Measuring Innovation in Education 2023
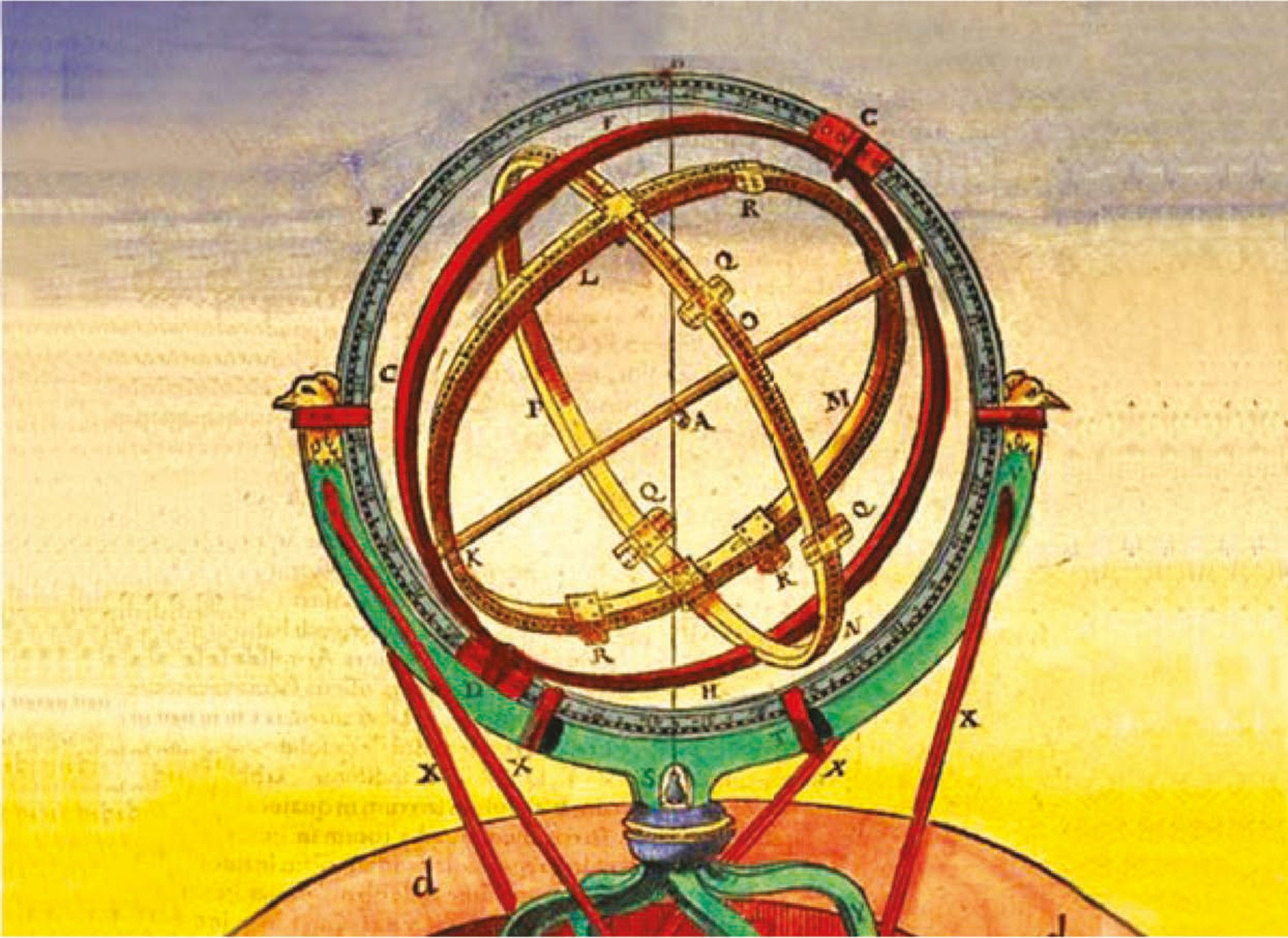
7. Measuring educational innovation through social media: An international exploration in the Twittersphere
Abstract
This chapter explores the possibilities to measure and analyse education innovation internationally using existing social media data. It proposes a method and a proof‑of‑concept regarding the measurement of the nature and intensity of exchanges around educational innovation using big data from a widely used social media: Twitter. Using social network analysis, it uncovers the nature of the networks that drive the discussion on educational innovation in Twitter, identifies the educational innovation areas that were discussed, and analyses the similarities and differences across three different languages and geographic zones: English, French and Spanish. The chapter points to the advantages (and limitations) of this method, notes how it could be expanded to other areas of interest, and illustrates how big data could be used for international comparative work.
Alan Daly, Miguel Del Fresno and Martin Rehm contributed equally to the chapter and are the first authors.
Introduction
The understanding of innovation is essential to the improvement of education. Developing the ability to measure and identify educational innovation and link it to its drivers and effects is a first step to refining and improving countries’ innovation policies in the education sector. This could also support state and local education administrators in strengthening their improvement strategies and how they empower stakeholders to innovate.
Cumulative knowledge on educational innovation and educational innovation policy requires to establish and provide countries with indicators that can be regularly updated over time, and/or a robust methodology to develop those indicators. While this can partially rely on the use of existing international data sets, could be based on the development of new surveys, this also implies to analyse and better understand the drivers of innovation in the education sector, where countries stand in this area, and to expand the methodologies and data sources to measure innovation in an accurate and comprehensive way.
The OECD explored different ways to understand and measure important dimensions of the innovation process and output in education. The 2014 edition of Measuring Innovation in Education (OECD, 2014[1]) computed measures of innovation allowing the comparison of innovation in education and in other sectors of society – showing high levels of innovation in knowledge and methods in education (and particularly higher education) compared to other sectors, but lower levels in most other types of innovation. The 2019 edition of Measuring Innovation in Education (Vincent-Lancrin et al., 2019[2]) focused on identifying the introduction of new teaching and school practices as well as of significantly changed practices and highlighted a moderate level of innovation in the classroom and in schools as measured by the change in practices that are considered as the most important to explain learning outcomes in mathematics, science and literacy.
This chapter proposes a new methodological approach to use existing data to measure the nature and processes of innovation in education. Instead of working with statistically designed datasets, it works with “big data” that users made publicly available on the Internet. In this particular case, we use Twitter, a social media platform, to identify the nature of the discussion on educational innovation across linguistic areas (English, French and Spanish) as well as the structure of the networks. This approach casts new light on how educational innovation is discussed, spread and by whom in different countries and languages.
The first section of the chapter recalls what educational innovation means. The second section puts the proposed methodological approach in perspective by contrasting it with the “survey” approach. We then present “social media”, the data and data analysis methodology before zeroing in on three separate analyses based on three different languages allowing the comparison of the “social networks” interested in educational innovation, the types of content related to educational innovation discussed across countries, but also the actors involved in sharing or re-sharing these ideas. In conclusion, we summarise the findings and draw the lessons of this methodology for further work in this area.
Conceptualising educational innovation
Innovation is crucial for individuals and societies to make progress and be able to face new and unprecedented challenges (Serdyukov, 2017[3]). This is true in education as in any other sector of society. However, innovation in education is more or less desired depending on time and stakeholders. For example, policy makers often suggest that educational systems are sluggish in adjusting to changes and often reluctant to innovation within a subjectively defined, reasonable amount of time. Yet, teachers and other educational professionals paint a different picture. From their perspective, there are too many – superficial – changes and supposedly innovations that are externally imposed on them in a top-down fashion without an indication of policy makers being (fully) aware of the circumstances in which teachers and pupils teach and learn (OECD, 2014[1]). Consequently, they often feel neglected in the process of policy development and only partially supported in the practical implementation of policy recommendations and strategies (Ketelaar et al., 2012[4]; Lewin and Stuart, 1993[5]). The COVID pandemic has shown that education systems could innovate and adjust very quickly to new realities when forced to though – see (Vincent-Lancrin, Cobo Romaní and Reimers, 2022[6]; Thorn and Vincent-Lancrin, 2021[7]).
While a debate about how much, when and where innovation is needed exists, there still appears to be a common ground. All participating parties share the belief and conviction that educational systems are running up against a wide range of challenges that need to be addressed in order to provide the best possible education for younger generations. Furthermore, the scientific community already stipulated and provided empirical evidence that there is an increasing need for teachers to develop and implement new, collaborative, approaches to learning (Finsterwald et al., 2013[8]; Rehm et al., 2020[9]). Previous OECD reports suggested four reasons why educational innovation matters, namely educational innovations can i) improve learning outcomes and the quality of education, ii) contribute to the enhancement of equity and equality, iii) have positive effects of efficiency, and iv) update the educational system to keep track and not loose pace compared to societal and economic changes that are occurring simultaneously (OECD, 2014[1]; OECD, 2016[10]; Vincent-Lancrin et al., 2019[2]); see also chapter 2 of this report (Van Lieshout, Arundel and Vincent-Lancrin, 2023[11]).
When considering the improvement of learning outcomes, concepts like self-regulated learning (e.g. (Garcia, Falkner and Vivian, 2019[12]) and personalised learning experiences and environments (e.g. (Prain et al., 2013[13]) have been suggested. Exemplary educational innovations that may be able to enhance issues of equity and equality can be centred around the digitalisation of education (e.g. (McLay and Reyes, 2019[14]) and the resulting ability of pupils and students to more easily access information and produce their own learning materials – e.g. (Lech et al., 2017[15]). The concept of efficiency, while highly important from a socio-economic perspective, has often been disconnected from educational science and the realities of educational professionals (Serdyukov, 2017[3]). Moreover, scholars have generally suggested the existence of a “productivity paradox” – e.g. (Polák, 2017[16]), which suggests that the advancement of technology is not matched with proportional increases in the productivity of learning. Interestingly, this notion can be related to “cognitive load theory” – e.g. (Kalyuga and Singh, 2016[17]) from the realm of educational science. In a nutshell, this theory postulates that too much extraneous information, such as an overload of information from social media – e.g. (Choo et al., 2015[18]), distracts the working memory. As a consequence, people can easily feel overwhelmed by information that, in essence, might not necessarily contribute to the actual learning process. Furthermore, if the level of extraneous information is too high, people are more prone to fall back on their long-term memory, more easily digest confirmatory information and replicate their established routines. Consequently, the chances of engaging into transformative processes, which incorporate new and even challenging information, might be diminished. In the context of educational systems meeting the pace of broader societal changes, scholars have again indicated the need for schools to prepare pupils for the ongoing digitalisation of the workplace, in order to reap the benefits it brings, but also to deal with the eminent disadvantages and pitfalls – e.g. (Dumont, Istance and Benavides, 2010[19]; Schleicher, 2012[20]).
Generally, innovation can be defined as “a new or improved product or process (or combination thereof) that differs significantly from the unit’s previous products or processes and that has been made available to potential users (product) or brought into use by the unit (process)” (OECD/Eurostat, 2018[21]). Following the fourth edition of the Oslo Manual (OECD/Eurostat, 2018[21]), this definition can be simplified into two main types of innovation, namely, i) product and ii) process innovation. Product innovation refers to innovation in goods and services, which are often intertwined in the context of digitalisation. From an educational perspective, these take on the form of new educational resources, such as e-textbooks, or educational formats, including e- and blended learning scenarios. Process innovation refers to innovation in production processes or activities. Examples from the educational realm include innovative processes to organise professional development of teachers, or new ways to foster collaboration between educational professionals. While these definitions provide a valuable basis for further considerations and conceptualisations, the practical ramifications are inherently difficult to measure.
Determining the degree of educational innovation – from surveys to social media
Generally, two approaches to measure and determine the degree of educational innovation are used.
First, the adaptation to the context of education of national innovation surveys, such as the EU Community Innovation Survey1. These tools are well established and have been used for decades in the private sector (OECD/Eurostat, 2018[21]). Moreover, there already have been efforts to adapt these survey to better represent the public sector, in general – e.g. (Bloch, Genicot and Ray, 2008[22]) , and the educational sector in more detail – e.g. (Haelermans, 2010[23]; Halász, 2018[24]; OECD, 2014[1]). In the latter case, the applicable studies often tried to relate the indicators for innovation to performance measures of pupils and students. This approach was adopted to develop survey instruments in the first part of this book.
The second broad approach to measure innovation is rooted in the assessment of organisational change. Here, survey tools are designed, distributed and analysed that deal with the dissemination of specific innovations in work practices, for example the introduction of (new) computers or organisational practices – e.g. (Greenan and Lorenz, 2013[25]). In contrast to the first approach, here the focus shifts more towards micro-level data and comparison of reports across time – e.g. (Adams Becker et al., 2018[26]). (OECD, 2014[1]) presents a possible implementation of this approach.
These approaches have undoubtedly contributed to our understanding of how educational innovations is introduced and implemented in practice. However, while innovations aim at improving something or offering new sets of opportunities to face challenges, there is no guarantee that a certain set of chosen changes or altered processes will indeed yield the envisioned results (Vincent-Lancrin, 2020[27]). Moreover, the two indicated types of measures tend to be summative and evaluate the situation after an educational innovation has been developed and implemented. This typically requires some time.
Involving all relevant actors already from the start of the underlying innovation process is considered key to successful innovation (Boahin and Hofman, 2012[28]; Ketelaar et al., 2012[4]; Prince Machado, Tenorio Sepúlveda and Ramirez Montoya, 2016[29]). To this effect, one of most widely used and simplest strategies is to engage communities through communication and collaboration, which involves accessing just in time information (e.g., news, ideas, approaches) and the exchange of information, knowledge, and strategies regarding the best practices from schools and communities (OECD, 2013[30]).
It is therefore important to also capture, monitor and evaluate the processes underlying the development and implementation of educational innovation – and to do it in real time if possible. Social media offers multiple parties (e.g. teachers and other educational professionals) the opportunity to start bottom-up initiatives and innovations (Rehm et al., 2020[31]; Rehm et al., 2020[9]; Rehm and Notten, 2016[32]). If educators and others feel marginalised from official policy processes and developments, they now have the possibility to publicly voice their concern and openly discuss both product and process innovations. Additionally, these same individuals are also able to exchange information, resources and experiences about the interplay between the top-down and bottom-up innovation. More specifically, educational professionals can easily discuss specific educational practices (e.g. video conferencing in times of home-schooling) using social media. They can also share views and best-practices on how they have been introduced and supported within their local settings, which forms the heart of innovation. For example, a recent study investigated whether these types of networks and communicative exchanges are able to exert real influence on (educational) policy processes. Investigating the national discussion on Twitter about the introduction of the Common Core in the United States, a science curriculum adopted in several US states, Supovitz and colleagues (2015[33]) suggest that informal networks in social media can have an impact on current affairs in the (educational) policy process.
Additionally, there has been a shift from government to governance in the past few decades (Ball and Junemann, 2012[34]). As a result, (horizontal) co-operation between the governmental bodies and networks of relevant actors (private/public) have become central. In particular, the network governance approach focuses on the informal, horizontal nature and mutual dependency between the various actors in the development and implementation of policy (Klijn and Koppenjan, 2012[35]). In these policy processes, governmental actors become facilitators and coordinators of discussions about policy processes (Pierre and Peters, 2000[36]). These discussions increasingly take place on social media platforms, such as Facebook and Twitter. This allows everyone who is interested to closely follow developments, share views and opinions, critically reflect on practice, and possibly assert influence on education policy processes (Cornelissen et al., 2011[37]). It is therefore of crucial importance to better understand how the underlying communication flows and patterns develop and evolve over time, as policy processes will gradually become more and more informed by discussions on social media.
Social opportunity spaces
The rise of social media has led to a panoply of online communication spaces or sites, such as Facebook, LinkedIn and Twitter, wherein individuals can engage into the latter type of activities and therefore engage into deliberative learning. On the basis of their structure and general characteristics, these platforms connect individuals via networked devices, such as computers (Wellman, 2001[38]). Consequently, these platforms are also referred to as social networking sites (SNS). Apart from recreational purposes (e.g. sharing holiday photos and pet videos), these spaces are increasingly used as places for professionals to meet and discuss current topics and problems relevant to their profession. Additionally, there has been a growing amount of research that investigated the potential of SNS for informal learning. Owen and colleagues (2016, p. 2[39]) postulate that social media provides teachers with a means to “scale-up their professional learning”. Moreover, a growing number of studies have shown that teachers use SNS, such as Twitter, to keep up to date with the latest news on education and share resources with colleagues (Risser, 2013[40]). This observation is paired with more theoretical considerations by scholars like Marotzki (2004[41]), who suggest that social media provides us with an unprecedented opportunity to exchange information and experiences, while connecting with other people and learning from and with each other.
These social media platforms essentially provide informal learning spaces that can initiate professional development processes (Spanhel, 2010[42]). However, in contrast to formal learning spaces, the focus here is not primarily on the acquisition and transfer of knowledge. Instead, it is rather a question of the "contextualization, flexibility, decentralization, pluralization of knowledge and experience patterns, or [...] the opening of indeterminacy spaces" (Marotzki and Jörissen, 2008, p. 100[43]). In that sense, there is considerable similarity with the conceptualisations of other scholars, who theorised and contemplated about online (learning) spaces. For example, Gee (2005, p. 223[44]) used the term affinity spaces. He introduced this term as a result of his disagreement with concepts like “community”, which in his opinion focused too much on membership. According to the author this carries the connotation of “close-knit personal ties among people which do not necessarily always fit [the situation]” (p. 214[44]). However, his work is largely rooted in observations from and around real-time strategy computer games. Consequently, it can be argued that affinity spaces only have limited relevance for situations where individuals engage into deliberative professional learning. Alternatively, Howard Rheingold (2007[45]) has promoted the term smart mobs. Yet, while there are again conceptual similarities, Rheingold’s work has mainly been used in conjunction with topics like political engagement (Hart and Sharma, 2004[46]) and smart (technical) systems (Lee et al., 2006[47]). Ito and colleagues (2012[48]) refer to connected learning, which is fostered in a (online) space and “[…] seeks to build communities and collective capacities for learning and opportunity” (p. 8[48]). Consequently, learning spaces can therefore be described as being embedded in the immediate environments of individuals and enable them to explicate their own ideas and experiences, which in turn contributes to a growing pool of resources and information that everyone can benefit from (Kolb and Kolb, 2017[49]).
In the context of social media, it has been argued that a possible advantage of such online learning spaces is that they can create “persistent, predictable, multi-user connections that support a wide range of user interaction and collaborative activity” (Mynatt, O’Day and Adler, 1998, p. 124[50]). Additionally, some authors have suggested that they constitute a combination of personal learning spaces that are socially connected and provide a collaborative foundation for informal learning (McPherson, Budge and Lemon, 2015[51]). However, when you enter such spaces, neither learning nor knowledge creation are guaranteed. Instead, they provide an opportunity for informal, professional development by enabling individuals to engage into discussions with a wide variety of other individuals (Tynjälä, 2013[52]) and by stimulating them to critically reflect on their actions (Kolb, 1984[53]). We therefore argue that social networking sites constitute social opportunity spaces, which provide the meta-context wherein knowledge creation is fostered and learning processes are stimulated by the complex interplay of various underlying relations and factors (Spanhel, 2010[42]). Akkerman and Bakker (2011, p. 133[54]) have termed this possibility "boundary crossing", in order to describe a situation where individuals are enabled to expand their horizon and looking outside of their “narrow daily existence" (Williams, 2006, p. 600[55]). Lohman (2005, p. 505[56]) calls this process as "environmental scanning".
Yet, while previous research has already touched upon this topic, these studies can be criticised on the basis of three main issues. First, numerous studies have been conducted among students, and while these studies have provided valuable insights on the topic, research in the context of a wider audience and informal learning remains scarce. Second, previous research on SNS has largely dealt with Facebook and has neglected other SNS, such as Twitter. Third, research communities have started to use social networks to investigate patterns of relations among actors in education.
Yet, while networks are gaining interest in recent years, the intersection between educational innovation and social networks has received limited attention. This chapter addresses these shortcomings by investigating whether and how discussions about educational innovation can be traced and mapped on Twitter in a variety of international settings. Specifically, we were interested in possible difference between English-speaking countries (primarily the United States and the United Kingdom) and French-speaking countries (primarily France), as the applicable educational systems show clear differences in terms of organisational structures, financial endowment and characteristics such as the degree of internationalization (OECD, 2018[57]). The approach was then replicated for Spanish-speaking countries. This first of its kind exploration is meant to provide insights into the educational innovation space as well as suggest a provide a proof-of-concept for broader endeavour and investigation drawing on big data from Twitter at the international level.
The objectives was to identify the types of practices related to educational innovation in the social media space, what types of educational innovation stakeholders discuss, how the networks around different types of innovation relate to each other, and whether the mechanisms of introduction and diffusion of educational innovation in the social media space appear similar (or not) across countries.
Data
Twitter (http://www.twitter.com) is a free online global social network that combines elements of blogging, text messaging and broadcasting. Users write short messages limited to 280 characters, known as tweets, which are delivered to everyone who has chosen to receive that user’s tweets. This type of communication has greatly contributed to the ease and flexibility with which information can be shared among large groups of people, irrespective of time and place (Ye et al., 2012[58]). Within each tweet, it is possible to include links to other media or to embed video, images and hashtags (a word or a phrase prefixed with the symbol #). Including hashtags in tweets has become common practice on Twitter and allows individuals to include their contributions in a larger conversation about a certain topic, which enhances their possibility to access networks and further develop their already existing ones (Letierce et al., 2010[59]). Twitter users can interact and communicate in different ways, and users are finding new and creative ways to get the most out of each tweet. First, they can write simple messages, called tweets, adding images, videos, hashtags, etc. Second, tweets can be further disseminated when recipients repost them through their timelines. This technique, called retweeting, refers to the verbatim forwarding of another user’s tweet. A third type of messaging is a variant of tweeting and retweeting, called mentioning. Mentions include a reference to another Twitter user’s username, also called a handle, denoted by the use of the “@” symbol. Mentions can occur anywhere within a tweet, signalling attention or referring to that particular Twitter user.
To collect data on keywords related to educational innovation, we utilised a customised data collection tool developed by two of our co-authors (Daly and del Fresno), called Social Runner Lab. Social Runner Lab allowed us to download data in real time directly from Twitter’s Application Programming Interface (API) based on tweets using specified users, keywords, key phrases, or hashtags. Examples of the parameters include #eduinov, #edtech, #pedagogiesactives, @MPLS_national and @HBP_education. Using Twitter’s application programming interface (API), we conducted two data collection runs. First, we collected all tweets and user profile info from 15 June until 15 December 2019 as a pilot test run to determine proof-of-concept in this space. We captured Twitter profile names as well as the tweets, retweets, and mentions posted. Our data include messages that are public on Twitter, but not private messages between individuals, nor from accounts which users have made private or direct messages. Overall, this resulted in 643 332 Tweets. However, this included a wide variety of noisy data. Consequently, we applied filters to really focus on educational innovation and removing users that might skew the overall findings (e.g. Minister of Education in France). This filtering process resulted in a total of 168 534 tweets, of which 157 849 were from an English-speaking context and 10 685 were from a French-speaking context. Second, and using the experience from the initial proof-of-concept, we extended our analyses to also incorporate another globally spoken language, Spanish. This time, we captured data over a three-month period (mid-June 2020 to mid-September 2020). Departing from a search for the keyword education, we again used filter mechanisms to zoom in on innovation, which then resulted in a total of 4 389 tweets.
Social network analysis: methodology
Social network analysis
In order to determine the underlying structure of the Twitter conversations, we used social network analyses (also referred to as SNA). Social network analysis is grounded in the larger idea of social network theory (e.g. (Wasserman and Faust, 1994[60])) and draws on a set of metrics to examine the pattern of connections, or ties, between individuals that create a larger social network. This network forms a social structure of relationships, which can facilitate or inhibit an individual’s access to both physical and intellectual resources such as knowledge, ideas, and opinions (Daly et al., 2010[61]; Rehm et al., 2020[31]; Schlager et al., 2009[62]). This structure allows for analyses at the individual, dyad, small group, and overall network level and as such provides insights into patterns of interactions that are not readily visible (Scott, 2017[63]).
For the purpose of this study, we computed three commonly used Social Network Analysis indicators, namely in-, out-, and overall degree centrality of all users (nodes) taking part in the applicable discussions (Grabowicz et al., 2014[64]). In-Degree centrality measures how often a user is contacted by others. In the case of Twitter this translates into a user being mentioned or replied to by another user. Out-Degree centrality captures how often a user contributed to the discussion. In other words, how often she mentioned or replied to another user. It is important to note at this stage that Tweets do not provide explicit connections to others. If person A tweets something without mentioning or replying to person B, they do of course contribute to the discussion. However, as such a contribution is not directly targeted at another user, they do not contribute to the networks relational structure. Overall degree centrality combines in- and out-degree centrality and aggregates the two.
These metrics enable us to better understand whether there were actors that were more active in the social network. Having greater centrality in a network suggests an individual actor has disproportionate influence over the exchanges in that network and, as such, that his or her opinion carries more “weight”. In this context, we distinguish between three distinct types of actors, namely i) transmitters, ii) transceivers, and iii) transcenders (Fresno García, Daly and Segado Sánchez-Cabezudo, 2016[65]).
Transmitters are individuals who send out a large number of mentions and replies, which translates into a high out-degree. Out-degree is not related to the number of followers a transmitter has but is strictly a measure of how many applicable messages an individual posts.
Transceivers are a different kind of actor. More specifically, they receive a high degree of mentions and replies. This in turn will contribute to an individual’s in-degree centrality.
Transcenders are individuals that score high in both in- and out-degree centrality and can also be described as the “elite” of a network.
We were also interested in the inner structure and clustering of the interactions within the larger connected networks. Consequently, we ran a modularity community detection algorithm to identify and represent factions (a “faction” in this sense is a group with more ties within than across groups, although even those group boundaries are somewhat porous) (Newman, 2006[66]; Noack, 2009[67]). Within the identified communities, we then focused on individuals with prominent roles (highly degree centrality) in the network (e.g. Burt (2010[68]) and (Lee et al., 2014[69])). In the context of this study, we follow earlier work and consequently zoomed in on the top 1% of users for this particular type of consideration (Moukarzel, Rehm and Daly, 2020[70]; Moukarzel et al., 2020[71])2.
The collection of data from social media has sometimes raised questions of ethical concern among the research community. More specifically, some scholars are concerned about the confidentiality of information gathered from human subjects, as well as the public confidence and trust in researchers’ work (Koene et al., 2015[72]). While acknowledging the importance of these types of concerns, we are proponents of the work by, among others, Moreno and colleagues (2013[73]), who define a human subject as “a living individual about whom an investigator obtains data through interaction with the individual or identifiable private information” (p. 709[73]). Based on this definition, they argue that data from social media, particularly Twitter, qualifies as an exemption from usual guidelines and considerations about informed consent that apply to design research datasets. Participants generally use these types of platforms to publicly disseminate their thoughts, ideas and experiences. Consequently, as in our case, if researchers only collect publicly available data from social media, which requires no password to obtain, concerns about confidentiality and trust can be relaxed.
Hashtags & media
Tweets, Mentions and Replies do not only contain information about users. As indicated before images, videos, and hashtags are other prominent parts of tweets that are commonly used. Twitter data therefore constitutes a so-called “tripartite graph structure” (Halpin, Robu and Sheperd, 2007[74]), which is often found in the context of online collaborative platforms. In other words, Twitter contains data on i) users, ii) hashtags (which essentially are a categorising mechanism to assign Tweets to discussions) and iii) media (e.g. online resources, such as videos, blog posts, articles that are shared). We are then considering 2- and 3-mode network analyses in which there are two or all three types of data (Wasserman and Faust, 1994[60]). For the purpose of this work, we decided to focus on hashtags and media separately. In terms of hashtags, we first determined a 2-mode network of users and hashtags (Latapy, Magnien and Del Vecchio, 2008[75]). Here, relationships between individuals are not necessarily established based on direct contact (e.g. mentions or replies), but rather on the basis of discourse (e.g. unknowingly using the same hashtag). Based on this network, we were then able to construct a one-mode projection, which showed connections between hashtags. Similarly to our users’ analyses, we then also ran a community detection algorithm, in order to identify and represent clusters of hashtags that might represent a common topical focus. In terms of the media resources being shared, we aggregated the most commonly shared uniform resources locators (URLs) and considered exemplary cases of what was being shared. For the applicable analyses, we again used the combination of R and gephi to determine and produce the relevant results.
Results
In this section, we present the results of the analysis applied to the English and French discussions around educational innovation in the Twitter space. We present the English- (which proves to be mainly a US-) network structure, then the French-based network, before presenting the extension of the approach to the Spanish case. We then highlight some of the commonalities and differences among those three online spaces.
Analysis of the overall (English- and French-based) network
Before considering the specific cases of the English- and French-based Twitter network structures, Table 7.1 provides an overview of the main Social Network Analysis metrics. As can be seen, there is a high level of variance in the data sample. Additionally, by considering the quartiles, it becomes apparent that the distribution across all levels of degree is highly skewed, indicating that the majority of people were following the applicable hashtags while not pro-actively taking part (out-degree) or being overly included (in-degree) in the discussions. This is further emphasised by, on average, low levels for all three types of degrees. Finally, the high maximum values for the degrees indicated that there are key users that are at the centre of the discussions and really driving the discussions.
Figure 7.1 provides an overall sociogram merging both samples. As can be seen, while the English-based Twitter discussion related to educational innovation is considerably larger, in terms of users (dots) and connections (lines), both discussions show an overlap with each other (Figure 6.1b). This means that irrespective of any region-specific considerations and networks, there is a group of users that are linked across networks and therefore access information and contribute to the discussion of educational innovation. Zooming in on the most active users, based on their overall-degree centrality (Figure 7.2), we again see, as to be expected, more user accounts from the US-based discussion. However, we also discovered a small group of user accounts from the French-based discussion that appear to form their own community, are all interconnected with each other and also linked with central user accounts from the English-based discussion.
Table 7.1. Descriptive social network analysis statistics (Overall network)
|
In-Degree centrality |
Out-Degree centrality |
Overall Degree centrality |
---|---|---|---|
Mean |
1 584 |
1 584 |
3 168 |
StDev |
10 906 |
16 130 |
23 046 |
Quantiles |
|
|
|
1st |
0 |
0 |
1 |
2nd |
1 |
1 |
1 |
3rd |
1 |
1 |
2 |
4th |
1 325 |
1 925 |
2 718 |
Min |
0 |
0 |
0 |
Max |
1 325 |
1 925 |
2 718 |
Figure 7.1. Overall network: English- and French-based Twitter discussion
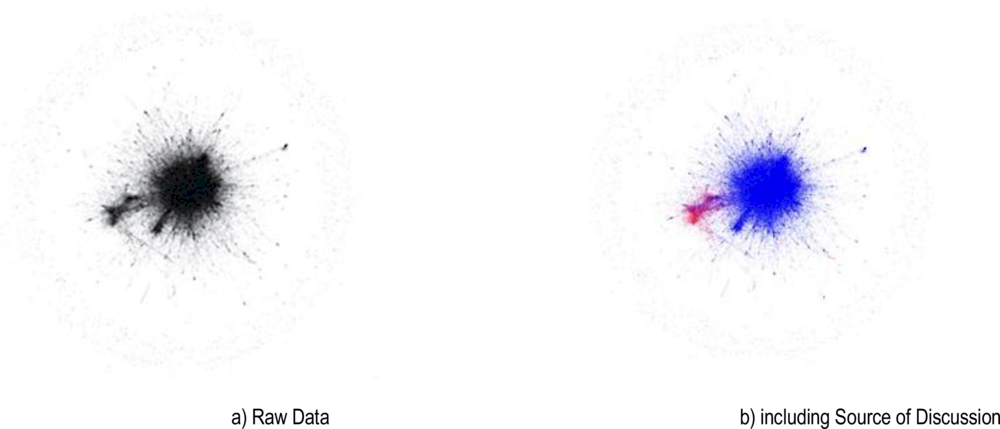
Note: Blue – English-based Twitter; Red: French-based Twitter
Note: Overall network: Users (Nodes): 66 701; Mentions & Replies (Edges): 142 062; Tweets: 168 534
Figure 7.2. Overall network (Top 1% overall-degree)
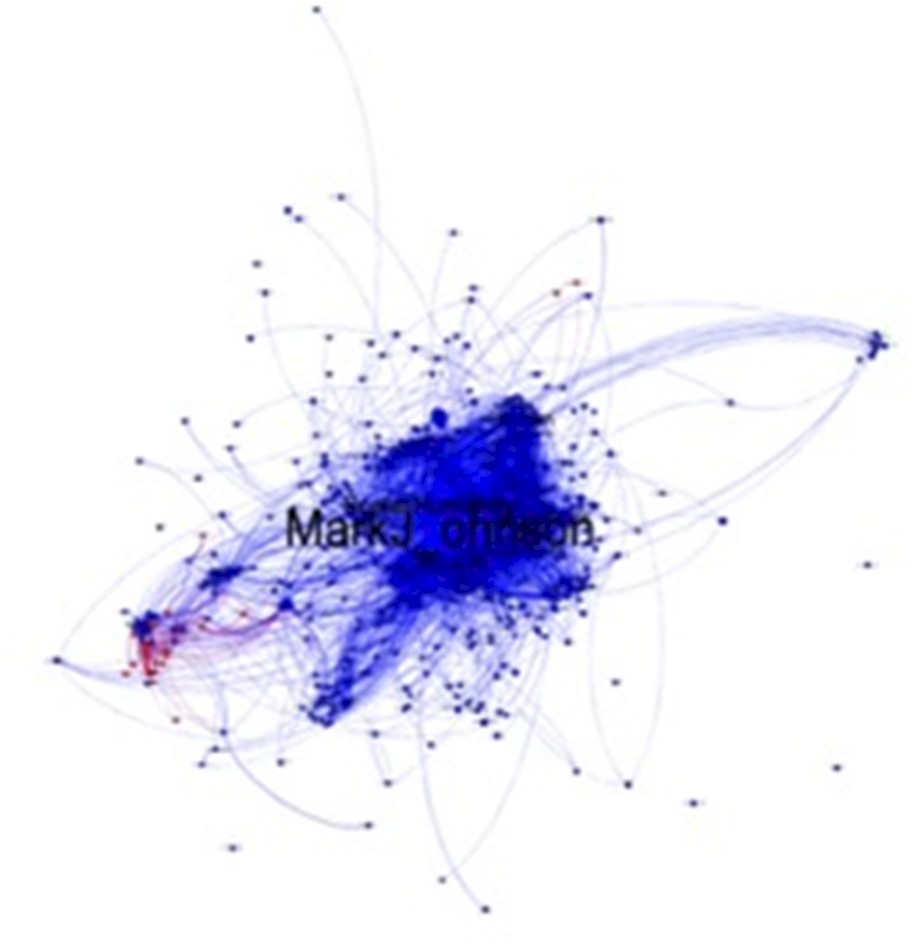
Note: Users (Nodes): 667; Mentions & Replies (Edges): 4.280; English-based Twitter: 71.73% Users; French-Based Twitter: 28.27% of Users
Analysis of English-based (US-) network
This section focuses on the English-based Twitter network, whose analysis shows that it is mainly a US-based network.
Users
The overall distribution of Tweets across the selected time period are visualised in Figure 7.3 below. As can be seen, while there has not been a constant level of interaction, there are a wide selection of peaks where the topic has been actively discussed by the applicable network.
Figure 7.3. Timeline: English

As a next step, we constructed sociograms for the entire network and determined factions using the chosen community clustering algorithm (Figure 7.4). Our findings suggest that there is a wide range of communities (communities are shown in differing colours) that are predominantly connected with each other, which is exemplified by the numerous connections between the communities (Figure 7.4). Moreover, as the algorithm identified all these different communities, we can preliminarily stipulate that these communities, while being connected, have something specific or unique about them. For example, it could be a certain group of people that already know each other offline, or a set of users that are particularly interested in a certain subtopic of educational innovation.
Based on the overall network structure, we determined the in-, out- and overall centrality metrics, in order to filter the sociograms and focus on the previously indicated three distinct types of actors, namely i) transmitters (Figure 7.5), ii) transceivers (Figure 7.6), and iii) transcenders (Figure 7.7).
Our results suggest that five Twitter accounts, from five different communities, have been particularly active in transmitting information (Figure 7.5). More specifically, @ScalarHumanity, @ericcurts, @MarkJ_ohnson, @mtholfsen, and @MynaEdu exhibit high out-degrees. On closer inspection, these accounts can be classified into personal accounts (@ericcurts, @MarkJ_ohnson, @mtholfsen) and organisational accounts (@ScalarHumanity, @MynaEdu). Moreover, when considering the indicated geographical regions of these accounts, all personal accounts appear to be based in the United States, while one organisational account indicates to be located in Australia (@MynaEdu).
When considering Transceivers (Figure 7.6) a similar picture emerges, with five accounts, from five communities, exhibiting significant, in this case, in-degree centrality. Here, @MarkJ_ohnson, @Alex_Corbitt, @MynaEdu, @ICTEvangelist, and @MindShiftKQED are noticeable. Interestingly, @MarkJ_ohnson and @MynaEdu appear again in this list. A closer look at the previously not mentioned accounts revealed again two personal accounts (@Alex_Corbitt and @ICTEvangelist) and one organisational account (@MindShiftKQED) that are US- (@Alex_Corbitt and @MindShiftKQED) and UK-based (@ICTEvangelist). Combining the two previous roles exhibiting high degrees, resulted in the Transcenders depicted in Figure 7.7. The applicable results suggest that the previously indicated users are really taking on an active role in the discussion about educational innovation (e.g. high out-degree) and are, as such, apparently also perceived as notable accounts by the community (e.g. high in-degree).
Figure 7.4. Overall network: English
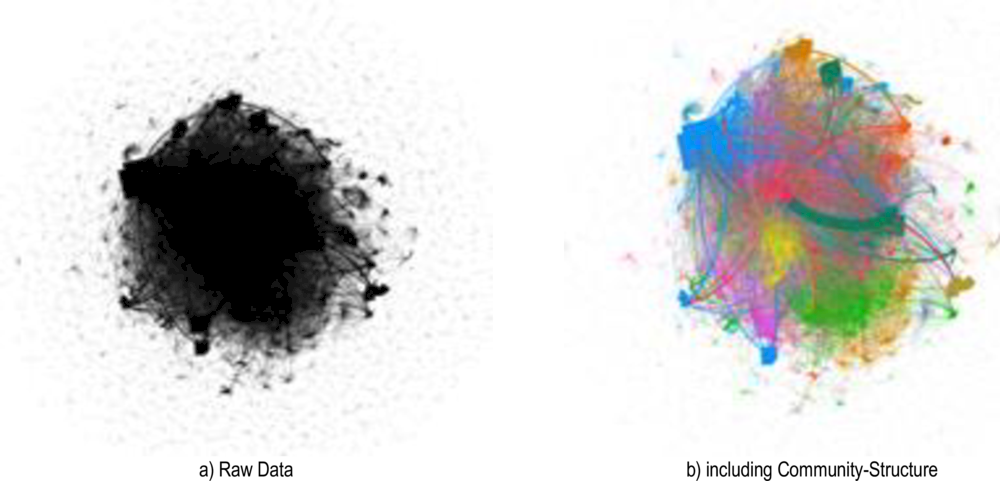
Note: Users (Nodes): 37 040; Mentions & Replies (Edges): 55 060
Figure 7.5. Out-degree network (Top 1 %): English (Transmitters)
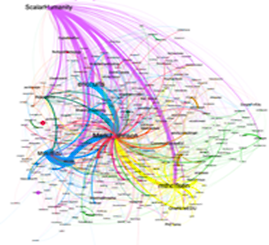
Note: Users (Nodes): 364; Mentions & Replies (Edges): 1 842
Figure 7.6. In-degree network (Top 1 %): English (Transceivers)
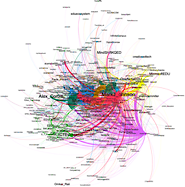
Note: Users (Nodes): 381; Mentions & Replies (Edges): 1 917
Figure 7.7. “Elite Network” high in- and out-degree (Top 1%): English (Transcenders)
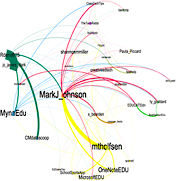
Note: Users (Nodes): 36; Mentions & Replies (Edges): 135
Table 7.2 summarises the applicable descriptive statistics that are depicted in Figures 7.5 to 7.7. Here, in addition to the already mentioned findings, a similar picture emerges as for the overall network. While the mean values are significantly higher than before, across all types of users, a noticeable degree of variance within the subsets remains. This is particularly pronounced in case of the Transcenders. Moreover, albeit less strongly pronounced, the data continues to be highly skewed. Again, this is mainly driven by a number of key users that putting considerable upward pressure on the means. This suggests that, while the consideration of Transmitters and Transceivers and Transcenders is a valuable tool to get a better picture of the underlying communication flows, the real core of the discussion is comprised of a still smaller group than the currently defined top one percent.
Table 7.2. Descriptive social network analysis statistics (English)
|
Transmitters |
|
Transceivers |
|
Transcenders |
||
---|---|---|---|---|---|---|---|
|
Out-Degree |
|
In-Degree |
|
In-Degree |
Out-Degree |
Overall Degree |
Mean |
55.06 |
|
46.66 |
|
181.58 |
309.67 |
491.25 |
StDev |
173.14 |
|
93.12 |
|
244.22 |
488.02 |
611.39 |
Quantiles |
|||||||
1st |
17 |
|
18 |
|
48.25 |
83 |
196 |
2nd |
22 |
|
24 |
|
111.5 |
129.5 |
224 |
3rd |
38.75 |
|
39 |
|
189.25 |
208 |
436.75 |
4th |
1 925 |
|
1 325 |
|
1 325 |
1 925 |
2 718 |
Min |
13 |
|
15 |
|
22 |
41 |
137 |
Max |
1 925 |
|
1 325 |
|
1 325 |
1 925 |
2 718 |
Hashtags
After mapping the network structure for the user accounts, we shifted our attention to the content of what was being tweeted. Figure 7.8 below represents the one-mode projection for the identified hashtags in the Tweets. Based on the chosen community detection algorithm, we are able to show “content communities” that seem to be interested in particular (combination of) sub-topics of educational innovation (as shown by the different coloured accounts nodes). When taking a closer look at the three largest “content communities” (Figure 7.9), we found that they cover three particular aspects that are related to educational innovation. The first, and largest community, deals with topics around science, technology, engineering and mathematics (STEM) (Figure 7.9a). The second community appears to have a focus on educational technology and how concepts like artificial intelligence, augmented-reality and virtual-reality can be used in education (Figure 7.9b). Finally, the third community covers topics around cybersecurity and ICT (infrastructure) (Figure 7.9c).
Figure 7.8. Overall hashtag network: English (including community-structure)
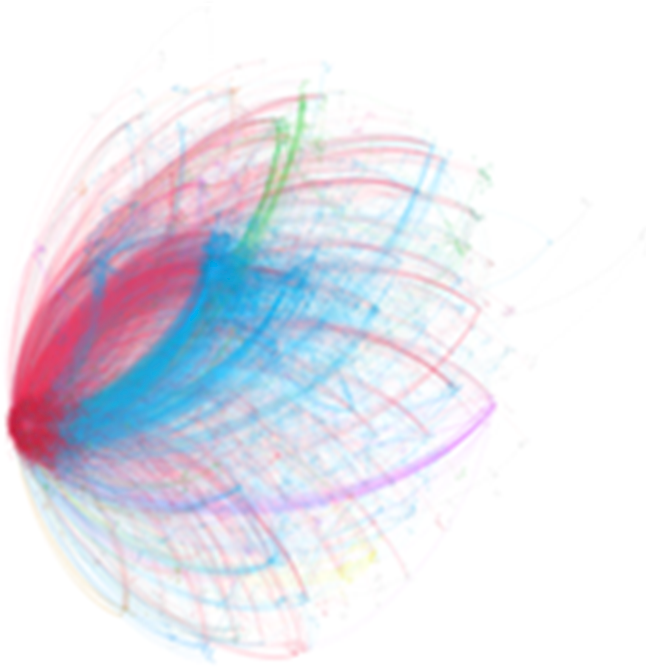
Note: Hashtags (Nodes): 11.202; Connections (Edges): 47.091
Figure 7.9. Hashtag networks: English (Top communities)
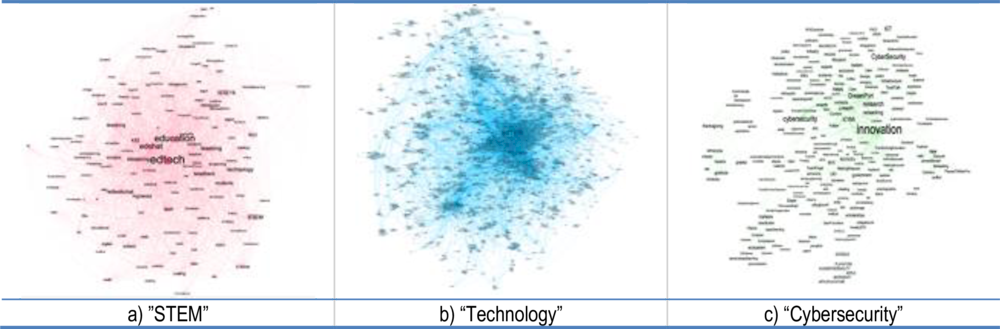
Note: „STEM“: Hashtags (Nodes): 6 819, Connections (Edges): 25 486; „Technology“: Hashtags (Nodes): 3 355, Connections (Edges): 9 301; „Cybersecurity“: Hashtags (Nodes): 255, Connections (Edges): 511.
Table 7.3 shows the most commonly used hashtags and allows to unpack the hashtag communities a bit more. Educational technology (#edtech, #EdTech and #Edtech) has a strong presence and makes up 8.79% of all hashtags. This is remarkable, as it is about triple the amount of activity the second largest hashtag has been included, namely education (#education and #Education) is engaged.
Table 7.3. Most commonly used hashtags (English)
Rank |
Hashtag |
Degree |
Rank |
Hashtag |
Degree |
|
---|---|---|---|---|---|---|
1 |
edtech |
5 917 |
11 |
AI |
424 |
|
2 |
education |
2 099 |
12 |
teachers |
419 |
|
3 |
EdTech |
1 876 |
13 |
ISTE19 |
386 |
|
4 |
edchat |
1 125 |
14 |
innovation |
381 |
|
5 |
Education |
773 |
15 |
edtechchat |
377 |
|
6 |
Edtech |
632 |
16 |
tech |
341 |
|
7 |
learning |
527 |
17 |
k12 |
327 |
|
8 |
STEM |
458 |
18 |
teaching |
312 |
|
9 |
elearning |
426 |
19 |
highered |
291 |
|
10 |
technology |
425 |
20 |
students |
290 |
Media/URLs
The preliminary analysis of the hashtags already suggested some more specific topics that were prevalent in the context of the English-based Twitter discussion. Table 7.4 presents the most frequently shared URLs per defined type of user and indicates a considerable amount of overlap between the three categories. Based on how the three types of users are defined, this was to some extend to be expected, as particularly the Transcenders will, by definition, include a range of users from the other two categories. Moreover, on closer inspection, some nuanced differences can be observed. While Transmitters are the only ones sharing resources from “educatorstechnology.com” (a platform for educational web tools and apps for teachers), Transceivers’ unique top URL is from “ andrewscampbell.com” (the blog of a Canadian educator and writer), and Transcenders are unique in sharing the URL “freetech4teachers.com” (the website of a US high school computer science teacher).
Table 7.4. Most frequently shared URLs per type of user (English)
|
Transmitters |
|
Transceivers |
|
Transcenders |
|||
---|---|---|---|---|---|---|---|---|
Rank |
Domain |
N |
|
Domain |
N |
|
Domain |
n |
1 |
controlaltachieve.com |
211 |
|
controlaltachieve.com |
210 |
|
controlaltachieve.com |
211 |
2 |
paper.li |
85 |
|
paper.li |
86 |
|
paper.li |
86 |
3 |
eraser |
49 |
|
rdene915.com |
45 |
|
eraser |
49 |
4 |
rdene915.com |
45 |
|
medium.com |
36 |
|
rdene915.com |
45 |
5 |
medium.com |
36 |
|
edsurge.com |
29 |
|
medium.com |
37 |
6 |
edsurge.com |
27 |
|
docs.google.com |
14 |
|
edsurge.com |
31 |
7 |
educatorstechnology.com |
24 |
|
amazon.com |
14 |
|
educatorstechnology.com |
24 |
8 |
amazon.com |
15 |
|
anchor.fm |
13 |
|
amazon.com |
14 |
9 |
anchor.fm |
13 |
|
youtube.com |
8 |
|
anchor.fm |
13 |
10 |
docs.google.com |
12 |
|
andrewscampbell.com |
6 |
|
freetech4teachers.com |
13 |
In order to shed some more light on this issue, we also looked at a sample of URLs and media that were shared within the Tweets. Some exemplary screenshots are provided in Figure 7.8. As can be seen, the shared resources deal with coding (Figure 7.10a), virtual reality (Figure 7.10b), educational transformation (Figure 7.10c) and recommendations on how to use e.g. Twitter in (high school) education (Figure 7.10d).
Figure 7.10. Screenshots of most commonly shared media/URLs: English
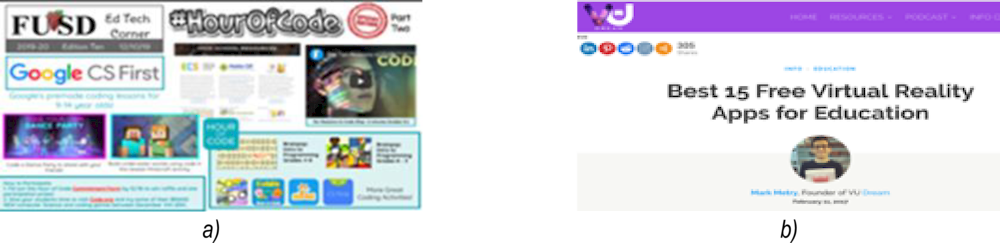
Measuring educational innovation through social media: An international exploration in the Twittersphere
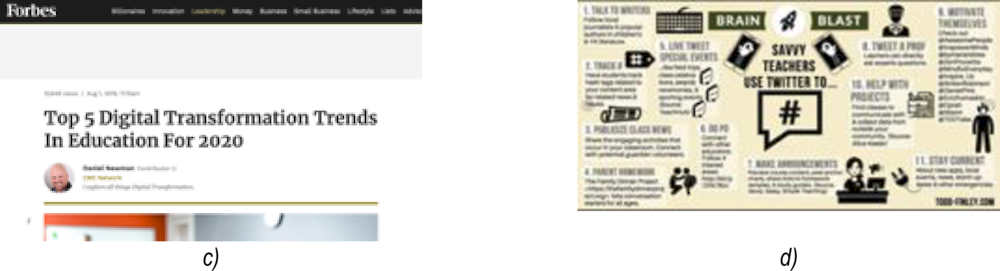
Source: Fontana Unified School District (a); https://markmetry.medium.com/the-best-15-free-virtual-reality-apps-for-education-29b4e68a2917 (b); Forbes (c); Todd Finley (@finleyt) (d).
Summary
Our analysis of discussions on educational innovation in the English-speaking Twitter space shows that, in 2019, those exchanges were mainly driven by a small community located in the United States, that its intensity fluctuated over time, with periodic peaks, rather than being a constant stream of discussion. The analysis of the content of those discussions allowed to identify three main “communities”: the largest one discussed topics around science, technology, engineering and mathematics (STEM), then education technology, and finally cybersecurity.
Analysis of French-based network
This section focuses on the French-based Twitter network.
Users
Similarly to the case of the English-based Twitter discussion on educational innovation, in the French case there also is not a constant level of interaction (Figure 7.11). Instead, the observed interaction is subject to regularly occurring communication peaks.
Figure 7.11. Timeline: French

Another similarity between the English and French-based Twitter discussions becomes apparent when considering the sociograms presented in Figure 12 below. Again, we discovered a wide range of communities that are predominantly connected with each other. Departing from these overall network findings, we then also considered i) transmitters (Figure 7.13), ii) transceivers (Figure 7.14), and iii) transcenders (Figure 7.15). Again, focusing on the five Twitter accounts that have been particularly active in transmitting information (Figure 7.13), revealed two types of accounts. However, in contrast to the English-based discussion, there was only one organisational account (@EdtechFrance), while the remaining four accounts (@mdrechsler, @AxelJean77, @DuchanoisG, @FrancoisTaddei) were personal accounts. Moreover, the profile descriptions suggest that, while all accounts appear to be located in France, there tends to be a geographical focus on the country’s capital city Paris. When considering Transceivers (Figure 7.14), we again find five accounts from five communities that exhibiting significant, in this case, in-degrees. While the communities stay the same, the representatives of these community somewhat change. More specifically, @EdtechFrance, @mdrechsler, @FrancoisTaddei continue to be notable users accounts (from the red, yellow and blue community in Figure 7.14, respectively). However, in the green community @lab110bis (an innovation lab) has taken the top position and @diversifier (an author in educational innovation) in the purple community. Considering the role of Transcenders (Figure 7.15), we found similar results as in the US-based discussion, with previously indicated users again taking on active roles and being perceived accordingly by the community.
Figure 7.12. Overall network: French
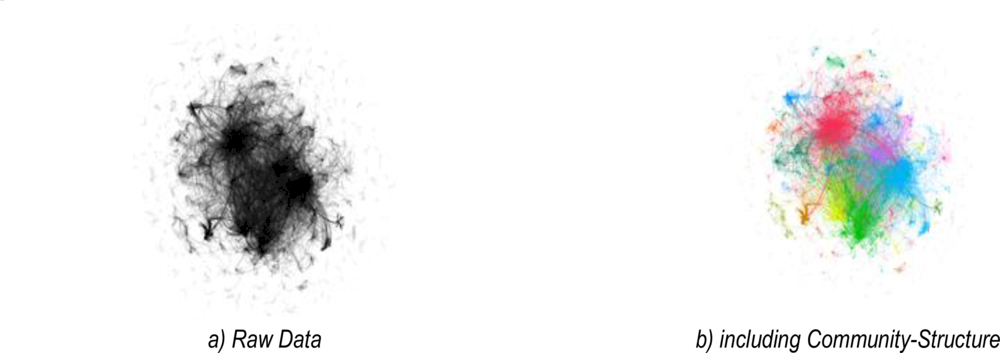
Note: Users (Nodes): 5.290; Mentions & Replies (Edges): 13.941
Figure 7.13. Out-degree network (Top 1%): French (Transmitters)
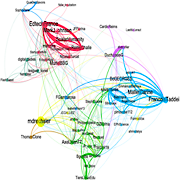
Note: Users (Nodes): 54; Mentions & Replies (Edges): 333
Figure 7.14. In-degree network (Top 1%): French (Transceivers)
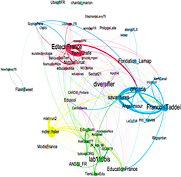
Note: Users (Nodes): 53; Mentions & Replies (Edges): 247
Figure 7.15. “Elite Network” – high in- and out-degree (Top 1%): French (Transcenders)
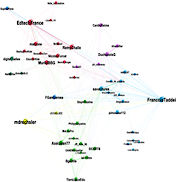
Note: Users (Nodes): 100; Mentions & Replies (Edges): 420
Table 7.5 presents the descriptive statistics for the three types of users. As before, while the overall level of communication is somewhat lower in the French-based data sample, we again see a very similar story as in the English-based data. Again, there remains to be a noticeable degree of variance within the subsets and a highly skewed distribution of all levels of degree. Interestingly, there seems to be a difference in the composition of the overall degree when comparing this data with the English-based version. More specifically, while within the French-based network, the role of the Transceivers is more pronounced (as indicated by a higher mean in-degree), the English-based data suggests that the Transmitters are more active (as underlined by a higher mean out-degree; see Table 7.3). Hence despite the similarities there also appears to be a noticeable difference in the structure of the underlying networks.
Table 7.5. Descriptive social network analysis statistics (French)
|
Transmitters |
|
Transceivers |
|
Transcenders |
||
---|---|---|---|---|---|---|---|
|
Out-Degree |
|
In-Degree |
|
In-Degree |
Out-Degree |
Overall Degree |
Mean |
22.89 |
|
68.63 |
|
43.63 |
18.79 |
62.42 |
StDev |
16.64 |
|
101.37 |
|
99.68 |
17.08 |
102.90 |
Quantiles |
|
|
|
|
|
|
|
1st |
15 |
|
29.5 |
|
11 |
11 |
25.75 |
2nd |
17 |
|
40 |
|
19.5 |
14 |
33 |
3rd |
25 |
|
64 |
|
36.25 |
22 |
60.25 |
4th |
152 |
|
904 |
|
904 |
152 |
930 |
Min |
13 |
|
24 |
|
5 |
9 |
18 |
Max |
152 |
|
904 |
|
904 |
152 |
930 |
Hashtags
Figure 7.16 visualises the one-mode projection of identified hashtags within the Tweets. The most commonly used hashtags are summarised in Table 6. In contrast to the English-based sample, here we found a larger number of “content communities”, covering i) ”EdTech” (Figure 7.17a), ii) “Education” (Figure 7.17b), iii) “Innovative (School) Projects” (Figure 7.17d), iv) “massive open online courses (MOOCs)” (Figure 7.17e), iv) “Inclusion” (Figure 7.17f) and v) a “Canadian (Weekly) Chat” (Figure 7.17c). The latter is particularly interesting as it extends the otherwise supposedly European discussion into North America.
Figure 7.16. Overall hashtag network: French (including community-structure)
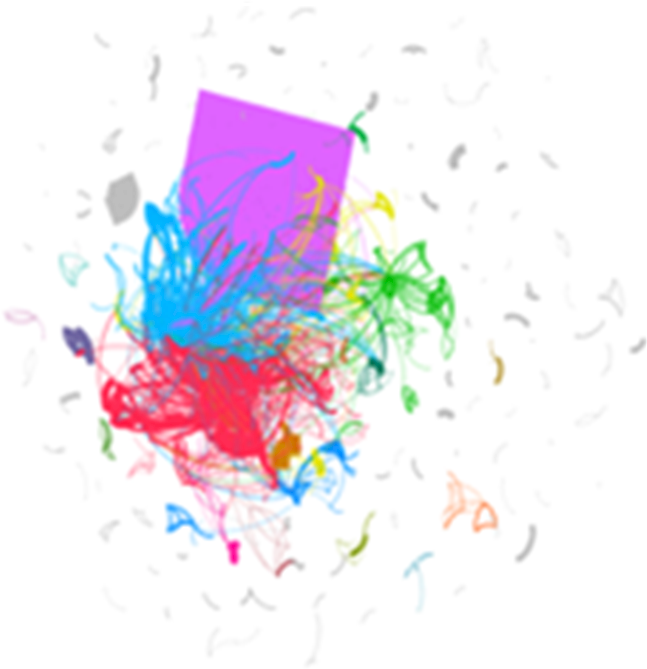
Note: Hashtags (Nodes): 1.122, Connections (Edges): 2.160
Figure 7.17. Hashtag networks: French (for Top 6 communities)
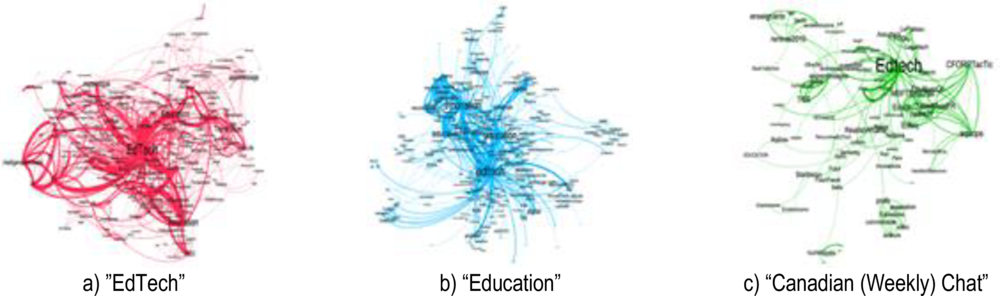
Measuring educational innovation through social media: An international exploration in the Twittersphere
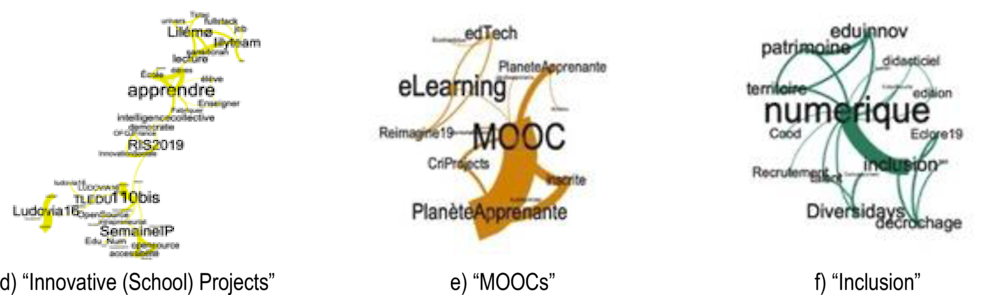
Note: „EdTech “: Hashtags (Nodes): 283, Connections (Edges): 597; „Education“: Hashtags (Nodes): 271, Connections (Edges): 572; „Canadian (Weekly) Chat“: Hashtags (Nodes): 84, Connections (Edges):171; „ Innovative (School) Projects“: Hashtags (Nodes): 44, Connections (Edges): 55; „ MOOCs “: Hashtags (Nodes): 55, Connections (Edges): 87; „Inclusion“: Hashtags (Nodes): 34, Connections (Edges): 44.
Table 7.6 provides an overview of the most commonly used hashtags from the French-based data sample. Again, as compared to the English-based sample, educational technology is the most commonly used and dominant hashtag. Interestingly, innovation and educational innovation rank higher as compared to the English-based case, suggesting a more prevalent explicit use of the innovation notion in the French-speaking educational sphere. Discussions around education technology (#edtech, #EdTech and #Edtech), the digital transformation (#numerique, #TransfoNum, #digital) and AI (#IA, #AI) show the strong association of innovation to education technology. Furthermore, hashtags like #formation, #education and #apprendre suggest a higher level of consideration for how to implement (educational) innovation in practice. The IDEFI refers to the excellence initiative in innovating training, a large competition funded by the French government.
Table 7.6. Most commonly used hashtags (French)
Rank |
Hashtag |
Degree |
|
Rank |
Hashtag |
Degree |
---|---|---|---|---|---|---|
1 |
edtech |
194 |
|
11 |
Formation |
31 |
2 |
EdTech |
189 |
|
12 |
IDEFI |
27 |
3 |
Education |
86 |
|
13 |
Eduinov |
26 |
4 |
innovation |
79 |
|
14 |
TransfoNum |
25 |
5 |
Edtech |
72 |
|
15 |
digital |
21 |
6 |
education |
67 |
|
16 |
apprentissage |
20 |
7 |
eduinov |
55 |
|
17 |
Startup |
19 |
8 |
Innovation |
49 |
|
18 |
AI |
18 |
9 |
numerique |
48 |
|
19 |
IntelligenceArtificielle |
16 |
10 |
IA |
37 |
|
20 |
Apprendre |
16 |
Note: English translations of the hashtags: numérique: digital; Formation: Training; IDEFI: Innovation competition in France; TransfoNum: Digital transformation: Intelligence Artificial: AI; apprendre: to learn.
Media/URLs
Finally, we again considered a sample of URLs and media that were shared within the French-based Twitter discussion. Table 7.7 shows the most frequently shared URLs among the three defined types of users. Similarly to the English-based sample, we again identified a considerable amount of overlap between the three categories. Even more so, Transceivers and Transcenders even have the same frequency of shares among the top 10 URLs. This is likely to be related to the fact that, again the two types of groups will inherently have an overlap, and because the amount of active users is comparatively small compared to the English-based sample. Consequently, the same information is more likely to make the rounds. Interestingly, the URLs being shared represent a mix of English and French resources. Moreover, in the latter case, the resources are both seemingly from France and Canada, which underlines the findings from the applicable hashtag analyses.
Table 7.7. Most frequently shared URLs per type of user
|
Transmitters |
|
Transceivers |
|
Transcenders |
|||
---|---|---|---|---|---|---|---|---|
Rank |
Domain |
N |
|
Domain |
N |
|
Domain |
N |
1 |
paper.li |
34 |
|
rire.ctreq.qc.ca |
10 |
|
rire.ctreq.qc.ca |
10 |
2 |
ici.radio-canada.ca |
9 |
|
edutopia.org |
10 |
|
edutopia.org |
10 |
3 |
les-savanturiers.cri-paris.org |
9 |
|
les-savanturiers.cri-paris.org |
9 |
|
les-savanturiers.cri-paris.org |
9 |
4 |
edtechfrance.fr |
4 |
|
ecolebranchee.com |
8 |
|
ecolebranchee.com |
8 |
5 |
podcasts.apple.com |
4 |
|
carrefour-education.qc.ca |
5 |
|
carrefour-education.qc.ca |
5 |
6 |
lapresse.ca |
4 |
|
ludomag.com |
5 |
|
ludomag.com |
5 |
7 |
ecolebranchee.com |
3 |
|
weareteachers.com |
5 |
|
weareteachers.com |
5 |
8 |
business.lesechos.fr |
2 |
|
edcan.ca |
4 |
|
edcan.ca |
4 |
9 |
outilstice.com |
2 |
|
kqed.org |
4 |
|
kqed.org |
4 |
10 |
cpformation.com |
2 |
|
youtube.com |
4 |
|
youtube.com |
4 |
Figure 7.18 provides some exemplary screenshots. Interestingly, the topics differ noticeably from the English-based discussion. More specifically, in this particular context we found shared resources that deal with a call for applications for the national day of educational innovation, an event organised by the French ministry of education that typically highlights educational innovation by awarding innovation prizes (Figure 7.18a), a critical reflection about educational innovation, in general, and educational technology, in particular (Figure 7.18b), practical considerations from instructional design theories (Figure 7.18c) and platforms to exchange best educational practices, supported by the French ministry of higher education (Figure 7.18d). Indeed, more resources relate to governmental initiatives compared to the US examples.
Figure 7.18. Screenshots of most commonly shared media/URLs: French
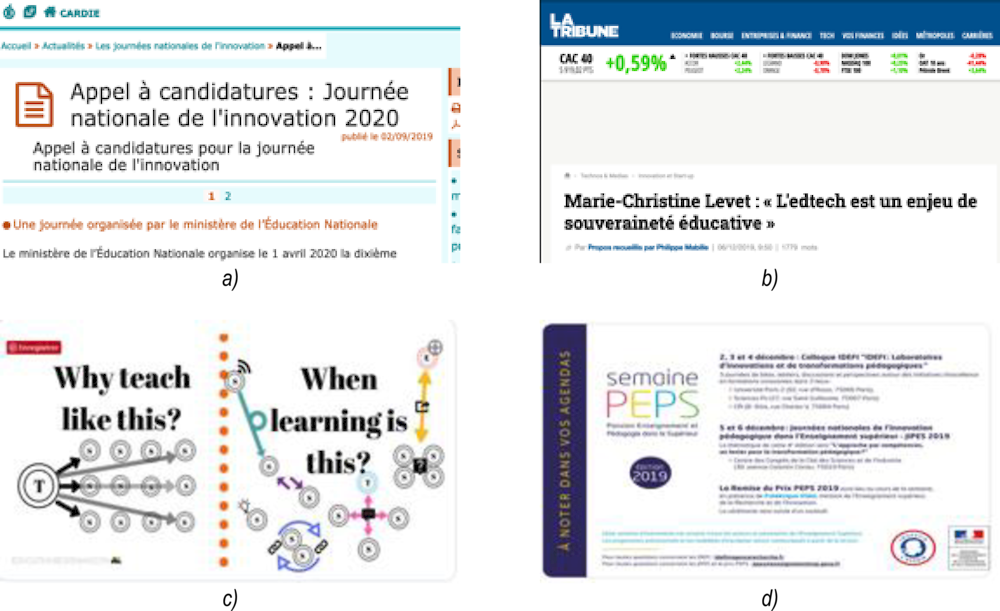
Source: French Ministry of Education (Ministère de l'Éducation nationale) (a); La Tribune (b) ; Education Rickshaw, https://educationrickshaw.com/2017/12/02/after-100-years-of-the-same-teaching-model-its-time-to-throw-out-the-playbook/ (c) ; French Ministry of Higher Education and Science (Ministère de l’enseignement supérieur, de la recherche et de l’innovation) (d).
Summary
Our analysis of discussions on educational innovation in the French-speaking Twitter space shows that, in 2019, those exchanges were much less frequent than in the English space, mainly driven by occasional exchanges in France and Canada, but with more clearly identifiable “content” communities. While digital technology and transformation are key aspects of discussions on innovation, more non-technological exchanges also occurs, for example around innovative school projects or governmental competitions on pedagogical innovation.
Extension of the original analyses to Spanish-based network
In order to explore another Twitter space but also to test the replicability of our initial analyses to other languages, in 2020 we replicated a similar approach using Spanish terms.
Users
In the Spanish-based sample, using the broad search term “education”, resulted in 1 269 million unique tweets from 592 421 unique authors. A subsequent filtering limiting the query to (innovation OR innovation) AND (education OR education)3 produced a data sample of 4 389 unique Tweets and 2 663 unique authors. The applicable distribution of Tweets across time is shown in Figure 7.19, below4. In comparison to the analysis we did in the English and French based samples, we again found considerable fluctuations in communication. Moreover, the discussion about educational innovation specifically, was less pronounced than the overall discussion about education.
Figure 7.19. Timeline: Spanish
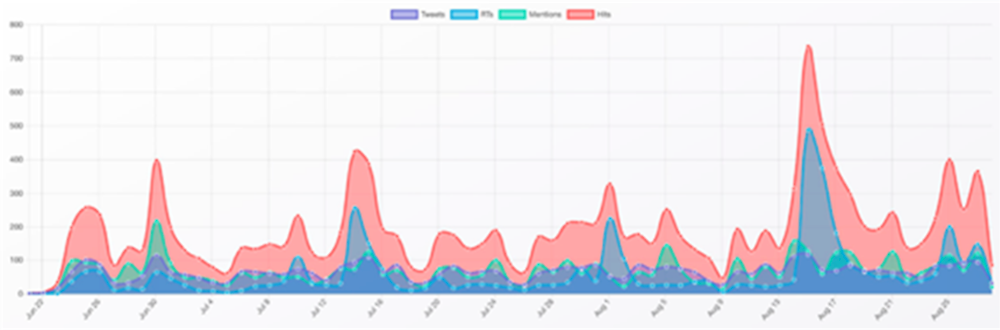
Table 7.8. Descriptive social network analysis metrics (Spanish)
|
In-Degree |
Out-Degree |
Overall Degree |
---|---|---|---|
Mean |
1,075 |
1,075 |
2,151 |
StDev |
7,503 |
2,088 |
7,990 |
Quantiles |
|
|
|
1st |
0 |
0 |
1 |
2nd |
0 |
1 |
1 |
3rd |
1 |
1 |
2 |
4th |
376 |
59 |
386 |
Min |
0 |
0 |
0 |
Max |
376 |
59 |
386 |
Another similarity between the English- and French-based Twitter discussions is exemplified by the results provided in Table 7.8 and Figure 7.20. Table 7.8 summarizes the main network metrics. Similarly to the English and French cases, the discussion in the Spanish case was subject to noticeable variance in frequency and highly skewed. The latter is underlined by the results on the quartiles and again suggests that only a small percentage of users was heavily invested in pro-actively joining and contributing to the discussions. The sociograms in Figure 7.20 visualise again the existence of a wide range of communities. Yet, in the Spanish case these communities are predominantly not connected with each other. The most probable cause for this observation of lower structural cohesion is related to the fact that the Spanish language is an extensive community of 21 countries with very different types of social structures, educational systems and different rates of Internet access. This is also true for English and French, but in these cases the findings ended up clearly zeroing in on the higher income countries speaking the languages.
Figure 7.20. Overall network: Spanish
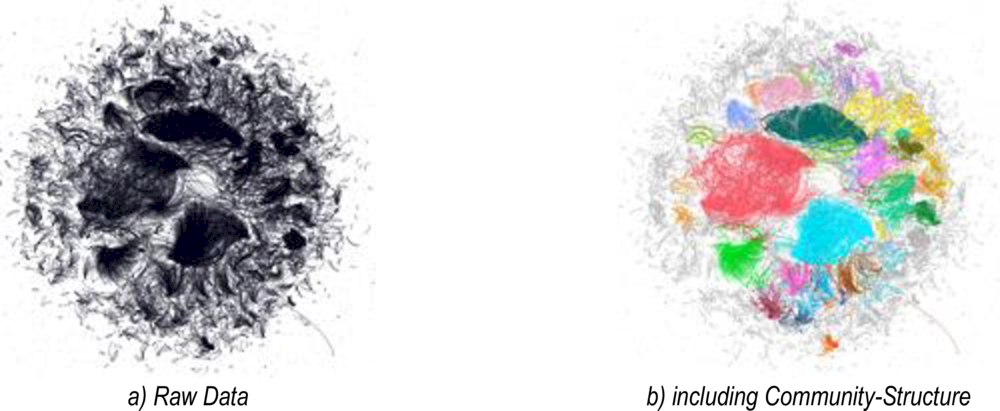
Note: Users (Nodes): 8 637; Mentions & Replies (Edges): 11 566.
Departing from these overall network findings, we then also considered again i) transmitters (Figure 7.21), ii) transceivers (Figure 7.22), and iii) transcenders (Figure 7.23).
In the top 1% of the transmitters network (Figure 7.21), although there are a greater number of prominent individual nodes than in other languages, there are also fewer relationships between them. The possible explanation for the multiplicity of countries also seems to be the most reasonable explanation in this case. Among the most prominent profiles of this network were @Claudiashein (Head of Government of Mexico City 2018-2024), @nefeerr (Spanish, human rights activist), @somosroliev (a network of Latin American organisations interested in innovating in education), @enlight_ED (an international network of Education, Innovation and EdTech promoted by @FundacionTef, @IEuniversity, @fundsantillana and @south_summit), @asociacionminu (an Argentine association for the development of projects developed by young people, for young people and projects educational programs that promote participation and inclusion), @MovimientoSTEAM (initiative in Mexico to promote innovation and the development of skills to compete in the 21st century world of work), and @Reynaldo_VZ (Cuban university academic leader). Again, these short descriptions of the applicable users accounts clearly highlight the equal dispersion of the discussions across several countries (or networks) of the Spanish language. As in the French-based sample, we see first indications that the overall data can be subdivided into the subsets that appear to be driven by geographical regions (e.g. Spain, Mexico, Chile and other South American countries).
Figure 7.21. Out-degree network (Top 1%): Spanish (Transmitters)
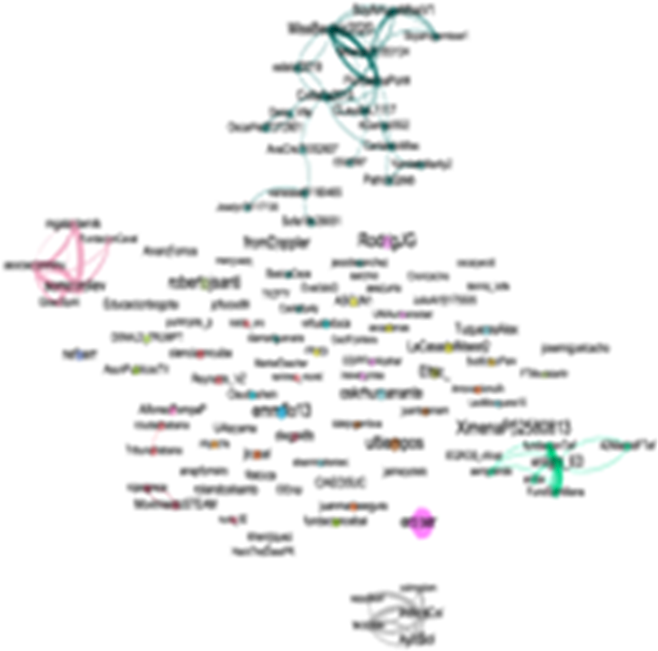
Note: Users (Nodes): 109; Mentions & Replies (Edges): 96
Considering the top 1% of Transceivers network (Figure 7.22), we again discovered different regional cliques. Furthermore, users like @Claudiashein (Head of Government of Mexico City 2018-2024) were again central to the discussion. Additionally, @omarfayad (Constitutional governor of the Mexican state of Hidalgo), @JorgeLuisPerd20 (Minister of Communications of the Republic of Cuba), @InesMChapman (Vice Prime Minister of the Republic of Cuba), @nefeerr (Spanish, human rights activist), and @fpaisdigital (Chilean foundation for development and the dissemination of a digital culture to improve people's quality of life) were among the other central users from the discussion. Interestingly, in comparison to the English and France cases a closer inspection confirms our earlier finding that the individual communities were less frequently connected. Even more so, the central users are also less likely to connect with each other, as compared to the English- and French-based samples.
Finally, Figure 7.22 provides a sociogram that zooms in on the Transcenders network. As to be expected, we found very similar accounts as in the previous two types of networks. In addition, the notion of the geographic fragmentation was again supported. This suggests that issues related to education and innovation are under the control and influence of small cliques and likely country-determined, which results in a less interconnected network, and seems to reflect interactions deeply rooted in the local education systems and its challenges in the context of educational innovation.
Figure 7.22. In-degree network (Top 1%): Spanish (Transceivers)
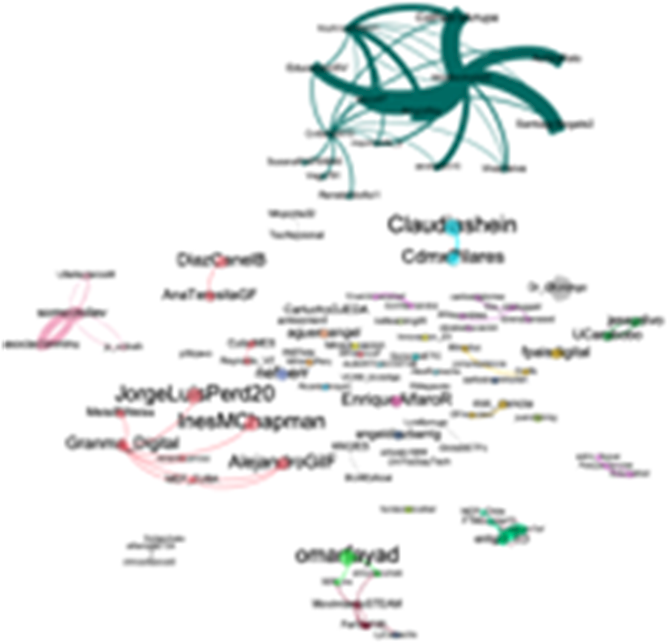
Note: Users (Nodes): 94; Mentions & Replies (Edges): 94
Figure 7.23. “Elite Network” – high in- and out-degree (Top 1%): Spanish (Transcenders)

Note: Users (Nodes): 83; Mentions & Replies (Edges): 177.
Table 7.9 provides the underlying descriptive Social Network Analysis metrics for Figures 7.21 to 7.23. As in the English and French cases, the mean values are significantly higher than for the overall network, we continued to observe a considerable degree of variance, and the data remained heavily skewed. Interestingly, the variance within the group of Transcenders for the out-degree was comparatively low, which suggests a commonality among the applicable users in terms of communicative behaviour. This is even more interesting as we already discovered that these users were separated within the overall discussion based on country location and seemingly content-related terms.
Table 7.9. Descriptive social network analysis statistics – Types of user (Spanish case)
|
Transmitters |
|
Transceivers |
|
Transcenders |
||
---|---|---|---|---|---|---|---|
|
Out-Degree |
|
In-Degree |
|
In-Degree |
Out-Degree |
Overall Degree |
Mean |
14,97 |
|
41,21 |
|
13,06 |
12,26 |
25,32 |
StDev |
10,15 |
|
62,70 |
|
43,13 |
8,08 |
44,01 |
Quantiles |
|
|
|
|
|
|
|
1st |
10 |
|
14 |
|
2 |
8 |
12 |
2nd |
11 |
|
18 |
|
4 |
10 |
16 |
3rd |
14 |
|
33,75 |
|
10 |
13 |
25 |
4th |
59 |
|
376 |
|
376 |
59 |
386 |
Min |
8 |
|
12 |
|
1 |
7 |
8 |
Max |
59 |
|
376 |
|
376 |
59 |
386 |
Hashtags
Figure 7.24 visualises the one-mode projection of identified hashtags within the Tweets. The most commonly used hashtags are summarised in Figure 7.25. Here, in contrast to the French case but similar to the English one, we found a limited number of “content communities”, covering and dominating the central topics of innovation and education, followed with a mixed network of hashtags in which both English and Spanish hashtags were used. The third and fourth big communities are related with education technology companies and the digital transformation in education, but also with business management, leadership and entrepreneurship.
Figure 7.24. Overall hashtag network: Spanish (including community-structure)
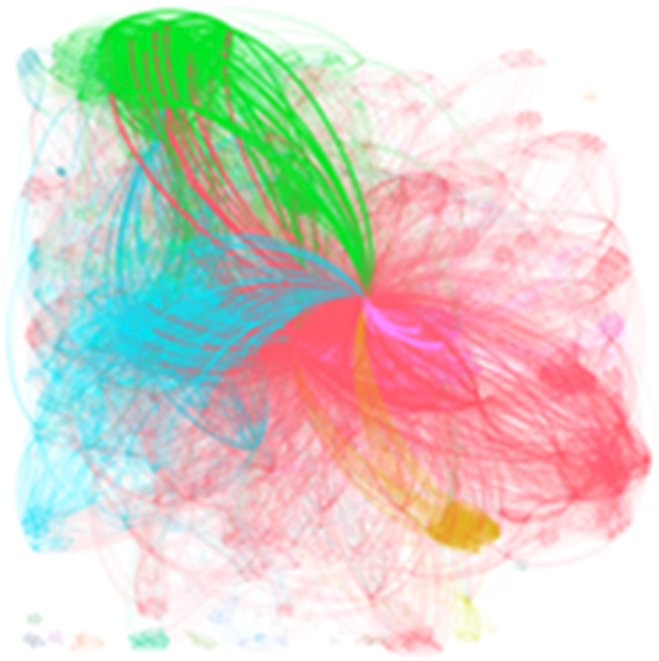
Note: Hashtags (Nodes): 2 282, Connections (Edges): 20 834.
Figure 7.25. Hashtag networks: Spanish (for Top 4 communities)
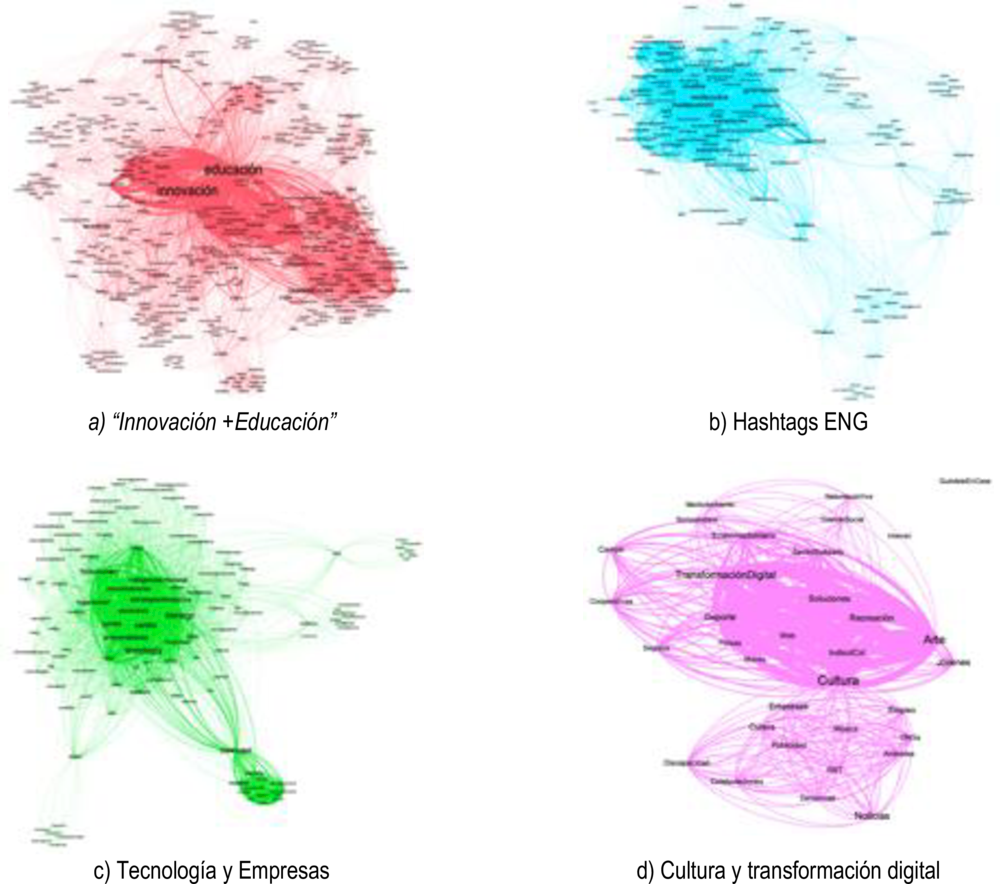
Note: „Innovación + Educación“ (innovation and education): Hashtags (Nodes): 401, Connections (Edges): 5 708; „Hashtag ENG“: Hashtags (Nodes): 144, Connections (Edges): 2 333; „Tecnología y Empresas“ (technology and companies): Hashtags (Nodes): 116, Connections (Edges):2 309; „ Cultura y transformación digital“ (digital culture and Transformation): Hashtags (Nodes): 44, Connections (Edges):490.
Table 7.10 shows the most commonly used hashtags in the Spanish case, allowed to attain a more nuanced view of the larger community structures. As can be seen, innovation and education are among the most commonly used hashtags, which was to be expected given our search terms – but was not the case in the English case. We also discovered similarities with the English- and French-based samples, as different aspects of technology were also commonly discussed. Interestingly, and this constitutes a noticeable difference to the English and French cases, there was a stronger focus on topics such as change (e.g. #cambio), leadership (e.g. #liderazgo) and experiences (e.g. #experiencias). A clear subset of the discussions related not only to innovation in education but also to educating for innovation, that is, how to prepare students for entrepreneurship and business management.
Table 7.10. Hashtag networks: Spanish (for Top 3 communities)
Rank |
Hashtag |
Degree |
Rank |
Hashtag |
Degree |
---|---|---|---|---|---|
1 |
innovación |
2552 |
16 |
comunicación |
170 |
2 |
educación |
2544 |
17 |
InteligenciaEmpresarial |
168 |
3 |
tecnología |
346 |
18 |
estrategiasdenegocios |
166 |
4 |
Liderazgo |
262 |
19 |
COVID19 |
154 |
5 |
innovation |
256 |
20 |
formación |
154 |
6 |
Tecnología |
254 |
21 |
TIC |
146 |
7 |
education |
228 |
22 |
Experiencias |
146 |
8 |
cambio |
226 |
23 |
Aprendizaje |
142 |
9 |
Elearning |
224 |
24 |
emprendimiento |
142 |
10 |
QuedataEnCa |
206 |
25 |
Escuela |
138 |
11 |
creatividad |
200 |
26 |
RedesSociales |
138 |
12 |
pymes |
192 |
27 |
EDreform |
136 |
13 |
emprendedores |
184 |
28 |
Universidad |
132 |
14 |
edtech |
178 |
29 |
Colombia |
128 |
15 |
escenarios |
174 |
30 |
tech |
124 |
Note: Liderazgo: leadership; Cambio: change; Creatividad: creativity; Pymes; SMEs; Emprendedores: entrepreneurs; Escenarios: scenarios; Inteligencia empresarial: business intelligence; estrategios de negocios; commercial strategy; formación: training; Experiencias: experiences; Aprendizaje: learning; Emprendimiento: Entrepreneurship; Escuela: school; Redes sociales: social networks; Universidad: university.
Most Commonly Used Media/URLs
Finally, we again considered a sample of URLs and media that were shared within the Spanish-based Twitter discussion. Figure 7.26 provides some exemplary screenshots. Interestingly, the topics confirm the role of political individual and institutions (e.g. Cuba, Mexico, and Chile).
Figure 7.26. Screenshots of most commonly shared media/URLs: Spanish
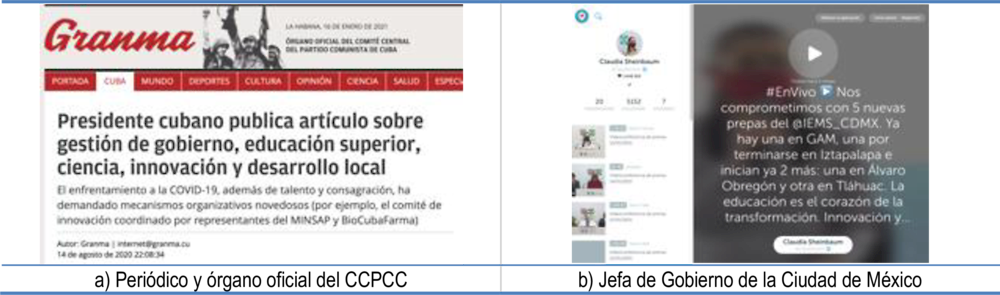
Measuring educational innovation through social media: An international exploration in the Twittersphere
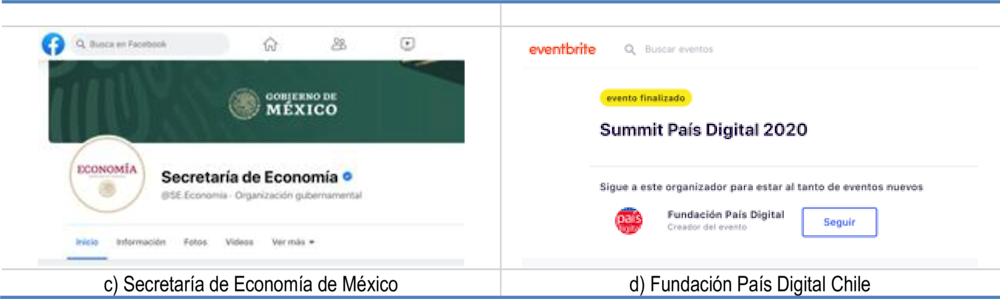
Note: a) Newsletter and official outlet of the Central Committee of the Communist Party of Cuba; b) Head of Mexico City government; c) Secretary of the economy of Mexico; d) Foundation País Digital (about developing a culture of digitalisation in Chile.
Summary
The Spanish-speaking Twitter space showed fewer exchanges than the English and French spaces, again mainly occasional, spread across a wide geographic area in Spain, Mexico and South America, probably explaining the lower interconnectedness of the different discussions. While educational innovation appear as an explicit content, digital technology and transformation are also key aspects of discussions, which also emphasise business management and entrepreneurship.
Comparative analysis
This section highlights some of the possibilities of our study for international comparisons, highlighting some of the differences and the similarities across the three linguistic Twitter discussions around educational innovation.
Differences
The analysis allowed us to discover some differences between the three samples.
First, in the context of the French and Spanish-based discussion, personal accounts appeared to play a more central role than organisational accounts. This is very interesting as it suggests a different culture of Twitter usage – e.g. (Park, Baek and Cha, 2014[76]). In the French-based sample, the personal accounts that were central to the discussion appeared to be geographically confined to the greater area of Paris. This closeness to the French (political) capital is again very interesting, as well as the relatively frequent relation of the top exchanged content with the ministry of education. The importance of political actors was even more pronounced in the Spanish case. (In the French case, we removed some of those actors though as they were confounders exchanging on topics that had little relevance for educational innovation.) Even more so, in this particular data sample, the impact of geographical regions was even more pronounced than in the French case, with political users largely disconnected from each other, seemingly on the basis of geographical regions. While Twitter allows users to participate irrespective of time and place (Rehm and Notten, 2016[32]), the access to offline, localised networks also seems to play a viable role in the attainment of centrality in the online network (Antheunis, Valkenburg and Peter, 2012[77]; Mesch and Talmud, 2006[78]). This also suggests the importance of geographic differences and the role of local contexts. The English (or US) case gave more prominence to organisational accounts from companies and foundations. There are thus different patterns in terms of who influences exchanges related to educational innovation in these different contexts.
Second, we observed differences in the level of interconnectivity (or openness) of the different networks. Openness matters for innovation policy, which tries to shape the conditions for knowledge, ideas, and good practices to flow within and across sectors. While all discussions were comprised of a wide range of communities, the Spanish sample showed very few signs of these communities being connected with each other. This suggests a strong internal focus on topics and circumstances that are relevant for the individual communities. In contrast, the English- and French-based samples show a strong degree of interconnectivity between the communities. The latter suggests that while there appear to be (nuanced) differences in the composition and topical foci of the individual communities, there is a general openness for other viewpoints, experiences and insights. Hence, these type of Twitter analyses provide valuable insights into the underlying architecture of discussions and how information spreads throughout the applicable networks. Moreover, policy makers can greatly benefit from this type of information, as it enables them to better understand whether and how different communities (e.g. possibly representing different perspective and points of view) are communicating with each other in an open social opportunity space.
Third, based on the hashtag analyses, it seemed that the English-based discussion focused on a few broader topics, while the French and Spanish-based discussions were more nuanced. We therefore stipulate that the use of the correct terminology is of vital importance when addressing the different spaces. While users from the English-based discussion seem to have agreed on a broad, “catch-all” terminology, the French and Spanish-based discussions had decidedly chosen for a more differentiated approach to discuss educational innovation. Furthermore, within the Spanish sample, we found indications that the discussions particularly served the purpose of sharing information and resources on educational leadership and (practical) experiences. Particularly, if policy makers are searching for information or even would like to share relevant information, this finding needs to be carefully considered, in order to ensure the highest possible uptake by the communities (Archibald and Clark, 2014[79]; McNeill, Harris and Briggs, 2016[80]). The variety of topics of discussion also confirms that educational innovation may concern different aspects across countries (Vincent-Lancrin et al., 2019[2]).
Fourth, the French and Spanish-based discussion showed more signs of organised exchanges of best-practices (e.g. a “content community” on this topic). Again, similar to the previous conclusion, this constitutes a valuable insight for policy makers, as they can better target the type and format of information that they would like to share, or simply get a better understanding of what type of educational innovation is currently discussed and implemented by practitioners. Indications of differences in discussions, e.g. inclusion, instructional design and a critical reflection of educational technology in schools, which were mainly present in the French-based discussion, seem to exemplify general differences in views on educational science depending on the country and educational system (De Corte, 1980[81]). A social media analysis can contribute very valuable insights and considerations to the discussion of different cultures regarding educational science, and, more generally, the approach to educational innovation.
Similarities
We were also able to identify some similarities between the English, French and Spanish cases.
First, the discussions related to educational innovation did not exhibit a constant level of interaction, but rather regularly occurred through peaks in communication. This is particularly interesting for policy makers and other actors in the space of educational innovation, as the timing of Tweets and the knowledge about fluctuations in the distribution of Tweets has been linked to the effectiveness of information campaigns (Dabeer, Karnink and Saroop, 2011[82]), the popularity and virality of content – e.g. (Pancer and Poole, 2016[83]), as well as the diffusion of information (Babcock, Villa Cox and Kumar, 2019[84]). In that sense, conducting social media analyses offers a type of “trend meter” that captures the current mood of the public about various aspects of educational innovation and indicates how they are perceived, as well as how persistent they continue to be of interest. Moreover, better understanding the “cadence” of communication patterns and how they are similar and different in a variety of contexts may suggest more opportune times to leverage networks.
Second, we found a limited number of communities that were driving the overall discussion in each of the linguistic contexts. This is a commonly observed phenomenon in social networks (e.g. Cross (2006[85]) and (2008[86])). Moreover, knowing about these communities and their (central) members can be valuable information for policy makers to specifically target these communities in an effort to enhance the reach of information (Bernhard and Dohle, 2018[87]; Cinelli et al., 2020[88]; Wekerle et al., 2018[89]). Being able to identify the communities, content, and most importantly the key users provides a set of opportunities to disseminate educational information, knowledge or practices to improve specific educational aims.
Third, all of the discussions were led by a combination of personal and organisational accounts. This is another indication that the topic of educational innovation is discussed by a wide variety of users and types of organisations. While organisational accounts suggest a larger interest in the topic and a degree of organisation and structure outside online social opportunity spaces, individual accounts show that really anybody can use Twitter to share their insights and contribute to a larger discussion. Differentiated strategies to engage with different types of users (organisations and individuals) reflects another key leverage point in the space. In the mix of personal and organisational accounts and relatively small numbers of influencers driving the discussion, none of them seem to be education research organisations, whether governmental or private. The French example may denote more influence of those governmental actors as governmental content seemed more largely shared. A question for policy makers to promote innovation based on evidence or gain evidence from innovation may include the design of stronger connections to these existing networks.
Fourth, a range of different sub-topics (as identified by different “content communities”) were discussed in the different samples. More specifically, the general topics of “educational technology”, “digital transformation” and the sharing of “good practices” appear to be a type of common denominator across the discussions. Finally, and particularly related to the latter general topic, a closer look at the URLs and media being shared revealed that sharing of best practices was a common phenomenon across samples. This ranged from suggestions on how to use (new) educational technology in classrooms, to very specific experience reports on how a certain educational technology (e.g. video-conferencing tools) have been effectively used in education. Interestingly, across the three cases there was a strong emphasis on the role of technology as core to notions around innovation. This is not surprising given the strong role of technology in driving innovation (and people’s association of the notions). In education, technology use was also one of the main observed changes in most countries’ classroom in the past decade (Vincent-Lancrin et al., 2019[2]).
Conclusions
This study set out to investigate whether and how different aspects of educational innovation are discussed on Twitter in a variety of international settings. It tried to measure the intensity of the discussion around educational innovation topics, the structure and drivers of the corresponding social networks, and the topics of the exchanged contents. Specifically, we considered possible difference between English-speaking (which ended up with a primarily US and UK focus), French-speaking (primarily France and a bit of Canada) and an additional run around Spanish speaking (which ended up with considerable focus on South American countries). The underlying motivation for this distinction was based on the prevalence of English, French and Spanish across OECD countries, and the observation that the corresponding education systems noticeably differ in terms of organisational structures, financial endowment and other characteristics such as the degree of internationalization (OECD, 2018[57]).
Our preliminary findings clearly suggest that Twitter is a social opportunity space (Rehm et al., 2020[31]), wherein the topic of educational innovation is widely discussed in all three samples. (This would also be the case for other social media.) By enabling users to engage into discussions with a wide variety of other individuals (Tynjälä, 2013[52]) and fostering a process of "environmental scanning" (Lohman, 2005, p. 505[56]), Twitter greatly contributes to the discussion about educational innovation. Not only do the applicable Twitter discussions contain information about the latest product and process innovations, but they also provide valuable insights into the interplay between the two, e.g. how educational leaders are introducing and supporting innovations in their immediate surroundings. This in turn has already been identified as a crucial aspect in contributing to make progress and further stimulate educational innovation (Serdyukov, 2017[3]).
Based on these early findings, we therefore conclude that social network analysis can be instrumental for policy makers to better understand and anticipate how communication patterns and discussions about educational innovation develop and evolve within social media spaces, such as Twitter. Furthermore, conducting these types of analyses allows us to potentially profile social media conversations and better understand what type of discussions draw what type of participants and how the dynamics might be influenced by this.
Finally, mapping social media structures can provide 1) topics and 2) information about central users that can be used to invite relevant representatives for a participatory discussion, e.g. EduCamps (Bernhardt and Kirchner, 2010[90]), about policy and make more informed policy decisions. Consequently, Twitter analyses allow tracking and understanding not only educational innovation in general, but also how it is implemented and supported within educational systems. Even more so, as the underlying communication flows resemble a bottom-up approach for educational professionals that often feel neglected in the formal policy processes – e.g. (Ketelaar et al., 2012[4]; Lewin and Stuart, 1993[5]), it can also serve as a vital source of information for policy makers to better understand the views and experiences about innovation of their target audience (e.g. educational professionals and leaders).
The current work should be considered a first step in a wider set of upcoming studies that depart from the indicated notions, conceptual frameworks and findings. Here, we have provided a proof-of-concept and highlighted how mapping out social network structures, visualizing connections and investigating the content that is being shared can contribute to our understanding of how (educational) innovation is discussed and applicable information and insights are being shared. These types of analyses are likely to become even more important and useful in the upcoming years. For example, the global COVID-19 pandemic has influenced new developments in social media. A wide range of preliminary studies has already shown that social media is being used to spread misinformation – e.g. (Brennen et al., 2020[91]; Kouzy et al., 2020[92]) and to counteract these developments – e.g. (Guest, Del Rio and Sanchez, 2020[93]; Van Bavel, Baicker and , 2020[94]). Yet, more detailed analyses are required to get a better understanding of online social opportunity spaces in general and how, beyond the ramifications of the current global pandemic, educational professionals are using them to share resources and eventually transform their daily practice.
Additionally, this study used data from a limited time frame and focused on three languages within the OECD area. Future studies should conduct analyses on data from more prolonged periods of time, in order to be able to more clearly indicate trends and movements. Furthermore, upcoming reports should further expand into other geographical regions (e.g. Asia, Africa, Europe) and incorporate a wider set of languages. One limitation of the approach compared to traditional comparative statistics is that it does not allow providing country statistics with precision – because an account may not be located where it has influence and the geography of the discussions should mainly be inferred by manual checks. For example, the weight of the United States in the English-speaking sample made it primarily a US discussion, in spite of the wide usage of English in other countries.
Possible next steps also include the consideration of other social media outlets, such as Facebook and YouTube, as well as more traditional communication channels, including town hall meetings, letters to the organizing body and newspaper coverage, or again, in the case of education of exchanges within large national or international platforms for teacher exchange. In order to provide a more holistic view on how educational innovation is discussed within a larger policy context, future studies should also strive to gather more data on the currently neglected types of information (Rehm et al., 2020[9]). Moreover, while this study has focused on educational innovation, Twitter discussions about innovation certainly encompass a wider set of disciplines, including leadership (Park, 2013[95]; Park and Kaye, 2017[96]), health (Chew and Eyesnbach, 2010[97]; Hawn, 2009[98]), technology (Chang, 2011[99]) and response to crisis (Acar and Muraki, 2011[100]; Lachlan et al., 2016[101]). Consequently, possible next steps include the possible expansion of our analyses into these spaces as well.
Furthermore, this study is purely based on quantitative Social Network Analysis data. Future research should consider applying a mixed-methods approach (Fröhlich et al., 2020). This can take on various forms. For example, by adding more advanced semantic analysis, e.g. topic modelling and sentiment analyses (Kabir, Karim and Newaz, 2018[102]; Rehm et al., 2020[31]; Yue et al., 2018[103]), future research could provide more detailed insights into the type of resources being shared, the perceptions of users about different types of (educational) innovation, and what type of experiences users have made when (trying to) introduce innovation in their local settings. Particularly the latter type of information could then further be explored by adding semi-structured interviews (Rehm, Cornelissen, Notten, et al., 2020), as well as qualitative coding of Tweets and resources being shared via Twitter (Moukarzel, Rehm and Daly, 2020[70]; Moukarzel et al., 2020[71]). This would not only allow to collect detailed information about how (educational) innovation is implemented, e.g. in schools, but also what type of hinderances and affordances educational professionals are facing in their local settings.
Finally, while Twitter analyses constitute an important aspect in understanding various aspects of educational innovation, social media are part of a social continuum (Daly et al., 2019[104]) that also includes offline spaces (e.g. within-school face-to-face settings). Hence, future studies should strive to also capture how educational professionals consider, implement and support educational innovation in their relevant and applicable offline spaces, and how the online and offline dimensions supplement each other. This would provide additional dimensions to the findings and contribute to a more holistic view of innovation in education.
Despite possible improvements, expansions and refinements of the methodology, the objective of the chapter was to show what type of information on educational innovation could be collected from a social media analysis for the purpose of international comparisons. It shows that approaching the dynamics of educational innovation within the online sphere is possible and could provide an informative and actionable complement to other forms of measurement and analysis of innovation in education, notably surveys.
References
[100] Acar, A. and Y. Muraki (2011), “Twitter for crisis communication: Lessons learned from Japan’s tsunami disaster”, International Journal of Web Based Communities, Vol. 7/3, pp. 392-402, https://doi.org/10.1504/IJWBC.2011.041206.
[26] Adams Becker, S., M. Brown, E. Dahlstrom, A, Davis, K. DePaul, V. Diaz and J. Pomerantz (2018), Horizon Report 2018 Higher Education Edition Brought to you by EDUCAUSE, https://www.learntechlib.org/p/184633/.
[54] Akkerman, S. and A. Bakker (2011), “Boundary Crossing and Boundary Objects”, Review of Educational Research, Vol. 81/2, pp. 132-169, https://doi.org/10.3102/0034654311404.
[77] Antheunis, M., P. Valkenburg and J. Peter (2012), “The quality of online, offline, and mixed-mode friendships among users of a social networking site”, Cyberpsychology: Journal of Psychosocial Research on Cyberspace, Vol. 6/3, https://doi.org/10.5817/CP2012-3-6.
[79] Archibald, M. and A. Clark (2014), “Twitter and nursing research: How diffusion of innovation theory can help uptake”, Journal of Advanced Nursing, Vol. 70/3, https://doi.org/10.1111/jan.12343.
[84] Babcock, M., R. Villa Cox and S. Kumar (2019), “Diffusion of pro- and anti-false information tweets: the Black Panther movie case”, Computational and mathematical Organization Theory, Vol. 25/1, pp. 72-84.
[34] Ball, S. and C. Junemann (2012), Networks, New Governance and Education, Policy Press.
[90] Bernhardt, T. and M. Kirchner (2010), Web 2.0 Meets Conference: The EduCamp as a New Format of Participation and Exchange in the World of Education, https://doi.org/10.4018/978-1-61520-678-0.ch011.
[87] Bernhard, U. and M. Dohle (2018), “Perceptual processes and political participation: Do the presumed reach and the presumed influence of social media affect political activities via Facebook and Twitter?”, Communications, Vol. 43/4, pp. 451-467, https://doi.org/10.1515/commun-2017-0052.
[22] Bloch, F., G. Genicot and D. Ray (2008), “Informal insurance in social networks”, Journal of Economic Theory, Vol. 143/1, pp. 36-58, https://doi.org/10.1016/j.jet.2008.01.008.
[28] Boahin, P. and A. Hofman (2012), “Implementation of innovations in higher education: the case of competency-based training in Ghana”, Innovations in Education and Teaching International, Vol. 49/3, pp. 283-293, https://doi.org/10.1080/14703297.2012.703021.
[91] Brennen, J., F. M. Simon, P. N. Howard and R. Kleis Nielsen (2020), Types, sources, and claims of COVID-19 misinformation.
[68] Burt, R. (2010), Structural Holes. The Social Structure of Competition, Harvard University Press, https://doi.org/10.4159/9780674029095.
[99] Chang, H. (2011), “A new perspective on Twitter hashtag use: Diffusion of innovation theory”, Proceedings of the American Society for Information Science and Technology, Vol. 47/1, https://doi.org/10.1002/meet.14504701295.
[97] Chew, C. and G. Eyesnbach (2010), “Pandemics in the Age of Twitter: Content Analysis of Tweets during the 2009 H1N1 Outbreak”, Plos One, Vol. 5/11, https://doi.org/10.1371/journal.pone.0014118.
[18] Choo, E., M. Ranney, T. Chan, N. Tueger, A. Walsh, K. Tegtmeyer, S. McNamara, R. Choi and C. Carroll (2015), “Twitter as a tool for communication and knowledge exchange in academic medicine: A guide for skeptics and novices”, Medical Teacher, Vol. 37/5, https://doi.org/10.3109/0142159X.2014.993371.
[88] Cinelli, M., S. Cresci, A. Galeazzi, W. Quattrociocchi and M. Tesconi (2020), “The limited reach of fake news on Twitter during 2019 European elections”, Plos One, Vol. 15/6, https://doi.org/10.1371/journal.pone.0234689.
[37] Cornelissen, F., J. van Swet, D. Beijaard and T. Bergen (2011), “Aspects of school–university research networks that play a role in developing, sharing and using knowledge based on teacher research”, Teaching and Teacher Education, Vol. 27/1, pp. 147-156, https://doi.org/10.1016/j.tate.2010.07.011.
[86] Cross, R. and K. Ehrlich (2008), “Managing Collaboration at the Point of Execution: Improving Team Effectiveness with a Network Perspective”, California Management Review, Vol. 50/4, pp. 74-98.
[85] Cross, R., T. Laseter, A. Parker and G. Velasquez (2006), “Using Social Network Analysis to Improve Communities of Practice”, California Management Review, Vol. 49/1, pp. 32-60.
[82] Dabeer, O., A. Karnink and A. Saroop (2011), Timing Tweets to Increase Effectiveness of Information Campaigns, https://doi.org/10.1609/icwsm.v5i1.14104.
[104] Daly, A., Y-W. Liou, M. del Fresno García and M. Rehm (2019), “Educational Leadership in the Twitterverse: Social Media, Social Networks, and the New Social Continuum”, Teachers College Record: The Voice of Scholarship in Education, Vol. 121.
[61] Daly, A., M. Molenaar, J. Bolivar and P. Burke (2010), “Relationships in reform: The role of teachers’ social networks”, Journal of educational administration, Vol. 48/3, pp. 359-391, https://doi.org/10.1108/09578231011041062.
[81] De Corte, E. (1980), “Processes of problem solving: Comparison of an American and a European view”, Instructional Science, Vol. 9/1, pp. 1-13.
[19] Dumont, H., D. Istance and F. Benavides (eds.) (2010), The Nature of Learning: Using Research to Inspire Practice, Educational Research and Innovation, OECD Publishing, Paris, https://doi.org/10.1787/9789264086487-en.
[8] Finsterwald, M., P. Wagner, B. Schober, M. Lüftenegger and C. Spiel (2013), “Fostering lifelong learning – Evaluation of a teacher education program for professional teachers”, Teaching and Teacher Education, Vol. 29/1, pp. 144-155, https://doi.org/10.1016/j.tate.2012.08.009.
[65] Fresno García, M., A. Daly and S. Segado Sánchez-Cabezudo (2016), “Identifying the new Infl uencers in the Internet Era: Social Media and Social Network Analysis”, Revista Española de Investigaciones Sociológicas, Vol. 153, pp. 23-40, https://doi.org/10.5477/cis/reis.153.23.
[12] Garcia, R., K. Falkner and R. Vivian (2019), “Systematic literature review: Self-Regulated Learning strategies using e-learning tools for Computer Science”, Computers & Education,, Vol. 123, pp. 150-163, https://doi.org/10.1016/j.compedu.2018.05.006.
[44] Gee, J. (2005), Semiotic social spaces and affinity spaces: from The Age of Mythology to today’s schools, Cambridge University Press.
[64] Grabowicz, P., J. Ramasco, B. Gonçlaves and V. Eguíluz (2014), “Entangling Mobility and Interactions in Social Media”, Plos One, Vol. 9/3, pp. 1-12, https://doi.org/10.1371/journal.pone.0092196.
[25] Greenan, N. and E. Lorenz (2013), Developing harmonized measures of the dynamics of organizations and work.
[93] Guest, J., C. Del Rio and T. Sanchez (2020), “The Three Steps Needed to End the COVID-19 Pandemic: Bold Public Health Leadership, Rapid Innovations, and Courageous Political Will”, JMIR Public Health Surveill, Vol. 6/2, https://doi.org/10.2196/19043.
[23] Haelermans, C. (2010), “Innovative power of Dutch secondary education”, Innovation-Management Policy & Practice, Vol. 12/2, pp. 154-165, https://doi.org/10.5172/impp.12.2.154.
[24] Halász, G. (2018), “Measuring innovation in education: The outcomes of a national education sector innovation survey”, European Journal of Education, Vol. 53/4, https://doi.org/10.1111/ejed.12299.
[74] Halpin, H., V. Robu and H. Sheperd (2007), The complex dynamics of collaborative tagging, https://doi.org/10.1145/1242572.1242602.
[46] Hart, S. and S. Sharma (2004), “Engaging fringe stakeholders for competitive imagination”, Academy of Management Perspective, Vol. 18/1, https://doi.org/10.5465/ame.2004.12691227.
[98] Hawn, C. (2009), “Take two aspirin and tweet me in the morning: how Twitter, Facebook, and other social media are reshaping health care”, Health Affairs, Vol. 28/2, pp. 361-368, https://doi.org/10.1377/hlthaff.28.2.361.
[48] Ito, M., K. Gutierrez, S. Livingstone, B. Penuel, J. Rhodes, K. Salen, J. Schor, J. Sefton-Green and S. Watkins (2012), Connected Learning: An Agenda for Research and Design.
[102] Kabir, A., R. Karim and S. Newaz (2018), “The Power of Social Media Analytics: Text Analytics Based on Sentiment Analysis and Word Clouds on R”, Informatica Economica, Vol. 22/1.
[17] Kalyuga, S. and A. Singh (2016), “Rethinking the Boundaries of Cognitive Load Theory in Complex Learning”, Educational Psychology Review, Vol. 28, pp. 831–852, https://doi.org/10.1007/s10648-015-9352-0.
[4] Ketelaar, E., D. Beijaard, H. Boshuizen and P. Den Brok (2012), “Teachers’ positioning towards an educational innovation in the light of ownership, sense-making and agency”, Teaching and Teacher Education, Vol. 28/2, https://doi.org/10.1016/j.tate.2011.10.004.
[109] Kirschner, P., J. Sweller, F. Kirshner and J. Zambrano (2018), “From Cognitive Load Theory to Collaborative Cognitive Load Theory”, International Journal of Computer-Supported Collaborative Learning, Vol. 13, pp. 213-233, https://doi.org/10.1007/s11412-018-9277-.
[35] Klijn, E. and J. Koppenjan (2012), “Governance Network Theory: Past, Present and Future”, Policy & Politics, Vol. 40/4, pp. 587-606, https://doi.org/10.1332/030557312X655431.
[72] Koene, A., E. Perez, C.J. Carter, R. Statache, S. Adolphs, C. O'Malley, T. Rodden and D. McAuley (2015), Research ethics and public trust, preconditions for continued growth of internet mediated research - public confidence in internet mediate research, SciTePress.
[49] Kolb, A. and D. Kolb (2017), “Learning Styles and Learning Spaces: Enhancing Experiential Learning in Higher Education”, Academy of Management Learning & Education, Vol. 4/2, pp. 193-212, https://doi.org/10.5465/amle.2005.17268566.
[53] Kolb, D. (1984), Experiential Learning: Experience As The Source Of Learning And Development, Prentice-Hall.
[92] Kouzy, R., J. Abi Jaoude, A. Kraitem, M. B. El Alam, B. Karam, E. Adib, J. Zarka, C. Traboulsi, E. W. Akl and K. Baddour (2020), “Coronavirus Goes Viral: Quantifying the COVID-19 Misinformation Epidemic on Twitter”, Cureus, Vol. 12/3, https://doi.org/10.7759/cureus.7255.
[101] Lachlan, K., P. Spence, X. Lin, K. Najarian and M. Del Greco (2016), “Social media and crisis management: CERC, search strategies, and Twitter content”, Computers in Human Behavior, Vol. 54, pp. 647-652, https://doi.org/10.1016/j.chb.2015.05.027.
[75] Latapy, M., C. Magnien and N. Del Vecchio (2008), “Basic notions for the analysis of large two-mode networks”, Social Networks, Vol. 30/1, pp. 31-48, https://doi.org/10.1016/j.socnet.2007.04.006.
[15] Lech, K., L. Hoople, K. Abiker, M. Mitchell and P. Mooney (2017), “Students as producers and active partners in enhancing equality and diversity: ‘culturosity’ at Canterbury Christ Church University”, Journal of Educational Innovation, Partnership and Change, Vol. 3/2, pp. 2055-4990, https://doi.org/10.21100/jeipc.v3i2.533.
[69] Lee, J., M. DeCamp, M. Dredze, M. Chisolm and Z.D. Berger (2014), “What are health-related users tweeting? A qualitative content analysis of health-related users and their messages on twitter”, Journal of medical Internet Research, Vol. 16/10, https://doi.org/10.2196/jmir.3765.
[47] Lee, U., B. Zhou, M. Gerla, E. Magistretti, P. Bellavista and A. Corradi (2006), “Mobeyes: smart mobs for urban monitoring with a vehicular sensor network”, IEEE Wireless Communications, Vol. 10/5, pp. 52-57, https://doi.org/10.1109/WC-M.2006.250358.
[59] Letierce, J., A. Passant, S. Decker and J.G. Breslin (2010), “Understanding how Twitter is used to spread scientific messages”.
[5] Lewin, K. and J. Stuart (1993), Educational Innovation in Developing Countries: Case-Studies of Changemakers, Springer.
[56] Lohman, M. (2005), “A survey of factors influencing the engagement of two professional groups in informal workplace learning activities”, Human Resource Development Quarterly, Vol. 16, pp. 501-527, https://doi.org/10.1002/hrdq.1153.
[41] Marotzki, W. (2004), Von der Medienkompetenz zur Medienbildung.
[43] Marotzki, W. and B. Jörissen (2008), Medienbildung, https://doi.org/10.1007/978-3-531-91158-8_11.
[14] McLay, K. and V. Reyes (2019), “Identity and digital equity: Reflections on a university educational technology course”, Australasian Journal of Educational Technology, Vol. 35/6, pp. 15-29, https://doi.org/10.14742/ajet.5552.
[80] McNeill, A., P. Harris and P. Briggs (2016), “Twitter Influence on UK Vaccination and Antiviral Uptake during the 2009 H1N1 Pandemic”, Frontiers in Public Health, Vol. 4, https://doi.org/10.3389/fpubh.2016.00026.
[51] McPherson, M., K. Budge and N. Lemon (2015), “New practices in doing academic development: Twitter as an informal learning space”, International Journal for Academic Development, Vol. 20/2, pp. 126-136, https://doi.org/10.1080/1360144X.2015.1029485.
[78] Mesch, G. and I. Talmud (2006), “The Quality of Online and Offline Relationships: The Role of Multiplexity and Duration of Social Relationships”, The Information Society, Vol. 22/3, pp. 137-148.
[73] Moreno, M., N. Goniu, P. S. Moreno and Douglas Diekema (2013), “Ethics of Social Media Research: Common Concerns and Practical Considerations”, Cyberpsychology, Behavior, and Social Networking, Vol. 16/9, pp. 708-713, https://doi.org/10.1089/cyber.2012.0334.
[71] Moukarzel, S., M. Rehm, M. del Fresno and A. Daly (2020), “Diffusing science through social networks: The case of breastfeeding communication on Twitter”, Plos One, https://doi.org/10.1371/journal.pone.0237471.
[70] Moukarzel, S., M. Rehm and A. Daly (2020), “Breastfeeding promotion on Twitter: A social network and content analysis approach”, Maternal & Child Nutrition, Vol. 16/4, https://doi.org/10.1111/mcn.13053.
[50] Mynatt, E., V. O’Day and A. Adler (1998), “Network Communities: Something Old, Something New, Something Borrowed ...”, Computer Supported Cooperative Work (CSCW), pp. 123-156, https://doi.org/10.1023/A:1008688205872.
[66] Newman, M. (2006), “Modularity and community structure in networks”, Proceedings of the National Academy of Sciences, Vol. 103/23, pp. 8577-5852, https://doi.org/10.1073/pnas.0601602103.
[67] Noack, A. (2009), “Modularity clustering is force-directed layout”, Physical Review E, Vol. 79/2, https://doi.org/10.1103/PhysRevE.79.026102.
[57] OECD (2018), OECD Handbook for Internationally Comparative Education Statistics 2018: Concepts, Standards, Definitions and Classifications, OECD Publishing, https://doi.org/10.1787/9789264304444-en.
[10] OECD (2016), Innovating Education and Educating for Innovation: The Power of Digital Technologies and Skills, Educational Research and Innovation, OECD Publishing, Paris, https://doi.org/10.1787/9789264265097-en.
[1] OECD (2014), Measuring Innovation in Education: A New Perspective, Educational Research and Innovation, OECD Publishing, Paris, https://doi.org/10.1787/9789264215696-en.
[30] OECD (2013), Leadership for 21st Century Learning, Educational Research and Innovation, OECD Publishing, Paris, https://doi.org/10.1787/9789264205406-en.
[21] OECD/Eurostat (2018), Oslo Manual 2018: Guidelines for Collecting, Reporting and Using Data on Innovation, 4th Edition, The Measurement of Scientific, Technological and Innovation Activities, OECD Publishing, Paris/Eurostat, Luxembourg, https://doi.org/10.1787/9789264304604-en.
[39] Owen, N., A. Fox and T. Bird (2016), “The development of a small-scale survey instrument of UK teachers to study professional use (and non-use) of and attitudes to social media”, International Journal of Research & Method in Education, Vol. 39/2, pp. 170-193, https://doi.org/10.1080/1743727X.2015.1041491.
[83] Pancer, E. and M. Poole (2016), “The popularity & virality of political social media: Hashtags, mentions, and links predict likes & retweets of 2016 U.S. presidential nominees’ tweets”, Social Influence.
[95] Park, C. (2013), “Does Twitter motivate involvement in politics? Tweeting, opinion leadership, and political engagement”, Computers in Human Behavior, Vol. 29/4, pp. 1641-1648, https://doi.org/10.1016/j.chb.2013.01.044.
[96] Park, C. and B. Kaye (2017), “The tweet goes on: Interconnection of Twitter opinion leadership, network size, and civic engagement”, Computers in Human Behaviour, Vol. 69, pp. 174-180, https://doi.org/10.1016/j.chb.2016.12.021.
[76] Park, J., Y. Baek and M. Cha (2014), “Cross‐cultural comparison of nonverbal cues in emoticons on Twitter: Evidence from big data analysis”, Journal of Communication, Vol. 64/2, pp. 333-354, https://doi.org/10.1111/jcom.12086.
[36] Pierre, J. and G. Peters (2000), Governance, Politics and the State, Macmillan.
[16] Polák, P. (2017), “The productivity paradox: A meta-analysis”, Information Economics and Policy, Vol. 38, pp. 38-54.
[13] Prain, V., P. Cox, C. Deed, J. Dorman, D. Edwards, C. Farrelly, M. Keeffe, V. Lovejoy, L. Mow, P. Sellings, B. Waldrip and Z. Yager (2013), “Personalised learning: lessons to be learnt”, British educational research journal, Vol. 39/4, pp. 654–676.
[29] Prince Machado, M., G. Tenorio Sepúlveda and M. Ramirez Montoya (2016), “Educational innovation and digital competencies: the case of OER in a private Venezuelan university”, International Journal of Educational Technology in Higher Education, Vol. 13/1, https://doi.org/10.1186/s41239-016-0006-1.
[31] Rehm, M., F. Cornelissen, A. Daly and M. Del Fresno García (2020), “Drinking from the Firehose: The Structural and Cognitive Dimensions of Sharing Information on Twitter”, American Journal of Education, Vol. 127/1, https://doi.org/10.1086/711014.
[9] Rehm, M., F. Cornelissen, A. Notten, A. Daly and J. Supovitz (2020), Power to the People?! Twitter Discussions on (Educational) Policy Processes.
[32] Rehm, M. and A. Notten (2016), “Twitter as an informal learning space for teachers!? The role of social capital in Twitter conversations among teachers”, Teaching and Teacher Education, Vol. 60, pp. 215-223, https://doi.org/10.1016/j.tate.2016.08.015.
[107] Resta, P., T. Laferrière, R. McLaughlin and A. Kouraogo (2018), Issues and Challenges Related to Digital Equity: An Overview, Springer, https://doi.org/10.1007/978-3-319-71054-9_67.
[45] Rheingold, H. (2007), Smart mobs: The next social revolution, Basic Books.
[106] Richardson, J. (ed.) (2019), Bringing Innovative Practices to Your School, Routledge, https://doi.org/10.4324/9780429197062.
[40] Risser, H. (2013), “Virtual induction: A novice teacher’s use of Twitter to form an informal mentoring network”, Teaching and Teacher Education, Vol. 35, pp. 25-33, https://doi.org/10.1016/j.tate.2013.05.001.
[105] Rovers, S., G. Clarebout, H. C. M. Savelberg, A. de Bruin and J. van Merriënboer (2019), “Granularity matters: comparing different ways of measuring self-regulated learning”, Metacognition and Learning, Vol. 14, pp. 1-19, https://doi.org/doi.org/10.1007/s11409-019-09188-6.
[62] Schlager, M., U. Farooq, J. Fusco, P. Schank and N. Dwyer (2009), “Analyzing Online Teacher Networks: Cyber Networks Require Cyber Research Tools”, Journal of Teacher Education, Vol. 60/1, pp. 86-100, https://doi.org/10.1177/0022487108328487.
[20] Schleicher, A. (ed.) (2012), Preparing Teachers and Developing School Leaders for the 21st Century: Lessons from around the World, International Summit on the Teaching Profession, OECD Publishing, Paris, https://doi.org/10.1787/9789264174559-en.
[63] Scott, J. (2017), Social Network Analysis, SAGE Publications Ltd, https://doi.org/10.4135/978152971659.
[3] Serdyukov, P. (2017), “Innovation in education: What works, what doesn’t, and what to do about it”, Journal of Research in Innovative Teaching & Learning, Vol. 10/1, pp. 4-33, https://doi.org/10.1108/JRIT-10-2016-0007.
[42] Spanhel, D. (2010), Mediale Bildungsräume. Ihre Erschließung und Gestaltung als Handlungsfeld der Medienpädagogik, Kopaed.
[33] Supovitz, J., A. Daly and M. del Fresno (2015), #CommonCore: How social media is changing the politics of education, https://repository.upenn.edu/hashtagcommoncore/1/.
[108] Tang, H. and A. Carr-Chellman (2016), “Massive Open Online Courses and Educational Equality in China: A Qualitative Inquiry”, Journal of Educational Technology Development and Exchange (JETDE), Vol. 9/1, pp. 49-66, https://doi.org/10.18785/jetde.0901.04.
[7] Thorn, W. and S. Vincent-Lancrin (2021), Schooling During a Pandemic: The Experience and Outcomes of Schoolchildren During the First Round of COVID-19 Lockdowns, OECD Publishing, Paris, https://doi.org/10.1787/1c78681e-en.
[52] Tynjälä, P. (2013), “Toward a 3-P Model of Workplace Learning: a Literature Review”, Vocations and Learning, pp. 11-36, https://doi.org/10.1007/s12186-012-9091-z.
[94] Van Bavel, J., K. Baicker and B. (2020), “Using social and behavioural science to support COVID-19 pandemic response”, Nature Human Behaviour, Vol. 4, pp. 460-471, https://doi.org/10.1038/s41562-020-0884-z.
[11] Van Lieshout, K., A. Arundel and S. Vincent-Lancrin (2023), "Measuring innovation through surveys: Main considerations and applications to education" in Vincent-Lancrin, S. (ed.), Measuring Innovation in Education 2023: Tools and Methods for Data-Driven Action and Improvement, OECD Publishing.
[110] Venkatesh, V., M. Morris, G. Davis and F. Davis (2003), “User Acceptance of Information Technology: Toward a Unified View”, MIS Quarterly, Vol. 27/3, pp. 425-478.
[27] Vincent-Lancrin, S. (2020), “Monitoring innovation in education to improve education policies and outcomes in OECD countries”, IUL Research, Vol. 1/1, https://doi.org/10.57568/iulres.v1i1.44.
[6] Vincent-Lancrin, S., C. Cobo Romaní and F. Reimers (eds.) (2022), How Learning Continued during the COVID-19 Pandemic: Global Lessons from Initiatives to Support Learners and Teachers, OECD Publishing, Paris, https://doi.org/10.1787/bbeca162-en.
[2] Vincent-Lancrin, S., J. Urgel, S. Kar and G. Jacotin (2019), Measuring Innovation in Education 2019: What Has Changed in the Classroom?, Educational Research and Innovation, OECD Publishing, Paris, https://doi.org/10.1787/9789264311671-en.
[60] Wasserman, S. and K. Faust (1994), Social Network Analysis: Methods and Applications, Cambridge University Press, https://doi.org/10.1017/CBO9780511815478.
[89] Wekerle, C., N. Vakili, S. Stewart and T. Black (2018), “The utility of Twitter as a tool for increasing reach of research on sexual violence.”, Child Abuse & Neglect, Vol. 85, https://doi.org/10.1016/j.chiabu.2018.04.019.
[38] Wellman, B. (2001), “Computer Networks As Social Networks”, Science, Vol. 293/5537, pp. 2031-2034, https://doi.org/10.1126/science.1065547.
[55] Williams, D. (2006), “On and off the ’Net: Scales for Social Capital in an Online Era”, Journal of Computer-Mediated Communication, Vol. 11/2, pp. 593–628, https://doi.org/10.1111/j.1083-6101.2006.00029.x.
[58] Ye, Q., B. Fang, W. He and J. Po-An Hsieh (2012), “Can Social Capital be Transferred Cross the Boundary of the Real and Virtual Worlds? An Empirical Investigation of Twitter”, Journal of Electronic Commerce Research, Vol. 13/2, pp. 145-156, http://www.jecr.org/node/51.
[103] Yue, L., W. Chen, X. Li, W. Zuo and M. Yin (2018), “A survey of sentiment analysis in social media”, Knowledge and Information Systems, Vol. 60, pp. 617–663, https://doi.org/10.1007/s10115-018-1236-4.
Notes
← 1. https://ec.europa.eu/eurostat/web/products-eurostat-news/-/DDN-20190312-1.
← 2. The applicable metrics and Social Network Analysis visualisations were determined using a combination of software packages. On the one hand, we used the statistical software environment R. Here, we used the libraries “data.table”, “stringr” and “igraph”, to clean and transform the raw Twitter data into Social Network Analysis compatible formats. On the other hand, we used the open graph visualisation platform gephi to produce sociograms.
← 3. (innovación OR innovación) AND (educación OR educación).
← 4. Please note that the Social Runner Lab was updated in between data collection runs. Consequently, the figures representing the distribution across time look slightly different.