This chapter explores the different levels of confidence and associated uncertainties influencing understanding of risks of losses and damages from climate change. Specifically, it looks at different levels of confidence in projections of the physical climate and in expectations of future vulnerability and exposure. These include and/or relate to uncertainties on emissions trajectories; on how the climate system responds to those emissions; climate variability and uncertainty about socio-economic development patterns. The chapter includes worked analytical examples that unpack and illustrate the different types of uncertainties. It ends by examining how these uncertainties need to be considered together for an effective communication of climate risk.
Managing Climate Risks, Facing up to Losses and Damages
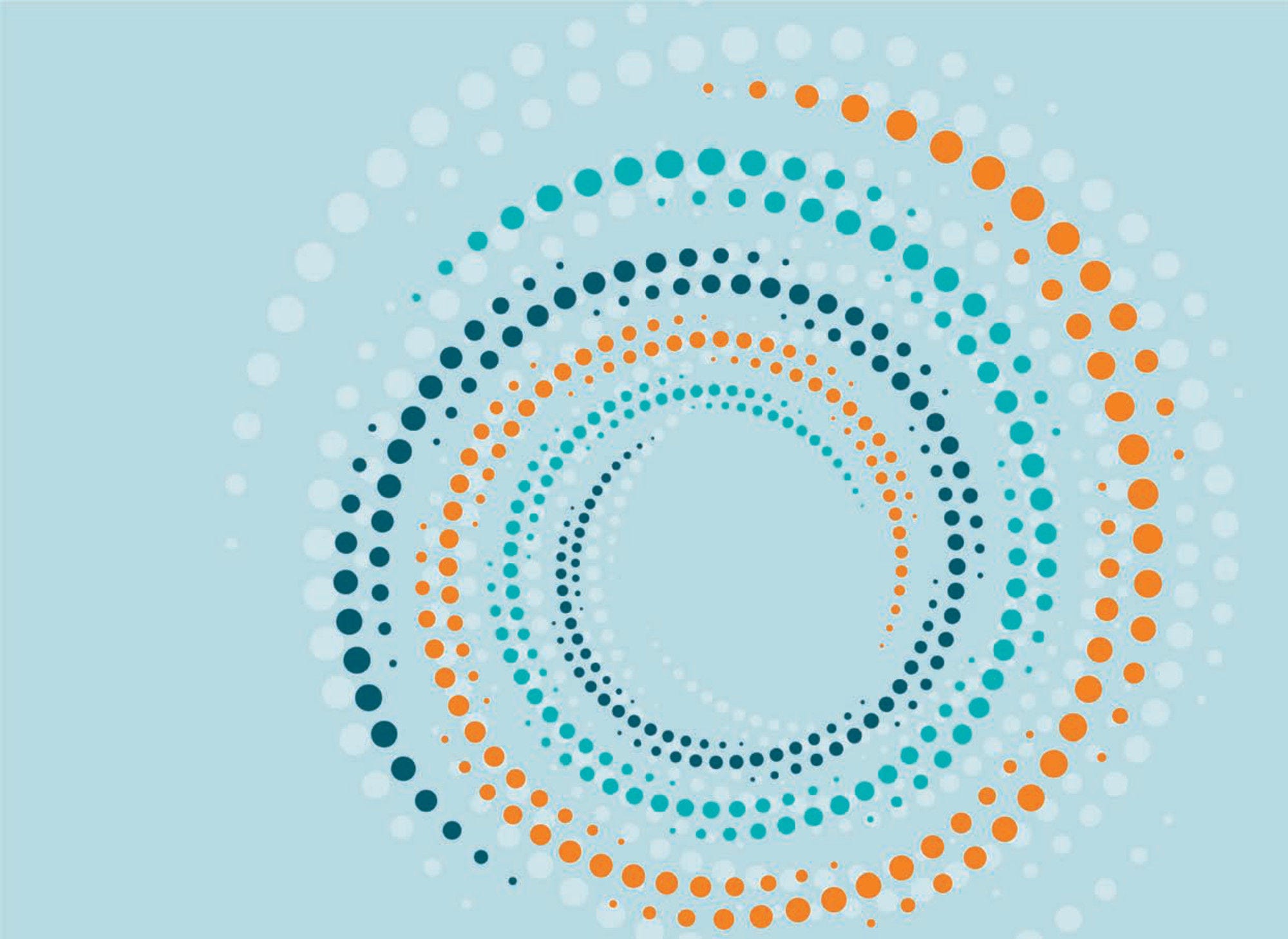
2. Types of uncertainties and understanding of risks of losses and damages
Abstract
In Brief
Human influence on the warming of the climate system is unequivocal. There is very high confidence on the thermodynamic aspects of climate change and the severity of the associated impacts. There are deep uncertainties in relation to important aspects of the physical climate response to climate forcing, and to vulnerability and exposure of socio-economic systems. However, these uncertainties are not a reason for delaying action.
Climate change is a result of complex physical processes. Deep scientific understanding about these processes is reserved to a limited group of highly specialised experts and scholars. While policy makers in the area of climate change do not require such understanding, they do often need to link science and policy. Therefore, they must deal with a number of different types and forms of uncertainties. A policy maker well-informed about the nuances of climate risk is one well-placed to identify effective and robust courses of action to reduce and manage that risk. These different types of uncertainties include:
Uncertainties in relation to quality and quantity of observational data: Earth is more extensively and systematically observed today than at any other time. Yet, there is still a long way to go before establishing a comprehensive, systematic global climate monitoring system. Any progress in monitoring is a step forward with potential short-term benefits.
Uncertainties in equilibrium climate sensitivity (ECS) and transient climate response (TCR): A first-order question about a climate plan or strategy is how effective it will be in mitigating climate change. The ranges of ECS and TCR estimates represent the uncertainties around the scale of the response of the climate to greenhouse gas emissions. The urgent need for stringent emissions reductions becomes even more evident in light of these uncertainties.
Uncertainties in projections of global and regional climate change: Despite high confidence in the thermodynamic aspects of climate change and the severity of the associated impacts, there is far less confidence in the detail of when, where and how these hazards will occur. However, short-term projected regional changes are still useful. They provide a range of potential changes to which human and natural systems can be subject to, even if the exact date and extent of those changes are not accurately predicted. Therefore, from a policy-making perspective, climate modelling informs on broad patterns and the potential for how climate change could unfold locally. This information can feed directly into the shaping of policies dealing with climate-related risks, including physical, behavioural and cultural adaptation to expected changes.
Uncertainties related to socio-economic data: Socio-economic data should underlie every policy decision to help infer the possible consequences of the policy. Often, however, crucial data are unavailable. In these cases, policy makers may rely instead on proxy variables and regression estimations among other approaches, introducing further uncertainties. Broader and deeper understanding of the issues can be achieved by jointly conducting quantitative and qualitative analyses.
Uncertainties related to socio-economic projections: Another important source of uncertainty is that related to the socio-economic outcomes. Integrated assessment models are the most influential approach in the climate policy arena to analysing alternative futures. These models integrate relevant components of the climate system and the economy in a single model. While they are useful to compare the future effects of different policies, they are constrained because they rarely model physical and socioeconomic uncertainty, especially the way the uncertainties interact. Therefore, other models need to complement integrated assessment models. These alternatives include agent-based models or resample and reweighting models. Projections are also sensitive to the role of nature in socio-economic systems, such as the intrinsic value of nature and its role in economic production and human well-being. These elements, therefore, should be carefully considered.
Other socio-economic uncertainties: There are other socio-economic uncertainties, such as those relating to socio-economic tipping points and social discount rates. Socio-economic tipping points are abrupt changes in society out of proportion to their origin (non-linear). Such tipping points could enhance or threaten the effectiveness of climate policy; by nature, they are extremely difficult to predict. Policies should aim to encourage the crossing of tipping points that help enhance the resilience of societies. The social discount rates need to reflect local values and cultures. They essentially describe the weight of the future in relation to the present: uncertain settings or high damages imply low social discount rates.
Uncertainties underlying the risks of losses and damages have decreased in the past decades. However, they remain an important factor in the perception of risk and in how climate change action responds to that perception. Given the compelling accumulation of scientific evidence about climate change and its potential consequences, lack of confidence in for example how, when and where a hazard will occur cannot be a reason for inaction. Indeed, this chapter shows that larger uncertainties can lead to a larger range of potential climate risk. The uncertainties and ambiguities surrounding some aspects of climate change projections should therefore amplify – not weaken – the case for strong climate action.
2.1. Introduction
Chapter 1 summarises understanding of climate change, highlighting key aspects of how the physical climate responds to anthropogenic greenhouse gas (GHG) emissions and how vulnerability and exposure can contribute to higher climate risk and socio-economic impacts. The Sixth Assessment Report (AR6), Working Group 1 (WG1) of the Intergovernmental Panel on Climate Change (IPCC) concluded that, “it is unequivocal that human influence has warmed the atmosphere, ocean and land”. Additionally it assessed that “the scale of recent changes across the climate system as a whole and the present state of many aspects of the climate system are unprecedented over many centuries to many thousands of years” (IPCC, 2021[1]).
Given these assessments, there is extremely high confidence about the thermodynamic aspects of climate change relating to the balance of energy flows into and out of the Earth system. Higher emissions, for example, lead to higher concentrations of greenhouse gases in the atmosphere and therefore a higher climate forcing. This, in turn, leads to higher mean surface temperature (IPCC, 2013[2]; Hausfather et al., 2020[3]). These thermodynamic changes also drive far-reaching changes in the dynamics of the ocean and atmosphere. Such changes notably affect the water cycle and precipitation patterns. These changes, in turn, lead to increased frequency and magnitude of many extreme weather events, such as heavy precipitation and floods, as well as droughts and heat waves.
Increased climate forcing of the Earth system due to enhanced GHG concentrations also drives irreversible long-term changes in sea level (mainly due to both thermal expansion and the ice loss from glaciers and ice sheets) (IPCC, 2021[1]). If it continues unchecked, climate change will also eventually activate tipping points, which would completely change the behaviour of the climate system. Chapter 3 explores one such potential change: the shutting off of the Atlantic Meridional Overturning Circulation (AMOC), a major ocean circulation that transfers heat from the tropics to high northern latitudes which is very likely to weaken in the 21st century (IPCC, 2021[1]).
There is high confidence these physical changes in the climate will cause different types of socio-economic impacts and associated losses and damages, including direct impacts on peoples’ livelihoods. These could occur, for example, through changes in rainfall patterns, temperatures and the distribution of biodiversity and ecosystem services. More indirect impacts on livelihoods are also possible through, for example, changes in the demand for goods and services (Granderson, 2014[4]).
There is also evidence that disadvantageous socio-economic conditions worsen vulnerability and exposure within countries through various channels. These could lead to more severe losses and damages (Hallegatte, Bangalore and Vogt-Schilb, 2016[5]). Impacts from climate change can also translate into loss of personal safety, place-based practices, identities and cultural heritage, and lead to displacement (Barnett et al., 2016[6]) (Adger et al., 2012[7]). These losses and damages are less easily quantifiable but may be equally or more important, as non-economic losses and damages occur at varying degrees and are perceived very differently by different societies.
There is high confidence on the thermodynamic aspects of climate change and the severity of the associated impacts. However, there is far less confidence in the detail of when, where and how these hazards will occur. This is due to the enormous challenges of modelling the highly complex and non-linear climate system. Compounding this challenge (and perhaps in some circumstances dominating this), there is also uncertainty regarding the future socio-economic conditions under which the hazards will manifest, which are key to understanding the levels of vulnerability and exposure to future climate change, and hence the risks of losses and damages on different timescales (Riahi et al., 2017[8]).
According to the IPCC, “uncertainty denotes a cognitive state of incomplete knowledge that results from a lack of information and/or from disagreement about what is known or even knowable” (Kunreuther et al., 2014[9]). Some aspects of uncertainty about a given future climate or socio-economic variable may be quantifiable and have a well-defined probability distribution. Other aspects of uncertainty may not be quantifiable. Both kinds of uncertainty are discussed in this chapter. Given the compelling evidence about the scale of the climate challenge, these uncertainties are no reason to delay action. On the contrary, climate-related damages are likely to increase in a non-linear manner away from present conditions with the magnitude of climate change. Therefore, uncertainty about the future climate on these regional to local scales is actually a reason for greater, not lesser concern.
Model projections of future precipitation illustrate this uncertainty. In some spatial regions, climate models have a high degree of agreement on the direction of change (Zappa, Bevacqua and Shepherd, 2021[10]). In some regions, however, models either disagree on the direction of change or project only a small change. The nature of the uncertainties has different policy implications for adaptation. In one region, the direction of change could be uncertain (e.g. a range of climate model projections on either side of a mean outcome of zero). Such a region faces different choices than one with a high level of confidence in the direction of change and where that change is relatively small and close to zero. In other words, the absence of confidence in how a change will happen is not the same as confidence in the absence of a change.
Indeed, in dealing with uncertainty in climate risk, one must strike a balance in guarding against two types of potential errors. The first error is the possibility of false positives (i.e. avoiding the possibility of overestimating the risk that an impact will happen). The second error is the possibility of false negatives [i.e. avoiding the possibility of underestimating that same risk (Shepherd, 2019[11])]. Finding this balance is not trivial. It requires a good understanding of different levels and types of uncertainties and how to best use this uncertainty to inform decision making.
The following sections explore uncertainties in relation to the physical climate response to forcing and to vulnerability and exposure of socio-economic systems (Figure 2.1). They aim to characterise information available to policy makers in light of uncertain knowledge, and how policy makers can more accurately shape the communication of risk to help spur action. Section 2.2 looks at the confidence in projections of the physical climate. Section 2.3 explores confidence in expectations of future vulnerability and exposure. Finally, Section 2.4 discusses how these uncertainties need to be considered together for an effective communication of climate risk.
Figure 2.1. Types of uncertainties influencing understanding of risks of losses and damages

2.2. Confidence and uncertainties in projections of the physical climate
Climate change is a result of complex physical processes. Deep scientific understanding of these processes is largely limited to a small group of highly specialised experts and scholars. While such understanding is not required, policy makers in the area of climate change often need to link science and policy. Therefore, they must deal with a number of different types and forms of uncertainties. A policy maker well-informed about nuances in understanding climate risk is one well-placed to identify effective and robust action to reduce and manage that risk.
This subsection sets out understanding of risks of losses and damages to motivate ambitious climate action from policy makers. It explores uncertainties of different nature, including those pertaining to the climate system itself and those arising from unknown future social and political choices determining climate forcing. These include those arising from availability of observational data and related to climate sensitivity, as well as future emissions projections and uncertainties in regional and global climate change projections. Some uncertainties may be reduced by better scientific tools including observation and modelling; others may be reduced only by political commitment or by the passage of time.
2.2.1. Quality and quantity of observational data
Past and present weather and climate observations
Data and observations of past and present weather and climate are key to understanding variability and change in the climate system and provide a benchmark against which to monitor changes. High-quality data and observations are also key in supporting research, which informs understanding of present and future climate change.
Observations of past behaviour of a number of climate-related variables are used to develop and improve the climate models that provide projections of the future climate system (Flato, 2013[12]). Based on the premise that if models are not able to predict the past well, then they cannot be expected to predict the future, a direct approach to evaluate the effectiveness of a climate model is to compare model output with historical observations. Data from the deep past (from millions to hundreds of years ago) – so-called paleoclimate data – are also important in evaluating climate models outside of the range of more recent climate observations that were used to develop them (Braconnot et al., 2012[13]).
Such an approach reveals that climate models reproduce well general features and aspects of global mean surface temperature from the past. Conversely, they perform less well for the patterns of precipitation at large scale and not well for precipitation at regional scale (Flato, 2013[12]). In analysing the difference between model output and observations, a good knowledge and understanding is needed of uncertainties and potential errors.
Global climate monitoring is costly. It is therefore important to consider the need for climate monitoring in light of societal goals (Goody et al., 2002[14]). There are broadly three different timescales at which climate monitoring is needed. Each has implications for different societal goals (Goody et al., 2002[14]):
First, the monitoring of present climate serves a number of societal goals. For example, it can support business activities in the agricultural or industrial sectors leading to short-term successes and gains. It can also inform action to protect and avoid losses of lives, livelihoods and assets.
Second, climate monitoring can inform prediction of short-term (e.g. one-two years) future climate, such as predictions of the El Niño phenomenon. This type of phenomena is linked to a number of natural disasters potentially at global scale, which means their monitoring is well motivated and linked to societal goals.
Third, climate data and monitoring indirectly informs projections of future climate at longer temporal scales. (50-100 years and beyond) as it allows for calibration and evaluation of models. Model results inform judgements on the long-term implications of human interference with the climate system. They have had significant implications for the international climate process, amplified by their central role in IPCC assessments (e.g. informing the adoption of long-term global temperature goals of the Paris Agreement).
Earth is more extensively and systematically observed today than at any other time. The ability to observe the climate has greatly improved in recent years thanks to improved technology. These include advances in measurements of, for example, sea level and temperatures, and Earth information technology such as satellite remote sensing (Guo, Zhang and Zhu, 2015[15]).
Improved methodology for analysing climate observations from meteorological stations has also led to better and more accurate climate observations over the past century (Mitchell and Jones, 2005[16]). Multiple datasets for global mean temperature have been constructed using different methods and a variety of proxies, with high agreement. This confirms the overall picture of increasing global surface temperatures (Rahmstorf, Foster and Cahill, 2017[17]). The emergence and successes of big data analytics have also been increasingly applied to both weather and climate science (Hassani, Huang and Silva, 2019[18]).
Monitoring weather provides valuable information on key climate variables, such as temperature. However, monitoring climate requires tracking a larger number of variables with more accuracy and low bias (National Research Council, 2012[19]). Many nations around the world recognise the need to maintain and improve climate observations in order to better understand how climate change is unfolding today and in support of better climate change predictions. This is evidenced by acceptance of the World Meteorological Organization (WMO)’s Global Climate Observing System (GCOS) Implementation plan (GCOS, 2016[20]), submitted to the COP22 in Marrakesh.
The GCOS Implementation plan “sets out a way forward for scientific and technological innovations for the Earth observation programmes of space agencies and for the national implementation of climate observing systems and networks”. In particular, the plan identifies actions needed for climate monitoring. It considers the increasing requirements of scientific research, the United Nations Framework Convention on Climate Change (UNFCCC) and other multilateral agreements. In short, it identifies a number of Essential Climate Variables (ECVs), summarised in Table 2.1, and defines target requirements for measuring them.
Table 2.1. Essential Climate Variables as defined by GCOS
Measurement domain |
Essential Climate Variables (ECVs) |
---|---|
Atmospheric |
Surface - air temperature, wind speed and direction, water vapour, pressure, precipitation, surface radiation budget. Upper-air: temperature, wind speed and direction, water vapour, cloud properties, Earth radiation budget, lightning. Composition: carbon dioxide (CO2), methane (CH4), other long-lived GHGs, ozone, aerosol, precursors for aerosol and ozone. |
Oceanic |
Physics - temperature: sea surface and subsurface. Salinity: sea surface and subsurface; currents, surface currents, sea level, sea state, sea ice, ocean surface stress, ocean surface heat flux. Biogeochemistry: inorganic carbon, oxygen, nutrients, transient tracers, nitrous oxide (N2O), ocean colour. Biology/ecosystems: plankton, marine habitat properties. |
Terrestrial |
Hydrology - river discharge, groundwater, lakes, soil moisture. Cryosphere: snow, glaciers, ice sheets and ice shelves, permafrost. Biosphere: albedo, land cover, fraction of absorbed photosynthetically active radiation, leaf area index, above-ground biomass, soil carbon, fire, land surface temperature. Human use of natural resources: water use, GHG fluxes. |
Source: (GCOS, 2016[20]).
In addition to this effort, the WMO has provided updated information since 1993 in its Status of the Global Climate series (for the most recent, (Kennedy et al., 2021[21]). It provides these data through the Commission for Climatology that guides the World Climate Programme, and in co‑operation with its members. The publication provides key information on the so-called global climate indicators (Trewin et al., 2021[22]), which represent a subset of the ECVs. In so doing, it provides credible scientific information on climate and its variability.
This monitoring shows that levels of uncertainties, quality and extent of data vary highly for different indicators. For example, global mean surface temperature changes can be determined with low levels of uncertainties and for long periods into the past. In contrast, ocean acidification has limited underlying observational data with only a low number of stations monitoring this indicator worldwide and for a limited number of years. Meanwhile, sea ice extent observations depend on satellite data, which are available over the last 40 years.
Climate reanalysis, which combines models with observations, has also made an important contribution in the past decades. It has provided globally complete estimates of many key climate variables with high frequency and spatial resolutions. Different climate centres produce several reanalyses using different models (Kalnay et al., 1996[23]; Dee et al., 2011[24]; Kobayashi et al., 2015[25]; Randles et al., 2017[26]). Among estimates in these models are atmospheric parameters. These range from air temperature, pressure and wind to surface parameters such as rainfall, soil moisture and sea-surface temperature. Such datasets are valuable in that they produce consistent time series of gridded data that would be virtually impossible to measure and analyse directly.
Reanalyses are a cleaned, standardised and interpolated form of past data used as input for many studies of historical climatic change. The limitations and biases in observational data and models used as input for creating these datasets are inevitably passed on to the final reanalysed dataset. In other words, the reliability of the dataset will be as good as its inputs and should not be taken as historical truth.
Systems and networks for gathering climate information are increasing and becoming more efficient. However, there is still a long way to go before establishing a comprehensive, systematic global climate monitoring system. Any progress in monitoring is a step forward with potential short-term benefits.
Since many gaps remain in the system, priorities must be assigned. In particular, quality and availability of data are highly heterogeneous geographically and temporally as well as for different types of climate variables. This problem results in differing quality of weather forecasts in different regions, often among the most vulnerable, as well as limiting climate research. Unequal data coverage, stemming from a range of political and economic issues, leads inevitably to larger uncertainties. For example, estimating future climate change in areas that are less represented has implications for climate justice (Brönnimann and Wintzer, 2018[27]).
Improvements in climate monitoring can have a direct impact on decision making related to climate risk management and resilience measures, among other issues. This is because these decisions need to answer questions relating to placement of infrastructure or areas to prioritise for soil or water conservation measures – questions that climate observations can directly inform. Better data, over long periods, may also inform the testing and development of models that can provide better predictions in the mid and longer term.
Observational records of specific impactful events
High-quality observational data are needed to do statistics of different types of extreme events and for detecting and attributing climate change (Otto, 2016[28]). This then enables researchers to draw conclusions (with varying degrees of confidence) about whether a given hazard occurred or was made more intense by human-made climate change overlaid on current climate variability. Such climate attribution studies are most often initiated on the basis of an event causing significant negative impacts for local communities (Philip et al., 2020[29]). Yet the monitoring and reporting of impacts associated with different classes of extreme weather can often be sparse and inconsistent between poorer and wealthier countries (Visser, Petersen and Ligtvoet, 2014[30]; Noy, 2015[31]; Noy and duPont IV, 2018[32]; Tschumi and Zscheischler, 2019[33]). Valid concerns exist about the mixed quality of impact data between different classes of extreme weather within high income countries (Tschumi and Zscheischler, 2019[33]). However, many other regions of the world fail to record any impacts whatsoever for entire classes of extreme weather (Noy, 2015[31]), such as heatwaves (Harrington and Otto, 2020[34]).
Observational data for impactful events are also important for calibration and evaluation of models for the future prediction of such events. Global Climate Models (GCMs) are effective in accurately predicting global increases in mean surface temperature increase as a result of GHG emissions. For example, at the global scale, these models are not meant to and cannot predict singular extreme events, such as a heatwave (see Section 2.2.4) or a flooding at a particular location (at least yet).
Secondary models connect global change projections from GCMs with local, specific events. Downscaling models, for example, aim mainly to improve the numerical resolutions. Impact models may additionally model the effect on other physical or human variables. Impact models also take in the outputs (e.g. projected changes in temperature, precipitation or sea-level rise) from GCMs. They then explore possible impacts of these environmental changes on various sectors of the economy (e.g. agriculture, water resources, forestry). Like for GCMs, both impact and downscaling models need to be tested and calibrated against observations from the past to make better predictions (Xu, Han and Yang, 2018[35]). Good observational data about small-scale and local climatic extremes and climate impacts therefore provide key information to these models.
Table 2.2. Quantifying impacts for different categories of extreme weather
Event type |
Easy-to-measure impacts |
Difficult-to-measure impacts |
Rarely-measured impacts |
---|---|---|---|
Heatwave |
N/A |
Excess mortality from heat, heat-related hospitalisations, infrastructure damage.* |
Productivity and other indirect economic losses. |
Flooding |
Insured asset damage, direct mortality. |
Uninsured asset damage. Crop losses. |
Mental health impacts, disease outbreaks. |
Drought |
Crop losses. |
Food insecurity and malnutrition. |
Heat-related impacts during drought. Other indirect economic losses. |
Wildfire |
Insured asset damage, direct mortality. |
Uninsured asset damage. Adverse health outcomes from related air pollution. |
Productivity and other indirect economic losses. |
Reporting coverage |
All countries |
High income countries only |
Bespoke studies only |
Note: A schematic representation of how uncertainties in quantifying the impacts of extreme weather differs depending on the category of extreme weather considered (rows), alongside the frequency with which different categories of impacts are reported by different countries (colours). The four event classes have been ordered on the basis of how often they are the subject of an attribution analysis.
*Because floods are experienced more frequently and by a larger fraction of the global population than wildfires1, more information is available about which impacts are well measured or poorly quantified in different settings. This explains the different levels of “impact reporting coverage” between the two categories in the table.
Blue boxes show event impacts commonly reported by all countries.
Orange boxes show event impacts typically reported only by high income countries.
Red boxes show extreme event impacts not routinely reported, and that require bespoke analyses (often published in peer-reviewed literature) to yield an impact estimate.
The next sub-sections attempt to summarise the different dimensions of uncertainty that exist when quantifying the impacts of on different classes of extreme weather, as an example of physical hazards (Table 2.2). Gaps in the assessment of other types of hazards, such as slow-onset events and tipping points (see Chapter 3), also exist but are not covered in this section. In addition, this discussion is not intended to provide a comprehensive review of the many publications within the disaster impact literature. Rather, it seeks to understand the relative magnitude of reporting gaps in extreme weather impact databases.
Flooding
Of the types of extreme weather most commonly analysed from an attribution perspective (see Table 2.2), the impacts associated with extreme rainfall events and subsequent flooding are the most well-defined in available databases (Tschumi and Zscheischler, 2019[33]). One reason why flooding events – either due to “storms” or “tropical cyclones” – are so well-represented in databases like EM-DAT, Sigma Explorer or DesInventar relates to the characteristics of their impacts. Physical assets, like property, roads or other infrastructure, are most commonly damaged during floods (Hallegatte et al., 2013[36]). Such damages share the beneficial qualities of having:
well-defined economic value (and insurance coverage, in the case of wealthier countries)
definitive spatial boundaries within which most impacts took place (i.e. locations that become inundated)
little-to-no time lag in the emergence of impacts post-event peak.
When combined with satellite and other data products, these characteristics enable relatively rapid assessments of most impacts associated with extreme floods (Ward et al., 2017[37]). Many other significant impacts of flooding are known to be poorly captured in extreme event impact databases. These include the mental health impacts of repeat events (English National Study of Flooding and Health Study Group, 2019[38]), flood-related displacement (Tong, 2017[39]), or the well-documented disease burden of floods (Brown and Murray, 2013[40]; Marcheggiani et al., 2010[41]; English National Study of Flooding and Health Study Group, 2019[38]). The studies that have identified these impacts are constrained by the need for high-quality health data covering multiple different floods to improve statistical power. Estimates of these more diffuse or difficult-to-quantify impacts of flood events are rarely available until years afterward, and often for bespoke cases only.
Wildfires
Wildfire impact profiles share at least three similarities with flooding. First, they expose well-defined economic assets, which are often insured in wealthier countries. Second, definitive spatial boundaries often disaggregate where most impacts take place (i.e. buildings burnt down versus those that did not). Third, there are smaller temporal lags in the emergence of impact.
Uncertainties similarly exist around the wider health impacts of wildfires. Preliminary analysis of the air pollution from the 2019/20 Australian bushfires, for example, found approximately 400 excess deaths and over 1 000 hospitalisations attributed to bushfire smoke exposure (Borchers Arriagada et al., 2020[42]).
Floods are experienced more frequently and by a larger fraction of the global population than wildfires.1 Therefore, more information is available about which impacts are well measured or poorly quantified in different settings. This explains the different levels of “impact reporting coverage” between the two categories of event in Table 2.2.
Heatwaves
Unlike for flooding events, directly observable impacts from heatwaves rarely exist. Previous well-studied events, however, offer insight into impacts that arise during heatwaves. Of special note are exceptional heatwaves affecting southeast Australia in January 2009 (Steffen et al., 2019[43]). Re-assessment of the Australian heatwaves over subsequent years identified at least four significant categories of impacts (Zander et al., 2015[44]):
excess mortality associated with extreme heat (estimates of more than 500 deaths)
excess morbidity, with another estimated 3 000 hospitalisations from people suffering heat-related illnesses
economic losses from electricity and transport infrastructure impacts totalled AUD 800 million, with heat-related blackouts affecting half a million people, widespread transport disruption from rail infrastructure malfunctions and road closures due to bitumen melting
wider labour productivity losses estimated at hundreds of millions of Australian dollars.
Unfortunately, even the most comprehensive heatwave impact reports offered to disaster databases like EM-DAT are typically limited to one of the four factors: excess-mortality calculations. Moreover, only countries in Western Europe routinely provide such numbers (Guha-Sapir, Hargitt and Hoyois, 2004[45]; Guha-Sapir, Hoyois and Below, 2016[46]). Meanwhile, for the majority of low- and lower-middle income countries, no real-time monitoring mechanisms are in place for any of these four (or more) categories of heatwave impacts. As a consequence, heatwave impacts are often not reported at all, despite clear meteorological evidence and longer-term retrospective analyses pointing to the opposite conclusions (Russo et al., 2016[47]; Ozturk, Saygili-Araci and Kurnaz, 2021[48]; Zittis et al., 2021[49]).
In short, robust evidence suggests that any instances of extremely high relative temperatures that persist for more than several days – in other words, a heatwave (Perkins and Alexander, 2013[50]) – can cause substantive social, health and economic impacts, irrespective of the location considered (Gasparrini et al., 2015[51]; Ebi et al., 2021[52]). The direct and indirect economic costs of a truly unprecedented heatwave (akin to the 2003 Europe event) affecting an unprepared (Fouillet et al., 2008[53]; Hess et al., 2018[54]) megacity in the Global South, for example, would likely approach hundreds of millions of dollars (Steffen et al., 2019[43]). In such an event, thousands of people could die from heat stress (Whitman et al., 1997[55]; Robine et al., 2008[56]; Mora et al., 2017[57]) and tens of thousands more could suffer from heat-related morbidity (Vaidyanathan et al., 2019[58]).
As it stands, few countries of the world have the capacity to provide real-time or near-real-time reports of the economic or morbidity impacts of heatwaves to impact databases (Tschumi and Zscheischler, 2019[33]). Indeed, many of the world’s most vulnerable countries lack any monitoring capability to detect impacts of heatwaves. This results in no heatwave “events” being reported to disaster databases (Harrington and Otto, 2020[34]). These reporting deficits contribute to mistaken perceptions. They suggest that extreme heatwaves either do not occur, or do not cause significant economic or health impacts in lower income or climatologically hot regions (Tschumi and Zscheischler, 2019[33]; Otto et al., 2020[59]). Until such reporting gaps are closed, thousands of excess deaths due to extreme heat will likely continue to go unreported every year. Despite forecasts a week in advance, clear warnings and community actions in a developed country region, the province of British Columbia alone reported that excess deaths had tripled to 719 during the heatwave of June/July 2021 (Government of British Columbia, 2021[60]).
Drought
Many lower income countries have detailed monitoring frameworks for direct crop losses from drought. This is due to the importance for non-governmental organisations to identify food insecurity and subsequent humanitarian concerns (Benson and Clay, 1998[61]; Clay and Stokke, 2000[62]; Harrington and Otto, 2020[63]).
Beyond the monitoring of direct crop losses and potential water scarcity in vulnerable regions, many economic impacts associated with drought are highly diffuse. Indirect economic losses can sometimes take several years to emerge. This lag creates problems when trying to compile a catalogue of impacts soon after an event occurs. In addition, complex macroeconomic modelling tools are often needed to successfully quantify such losses (Kamber, McDonald and Price, 2013[64]; Edwards, Gray and Hunter, 2019[65]). This creates further disparities in reporting quality between those countries that can routinely identify these impacts, and those that cannot.
2.2.2. Variation in modelled climate sensitivity
Equilibrium climate sensitivity (ECS) refers to the change in global mean temperature following a doubling of atmospheric CO2-equivalent (CO2-eq2) concentration (Kattenberg et al., 1996[66]). In other words, if one doubles the amount of CO2-eq concentration in the atmosphere and waits for the climate to respond, the resulting temperature increase once the climate reaches equilibrium again is the ECS.
Another type of sensitivity of the Earth system is the so-called transient climate response (TCR). TCR differs from ECS as it refers to the amount of warming that occurs at the time the CO2-eq concentration doubles following a linear and steady increase in emissions (having increased gradually by 1% each year), as opposed to when the system has reached equilibrium. TCR is more closely related to the way cumulative GHG emissions have changed in the more recent past.3
The ECS and the TCR are not simple metrics. Rather, they are the result of complex response within the Earth system and have been the subject of research over many decades. For the last 40 years, there has been high confidence that ECS is somewhere between 1.5-4.5°C (Ad Hoc Study Group on Carbon Dioxide and Climate et al., 1979[67]; IPCC, 2013[2]). The most recent IPCC Assessment updates this to a likely range of 2.5-4°C (high confidence), with a best estimate of ECS of 3°C. This update is based on improved knowledge of climate processes, paleoclimate evidence and the response of the climate system to increasing radiative forcing. In other words, a doubling of CO2-eq emissions concentrations in the atmosphere would result in an increase of 2.5-4°C in surface mean temperature of the planet (IPCC, 2021[1]).
TCR is lower than ECS within a likely range as 1.0°C to 2.3°C (that is, each1000 PgC of cumulative CO2 emissions is assessed to likely cause a increase in global surface temperature with a best estimate within that range), with a best estimate of 1.65°C (IPCC, 2021[1]). This reflects delays and lags in the climate system such as the time required to transfer heat to the deeper levels of the ocean and reach a new equilibrium. The size of the temperature increase these emissions determines the extent and severity of climate change associated with anthropogenic emissions. Consequently, ECS and TCR are key metrics for understanding present and future human-made climate change.
A first-order question about a climate plan or strategy is its likely effectiveness. Uncertainties surrounding ECS and TCR have direct consequences for the scale of the response of the climate to GHG emissions (Figure 2.1). The place of ECS within the 1.5-4.5ºC range will have a direct consequence on the severity, timing and scale of climate impacts with important societal ramifications.
Pledges by countries to reduce emissions are inadequate to achieve the long-term temperature goal of the Paris Agreement (UNFCCC, 2015[68]). If realised ECS falls within the high end of the distribution of current ECS estimates, then, resulting emissions levels could lead to even higher warming (and impacts) than currently projected in the second half of the century. In that case, emissions reductions would need to be accelerated or carbon dioxide reduction (CDR) technologies deployed earlier and at a greater scale than might otherwise have been the case. Some recent literature suggests that even considering these uncertainties, the 1.5ºC temperature goal of the Paris Agreement is physically achievable (Holden et al., 2018[69]; CONSTRAIN, 2020[70]), though immense effort and luck would both be required. A good understanding of ECS uncertainty is therefore crucial for any climate risk assessment and policy making in relation to both mitigation and adaptation. Despite many efforts to narrow the ECS uncertainty range, it has remained large over the past four decades.
There are many ways and lines of evidence used to determine ECS and TCR, each based on multiple studies, models and data sets. These include data from the deep past (paleoclimate), recent observations and outputs of climate models.4 A recent study, using these multiple lines of evidence, has narrowed the range down in a meaningful way (Sherwood et al., 2020[71]). The study concludes that values on the low-end of the ECS uncertainty range (i.e. below 1.5 degrees), where the climate response to anthropogenic emissions is lower, are very unlikely. It suggests less than a 5% chance of an ECS lower than 2 degrees. By contrast, it concludes there is a more than 5% chance of values above the higher end of the AR5 range (over 4.5 degrees). As in previous assessments, the warming by the end of the century could be higher than the upper range of warming levels expected today for any given scenario for GHG concentrations over the course of the century.5
Other studies also suggest the response of the climate to increases in GHG concentration is “fat-tailed”. In other words, there is a slim, but sufficiently large, probability of extreme warming (Ackerman, Stanton and Bueno, 2010[72]; Wagner and Weitzman, 2018[73]; Weitzman, 2009[74]). ECS uncertainties have been partially quantified from ensembles of climate model runs. These assess the robustness of the results given different available models and plausible parameter ranges. The shape of the distribution of ECS estimates from such methods indicates the levels of uncertainty. It also indicates the chance that ECS will be realised on the high end of the range. However, the tails of the distribution are sensitive to assumptions. Box 2.1 explores the implications of different ECD distributions. ECS, rather than an input, is an outcome of a model run. It depends on and changes across models based on how complex climate system dynamics are modelled.
Box 2.1. Implications of different ECS distributions
One way to estimate ECS is through climate models. Deterministic projections (i.e. cause and effect predictions) of changes in the climate are subject to a large number of uncertainties. These arise from incomplete understanding of the climate system, as well as imprecise models and the sensitivity of models and model runs to initial conditions set by the modeller (Collins et al., 2013[75]). There are also only a relatively small number of models and they disagree significantly. This is relevant for the way ECS is estimated.
To illustrate the understanding of uncertainties in ECS arising from models, Figure 2.2a compares the probability distribution of ECS as per Sherwood et al. (2020[71]). The most recent ECS estimates are under CMIP6 (Meehl et al., 2020[76]). First, Figure 2.2a shows that CMIP6 ECS estimates from climate models are higher than the AR5 1.5-4.5°C range, with only very few values falling below 1.5°C and many values surpassing the upper limit of 4.5°C. This increase is well documented in the literature. It stems mainly from stronger positive cloud feedbacks from decreasing extratropical low cloud coverage and albedo in models (Zelinka et al., 2020[77]).
Beyond the absolute values of ECS, a visual interpretation of the histogram in Figure 2.2a and b shows fewer lower values of ECS than higher values. This suggests the shape of the distribution fitting those values is fat-tailed towards higher estimates. Figure 2.2b fits, for illustrative purpose only, two types of naïve distributions to the CMIP6 ECS data. Figure 2.2b shows that assuming a fat-tailed, lognormal distribution would lead to higher probabilities of higher values. Conversely, assuming a Gaussian curve (normal distribution) would decrease the probability of these higher values. Therefore, the assumed shape of this distribution influences the estimated probability of higher levels of warming, an understanding important for informing measures dealing with climate risk.
This distribution fitting is by no means proposed as alternative estimations of ECS distributions to the one presented in Sherwood et al. (2020[71]). The Sherwood study uses multiple lines of evidence and constitutes state-of-art estimates of ECS. The difference between the two panels reflects the effect of the additional data available to a more sophisticated (Sherwood) method, in particular the effect on the tails. Any such distribution is an attempt to visualise our present uncertainty and (lack of) knowledge, reflecting a summary of many expert judgements rather than a quantification of some physical truth ( (Jebeile and Crucifix, 2020[78])).
Figure 2.2. Distribution fitting of CMIP6 Equilibrium Climate Sensitivity estimates

Note: CMIP6 estimates were obtained from (Meehl et al., 2020[76]) and span over 1.1-5.6ºC. Distribution fitting in panel b was performed using R, package “fitdist” by maximum likelihood estimation over variable parameters (mean, standard deviation) within the class of models.
Estimated ECS and TCR levels have direct implications for estimates of the remaining carbon budgets consistent with different long-term temperature goals. Uncertainties in ECS and TCR, which are properties of the climate system as a whole, introduce high levels of uncertainty for mitigation pathways globally and for implications regionally/locally.
The need for urgent and stringent emissions reductions at all levels becomes even more evident in light of these uncertainties. This is especially true as the most recent research rules out low (less-damaging) values while maintaining the possibility of high (more-damaging) ones. As a result of continued research into climate sensitivity, understanding of emissions budgets and the physical processes that could lead to high-end outcomes have evolved and improved substantially.
Given the above, working emissions scenarios and their implication for global mitigation and local adaptation are not really independent from an understanding of ECS and TCR. Closely monitoring changes in emissions and changes in temperatures and impacts is therefore crucial. This monitoring can allow for emissions scenarios to be incrementally improved to match the reality of climate change and for action to swiftly respond to the improvement of knowledge.
A slow response time in climate politics is a high-risk approach, which assumes the lower end of possible carbon budgets in the hope that the lower end could become the higher end with further information. But it could go the other way. Budgets set at a 66% chance, or even 50% chance, of meeting the global temperature goal, are more vulnerable to changes than those that take a more robust attitude towards the need to meet the target.
2.2.3. Uncertainties in future emissions projections and climate forcing
In addition to the uncertainties on how the climate responds to climate forcing, detailed prediction of future climate change is also limited by i) uncertainties in the projection of future GHG emissions levels; and ii) uncertainties in how those emissions translate into GHG concentrations in the atmosphere, which will determine the amount of climate forcing (Figure 2.1).
First, uncertainties in projected emissions stem from the imperfect ability to predict future activities around the world and therefore the resulting emissions. This is due to the complex interplay of rapidly changing societal, technological, economic and other political choices for governments, countries and citizens in the short-, mid- and long-term.
Second, uncertainties related to how emissions will ultimately translate into climate forcing are directly linked to the current understanding of carbon cycle. In other words, they are linked to the series of processes by which carbon compounds, including CO2, are interconverted and incorporated in the environment and returned to the atmosphere (see Box 2.2)
Estimation of future emissions of GHGs, aerosols and their precursors is crucial for any analysis of future climate change. However, the prediction of future anthropogenic emissions is mainly governed by political, economic and societal choices. Therefore, the exercise of predicting short-, mid- and long-term emissions is inherently speculative, based on understanding of socio-economic systems and assumptions about potential choices. Because politically-determined targets and aspirations do not necessarily translate into perfect regulation of human activities, the question is not simply to predict what kind of future will result from each action, select the future most wanted and determine emissions accordingly. Instead, there is a messy interface between the predictions of science, the aspirations of politics, and the real-world actions of individuals and organisations. Nevertheless, one should beware of treating social change as a problem of prediction rather than action: leadership will be required to orient the social and economic world towards emission reductions, and the assumption that no leadership exists may be an unfortunately self-fulfilling prophecy.
Climate modelling of future GHG emissions relies therefore on building “scenarios”, each associated with different plausible narratives describing how societies may evolve. Scenarios will then be used to estimate different climate change futures that can inform action and actual choices of societies and governments.
Box 2.2. Carbon cycle uncertainties and RCP scenarios
The RCP scenarios are emissions concentrations pathways. While strongly correlated, GHG emissions and GHG concentrations in the atmosphere are not the same. As such, there is not one emissions pathway that is linked to one RCP scenario.
Figure 2.3. Time series of annual emissions compatible with RCP scenarios

Note: Compatible fossil fuel emissions simulated by the CMIP5 models for the four RCP scenarios. Time series of annual emission (PgC yr–1). Dashed lines represent the historical estimates and RCP emissions calculated by the IAMs used to define the RCP scenarios; solid lines and plumes show results from CMIP5 Earth System Models (model mean, with one standard deviation shaded).
Source: (Stocker et al., 2013[79]).
Indeed, projections of GHG concentrations over the 21st century rely on simplifying assumptions about biogeochemical cycles, including the carbon cycle. Uncertainties about the carbon cycle include the relation between increased CO2 emissions and terrestrial carbon uptake and storage and the role of “tipping point” feedback processes such as permafrost thawing, forest dieback and the weakening of land and ocean carbon sinks (Steffen et al., 2018[80]). Considering these uncertainties, a range of possible emissions trajectories could lead to a certain level of concentration (Figure 2.3). These uncertainties have implications for policy making (and not concentrations) as human activities lead to emissions. Climate policy and action can therefore only directly regulate emissions.
Uncertainties about the carbon cycle are potentially much larger than assumed (Higgins and Harte, 2012[81]). This could effectively mean that carbon cycle responses could lead to higher levels of warming than expected. In this sense, using concentrations instead of emissions may lead to an underestimation of warming and neglect research and understanding of possible feedback processes. It is important to assess these ranges of emissions systematically so they can be narrowed over time.
In turn, how these emissions will translate into concentrations will determine the extent of climate change. From a physical point of view, and for the purpose of climate modelling, a focus on concentration pathways makes sense as it reduces one level of uncertainties. However, these uncertainties can have important implications for policy making and cannot be ignored.
Climate policy commitments and pledges worldwide indicate where emissions are headed over time. Nationally Determined Contributions (NDCs) under the Paris Agreement often include emissions reductions commitments. These limit emissions (or emissions intensity of, for example, economic output) to a target emissions range at a target year or period. In addition to NDCs, a large number of countries have put forward commitments to reach net-zero CO2 or GHG emissions by mid-century. Such commitments also serve as a signpost for where emissions are heading.
Emissions levels in line with those commitments do not necessarily need to directly inform the development of scenarios. However, they provide important information in relation to the plausibility of different existing scenarios. In May 2021, analysis showed that NDCs and longer-term climate targets put forward by countries would lead to warming of about 2.4ºC (1.9-3ºC) by the end of the century (CAT, 2021[82]).
The IPCC Fifth Assessment Report developed four Representative Concentration Pathways (RCPs). The low-carbon mitigation scenario comprises stringent climate and energy system interventions, while a high-carbon scenario assumes continued growth in emissions. These were later complemented with an additional scenario in line with the goal to pursue “efforts to limit the temperature increase to 1.5°C above pre-industrial levels”, adopted in the Paris Agreement (UNFCCC, 2015[68]) (see Chapter 1, section 1.2.2 and Figure 1.1).
Integrated Assessment Models (IAMs) produce global emissions scenarios. These models consider the potential evolution of energy system, population growth, economic development and other drivers of emissions such as agriculture and land use. IAMs are traditionally used to examine the cost-effectiveness of achieving mitigation goals. Overall, they are useful and widely used tools for answering policy-relevant questions at different levels although they also have blind spots and limitations which require consideration e.g. (Gambhir, 2019[83]).
Based on results from IAMs, a second set of scenarios, the Shared Socio-economic Pathways (SSPs), have been constructed. The SSPs show in more detail the socio-economic and technological factors that result in different emissions and thus concentration pathways (see also Section 2.3.2). Importantly, the different SSP scenarios provide critical information on potential global socio-economic and technological choices. These can be put into the context of NDCs and longer-term net-zero targets. Figure 2.4 shows global trajectories for nine RCP/SSP scenario combinations for CO2 emissions that form a basis for the physical study of different future worlds, with different levels of warming and climate change impacts.
Figure 2.4. Global CO2 emissions in different RCP/SSP scenario combinations

Note: Harmonised trajectories of Global CO2. Scenario names refer to combination of SSP/RCP scenarios as such: SSP1 1.9 denotes SSP1/RCP1.9 scenario combination and so forth. OS stands for “overshoot” and LowNTCF for low near-term climate forcing species emission.
Source: (Gidden et al., 2019[84]).
There are no probabilities attached to the scenarios as they rely on a number of choices and assumptions (e.g. spread of a technology), which are not describable as probabilities. In this sense, there are no right or wrong scenarios. Instead; they can be more or less plausible based on, for example, real world policy, socio-economic and technological choices and describe potential implications of those. Recent work has questioned the plausibility of the highest scenario (Hausfather and Peters, 2020[85]). RCP8.5, the highest concentration pathway in the scenario set, would be unlikely on the basis of emissions alone. It would require major players to walk away from their commitments and burn fossil fuels beyond the likely economic availability of the resource.
In general, as time advances the scenario space becomes more constrained. In future, therefore, new sets of scenarios will reflect the new dominant axes of social and political uncertainty. These scenarios should be constructed in a way that is useful for policy makers. For example, they could distinguish feasible social and political choices or outcomes, by highlighting barriers, opportunities, synergies and trade-offs across different pathways, or by incorporating the systemic effects of losses and damages. A wider range of futures analysis techniques may be required to complement information from energy and integrated assessment models (Gambhir, 2019[83])
It is impossible to know what choices governments and societies in general will make in the short‑, mid- and long-term. However, RCP and SSP scenarios together provide policy makers with important insights on how these choices determine the type of transition for the world in the next decades. In so doing, they can help inform these choices. For example, there are different ways to achieve the goal of limiting warming to 1.5°C by 2100 (IPCC, 2018[86]). These depend on choices of different socio-economic potential trajectories, resource efficiency measures, and societal and technological developments. They also consider trade-offs between early, deep reductions and the use of atmospheric CDR technologies. Pathways relying on low energy demand and low material consumption use only a limited amount of CDR. On the other end, scenarios with high levels of future energy demand show lower levels of CO2 emissions reductions to 2030; they rely to a much greater extent on the use of CDR technologies later in the century [ (IPCC, 2018[86]), and Figure 6.4)].
Put another way, the assumed extensive use of large-scale CDR is the only way for high-consumption pathways to meet the Paris ambition of limiting warming to 1.5ºC. There is no certainty these technologies can be deployed at reasonable costs or risks. Therefore, the high emissions growth pathways carry higher implementation risk for the Paris goal.
Scenarios frameworks, such as the SSP/RCP scenarios and their predecessor SRES scenarios (IPCC, 2000[87]), can be useful frameworks. They can shape policy makers’ understanding of global transformation trends over long time horizons needed to avoid the most dangerous impacts from climate change. At the international level, the RCP scenario framework informed the work of international policy makers. For example, in the context of international climate negotiations, the framework contributed to adoption of common and global temperature goals of the Paris Agreement.
The usability of such scenarios is less direct and evident for policy makers at the national level. For example, a national group that plays the crucial role of turning objectives into actual emissions reductions can act in their jurisdictions and localities, such as cities or states. However, information provided by these global scenarios is not directly fit for their purpose. First, temporal and spatial scales in these are too coarse for policy making at finer scales. In addition, climate policy action will depend on a range of factors that go beyond what these scenarios consider and capture even though efforts are underway to include more areas of relevance for decision making at national level.
The use of scenarios is intended to broaden a debate and capture the most important feasible axes of uncertainty. This is useful in simplifying the wide range of possible outcomes to a tractable plausible set. These scenarios inform and frame debates about socio-economic and technological development over the next century, identifying trade-offs and the most crucial elements of the low-carbon transition.
In collapsing the range of plausible futures to a handful of possible outcomes, however, other uncertainties are necessarily disregarded that may turn out to be significant. For example, the emissions implied by RCP8.5 are now thought to be implausible (Hausfather and Peters, 2020[85]). However, carbon cycle feedbacks could one day result in RCP8.5-like concentrations with lower anthropogenic emissions (see also Section 2.2.2 and Box 2.2). On the socio-economic side, some researchers consider that systematic effects of abrupt social, political or technological changes should be more directly incorporated into energy modelling approaches (McCollum et al., 2020[88]). Indeed, to inform policies most effectively, decision makers must keep in mind the possibility of higher impact, lower probability unfolding of climate change.
There are also a number of well-known shortcomings associated with IAMs in failing to model certain key aspects of the real world. For example, IAMs only implicitly considers politics as a driver for emissions reductions or delay of action (Peng et al., 2021[89]). A range of political and socio-economic aspects determines the extent and types of action of countries, businesses and societies that global scenarios do not currently capture. For example, a central challenge in the world today is reducing economic inequality. How countries achieve that societal objective may have important implications for decarbonisation. Peng et al. (2021[89]) explore concrete areas that could improve these models, as for example the inclusion of public opinion or how trade and investment policies can be captured.
Projections based on real world drivers can indeed help shape action at the local, subnational and national levels. These projections are made in the context of emissions reductions needed globally to avoid the most dangerous impacts of climate change. In addition to global scenarios, confident information about where emissions are heading can answer a number of questions relevant to policy makers.
First, confident information can provide a good indication of whether a policy or a strategy will be effective in reducing emissions in line with national commitments. This can be a crucial step in leveraging buy-in of the policy plan by decision makers and by the public. Second, a roadmap towards commitments, in particular long-term commitments, provides concrete policy options that clarifies how the country plans to achieve the target. This makes them more credible (Rogelj et al., 2021[90]). Such roadmaps can support the formulation of concrete implementation plans. As such, they rely on the projection of emissions and explore policy options leading to needed levels of reductions. Lastly, such roadmaps are also the basis for evaluation of fairness and adequacy of commitments from a country perspective. This can help advance discussions around the issue of equity (Rogelj et al., 2021[90]).
2.2.4. Uncertainties in projections of global and regional climate change
As highlighted in Chapter 1, climate risk is a function of hazards, vulnerability and exposure. In order to better characterise the climate risk, and the risk of losses and damages, an important effort is to better characterise how, where and by when different types of potential hazards (e.g. extreme weather events such as heatwaves, flooding or droughts) may occur. As discussed in previous sections, this work is done using different models. These range from Global Circulation Models (GCMs) to downscaled models, regional models and models focusing on particular sectors of the economy and types of impacts.
At the global level, much of that exercise involves many academic and operational institutions around the world in the context of model intercomparison projects. These include the Coupled Model Intercomparison Project (CMIP), co‑ordinated by the World Climate Research Programme (for details see to Box 2.3).
These projects have helped construct a consistent and policy-relevant knowledge base and a foundation for the IPCC assessments. They compare the outputs of different models and show how, for different future warming scenarios (see Section 2.2.3), climate change may unfold around the globe for different regions and timeframes. The level of confidence in these different projections depends on a range of factors. Effective and accountable model-informed decision making needs to consider the varying degrees of confidence and value of the information for different types of decisions (Nissan et al., 2019[91]).
The next sub-sections explore different levels of confidence in GCM data in two ways. First, they examine different spatial and time resolutions. Second, they look at type of processes and hazards to examine where larger uncertainties can lead to a larger range of potential climate risk.
Different levels of confidence in projections at different temporal and spatial scales: The case of extreme temperature projections
With mounting impacts from climate change threatening human and natural systems, there is an increasing need for information on future climate change to support policy planning and climate risk management strategies (Wang et al., 2016[92]; Donatti et al., 2016[93]; Giuliani et al., 2017[94]; Finn, 2020[95]). The varying temporal and spatial scales at which this planning is necessary require different types of information, informing different types of responses. For example, long-term mitigation strategies at the global level require information in substantially longer temporal and larger spatial scales than responses to immediate localised threats. Using an illustrative example of temperature projections from state-of-the-art climate models (CMIP6), this subsection explores how the information from these models can best inform climate action and strategies.
Chapter 1 discussed the high confidence in the relationship between human-made emissions and the rise of average global temperatures. Indeed, climate models have performed well in predicting global mean surface temperature rise on the basis of anthropogenic emissions scenarios for the last five decades (Hausfather et al., 2020[96]). This suggests that climate models capture well the key underlying physical processes driving the rise in temperatures (Hausfather et al., 2020[96]). This gives confidence in the ability of climate models to project temperature increase resulting from different future emissions levels, albeit with some uncertainty.
Climate change will not unfold uniformly around the planet. Warming will be larger over land than oceans and at Arctic latitudes, for example (Collins et al., 2013[75]). However, climate-related risks in general increase with degrees of average warming of the planet.
Indeed, climate risks for natural and human systems are higher for warming at 1.5°C than at present at 0.87ºC6, and higher again at 2°C (IPCC, 2018[97]). Global average temperature increase is therefore a key metric for the approximate magnitude of climate change impacts in different potential worlds of changed climate. The timing of potential crossing of thresholds of increased risks of physical hazards, such as extreme or slow-onset events or tipping points, is a simple metric to discuss with policy makers and stakeholders. These thresholds could include, for example, crossing tipping points and the increased frequency and severity of extreme weather events.
Global average temperature, however, is not a metric that can directly inform policy makers of specific localised temperature events for reasons discussed earlier. These events are related to the less well-understood climate dynamics that are driven by the increased energy flow into the climate system overlaid on local weather patterns.
Indeed, while the frequency and severity of many of such events, including hot extremes, marine heatwaves, and heavy precipitation, increase with average warming of the planet, severe heatwaves affecting human lives and livelihoods happen already at current – relatively low – levels of average warming (Stott, Stone and Allen, 2004[98]; Vautard et al., 2020[99]; Le Tertre et al., 2006[100]). In the last days of June 2021, for example, Pacific coast areas of the United States and Canada experienced record temperatures. A recent study shows these heatwaves were virtually impossible without human-induced climate change: the event was statistically estimated to be about a 1 in 1 000 year event in the current climate (Sjoukje Philip et al., 2021[101]).
Yet global average temperature projections in models are calculated as the average of a number of discrete projections of temperature made at the grid-level of the models7 at much higher temporal and spatial resolutions. Thus, a focus on the global average temperature change alone would obscure the wealth of more granular information available from model projections at these higher resolutions.
The section uses the most recent projections for extreme temperatures from GCMs in the CMIP6 database (Box 2.3) to explore these underlying model projections. Specifically, it explores the level of variability and agreement across models. Ultimately, it examines the confidence in these projections at different temporal and spatial scales for a subset of the models comprising the CMIP6 database. In so doing, it aims to shed light on the value of these climate projections for guiding short-term decisions to reduce those risks through, for example, adaptation measures.
Box 2.3. Overview of climate model intercomparison projects
The overall goal of the Coupled Model Intercomparison Project (CMIP) is to better understand the past, present and future of the climate system resulting from natural factors or from changes in climate forcing in the atmosphere caused by human and other activities. To that end, it uses a number of coupled atmosphere-ocean General Circulation Models (GCMs). GCMs represent physical processes in the atmosphere, ocean, cryosphere, biosphere and land, considering the interactions between them, for predicting climate at near-(next decades) or long-term (next centuries). CMIP, established in 1995 and in its sixth phase (CMIP6 – https://pcmdi.llnl.gov/CMIP6/), involves a large number of research institutions. They run their climate models under a standard experimental framework or use a common set of input parameters (Eyring et al., 2016[102]) to study and compare their outputs. This means that outputs from different models can be directly compared.
The CMIP exercise enables assessment of model performance, including by evaluating models’ strengths and weaknesses, to foster development and review of GCMs. CMIP also makes the resulting multi-model output datasets available for analysis and application in a variety of impact studies. The climate projections produced in the context of CMIPs underpin IPCC assessments on climate change.
For its part, CMIP6 provides the basis for scientific assessment on climate change in the IPCC’s Sixth Assessment Reports (AR6). CMIP6 uses over 100 models from 50 institutions to estimate how the climate will respond to different concentration scenarios, based on different socio-economic scenarios. GCMs project a range of climate variables around the globe at varying temporal and spatial resolutions, which can be analysed collectively in the CMIP context. Approaches have been developed to distil the most credible information on impacts and risks for stakeholders and policy makers (Eyring et al., 2019[103]).
CMIP is the largest and most comprehensive intercomparison project, but there are dozens of others. They range from the official and highly-coordinated to small unofficial projects. The others have similar aims and take a systematic approach to comparing different aspects of model inputs, outputs and performance.
Figure 2.5 shows extreme temperature projections for the cities of Hyderabad (India) and Paris (France) for three different scenarios of warming. These are: i) a low-carbon scenario in line with the 1.5ºC in the Paris Agreement (RCP 1.9); ii) a middle scenario (RCP 4.5); and iii) a very high-carbon scenario assuming continued growth in emissions (RCP 8.5). The locations explored in this section were chosen as broadly representative of different types of climates and levels of socio-economic vulnerabilities. The Indian State capital, for example, has higher vulnerability than the French capital (Kadiyala et al., 2020[104]). In addition, both cities experience more than global average impacts of climate change. For example, average yearly temperature in the French territory increased by 0.95°C between 1901 and 2000 – about 20% higher than the global average of 0.74°C (MEEDDM, 2009[105]).
Figure 2.5. Projected annual maximum temperatures and decadal averages for Paris and Hyderabad

Note: Annual surface temperature extreme and their decadal averages in Paris (yearly maximum extreme average in 48-49ºN 2-3ºE) and Hyderabad (annual maximum extreme average in 17-18ºN 78-79ºE). Data were obtained from CMIP6 database (https://esgf-node.llnl.gov/projects/esgf-llnl/) for daily extreme temperature (variable “tasmax”); for scenario combinations RCP1.9 (SSP1), RCP 4.5 (SSP2) and RCP 8.5 (SSP5); and for models CAMS-CSMi-0 (variant ‘r2i1p1f1’), EC-Earth-3 (variant ‘r4i1p1f1’), MIROC6 (variant ‘r1i1p1f1’) and UK-ESM1-0-LL (variant ‘r1i1p1f2’). Note that first decadal average point is calculated using only 5 data points (2015-2019).
Figure 2.5 shows, for all three scenarios, that annual maximum temperatures for both locations vary considerably on an annual time scale throughout the century. This is familiar from most people’s everyday experience: some summers are hotter than others. The average maximum annual temperature, however, increases over time as average global temperature increases. The trend is much clearer for the higher concentration scenarios RCP4.5 and RCP8.5. Different models show more or less change for each scenario for a number of reasons. These relate to how each of the models portrays the global climate historically and into the future. This section does not explore these implications, but policy makers should be aware of the high degree of variability in the annual data.
The models realistically show that extreme temperatures can already happen at lower levels of warming and in the near term. Because of this annual variability (Box 2.4) and the initial conditions used in the model runs, the precise timing of occurrence of heatwave events is expected to differ across different realisations of the same model and across different models. Despite these differences, trends should be reasonably consistent. Indeed, Figure 2.6 (panels 1a and 2a) shows a large spread and no correlation in the timing of yearly maxima anomalies when considering models together. This indicates that climate models cannot predict the exact timing of individual heatwave events with any skill.
Moving towards coarser temporal resolutions, such as the smoother decadal averages, reveals much greater agreement between models (see Figure 2.5 and the decadal average anomalies in Figure 2.6, panels 1d and 2d). With the large annual variability removed, the long-term trend is clearer and consistent between models. The decadal temperature extreme averages rise over time along with global average warming. In the highest warming scenario (RCP8.5) towards the end of the century, the severity of heat in even the coolest years could exceed the severity of the most extreme heat experienced today. In the strong-mitigation scenario (RCP1.9), modelled extreme heat remains similar to or only a little above the present climate. This provides an opportunity to reduce risks of physical hazards through stringent emissions reductions.
Figure 2.6 (panels 1a and 2a) draws a picture of scenarios in terms of temperature anomalies.8 It shows that in higher warming scenarios (RCP4.5 and RCP8.5) temperature extremes can exceed the average extremes in the 20-year baseline period by up to roughly 10ºC for both cities studied here. The same difference in anomaly may, however, mean different things for both cities. This is due to their different geographical locations and overall climate.
Paris has an oceanic and tropical wet climate. In recent years, the city has been hit by severe heat waves [most notably the deadly heatwave in 2003 (Le Tertre et al., 2006[100])]. An increase in the severity and frequency of those events can undoubtedly lead to high impacts. This is especially the case in the absence of continuous improvements in resilience.
Hyderabad has a dry climate with temperatures already significantly higher than in Paris due to its location. The same anomaly increase, then, could result in levels of temperatures that human and natural systems cannot physiologically endure. This could lead to the crossing of physical adaptation limits or major transformations in human societies and ecosystems (Hanna and Tait, 2015[106]; Andrews et al., 2018[107]; Stillman, 2019[108]). The relationship of geographic location and overall climate has important implications for the understanding of climate risk in these regions and for the risk of losses and damages.
Figure 2.6. Extreme temperature anomalies at different spatial and temporal scales

Note: Yearly surface temperature extreme anomalies (increase above baseline) at different spatial resolutions around Paris (France) and Hyderabad (India). Small, medium and large-scale temperatures result from average extreme temperature over a 1x1, 15x15 and 50x50-degree cells (48-49ºN 2-3ºE, 41-56ºN 5ºW-10ºE and 24-73ºN 22ºW-27ºE for Paris, respectively; 17-18ºN 78-79ºE, 10-25ºN 71-86ºE and 7ºS-42ºN 54-103ºE for Hyderabad, respectively). Data were obtained from CMIP6 database (https://esgf-node.llnl.gov/projects/esgf-llnl/) for daily extreme temperature (variable “tasmax”); for scenario combinations RCP1.9 (SSP1), RCP4.5 (SSP2) and RCP8.5 (SSP5); for models CAMS-CSMi-0 (variant r2i1p1f1); EC-Earth-3 (variant r4i1p1f1); MIROC6 (variant r1i1p1f1); and UK-ESM1-0-LL (variant r1i1p1f2). Extreme temperature anomalies were calculated as the difference of yearly maximum at any given year from the long-term average of yearly maxima for the period of 1986-2005. The choice of the baseline period is in line with baseline period used for temperature anomalies presented in AR5. Note that first decadal average point is calculated using only 5 data points (2015-19).
As with temporal scales, confidence in temperature extreme projections is lower at higher spatial resolution. Figure 2.6 shows that for both regions, the variability in projections is lower if spatial scales are larger. Like for temporal scales, climate variability determines the detail of where extreme temperatures occur at the small scale (Box 2.4). In this way, each model run is just one potential realisation of this (model-dependent) climate variability. The model can be different from how these heat events will be realised in the real world.
Figure 2.6 also shows that for larger temporal scales (panels 1d and 2d) and spatial scales (panels 1b and c and 2b and c), patterns in temperature are smoothed (i.e. they are flattened and less spikey). There is higher confidence in these smoother patterns to describe average features of the climate system. However, because they result from averaging, the patterns cannot be considered as direct indications of local climate at a certain point in time.
From a policy-making perspective therefore, high-resolution modelling shows the potential for many regions to experience episodes of extreme heat over the next century. However, it cannot predict the detail of exactly when and where they will occur beyond a timescale of weeks (Nissan et al., 2019[91]).
Information at more detailed temporal scales is still valuable for policy making. It informs on broad patterns and the potential for how climate change could unfold locally. As such, it can feed directly into the shaping of policies dealing climate-related risks including physical, behavioural and cultural adaptation to expected changes. Projections of extreme temperature per se do not completely determine impact. This is also a function of humidity, duration of heatwave, minimum overnight temperatures, wind speed and so on, plus any adaptation measures (Nissan et al., 2019[91]).
The prospects for improvement of such information are mixed. There is no likelihood of anything other than estimates of statistical risk for longer-term projections (decades to centuries). However, improvements to modelling can improve estimates of that statistical risk. This is especially true for specific geographical locations and quantifying more accurately the risk of extreme events that are rarely observed. This may inform adaptation plans, climate risk assessments, insurance provision and infrastructure planning.
Medium-range initialised climate projections (months to several years) may benefit from large-scale investments in climate model improvements, improving the ability to produce medium-range and more locally informative climate projections. Preliminary results from such initialised climate model runs suggest the potential availability of a small amount of statistical skill in forward prediction on seasonal to interannual and even decadal timescales (Smith et al., 2019[109]; Dunstone et al., 2020[110]).
Statistical skill, however, cannot be directly translated into predictive utility. For example, seasonal forecasts that offer a “40% chance of a hotter than average summer” are of quantitative use only to a small minority of stakeholders. For short-term forecasts (weeks to months), increasing the time scale of accurate weather forecasting by model improvement is of potentially immense benefit (Nissan et al., 2019[91]). This allows rapid measures to be taken in advance of forecasted events by, for example, setting up cooling centres or distributing information and water.
Box 2.4. Climate variability
Climate is defined as long-term average characteristics of weather in a given area over decades (usually 30 years). Climate variability refers to natural variations in the statistics of climate occurring on all timescales. The IPCC notes that “variability may be due to natural internal processes within the climate system (internal variability), or to variations in natural or anthropogenic external forcing (external variability)” (IPCC, 2018[111]). In everyday experience, one winter may be cold and wet and another mild and dry. However, this only represents actual climatic change when the average trend is sustained over many years. This also means, perhaps counter-intuitively, that colder years may occur “randomly” even in a warmer climate. Similarly, extreme rainfall may occur sometimes even if the climatic trend is towards drying.
The most well-known example of natural variability due to internal processes of the climate system is the rapid periodic warming of the eastern Pacific Ocean known as El Niño. El Niño and its “opposite”, La Niña, are patterns of changes to temperature, rain and wind patterns in many regions that last for months or years. An example of external natural variability is a major volcanic eruption, like Mount Pinatubo in 1991. This eruption led to the injection of large quantities of sulphur dioxide (SO2), into the stratosphere, causing a period of cooler than average global temperatures (Council, 2001[112]). In general, external factors are estimated separately and then given to models as an input. Conversely, internal factors are generated dynamically by models themselves. Some factors may be treated in one model as external, and in other models as internal. In either case, they are an additional source of variation between models.
Climate variability is sometimes referred to aleatoric or random uncertainty. This is the actual realisation of the climate, either in model simulations or in nature, which is subject to internal variability and the chaotic nature of atmospheric and oceanic dynamics. Each model will generate a different pattern of internal variability.
The scale of internal variability is relatively consistent and can be compared with observations of real-world variability. Nevertheless, the variability implies that each model run may predict differently how the climate will be realised. This means that even if one had complete knowledge of the physics and statistics of an event, it is still a long way from being able to predict exactly when they will occur.
Variability, then, has important implications for losses and damages. For example, as a result of climate variability, an area that is prone to hurricanes could be hit by no hurricanes in ten years or be hit by three hurricanes in one year. This would have consequences for adaptation policy measures or insurance.
Figure 2.5 and Figure 2.6, panels a and b, compare internal variability in four state-of-the-art climate models. When averaged over larger areas (panel c) and longer timescales (panel d) or over multiple models (coloured lines), natural variability is “averaged out”. Instead, the overall trend is visible. Both the trend and the superimposed variability are of high importance for the actual realised climate and weather experienced in any given location.
Different levels of confidence on projections of different types of processes: Temperature vs. rainfall
As discussed in the previous section, there is a high level of agreement and important features that are robust across climate models when projecting temperature at sufficiently large temporal and spatial scales. For example, global warming continues in the 21st century for all of the RCP scenarios. Temperature increase levels are similar during the decade after 2015. Warming rates increase significantly faster for higher concentration scenarios thereafter (IPCC, 2021[1]). In addition, temperature change will not be regionally uniform. There are also robust features in large-scale warming patterns across models. These include larger warming over land than seas; amplified surface warming in Arctic latitudes; and minima in surface warming in the North Atlantic and Southern Ocean [ (Collins et al., 2013[75]; IPCC, 2021[1]) and Figure 2.7]. This means that average land surface temperatures and those in the far North, in general, can be expected to increase by significantly more than the global average. Since the world’s human population live on land rather than in the ocean, the increase in average land temperatures is particularly important. In addition, almost half of the population lives in coastal areas or in close proximity to the coast, being directly or indirectly dependent on the ocean. Ocean warming can also therefore have devastating consequences, including through not only sea level rise but also, for example, by strongly intensified cyclones and changes to marine ecosystems.
Figure 2.7. Annual mean temperature change (ºC) relative to 1850-1900 for different warming worlds

Note: Simulated annual mean temperature change (°C) at global warming levels of 1.5°C, 2°C and 4°C (20-yr mean global surface temperature change relative to 1850–1900), based on CMIP6 multi-model mean change.
Source: (IPCC, 2021[1])
Climate models predict that global precipitation will increase with global mean surface temperature. These predictions are made in accordance with the basic physics of increased moisture held by warmer air. Estimates suggest the magnitude of this effect could be an increase of around 1-3% for every degree of warming (Siler et al., 2018[113]). In temperature projections, climate models show a general agreement about the above broad patterns of regional temperature change. However, there is less confidence on how changes in precipitation will be distributed spatially around the globe. Robust patterns emerge from projections of ensembles of models only in certain regions, with large areas and low latitudes in particular showing a lack of model agreement on even on the direction of precipitation changes (Figure 2.8).
The response of the climate in terms of precipitation involves more complex physical processes than that of surface temperature response to forcing. This includes, for example, the increase of the atmospheric concentration in water vapour with global warming, and cloud formation. Significant differences in cloud physics between models are partly responsible for the variation in climate sensitivity (Zelinka et al., 2020[77]). Other factors such as changes in atmospheric dynamics and water availability must also be considered (Collins et al., 2013[75]).
Due to these multiple interacting drivers, changes are not regionally uniform. What is less well understood is the considerably higher level of disagreement across models and the main reasons for the spread in precipitation projections for different regions of the world (Collins et al., 2013[75]; Shepherd, 2014[114]; Bony et al., 2015[115]; Zappa, Bevacqua and Shepherd, 2021[10]). Figure 2.8 shows where large changes in precipitation as a result of climate change are projected for different regions of the world. The plot distinguishes regions where models agree on the direction of change (full stippling) or those where a large response is projected but there is less agreement about the direction of change (open stippling).
To make use of the available information, multiple possibilities must be considered simultaneously. Different models can project changes in precipitation of different signs. In other words, the same region may be projected to become wetter or drier in a warmer world according to different models (Collins et al., 2013[75]). These discrepancies can be due to a number of reasons, including differences across models or, in some cases, a result of small ensemble size from each model (Rowell, 2011[116]).
Taking averages across models reduces the variation but hides important uncertainties. For precipitation projections of different signs, averaging could lead to a misleading picture of change. Specifically, it could result in values close to zero. This could lead to a false confidence on the absence of change, whereas large changes are actually being projected, only in different directions (Zappa and Shepherd, 2017[117]; Zappa, Bevacqua and Shepherd, 2021[10]). In addition, in a number of regions, changes in precipitation can also vary in sign within a year. For instance, the UK Climate Projections (Lowe et al., 2018[118]) project “hotter, drier summers and warmer, wetter winters”. Considering only annual mean values may hide some of these seasonal changes (Collins et al., 2013[75]), which are particularly important for loss and damage and for adaptation planning.
Figure 2.8. Projections of precipitation change based on CMIP6 model

Note: Projected change (percentage) in the annual mean precipitation by 2081-2100 in the SSP5-8.5 scenario as portrayed. Projected changes are quantified as the mean of the signal-to-noise of individual model responses (noting that “signal” refers to the mean response to climate change whereas “noise” refers to the unforced internal variability), rather than the signal-to-noise of the mean response. This aims to avoid the compensation arising from discordant individual model responses on the multi-model mean. Projected changes based on CMIP6 are shown as full stippling to indicate a robust response (≥90% of models agree on the direction of precipitation change). Conversely, the open stippling indicates a plausibly large response in a non-robust projection, that is, in either direction of precipitation change.
Comparing temperature and rainfall projections in GCMs is useful for policy makers and stakeholders. It helps illustrate the implications of using scientific information to support decision making in terms of quantitative regional and local climate predictions. While projections are available, they are subject to different types and considerable amounts of uncertainties. Efforts have been made in trying to unpack and better understand these uncertainties for temperature and precipitation (Hawkins and Sutton, 2009[119]; Hawkins and Sutton, 2010[120]):
Uncertainty in future emissions and therefore future radiative forcing, due to uncertainty over decisions affecting the future such as socio-economic and political choices (as discussed in Section 2.2.3).
Randomness in internal variability of the climate system, or realisation uncertainty, which is the natural variability of the climate system. This can arise even in the absence of changes to radiative forcing. Such variability is thought to be largely independent from future anthropogenic emissions but is superimposed onto the underlying anthropogenic trend (Box 2.4). Initialisation of models to present conditions may render the first months to years of internal variability marginally predictable.
Disagreement between models: as seen in Figure 2.5 and Figure 2.6, individual climate models will model somewhat different changes for the same radiative forcing because of how they treat the physical features of the climate system differently.
Discrepancy between models and real world: the set of climate models available share common assumptions and biases. This means the real outcome may not be perfectly represented by any of the models. It does not mean the models are worthless, only that model-derived information needs to be treated with care and be consistent with other evidence.
For both temperature and precipitation projections, the relative importance of the first three of the above sources of uncertainties in climate change projections varies significantly with region, time horizon of predictions and the temporal scale over which projections are being averaged (Hawkins and Sutton, 2009[119]; Hawkins and Sutton, 2010[120]).
Interestingly, for the two climate variables, the relative importance of these uncertainties over time varies in different ways. Figure 2.9 shows climate internal variability and model uncertainties are the dominant sources of uncertainties of temperature and precipitation for roughly two decades’ lead time of projections. Thereafter, scenario uncertainty plays a dominant role in projections of temperature. Conversely, for precipitation, model uncertainty remains the main source of uncertainty. Although these plots show changing fractional contributions to uncertainty, the absolute magnitude of all uncertainties increases with lead time. The analysis does not measure any discrepancy between models and real world.
Figure 2.9. Different types of uncertainties in global mean temperature and precipitation projections

Note: Fraction of total variance in decadal mean projections explained by internal variability (brown), model uncertainty (blue) and scenario uncertainty (green), for global mean surface air temperature (a) and precipitation (b)
The relative importance in the sources of uncertainties – more so than their exact quantification – is relevant for how climate change projections can best inform decision making. If mostly influenced by climate internal variability, climate projections over the next two decades need to be considered carefully. Impacts will be exacerbated by forcing resulting from emissions already in the system. However, how impacts will be realised still depends strongly on highly complex chaotic dynamics of the climate system that are difficult, if not impossible, to predict (Box 2.4).
The unexpected nature of recent climatic events, such as extreme rainfall in the People’s Republic of China and Germany, and extreme heat in Canada, underlines this unpredictability and the high importance of the variability superimposed on the trend. Short-term projected regional changes are therefore useful to provide a range of potential changes that might affect communities and ecosystems. The exact date and extent of changes or of extreme events cannot, however, be derived from these models.
State-of-the-art initialised climate models give only low (albeit statistically significant) levels of skill. They will be of interest mainly to highly quantitative, portfolio-averaged stakeholders such as large insurance companies. However, they will not likely be useful for individual adaptation decisions. To respond to these impacts, a focus on the resilience and adaptability of human and natural systems to possible changes and events, rather than on seeking to predict in detail, would better serve policy measures for losses and damages.
Over longer time horizons (about four decades), the main source of uncertainty in global and regional projections of temperatures lies in the emissions or concentration scenarios assumed. In considering these potential changes, policy makers could usefully consider the full plausible range of changes, including all evidence and not solely derived from numerical models. This, in turn, would permit an understanding of the minimum and maximum amount of change the system may have to adapt to.
The bounding scenarios, reflecting the decision makers’ tolerance for risk, can inform the time horizon from and over which the decision and policy measure need to be operational (Kotamarthi et al., 2016[121]). A city planner may treat emissions scenarios as fully external to her operational boundaries. Conversely, an international climate policy negotiator may treat them as a yet-to-be-determined choice.
For precipitation in many regions, disagreement between models remains the main source of uncertainty of regional and global change projections, with implications for policy making. Future changes in the frequency and intensity of drought and flood events are, however, critical to the resilience of many communities and ecosystems. Planning for a drought or for a flood entail different policy measures. As a result, projections remain difficult for regions where different models project different types of change altogether. It is important to note that for some regions such as the UK (Lowe et al., 2018[118]) both increased drought and increased flooding may be projected at different times of the year and seasons. In this case, measures need to tackle both types of hazards.
GCMs are thus indispensable tools for the understanding of the climate change phenomenon. However, while many qualitative results are highly robust and confident, many regional projections of detailed change are inherently uncertain in differing ways. The exploration and understanding of the remaining uncertainty is therefore in itself a highly policy-relevant output of modern climate science. Certainly, modellers have deep but incomplete understanding of the complex climate system. Furthermore, those models are limited by the extent of that knowledge. The uncertainty in future choices of societies and governments, which are actually the main driver of climate risk, is considerably more important for policy makers.
In light of this latter type of uncertainty, GCMs can be highly effective tools. They can strengthen collective understanding of potential different futures and of how decisions today can influence the risk of climate change tomorrow. Greater and more co‑ordinated investment in climate modelling capability could help tackle these uncertainties directly. In so doing, they could improve the relevance of scientific programmes (Palmer and Stevens, 2019[122]). The presumption that simply increasing computational effort will necessarily improve the reliability of modelling output is, however, disputed (Stainforth and Calel, 2020[123]). Other approaches based on narratives (Dessai et al., 2018[124]) and storylines (Shepherd et al., 2018[125]), which treat uncertainty in less rigid frameworks, are also active research areas with direct application to adaptation planning (Bhave et al., 2018[126])where model-based methods are subject to high levels of uncertainty.
2.3. Uncertainties relating to socio-economic exposure and vulnerability
The hazard component of risk is associated with uncertainties around the sensitivity of climate to GHG emissions (see Section 2.2). However, other components of risk – exposure and vulnerability9 – are influenced by socio-economic, institutional, political and cultural characteristics. Therefore, they are associated with the uncertainty underlying these characteristics. As opposed to physical hazards, socio-economic processes do not obey the laws of physics because they are a result of human choices. This makes it easier to influence these processes through policy and economy. However, it also makes it immensely more difficult to project them, compared to hazards.
While uncertainties in the physical climate and those pertaining to socio-economic systems are usually discussed separately, they interact in complex ways to determine risks of losses and damages. A better understanding is essential of the different levels and types of uncertainties underlying the vulnerability and exposure components of climate risk. This can inform policy makers’ decisions on how to manage those risks and take the right action. This section explores five broad types of uncertainties related to socio-economic vulnerability and exposure. It also introduces methods to characterise and possibly alleviate these uncertainties, while exploring implications for policy makers.
Different sources of uncertainties limit the understanding of how climate change will unfold. This complicates the assessment of the appropriate mitigation and adaptation actions to address it (Congressional Budget Office, 2005[127]; Gillingham et al., 2015[128]). Policy makers inevitably face uncertainties in making decisions. It is key to evaluate policies in light of that uncertainty. One can alleviate, but never eliminate uncertainties; the future, especially of socio-economic outcomes, will always be uncertain to an extent. The far future is even more uncertain. Examination of uncertainties, however, can still be productive. Better characterising – and potentially even decreasing – uncertainties informs on the potential outcomes of a policy action. More or better quality of information relating to uncertainties enables policies to be less risky and more effective.10 In addition, understanding uncertainties serves as a communication tool for the political process. Once uncertainties are characterised, there is common ground for policy disputes on how to act in light of them. Without characterisation, disputes are less likely to be transparent and, as a consequence, less likely to be fruitful.
There are potentially different methodologies to address different types of socio-economic uncertainties. Table 2.3 summarises the types of uncertainties included in this section with ways to address them and implications for policies. The characterisation of different types of uncertainties requires different methods and provides different types of information, ranging from quantitative to qualitative. Box 2.5 provides a selective overview of the estimated losses and damages from climate change with diverse range of methodologies, which also illustrates the impact of uncertainty on projected losses and damages. It is key to consider and meaningfully combine these different information types and methods without imposing a hierarchy. For example, qualitative information should not be secondary to quantitative information.
Table 2.3. Uncertainties and their methodological and policy implications
Source of uncertainty |
Methods to address it |
Policy implications |
---|---|---|
Quantitative data |
Statistical, econometrical approaches |
Update surveys with climate vulnerability and exposure questions. Use innovative data sources to complement traditional measures. Where there is lack of knowledge, use proxy variables, interpolation or statistical estimation. Use the estimated uncertainty in policy making process. |
Qualitative data |
Place-based approaches |
Complement quantitative analyses with qualitative ones to improve the design, targeting and implementation of policies. |
Projections of economic damages |
Computational models, such as integrated assessment models (IAMs), agent-based models, and reweight and resample techniques |
IAMs are immensely useful to compare the costs of different policies in the long run, but they do not examine uncertainty in detail. Complementing IAM analyses with models that explicitly assess the uncertainty of projections would help broaden policy making. |
Socio-economic role of nature |
Mix of methods: econometrics, place-based approaches, computational models |
The estimated costs of climate change are sensitive to the non-market value of nature and the role of nature in production. Each of these should be carefully considered in welfare and in the economy when designing policies. High uncertainty warrants precautionary approaches. |
Other |
Theoretical reflection, place-based approaches |
Policies should encourage triggering socio-economic tipping points, as they are needed for a long-term transformation to increase resilience. Social discount rates need to reflect the local values and cultures. Uncertain settings and sufficiently high damages imply low social discount rates. |
Box 2.5. Illustration of the effect of methodological choice through selected estimates
As an indication of the associated uncertainties, Table 2.4 shows a very selective overview of the impacts of climate change estimated in the literature. These pieces all look at different aspects of climate change, examining different hazards, sectors, regions or outcomes, and accordingly approaching the question with different methods and assumptions. As such, it is impossible to aggregate or average these numbers even if they aim to estimate similar outcomes. For example, both OECD (2015[129]) and Burke, Hsiang and Miguel (2015[130]) aim to look at the economic effects of climate change. However, Burke et al. (2015[130]) takes a top-down approach using econometric modelling, and examining how temperature changes are associated with GDP, and based on that association how changing temperatures would impact GDP. OECD (2015[129]), on the other hand, uses a computable general equilibrium model, which allows the inclusion of multiple types of hazards, and for a detailed examination of the mechanisms through which the impacts take place (e.g. decreasing labour productivity). However, both approaches have shortcomings, for example, they do not include the effects of possible droughts, nor indirect impacts through the effect of climate on ecosystem services. Neither aimed at providing estimates of the impacts associated with crossing climate tipping points.
Since the methods covered by the literature are different, and differ considerably, e.g. which hazard they examine or how do they take into account adaptation, they cannot be considered equivalent, cannot be added and will overlap to an extent. Note that all of the estimates are uncertain and incomplete, and can be taken only as an indicative estimate of the lower boundary of impacts. They show considerable range of estimated impacts, which illustrates the extent of physical and socio-economic uncertainty. Taken together, they point to sizeable climate impacts, motivating ambitious climate action.
Table 2.4. Selection of projected losses and damage estimates
Source |
Method |
Hazard |
Area of focus |
Region |
Estimate |
---|---|---|---|---|---|
Jafino et al. (2020[131]) |
Resampling and reweighing model |
Temperature increase and extreme events |
Levels of poverty |
Global |
In the range of 30 and 130 million additional people in extreme poverty until 2030 |
Sub-Saharan Africa |
In the range 10 and 50 million additional people in extreme poverty until 2030 |
||||
South Asia |
In the range of 10 and 60 million people in extreme poverty until 2030 |
||||
OECD |
CGE modelling |
Temperature rise, sea-level rise, cyclones and extreme temperatures |
Economic effects |
Global |
Around 1.5% of GDP until 2050 (1-3%) |
Sub-Saharan Africa |
Around 3% of GDP until 2050 |
||||
South and South-East Asia |
Around 2.5% of GDP by 2050 |
||||
Halegatte, Rentschler and Rozenberg (2019[132]) |
Econometric and modelling |
Extreme weather events |
Infrastructure damage |
Selected developing countries in Africa, Asia and South-America |
Around USD 1 trillion between 2020 and 2030 |
Burke, Hsiang and Miguel (2015[130]) |
Econometric |
Temperature increase |
Economic effects |
Global |
23% of GDP per capita until 2100, 10% until 2050 |
Sub-Saharan Africa |
Around 30% of GDP per capita until 2050, around 90% until 2100 |
||||
South-East Asia |
Around 30% of GDP per capita by 2050, around 90% until 2100 |
||||
Bastien-Olvera and Moore (2020[133]) |
IAM |
Temperature increase |
Non-economic damages to ecosystems |
Global |
Around USD 190 trillion of non-economic damages to ecosystems until 2050 |
Gasparrini et al (2017[134]) |
Statistics and computational projections |
Extreme temperatures through heat-related mortality |
Mortality |
Selected areas of Europe, Asia, Oceania, Americas |
Up to 10-15% increase in annual heat related mortality |
WHO |
Climate-health models |
Temperature rise, variable rainfall patterns and extreme temperatures, affecting malnutrition, heat stress, diarrhoea and malaria |
Healthcare costs |
Global |
USD 2-4 billion increase of annual healthcare costs between 2030 and 2050 |
Mortality through malnutrition, heat stress, diarrhoea and malaria |
Global |
250,000 excess deaths per year between 2030 and 2050 |
|||
Hayes et al (2018[136]) |
Literature review |
Extreme events |
Mental health |
Global |
Large effects on mental health, disproportionately affecting the most marginalised groups |
Tschakert et al (2019[137]) |
Systematic case analysis |
Climate change |
Non-economic losses and damages |
Global |
Intangible losses and at-risk sentiments are pervasive across the world |
Note: Percentages refer to the level of the shown year, i.e. a 90% decrease means in 2050 means that, if without climate change there the value would be 100 in 2050, with climate change it is purported to be 10 instead.
Source: (Bastien-Olvera and Moore, 2020[133]), (Burke, Hsiang and Miguel, 2015[130]), (Gasparrini et al., 2017[134]), (Hallegatte et al., 2015[138]), (Hallegatte, Rentschler and Rozenberg, 2019[132]), (Hayes et al., 2018[136]), (Jafino et al., 2020[131]), (OECD, 2015[129]) (Tschakert et al., 2019[137]), (WHO, 2014[135]) and author calculations.
2.3.1. Uncertainties related to the availability of socio-economic data
Data are a key component of the policy-making process. For example, data can be used to gauge past regularities and link the past with the future, providing a vantage point for projections (Haug et al., 2009[139]; Riahi et al., 2017[8]). Relevant data for exposure and vulnerability can be quantitative (unemployment rate or gross domestic product [GDP] growth) or qualitative (data from interviews or surveys and narratives). In addition, innovative data sources, such as satellite data or text-based data, can also advance knowledge on exposure and vulnerability. Where direct measurement is impossible, proxy variables, interpolation or statistical estimation can be used.
This subsection explores these different types of data. It looks at how they can contribute to better understanding of exposure and vulnerabilities. It also examines where remaining gaps and lack of information contribute to uncertainties.
Quantitative data
One source of uncertainty in understanding present and future vulnerability and exposure relates to availability of quantitative information. Specifically, the extent and quality of data on exposure or vulnerabilities are below average in regions where some types of hazards are projected to be harshest (IPCC, 2018[97]).11 For example, there are no official subnational population data available in some of the least developed countries, which could be an indicator of exposure of lives and livelihoods (World Bank, 2021[140]). Similarly, up-to-date detailed vulnerability data – for example, on hospital capacities or income –are available only in developed countries (WHO, 2021[141]). Any data about vulnerabilities or exposure cannot be fully complete. As explained in Chapter 1, vulnerability and exposure are multifaceted concepts and may be both direct and indirect; it is impossible to be sure to have captured all of the rapidly evolving aspects.
Even when using multiple sources of data, physical, mental and cultural vulnerabilities cannot be fully captured because of their varied, ever-changing – and sometimes subjective – nature. Still, in managing climate risks, policy makers must strive to obtain as much information to make robust decisions. This can put climate policies on firmer ground, making climate policies better targeted, more effective and more efficient, which leads to improved socio-economic outcomes. Traditional data sources, such as surveys and administrative data, could be used to inform levels of exposures and vulnerabilities (Brouwer et al., 2007[142]; Hallegatte and Rozenberg, 2017[143]). A range of data routinely collected by governments can inform on vulnerability and exposure (Deschênes, Greenstone and Guryan, 2009[144]) and on policies aiming at reducing vulnerabilities and exposure. For example, tax records show the size and incomes of households, which indicate exposure of lives and livelihoods in a given area. Household surveys usually collect information on home ownership, for example, which provide information on vulnerability (Taupo, Cuffe and Noy, 2018[145]).
Information from surveys, however, is not comprehensive and there is room for improvement. For example, surveys can be complemented with direct questions on awareness of climate exposures and vulnerabilities of firms and households. One can formulate a range of specific questions for a survey that address vulnerability and exposure. However, the specificity and complexity of questions must be balanced with the potential for reaching the largest share of the population possible. Indeed, survey questions should be as simple as possible to discourage respondents from opting out (Blair, Czaja and Blair, 2014[146]). Household surveys are considered to be one of the great innovations of social science research in the past century, upon which many policies were and are based. However, they also show a decline in quality recently. Non-response rates, for example, have increased (Meyer, Mok and Sullivan, 2015[147]; Brown et al., 2014[148]).
Composite indices are a way to use traditional data in a more comprehensive way (Nardo et al., 2005[149]). These indices are built from components (usually relying on traditional data). Thus, they can be comprehensive and provide a useful ranking for the most or least resilient regions or countries. For instance, Climate Risk Index, developed by Germanwatch, uses a reinsurance data on past extreme events. This is useful to measure exposure and vulnerability (particularly to extreme events) (Eckstein, Künzel and Schäfer, 2021[150]). Different indices, such as the ND-GAIN index or The Hague Centre for Strategic Studies’ Climate Vulnerability Index, include climate projections or potential readiness for various climate futures (Usanov and Gehem, 2014[151]; Chen et al., 2015[152]).
Index numbers tend to rely on ad hoc assumptions, such as what elements to include or the weighting of those elements. This leads to difficulty in interpretation. For example, it is not always clear what 10% difference between two countries means. For comparisons, all countries or regions should face the same weighing scheme for the index. In reality, different exposures and vulnerabilities might be important for different regions. Sea-level rise, for example, will be important for Small Island Developing States but less so for landlocked developed countries. Thus, index numbers are useful to provide a comprehensive snapshot and ranking but should be interpreted with caution.
Innovative data sources, such as satellite images, can complement traditional data. These sources often provide an option where traditional data are lacking or insufficient, enabling assessment of alternative measures of socio-economic exposure and vulnerabilities. For example, satellite data can provide subnational estimates of population and assets that are exposed. They can also inform on the level of vulnerabilities. For example, they can infer the area of affected crops and physical vulnerability of assets from the hues and shades of satellite images (Brown, de Beurs and Marshall, 2012[153]; Ceola, Laio and Montanari, 2014[154]). The World Bank also uses satellite images in cases where no adequate data are available about subpopulation from national statistical offices (World Bank, 2021[140]). Moreover, satellite data are usually freely available on the Internet (Turner, 2013[155]; LaJeunesse Connette et al., 2016[156]). These types of data enable a wide-ranging coverage of certain climate risks. However, again, they cannot be comprehensive, simply because exposure and vulnerability are multifaceted concepts.
Where direct data on vulnerability are unavailable, it can be estimated indirectly. Climate impact (risk) has three components: hazard, exposure and vulnerability (see Chapter 1). If the hazard is given, the remaining risk will describe the combination of exposure and vulnerability. In 2017, for example, Hurricane Maria caused estimated economic damage valued at more than 200% of the annual GDP in Dominica (Government of the Commonwealth of Dominica, 2017[157]). Thus, exposure and vulnerability of GDP to this hazard was quite large.
If exposure is also given, it is known the whole island was exposed to this hazard. If all of Dominica is exposed to a hazard like Hurricane Maria, for example, the economic costs exceed 200% of the GDP (IFRC, 2017[158]). This would be an indirect estimate of vulnerability for a given hazard and exposure. It can also work, the other way around: if there is information on vulnerability, it is possible to provide an indirect estimate of exposure.
A regression framework is a systematic way to make indirect assessment. In the case of Dominica, the framework would need data about the impact and exposure of similar events (e.g. Hurricanes David, Lenny and Erika). The multitude of data enables statistical abstraction from hazards, exposures and other relevant variables (e.g. macroeconomic conditions) to estimate vulnerability. For example, using a regression framework, the average hurricane strike is estimated to decrease the economic growth rate by at least 0.83 percentage points in Central America and the Caribbean (Strobl, 2012[159]). As another example, the annual cost of the Australian heatwaves during the end of 2013 and beginning 2014, has been estimated at USD 650 per person (Zander et al., 2015[44]); this estimates vulnerability of labour income to heatwaves. The hazards are given (the heatwave season of 2013/14); population exposure is abstracted away by examining the income per person.
As an advantage of this statistical approach, the only required variables are an impact variable (such as GDP or mortality), the hazards, and either exposures or vulnerabilities. In addition, statistical estimation of exposure of vulnerability is likely to be more comprehensive than using a direct measure. This is because it can capture all elements related to the impact variable but unrelated to hazards and exposures or vulnerabilities.
The approach also has disadvantages. First, the impact variable limits the indirect estimate. Impact on GDP, for example, will not capture non-economic vulnerabilities. The estimated impact of past climates on socio-economic outcomes is subject to statistical imprecision and sensitivity of results to assumptions (Newell, Prest and Sexton, 2021[160]). These statistical uncertainties can be explicitly assessed by statistical and econometric approaches. Knowledge on statistical uncertainties is often not used. Decision makers and academics tend to focus on the central results, even though statistical uncertainties carry important information for policy (Romer, 2020[161]). Finally, by nature, indirect estimate provides information on an aggregate level, with potentially low spatial and temporal resolutions than an individual data source. This makes its use difficult to assess local vulnerabilities and thus to tailor policies to local needs.
Other approaches are possible when direct measurement of vulnerability or exposure is impossible on the desired spatial or temporal scales. Interpolation techniques, for example, could support decision making. These techniques take exposure or vulnerability data on a more spatially or temporally aggregated scale, estimating exposure and vulnerability on finer scales. Information on finer scale could be important to accommodate local needs in policy making. For example, Uddin et al (2019[162]) create a risk map of the coastal region of Bangladesh on a fine spatial scale using interpolation. This could provide a roadmap for policies tackling flood risks. In Europe, similar methodology is used to monitor exposure to polluted air (EEA, 2008[163]). For policy makers, these methods help fine-tune policies to localities. However, interpolation makes more data based on existing sources. As such, it requires assumptions, which should be carefully assessed.
In other cases, a proxy variable may be preferable. This would not measure the variable of interest but rather a phenomenon that moves together (correlates) with the variable of interest. In the case of GDP, nightlight images from satellites were used to provide an approximation of subnational GDP levels in East Africa (Henderson, Storeygard and Weil, 2012[164]). Nightlight images were also used to estimate worldwide exposure to floods (Ceola, Laio and Montanari, 2014[154]) or to look at the short-term economic impacts of extreme weather events (Ishizawa, Miranda and Strobl, 2017[165]).
The frequency of certain words or phrases is another possible proxy variable to approximate vulnerabilities or exposure. Such words are found in official documents, journal articles, newspaper pieces, social media and other written communication. Here, the researcher compiling the data would search for expressions that indicate vulnerability or exposure in newspapers or Internet articles. How frequently are they mentioned at different times and places? In what contexts are they mentioned? What is their emotional connotation? Regions with higher climate vulnerability will have more discussion on the Internet and in newspapers about those issues (Archibald and Butt, 2018[166]; Bromley-Trujillo and Poe, 2020[167]).
The challenges posed by data availability are more pronounced in developing countries where a larger share of the economy tends to be informal. Generally, central and local governments have fewer resources and less capacity to conduct assessments. India, one of the countries most affected by extreme events, is projected to incur one of the highest costs from climate change (Kreft, Eckstein and Melchior, 2016[168]) and face specific challenges. Box 2.6 discusses these challenges, touching on migrant workers, informal economy, non-economic and indirect losses, and damages.
Box 2.6. Challenges of estimating losses and damages in India
Abundant evidence reveals that India has frequently experienced both extreme events and slow-onset processes induced by climate change. Such events affect lives and livelihoods, infrastructure, ecosystems, biodiversity and cultural heritage, among others (Government of India, 2011[169]). In fact, India is ranked among the ten worst countries affected by natural disasters. Three-fourths of its states and union territories are prone to disasters such as cyclonic storms, floods and droughts (Government of India, 2011[169]). Recently, both national and state governments have drafted climate change and disaster mitigation plans such as the National Action Plan on Climate Change and the State Action Plan on Climate Change. Disaster management plans have also been prepared at the district level. Risk-hazard mapping is one of the goals of the Prime Minister’s ten-point agenda for disaster risk reduction.
Migrant workers
Both climatic and non-climatic factors are responsible for rural-urban migration in India (Viswanathan and Kavi Kumar, 2015[170]). Migrant workers, including permanent and seasonal ones, are living in highly susceptible regions and exposed to climatic change. The informal sector employs a large proportion of migrants. These informal workers do not have voting rights in the city since their native village is listed on their identity cards, i.e., Voter card, Aadhaar card, driver’s’ licence, bank passbook, etc. Hence, they are denied relief and compensation that follows an extreme event, such as the 2015 Chennai flood [see (Patankar, 2019[171])]. Thus, losses and damages incurred by migrant workers are not included in loss and damage assessments. Not surprisingly, reverse migration is rampant following an extreme event. Such a reverse flow creates a shortage of labour in urban areas and delays recovery for the business units in the city. For example, around 83% of textile owners in Surat reported that labour shortage was a major issue during the recovery period (Bahinipati et al., 2017[172]).
Informal economy
Estimating losses and damages for the informal sector is also challenging. Most micro, small and medium-sized enterprises operate informally in India and absorb a large number of migrant workers. The informality implies that pre-disaster information related to stock and flow of capital assets, employment or supply chain is not available for a particular location hit by an extreme event. In addition, the workers are unlikely to be covered insurance. Thus, insurance claims cannot be used as a proxy for losses and damages. For instance, according to Patankar (2019[171]), around 93% of business units in Mumbai do not have insurance coverage for floods. Meanwhile, Bahinipati et al. (2017[172]) find that only one-fourth of total losses and damages were covered through insurance and government supported compensation for textile units in Surat. The flood that occurred in Chennai during 2015 led to economic losses and damages to smaller firms in the ballpark of INR 8.4 billion per week (KPMG, 2016[173]). Further, a study found that around 14 000 micro industries and business units were badly affected by flooding in Uttarakhand in 2013. This led to an estimated USD 631 million (Singh, 2018[174]) in loss and damages.
Non-economic losses and damages
In the case of non-economic losses and damages, the major challenges are conceptualising, accounting for and, if possible, monetising them (IPCC, 2012[175]; Serdeczny, Waters and Chan, 2016[176]). Since the stock of non-economic goods is not recorded, it is difficult to attribute ex post non-economic losses and damages from a natural disaster perspective. Methodologically, it is a challenge to estimate non-economic losses and damages in monetary terms, i.e. to price non-economic goods. Since these goods are not traded on the market, stated preference methods such as contingent valuation and choice modelling could be adopted to estimate losses and damages. Banihipati (2020[177]) opted for contingent valuation to estimate non-economic losses and damages in the case of drought-affected households in western India. Surprisingly, it observed that households consider immediate economic losses and damages to be more important. Another challenge is attributing non-economic losses and damages to climatic or non-climatic causes. Some of these losses, such as school dropout rates, migration, loss of identity and psychosocial stress, are generic in nature. Most often, they are not perceived by an affected entity as an effect of climatic events. For instance, scarcity of water in the cities is perceived to be due to poor land-use practices, population increase and environmental degradation rather than climatic shocks (Singh et al., 2021[178]). Similarly, households in the drought-affected regions of western India are not able to relate seasonal migration, school dropout rates to the occurrence of droughts and instead view it as a regular outcome of the economic process (Banihipati, 2020[177]). Often, non-economic losses and damages can be better described by qualitative methods (explored in the next subsection).
Indirect losses and damages
There is also a challenge to estimate losses and damages associated with indirect impact or spill over effects (e.g. a drought causing crop damage that impacts food prices, and hence consumption expenditure and nutrition). To date, relatively few studies have estimated indirect losses and damages (Ranger et al., 2010[179]). Thus, such estimates are often missing in disaster assessment reports. Yet such losses can be sizeable. During the 2006 floods in Surat, the mean losses and damages for textile units were around INR 1.98 million as per 2013 prices – the direct effects were INR 0.98 million, and the indirect effects were INR 1 million (Bahinipati et al., 2017[172]).
Qualitative data
Some key aspects of losses and damages cannot be quantified, such as sense of place, identity or security (Barnett et al., 2016[6]). Such aspects require knowledge of local socio-economic or cultural contexts and qualitative approaches to assess them, with researchers often conducting fieldwork in the affected area. Here, the data are typically collected through in-depth interviews, surveys or focus group discussions. Data emerging from such assessments are often in the form of narratives or stories. They reveal aspects of the lives that people consider to be the most important. These are as relevant for climate policy making as quantitative data (Tschakert et al., 2019[137]).
The broad variety of qualitative methods can draw out the complexities of a given policy situation, which quantitative analyses hardly achieve with certainty. In providing objective data, quantitative analyses may provide a false sense of confidence and objectivity since morality numbers and the like are associated with high levels of uncertainty (see the first subsection of 2.3.1). Qualitative data are inherently different, answering equally important questions regarding losses and damages. Thus, both quantitative and qualitative data should be considered. Indeed, the two approaches should be integrated to avoid imposing a hierarchy between methods.
Generally, qualitative data can add depth and explanation to what can be observed quantitatively, thereby decreasing uncertainty related to interpretation. For example, two different studies found a positive quantitative association between precipitation and conflicts (Witsenburg and Adano, 2009[180]; De Juan, 2015[181]). However, their interpretation of this positive association is different. One study conducts interviews with locals and concludes that raids are easier to carry out in wet weather (Witsenburg and Adano, 2009[180]). The other study argues that rains provide valuable resource (water) during droughts, thus increasing competition and conflict (De Juan, 2015[181]). While the quantitative results are similar, the qualitative analysis shows that policy implications are completely different.
Narratives and other qualitative information can also influence decision makers, sometimes more so than only numbers or figures. For example, images of a marine biologist removing a plastic straw from a sea turtle’s nostril inspired plastic straw bans around the world (Houck, 2018[182]). In the climate arena, the picture of a starving polar bear commanded more attention and sparked a larger interest than scientific studies on the same topic published in the same year (Rode et al., 2015[183]; Whiteman et al., 2015[184]).
However, any policy judgement will be inherently based on a comparison of implicit sets of values, as implementing a policy means not implementing another one with different effects. Therefore, decision makers and other stakeholders may prefer a vantage point that enables them to compare these trade-offs in policies. Often, this takes the form of figures or indices to justify the choice of one policy over another and motivate policy action.
Full quantification of the effects of climate change in monetary terms is impossible. This is due both to lack of sufficient knowledge but also the context-specific or idiosyncratic nature of some values (Tschakert et al., 2017[185]). Some social effects can be quantified but in different ways than economic damages. For example, lives lost and psychological scales of well-being [e.g. Grief Intensity Scale, PG-13 (Prigerson and Maciejewski, 2006[186])] present qualitative information in a comparable way. By considering these qualitative aspects, policy makers could have a fuller picture of the impacts of climate change and thus design more effective policies.
2.3.2. Uncertainty related to projections
Modelling future economic damages of climate change and uncertainties
How carbon emissions translate to damages has been a key question for climate economics for decades. It is a complex problem, in part, because there are different types and intensities of climate change hazards, ranging from heat waves, sea-level rise to the crossing of a climate tipping point (see Chapter 3). Additionally, the potential of damages from climate change spans different sectors of the economy. It also cascades over time, and from local to regional and global levels. Potentially, it also cascades from global to the local level; global food prices will have a local impact on availability (see Section 3.3).
Damage estimates will inevitably be uncertain, but they are still useful. The exercise allows direct comparison of the costs of not implementing climate policies – i.e. costs incurring from damages – with the costs of implementing policies that will reduce damage costs. Inquiries of this type have contributed to the wealthy body of literature on cost-benefit analysis of mitigation scenarios. For decades, these have been used to stimulate (or argue against) stringent action on climate change. There are different types of modelling tools and approaches that are used to assess this question. This subsection will explore some of these, highlighting their strengths and limitations.
IAMs are the most widely used type of model for estimating damages from climate change12 (Wei, Mi and Huang, 2015[187]). IAMs provide a representation of economic, energy, land and climate systems. In so doing, they can help illustrate and analyse the interdependencies and trade-offs between choices made in these systems. The DICE and PAGE models are among the most influential IAMs used for estimating damages costs from climate change (Nordhaus, 1992[188]) (Stern, 2006[189]) . These models allow cost estimates for avoiding climate change for different levels of temperature increase, providing a cost-benefit analysis of mitigation scenarios. Given assumptions on GDP growth, population growth policies and technology, among other factors, IAMs inform researchers and policy makers about economic and environmental outcomes, as well as energy pathways and land use. This analysis is based on simplified firm and household decisions.
The climate module of an IAM describes climate impacts, which will be translated into economic damages through a damage function. A damage function in an IAM links carbon emissions and economic damages. It aims to capture all impacts of the carbon emission in a single function.
Over the years, formulations of damage functions have improved, which has contributed to more accurate descriptions and potentially better damage estimates. In early IAMs, damage functions often relied on ad hoc assumptions without much empirical basis. For example, Nordhaus (1992[188]) specified a squared damage function that multiplied economic output. This was based on early empirical work. Conversely, Kalkuhl and Wenz (2020[190]) use the latest advances in climate econometrics with detailed subnational data to provide updated estimates of Nordhaus’ parameterisation. They find the damage caused by emission of a single tonne of CO2 is around USD 70-140, more than twice the estimate provided by Nordhaus (1992[188]).
The severe damages caused by high temperatures have been supported by other empirical works (Burke, Hsiang and Miguel, 2015[130]) (Hsiang, 2016[191]). These empirical estimates are helpful guides to reduce the uncertainties around climate damages. Comparing damage functions across different IAMs may also be helpful in setting bounds.
However, the appropriate form for the damage function is still unclear. Most IAMs, starting from Nordhaus (1992[188]), a use a multiplicative function. This multiplies damages by level of consumption, implying consumption is impaired by damages but cannot be decreased to zero. In contrast, an additive function of damages can completely counteract the welfare provided by consumption (Weitzman, 2009[74]). Additive form is more appropriate for settings where environmental goods and services are difficult to substitute (see second subsection of 2.3.2 for a more detailed discussion of the socio-economic role of nature).
Damages, as estimated by IAMs, unquestionably vary widely and are attached to high levels of uncertainties. A single function cannot capture all of the wide range of economic impacts from climate change. Some critics argue the very concept of a damage function is misleading (Pindyck, 2017[192]).
Fundamentally, the exact damage function is not knowable. Each of the three components of risks (hazards, exposure and vulnerability) are uncertain in the future. They will become still more uncertain as estimates look further into the future (Neumann et al., 2020[193]).
As another potential limitation, IAM results use coarse temporal resolution (typically time steps of a decade or more). In addition, they cannot model the transition pathway between two subsequent time steps, which adds to the level of uncertainty associated with such damage estimates (Monasterolo, Roventini and Foxon, 2019[194]). The structure of IAMs also makes it difficult to use econometric or statistical tools to assess their validity (Nordhaus, 2018[195]).
Uncertainties attached with such damage estimates and functional form assumption, therefore, need to be considered carefully. In addition, policy makers need to understand what types of questions these models can usefully inform. They must also recognise where output from these models is not fit for policy making.
Using IAMs to estimate economic costs of climate change in the near term, say, for the length of a political cycle or a mandate, will most likely produce meaningless results. Comparing different IAMs for the cost-effectiveness analysis of different mitigation pathways can, beyond high levels of uncertainties, inform international climate policy where global temperature goals can drive action in countries. If used in the appropriate manner, these models can be informative. Uncertainties associated with results are by no means a barrier to action. While the exact damages are unknowable, they will not be zero; and accumulating evidence suggests they are likely to be sizeable.
IAMs will likely underestimate the vulnerability and exposure. This occurs because certain elements are difficult to include in economic models, such as ecosystem responses, extreme events or tipping points. Thus, these are often not treated within models, leading to an underassessment of risks (Stern, 2013[196]). There are exceptions, for example IAMs with stochastic damages (Cai, Lenton and Lontzek, 2016[197]).
Economic models have still not caught up to the latest climate models. For example, they assume too long of a delay between carbon emissions and warming (Dietz et al., 2021[198]). A model is a simplified version of reality, which means some aspects will be left out or streamlined. However, in IAMs the simplifying assumptions will lead to underestimation of climate risks. Therefore, for IAM estimates, the uncertainty will be the extent to which the true effect will exceed the modelled one.
Other modelling approaches can approach uncertainty of climate change and socio-economic interactions in a more flexible manner compared to IAMs (Farmer et al., 2015[199]; Hallegatte and Rozenberg, 2017[143]). These include models that start from the subcomponents to build up their structure (bottom-up models). Two such approaches are discussed here: agent-based models and resampling and reweighting models.
Agent-based models assume multiple different agents, such as firms, households or individual consumers, who interact with each other and with their environment. Each agent has its own decision problems and behaviour rules on how to approach them. The outcomes of the model arise from the complex interplay of the different agents. The interplay can be complex. Often, multiple runs of the same model leads to different outcomes, which can be regarded as a characterisation of uncertainty.
The flexibility of agent-based models allows modellers to examine the outcomes at different levels of aggregation. They can thus compare how simulated agent interactions at the micro-level give rise to macro-level outcomes. Disentangling at different levels is useful for locating the source of uncertainties surrounding exposure and vulnerability and assessing what influences them. Thus, for policy makers, agent-based models provide a comprehensive approach to elicit the effects of policy or climate shocks, and assess the surrounding uncertainties (Kniveton, Smith and Wood, 2011[200]; Rai and Henry, 2016[201]; Hailegiorgis, Crooks and Cioffi-Revilla, 2018[202]).
Moreover, these models can provide a detailed description of different uncertainties, impacts and how they interact. Thus, compared to IAMs, for example, they are more appropriate to examine the effects of compound events, the comprehensive mechanisms and transmission channels. The examination of transmission channels is often needed to fine-tune policies.
Agent-based models, however, are limited by their behavioural assumptions, which are difficult to verify. The same behavioural rules can lead to similar outcomes at the macro level, where the verification is easier. Because of this, they often require in-depth knowledge of the modelled situation to inform the model.
These models are mostly applied to well-defined local problems (Kniveton, Smith and Wood, 2011[200]; Hailegiorgis, Crooks and Cioffi-Revilla, 2018[202]). They also provide a stylised description of a wider, complex issue, which is difficult to do with other types of approaches. For example, an agent-based model has revealed the costs of global supply chain disruptions caused by natural disasters are comparable to the disasters’ direct cost impacts (Otto et al., 2017[203]). This type of analysis enables policy makers to identify bottlenecks and make their supply chains more resilient.
The second modelling approach is to assess the impact of socio-economic effects and the uncertainty surrounding them in a resampling and reweighting model. First, the approach uses quantitative data to estimate the effects of climate change on multiple socio-economic outcomes, such as household income, labour productivity or food prices. In the second step, it simulates households’ future vulnerability and exposure using the IPCC’s Shared Socioeconomic Pathways as a basis (Hallegatte and Rozenberg, 2017[143]) (see subsection below). In the third step, the simulation is done for a world with severe and moderate climate change using the IPCC’s Representative Concertation Pathways (see Section 2.2.3).
The difference between the severe and moderate climate change scenarios gives an estimate of the effect of climate change on each outcome (e.g. household income, labour productivity, food prices). To assess the uncertainty, the second and third steps are run several thousand times, each time changing the future composition of households and climate model used. This part enables assessment of the uncertainty associated with each socio-economic outcome modelled. For example, it has been shown that the expected effect of climate change on poverty through extreme events is projected to be three times higher than the effect through labour productivity.
The productivity effect is much larger than the effects of extreme events in some scenarios (Jafino et al., 2020[131]). Therefore, an extreme weather event will likely have a larger effect than productivity. However, it is not possible to rule out that the productivity effect will overwhelmingly dominate.
Hence, resampling and reweighting models perform well in assessing uncertainties. They approach scientific and socio-economic uncertainties in a single framework. This is often important because for some regions different climate models predict completely different hazards [e.g. droughts or floods in West Africa (World Bank, 2021[204])].
The drawback of these types of models is their lack of a consistent macroeconomic framework. This makes it difficult to assess how policies could alter outcomes in the longer run. As another drawback, they can only estimate the effects of individual channels rather than compound impacts. This is important because the total effect of climate change is likely to be more than the sum of individual impacts.
Uncertainties related to the socio-economic role of nature
The way computational models treat ecosystems, and the environment more broadly, profoundly affects the estimated impacts of climate change. Climate change puts several ecosystems at risk, which in turn affects socio-economic well-being (van der Geest et al., 2019[205]). In particular, if the global mean temperature were to rise 1.5°C or less instead of 2°C, significant ecosystem damaged is avoided (IPCC, 2018[86]).
However, how changes in ecosystems respond to climate change and how such changes subsequently affect socio-economic well-being are not always clear. There are two crucial issues, which give rise to uncertainty about exposure and vulnerability: the role of relative prices with respect to environmental services; and the non-economic value of nature.
First, the role of relative prices is important because it determines the difficulty of future access to environmental goods. Computational models need to make assumptions about the production process; how various goods can be substituted in production; and consumption. Investigations have shown that estimated impacts are sensitive to assumptions about substitutability of nature with other factors, even if climate impacts are temporary (Hoel and Sterner, 2007[206]; Sterner and Persson, 2008[207]). If substitution of environmental and other goods is not perfect, environmental services, such as access to clean water, will get more expensive relative to other goods such as mobile phones.
Estimated costs quickly rise with the difficulty of substitution but are severe even with modest assumptions. For example, Sterner and Persson (2008[207]) assume that the environmental and other goods are substitutable but not perfectly13 in a simple DICE model. They find the world needs to be carbon neutral optimally before 2100. This finding contrasts with the original DICE model, which prescribes that carbon emissions should peak around the same time. Accordingly, economic damages are likely to be underestimated within most IAMs, which assume that environmental and other goods are perfectly substitutable.
Given these results, policy makers should carefully examine the use of environmental goods in the economy and if such goods can be substituted. Potential investments could decrease vulnerability by making it easier to substitute some environmental goods. For example, access to clean drinking water could be a serious problem to poorer households, due to rising water prices. Thus, investments in water infrastructure might alleviate uncertainties connected to relative prices and make populations more resilient. Such infrastructure could include wastewater treatment plants or desalination plants and processes. Other environmental goods and services might be more difficult to substitute, such as carbon sequestration from the Amazon rainforest.
The second important source of uncertainty is the non-economic value of ecosystems and environmental goods. As mentioned in Section 2.3.1, the most important impacts will likely be non-economic in nature. Many argue against putting a monetary value on ecosystems on principle (Costanza et al., 2017[208]; Des Jardins, 2013[209]). However, the needs of policy makers to conduct cost-benefit analyses of environmental policies prompted economists to devise methods of such valuation (Perman et al., 2011[210]). Thus, these impacts are essentially qualitative impacts, approximated with quantitative methods.
Several research papers have shown that ecosystems or even single species carry value to people who might not use or even see them (Bateman et al., 2002[211]; Fankhauser, Dietz and Gradwell, 2014[212]). For example, the average willingness-to-pay for the preservation of threatened species is around USD 400 per household, according to a recent review (Subroy et al., 2019[213]). However, these appraisals are quite uncertain and the results are sensitive to the choice of methodologies (Bastien-Olvera and Moore, 2020[133]).
Recent research finds that estimated non-economic impacts are about four times higher than economic impacts. For policy makers, this implies high uncertainty regarding how non-economic costs will affect the population. As the ultimate goal of policies is usually some form of welfare, policy makers must consider non-economic damages in their appraisals.
Conducting appraisals of non-economic damages using established methods (e.g. contingent valuation, choice experiments) would decrease uncertainties. In so doing, they would help tailor policies to population needs. An area might be economically resilient to certain types of hazards. However, it could be vulnerable to non-economic impacts such as forest dieback or species extinction. Therefore, investments should go beyond protecting ecosystems that contribute directly to economic production (e.g.agriculture, tourism, fishery): they should also protect ecosystems with no direct economic value. This point relates back to the qualitative impacts discussed in Section 2.3.1.
Shared Socioeconomic Pathways as a measure of uncertainty
As discussed in Section 2.2.3, IPCC has created a set of scenarios for socio-economic systems (similarly to RCPs). These will underlie some of the discussion in the forthcoming Sixth Assessment Report. The SSPs describe five consistent narratives of future developments in the world (Riahi et al., 2017[8]). The SSPs are not predictions, and do not aspire to describe the future. Rather, they provide consistent scenarios based on assumptions of trends.
In the same vein, since the pathways are not forecasts based on the current state, there are no probabilities attached to them. The future might not resemble the past or the present. SSPs explore a range of different futures to assess what these plausible worlds could mean for natural and human systems.
The SSPs were built by groups of experts by first creating five general storylines about the ways in which the world can develop (Riahi et al., 2017[8]). Then, to guide the interpretation and assumptions, the storylines were translated to quantitative input tables. Based on the input tables, the narratives were converted to quantitative projections about three socio-economic drivers: GDP, population and urbanisation. Each of these is difficult to project, especially in the longer term.
Figure 2.10. Main outcomes of the Shared Socioeconomic Pathways

Note: The figure shows the (baseline marker) projections of population, GDP, urbanisation and GDP per capita.
Source: (Riahi et al., 2017[8]); Population: (Samir and Lutz, 2017[214]); GDP: (Dellink et al., 2017[215]); Urbanisation: (Jiang and O’Neill, 2017[216]).
The five pathways describe quite different worlds. Even within individual pathways, different models yield different outcomes, an indication of considerable uncertainty. While the exact projections of the five scenarios are uncertain, general trends are clear – see Figure 2.10 for projections principally used by the IPCC in discussions (baseline marker projections):
SSP1 (green) depicts a sustainable development pathway. It is a relatively optimistic scenario, where socio-economic systems (such as infrastructures and lifestyles) undergo a rapid shift to a less carbon-intensive operation, enabled by technology (see Chapter 6). This decreases the hazards and exposures considerably. Thus, GDP per capita and urbanisation are among the highest, and population growth is among the lowest of the SSPs (van Vuuren et al., 2017[217]).
SSP2 (brown) illustrates a world in which historical trends continue. Hazards and exposure increase gradually but are somewhat offset by more adaptation options, which decreases vulnerabilities slowly. This pathway is in the middle, compared to other SSPs, in terms of socio-economic outcomes (Fricko et al., 2017[218]).
SSP3 (grey) point to considerable increases in both exposure and vulnerabilities. This pathway is the worst overall in terms of global socio-economic outcomes; GDP levels are the lowest, population is the highest (Fujimori et al., 2017[219]).
SSP4 (red) describes a world with increasing hazards and exposure. It has decreasing vulnerabilities but only for developed countries. This worsens inequalities across and within countries, increasing poverty and generating political and social tensions. In this world, environmental problems are fundamentally local. Accordingly, GDP and GDP per capita is in the lower half of the SSPs (Calvin et al., 2017[220]).
SSP5 (purple), labelled “fossil-fuelled development”, has the highest GDP and GDP per capita, and optimistic projections for high population growth and urbanisation. In this pathway, rapid development takes place, which relies on the continuing exploitation of natural resources. Such rapid development could lead to decreased vulnerability in some places. However, it would uphold high-carbon status quo and necessarily lead to more impacts compared to pathways that encompass a transition towards low-carbon economies (Kriegler et al., 2017[221]).
Uncertainty also pervades each individual SSP. Recall that Figure 2.10 only depicts the baseline marker scenarios and that different modelling groups develop the scenarios in different ways. This includes in relation to the different mitigation scenarios used in physical climate models (see Section 2.2).
Accordingly, except for SSP3, all of the pathways could achieve warming below 1.5°C (compared to pre-industrial levels) (IPCC, 2018[86]). Figure 2.11 shows the net carbon emissions (emissions minus removal) associated with the SSPs, based on several models (Riahi et al., 2017[8]). Green lines show pathways where the 1.5°C target is achieved; blue lines show other pathways; and dark blue shows the baseline marker models.
The nature of uncertainties and risks is different for each. For example, achieving the 1.5°C target carries fewer risks in SSP1 than in SSP5. In SSP1, emissions decline swiftly slowing the warming, whereas they initially continue at the current levels or increase in SSP5. For SSP5 the target is achieved if sufficient carbon can be removed from the atmosphere (net carbon emissions are deeply negative).
Since the far future is more uncertain than the near future, edging towards a pathway that relies on technology in the far future is more uncertain and riskier. For policy makers, such a path implies that risks can be decreased by acting sooner rather than later. It also implies they favour policies that bring the world closer to one of the less risky worlds. The SSPs provide a common grounding for policy makers for discussions of socio-economic aspects and surrounding issues, which provides more clarity to these debates.
Figure 2.11. Carbon emissions in Shared Socioeconomic Pathways

Note: The graph shows the net carbon emissions (Mt) in each year, according to the various simulations for each SSP scenario (Riahi et al., 2017[8]). Dark blue shows the baseline; green lines show the pathways compatible with 1.5°C warming; and light blue lines show other pathways.
Source: IIASA SSP Public Database (Riahi et al., 2017[8]).
2.3.3. Other uncertainties
Uncertainty arises from the complex nature of socio-economic systems. Thus, projections describe a possible future development. However, they cannot and will not be realised simply because of unforeseen random shocks. Projections cannot account or assign probabilities for all random shocks. This is equally true whether they are trade shocks (from the global economic systems) or exceptional circumstances (such as the COVID-19 pandemic). Thus, projections should be carefully considered but not taken as predictions. Resilience should be built to random shocks, for example, by diversifying supply chains.
An extreme case of uncertain shocks occurs when such shocks lead to impacts out of proportion with their origin (non-linear), especially socio-economic tipping points. Socio-economic tipping points are thresholds in socio-economic systems, which lead to abrupt changes and upset whole systems, leading to new equilibria.14
Socio-economic tipping points are discussed separately from projections because they are so difficult or impossible to project but have immense consequences. A projection may predict general trends perfectly, but a random shock from the world economy might trigger a socio-economic tipping point that could shift them.
Knowledge of when and how tipping points will be crossed is unknown. However, policy makers can encourage their tipping through comprehensive policies. For example, they could invest in renewable technologies but also remove fossil fuel subsidies (Otto et al., 2020[222]). According to experimental evidence, a roughly 25% committed minority in a group can tip the group majority to a different behaviour (Centola et al., 2018[223]).
Importantly, socio-economic tipping points can be tipped both ways. On the one hand, ground-breaking research in 1973 revealed ozone depletion in the atmosphere. This led to signing the Montreal Protocol only 14 years later, despite objections from affected industries. On the other, a new fuel tax in France in 2019 led to resistance and overturning of a policy.
Tipping points remain unknowable, even if it is possible to broadly categorise them. David Tábara et al. (2018[224]), for example, identify six types of tipping points relating to different parts of the socio-economic system. These are energy systems, governance, socio-cultural, technological systems, resource systems and economy. The number of tipping points increases with the complexity of societies. This means that multiple tipping points will likely need to be tipped for transformation (Otto et al., 2020[222]).
The social discount rate provides another uncertainty for policy design. The social discount rate determines the weight of future – compared to the present – in cost-benefit assessments. If future generations are just as important as current ones, the social discount rate is zero. If the next year counts for half as much as this year, the social discount rate is around 30%. As it increases, the well-being of future people carries less weight in these assessments. Social discount rates received special attention in the climate policy arena because actions taken today influence well-being in the coming decades or even centuries. Despite the scholarly attention, no clear consensus has been reached (Kelleher, 2017[225]; Varian, 2006[226]). Thus, the social discount rate is uncertain and it is likely to be different in different societies. However, research has provided a framework to consider social discount rates in a consistent way. For most, the social discount rate has two components: preference for the present and the inequality aversion across generations (Kolstad et al., 2015[227]).
First, preference for the present means that a given monetary is worth more today than it will be next year. Ultimately, this preference can be derived from forgone gains: a sum of money can be invested today, which will yield gains next year. This means the sum of money today is worth more than the same sum next year. This only holds for goods traded on the market. It does not hold for non-market goods, such as lives or livelihoods. It is unclear if ten lives lost next year are preferable to ten lives lost today.
Second, inequality aversion across generations refers to the expectation that economic growth will continue and people in the future are likely to be wealthier. Consequently, they can bear the economic costs more easily. This expectation is debatable. It is unclear whether the economy will continue to grow indefinitely and by how much is uncertain (Dellink et al., 2017[215]).
This uncertainty increases further out in the future. This implies the social discount rate will decline over time for two reasons. First, governments are risk-averse (see Chapter 1) and would prefer not to make overly strong assumptions about future wealth. This also relates to governments’ general aversion of creating economic inequalities across generations; a high discount rate implies that future generations will bear the brunt of costs (Gollier, 2015[228]). It also relates to sustainability concerns that point to the possibility of future generations being worse off than current ones (Asheim and Mitra, 2010[229]). These considerations are ultimately political. Second, the mathematics of the expected value shows that discount rates need to be conservative under uncertainty (Gollier, 2002[230]).15 Thus, without any value judgement, the discount rates should be declining as uncertainty increases.Uncertainty is likely to increase as projections go further into the future. Of course, this lesson also translates across countries: policy makers in countries facing more uncertainty should use lower discount rates than those in less uncertain countries.
2.4. The communication of climate-related risk and implications for policy processes
Sections 2.2 and 2.3 explore the high level of confidence in knowledge of climate change. At the same time, they highlight different levels and types of uncertainties in the understanding of certain features of the climate system. They also explore implications for the understanding of climate-related risk of losses and damages.
There are long-standing challenges in communicating climate change. In the face of climate scepticism, disputes over the integrity of climate scientists and heated debate on appropriate policy responses, the IPCC has always emphasised the degree of confidence associated with its statements. Its landmark Fourth Assessment Report (AR4) in 2007 concluded that warming of the climate system was unequivocal (IPCC, 2007[231]). Subsequent reviews have only strengthened levels of confidence around the reality of climate change and that it is driven by human activity.
Uncertainties underlying the risks of climate change have decreased in the past decades. This is evidenced by the shift towards higher confidence levels in IPCC Assessment reports’ Summaries for Policy makers (Molina and Abadal, 2021[232]), for example. However, uncertainties remain an important factor in how the risk is perceived and in how action on climate change is taken as a consequence. Indeed, public acceptance (e.g. of climate scientists’ predictions of sea-level rise) is influenced by how uncertainty is communicated and also to what extent. When scientists acknowledge the full extent of inevitable uncertainty, the public’s level of trust in those predictions decreases (Howe et al., 2019[233]).
The profound understanding today of climate change is owed to years of climate research and advances in knowledge of the Earth’s climate system. Hence, communication of climate change knowledge is subject to the practice of scientific communication. As such, it often involves efforts to be comprehensive, balanced and conservative. This may have the unintended consequence of eroding trust in the message among non-experts (Ho and Budescu, 2019[234]).
The predictability of the climate system especially at finer spatial and temporal scales remains limited, due to, among other factors, inherently variable and complex chaotic dynamics (Box 2.4). Climate projections can be improved with scientific advances. However, they will always be subject to types and levels of uncertainty. Further, their accurate communication will inevitably involve the consideration of these uncertainties.
As a summary of recent cutting-edge research, the IPCC assessment focuses on the most recent (and therefore most uncertain) results and on future research needed to tackle the remaining unknowns. The IPCC assessment does not spend thousands of pages summarising the extremely high confidence of scientists in the greenhouse effect. By definition, it is tasked to consider the more uncertain aspects of change. If uncertainty by itself is perceived as a reason for doubt, then this could lead to a mistrust of the whole message.
Issues around trust could suggest the climate change problem is facing an unsolvable dilemma: where there is uncertainty, there is little trust, and where there is little trust, there is little action. Other scientific communities have faced such problems in the past (Oreskes and Conway, 2010[235]). The climate change challenge, while informed by science, is not a purely scientific problem per se. Indeed, it is influenced by a series of other factors, such as vulnerability and exposure. Such factors fall outside the pure climate science domain. As such, they require action and answers that go beyond that which can be informed by science. Indeed, uncertainties associated with such factors may be far greater than uncertainties in physical climate projections.
This challenges the notion that more accurate scientific predictions of climate change impacts are a requirement for action on climate. Climate science informs policy makers on potential ways physical climate change can unfold for different parts of the world. It does this even if individual models do not always agree on the direction of important changes in a specific region. Yet solutions for climate change transcend the advances of scientific knowledge, however extremely valuable these are.
Indeed, the exact predictions of when and where hazards will occur is not a requirement in other areas of risk management. Governments do not expect to know when an individual or a group of people will be infected by a pathogen before they enact measures to counteract pandemics. Nor is it even necessary to assess probability to be high: one may buy fire insurance for a house even though the probability of fire is small.
The same rationale is applicable to the climate challenge. There is certainty that climate change is happening and good knowledge about how and what types of hazards can occur in different regions and over different timeframes. In other words, despite uncertainties, current knowledge of climate change is sufficient to support implementation of many risk management measures. Of course, as in the other cases, the availability of more detailed information would be highly valuable.
Since the likely damages increase disproportionately as one moves from the mean of potential responses, larger uncertainties can lead to a larger range of potential climate risk that are to be taken into account through management approaches. From a risk management perspective, when dealing with uncertainties, one needs to balance the possibilities and consequences of overestimating or underestimating the risk. In other words, one needs to minimise both false alarms and missed warnings (Shepherd, 2019[11]).
Finding a balance between these two extremes is not trivial. There is no single probabilistic threshold that can guarantee a risk is not being over- or underestimated, for example. Multiple factors, including value judgements and risk tolerance, can influence this threshold.
The IPCC terminology of likelihoods (Table 2.5) embeds an assessment of what probability levels mean in terms of certainty about an outcome. These levels have direct consequences for policy making. They imply different levels of “call for action” (Molina and Abadal, 2021[232]) and can be considered more or less important in risk management strategies.
However, likelihoods are not the same as risk. Since climate change is essentially a risk management problem, policy makers need to consider these likelihoods carefully and critically. Indeed, Sutton (2019[236]) argues that climate science must “take the needs of risk assessment much more seriously”.
Table 2.5. IPCC likelihood terminology
Likelihood terminology |
Likelihood of the occurrence/outcome |
---|---|
Virtually certain |
99-100% probability |
Extremely likely |
95-100% probability |
Very likely |
90-100% probability |
Likely |
66-100% probability |
More likely than not |
>50-100% probability |
About as likely as not |
33-66% probability |
Unlikely |
0-33% probability |
Very unlikely |
0-10% probability |
Extremely unlikely |
0-5% probability |
Exceptionally unlikely |
0-1% probability |
Source: Box TS.1 in (Stocker et al., 2013[79]).
In working and considering the IPCC likelihoods in a risk management strategy, one could reasonably assume the projection of impacts for which probabilities are high. In other words, probabilities backed up by consistent, multiple and independent lines of high-quality evidence will be considered. In this case, terms such as “virtually certain”, “extremely unlikely” or “very likely” will be usable directly as meaningful probabilities in a risk assessment.
As likelihoods in the projections decrease, inclusions in the risk assessment will depend on the relevant magnitude of the impact in question. For example, the Atlantic Meridional Overturning Circulation has an up to 10% probability of collapsing during the 21st century (IPCC, 2019[237]), with potentially impactful consequences. In this case, probability may be high enough for it to be considered in risk assessments. Conversely, a 50% probability of less rainfall in a deserted uninhabited area may be regarded as negligible, considering the lower potential for losses and damages.
Terminologies assigned to the middle and lower range of probabilities, therefore, need to be considered with care and are not always presented in a systematic manner. Given any probability distribution, one could frame a result with any degree of likelihood. A 1% chance of temperature above 40 degrees, a 10% chance of temperature above 35 degrees, a 50% chance of temperature above 30 degrees, and a 99% chance of temperature about 20 degrees may all refer to the same forecast in the same place on the same day. There is a subjective element to the presentation of likelihoods and the choice of what variables to communicate.
In many other areas of life and of decision making, a significantly more prudent approach is taken against the same odds the world faces with climate change. For example, if an aircraft had an up to 33% probability of not arriving at its destination, this would not be considered an unlikely outcome. Climate pathways that take carbon budgets with only a 66% chance of meeting the Paris “target” betray a loose interpretation of the word “target”. This deserves greater scrutiny by policy makers as to whether that is truly the intended meaning.
Similarly, even though the probability of fire in an individual private home may be lower than 1%, the potential damage of such an event is high. Therefore, most people purchase insurance to protect their homes. The same rationale applies to government and societal decisions and action to address climate change. Uncertainty will always be present; decisions must be taken regardless.
Paradoxically, these uncertainties and ambiguities are the very aspects of climate change that are critical to understand better. More understanding can forge effective responses to climate risks and reduce sceptical reactions to the compelling accumulation of scientific evidence (Pidgeon, 2012[238]; Poortvliet et al., 2020[239]). Therefore, greater recognition is needed of both the strengths and limitations of current physical climate models.
Collectively, both the planet and human societies are driven to conditions never before observed in human history. There is also need for approaches to policy and decision making in relation to adaptation that can cope with inevitable uncertainties. These uncertainties arise from the dynamics of the physical climate system closely interacting with complex and equally dynamic social and economic systems. The uncertainties and ambiguities surrounding some aspects of climate change projections should also amplify – not weaken – the case for strong climate mitigation action, undoubtedly the safest and most effective way to reduce the risks of losses and damages.
References
[72] Ackerman, F., E. Stanton and R. Bueno (2010), “Fat tails, exponents, extreme uncertainty: Simulating catastrophe in DICE”, Ecological Economics, Vol. 69/8, pp. 1657-1665, http://dx.doi.org/10.1016/j.ecolecon.2010.03.013.
[67] Ad Hoc Study Group on Carbon Dioxide and Climate et al. (1979), Carbon Dioxide and Climate, National Academies Press, Washington, DC, http://dx.doi.org/10.17226/12181.
[7] Adger, W. et al. (2012), “Cultural dimensions of climate change impacts and adaptation”, Nature Climate Change, Vol. 3/2, pp. 112-117, http://dx.doi.org/10.1038/nclimate1666.
[92] Álvarez, I. (ed.) (2016), “Locally downscaled and spatially customizable climate data for historical and future periods for North America”, PLOS ONE, Vol. 11/6, p. e0156720, http://dx.doi.org/10.1371/journal.pone.0156720.
[107] Andrews, O. et al. (2018), “Implications for workability and survivability in populations exposed to extreme heat under climate change: A modelling study”, The Lancet Planetary Health, Vol. 2/12, pp. e540-e547, http://dx.doi.org/10.1016/s2542-5196(18)30240-7.
[166] Archibald, C. and N. Butt (2018), “Using Google search data to inform global climate change adaptation policy”, Climatic Change, Vol. 150/3-4, pp. 447-456, http://dx.doi.org/10.1007/s10584-018-2289-9.
[229] Asheim, G. and T. Mitra (2010), “Sustainability and discounted utilitarianism in models of economic growth”, Mathematical Social Sciences, Vol. 59/2, pp. 148-169, http://dx.doi.org/10.1016/j.mathsocsci.2009.08.003.
[172] Bahinipati, C. et al. (2017), “Flood-induced loss and damage to textile industry in Surat City, India”, Environment and Urbanization ASIA, Vol. 8/2, pp. 170-187, http://dx.doi.org/10.1177/0975425317714903.
[177] Banihipati, C. (2020), “Assessing the costs of droughts in rural India: A comparison of economic and non-economic loss and damage”, Current Science, Vol. 118/11, pp. 1832-1841.
[6] Barnett, J. et al. (2016), “A science of loss”, Nature Climate Change, Vol. 6/11, pp. 976-978, http://dx.doi.org/10.1038/nclimate3140.
[133] Bastien-Olvera, B. and F. Moore (2020), “Use and non-use value of nature and the social cost of carbon”, Nature Sustainability, Vol. 4/2, pp. 101-108, http://dx.doi.org/10.1038/s41893-020-00615-0.
[211] Bateman, I. et al. (2002), Economic Valuation with Stated Preference Techniques, Edward Elgar Publishing, Cheltenham, UK, https://ideas.repec.org/b/elg/eebook/2639.html (accessed on 6 January 2021).
[61] Benson, C. and E. Clay (1998), “The impact of drought on sub-saharan African economies”, World Bank Technical Papers, World Bank, Washington, DC, https://elibrary.worldbank.org/doi/abs/10.1596/0-8213-4180-4.
[126] Bhave, A. et al. (2018), “Water Resource Planning Under Future Climate and Socioeconomic Uncertainty in the Cauvery River Basin in Karnataka, India”, Water Resources Research, Vol. 54/2, pp. 708-728, http://dx.doi.org/10.1002/2017wr020970.
[146] Blair, J., R. Czaja and E. Blair (2014), Designing Surveys: A Guide to Decisions and Procedures, SAGE Publications, Thousand Oaks, CA.
[115] Bony, S. et al. (2015), “Clouds, circulation and climate sensitivity”, Nature Geoscience, Vol. 8/4, pp. 261-268, http://dx.doi.org/10.1038/ngeo2398.
[42] Borchers Arriagada, N. et al. (2020), “Unprecedented smoke‐related health burden associated with the 2019-20 bushfires in eastern Australia”, Medical Journal of Australia, Vol. 213/6, pp. 282-283, http://dx.doi.org/10.5694/mja2.50545.
[13] Braconnot, P. et al. (2012), “Evaluation of climate models using palaeoclimatic data”, Nature Climate Change, Vol. 2/6, pp. 417-424, http://dx.doi.org/10.1038/nclimate1456.
[167] Bromley-Trujillo, R. and J. Poe (2020), “The importance of salience: Public opinion and state policy action on climate change”, Journal of Public Policy, Vol. 40/2, pp. 280-304, http://dx.doi.org/10.1017/S0143814X18000375.
[27] Brönnimann, S. and J. Wintzer (2018), “Climate data empathy”, Wiley Interdisciplinary Reviews: Climate Change, Vol. 10/2, http://dx.doi.org/10.1002/wcc.559.
[142] Brouwer, R. et al. (2007), “Socioeconomic vulnerability and adaptation to environmental risk: A case study of climate change and flooding in Bangladesh”, Risk Analysis, Vol. 27/2, pp. 313-326, http://dx.doi.org/10.1111/j.1539-6924.2007.00884.x.
[148] Brown, L. et al. (2014), “The past, present, and future of federal surveys: Observations from the Committee on National Statistics”, Conference Proceedings from JSM (Joint Statistical Meeting) 2014 - Social Statistics Section), Committee on National Statistics, Washington, DC.
[40] Brown, L. and V. Murray (2013), “Examining the relationship between infectious diseases and flooding in Europe”, Disaster Health, Vol. 1/2, pp. 117-127, http://dx.doi.org/10.4161/dish.25216.
[153] Brown, M., K. de Beurs and M. Marshall (2012), “Global phenological response to climate change in crop areas using satellite remote sensing of vegetation, humidity and temperature over 26 years”, Remote Sensing of Environment, Vol. 126, pp. 174-183, http://dx.doi.org/10.1016/j.rse.2012.08.009.
[130] Burke, M., S. Hsiang and E. Miguel (2015), “Global non-linear effect of temperature on economic production”, Nature, Vol. 527/7577, pp. 235-239, http://dx.doi.org/10.1038/nature15725.
[197] Cai, Y., T. Lenton and T. Lontzek (2016), “Risk of multiple interacting tipping points should encourage rapid CO2 emission reduction”, Nature Climate Change, Vol. 6/5, pp. 520-525, http://dx.doi.org/10.1038/nclimate2964.
[220] Calvin, K. et al. (2017), “The SSP4: A world of deepening inequality”, Global Environmental Change, Vol. 42, pp. 284-296, http://dx.doi.org/10.1016/j.gloenvcha.2016.06.010.
[82] CAT (2021), Warming Projections Global Update, Climate Action Tracker.
[223] Centola, D. et al. (2018), “Experimental evidence for tipping points in social convention”, Science, Vol. 360/6393, pp. 1116-1119, http://dx.doi.org/10.1126/science.aas8827.
[154] Ceola, S., F. Laio and A. Montanari (2014), “Satellite nighttime lights reveal increasing human exposure to floods worldwide”, Geophysical Research Letters, Vol. 41/20, pp. 7184-7190, http://dx.doi.org/10.1002/2014GL061859.
[152] Chen, C. et al. (2015), “University of Notre Dame Global Adaptation Index”, Technical Report, https://gain.nd.edu/assets/254377/nd_gain_technical_document_2015.pdf.
[62] Clay, E. and O. Stokke (2000), Food Aid and Human Security, Frank Cass, Portland, OR.
[75] Collins, M. et al. (2013), “Long-term climate change: Projections, commitments and irreversibility”, in Stocker, T. et al. (eds.), Climate Change 2013: The Physical Science Basis. Contribution of Working Group I to the Fifth Assessment Report, Intergovernmental Panel on Climate Change, Geneva.
[127] Congressional Budget Office (2005), “Uncertainty in analyzing climate change: Policy implications”, A CBO Paper, Congressional Budget Office, Washington, DC.
[70] CONSTRAIN (2020), A New Generation of Climate Models, COVID-19 and the Paris Agreement, ZERO IN ON Reports, The CONSTRAIN Project.
[208] Costanza, R. et al. (2017), “Twenty years of ecosystem services: How far have we come and how far do we still need to go?”, Ecosystem Services, Vol. 28, pp. 1-16, http://dx.doi.org/10.1016/J.ECOSER.2017.09.008.
[112] Council, N. (2001), Climate Change Science: An Analysis of Some Key Questions, National Academies Press, Washington, DC, http://dx.doi.org/10.17226/10139.
[224] David Tàbara, J. et al. (2018), Positive Tipping Points in a Rapidly Warming World, Elsevier B.V., http://dx.doi.org/10.1016/j.cosust.2018.01.012.
[181] De Juan, A. (2015), “Long-term environmental change and geographical patterns of violence in Darfur, 2003-2005”, Political Geography, Vol. 45, pp. 22-33, http://dx.doi.org/10.1016/J.POLGEO.2014.09.001.
[24] Dee, D. et al. (2011), “The ERA-Interim reanalysis: Configuration and performance of the data assimilation system”, Quarterly Journal of the Royal Meteorological Society, Vol. 137/656, pp. 553-597, http://dx.doi.org/10.1002/qj.828.
[215] Dellink, R. et al. (2017), “Long-term economic growth projections in the Shared Socioeconomic Pathways”, Global Environmental Change, Vol. 42, pp. 200-214, http://dx.doi.org/10.1016/j.gloenvcha.2015.06.004.
[209] Des Jardins, J. (2013), Environmental Ethics: An Introduction to Environmental Philosophy (Fifth Edition), Cengage Learning, Belmont, CA, https://digitalcommons.csbsju.edu/academic_books/5 (accessed on 6 January 2021).
[144] Deschênes, O., M. Greenstone and J. Guryan (2009), “Climate change and birth weight”, American Economic Review, Vol. 99/2, pp. 211-217, http://dx.doi.org/10.1257/aer.99.2.211.
[124] Dessai, S. et al. (2018), “Building narratives to characterise uncertainty in regional climate change through expert elicitation”, Environmental Research Letters, Vol. 13/7, p. 074005, http://dx.doi.org/10.1088/1748-9326/aabcdd.
[198] Dietz, S. et al. (2021), “Are economists getting climate dynamics right and does it matter?”, Journal of the Association of Environmental and Resource Economists, Vol. 8/5, pp. 895-921, http://dx.doi.org/10.1086/713977.
[93] Donatti, C. et al. (2016), “What information do policy makers need to develop climate adaptation plans for smallholder farmers? The case of Central America and Mexico”, Climatic Change, Vol. 141/1, pp. 107-121, http://dx.doi.org/10.1007/s10584-016-1787-x.
[110] Dunstone, N. et al. (2020), “Skilful interannual climate prediction from two large initialised model ensembles”, Environmental Research Letters, Vol. 15/9, p. 094083, http://dx.doi.org/10.1088/1748-9326/ab9f7d.
[52] Ebi, K. et al. (2021), “Burning embers: Synthesis of the health risks of climate change”, Environmental Research Letters, Vol. 16/4, p. 044042, http://dx.doi.org/10.1088/1748-9326/abeadd.
[150] Eckstein, D., V. Künzel and L. Schäfer (2021), Global Climate Risk Index 2021, Germanwatch, Bonn, https://germanwatch.org/sites/default/files/Global%20Climate%20Risk%20Index%202021_2.pdf.
[65] Edwards, B., M. Gray and B. Hunter (2019), “The social and economic impacts of drought”, Australian Journal of Social Issues, Vol. 54/1, pp. 22-31, https://doi.org/10.1002/ajs4.52.
[163] EEA (2008), “European scale exceedance mapping for PM10 and ozone based on daily interpolation fields”, ETC/ACC Technical Paper, No. 2007/08, European Environmental Agency, Copenhagen.
[38] English National Study of Flooding and Health Study Group (2019), “Impact of repeat flooding on mental health and health-related quality of life: A cross-sectional analysis of the English National Study of Flooding and Health”, BMJ Open, Vol. 9/11, p. e031562, http://dx.doi.org/10.1136/bmjopen-2019-031562.
[102] Eyring, V. et al. (2016), “Overview of the Coupled Model Intercomparison Project Phase 6 (CMIP6) experimental design and organization”, Geoscientific Model Development, Vol. 9/5, pp. 1937-1958, http://dx.doi.org/10.5194/gmd-9-1937-2016.
[103] Eyring, V. et al. (2019), “Taking climate model evaluation to the next level”, Nature Climate Change, Vol. 9/2, pp. 102-110, http://dx.doi.org/10.1038/s41558-018-0355-y.
[212] Fankhauser, S., S. Dietz and P. Gradwell (2014), “Non-economic losses in the context of the UNFCCC work programme on loss and damage”, Technical Paper, No. FCCC/TP/2013/2, United Nations Framework Convention on Climate Change, Geneva.
[199] Farmer, J. et al. (2015), “A third wave in the economics of climate change”, Environmental and Resource Economics, Vol. 62/2, pp. 329-357, http://dx.doi.org/10.1007/s10640-015-9965-2.
[95] Finn, D. (2020), How do we build collaborative science for better urban planning and climate change adaptation?, American Geophysical Union, Washington, DC.
[12] Flato, G. (2013), “Evaluation of climate models”, in Stocker, T. (ed.), Climate Change 2013: The Physical Science Basis. Contribution of Working Group I to the Fifth Assessment Report of the Intergovernmental Panel on Climate Change, Cambridge University Press, Cambridge, United Kingdom and New York, NY.
[53] Fouillet, A. et al. (2008), “Has the impact of heat waves on mortality changed in France since the European heat wave of summer 2003? A study of the 2006 heat wave”, International Journal of Epidemiology, Vol. 37/2, pp. 309-317, http://dx.doi.org/10.1093/ije/dym253.
[218] Fricko, O. et al. (2017), “The marker quantification of the Shared Socioeconomic Pathway 2: A middle-of-the-road scenario for the 21st century”, Global Environmental Change, Vol. 42, pp. 251-267, http://dx.doi.org/10.1016/j.gloenvcha.2016.06.004.
[219] Fujimori, S. et al. (2017), “SSP3: AIM implementation of Shared Socioeconomic Pathways”, Global Environmental Change, Vol. 42, pp. 268-283, http://dx.doi.org/10.1016/j.gloenvcha.2016.06.009.
[83] Gambhir, A. (2019), “Planning a Low-Carbon Energy Transition: What Can and Can’t the Models Tell Us?”, Joule, Vol. 3/8, pp. 1795-1798, http://dx.doi.org/10.1016/j.joule.2019.07.016.
[51] Gasparrini, A. et al. (2015), “Mortality risk attributable to high and low ambient temperature: A multicountry observational study”, The Lancet, Vol. 386/9991, pp. 369-375, http://dx.doi.org/10.1016/s0140-6736(14)62114-0.
[134] Gasparrini, A. et al. (2017), “Projections of temperature-related excess mortality under climate change scenarios”, The Lancet Planetary Health, Vol. 1/9, pp. e360-e367, http://dx.doi.org/10.1016/s2542-5196(17)30156-0.
[20] GCOS (2016), The Global Observing System for Climate: Implementation Needs, GCOS, https://library.wmo.int/doc_num.php?explnum_id=3417 (accessed on 27 May 2021).
[84] Gidden, M. et al. (2019), “Global emissions pathways under different socioeconomic scenarios for use in CMIP6: A dataset of harmonized emissions trajectories through the end of the century”, Geoscientific Model Development, Vol. 12/4, pp. 1443-1475, http://dx.doi.org/10.5194/gmd-12-1443-2019.
[128] Gillingham, K. et al. (2015), Modeling Uncertainty in Climate Change: A Multi-Model Comparison, National Bureau of Economic Research, Cambridge, MA, http://dx.doi.org/10.3386/w21637.
[94] Giuliani, G. et al. (2017), “Spatially enabling the Global Framework for Climate Services: Reviewing geospatial solutions to efficiently share and integrate climate data & information”, Climate Services, Vol. 8, pp. 44-58, http://dx.doi.org/10.1016/j.cliser.2017.08.003.
[228] Gollier, C. (2015), “Discounting, inequality and economic convergence”, Journal of Environmental Economics and Management, Vol. 69, pp. 53-61, http://dx.doi.org/10.1016/j.jeem.2014.10.005.
[230] Gollier, C. (2002), “Discounting an uncertain future”, Journal of Public Economics, Vol. 85/2, pp. 149-166, http://dx.doi.org/10.1016/S0047-2727(01)00079-2.
[14] Goody, R. et al. (2002), “Why monitor the climate?”, Bulletin of the American Meteorological Society, pp. 873-878, https://journals.ametsoc.org/view/journals/bams/83/6/1520-0477_2002_083_0873_wwsmtc_2_3_co_2.xml (accessed on 27 May 2021).
[60] Government of British Columbia (2021), “Chief coroner’s updated statement on public safety during heat wave”, 2 July, Statement, Public Safety and Solictor General, Government of British Columbia, Victoria, https://news.gov.bc.ca/releases/2021PSSG0062-001295.
[169] Government of India (2011), Disaster Management in India, Ministry of Home Affairs, New Delhi.
[157] Government of the Commonwealth of Dominica (2017), PDNA: Post-Disaster Needs Assessment Hurricane Maria September 18, 2017, Government of the Commonwealth of Dominica.
[4] Granderson, A. (2014), “Making sense of climate change risks and responses at the community level: A cultural-political lens”, Climate Risk Management, Vol. 3, pp. 55-64, http://dx.doi.org/10.1016/j.crm.2014.05.003.
[45] Guha-Sapir, D., D. Hargitt and P. Hoyois (2004), Thirty Years of Natural Disasters 1974-2003: The Numbers, Centre for Research on the Epidemiology of Disasters, Brussels.
[46] Guha-Sapir, D., P. Hoyois and R. Below (2016), Annual Disaster Statistical Review 2015: The Numbers and Trends, Centre for Research on the Epidemiology of Disasters, Brussels.
[15] Guo, H., L. Zhang and L. Zhu (2015), “Earth observation big data for climate change research”, Advances in Climate Change Research, Vol. 6/2, pp. 108-117, http://dx.doi.org/10.1016/j.accre.2015.09.007.
[202] Hailegiorgis, A., A. Crooks and C. Cioffi-Revilla (2018), “An agent-based model of rural households’ adaptation to climate change”, Journal of Artificial Societies and Social Simulation, Vol. 21/4, p. 4, http://dx.doi.org/10.18564/jasss.3812.
[138] Hallegatte, S. et al. (2015), Shock Waves: Managing the Impacts of Climate Change on Poverty, Washington, DC: World Bank, http://dx.doi.org/10.1596/978-1-4648-0673-5.
[5] Hallegatte, S., M. Bangalore and A. Vogt-Schilb (2016), Socioeconomic Resilience: Multi-Hazard Estimates in 117 Countries, World Bank, Washington, DC, http://dx.doi.org/10.1596/1813-9450-7886.
[36] Hallegatte, S. et al. (2013), “Future flood losses in major coastal cities”, Nature Climate Change, Vol. 3/9, pp. 802-806, http://dx.doi.org/10.1038/nclimate1979.
[132] Hallegatte, S., J. Rentschler and J. Rozenberg (2019), Lifelines: The Resilient Infrastructure Opportunity, Washington, DC: World Bank, http://dx.doi.org/10.1596/978-1-4648-1430-3.
[143] Hallegatte, S. and J. Rozenberg (2017), “Climate change through a poverty lens”, Nature Climate Change, Vol. 7/4, pp. 250-256, http://dx.doi.org/10.1038/nclimate3253.
[106] Hanna, E. and P. Tait (2015), “Limitations to thermoregulation and acclimatization challenge human adaptation to global warming”, International Journal of Environmental Research and Public Health, Vol. 12/7, pp. 8034-8074, http://dx.doi.org/10.3390/ijerph120708034.
[34] Harrington, L. and F. Otto (2020), “Reconciling theory with the reality of African heatwaves”, Nature Climate Change, Vol. 10/9, pp. 796-798, http://dx.doi.org/10.1038/s41558-020-0851-8.
[63] Harrington, L. and F. Otto (2020), “Reconciling theory with the reality of African heatwaves”, Nature Climate Change, Vol. 10/9, pp. 796-798, http://dx.doi.org/10.1038/s41558-020-0851-8.
[18] Hassani, H., X. Huang and E. Silva (2019), “Big data and climate change”, Big Data and Cognitive Computing, Vol. 3/1, p. 12, http://dx.doi.org/10.3390/bdcc3010012.
[139] Haug, C. et al. (2009), “Navigating the dilemmas of climate policy in Europe: Evidence from policy evaluation studies”, Climatic Change, Vol. 101/3-4, pp. 427-445, http://dx.doi.org/10.1007/s10584-009-9682-3.
[3] Hausfather, Z. et al. (2020), “Evaluating the performance of past climate model projections”, Geophysical Research Letters, Vol. 47/1, http://dx.doi.org/10.1029/2019GL085378.
[96] Hausfather, Z. et al. (2020), “Evaluating the performance of past climate model projections”, Geophysical Research Letters, Vol. 47/1, http://dx.doi.org/10.1029/2019GL085378.
[85] Hausfather, Z. and G. Peters (2020), “Emissions – the ‘business as usual’ story is misleading”, Nature, Vol. 577/7792, pp. 618-620, http://dx.doi.org/10.1038/d41586-020-00177-3.
[120] Hawkins, E. and R. Sutton (2010), “The potential to narrow uncertainty in projections of regional precipitation change”, Climate Dynamics, Vol. 37/1-2, pp. 407-418, http://dx.doi.org/10.1007/s00382-010-0810-6.
[119] Hawkins, E. and R. Sutton (2009), “The potential to narrow uncertainty in regional climate predictions”, Bulletin of the American Meteorological Societ, Vol. 90/8, pp. 1095-1107.
[136] Hayes, K. et al. (2018), “Climate change and mental health: risks, impacts and priority actions”, International Journal of Mental Health Systems, Vol. 12/1, http://dx.doi.org/10.1186/s13033-018-0210-6.
[164] Henderson, J., A. Storeygard and D. Weil (2012), “Measuring economic growth from outer space”, American Economic Review, Vol. 102/2, pp. 994-1028, http://dx.doi.org/10.1257/aer.102.2.994.
[54] Hess, J. et al. (2018), “Building resilience to climate change: Pilot evaluation of the impact of India’s first heat action plan on all-cause mortality”, Journal of Environmental and Public Health, Vol. 2018, pp. 1-8, http://dx.doi.org/10.1155/2018/7973519.
[81] Higgins, P. and J. Harte (2012), “Carbon cycle uncertainty increases climate change risks and mitigation challenges”, Journal of Climate, Vol. 25/21, pp. 7660-7668, http://dx.doi.org/10.1175/jcli-d-12-00089.1.
[234] Ho, E. and D. Budescu (2019), “Climate uncertainty communication”, Nature Climate Change, Vol. 9/11, pp. 802-803, http://dx.doi.org/10.1038/s41558-019-0606-6.
[206] Hoel, M. and T. Sterner (2007), “Discounting and relative prices”, Climatic Change, Vol. 84/3-4, pp. 265-280, http://dx.doi.org/10.1007/s10584-007-9255-2.
[69] Holden, P. et al. (2018), “Climate–carbon cycle uncertainties and the Paris Agreement”, Nature Climate Change, Vol. 8/7, pp. 609-613, http://dx.doi.org/10.1038/s41558-018-0197-7.
[182] Houck, B. (2018), “Why the world Is hating on plastic straws right now”, 12 July, Eater.
[233] Howe, L. et al. (2019), “Acknowledging uncertainty impacts public acceptance of climate scientists’ predictions”, Nature Climate Change, Vol. 9/11, pp. 863-867, http://dx.doi.org/10.1038/s41558-019-0587-5.
[191] Hsiang, S. (2016), “Climate Econometrics”, Annual Review of Resource Economics, Vol. 8/1, pp. 43-75, http://dx.doi.org/10.1146/annurev-resource-100815-095343.
[158] IFRC (2017), “Dominica: Hurricane Maria”, Information Bulletin, International Federation of Red Cross and Red Crescent Societies, Geneva.
[237] IPCC (2019), “Summary for Policymakers”, in H.-O. Pörtner et al. (eds.), IPCC Special Report on the Ocean and Cryosphere in a Changing Climate, Intergovernmental Panel on Climate Change, Geneva.
[111] IPCC (2018), “Annex I: Glossary [Matthews, J.B.R. (ed.)]”, in Masson-Delmotte, V. et al. (eds.), Global Warming of 1.5°C. An IPCC Special Report on the impacts of global warming of 1.5°C above pre-industrial levels and related global greenhouse gas emission pathways, in the context of strengthening the global response to the threat of climate change, Intergovernmental Panel on Climate Change, Geneva.
[97] IPCC (2018), “Summary for Policymakers”, in Masson-Delmotte, V. et al. (eds.), Global Warming of 1.5°C. An IPCC Special Report on the impacts of global warming of 1.5°C above pre-industrial levels and related global greenhouse gas emission pathways, in the context of strengthening the global response to the threat of climate c [...], World Meteorological Organization, Geneva, Switzerland.
[2] IPCC (2013), “Summary for Policymakers”, in Stocker, T. et al. (eds.), Climate Change 2013: The Physical Science Basis. Contribution of Working Group I to the Fifth Assessment Report of the Intergovernmental Panel on Climate Change, Cambridge University Press, Cambridge, United Kingdom and New York, NY.
[175] IPCC (2012), Managing the Risks of Extreme Events and Disasters to Advance Climate Change Adaptation. A Special Report of Working Groups I and II of the Intergovernmental Panel on Climate Change, Cambridge University Press, Cambridge, United Kingdom, https://www.ipcc.ch/pdf/special-reports/srex/SREX_Full_Report.pdf.
[231] IPCC (2007), “Summary for Policymakers”, in Solomon, S. (ed.), Climate Change 2007: The Physical Science Basis. Contribution of Working Group I to the Fourth Assessment Report of the Intergovernmental Panel on Climate Change, Cambridge University Press, Cambridge, United Kingdom and New York, NY.
[87] IPCC (2000), IPCC Special Report Emissions Scenarios – Summary for Policymakers, Intergovernmental Panel on Climate Change, Geneva.
[165] Ishizawa, O., J. Miranda and E. Strobl (2017), The Impact of Hurricane Strikes on Short-Term Local Economic Activity: Evidence from Nightlight Images in the Dominican Republic, World Bank, Washington, DC, http://dx.doi.org/10.1596/1813-9450-8275.
[131] Jafino, B. et al. (2020), “Revised estimates of the impact of climate change on extreme poverty by 2030”, Policy Research Working Paper, No. 9417, World Bank, Washington, DC, https://openknowledge.worldbank.org/handle/10986/34555.
[78] Jebeile, J. and M. Crucifix (2020), “Multi-model ensembles in climate science: Mathematical structures and expert judgements”, Studies in History and Philosophy of Science Part A, Vol. 83, pp. 44-52, http://dx.doi.org/10.1016/j.shpsa.2020.03.001.
[216] Jiang, L. and B. O’Neill (2017), “Global urbanization projections for the Shared Socioeconomic Pathways”, Global Environmental Change, Vol. 42, pp. 193-199, http://dx.doi.org/10.1016/j.gloenvcha.2015.03.008.
[104] Kadiyala, M. et al. (2020), “Assessment of climate change and vulnerability in Indian state of Telangana for better agricultural planning”, Theoretical and Applied Climatology, Vol. 143/1-2, pp. 309-325, http://dx.doi.org/10.1007/s00704-020-03425-8.
[190] Kalkuhl, M. and L. Wenz (2020), “The impact of climate conditions on economic production. Evidence from a global panel of regions”, Journal of Environmental Economics and Management, Vol. 103, p. 102360, http://dx.doi.org/10.1016/j.jeem.2020.102360.
[23] Kalnay, E. et al. (1996), “The NCEP/NCAR 40-year reanalysis project”, Bulletin of the American Meteorological Society, Vol. 77/3, http://dx.doi.org/https://journals.ametsoc.org/view/journals/bams/77/3/1520-0477_1996_077_0437_tnyrp_2_0_co_2.xml.
[64] Kamber, G., C. McDonald and G. Price (2013), Drying out: Investigating the economic effects of drought in New Zealand, Reserve Bank of New Zealand, Wellington, https://www.nzae.org.nz/wp-content/uploads/2014/05/price.pdf.
[66] Kattenberg, A. et al. (1996), “Climate models - Projections of future climate”, in Climate Change 1995: The Science of Climate Change, Contribution of Working Group I to the Second Assessment Report of the Intergovernmental Panel on Climate Change, Cambridge University Press, Cambridge, United Kingdom.
[225] Kelleher, J. (2017), “Descriptive versus prescriptive discounting in climate change policy analysis”, Georgetown Journal of Law & Public Policy, Vol. 15, https://heinonline.org/HOL/Page?handle=hein.journals/geojlap15&id=972&div=&collection= (accessed on 28 April 2021).
[21] Kennedy, J. et al. (2021), State of the Global Climate 2020, World Meteorological Organization, Geneva.
[200] Kniveton, D., C. Smith and S. Wood (2011), “Agent-based model simulations of future changes in migration flows for Burkina Faso”, Global Environmental Change, Vol. 21/SUPPL. 1, pp. S34-S40, http://dx.doi.org/10.1016/j.gloenvcha.2011.09.006.
[25] Kobayashi, S. et al. (2015), “The JRA-55 Reanalysis: General Specifications and Basic Characteristics”, Journal of the Meteorological Society of Japan. Ser. II, Vol. 93/1, pp. 5-48, http://dx.doi.org/10.2151/jmsj.2015-001.
[227] Kolstad, C. et al. (2015), “Social, economic, and ethical concepts and methods”, in Intergovernmental Panel of Climate Change Working Group 3, Cambridge University Press, Cambridge, United Kingdom, http://dx.doi.org/10.1017/cbo9781107415416.009.
[121] Kotamarthi, R. et al. (2016), Use of Climate Information for Decision-Making and Impacts Research: State of Our Understanding, prepared for the Department of Defense, Strategic Environmental Research and Development Program.
[173] KPMG (2016), “Preparing MSMEs for effective disaster management”, April, KPMG, Amstelveen, The Netherlands, https://assets.kpmg/content/dam/kpmg/pdf/2016/05/Disaster-Management-Preparedness-SME.pdf.
[168] Kreft, S., D. Eckstein and I. Melchior (2016), Global Climate Risk Index 2017: Who Suffers Most From Extreme Weather Events? Weather-related Loss Events in 2015 and 1996 to 2015, Germanwatch, Bonn.
[221] Kriegler, E. et al. (2017), “Fossil-fueled development (SSP5): An energy and resource intensive scenario for the 21st century”, Global Environmental Change, Vol. 42, pp. 297-315, http://dx.doi.org/10.1016/j.gloenvcha.2016.05.015.
[9] Kunreuther, H. et al. (2014), “Integrated risk and uncertainty assessment of climate change response policies”, in Edenhofer, O. et al. (eds.), Climate Change 2014: Mitigation of Climate Change. Contribution of Working Group III to the Fifth Assessment Report of the Intergovernmental Panel on Climate Chang, Cambridge University Press, Cambridge, United Kingdom and New York, NY.
[156] LaJeunesse Connette, K. et al. (2016), “Assessment of mining extent and expansion in Myanmar based on freely-available satellite imagery”, Remote Sensing, Vol. 8/11, p. 912, http://dx.doi.org/10.3390/rs8110912.
[100] Le Tertre, A. et al. (2006), “Impact of the 2003 heatwave on all-cause mortality in 9 French cities”, Epidemiology, Vol. 17/1, pp. 75-79, http://www.jstor.org/stable/20486164.
[118] Lowe, J. et al. (2018), UKCP18 Science Overview Report, MET Office.
[41] Marcheggiani, S. et al. (2010), “Risks of water-borne disease outbreaks after extreme events”, Toxicological & Environmental Chemistry, Vol. 92/3, pp. 593-599, http://dx.doi.org/10.1080/02772240903252140.
[1] Masson-Delmotte, V. et al. (eds.) (2021), Climate Change 2021: The Physical Science Basis. Contribution of Working Group I to the Sixth Assessment Report of the Intergovernmental Panel on Climate Change, Cambridge University Press, In press.
[86] Masson-Delmotte, V. et al. (eds.) (2018), Global Warming of 1.5°C. An IPCC Special Report on the impacts of global warming of 1.5°C above pre-industrial levels and related global greenhouse gas emission pathways, in the context of strengthening the global response to the threat of climate change, sustainable development, and efforts to eradicate poverty.
[88] McCollum, D. et al. (2020), “Energy modellers should explore extremes more systematically in scenarios”, Nature Energy, Vol. 5/2, pp. 104-107, http://dx.doi.org/10.1038/s41560-020-0555-3.
[105] MEEDDM, G. (2009), Evaluation du coût des impacts du changement climatique et de l’adaptation en France, http://www.onerc.gouv.fr (accessed on 4 July 2021).
[76] Meehl, G. et al. (2020), “Context for interpreting equilibrium climate sensitivity and transient climate response from the CMIP6 Earth system models”, Science Advances, Vol. 6/26, p. eaba1981, http://dx.doi.org/10.1126/sciadv.aba1981.
[147] Meyer, B., W. Mok and J. Sullivan (2015), “Household Surveys in Crisis”, Journal of Economic Perspectives, Vol. 29/4, pp. 199-226, http://dx.doi.org/10.1257/jep.29.4.199.
[16] Mitchell, T. and P. Jones (2005), “An improved method of constructing a database of monthly climate observations and associated high-resolution grids”, International Journal of Climatology, Vol. 25/6, pp. 693-712, http://dx.doi.org/10.1002/joc.1181.
[232] Molina, T. and E. Abadal (2021), “The evolution of communicating the uncertainty of climate change to policymakers: A study of IPCC synthesis reports”, Sustainability, Vol. 13/5, p. 2466, http://dx.doi.org/10.3390/su13052466.
[194] Monasterolo, I., A. Roventini and T. Foxon (2019), “Uncertainty of climate policies and implications for economics and finance: An evolutionary economics approach”, Ecological Economics, Vol. 163, pp. 177-182, http://dx.doi.org/10.1016/j.ecolecon.2019.05.012.
[57] Mora, C. et al. (2017), “Global risk of deadly heat”, Nature Climate Change, Vol. 7/7, pp. 501-506, http://dx.doi.org/10.1038/nclimate3322.
[149] Nardo, M. et al. (2005), Handbook on Constructing Composite Indicators, OECD, Paris, https://www.oecd.org/sdd/42495745.pdf.
[19] National Research Council (2012), “Integrated climate observing system and Earth system analysis”, in A National Strategy for Advancing Climate Modeling, National Academies Press, Washington, DC, http://dx.doi.org/10.17226/13430.
[193] Neumann, J. et al. (2020), “Climate damage functions for estimating the economic impacts of climate change in the United States”, Review of Environmental Economics and Policy, Vol. 14/1, pp. 25-43, http://dx.doi.org/10.1093/reep/rez021.
[160] Newell, R., B. Prest and S. Sexton (2021), “The GDP-Temperature relationship: Implications for climate change damages”, Journal of Environmental Economics and Management, p. 102445, http://dx.doi.org/10.1016/j.jeem.2021.102445.
[91] Nissan, H. et al. (2019), “On the use and misuse of climate change projections in international development”, Wiley Interdisciplinary Reviews: Climate Change, Vol. 10/3, p. e579, http://dx.doi.org/10.1002/wcc.579.
[195] Nordhaus, W. (2018), “Evolution of modeling of the economics of global warming: Changes in the DICE model, 1992-2017”, Climatic Change, Vol. 148/4, pp. 623-640, http://dx.doi.org/10.1007/s10584-018-2218-y.
[188] Nordhaus, W. (1992), “An optimal transition path for controlling greenhouse gases”, Science, Vol. 258/5086, pp. 1315-1319, http://dx.doi.org/10.1126/SCIENCE.258.5086.1315.
[31] Noy, I. (2015), “Natural disasters in the Pacific Island Countries: new measurements of impacts”, Natural Hazards, Vol. 84/S1, pp. 7-18, http://dx.doi.org/10.1007/s11069-015-1957-6.
[32] Noy, I. and W. duPont IV (2018), “The long-term consequences of disasters: What do we know, and what we still don’t”, International Review of Environmental and Resource Economics, Vol. 12/4, pp. 325-354, http://dx.doi.org/10.1561/101.00000104.
[129] OECD (2015), The Economic Consequences of Climate Change, OECD Publishing, Paris, https://dx.doi.org/10.1787/9789264235410-en.
[235] Oreskes, N. and E. Conway (2010), Merchants of Doubt: How a Handful of Scientists Obscure The Truth on Issues from Tobacco Smoke to Global Warming, Bloomsbury Press, New York.
[203] Otto, C. et al. (2017), “Modeling loss-propagation in the global supply network: The dynamic agent-based model acclimate”, Journal of Economic Dynamics and Control, Vol. 83, pp. 232-269, http://dx.doi.org/10.1016/J.JEDC.2017.08.001.
[28] Otto, F. (2016), “The art of attribution”, Nature Climate Change, Vol. 6/4, pp. 342-343, http://dx.doi.org/10.1038/nclimate2971.
[59] Otto, F. et al. (2020), “Challenges to understanding extreme weather changes in lower income countries”, Bulletin of the American Meteorological Society, Vol. 101/10, pp. E1851-E1860, http://dx.doi.org/10.1175/bams-d-19-0317.1.
[222] Otto, I. et al. (2020), “Social tipping dynamics for stabilizing Earth’s climate by 2050”, Proceedings of the National Academy of Sciences of the United States of America, Vol. 117/5, pp. 2354-2365, http://dx.doi.org/10.1073/pnas.1900577117.
[48] Ozturk, T., F. Saygili-Araci and M. Kurnaz (2021), “Projected changes in extreme temperature and precipitation indices over CORDEX-MENA domain”, Atmosphere, Vol. 12/5, p. 622, http://dx.doi.org/10.3390/atmos12050622.
[122] Palmer, T. and B. Stevens (2019), “The scientific challenge of understanding and estimating climate change”, Proceedings of the National Academy of Sciences, Vol. 116/49, pp. 24390-24395, http://dx.doi.org/10.1073/pnas.1906691116.
[171] Patankar, A. (2019), “Impacts of natural disasters on households and small businesses in India”, SSRN Electronic Journal, http://dx.doi.org/10.2139/ssrn.3590902.
[89] Peng, W. et al. (2021), “Climate policy models need to get real about people — here’s how”, Nature, Vol. 594/7862, pp. 174-176, http://dx.doi.org/10.1038/d41586-021-01500-2.
[50] Perkins, S. and L. Alexander (2013), “On the Measurement of Heat Waves”, Journal of Climate, Vol. 26/13, pp. 4500-4517, http://dx.doi.org/10.1175/jcli-d-12-00383.1.
[210] Perman, R. et al. (2011), Natural Resource and Environmental Economics -, Addison Wesley, https://www.amazon.fr/Natural-Resource-Environmental-Economics-Perman/dp/0321417534 (accessed on 6 January 2021).
[29] Philip, S. et al. (2020), “A protocol for probabilistic extreme event attribution analyses”, Advances in Statistical Climatology, Meteorology and Oceanography, Vol. 6/2, pp. 177-203, http://dx.doi.org/10.5194/ascmo-6-177-2020.
[238] Pidgeon, N. (2012), “Climate change risk perception and communication: Addressing a critical moment?”, Risk Analysis, Vol. 32/6, pp. 951-956, http://dx.doi.org/10.1111/j.1539-6924.2012.01856.x.
[192] Pindyck, R. (2017), “The use and misuse of models for climate policy”, Review of Environmental Economics and Policy, Vol. 11/1, pp. 100-114, http://dx.doi.org/10.1093/reep/rew012.
[239] Poortvliet, P. et al. (2020), “Communicating Climate Change Risk: A Content Analysis of IPCC’s Summary for Policymakers”, Sustainability, Vol. 12/12, p. 4861, http://dx.doi.org/10.3390/su12124861.
[186] Prigerson, H. and P. Maciejewski (2006), Prolonged Grief Disorder (PG-13), Weill Cornell Medicine Center for Research on End-of-Life Care, New York.
[17] Rahmstorf, S., G. Foster and N. Cahill (2017), “Global temperature evolution: Recent trends and some pitfalls”, Environmental Research Letters, Vol. 12/5, p. 054001, http://dx.doi.org/10.1088/1748-9326/aa6825.
[201] Rai, V. and A. Henry (2016), Agent-based Modelling of Consumer Energy Choices, Nature Publishing Group, http://dx.doi.org/10.1038/nclimate2967.
[26] Randles, C. et al. (2017), “The MERRA-2 Aerosol Reanalysis, 1980 onward. Part I: System description and data assimilation evaluation”, Journal of Climate, Vol. 30/17, pp. 6823-6850, http://dx.doi.org/10.1175/jcli-d-16-0609.1.
[179] Ranger, N. et al. (2010), “An assessment of the potential impact of climate change on flood risk in Mumbai”, Climatic Change, Vol. 104/1, pp. 139-167, http://dx.doi.org/10.1007/s10584-010-9979-2.
[8] Riahi, K. et al. (2017), “The Shared Socioeconomic Pathways and their energy, land use, and greenhouse gas emissions implications: An overview”, Global Environmental Change, Vol. 42, pp. 153-168, http://dx.doi.org/10.1016/j.gloenvcha.2016.05.009.
[56] Robine, J. et al. (2008), “Death toll exceeded 70,000 in Europe during the summer of 2003”, Comptes Rendus Biologies, Vol. 331/2, pp. 171-178, http://dx.doi.org/10.1016/j.crvi.2007.12.001.
[183] Rode, K. et al. (2015), “Can polar bears use terrestrial foods to offset lost ice-based hunting opportunities?”, Frontiers in Ecology and the Environment, Vol. 13/3, pp. 138-145, http://dx.doi.org/10.1890/140202.
[90] Rogelj, J. et al. (2021), “Net-zero emissions targets are vague: Three ways to fix”, Nature, Vol. 591/7850, pp. 365-368, http://dx.doi.org/10.1038/d41586-021-00662-3.
[161] Romer, D. (2020), “In praise of confidence intervals”, AEA Papers and Proceedings, Vol. 110, pp. 55-60, http://dx.doi.org/10.1257/pandp.20201059.
[116] Rowell, D. (2011), “Sources of uncertainty in future changes in local precipitation”, Climate Dynamics, Vol. 39/7-8, pp. 1929-1950, http://dx.doi.org/10.1007/s00382-011-1210-2.
[47] Russo, S. et al. (2016), “When will unusual heat waves become normal in a warming Africa?”, Environmental Research Letters, Vol. 11/5, p. 054016, http://dx.doi.org/10.1088/1748-9326/11/5/054016.
[214] Samir, K. and W. Lutz (2017), “The human core of the Shared Socioeconomic Pathways: Population scenarios by age, sex and level of education for all countries to 2100”, Global Environmental Change, Vol. 42, pp. 181-192, http://dx.doi.org/10.1016/j.gloenvcha.2014.06.004.
[176] Serdeczny, O., E. Waters and S. Chan (2016), “Non-economic loss and damage in the context of climate change”, Discussion Paper, No. 3, German Development Institute, Bonn.
[240] Sharpe, S. and T. Lenton (2021), “Upward-scaling tipping cascades to meet climate goals: Plausible grounds for hope”, Climate Policy, Vol. 21/4, pp. 421-433, http://dx.doi.org/10.1080/14693062.2020.1870097.
[11] Shepherd, T. (2019), “Storyline approach to the construction of regional climate change information”, Proceedings of the Royal Society A: Mathematical, Physical and Engineering Sciences, Vol. 475/2225, p. 20190013, http://dx.doi.org/10.1098/rspa.2019.0013.
[114] Shepherd, T. (2014), “Atmospheric circulation as a source of uncertainty in climate change projections”, Nature Geoscience, Vol. 7/10, pp. 703-708, http://dx.doi.org/10.1038/ngeo2253.
[125] Shepherd, T. et al. (2018), “Storylines: an alternative approach to representing uncertainty in physical aspects of climate change”, Climatic Change, Vol. 151/3-4, pp. 555-571, http://dx.doi.org/10.1007/s10584-018-2317-9.
[71] Sherwood, S. et al. (2020), “An Assessment of Earth’s Climate Sensitivity Using Multiple Lines of Evidence”, Reviews of Geophysics, Vol. 58/4, http://dx.doi.org/10.1029/2019rg000678.
[113] Siler, N. et al. (2018), “Revisiting the surface-energy-flux perspective on the sensitivity of global precipitation to climate change”, Climate Dynamics, Vol. 52/7-8, pp. 3983-3995, http://dx.doi.org/10.1007/s00382-018-4359-0.
[174] Singh, C. (2018), Assessing India’s Mounting Climate Losses to Financial Institutions, Action on Climate Today, http://www.indiaenvironmentportal.org.in/files/file/climate-losses-revised.pdf.
[178] Singh, C. et al. (2021), “Losses and damages associated with slow-onset events: Urban drought and water insecurity in Asia”, Current Opinion in Environmental Sustainability, Vol. 50, pp. 72-86, http://dx.doi.org/10.1016/j.cosust.2021.02.006.
[101] Sjoukje Philip, C. et al. (2021), “Rapid attribution analysis of the extraordinary heatwave on the Pacific Coast of the US and Canada June 2021”, World Weather Attribution, https://www.worldweatherattribution.org/wp-content/uploads/NW-US-extreme-heat-2021-scientific-report-WWA.pdf.
[109] Smith, D. et al. (2019), “Robust skill of decadal climate predictions”, npj Climate and Atmospheric Science, Vol. 2/1, http://dx.doi.org/10.1038/s41612-019-0071-y.
[123] Stainforth, D. and R. Calel (2020), “New priorities for climate science and climate economics in the 2020s”, Nature Communications, Vol. 11/1, http://dx.doi.org/10.1038/s41467-020-16624-8.
[43] Steffen, W. et al. (2019), Compound Costs: How Climate Change is Damaging Australia’s Economy, Climate Council of Australia, Potts Point.
[80] Steffen, W. et al. (2018), “Trajectories of the Earth System in the Anthropocene”, Proceedings of the National Academy of Sciences, Vol. 115/33, pp. 8252-8259, http://dx.doi.org/10.1073/pnas.1810141115.
[207] Sterner, T. and U. Persson (2008), “An even Sterner Review: Introducing relative prices into the discounting debate”, Review of Environmental Economics and Policy, Vol. 2/1, pp. 61-76, http://dx.doi.org/10.1093/reep/rem024.
[196] Stern, N. (2013), “The structure of economic modeling of the potential impacts of climate change: Grafting gross underestimation of risk onto already narrow science models”, Journal of Economic Literature, Vol. 51/3, pp. 838-859, http://dx.doi.org/10.1257/jel.51.3.838.
[189] Stern, N. (2006), The Economics of Climate Change : The Stern Review, Cambridge University Press, Cambridge, United Kingdom.
[108] Stillman, J. (2019), “Heat waves, the new normal: Summertime temperature extremes will impact animals, ecosystems, and human communities”, Physiology, Vol. 34/2, pp. 86-100, http://dx.doi.org/10.1152/physiol.00040.2018.
[241] Stocker, T. et al. (2001), “Physical climate processes and feedbacks”, in Houghton, J. et al. (eds.), Climate Change 2001: The Scientific Basis – Contribution of Working Group I to the Third Assessment Report of the Intergovernmental Panel on Climate Change, Cambridge University Press, Cambridge, United Kingdom and New York, NY.
[79] Stocker, T. et al. (2013), “Technical summary”, in Stocker, T. et al. (eds.), Climate Change 2013: The Physical Science Basis. Contribution of Working Group I to the Fifth Assessment Report of the Intergovernmental Panel on Climate Change, Intergovernmental Panel on Climate Change, Geneva.
[98] Stott, P., D. Stone and M. Allen (2004), “Human contribution to the European heatwave of 2003”, Nature, Vol. 432/7017, pp. 610-614, http://dx.doi.org/10.1038/nature03089.
[159] Strobl, E. (2012), “The economic growth impact of natural disasters in developing countries: Evidence from hurricane strikes in the Central American and Caribbean regions”, Journal of Development Economics, Vol. 97/1, pp. 130-141, http://dx.doi.org/10.1016/j.jdeveco.2010.12.002.
[213] Subroy, V. et al. (2019), “The worth of wildlife: A meta-analysis of global non-market values of threatened species”, Ecological Economics, Vol. 164, p. 106374, http://dx.doi.org/10.1016/j.ecolecon.2019.106374.
[236] Sutton, R. (2019), “Climate science needs to take risk assessment much more seriously”, Bulletin of the American Meteorological Society, Vol. 100/9, pp. 1637-1642, http://dx.doi.org/10.1175/bams-d-18-0280.1.
[145] Taupo, T., H. Cuffe and I. Noy (2018), “Household vulnerability on the frontline of climate change: the Pacific atoll nation of Tuvalu”, Environmental Economics and Policy Studies, Vol. 20/4, pp. 705-739, http://dx.doi.org/10.1007/s10018-018-0212-2.
[39] Tong, S. (2017), “Flooding-related displacement and mental health”, The Lancet Planetary Health, Vol. 1/4, pp. e124-e125, http://dx.doi.org/10.1016/s2542-5196(17)30062-1.
[22] Trewin, B. et al. (2021), “Headline indicators for global climate monitoring”, Bulletin of the American Meteorological Society, Vol. 102/1, pp. E20-E37, http://dx.doi.org/10.1175/bams-d-19-0196.1.
[185] Tschakert, P. et al. (2017), “Climate change and loss, as if people mattered: Values, places and experiences”, WIREs Climate Change, Vol. 8/5, http://dx.doi.org/10.1002/wcc.476.
[137] Tschakert, P. et al. (2019), “One thousand ways to experience loss: A systematic analysis of climate-related intangible harm from around the world”, Global Environmental Change, Vol. 55, pp. 58-72, http://dx.doi.org/10.1016/j.gloenvcha.2018.11.006.
[33] Tschumi, E. and J. Zscheischler (2019), “Countrywide climate features during recorded climate-related disasters”, Climatic Change, Vol. 158/3-4, pp. 593-609, http://dx.doi.org/10.1007/s10584-019-02556-w.
[155] Turner, W. (2013), “Satellites: Make data freely accessible”, Nature, Vol. 498/7452, pp. 37-37, http://dx.doi.org/10.1038/498037c.
[162] Uddin, M. et al. (2019), “Mapping of climate vulnerability of the coastal region of Bangladesh using principal component analysis”, Applied Geography, Vol. 102, pp. 47-57, http://dx.doi.org/10.1016/j.apgeog.2018.12.011.
[68] UNFCCC (2015), Paris Agreement, United Nations Framework Convention on Climate Change, Paris, https://treaties.un.org/pages/ViewDetails.aspx?src=TREATY&mtdsg_no=XXVII-7-d&chapter=27.
[151] Usanov, A. and M. Gehem (2014), Innovation in a Waming World, The Hague Centre for Strategic Studies and TNO, https://hcss.nl/report/innovation-in-a-warming-world-research-tackling-the-grand-challenge-of-climate-change-in-europe/.
[58] Vaidyanathan, A. et al. (2019), “Assessment of extreme heat and hospitalizations to inform early warning systems”, Proceedings of the National Academy of Sciences, Vol. 116/12, pp. 5420-5427, http://dx.doi.org/10.1073/pnas.1806393116.
[205] van der Geest, K. et al. (2019), “The impacts of climate change on ecosystem services and resulting losses and damages to people and society”, in Mechler, R. et al. (eds.), Loss and Damage from Climate Change. Climate Risk Management, Policy and Governance, Springer, Cham, http://dx.doi.org/10.1007/978-3-319-72026-5_9.
[217] van Vuuren, D. et al. (2017), “Energy, land-use and greenhouse gas emissions trajectories under a green growth paradigm”, Global Environmental Change, Vol. 42, pp. 237-250, http://dx.doi.org/10.1016/j.gloenvcha.2016.05.008.
[226] Varian, H. (2006), “Economist seeks to recalculate the costs of global climate change”, New York Times, 14 December, https://www.nytimes.com/2006/12/14/business/worldbusiness/14iht-climate.3896788.html (accessed on 31 March 2021).
[99] Vautard, R. et al. (2020), “Human contribution to the record-breaking June and July 2019 heatwaves in Western Europe”, Environmental Research Letters, Vol. 15/9, p. 094077, http://dx.doi.org/10.1088/1748-9326/aba3d4.
[30] Visser, H., A. Petersen and W. Ligtvoet (2014), “On the relation between weather-related disaster impacts, vulnerability and climate change”, Climatic Change, Vol. 125/3-4, pp. 461-477, http://dx.doi.org/10.1007/s10584-014-1179-z.
[170] Viswanathan, B. and K. Kavi Kumar (2015), “Weather, agriculture and rural migration: Evidence from state and district level migration in India”, Environment and Development Economics, Vol. 20/4, pp. 469-492, http://dx.doi.org/10.1017/s1355770x1500008x.
[73] Wagner, G. and M. Weitzman (2018), “Potentially large equilibrium climate sensitivity tail uncertainty”, Economics Letters, Vol. 168, pp. 144-146, http://dx.doi.org/10.1016/j.econlet.2018.04.036.
[37] Ward, P. et al. (2017), “A global framework for future costs and benefits of river-flood protection in urban areas”, Nature Climate Change, Vol. 7/9, pp. 642-646, http://dx.doi.org/10.1038/nclimate3350.
[74] Weitzman, M. (2009), “Additive damages, fat-tailed climate dynamics, and uncertain discounting”, Economics: The Open-Access, Open-Assessment E-Journal, Vol. 3/2009-39, p. 1, http://dx.doi.org/10.5018/economics-ejournal.ja.2009-39.
[187] Wei, Y., Z. Mi and Z. Huang (2015), “Climate policy modeling: An online SCI-E and SSCI based literature review”, Omega, Vol. 57, pp. 70-84, http://dx.doi.org/10.1016/j.omega.2014.10.011.
[184] Whiteman, J. et al. (2015), “Summer declines in activity and body temperature offer polar bears limited energy savings”, Science, Vol. 349/6245, pp. 295-298, http://dx.doi.org/10.1126/science.aaa8623.
[55] Whitman, S. et al. (1997), “Mortality in Chicago attributed to the July 1995 heat wave”, American Journal of Public Health, Vol. 87/9, pp. 1515-1518, http://dx.doi.org/10.2105/ajph.87.9.1515.
[141] WHO (2021), “Hospital beds (per 10 000 population)”, Global Health Observatory, (database), https://www.who.int/data/gho/data/indicators/indicator-details/GHO/hospital-beds-(per-10-000-population) (accessed on 10 September 2021).
[135] WHO (2014), Quantitative risk assessment of the effects of climate change on selected causes of death, 2030s and 2050s, https://apps.who.int/iris/bitstream/handle/10665/134014/9789241507691_eng.pdf?sequence=1&isAllowed=y.
[180] Witsenburg, K. and W. Adano (2009), “Of rain and raids: Violent livestock raiding in northern Kenya”, Civil Wars, Vol. 11/4, pp. 514-538, http://dx.doi.org/10.1080/13698240903403915.
[204] World Bank (2021), Climate Change Knowledge Portal, website, https://climateknowledgeportal.worldbank.org/ (accessed on 10 September 2021).
[140] World Bank (2021), “Subnational population”, DataBank, (database), https://databank.worldbank.org/source/subnational-population (accessed on 10 September 2021).
[35] Xu, Z., Y. Han and Z. Yang (2018), “Dynamical downscaling of regional climate: A review of methods and limitations”, Science China Earth Sciences, Vol. 62/2, pp. 365-375, http://dx.doi.org/10.1007/s11430-018-9261-5.
[44] Zander, K. et al. (2015), “Heat stress causes substantial labour productivity loss in Australia”, Nature Climate Change, Vol. 5/7, pp. 647-651, http://dx.doi.org/10.1038/nclimate2623.
[10] Zappa, G., E. Bevacqua and T. Shepherd (2021), “Communicating potentially large but non‐robust changes in multi‐model projections of future climate”, International Journal of Climatology, Vol. 41/6, pp. 3657-3669, http://dx.doi.org/10.1002/joc.7041.
[117] Zappa, G. and T. Shepherd (2017), “Storylines of atmospheric circulation change for European regional climate impact assessment”, Journal of Climate, Vol. 30/16, pp. 6561-6577, http://dx.doi.org/10.1175/jcli-d-16-0807.1.
[77] Zelinka, M. et al. (2020), “Causes of higher climate sensitivity in CMIP6 models”, Geophysical Research Letters, Vol. 47/1, http://dx.doi.org/10.1029/2019gl085782.
[49] Zittis, G. et al. (2021), “Business-as-usual will lead to super and ultra-extreme heatwaves in the Middle East and North Africa”, npj Climate and Atmospheric Science, Vol. 4/1, http://dx.doi.org/10.1038/s41612-021-00178-7.
Notes
← 1. The Flood Observatory, https://floodobservatory.colorado.edu/.
← 2. A carbon dioxide equivalent or CO2 equivalent (CO2-eq) is a metric used to compare the emissions from various GHG to CO2 emissions. It is calculated by converting amounts of other gases to the equivalent amount of carbon dioxide on the basis of their global-warming potential (GWP).
← 3. For assessment of losses and damages while atmospheric concentrations of GHGs continue to rise and the climate is still changing, the TCR is the more useful metric to understand levels of global change such as climatic responses in the mid-21st century. For assessment of the long-term “new normal” resulting from a given level of climate change, after the Earth system has stabilised, the ECS is more useful to understand changes such as the long-term sea level rise commitment.
← 4. The uncertainty in the ECS range arises from the multiple processes and feedbacks in the climate system. These can amplify or dampen the amount of warming resulting from a direct increase in emissions and thus forcing. Atmospheric feedbacks, which largely control ECS, include water vapour in the atmosphere and clouds and their interaction with radiation. In addition, uncertainties in oceanic processes determining the transports of air-sea fluxes of heat and surface reflectivity, such as albedo of clouds, snow or land surfaces, are also at the origin of the uncertainty range in ECS (Stocker et al., 2001[241]). There is high confidence that in combination these feedbacks will lead to an amplification (rather than dampening) of the warming that would be expected by simple physics, especially over long-term scales (Flato, 2013[12]).
← 5. Note this is not inconsistent with the AR5 “likely” (66-100% probability) range being 1.5-4.5ºC, since there was up to a 34% chance of the outcome being outside the bounds.
← 6. Observed global mean surface temperature (GMST) provided for the decade 2006-15 relative to the average over the 1850-1900 period (IPCC, 2018[97]). The likely range is between 0.75°C and 0.99°C.
← 7. A GCM model divides up the Earth into slices, a so-called grid, at which a high number of complex processes of the Earth’s dynamics are modelled and projected. The spatial resolution of state-of-the-art GCMs is about 100 km.
← 8. Temperature anomalies mean a deviation from a baseline reference value. A positive anomaly means the temperature in question is above the baseline and a negative anomaly means the temperature is below that baseline. “Anomaly” is a scientific term and does not mean that anything is unexpected or unexplainable. The use of temperature anomalies minimises biases introduced by models and the effect of climate variability. This allows for more meaningful comparisons between locations and models, as well as more accurate calculations of temperature trends.
← 9. Exposure describes the assets and livelihoods that are exposed to climatic hazards, such as people living in low lying coastal areas. Vulnerability describes the propensity of exposed assets and livelihoods to be adversely affected by hazards, such as physical durability of buildings in low lying coastal areas (see Section 1.4 in Chapter 1).
← 10. There are exceptions, of course, such as the insurance market, where more information may collapse the market: for example, if an insurance provider knows exactly which households will be hit with floods in the next couple of years, it will refuse to provide insurance to those households. In turn, households that will not be affected will not take insurance. They know they are only being offered insurance because they will not be hit. This is because insurance essentially creates a market for uncertainty (see Chapter 5). Once uncertainty is sufficiently alleviated, they will not work. However, even these markets cannot work with a sufficient amount of information, which enables a risk transfer through the market. This is one reason why governments must strive to balance privacy of insureds and information that insurance companies can access.
← 12. There are different types of IAMs and different models for each type of IAM, developed by a number of institutions and research entities over the years. Full-scale IAMs describe the potential evolution of energy-system, population growth, economic development and other drivers of emissions such as agriculture and land use. These are traditionally used to examine the cost-effectiveness of achieving mitigation goals. Another type of IAMs, which are the focus of this section, are reduced-complexity. They make simplified assumptions around socio-economic drivers of emissions, costs of mitigations, while including costs of avoiding climate impacts.
← 13. Sterner and Persson (2008[207]) assume 0.5 for elasticity of substitution, meaning a 10% increase in environmental goods’ price would increase the demand for the other good by 5% – with perfect substitution the figure would be 10%.
← 14. “Abrupt” is difficult to define, especially in the social sciences. Some papers do not even consider the temporal aspects of socio-economic tipping points (David Tàbara et al., 2018[224]). Sharpe and Lenton (2021[240]) consider a length of 20 years as a tipping point. Otto et al. (2020[222]) define it as 30 years.
← 15. For example, if there is a 50% chance the 10% discount rate is appropriate, and a 50% chance the 0% discount rate is appropriate, then the expected value in 100 years is: . The implied certainty-equivalent social discount rate is r: Thus, or 1%, which is below 5% (the average of 10% and 0%).