The chapter analyses changes in assessed literacy and numeracy capabilities in artificial intelligence (AI) between 2016 and 2021. To that end, it compares the majority responses of the expert groups that completed the pilot and the follow-up assessments. In addition, it looks at how the AI evaluations of experts who participated in both studies changed over the period. The chapter also studies the level of experts’ agreement and the prevalence of uncertain answers in both assessments to compare the quality of group ratings obtained in 2016 and 2021. Subsequently, it analyses experts’ projections of how AI capabilities will evolve by 2026 to obtain information on the likely direction of AI progress in the near future.
Is Education Losing the Race with Technology?
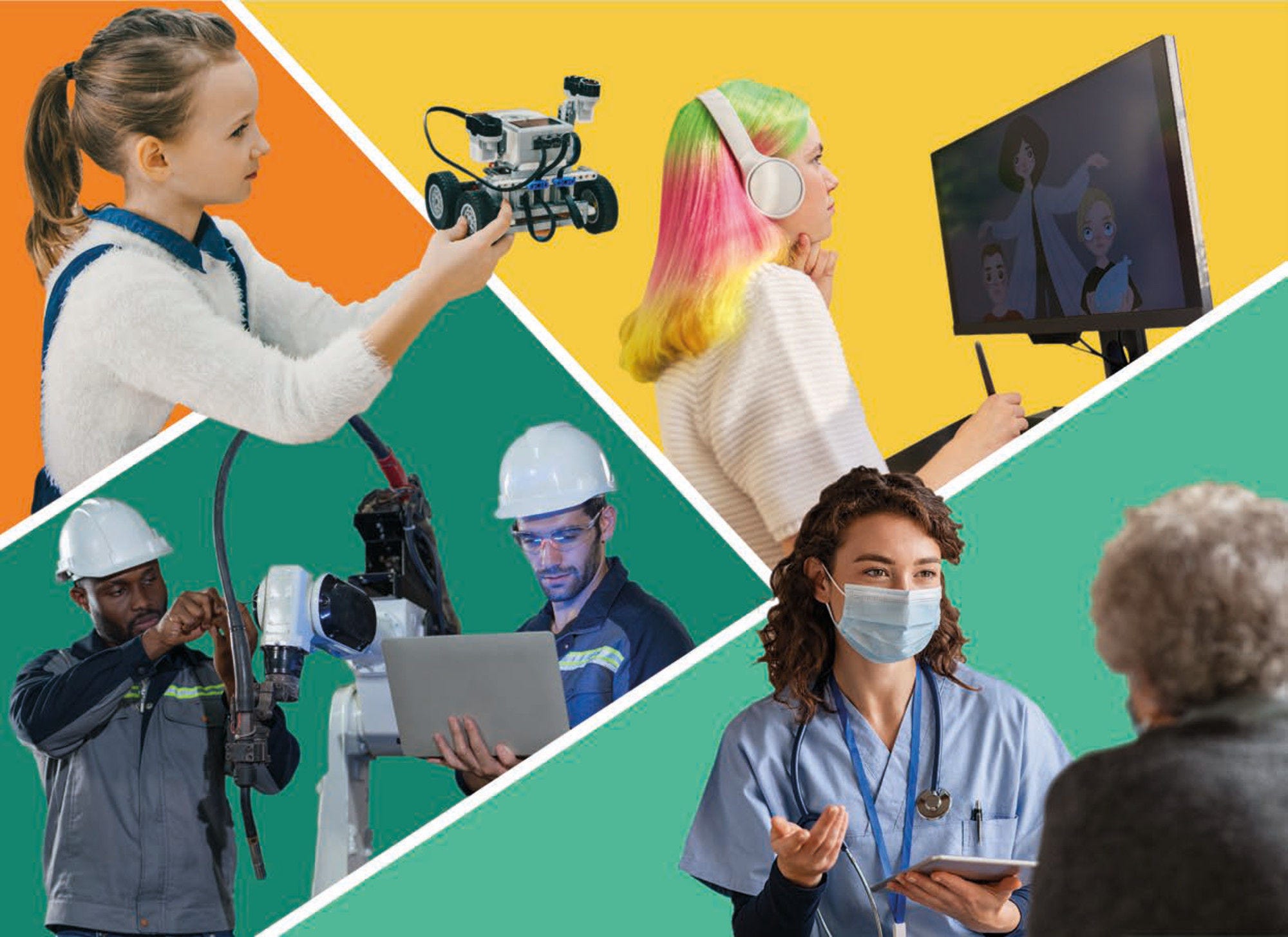
5. Changes in AI capabilities in literacy and numeracy between 2016 and 2021
Abstract
Tracking advances in artificial intelligence (AI) is important for anticipating the impacts of this technology on work and education. A periodical assessment of AI capabilities can provide information on the direction, pace and content of technological developments in the AI field. This knowledge base can help policy makers develop realistic scenarios about how jobs and skill demand will be redefined and how to reshape education and labour-market policies in response.
This chapter compares results of the 2016 and 2021 assessments to study how AI capabilities in literacy and numeracy developed over the period. In 2016, during a two-day workshop, 11 computer scientists rated potential AI performance on the literacy, numeracy and problem-solving tests of the Programme for the International Assessment of Adult Competencies (PIAAC). They rated whether AI could solve each test question with a Yes, Maybe or No. Three computer scientists also assessed whether AI could solve the questions in ten years’ time, that is, in 2026 (Elliott, 2017[1]).
In the follow-up assessment of 2021, 11 experts, six of whom participated in the pilot, assessed AI in literacy and numeracy in an online survey and discussed the results during a four-hour workshop. They followed similar instructions for rating as in 2016. However, they used a different rating scale, ranging from 0% (confident that AI cannot solve the question) to 100% (confident that AI can solve the question). Four other experts in mathematical reasoning of AI completed the numeracy assessment with revised rating instructions. In addition, all experts predicted the evolution of AI capabilities over the next five years.
The chapter first analyses changes in reported AI literacy capabilities since 2016, as well as experts’ predictions of how these capabilities will evolve by 2026. The chapter then compares AI numeracy performance ratings provided by the 11 experts in 2016 with those of the 15 experts in 2021 and presents forecasts for AI numeracy performance in the future.
Change in AI literacy capabilities over time
The follow-up assessment aimed at both providing comparability to the 2016 results and improving methods for collecting expert judgements on AI capabilities with PIAAC. Some notable improvements to the 2016 assessment include use of a facilitated discussion technique and a five-point scale to assess experts’ ratings and their confidence in these ratings. The scale is presented in Figure 5.1, together with the answer categories used in 2016. While the 0%- and 100%-categories represent confident negative and positive ratings, respectively, the 25%- and the 75%-answers express lower confidence. In the following, 0%- and 25%-ratings are grouped into a single category, as are 75%- and 100%-ratings. This makes the answer-categories used in 2021 comparable to the Yes-, Maybe- and No-categories used in 2016 (see Figure 5.1).
Figure 5.1. Answer categories used in the 2016 and 2021 assessments
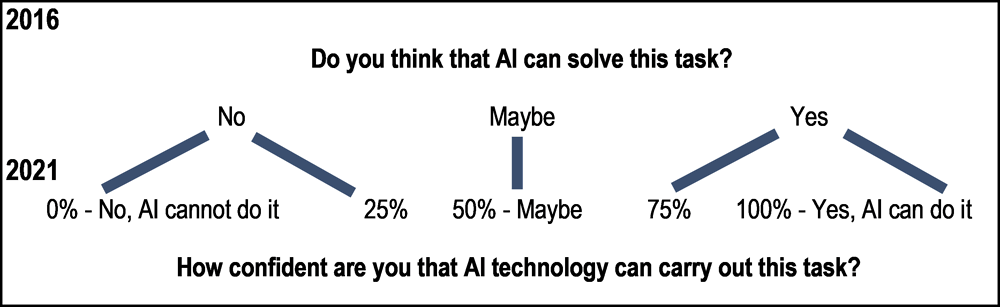
To aggregate experts’ ratings into a single AI measure, the study labels each literacy question as solvable or not solvable by AI following the evaluation of most experts. Subsequently, it estimates the share of literacy questions that AI can solve at each level of question difficulty.
Change in AI literacy performance between 2016 and 2021
Figure 5.2 compares the aggregate results in literacy in the 2016 and 2021 assessments.1 The measures rely on the majority between negative and positive ratings, omitting Maybe-ratings. The results suggest considerable improvement in AI performance in the literacy test. In 2016, AI could correctly answer 71% of Level 2 questions, 48% of Level 3 questions and 20% of questions at Level 4 and above, according to most experts. In 2021, the success rates at these difficulty levels ranged between 93% and 68%. This represents an improvement of 25 percentage points of AI on the entire PIAAC literacy test – from 55% of questions that AI can solve according to the majority of experts in 2016 to 80% in 2021.
Figure 5.2. AI literacy performance in 2016 and 2021, by question difficulty
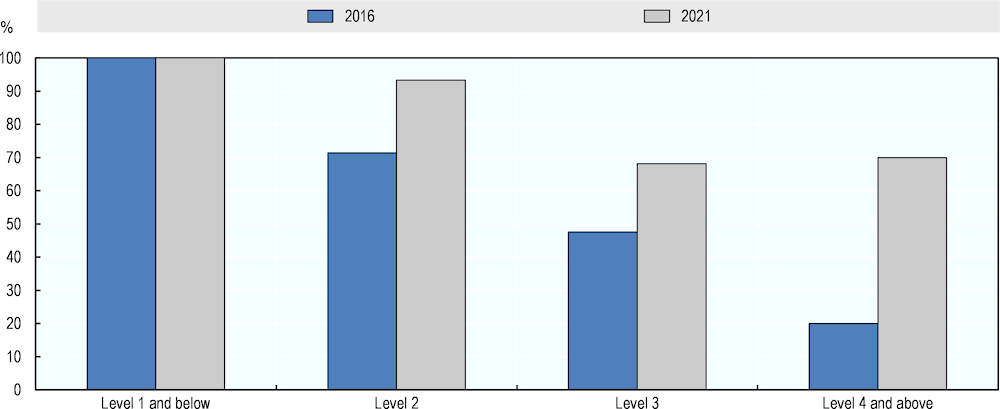
Source: Adapted from Elliott, S. (2017[1]), Computers and the Future of Skill Demand, https://doi.org/10.1787/9789264284395-en.
In addition, Figure 5.3 presents AI literacy measures from 2016 and 2021 that include Maybe-ratings as partial Yes-answers. Concretely, Maybe-answers are treated as Yes-votes weighted by 0.5. The vote that exceeds 50% of all ratings, including Maybe-ratings, is then used to determine AI’s success on each question. AI literacy scores for both years are higher when counting Maybe as a half Yes. However, the overall picture remains similar. In 2021, AI literacy performance exceeded performance assessed in 2016 at question difficulty Level 2 and higher.
These results reflect progress in the field of natural language processing (NLP) since 2016. As described in Chapter 2, NLP has seen tremendous advances since the introduction of large pre-trained language models in 2018. These include ELMo (Embeddings from Language Models) (Peters et al., 2018[2]), GPT (Generative Pre-Trained Transformer) (Radford et al., 2018[3]), and BERT (Bidirectional Encoder Representations from Transformers) (Devlin et al., 2018[4]). These models are trained on unprecedented amounts of data and are featured in systems for specific language tasks. Their introduction has pushed the state of the art of NLP forward, as measured by various benchmarks and tests for evaluating systems’ performance (see Chapter 2).
Figure 5.3. AI literacy performance in 2016 and 2021, counting Maybe as 50%-Yes
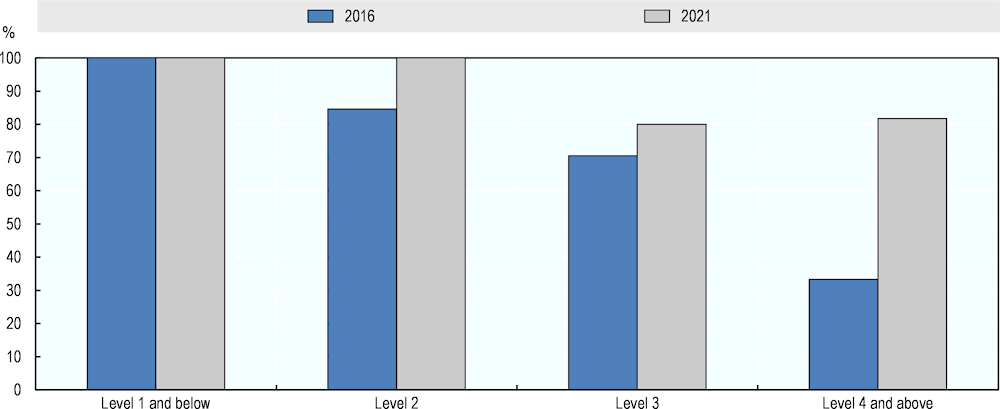
Source: Adapted from Elliott, S. (2017[1]), Computers and the Future of Skill Demand, https://doi.org/10.1787/9789264284395-en.
Change in AI literacy performance according to experts participating in both assessments
Comparisons of AI performance ratings over time may be biased by changes in the composition of the expert groups providing the ratings. If expert groups differ in the mix of optimists and pessimists, composition of expertise, or with regard to other characteristics relevant for the assessment, these differences would be reflected in the aggregate AI ratings and wrongly attributed to differences in AI capabilities between both time points. Information on such potential confounding factors is not available. However, an analysis of how the six experts who participated in both assessments changed their ratings over time would account for much of the potential bias related to the use of different expert groups across time points.
First, Figure 5.4 provides an overview of the average literacy ratings of all experts in the 2016 and 2021 assessments. Overall, the distributions of experts’ average ratings are similar across years, with ratings in 2021 being, on average, higher than in 2016. A two-sample independent t-test indicates a significant increase in experts’ average ratings (t(20) = 1.5; p = 0.08).
The blue bars in Figure 5.4 represent the average ratings of the experts who participate in only one assessment. The five experts who participated in the pilot, but did not continue in the follow-up, had middle to high average ratings in 2016 (Moshe Vardi, Mark Steedman, Rebecca Passonneau, Vijay Saraswat and Jill Burstein). Among the new experts in 2021, two had the lowest ratings (Michael Witbrock and José Hernández-Orallo), another two had the highest ratings (Yvette Graham and Antony Cohn) and one expert had a medium overall rating (Chandra Bhagavatula) in their expert group.
Bars in grey show the averages of the six experts who took part in both assessments – Ernest Davis, Ken Forbus, Art Graesser, Jerry Hobbs, Vasile Rus and Jim Spohrer. Their mean ratings represent different opinions on AI in both the 2016 and the 2021 assessments.
Figure 5.4. Average expert ratings in literacy in 2016 and 2021
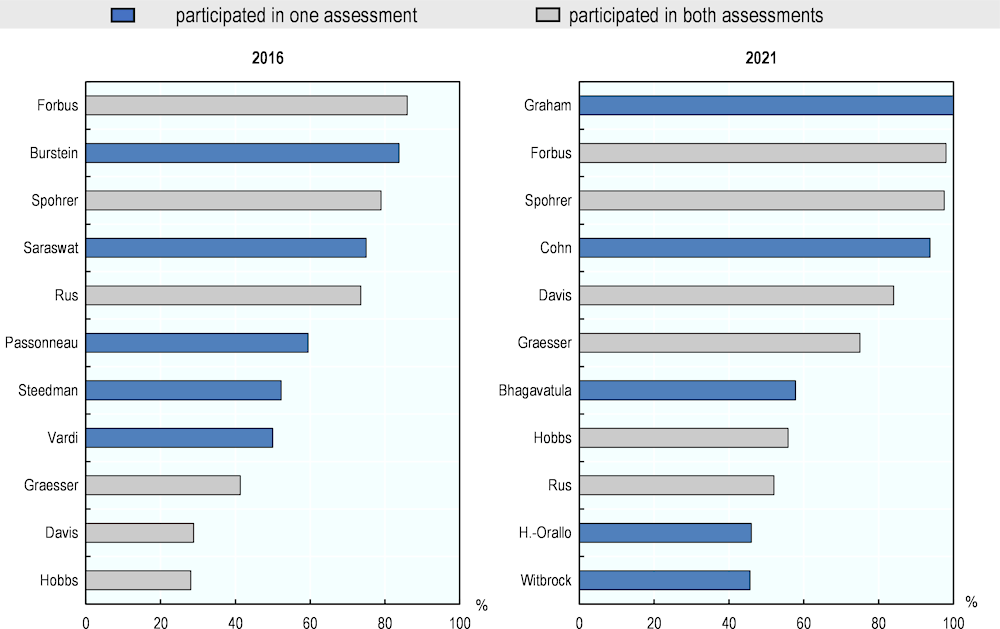
Source: Adapted from Elliott, S. (2017[1]), Computers and the Future of Skill Demand, https://doi.org/10.1787/9789264284395-en.
All but one of these experts – Vasile Rus – rated potential AI performance on the literacy test higher in 2021 than in 2016. A paired t-test shows that the “within-person” increase in ratings is significant (t(5) = 2; p = 0.05).
During the discussion, Rus made clear that his decrease in ratings is due to how he interprets the rating exercise rather than to how he evaluates the state of the art in NLP. Concretely, he assumed a more general system for literacy in 2021 that should perform literacy tasks as flexibly as humans. These higher expectations towards the system to be rated explain his lower ratings in 2021.
Figure 5.5 shows the aggregate AI literacy ratings based only on the ratings of the six experts who participated in both assessments. The results are in accordance with those obtained from the full expert groups, showing considerable increase in AI literacy performance in PIAAC over time. Compared to the full groups, the six experts provide somewhat more positive evaluations for 2021 and somewhat more negative ones for 2016 (see Figure 5.2). For 2016, the AI literacy performance estimated from their ratings is at 63% at Level 2, 33% at Level 3 and 22% at Level 4 and above. For 2021, these AI ratings are 93%, 76% and 78%, respectively. Overall, AI performance in the entire literacy test increased by 37 percentage points following these six experts’ judgements – from an estimated success rate at 48% in 2016 to a performance at 85% in 2021.
Figure 5.5. AI literacy performance in 2016 and 2021 according to experts who participated in both assessments
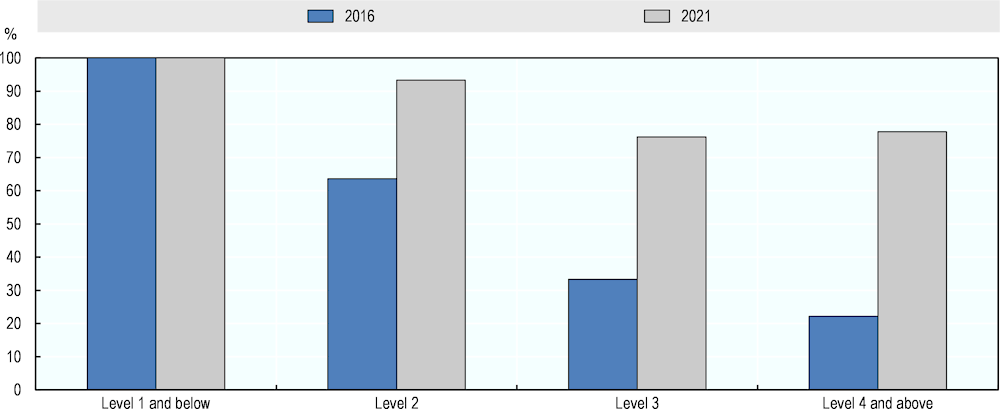
Source: Adapted from Elliott, S. (2017[1]), Computers and the Future of Skill Demand, https://doi.org/10.1787/9789264284395-en.
Comparison of experts’ agreement and confidence across literacy assessments
A valid comparison of AI capabilities across time requires sufficient agreement and certainty among experts regarding the state of the art in AI in each time point. This section looks at the level of agreement and the prevalence of uncertain answers in both literacy assessments to compare the quality of group ratings obtained in 2016 and 2021.
Figure 5.6 shows the average size of the majorities reached on the literacy questions of PIAAC in the pilot and follow-up assessments. For example, if an assessment includes ten experts rating two questions, A and B, and if A received six Yes and four No and B got two Yes and eight No, the average majority size would be 70%. This is the mean of the 60% majority reached on A and the 80% majority reached on B. This average is indicative for the overall level of agreement among the experts.
In 2021, the average size of the majorities reached across all literacy questions was 78%, close to the average majority size of 75% achieved in 2016. In both assessments, average agreement was highest at the easiest questions and decreased gradually with question difficulty. On average, across questions, majorities in 2021 are bigger than majorities in 2016 at Level 2 and Level 3 and smaller at Level 4 and above.
Figure 5.6. Average majority size in rating literacy questions in 2016 and 2021
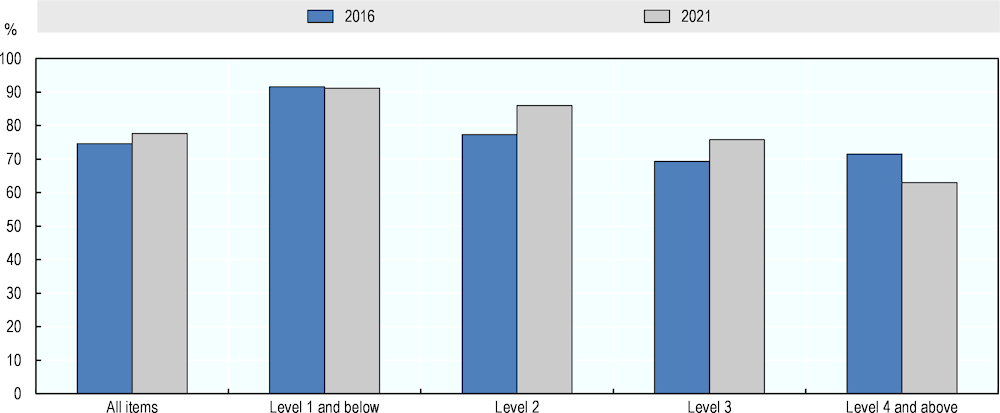
Source: Adapted from Elliott, S. (2017[1]), Computers and the Future of Skill Demand, https://doi.org/10.1787/9789264284395-en.
Figure 5.7. Share of literacy questions that receive three or more uncertain ratings in 2016 and 2021
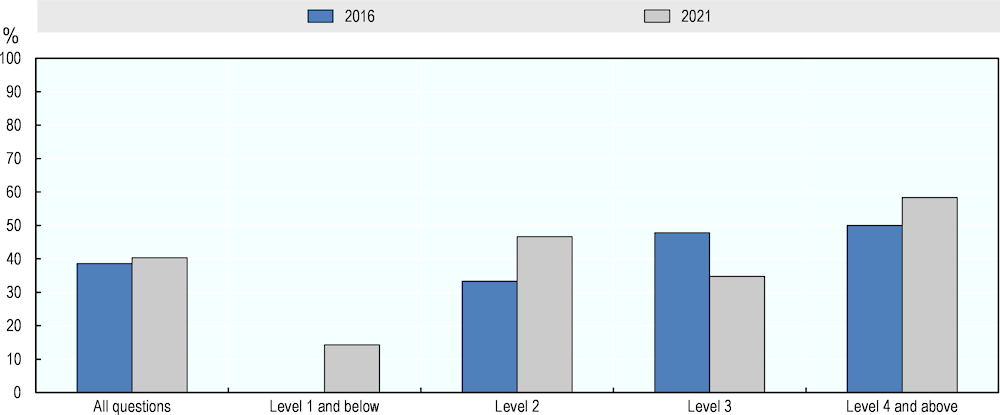
Source: Adapted from Elliott, S. (2017[1]), Computers and the Future of Skill Demand, https://doi.org/10.1787/9789264284395-en.
Although there is high agreement among those with certain ratings, some experts provide uncertain answers to many literacy questions. Figure 5.7 shows the shares of literacy questions at different difficulty levels that receive three or more Maybe- or Don’t know-ratings. In both 2016 and 2021, the shares of questions with uncertainty increase with question difficulty. Uncertainty is similar across assessments, with approximately 40% of all literacy questions in 2016 and 2021 receiving three or more uncertain ratings.
Projections of AI literacy performance for 2026
A repeated assessment of AI capabilities provides a sense for the direction and pace of the development of this technology. Another way to obtain information on the progress of AI is to ask experts to predict its capabilities in future. The pilot study asked three experts – Ernest Davis, Ken Forbus and Art Graesser – to rate the potential performance of AI on the literacy questions for 2026. In the follow-up assessment, all 11 experts provided predictions for the same year. The results are shown in Figure 5.8.
According to the majority of experts, computers will perform much better in literacy by 2026. The more recent projections are more optimistic than those made in 2016. Experts in the follow-up assessment expected AI to be able to perform all literacy questions in 2026. The predictions of the three experts in the pilot study suggest an AI performance of 91% at Level 3 and 80% at Level 4 and above for the same year.
Projections over a shorter time horizon are more likely to be precise given the rapid rate of change in AI technology. Moreover, experts pointed out they often provide predictions over three to five years when applying for research grants. Thus, they are more used to thinking of AI progress in terms of shorter time frames.
Figure 5.8. Projected AI literacy performance for 2026, by question difficulty
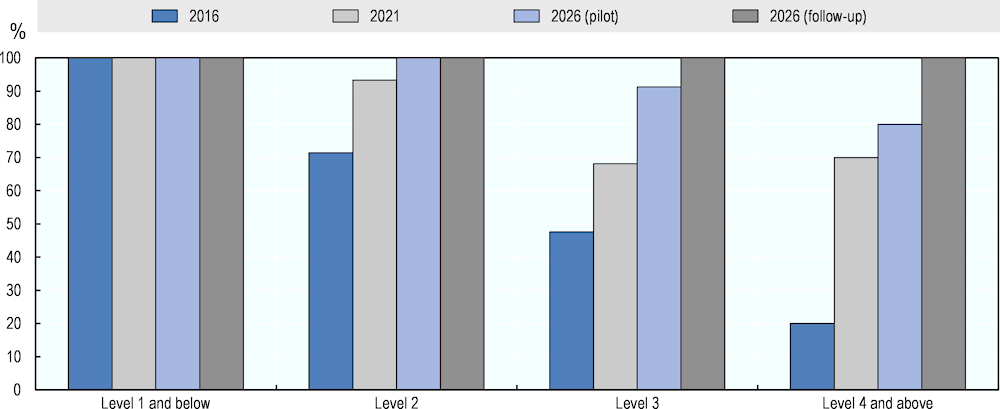
Source: Adapted from Elliott, S. (2017[1]), Computers and the Future of Skill Demand, https://doi.org/10.1787/9789264284395-en.
Change in AI numeracy capabilities over time
The follow-up study assessed AI in numeracy somewhat differently than the pilot study. The pilot study asked 11 experts to rate AI on each of the numeracy questions in PIAAC as part of a two-day assessment workshop (Elliott, 2017[1]). Conversely, the follow-up study collected the judgements of 15 experts in two assessment rounds. In the first round, 11 experts, 6 of whom took part in the pilot study, rated current AI performance, as well as expected performance for 2026, with regard to each numeracy question. They received instructions similar to those used in the pilot study. In the second round, four experts in mathematical reasoning of AI received more information on the PIAAC test and were asked to conceptualise a single system for addressing all numeracy questions. They subsequently provided ratings of current techniques on each numeracy question. In addition, they provided a single rating on whether AI could carry out the entire numeracy test in five years’ time.
This section compares ratings of current AI capabilities obtained from the 11 experts in 2016 with the aggregate ratings of the 15 experts who participated in the follow-up study. It then presents projection ratings for 2026 by viewing projections in the pilot study, the first and the second round of the follow-up study separately. As in the literacy analyses, experts’ answers from the follow-up study are grouped into the three categories of No (0% and 25%), Maybe (50%) and Yes (75% and 100%) to provide comparability to the pilot assessment (see Figure 5.1).
Change in AI numeracy performance between 2016 and 2021
Figure 5.9 compares AI numeracy ratings from the pilot and follow-up assessments, using measures based on Yes- and No-ratings only.2 The figure shows a decline in assessed AI performance in numeracy at all levels of question difficulty. The decline is smaller at Level 1 and below and Level 2 of question difficulty. It amounts to 19 and 35 percentage points at Level 3 and Level 4 and above, respectively. AI performance on the entire numeracy test has decreased by 14 percentage points between the assessments, according to experts’ majority opinion.
Figure 5.9. AI numeracy performance in 2016 and 2021, by question difficulty
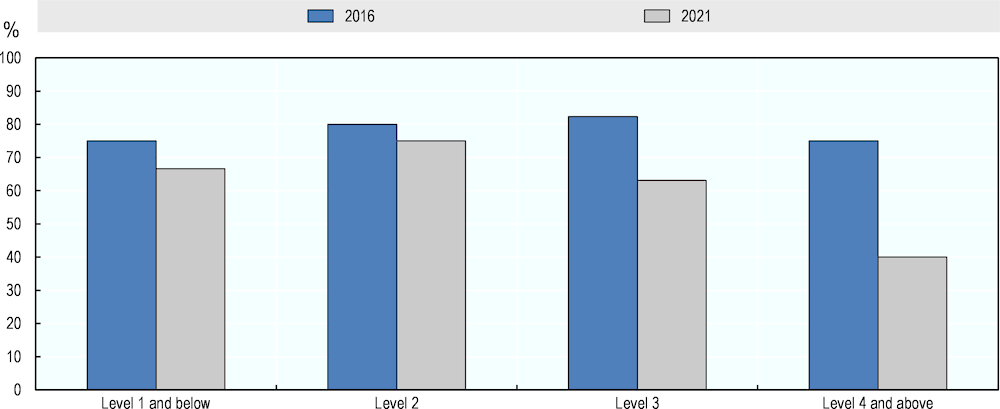
Source: Adapted from Elliott, S. (2017[1]), Computers and the Future of Skill Demand, https://doi.org/10.1787/9789264284395-en.
Figure 5.10 provides analogous results based on measures that include Maybe-answers as partial Yes-answers. When adding the Maybe-answers to the Yes-votes, the share of numeracy questions that receive a majority of positive answers increases at all difficulty levels. However, the gap between AI numeracy performance assessed in 2016 and 2021 remains: performance in 2021 is rated lower than in 2016, particularly at the higher levels of questions difficulty.
Differences in how experts interpret the ratings exercise may drive these counterintuitive findings. As described in Chapter 4, the follow-up assessment instructed experts to rate the capacity of one hypothetical system to solve the PIAAC numeracy test. The discussion showed this led some experts to assume a general AI system for numeracy that should solve diverse mathematical problems similarly to humans. These experts provided more negative ratings, given that current technology is not yet at this stage of generality.
Figure 5.10. AI numeracy performance in 2016 and 2021, counting Maybe as 50%-Yes
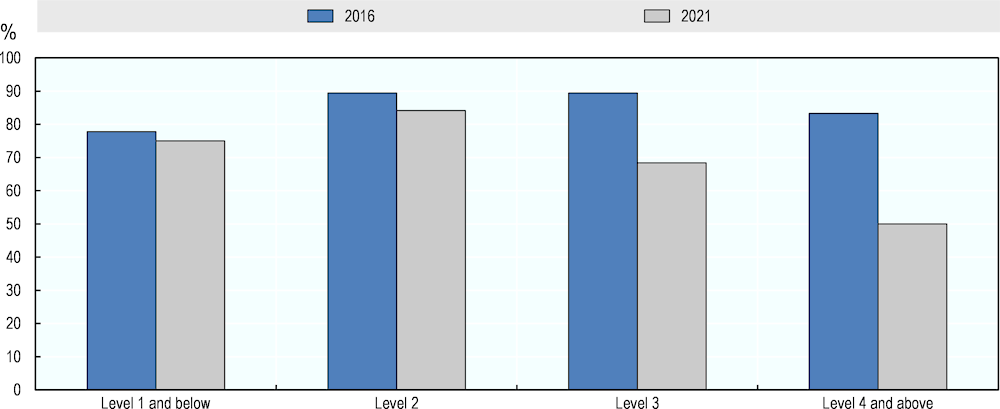
Source: Adapted from Elliott, S. (2017[1]), Computers and the Future of Skill Demand, https://doi.org/10.1787/9789264284395-en.
Change in AI numeracy performance according to experts participating in both assessments
Another explanation for the implausible decline in numeracy may relate to differences in the composition of experts in both assessments. For example, more pessimistic experts or experts with somehow different expertise may have joined the follow-up study. To account for such potential bias, the following analyses draw on ratings only from those six experts who participated in both assessments. The opinions of these experts may not fully represent the relevant expertise in the field. However, a within-person comparison should provide a better sense of the direction of change in AI since it eliminates potential confounding factors related to the use of different expert groups in both assessments.
Figure 5.11 presents the individual average ratings of all experts who completed the pilot and the follow - up assessments. It shows that experts’ average ratings in 2021 are more variable than average ratings in 2016. Ratings in 2021 are also, on average, lower than the ratings in 2016. However, a two- sample independent t-test shows this difference is not significant (t(24) = 0.89, p = 0.19).
A look at the six experts who participated in both assessments (bars in grey) shows that four of them rated AI performance in numeracy higher in 2021 than in 2016. These experts were Ken Forbus (51% in 2016 and 98% in 2021), Jim Spohrer (91% and 98%), Art Graesser (21% and 59%) and Ernest Davis (19% and 48%). One expert – Jerry Hobbs – had a high average rating of AI numeracy capabilities in both years (98% in 2016 and 95% in 2021). Another expert – Vasile Rus – rated AI low on almost all numeracy questions in the 2021 assessment (2%), which marked a sharp decline to his evaluation in 2016 (70%). He explained the decline by the higher degree of generality assumed for the hypothetical system to be rated in 2021 (see also above). A paired t-test shows that this “within-person” difference in ratings is not significant (t(5) = 0.49; p = 0.65).
Figure 5.11. Average expert ratings in numeracy in 2016 and 2021
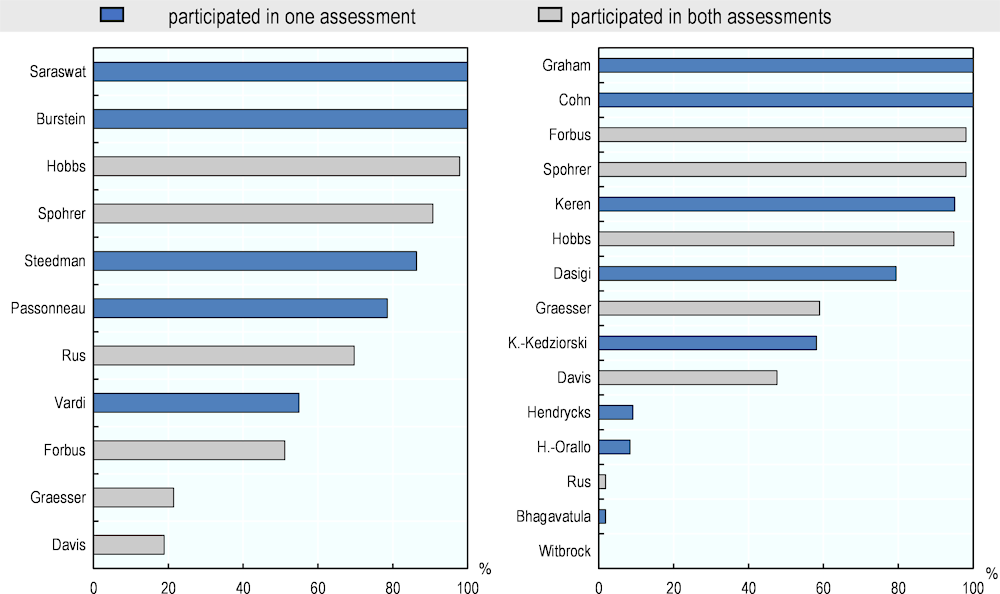
Source: Adapted from Elliott, S. (2017[1]), Computers and the Future of Skill Demand, https://doi.org/10.1787/9789264284395-en.
Figure 5.12 presents aggregate AI numeracy measures for 2016 and 2021 based on the ratings of the six experts who completed both assessments. In contrast to the ratings of the full expert groups, those of the six experts suggest an increase in potential AI performance on the numeracy test between 2016 and 2021. According to the majority opinion of the six experts, AI was expected to complete around 70% of questions at Level 2 and below, 54% of Level 3 questions and 20% of questions at Level 4 and above. For 2021, the corresponding performance ratings were assessed at 86%, 93% and 60%, respectively.
These results show that changes in AI numeracy capabilities over time are hard to define since findings are not robust to different specifications of the expert groups judging these capabilities.
Figure 5.12. AI numeracy performance in 2016 and 2021 according to experts who participated in both assessments
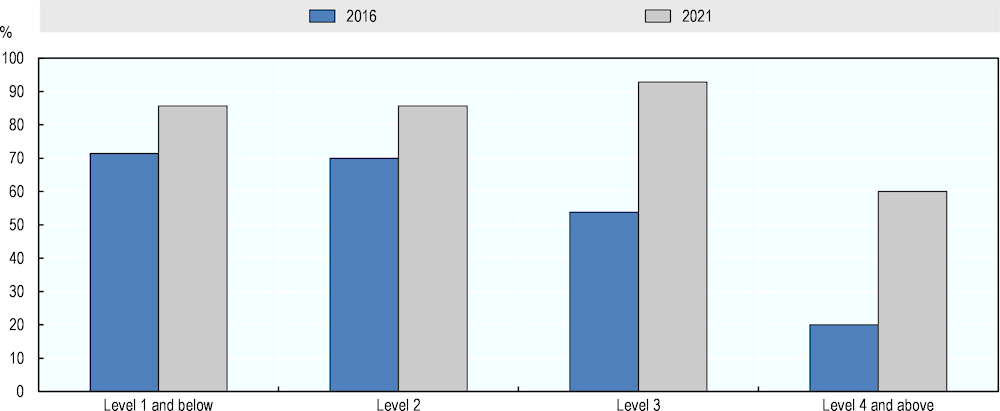
Source: Adapted from Elliott, S. (2017[1]), Computers and the Future of Skill Demand, https://doi.org/10.1787/9789264284395-en.
Comparison of experts’ agreement and confidence across numeracy assessments
As shown in Chapter 4, experts in the 2021 assessment disagreed about AI performance in numeracy. Two opposing groups emerged of five experts who evaluated AI negatively on almost all numeracy questions and four who provided mainly positive ratings (see Figure 5.11). This hindered consensus building in the quantitative evaluation of AI. In the following, consensus and experts’ confidence in numeracy ratings in the two assessments are compared. This can show whether disagreement is specific to the follow-up assessment or characteristic of the assessment of numeracy capabilities altogether.
Figure 5.13 shows the average size of the majorities reached on the numeracy questions of PIAAC in both assessments. In the follow-up, the majority opinion regarding AI numeracy capabilities included, on average across all questions, 62% of the experts who provided a positive or negative evaluation. This majority share is similar at different levels of question difficulty. By contrast, experts’ agreement in numeracy was higher in the pilot study. Across all questions on average, 74% of the experts with ratings different than “Maybe” or “Don’t know” formed the majority. This share is highest at Level 1 and below, at 84%, and decreases gradually to 64% at Level 4 and above.
Figure 5.13. Average majority size in rating numeracy questions in 2016 and 2021
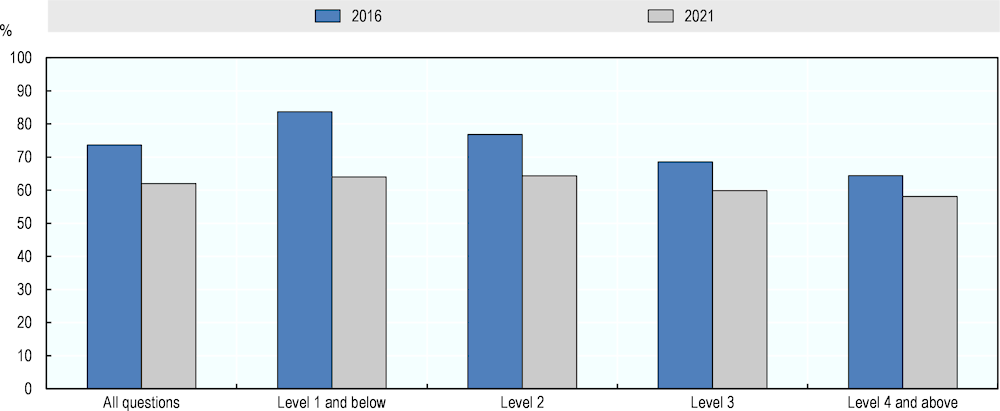
Source: Adapted from Elliott, S. (2017[1]), Computers and the Future of Skill Demand, https://doi.org/10.1787/9789264284395-en.
Figure 5.14. Share of numeracy questions that receive three or more uncertain ratings in 2016 and 2021
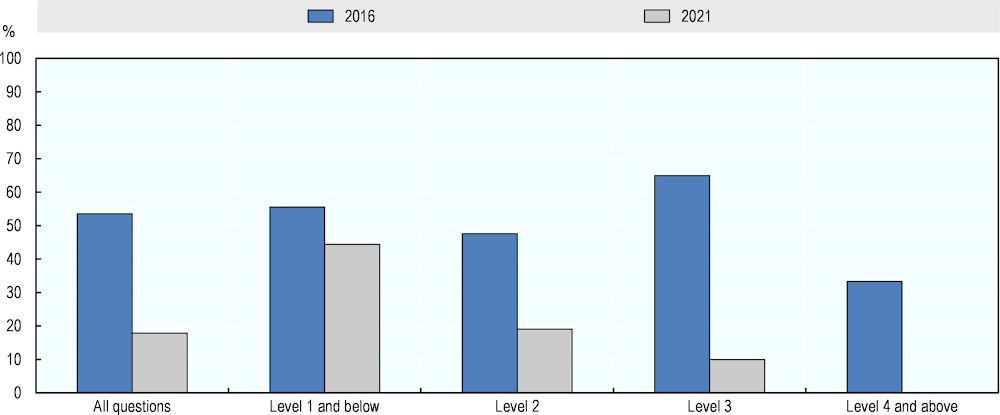
Source: Adapted from Elliott, S. (2017[1]), Computers and the Future of Skill Demand, https://doi.org/10.1787/9789264284395-en.
In addition, Figure 5.14 provides information on the prevalence of uncertain ratings in the pilot and follow-up assessments. It shows, that, in 2016, around half of the numeracy questions received three or more uncertain answers. In the follow-up assessment, uncertainty was lower, with only 18% of all questions having at least three Maybe- or Don’t know-answers. Uncertainty varied with question difficulty. In 2016, the share of questions with uncertainty was bigger at the first three levels of question difficulty and smaller at Level 4 and above. In the follow-up assessment, uncertainty was highest at the easiest questions and lowest at the hardest questions.
Overall, the numeracy assessment in 2016 is characterised by higher agreement – similar to the one achieved in the literacy domain in the same year – and low certainty in ratings. By contrast, experts in the follow-up assessment had more opposing views on AI numeracy capabilities but expressed more certainty in their evaluations. As described in Chapter 4, disagreement in the follow-up study had different reasons. Among others, these included varying interpretations of the rating instructions and differing assumptions about the systems to be rated. This may have driven the decline in experts’ agreement compared to 2016.
An alternative explanation may have to do with technological developments related to numeracy capabilities. In 2016, large language models have not yet been widely applied for mathematical reasoning. The limited information on AI’s capabilities related to quantitative reasoning may have caused uncertainty in the pilot assessment, but also consensus, as it is easier to agree on issues for which information is concise. Since 2016, the research field has expanded. The availability of more information relevant for the numeracy assessment may have decreased uncertainty in 2021. However, it may have increased disagreement as it is harder to agree on issues for which there is much and novel information.
Projections of AI numeracy performance for 2026
Figure 5.15 compares the numeracy ratings for 2016 and 2021 with experts’ projections for 2026. This can provide a sense of the likely development of AI numeracy capabilities.
Figure 5.15. Projected AI numeracy performance for 2026, by question difficulty
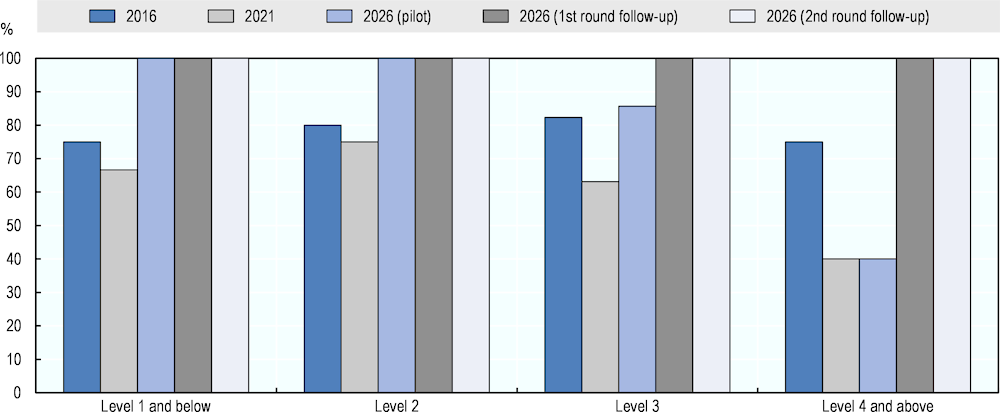
Source: Adapted from Elliott, S. (2017[1]), Computers and the Future of Skill Demand, https://doi.org/10.1787/9789264284395-en.
Three experts in 2016 rated potential AI performance on the numeracy test in 2026 – Ernest Davis, Ken Forbus and Art Graesser. They were most sceptical with regard to AI’s numeracy capabilities in 2016, as shown in Figure 5.11. Similarly, they were doubtful about AI’s performance in ten years’ time, providing majority ratings of 86% at Level 3 and 40% at Level 4 and above. The latter is below the full group’s rating of current AI numeracy performance for 2016.
All 11 experts who first rated AI in numeracy in the follow-up study provided projections for each of the test questions. Their majority rating suggests 100% successful AI performance in numeracy in 2026. The four experts in mathematical reasoning who completed the second assessment round provided only one rating for future AI performance. These ratings also indicate a 100% success rate of AI in numeracy in 2026.
References
[4] Devlin, J. et al. (2018), “BERT: Pre-training of Deep Bidirectional Transformers for Language Understanding”.
[1] Elliott, S. (2017), Computers and the Future of Skill Demand, Educational Research and Innovation, OECD Publishing, Paris, https://doi.org/10.1787/9789264284395-en.
[2] Peters, M. et al. (2018), “Deep contextualized word representations”.
[3] Radford, A. et al. (2018), Improving Language Understanding by Generative Pre-Training, https://s3-us-west-2.amazonaws.com/openai-assets/research-covers/language-unsupervised/language_understanding_paper.pdf (accessed on 1 February 2023).
Annex 5.A. Supplementary figures
Annex Table 5.A.1. List of online figures for Chapter 5
Table Number |
Table Title |
---|---|
Figure A5.1 |
Comparison of average and majority expert opinions on AI capabilities in literacy in 2016 and 2021 |
Figure A5.2 |
Comparison of average and majority expert opinions on AI capabilities in literacy in 2016 and 2021, counting Maybe as 50% |
Figure A5.3 |
Comparison of average and majority expert opinions on AI capabilities in numeracy in 2016 and 2021 |
Figure A5.4 |
Comparison of average and majority expert opinions on AI capabilities in numeracy in 2016 and 2021, counting Maybe as 50% |
Notes
← 1. The results for 2016 reported in this study differ from those in Elliott (2017[1]) because the studies use different approaches to aggregate experts’ ratings. The pilot study by Elliott (2017[1]) computes the aggregate literacy and numeracy measures by taking the mean of experts’ ratings on each question and then averaging these mean ratings across questions. This measure has the advantage of reflecting all experts’ opinions about AI capabilities. By contrast, the follow-up study classifies each question as solvable or not solvable by AI according to the majority of experts’ ratings and estimates the share of questions marked as solvable. This aggregation approach disregards minority opinions. However, its measures are more easily interpretable, and rely only on questions with majority agreement.
Figures A5.1 and A5.2 in Annex 5.A offer additional analyses that compare the results from both assessments following the aggregation rule used in 2016. They show that using the average of experts’ ratings as a measure of AI performance on PIAAC produces results similar to those following the majority rule. At each level of question difficulty, AI performance in literacy assessed with the average expert rating is higher in 2021 than in 2016. At Level 4 and above, the increase in literacy performance indicated by the “average” approach is smaller than the increase produced with the “majority” approach. This may have to do with the small number of questions and the bigger disagreement at this level, producing arbitrariness in results.
← 2. The results for numeracy for 2016 differ from those reported by Elliott (2017[1]) because they rely on the majority rating and not the average rating of experts (see note 1 above). Figures A5.3 and A5.4 in Annex 5.A present results for 2016 and 2021 obtained by averaging ratings across experts and questions, as done in Elliott (2017[1]). Similar to the results relying on the majority of experts’ ratings, the results relying on averages show lower AI numeracy performance in 2021 than in 2016. However, the decline in AI performance indicated by the “average” approach is smaller than the one indicated by the “majority” approach at Level 3 and higher. Questions at these difficulty levels received similar shares of Yes- and No-votes in both assessments. This leads to arbitrary results when using the majority vote as AI’s success on these questions is usually decided by a difference of only one vote. By contrast, the “average” approach produces measures close to 50% at these levels since it averages similar shares of 0% and 100% ratings, ignoring that these evaluations reflect disagreement rather than medium AI performance.