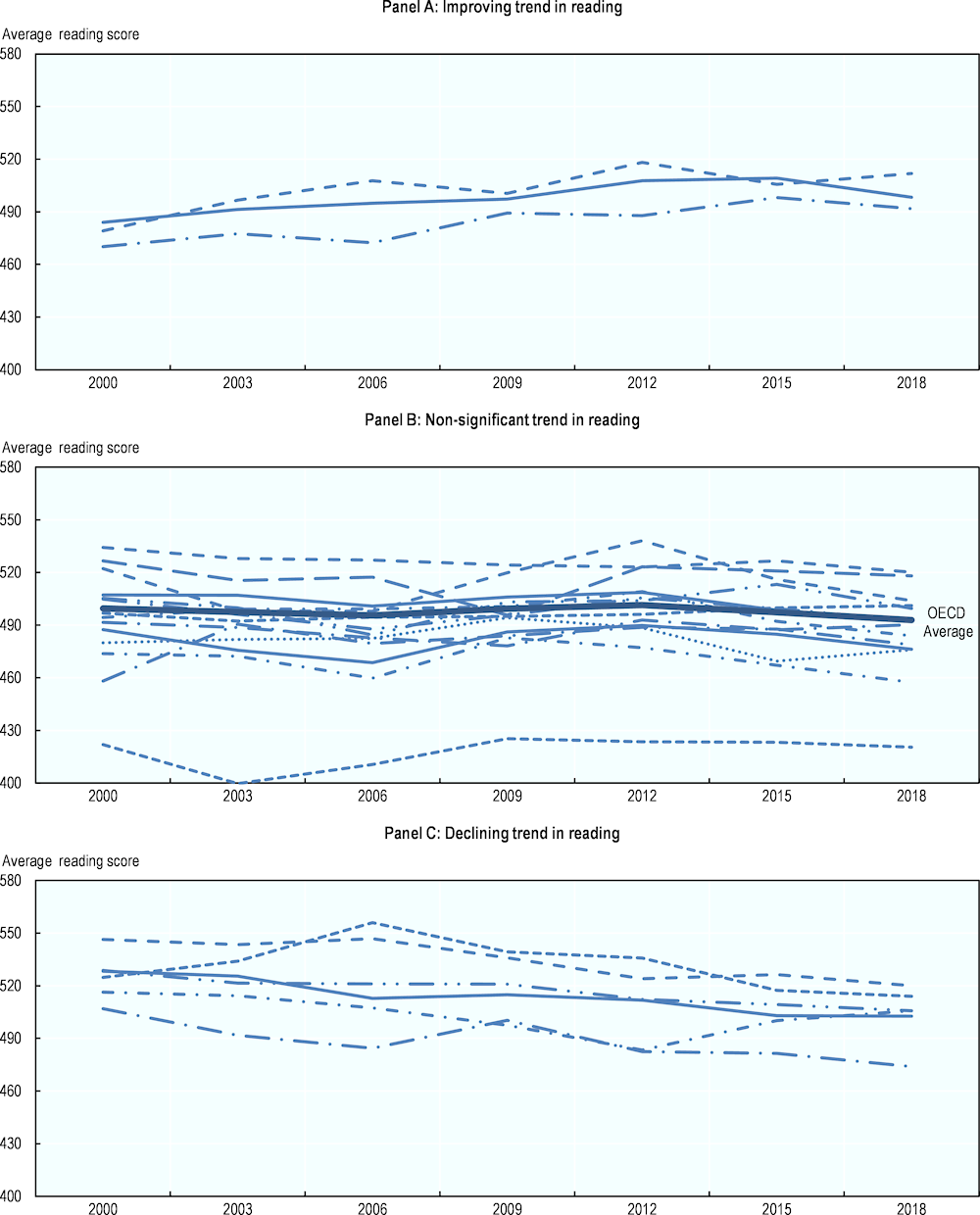
Note: Average reading scores of 23 OECD countries that took part in all PISA reading assessments since 2000. Countries' performance trends are classified as improving, not significantly changing or declining in accordance with the average three-year trend in mean performance. The average three-year trend is the average change, per three-year period, between the earliest available measurement in PISA and PISA 2018, calculated by a linear regression. Panel A presents countries with significantly positive three-year trends, Panel B presents countries with non-significant three-year trends, and Panel C shows countries with significantly negative trends.
Source: OECD (2019[8]), PISA 2018 Results (Volume I): What Students Know and Can Do, Table I.B1.10, https://doi.org/10.1787/5f07c754-en.
PISA defines reading literacy as the capacity “to understand, use, evaluate, reflect on and engage with texts in order to achieve one’s goals, develop one’s knowledge and potential, and participate in society” (OECD, 2019, p. 14[9]). Reading performance is scored in relation to the variation of the results observed across all test participants in the first main assessment. That is, scores do not have a substantive meaning. Instead, they are scaled to fit a normal distribution with a mean of 500 score points and a standard deviation of 100 score points.
Figure 2.3 presents trends in countries’ average reading scores since 2000. It focuses on 23 OECD countries that took part in all PISA reading assessments. Their performance trends are classified as improving, not significantly changing or declining in accordance with the average three-year change in mean performance between assessments. The average three-year trend in students’ reading performance is significantly positive in only three countries – Germany, Poland and Portugal (see Table A2.3, Annex 2.A). In most participating countries, young people’s reading skills have not changed significantly over time. Six countries – Australia, Finland, Iceland, Korea, New Zealand and Sweden – have declining trends in reading (see Table A2.3, Annex 2.A).
Changes in numeracy skills
It is not possible to compare data on numeracy from PIAAC and IALS. Because the numeracy domain in PIAAC is substantially different from the quantitative literacy domain included in IALS, it is not possible to construct a comparable scale for the earlier survey.
The numeracy assessment of the PIAAC is similar to that used in ALL in terms of the constructs measured and the test content (OECD, 2013[10]; Paccagnella, 2016[7]). Most of the numeracy test items used in PIAAC were used in ALL. The numeracy results of ALL have also been re-estimated to fit the measurement scale used in PIAAC. Such comparable data on numeracy is available for seven OECD countries – Canada, Hungary, Italy, the Netherlands, New Zealand, Norway and the United States.
Numeracy in PIAAC is assessed on the same 500-point scale as literacy, which, in the following, is again broken down into four proficiency levels. Respondents at a given proficiency level can solve approximately two-thirds of the questions at that level. They are also more successful on less difficult questions and less successful on more difficult ones. Questions at lower difficulty levels require respondents to perform simple, one-step operations, such as counting or sorting. Questions at higher levels of difficulty, by contrast, typically require understanding and integrating several mathematical procedures, such as reading graphs, calculating rate of change and applying formulae.
Figure 2.4. presents the distribution of the 16-65 year-old population across the four numeracy proficiency levels in ALL and PIAAC. Similar to the findings for literacy, most of the population of the observed countries has numeracy skills at medium proficiency levels (Levels 2 and 3). Comparing PIAAC to ALL results shows that, across the seven countries, on average, skills have shifted slightly from higher to lower proficiency levels. Specifically, the proportion of adults at Level 3 has decreased by three percentage points, and the proportion of adults with the poorest numeracy skills has increased by two percentage points. This trend is more strongly pronounced in Canada, Hungary, the Netherlands, Norway, and, to some extent, in the United States. Among the observed countries, numeracy skills of adults have improved only in Italy over time. However, this increase started from a large share of poorly skilled individuals.
Figure 2.4. Numeracy proficiency levels of 16-65 years-olds, ALL and PIAAC
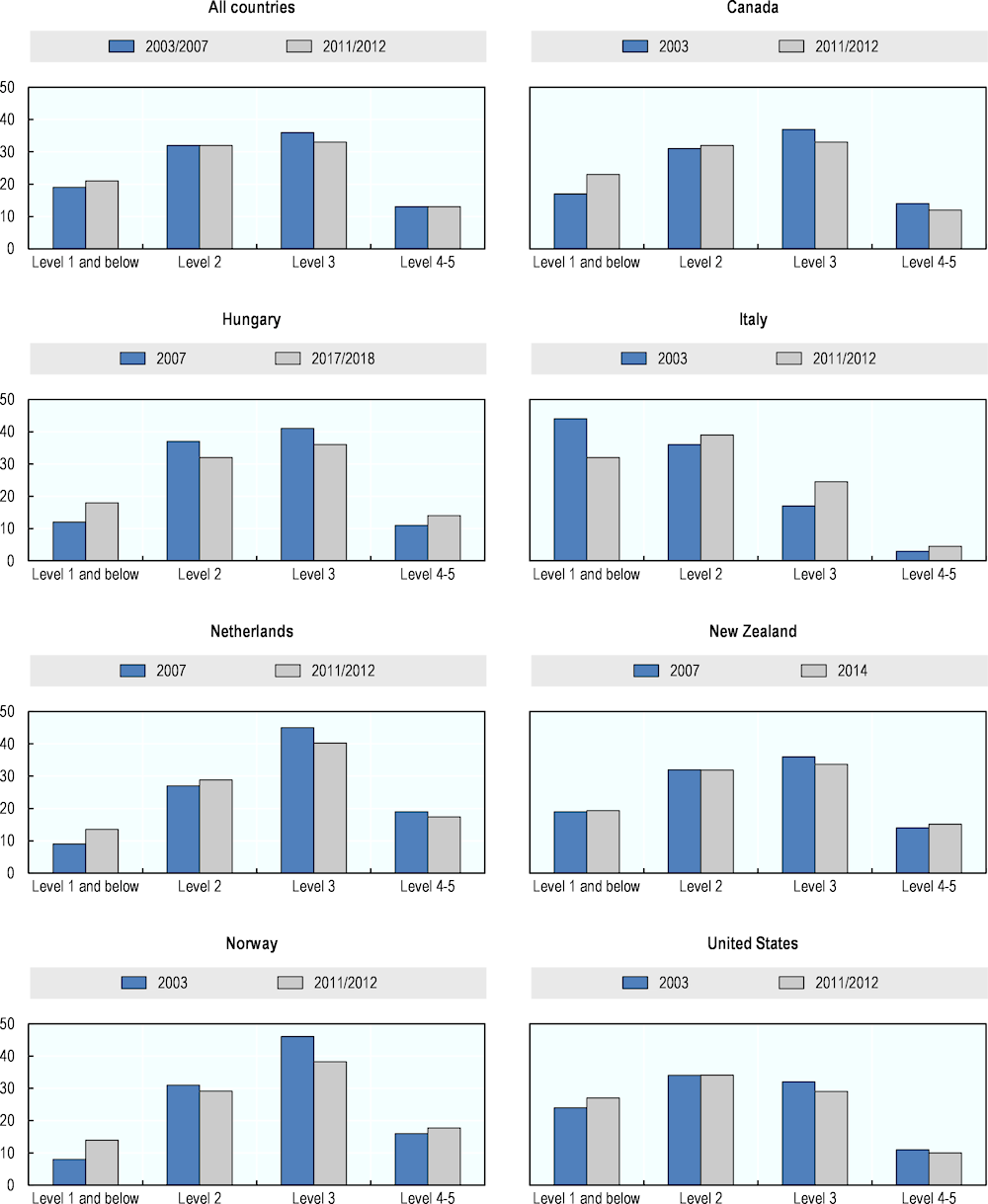
Source: US Department of Education, National Center for Education Statistics, Statistics Canada and OECD (2020[11]), Adult Literacy and Life Skills Survey (ALL) 2003-2008 and PIAAC 2012-2017 Literacy, Numeracy, and Problem Solving TRE Assessments, https://nces.ed.gov/surveys/piaac/ideuspiaac (accessed on 31 August 2022).
Figure 2.5. Numeracy proficiency levels of the working population, ALL and PIAAC
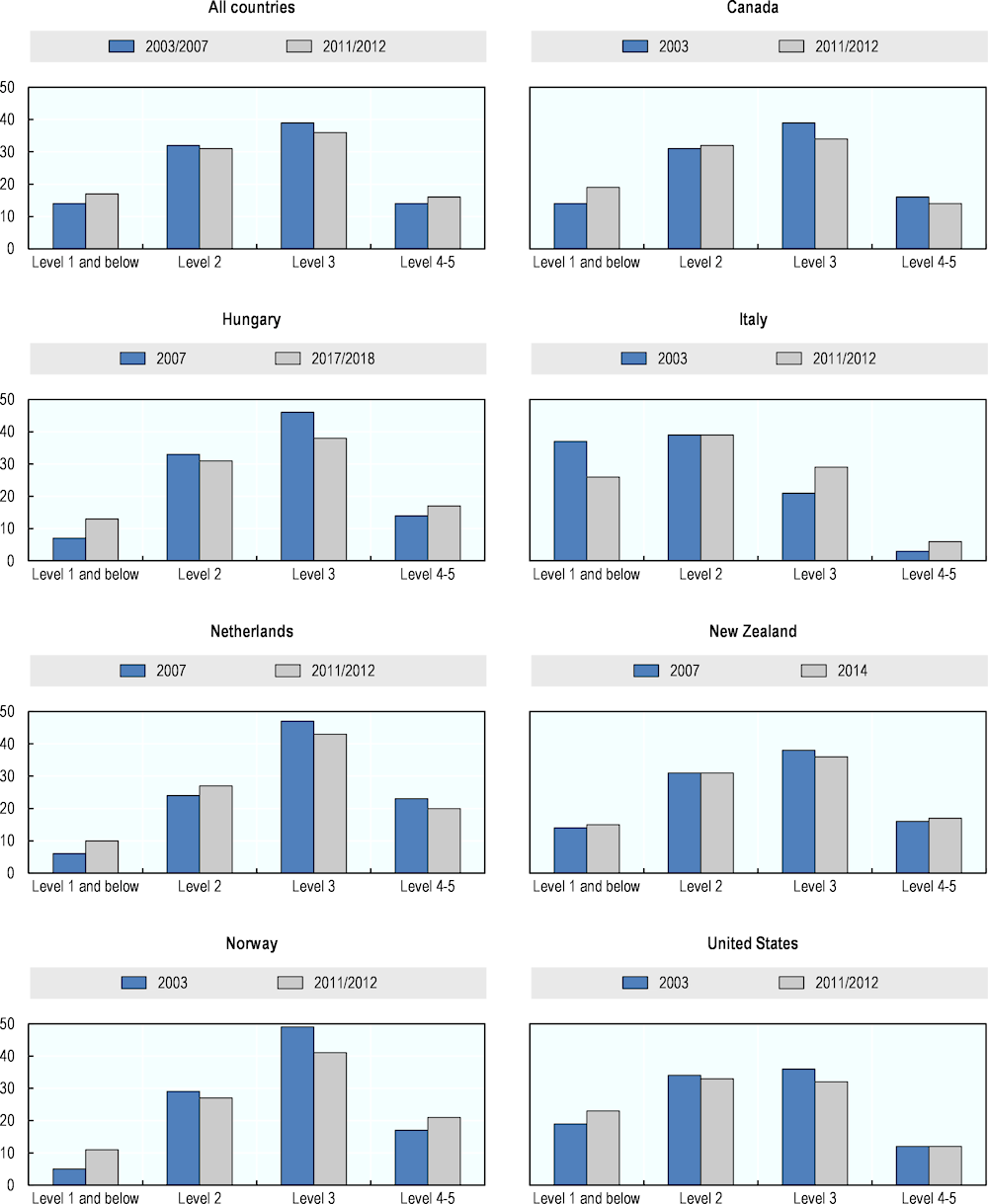
Source: US Department of Education, National Center for Education Statistics, Statistics Canada and OECD (2020[11]), Adult Literacy and Life Skills Survey (ALL) 2003-2008 and PIAAC 2012-2017 Literacy, Numeracy, and Problem Solving TRE Assessments, https://nces.ed.gov/surveys/piaac/ideuspiaac (accessed on 31 August 2022).
Figure 2.6. Long-term trends in average mathematics proficiency of 15-year-olds
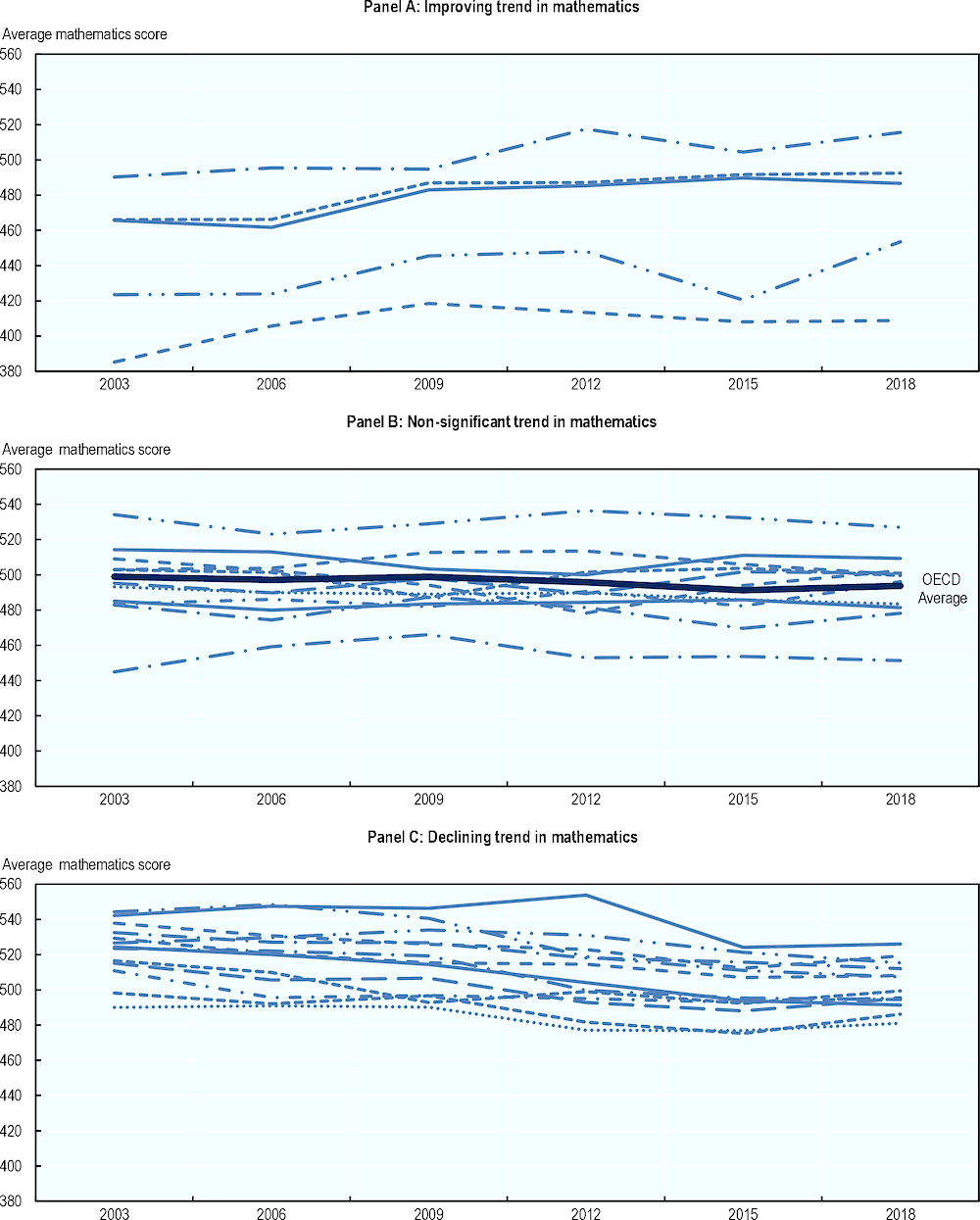
Note: Average mathematics scores of 29 OECD countries that took part in all PISA mathematics assessments since 2003. Countries' performance trends are classified as improving, not significantly changing or declining in accordance with the average three-year trend in mean performance. The average three-year trend is the average change, per three-year period, between the earliest available measurement in PISA and PISA 2018, calculated by a linear regression. Panel A presents countries with significantly positive three-year trends, Panel B presents countries with non-significant three-year trends, and Panel C shows countries with significantly negative trends.
Source: OECD (2019[8]), PISA 2018 Results (Volume I): What Students Know and Can Do, Table I.B1.11, https://doi.org/10.1787/5f07c754-en.
Figure 2.5 shows the numeracy proficiency of the working population and its change over time. Compared to the full adult population, the working population demonstrates higher numeracy skills, with smaller shares of workers having poor numeracy skills. Across all observed countries, on average, the shares of working adults with medium numeracy proficiency have slightly decreased over time. Meanwhile, the margins of the skills distribution – the shares of workers with the lowest and the higher numeracy proficiency – have increased. This pattern is observed for Hungary and Norway. In Canada and the Netherlands, the numeracy skills of working adults have shifted from higher (Levels 3-5) to lower (Level 1 and below and Level 2) proficiency level. Only Italy shows an improving trend in workers’ numeracy skills.
PISA has provided information on the mathematics skills of 15-year-old students. Comparisons of mathematics performance across time are possible from 2003 on. Mathematics skills in PISA are defined as students’ “capacity to formulate, employ and interpret mathematics in a variety of contexts” (OECD, 2019, p. 75[9]). This includes mathematical reasoning and the use of mathematical concepts and procedures to describe, explain and predict phenomena. Mathematics skills are assessed similarly to reading: scores are scaled to fit a normal distribution with a mean of 500 score points and a standard deviation of 100 score points. Students with the lowest scores can identify mathematical information that is clearly stated and perform routine mathematical procedures. Students with the highest proficiency can understand, use and conceptualise mathematical information of various types and apply advanced mathematical reasoning to solve complex problems (OECD, 2019[9]).
Figure 2.6 presents trends in average mathematical scores of 15-year-olds. The focus is on 29 OECD countries that participated in all mathematical assessments since 2003. Countries are grouped according to their average three-year score change into countries with significantly positive average change (Panel A), countries with a non-significant trend (Panel B) and countries with a significantly negative average three-year change (Panel C). The figure shows that only five of the observed countries experienced an improvement of young people’s mathematics performance since 2003 (Italy, Mexico, Poland, Portugal and Türkiye, see Table A2.4, Annex 2.A). Eleven countries have non-significant trends, while mathematical average scores declined over time in 13 countries.
In sum, numeracy and literacy skills have not changed much over time, either for the adult or the young population. Only a few countries experienced improvements in foundation skills. This may result from various factors, including population ageing, immigration or changing skill proficiency of particular groups (Paccagnella, 2016[7]). However, the small to moderate changes in literacy and numeracy show that lifting up the supply of skills is challenging for governments.
Recent developments in AI capabilities
In contrast to human skills, AI capabilities develop fast. Over the past decade, a wave of technological progress has occurred in many AI fields, including vision, NLP, speech recognition, image understanding, reinforcement learning and robotics (Littman et al., 2022[12]). This has led to the proliferation of AI applications in various contexts, such as translation, games, medical diagnosis, stock trading, autonomous driving and science. Some observers have labelled this recent uptake in AI development and deployment “a golden decade of deep learning” (Dean, 2022[13]).
The following sections briefly summarise technological developments that are relevant for the evolution of computer capabilities in the domains of literacy and numeracy. Specifically, recent progress in the fields of NLP and quantitative reasoning are described and discussed.
Recent developments in natural language processing
NLP is a major domain in AI. It aims at building computer capabilities that allow AI systems to process and interpret spoken and written language to perform different linguistic tasks. These include extracting information from large amounts of text data, correctly categorising and synthesising text content or communicating with humans.
The field consists of various sub-domains, each of which is centred around one major task or challenge. For example, Speech Recognition is a sub-domain that aims at reliably converting voice data into text, while Question-Answering deals with the automatic retrieval or generation of answers to questions posed in text or speech. Natural language technologies are typically developed within such narrow domains and focus on specific tasks. Their performance is evaluated accordingly – on domain-specific benchmarks that provide a standard for comparing different approaches in the domain. Benchmarks are test datasets, on which systems perform a task or a set of tasks (see example tasks in Box 2.1).
NLP has experienced a major surge in the last several years. In many domains, AI systems’ performance has outpaced the tests developed to measure it (Zhang et al., 2022[1]). In Question-Answering, AI systems improved so rapidly that researchers launched a more challenging version of the Stanford Question Answering Dataset (SQuAD) only two years after the benchmark’s initial release in 2016 (Rajpurkar et al., 2016[14]; Rajpurkar, Jia and Liang, 2018[15]). It took another year until systems reached human-level performance on SQuAD 2.0.3 Similarly, AI exceeded human performance on the General Language Understanding Evaluation (GLUE) benchmark within a year, and on its successor SuperGLUE soon after.4 Both benchmarks test systems on a number of distinct tasks, such as Question-Answering and commonsense reading comprehension (Wang et al., 2018[16]; Wang et al., 2019[17]).
Performance has also improved in Natural Language Inference, the task of “understanding” the relationship between sentences, e.g. whether two sentences contradict or entail each other. This is shown by benchmarks such as Stanford Natural Language Inference (SNLI) (Bowman et al., 2015[18]) and Abductive Natural Language Inference (aNLI) (Bhagavatula et al., 2019[19]). Considerable progress was also registered in text summarisation, translation and sentiment analysis (Zhang et al., 2022[1]).
This breakthrough in NLP was driven by the emergence of large pre-trained language models, such as Embeddings from Language Models (ELMo) by Peters et al. (2018[20]), Generative Pre-Trained Transformer (GPT) by Radford et al. (2018[21]) and Bidirectional Encoder Representations from Transformers (BERT) by Devlin et al. (2018[22]). These models are used to further develop specific NLP systems for particular tasks and domains. Specifically, they are trained once, on a large corpus of unlabelled text data to “learn” general language patterns and the semantic of words. The models can be then “fine-tuned” to downstream tasks, meaning they are adapted to a target task with additional training. This fine-tuning or extra training uses domain-specific training data to allow the general pre-trained models to learn the vocabulary, idioms and syntactic structures common in a new domain.
Box 2.1. Example tasks from natural language processing benchmarks
The General Language Understanding Evaluation dataset (SuperGLUE), Wang et al. (2019[17])
Text: Barq’s – Barq’s is an American soft drink. Its brand of root beer is notable for having caffeine. Barq’s, created by Edward Barq and bottled since the turn of the 20th century, is owned by the Barq family but bottled by the Coca-Cola Company. It was known as Barq’s Famous Olde Tyme Root Beer until 2012.
Question: Is Barq’s root beer a Pepsi product?
Answer: No
The Stanford Question Answering Dataset (SQuAD) 2.0, Rajpurkar, Jia and Liang (2018[15])
Text: Southern California, often abbreviated SoCal, is a geographic and cultural region that generally comprises California's southernmost 10 counties. The region is traditionally described as "eight counties", based on demographics and economic ties: Imperial, Los Angeles, Orange, Riverside, San Bernardino, San Diego, Santa Barbara, and Ventura. The more extensive 10-county definition, including Kern and San Luis Obispo counties, is also used based on historical political divisions. Southern California is a major economic centre for the state of California and the United States.
Question: What is a major importance of Southern California in relation to California and the United States?
Answer: economic centre
The Stanford Natural Language Inference (SNLI) Corpus, Bowman et al. (2015[18])
Text: A man inspects the uniform of a figure in some East Asian country.
Hypothesis: The man is sleeping.
Answer: contradiction
Choice of Plausible Alternatives (COPA), Roemmele, Adrian Bejan and S. Gordon (2011[23])
Premise: The man broke his toe. What was the CAUSE of this?
Alternative 1: He got a hole in his sock.
Alternative 2: He dropped a hammer on his foot.
Answer: Alternative 2
The Cloze Test by Teachers (CLOTH) benchmark, Xie et al. (2017[24])
Text: Nancy had just got a job as a secretary in a company. Monday was the first day she went to work, so she was very … and arrived early.
Question: A. depressed B. encouraged C. excited D. surprised
Answer: C
The introduction of these large-scale models has considerably pushed the state of the art of NLP forward. When ELMo was first introduced in 2018, it helped exceed system performance on various tasks in the domains of Question-Answering, textual entailment and sentiment analysis (Storks, Gao and Chai, 2019[25]). The release of GPT pushed forward AI performance on 12 benchmarks, including GLUE, SNLI and the Choice of Plausible Alternatives (COPA) benchmark (Roemmele, Adrian Bejan and S. Gordon, 2011[23]), which evaluates commonsense causal reasoning. Its later updated versions GPT-2 and GPT-3 further improved state-of-the-art results on numerous language modelling tasks. Similarly, BERT topped several benchmark performance rankings when first released, such as those of GLUE, SQuAD, COPA, Situations With Adversarial Generation (SWAG) (Zellers et al., 2018[26]) on commonsense reasoning and CLOze test by TeacHers (CLOTH) (Xie et al., 2017[24]), a collection of questions from middle and high school-level English language exams.
Remarkably, these models perform well on novel tasks without considerable additional training. When applied without any subsequent fine-tuning, GPT-3, for example, achieves strong performance on numerous language tasks. Indeed, in many cases, it outpaces state-of-the-art systems designed for the task (Brown et al., 2020[27]). Performance is even better in settings, where the model is provided with only one demonstration of the novel task or with few prior examples (typically 10-100).
As another remarkable feature, pre-trained language models can perform a huge variety of tasks, even without being explicitly trained for those tasks. In the above example, a GPT-3 without fine-tuning performed well on tasks as diverse as translation, Question-Answering, reading comprehension, reasoning or three-digit arithmetic. These qualities of language models are a gateway towards producing more general AI systems – systems that can adapt to new situations and solve problems from different domains without extensive additional training.
The success of pre-trained language models is mostly due to the use of self-supervised learning. This allows for training models on unprecedented amounts of training data. In self-supervised learning, neural network models are trained on texts, parts of which are made hidden. The task of the model is to predict the hidden words as function of the context in which they appear. In this way, the model “learns” grammar rules and semantics. This approach does not require a human to label training examples as true or false, thus enabling the use of more training data. Moreover, training occurs only once, which significantly reduces the costs and time to develop a system. Researchers can simply download a general pre-trained language model and fine-tune it for a specific task on a smaller amount of domain-specific data.
The Transformer architecture is one of the most advanced and widely used self-supervised approaches. Both BERT and GPT are pre-trained on Transformers. Its crucial feature is a “self-attention” mechanism that allows for capturing long-range dependencies between words (e.g. words far apart in a sentence) (Littman et al., 2022[12]). In addition, Transformers and similar architectures allow for learning the meaning of words in context. For example, the word “rose” would be represented differently in the sentences “Roses are red” and “The sun rose”. This is a considerable advantage over earlier pre-trained word-embedding models like word2vec (Mikolov et al., 2013[28]). In such models, words are represented with the same vector, independent of the context, in which they are used.
While these new approaches considerably advanced the field of NLP, they are still far from producing AI systems that can process language as humans do. The reason is that NLP systems still lack a deep understanding of speech and text. This limits their capacity to perform more sophisticated language tasks that require commonsense knowledge and complex reasoning.
Recent developments in mathematical reasoning of AI
Research on automating mathematical reasoning has a long history with important achievements. These include the development of tools, such as Maple, Mathematica and Matlab, that can perform numerical and symbolic mathematical operations. Significant effort has also gone into automated theorem proving, with major achievements, such as proving the four-colour theorem among others (Appel and Haken, 1977[29]). This section focuses narrowly on mathematical benchmarks that are comparable to the PIAAC numeracy test.
From the perspective of AI research, mathematical problems can be divided roughly into the following categories (Davis, 2023[30]):
Symbolic problems: problems formulated in mathematical notation with minimal use of natural language. For example, “Solve x3 − 6x2 + 11x − 6 = 0”.
Word problems: problems stated in (more than minimal) natural language, possibly in combination with symbols.
Purely mathematical word problems: problems with minimal reference to non-mathematical concepts. E.g. “Find a prime number p such that p+36 is a square number.”
Real-world word problems: problems whose solution requires using non-mathematical knowledge.
Commonsense word problems (CSW): problems involving significant use of commonsense knowledge and possibly common knowledge, but not encyclopedic or expert knowledge. Elementary CSWs require only elementary mathematics (Davis, 2023[30]).
Researchers have been trying to develop systems that solve mathematical word problems since the 1960s (Davis, 2023[30]). However, the dominance of machine-learning techniques over the past two decades has also affected AI research in mathematical reasoning. As a result, much recent work aims at generalising large-scale, pre-trained language models to mathematical problems and the quantitative aspects of natural language (Lewkowycz et al., 2022[31]; Saxton et al., 2019[32]). While acknowledging other types of AI research, this brief summary focuses on recent efforts, featuring some prominent benchmarks and the performance of deep/machine-learning systems on these (see Box 2.2).
Mathematical reasoning has received less attention in AI research than language modelling or vision because it has relatively less applicability and commercial use. However, some AI experts argue that mathematical reasoning poses an interesting challenge for AI (Saxton et al., 2019[32]). It requires learning, planning, inferring and exploiting laws, axioms and mathematical rules, among others. These capabilities can enable more powerful and sophisticated systems that can solve more complex, real-world problems.
Mathematics is generally considered hard for AI (Choi, 2021[33]). In 2019, researchers from DeepMind Technologies, an AI-focused company owned by Google, tested the performance of state-of-the-art NLP models in the domain of mathematics (Saxton et al., 2019[32]). For that purpose, they developed a test dataset of questions from the areas of algebra, arithmetic, calculus, comparisons and measurement, among others. In addition, they evaluated systems on publicly available mathematics exams for 16-year-old schoolchildren in Britain. The best-performing model in the study, the Transformer, achieved moderate results on the test dataset. It also failed the school maths exam, answering correctly only 14 of the 40 questions (O’Neill, 2019[34]).
To facilitate research in the field, researchers from University of California, Berkeley introduced MATH in 2021, a test dataset containing 12 500 challenging mathematics problems (Hendrycks et al., 2021[35]). The questions are in textual format and span different fields of mathematics. Large language models, pre-trained on mathematical content and presented with examples from MATH, achieved poor results on the benchmark at the time of release, with accuracy of 3‑6.9%. However, MATH is also challenging for humans. A three-time gold medallist in the International Mathematical Olympiad attained 90% on the test, while a PhD student in computer science achieved 40%.
Box 2.2. Example tasks from benchmarks on mathematical reasoning
MATH Dataset, Hendrycks et al. (2021[35])
Problem: Tom has a red marble, a green marble, a blue marble, and three identical yellow marbles. How many different groups of two marbles can Tom choose?
Solution: There are two cases here: either Tom chooses two yellow marbles (1 result), or he chooses two marbles of different colours (results). The total number of distinct pairs of marbles Tom can choose is 1 + 6 = 7.
GSM8K, Cobbe et al. (2021[36])
Problem: Beth bakes 4, 2 dozen batches of cookies in a week. If these cookies are shared amongst 16 people equally, how many cookies does each person consume?
Solution: Beth bakes 4 2 dozen batches of cookies for a total of 4*2=8 dozen cookies. There are 12 cookies in a dozen, and she makes 8 dozen cookies for a total of 12*8=96 cookies. She splits the 96 cookies amongst 16 people so they each eat 96/16=6 cookies.
Saxton et al. (2019[32])
Question: Solve -42*r + 27*c = -1167 and 130*r + 4*c = 372 for r.
Answer: 4
MathQA, Amini et al. (2019[37])
Question: A train running at the speed of 48 km/hr crosses a pole in 9 seconds. What is the length of the train? a) 140, b) 130, c) 120, d) 170, e) 160
Answer: C
NumGLUE, Mishra et al. (2022[38])
Question: A man can lift one box in each of his hands. How many boxes can a group of 5 people hold in total?
Answer: 10
Similarly, in 2021, researchers from OpenAI, a prominent AI research laboratory, released GSM8K, a dataset of 8 500 diverse mathematics problems at grade school-level in textual form (Cobbe et al., 2021[36]). The questions require a sequence of several simple arithmetic operations to solve. They are generally easier than MATH test questions. A well-performing middle-school student is expected to correctly solve the entire test, for example. Nevertheless, competing AI methods achieved low to moderate results on the test when it was released.
Some developments illustrate how large language models have been applied in the field of mathematical reasoning. In 2022, Google introduced Minerva, a large language model pre-trained on general natural language data and further fine-tuned on technical content (Lewkowycz et al., 2022[31]). Currently, the model tops the MATH benchmark (as of 21 February 2023).5 In addition, Minerva achieved good results on questions from engineering, chemistry, physics, biology and computer science, stemming from the Massive Multitask Language Understanding dataset (Hendrycks et al., 2021[35]). The model also obtained a score of 57% on the National Maths Exam in Poland, which corresponds to the average human performance in 2021.
In the same year, Codex (Chen et al., 2021[39]), a system developed by Open AI, achieved high accuracy on subsets of the MATH dataset (Hendrycks et al., 2021[35]), as well as on questions from university-level mathematics courses (Drori et al., 2021[40]). The model is a neural network pre-trained on text and fine- tuned on publicly available code. While the system’s achievement has been acknowledged by the community, its reported high performance has been questioned on numerous grounds (Davis, 2022[41]). Criticism includes that it is not the neural network that solves the problems but a mathematical tool that it invokes (a Python algebra package) and that the system may work based on correct answers recorded in the test corpus. In addition, as language models, both Minerva and Codex are limited to textual input, and cannot handle diagrams and graphs, which are often essential elements of mathematical problems.
More recently, in 2022, several datasets have been assembled in the collection LILA that combines 23 existing benchmarks and covers a variety of linguistic complexity and mathematical difficulty (Mishra et al., 2022[42]). Systems, such as Codex, GPT-3, Neo-P and Bashkara, perform with varying success in the different mathematical domains on LILA (Davis, 2023[30]).
Despite some successes, AI is still far from mastering mathematical problems. State-of-the-art results on the MATH or LILA datasets, for example, are still far below the benchmark’s ceiling. There is also room for improvement with regard to performance on GSM8K. To date, no AI system can solve elementary commonsense word problems reliably (Davis, 2023[30]). Moreover, prominent benchmarks in the field focus strongly on quantitative problems stated in text – “math word problems” – leaving other mathematical tasks unaddressed. In particular, mathematical tasks that include visual content, such as figures, tables, diagrams or other images, have received less attention.
The importance of measuring AI capabilities
The analysis presented in this chapter shows that AI capabilities in core domains develop much faster over time than human skills. Over the last two decades, literacy and numeracy skills of adults, of adult workers and of youth have increased in only but a few countries. This highlights the fact that uplifting the supply of skills in an economy is not a trivial task for policy makers and education providers. At the same time, AI technology develops quickly, excelling its capabilities and acquiring new ones. The last five years have seen tremendous breakthroughs in NLP, leading to improved capabilities of AI in literacy. In the domain of numeracy, technological progress, although at a smaller scale, is under way.
These developments give rise to important questions for policy and education:
Will new AI capabilities result in substantial numbers of people whose skills are below those of AI across important capabilities used at work?
What education and training will be needed for most people to develop some work-related capabilities beyond those of AI and robotics?
What human capabilities will be too difficult for AI and robotics to reproduce over the next few decades?
While this chapter focuses on the supply side of skills, much previous research studies how technological change affects the demand for human skills. The notion is often that technological change is task-biased, meaning that machines can substitute workers in some tasks better than in others (Autor, Levy and Murnane, 2003[43]; Frey and Osborne, 2017[44]). This would result in decreasing demand for workers for tasks that are automatable. At the same time, demand for workers in tasks that relate to the deployment and monitoring of machines at the workplace would increase. Many studies try to understand which tasks machines can automate (see Chapter 1). This has important implications:
Which occupations are at high risk of automation?
Will AI have a greater effect on the demand for low-skill or high-skill workers? Younger or older workers? Workers with more or less education?
How might new AI capabilities change the overall amount of education or the types of capabilities that people need for work?
A systematic assessment of AI and robotic capabilities that allows for comparisons with human skills can provide answers to the above questions. The following study demonstrates how the use of standardised skills assessments and expert knowledge can help track AI capabilities in core domains of human skills.
References
[37] Amini, A. et al. (2019), “MathQA: Towards Interpretable Math Word Problem Solving with Operation-Based Formalisms”.
[29] Appel, K. and W. Haken (1977), “The Solution of the Four-Color-Map Problem.”, Scientific American, Vol. 237/4, pp. 108-121, http://www.jstor.org/stable/24953967.
[43] Autor, D., F. Levy and R. Murnane (2003), “The Skill Content of Recent Technological Change: An Empirical Exploration”, The Quarterly Journal of Economics, Vol. 118/4, pp. 1279-1333, https://doi.org/10.1162/003355303322552801.
[19] Bhagavatula, C. et al. (2019), “Abductive Commonsense Reasoning”.
[18] Bowman, S. et al. (2015), “A large annotated corpus for learning natural language inference”.
[27] Brown, T. et al. (2020), “Language Models are Few-Shot Learners”.
[39] Chen, M. et al. (2021), “Evaluating Large Language Models Trained on Code”.
[33] Choi, C. (2021), 7 revealing ways AIs fail. Neural networks can be disastrously brittle, forgetful, and surprisingly bad at math, IEEE Spectrum for the Technology Insider, https://spectrum.ieee.org/ai-failures (accessed on 1 February 2023).
[36] Cobbe, K. et al. (2021), “Training Verifiers to Solve Math Word Problems”.
[30] Davis, E. (2023), Mathematics, word problems, common sense, and artificial intelligence, https://arxiv.org/pdf/2301.09723.pdf (accessed on 28 February 2023).
[41] Davis, E. (2022), Limits of an AI program for solving college math problems, https://arxiv.org/pdf/2208.06906.pdf (accessed on 5 February 2023).
[13] Dean, J. (2022), “A Golden Decade of Deep Learning: Computing Systems & Applications”, Daedalus, Vol. 151/2, pp. 58-74, https://doi.org/10.1162/daed_a_01900.
[22] Devlin, J. et al. (2018), “BERT: Pre-training of Deep Bidirectional Transformers for Language Understanding”.
[40] Drori, I. et al. (2021), “A Neural Network Solves, Explains, and Generates University Math Problems by Program Synthesis and Few-Shot Learning at Human Level”, https://doi.org/10.1073/pnas.2123433119.
[6] Elliott, S. (2017), Computers and the Future of Skill Demand, Educational Research and Innovation, OECD Publishing, Paris, https://doi.org/10.1787/9789264284395-en.
[44] Frey, C. and M. Osborne (2017), “The future of employment: How susceptible are jobs to computerisation?”, Technological Forecasting and Social Change, Vol. 114, pp. 254-280, https://doi.org/10.1016/j.techfore.2016.08.019.
[35] Hendrycks, D. et al. (2021), “Measuring Mathematical Problem Solving With the MATH Dataset”.
[31] Lewkowycz, A. et al. (2022), “Solving Quantitative Reasoning Problems with Language Models”.
[12] Littman, M. et al. (2022), “Gathering Strength, Gathering Storms: The One Hundred Year Study on Artificial Intelligence (AI100) 2021 Study Panel Report”.
[28] Mikolov, T. et al. (2013), “Efficient Estimation of Word Representations in Vector Space”.
[42] Mishra, S. et al. (2022), “Lila: A Unified Benchmark for Mathematical Reasoning”.
[38] Mishra, S. et al. (2022), “NumGLUE: A Suite of Fundamental yet Challenging Mathematical Reasoning Tasks”.
[2] OECD (2023), Adult education level (indicator), https://doi.org/10.1787/36bce3fe-en (accessed on 1 February 2023).
[9] OECD (2019), PISA 2018 Assessment and Analytical Framework, PISA, OECD Publishing, Paris, https://doi.org/10.1787/b25efab8-en.
[8] OECD (2019), PISA 2018 Results (Volume I): What Students Know and Can Do, PISA, OECD Publishing, Paris, https://doi.org/10.1787/5f07c754-en.
[4] OECD (2016), The Survey of Adult Skills: Reader’s Companion, Second Edition, OECD Skills Studies, OECD Publishing, Paris, https://doi.org/10.1787/9789264258075-en.
[5] OECD (2013), Technical Report of the Survey of Adult Skills (PIAAC), https://www.oecd.org/skills/piaac/_Technical%20Report_17OCT13.pdf (accessed on 1 February 2023).
[10] OECD (2013), The Survey of Adult Skills: Reader’s Companion, OECD Publishing, Paris, https://doi.org/10.1787/9789264204027-en.
[3] OECD (2012), Better Skills, Better Jobs, Better Lives: A Strategic Approach to Skills Policies, OECD Publishing, Paris, https://doi.org/10.1787/9789264177338-en.
[34] O’Neill, S. (2019), “Mathematical Reasoning Challenges Artificial Intelligence”, Engineering, Vol. 5/5, pp. 817-818, https://doi.org/10.1016/j.eng.2019.08.009.
[7] Paccagnella, M. (2016), “Literacy and Numeracy Proficiency in IALS, ALL and PIAAC”, OECD Education Working Papers, No. 142, OECD Publishing, Paris, https://doi.org/10.1787/5jlpq7qglx5g-en.
[20] Peters, M. et al. (2018), “Deep contextualized word representations”.
[21] Radford, A. et al. (2018), Improving Language Understanding by Generative Pre-Training, https://s3-us-west-2.amazonaws.com/openai-assets/research-covers/language-unsupervised/language_understanding_paper.pdf (accessed on 1 February 2023).
[15] Rajpurkar, P., R. Jia and P. Liang (2018), “Know What You Don’t Know: Unanswerable Questions for SQuAD”.
[14] Rajpurkar, P. et al. (2016), “SQuAD: 100,000+ Questions for Machine Comprehension of Text”.
[23] Roemmele, M., C. Adrian Bejan and A. S. Gordon (2011), Choice of Plausible Alternatives: An Evaluation of Commonsense Causal Reasoning, http://commonsensereasoning.org/2011/papers/Roemmele.pdf (accessed on 1 February 2023).
[32] Saxton, D. et al. (2019), “Analysing Mathematical Reasoning Abilities of Neural Models”.
[45] SQuAD2.0 (2023), The Stanford Question Answering Dataset. Leaderboard, https://rajpurkar.github.io/SQuAD-explorer/ (accessed on 21 January 2023).
[25] Storks, S., Q. Gao and J. Chai (2019), “Recent Advances in Natural Language Inference: A Survey of Benchmarks, Resources, and Approaches”.
[11] US Department of Education, National Center for Education Statistics, Statistics Canada and OECD (2020), Program for the International Assessment of Adult Competencies (PIAAC), Adult Literacy and Life Skills Survey (ALL) 2003-2008 and PIAAC 2012-2017 Literacy, Numeracy, and Problem Solving TRE Assessments, https://nces.ed.gov/surveys/piaac/ideuspiaac (accessed on 31 August 2022).
[17] Wang, A. et al. (2019), “SuperGLUE: A Stickier Benchmark for General-Purpose Language Understanding Systems”.
[16] Wang, A. et al. (2018), “GLUE: A Multi-Task Benchmark and Analysis Platform for Natural Language Understanding”.
[24] Xie, Q. et al. (2017), “Large-scale Cloze Test Dataset Created by Teachers”.
[26] Zellers, R. et al. (2018), “SWAG: A Large-Scale Adversarial Dataset for Grounded Commonsense Inference”.
[1] Zhang, D. et al. (2022), “The AI Index 2022 Annual Report”, https://aiindex.stanford.edu/wp-content/uploads/2022/03/2022-AI-Index-Report_Master.pdf (accessed on 20 February 2023).
Annex 2.A. Supplementary tables
Annex Table 2.A.1. List of online tables for Chapter 2
Table Number |
Table Title |
---|---|
Table A2.1 |
Distribution of adult population by level of literacy, IALS and PIAAC |
Table A2.2 |
Distribution of workers by level of literacy, IALS and PIAAC |
Table A2.3 |
Mean reading PISA score since 2000 and average 3-year trend in reading performance, by country |
Table A2.4 |
Mean mathematics PISA score since 2003 and average 3-year trend in mathematics performance, by country |
Notes
← 1. The countries or economies participating in both PIAAC and IALS comprise Australia, Canada, Chile, the Czech Republic, Denmark, England (United Kingdom), Finland, Flanders (Belgium), Germany, Ireland, Italy, the Netherlands, New Zealand, Northern Ireland (United Kingdom), Norway, Poland, Slovenia, Sweden and the United States.
← 2. The countries participating in both PIAAC and ALL comprise Canada, Hungary, Italy, the Netherlands, New Zealand, Norway and the United States.
← 3. The leaderboard of SQuAD 2.0 can be found under: https://rajpurkar.github.io/SQuAD-explorer/ (accessed on 21 January 2023)
← 4. The leaderboard of SuperGLUE can be found under: https://super.gluebenchmark.com/leaderboard (accessed on 21 January 2023)
← 5. A ranking of systems’ performance on MATH can be found under: www.paperswithcode.com/sota/math-word-problem-solving-on-math (accessed on 21 February 2023).