Digital tools can allow public employment services (PES) to be more effective and efficient. Such tools can enable PES to better meet the needs of their clients, including employers, jobseekers, and those at risk of unemployment. Indeed, such tools can free up resources for PES to support other areas of active labour market policies (ALMPs). This is particularly important in Latvia’s context where spending on ALMPs is low compared to other OECD countries. This chapter describes and assesses the current digital tools used at Latvia’s State Employment Agency (SEA). It focuses on the SEA’s jobseeker profiling tool that helps identify jobseekers’ distance from the labour market, the vacancy matching tool that supports employers and jobseekers, and the plans to introduce skills profiling tools.
Modernising Latvia’s Public Employment Service through Digitalisation
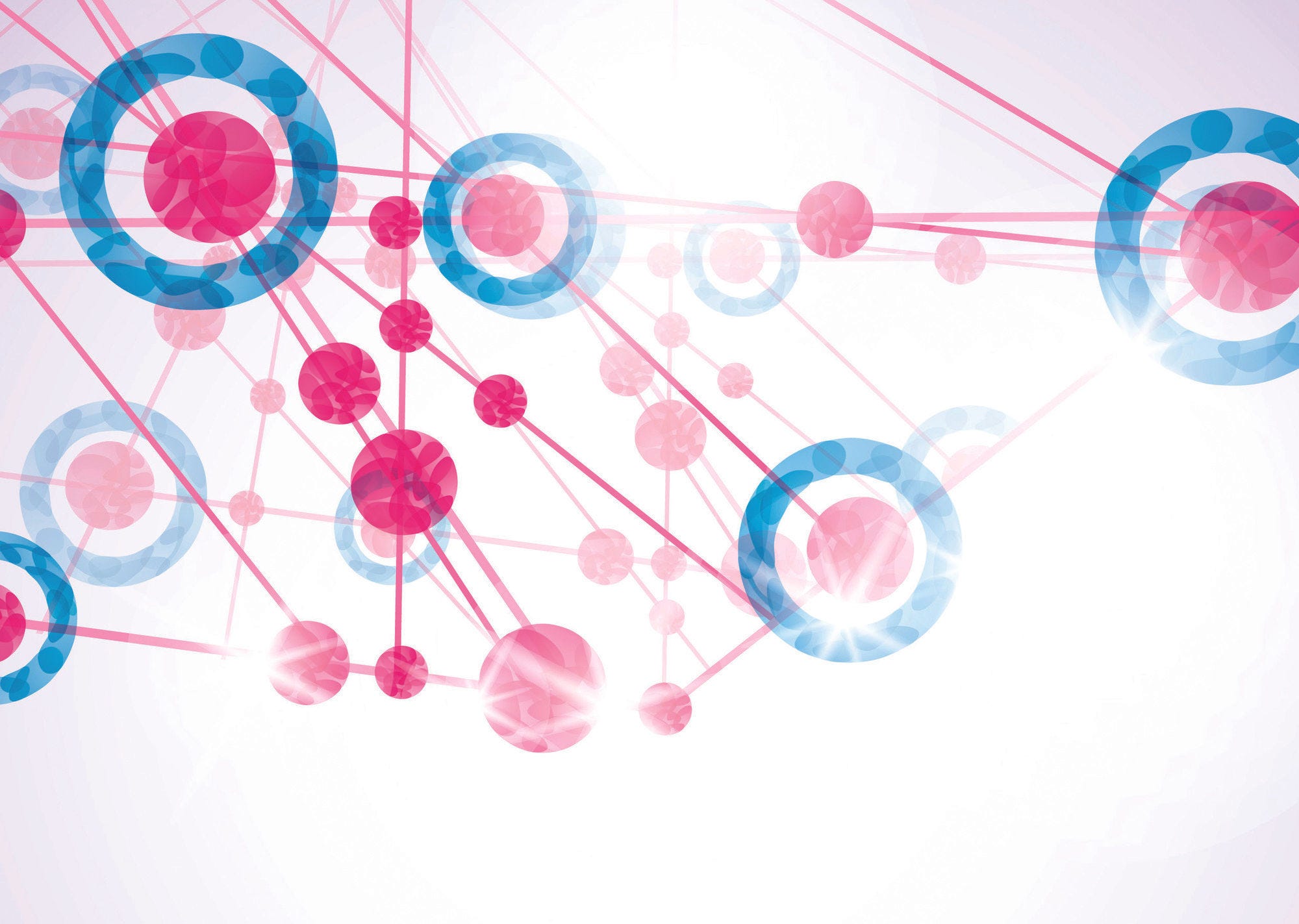
4. Digital tools at the State Employment Agency
Copy link to 4. Digital tools at the State Employment AgencyAbstract
4.1. Introduction
Copy link to 4.1. IntroductionPES specific digital tools can help PES to better support employers, jobseekers and those at risk of unemployment. Such tools can also free up resources, such as by saving counsellor’s time, which can then be redirected to other activities. PES specific tools differ from more general digital tools in that they offer functionalities that are only relevant to PES. This chapter describes and assesses the key PES specific digital tools used at the SEA. Specifically, it covers the profiling tool and the vacancy matching tool as well as the SEA’s needs and current plans for a skills profiling tool. The high-level IT infrastructure, data analytics and visualisation tools, and the associated practices and processes are covered in Chapter 3.
In recent years countries have made great strides in making use of digital tools (OECD, 2022[1]). Such tools can offer a range of different digital functions to meet the needs of jobseekers, employers, and PES staff. For jobseekers, functions can include online registration, CV creation, skills testing, career guidance, virtual training, access to job vacancies, and provision of information on PES services including through tools like chat bots. Employers might have access to features that allow them to find employees, post job advertisements, receive advice, apply for training incentives, and share information on filled positions. Finally, PES staff can use tools to access information on jobseekers, conduct job seeker profiling, manage individual action plans, match job seekers with employer vacancies, and supervise service providers.
The IT systems of the SEA support almost all of these functions (Annex A provides a full overview). There are a few functions that do not exist in Latvia, including some more advanced vacancy matching features and skills profiling systems. However, simply digitalising these functions is not sufficient, it is important that these tools are optimised meet the needs of users as effectively as possible. This chapter thus provides a detailed assessment on the design and implementation of the digital tools supporting the core service delivery in the SEA. In particular, the chapter focuses on the SEA’s jobseeker profiling process, the vacancy matching platform, and the SEA’s future plans to improve skills profiling.
Section 4.2 looks at the SEA’s profiling tool. This tool divides jobseekers into three categories from closest to furthest from the labour market. However, the tool is manual, it takes time for counsellors to fill in and does not take advantage of existing data and does not leverage analytical techniques (like machine learning or regression analysis) to provide an evidenced-based assessment of jobseekers’ prospects. Section 4.3 turns to the SEA’s vacancy matching platform. The platform uses relatively simple filters to allow jobseekers and counsellors to search for vacancies, while counsellors can see which jobseekers match a particular vacancy. While this platform does help meet the needs of the SEA clients, it could do so more efficiently especially with more automation. Lastly, Section 4.4 looks at the SEA’s plans to implement digital skills profiling. The SEA currently only collects very basic self-declared information on jobseekers’ skills and is in the process of implementing new skills profiling tools that will better support counsellors to understand their clients and jobseekers to understand themselves.
4.2. The SEA uses a basic tool to profile jobseekers that could be improved
Copy link to 4.2. <strong><em>The SEA uses a basic tool to profile jobseekers that could be improved</em></strong>All PES have clients with varying levels of need and distance to the labour market. Profiling allows PES to understand these needs and levels of job-readiness. Profiling can take several forms, from relying only on the judgement of caseworkers through to statistical profiling models that leverage rich administrative data and sophisticated analytics to estimate a jobseeker’s distance to the labour market (see Box 4.1 for a discussion of the different profiling approaches). The SEA uses a “rules-based” profiling tool: counsellors collect information on jobseekers “risk factors” through an interview, the risk factors are then counted, and jobseekers are divided into three groups from most to least job-ready based on the number of risk factors they have. This section describes and assesses this profiling tool.
4.2.1. The SEA uses profiling to inform service provision by grouping jobseekers based on their job-readiness
Copy link to 4.2.1. The SEA uses profiling to inform service provision by grouping jobseekers based on their job-readinessProfiling is part of the initial registration process at the SEA. Its purpose is to gauge the distance of jobseekers from the labour market, which informs service provision. The current profiling system was introduced in 2019 to replace an older system that was not meeting counsellors’ and jobseekers’ needs.
Figure 4.1 illustrates the SEA’s profiling process and Annex B shows a screenshot of the profiling tool which is in the form of a spreadsheet. Profiling occurs during the jobseeker’s first interview, which the SEA aims to conduct within 30 days of registration as unemployed. This interview collects data on risk factors, which span a range of areas including demographic information (e.g. being under 18 or over 50), employment history, skills (including qualifications), care responsibilities, social issues (like addiction or criminal history), health, and motivation.
Most of this information must be collected in the interview and is not contained in the information system of the SEA called BURVIS. There are only a few exceptions. Age and disability data are held in BURVIS, and for the subset of jobseekers who have uploaded CV information at the time of profiling there are data on their stated education, language skills and preferred occupation. However, even in these cases such data are not linked to the profiling tool (i.e. no data are linked to the profiling tool, although for variables like age, and disability etc., they can be looked up by the counsellor in the BURVIS system). Thus, even when the data do exist in BURVIS, as the data are not linked to the profiling tool they are not in practice very useful for profiling. For example, given the counsellor is already interviewing the client, there is not much time‑benefit (or even a time loss) to the counsellor pausing the interview to look up information on the client in BURVIS and then manually entering it into the profiling tool (i.e. the spreadsheet), compared to simply asking the jobseeker to re‑supply the information (e.g. asking the client their age, alongside the other questions which must be asked anyway as no such data exist in BURVIS).
Once the form has been filled out, the risk factors are then tallied, and clients are segmented into three groups based on the total number of identified risk factors – with some risk factors counting for double. For example, if a person is aged over 50 (counts as one risk factor) and cares for a young child (counts as two risk factors) and has no other risk factors, then this person has a total of three risk factors and is classified in group 2 (3‑6 risk factors see Figure 4.1). Those with two or fewer risk factors are placed in the most job-ready group, those with three to six risk factors in the medium group, and those with more than six risk factors in the least job-ready group. Counsellors also have the right to place jobseekers in a different category from the one that corresponds to the number of their risk factors if they deem it necessary. About half of clients are classified as most job-ready with a little over a third in the middle category and about 10% in the group farthest from the labour market (data are from Q3 2019) (SEA, 2020[2]).
For clients with disabilities, a separate questionnaire is administered to gain a more comprehensive understanding of the person’s health issues. This questionnaire collects information about suitable work hours (e.g. total hours, need for breaks), remote work possibilities, and appropriate or inappropriate conditions (e.g. working outdoors, loud environments, work with chemicals, fast-paced or monotonous work, etc.). This survey is voluntary, and the jobseeker is not obliged to complete it.
A different set of services is recommended for each of the three groups, which informs the drawing up of an individual action plan for the jobseeker. Those further from the labour market have a wider array of services such as training or participation in public works schemes that may be recommended. These services are only recommended, providing counsellors with flexibility to deviate if necessary, though those in higher risk groups have been found to get more services (SEA, 2020[2]). If a jobseeker is profiled as low risk but has not found employment after many months, the jobseeker is not “re‑profiled” into a different group, but rather the counsellor will be aware of the lengthening unemployment spell and the action plan can be updated.
Figure 4.1. Profiling at the SEA
Copy link to Figure 4.1. Profiling at the SEA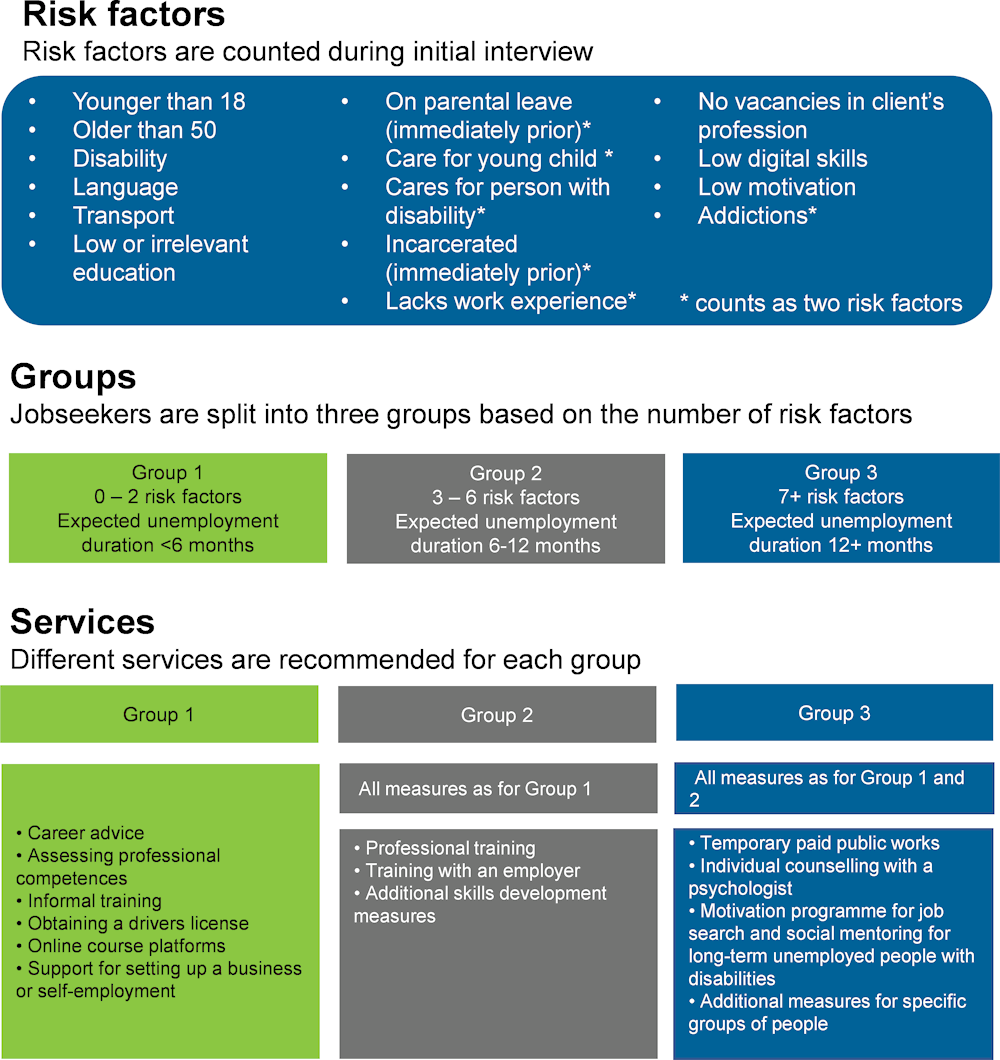
Source: OECD illustration based on information from Latvia’s State Employment Agency (SEA).
4.2.2. The SEA’s profiling system replaced an earlier needlessly complicated set up
Copy link to 4.2.2. The SEA’s profiling system replaced an earlier needlessly complicated set upThe current profiling system, introduced in 2019, is a significant improvement on the previous model. The prior profiling system was needlessly complex, dividing jobseekers into no fewer than 39 different groups (Table 4.1). Such a system was unwieldy to use, making it challenging to understand and explain the classification. The OECD recommended that this system be replaced by a more practical one (OECD, 2019[3]).
The current system dramatically streamlines this by reducing the number of groups to three. Additionally, the three groups follow a logical structure (most to least job-ready), using a simple and thus easy to understand algorithm to classify job readiness (simple tally of risk factors). This makes the profiling transparent and easy to understand.
One advantage of the previous profiling model, however, was that it made partial use of statistical profiling. That is, the profiling incorporated a statistical model that linked the characteristics of clients to the outcomes of similar clients in the past. This was partial statistical profiling as the tool used statistical profiling to group jobseekers by likelihood of finding a job, but the final classification also separated groups further based on their self-assessed skills and counsellor-assed motivation to arrive at the 39 different groups (OECD, 2019[3]). Statistical profiling is a key element of a modern profiling set-up (Box 4.1). However, SEA’s previous profiling system was so complicated (especially due to the 39 groups) that it was not useful for counsellors (SEA, 2020[2]). Moreover, the SEA had outsourced the model to an external provider. While outsourcing such expertise can be a sensible approach, in the experience of the SEA with the provider, the model was not able to be updated when needed and the SEA felt the model was out of date with respect to recent changes in the labour market. Ultimately replacing the previous tool was sensible given its lack of useability (SEA, 2020[2]; OECD, 2019[3]). However, the failings of the previous tool should not condemn statistical profiling per se, an approach which other countries have used with more success (OECD, 2018[4]). Rather the previous profiling tools shortcomings reflect implementation challenges (such as a poor experience with the provider) and other issues which are not limited to statistical profiling. Solutions to these potential issues are discussed below.
Table 4.1. The previous profiling tool used statistical profiling but could not be updated and divided clients into too many groups
Copy link to Table 4.1. The previous profiling tool used statistical profiling but could not be updated and divided clients into too many groupsComparison of current and previous profiling tool
Feature |
Old profiling tool |
Current profiling tool |
---|---|---|
Year introduced |
2013 |
2019 |
Linked to data in BURVIS? |
No |
No |
Used statistical profiling to link data on jobseekers to outcomes of previous clients with similar characteristic? |
Yes (partially) |
No |
How many profiling groups? |
39 |
3 |
Well understood by jobseekers and counsellors? |
No |
Yes |
Model maintenance outsourced to private provider? |
Yes but in practice the tool could not be updated as needed |
No/Not applicable as no statistical model |
Source: OECD compilation based on information provided by the SEA and in OECD (2019[3]), Evaluating Latvia’s Active Labour Market Policies, Connecting People with Jobs, https://doi.org/10.1787/6037200a-en.
4.2.3. Despite improvements the current profiling tool could be automated and make more use of evidence and data
Copy link to 4.2.3. Despite improvements the current profiling tool could be automated and make more use of evidence and dataWhile the simplification from the previous model is a positive development, the profiling tool still has several shortcomings. Most notably, it is a manual process and its measure of distance to the labour market is not data-driven, which could compromise accuracy.
Inputting profiling information manually is time consuming and does not allow data to be reused easily
Copy link to Inputting profiling information manually is time consuming and does not allow data to be reused easilyThe manual process of counsellors filling out a spreadsheet to count risk factors is not a modern and efficient approach. Firstly, it is time‑consuming, requiring counsellors to spend part of the first interview with the client asking questions in order to fill out the form. While some questions, the counsellor can refer to the SEA’s BURVIS information system this is not likely to save much time as the counsellor would have to copy manually the data into the Excel sheet for the profiling. Indeed, the remote online registration process collects some of the necessary profiling information digitally, which would facilitate the automation of filling in and processing profiling information.
Secondly, a manual process is potentially error-prone – e.g. if the number of risk factors is miscounted or if some risk factors are mistakenly missed. In contrast, an automated system could implement quality control checks that automatically detect errors and could potentially update or cross-verify information if data are updated in BURVIS.
A key drawback of the existing approach is not only that data entry is a manual task, but that its efficiency is scarcely better than a paper-based method. There is streamlined method for transferring the information from the Excel sheet into BURVIS, undermining what could be a key advantage of a digital solution. This means that the information gathered during profiling is not stored in a way that can be systematically searched digitally and used in later analytics. Only the final profiling score is retained in BURVIS, but the risk factors themselves – and therefore the reasoning behind the counsellor’s decision – are not well captured. Not properly integrating the risk factor data to BURVIS misses an opportunity to curate data on individuals that could be useful later. For example, in research and monitoring, detailed information on risk factors would enable the SEA to better understand outcomes (such as unemployment duration, likelihood of finding employment, and earnings among others) for different groups, the quality of services provided to them, and the effectiveness of these services and programmes (in the case of counterfactual impact evaluations). Such individual information might also be better presented to counsellors through data analytics and visualisation tools, aiding counsellors to better understand their portfolio of clients and the SEA management to better understand the distribution of risk factors identified.
The SEA recognises the need to automate profiling. An internal report in December 2020 recommended changes to BURVIS to automate aspects of profiling (using the information on risk factors already held in BURVIS), with the option for counsellors to further add and amend information (SEA, 2020[2]). However, due to resource constraints, these changes have yet to be implemented.
The SEA’s model is not data driven or as accurate in predicting unemployment duration as it could be
Copy link to The SEA’s model is not data driven or as accurate in predicting unemployment duration as it could beThe current “model” part of the profiling system – i.e. simply counting risk factors for longer unemployment duration – can be classified as an arbitrary rules-based algorithm. While this method is easy for counsellors to understand, it does not necessarily provide an accurate prediction of jobseekers’ unemployment duration.
For instance, by counting the number of risk factors, each risk factor is arbitrarily assigned a weight of one (or two in cases where the risk factor counts double) without quantifying the empirical link between the risk factor and unemployment duration. It seems improbable, for example, that lacking Latvian language skills, having a child under pre‑school age, and lacking digital skills represent the same risk of a long unemployment spell. Nor does it seem likely that those recently released from prison have similar employment prospects to those returning from paid parental leave or to someone who has never worked.
Indeed, during the introduction of the profiling scheme in 2019, an internal study of its effectiveness was conducted (SEA, 2020[2]). This study identified inaccuracies in the profiling model – noting that many people in each group found a job earlier or later than expected. As the study found that on average jobseekers found a job sooner than anticipated, the study recommended that the number of risk factors needed to qualify for a more disadvantaged group be increased. As a result, the cut-off for group three was raised from 6 to 7 risk factors, but this was an ad hoc approach and still treats risk factors with a weight of only 1 or 2 and does not account for interactions and interdependencies among risk factors and other variables.
An alternative approach would be to use a data-driven model, as is the case in many other OECD and EU countries that perform statistical profiling (OECD, 2018[4]; Desiere, Langenbucher and Struyven, 2019[5]) and as was used previously but ineffectively in Latvia (see Section 4.2.2 above). Such a data-driven approach can be expected to perform better and deliver more accurate predictions than the approach that the SEA currently uses which is not linked to data, with many countries achieving a high-degree of accuracy (Desiere, Langenbucher and Struyven, 2019[5]).
Of course, statistical models are not without their own limitations and, as discussed in Section 4.2.2, the SEA’s previous experience with statistical profiling highlighted several shortcomings. In particular the model was opaque and not found useful by counsellors, while there were also issues keeping the model up to date and accurate. Each of these shortcomings could be addressed with a different implementation approach.
For example, to ensure the model is up to date with the latest labour market trends, the model could be designed in a way that it can be easily updated by the SEA (without a private provider’s support), such as by designing the model so that it automatically re‑runs regressions (or a machine learning algorithm) on the latest data. However, this would require that recent data on jobseeker’s outcomes and characteristics can be provided to the model – as would also need to be done when relying on an external provider. Alternatively, funding could be allocated to regularly re‑estimate the model using recent data and an agreement reached with a private provider.
To ensure counsellors understand and use the tool adequate training, guidelines, and systematic user support should be provided. Indeed, any new profiling tool should involve counsellors in the development, testing, and roll-out phase (Desiere, Langenbucher and Struyven, 2019[5]). Effective engagement with counsellors in these ways could avoid another major issue the SEA had with its previous tool, namely that tool was not well understood or useful for counsellors.
Box 4.1. Many countries make use of statistical profiling tools to support jobseekers
Copy link to Box 4.1. Many countries make use of statistical profiling tools to support jobseekersMany countries use statistical profiling tools to help deliver PES services more efficiently (Desiere, Langenbucher and Struyven, 2019[5]; OECD, 2018[4]). Such tools use a data driven and evidenced-based approach to understanding how far a jobseeker is from the labour market.
Types of profiling
Copy link to Types of profilingAll PES work with clients who have varying needs and who are at different levels of job-readiness. To allocate resources effectively, PES use various forms of profiling, but not all of them use statistical profiling. Indeed, there are several different types of profiling including:
Caseworker judgement – relies on the judgment of caseworkers to assess needs.
Rules-based profiling – uses eligibility criteria (e.g. age, unemployment duration etc.).
Statistical profiling – uses a statistical model to predict disadvantage.
The advantage of statistical profiling over rules-based profiling and caseworker judgment is that it makes use of evidence and data (most often employment duration or probability of finding employment over a set period – e.g. the next six months). Caseworkers and such models can complement each other with the model informing caseworker decisions who may deviate from the profiling score if they think it is warranted (e.g. if they observe aspects of a clients situation not captured by the model).
Leveraging analytics and linked administrative data
Copy link to Leveraging analytics and linked administrative dataA profiling model aims to summarise a client’s situation and so requires a comprehensive picture of the client’s background. This requires data from many sources. While much useful information is held by the PES (e.g. demographic information and basic labour market histories captured during profiling), linking in administrative data from other agencies (e.g. on benefit histories, rich employment histories, and health to name a few) can provide an even more complete picture without the need for further questioning of jobseekers. The use of such linked data reduces the need for questionnaires, saving time for jobseekers and potentially counsellors if they administer the questionnaires.
While rich data form an excellent foundation for profiling, evidence‑based profiling requires analytical solutions to accurately measure how far a jobseeker is from the labour market. Commonly PES will use such data to predict employment outcomes based on previous jobseekers’ experiences. Such models can look at the complex association between clients’ characteristics and outcomes. Statistical models that do this include regression-based analysis, or more sophisticated methods such as machine learning models, with different PES trying different models.
Source: Desiere, Langenbucher and Struyven (2019[5]), “Statistical profiling in public employment services: An international comparison”, https://doi.org/10.1787/b5e5f16e-en; OECD (2018[4]), Profiling tools for early identification of jobseekers who need extra support, http://oecd.org/els/emp/OECD-Profiling-policy-brief-DEC-18.pdf.
4.3. The SEA uses a rules-based digital tool to help match jobseekers to vacancies
Copy link to 4.3. The SEA uses a rules-based digital tool to help match jobseekers to vacancies4.3.1. The SEA holds data on jobseekers and vacancies, with many vacancies added manually by counsellors
Copy link to 4.3.1. The SEA holds data on jobseekers and vacancies, with many vacancies added manually by counsellorsThe SEA maintains a database of both jobseekers and vacancies. Data on jobseekers are collected largely during the registration, the initial interview process and through updates to the jobseekers’ profile in the online CV and Vacancy Portal (CVVP). Data on job vacancies are provided by employers. Employers can list vacancies directly on the SEA’s CVVP which helps automate the process, however around half of vacancies are shared with the SEA via email or phone. In such cases counsellors must manually enter information on the vacancy into the system. State and local government authorities must list their vacancies with the SEA, however there is no requirement that private sector employers do so unless the vacancy is filled with a third-country national (i.e. individuals from who require a work permit to become employed). In such cases, the vacancy must have been listed with the SEA for at least 10 working days in order for the local workforce to potentially apply.1 The SEA’s vacancy database performs well at attracting vacancies. It is the largest in Latvia, with vacancy stock varying from around 18 200 to 26 600 in 2021 (with the trough in Q1 during Northern winter, and the peak in Q3 during Northern summer) or around 80% of the total vacancies in Latvia reported by Latvia’s central statistics bureau (European Commission, 2023[6]). Good outreach to employers is crucial to help meet their needs, maintain engagement, and to ensure employers continue to list their vacancies with the SEA.
Many employers advertise relatively lower skilled jobs with the SEA. The SEA would thus ideally like to attain more high skilled vacancies. This can be helpful both in increasing the availability of jobs to jobseekers and ensuring there are no stigmas or stereotypes associated with jobseekers registered at the SEA – i.e. that the SEA has a good reputation for filling vacancies at a range of skill levels.
4.3.2. Simple rules-based filters support matching
Copy link to 4.3.2. Simple rules-based filters support matchingCounsellors and jobseekers can browse vacancies and use filtering criteria to find vacancies. Figure 4.2 shows a screenshot of the vacancy matching portal available to jobseekers. Available criteria for matching include key word search, salary, industry (listed as “scope” in Figure 4.2), type of employment (e.g. indefinite contract, fixed term contract, among other contract types), location, company, application closing date (“current until”), remote working options, whether the job is seasonal, and whether it involves travel abroad. Jobseekers may also search for vacancies based on geographic location, through an interactive map. If a jobseeker is interested in applying for a vacancy, the contact details are listed allowing the jobseeker to directly apply for the job (except in rare circumstances where the employers mark the contact details as private, and the jobseeker must apply for the vacancy through the SEA). The job searching space withing the CVVP portal is open (anyone can access it) making it available for jobseekers who may not be registered as unemployed (for example those who are working and looking to change jobs). Indeed, jobseekers do not need even to create a profile to access this information.
While this system is simplistic in comparison to some of the more advanced matching algorithms available in other countries, the vacancy matching tool does supports counsellors to quickly identify the clients that are best placed to fill a vacancy. Counsellors can then present a shortlist of clients to employers who may further decide who to interview for the role. Possible areas that could be improved would be to increase the automatic collection and processing of vacancies. In some countries, more advanced matching algorithms are used to support matching of vacancies to jobseekers (Box 4.2).
Another issue identified with vacancy matching at the SEA is the challenge users face in comprehending the classification of occupations and their associated competencies. The SEA uses the European Union’s (EU’s) employment, skills competences, qualifications and occupations (ESCO) taxonomy. While PES within the EU are obliged to use the ESCO classification for the EU’s European Employment Services (EURES) job vacancy platform, it has at times been difficult for employers and jobseekers to use, as they do not always know which category they should classify their skills/occupations which are listed in a high degree of detail. One approach to improve on implementation could be to use AI rather than employers and jobseekers to connect the information in job advertisements and CVs to the ESCO classification system, with for example Finland using a matching system along these lines (OECD, 2022[1]).
Figure 4.2. The SEA provides an online portal to search for vacancies
Copy link to Figure 4.2. The SEA provides an online portal to search for vacanciesScreenshot of Latvia’s State Employment Agency’s (SEA) online vacancy portal (CVVP)
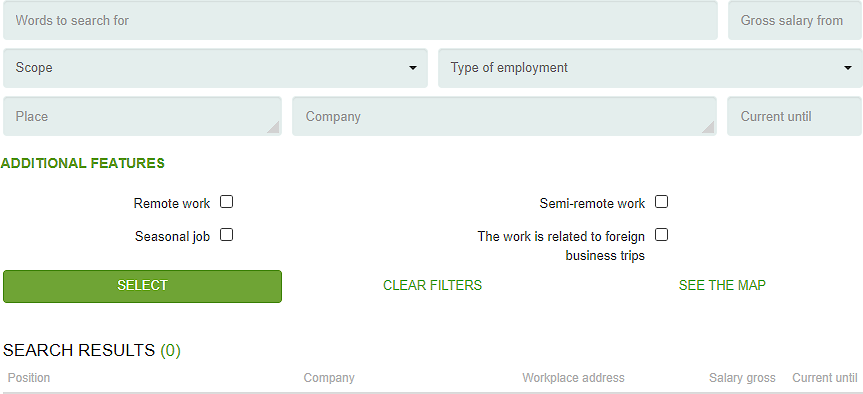
Note: Text has been matching translated into English from Latvian by the web browser. “Scope” could be better translated into “industry” – examples include construction, banking, security, etc.
Source: SEA (n.d.[7]), https://cvvp.nva.gov.lv/#/pub/vakances/saraksts (accessed on 30 June 2023).
The CVVP enables jobseekers to browse vacancies, while the BURVIS portal enables counsellors to browse jobseekers. This allows counsellors to search for those jobseekers that match the employers’ criteria. Matching criteria include:
Experience required in vacancy
Education
Language skills
Computer skills
Driving license
Driving license for heavy vehicles
Travel distance to workplace
Skills and competences (these are very basic – self-declared computer skills and driver’s license).
Wage
Work type (full time, part time).
Box 4.2. Some countries use a range of advanced digital tools to support vacancy matching
Copy link to Box 4.2. Some countries use a range of advanced digital tools to support vacancy matchingTo help facilitate matching, digital tools are used in OECD countries in a variety of ways (OECD, 2021[8]). These include to:
“Crawl” the internet for vacancies. Algorithms can search the internet and other vacancy databases to find vacancies that can automatically be added to the PES vacancy database. This has been done for example in the Netherlands and Austria.
Support employers and private employment services to automatically upload and share vacancies with the PES. For example, Finland is working on increasing its use of application programming interfaces (APIs) to collect more vacancies from private employment services, while Sweden works with the largest advertisers to collect more vacancies (links.api.jobtechdev.se/). The Flemish PES, VDAB, has tools that allow large employers and private employment agencies to automatically upload large numbers of vacancies.
Predict which companies are hiring. In a particularly innovative approach France has a tool, La Bonne Boîte (labonneboite.pole-emploi.fr), that predicts, based on past recruitment trends, which employers are likely to be hiring. Jobseekers are then encouraged to cold-call such prospective employers.
Perform advanced matching using AI rather than a simple rules-based approach. For example, VDAB has used an algorithm that predicts what vacancies jobseekers are likely to find most interesting so as to show jobseekers search results they may find most relevant. The tool uses a very wide variety of information, including not only detailed characteristics of the skills and qualifications required but also the “click” data from job search histories of the PES database and rich demographic information to predict what similar jobseekers would find interesting. This tool is discussed in detail in OECD (2021[8]).
Key information to assess such tools is missing making it hard to determine their effectiveness. To form a good assessment of such tools one would want strong evidence on the costs and benefits. However, unfortunately information on costs is rarely public – though the range is wide and some tools can be costly. VDAB for example has a 25‑person strong AI team to support its many digital tools (OECD, 2021[8]). On the benefit side one would ideally have rigorous counterfactual impact evaluations, but these too do not seem to exist or are otherwise not published.
Nevertheless, given that such tools could benefit many jobseekers and employers it is possible they allow for great improvements to PES service delivery, and the willingness of some PES to invest in these tools shows that PES believe the tools are worthwhile.
Source: OECD (2021[8]), New ways to reach out to employers, http://oecd.org/els/emp/Employers.pdf.
4.4. The SEA is looking to implement skills profiling
Copy link to 4.4. The SEA is looking to implement skills profilingDigital skills profiling tools measure jobseekers’ skills and can serve a wide range of purposes, from providing insights to counsellors and self-knowledge to jobseekers, to assisting with matching jobseekers to employers or to training programmes among other possible purposes (Box 4.3) Currently, SEA collects only very basic self-declared information on a small number of skills, such as computer skills and driver’s licenses. At present, the SEA is seeking to develop skills profiling to better support jobseekers and counsellors, with plans to introduce skills profiling tools in a staggered approach. This has been supported by this project including through the development of a technical specification for a new digital skills profiling tool (OECD, 2023[9]).
4.4.1. The SEA wishes to support counsellors and jobseekers better understand jobseekers’ skills
Copy link to 4.4.1. The SEA wishes to support counsellors and jobseekers better understand jobseekers’ skillsGiven the range of different purposes that skills profiling tools can serve, it is essential to clarify early in the process of designing and adopting such tool the specific purpose it will serve. The OECD, DG REFORM, the SEA and the MoW, jointly organised a workshop on skills profiling in March 2023.2 At this workshop the MoW and the SEA tentatively agreed that the primary purpose of the tool would be to support counsellors to better and more quickly understand their clients, and to help jobseekers gain self-knowledge of their skills and interests and explore ideas for occupations they should seek (OECD, 2023[9]). By using a skills profiling tool, people can better assess their suitability for different occupations.
To allow counsellors to access the results of this test of jobseekers, the tool will need jobseekers to provide an identity to link the data to (such as the Latvian e‑identity) and then an API to link data from the tool into BURVIS (OECD, 2023[9]). This would also allow the skills profiling data to be used in other data analysis exercises (such as monitoring or evaluation).
Box 4.3. Skills profiling tools are used in many countries to support PES
Copy link to Box 4.3. Skills profiling tools are used in many countries to support PESSkills profiling tools can serve a range of purposes in PES including to:
Provide insights and self-knowledge to jobseekers.
Support jobseekers to explore occupations and jobs that match their skills and interests.
Match jobseekers to appropriate training which may be hosted externally.
Support employment counsellors: This could be in a variety of ways including through helping employment counsellors understand their clients or by suggesting suitable occupations (job search strategies, training, or vacancies).
Support PES management and policy makers by proving an overall picture of the skills of PES clients (i.e. information on skills supply on the labour market).
Accredit jobseekers with a certificate so they can demonstrate their skills to potential employers (e.g. on their CVs).
Form follows function – skills profiling tool purpose should determine other design choices. Deciding which of these potential purposes the tool aims to meet is thus critical as this informs all other design choices. Such design choices include how skills are measured (e.g. self-described, a more objective knowledge-based test, or a psychological test to measure personal fit), the types of skills measured, who will take the test (e.g. whether it is public), whether data is linked to other PES systems (e.g. so counsellors can access it), and whether the test is one test or rather made up of many optional modules.
4.4.2. Introducing skills profiling in a staggered approach
Copy link to 4.4.2. Introducing skills profiling in a staggered approachTo support skills profiling the SEA is initially seeking to digitise two tests. One test will focus on profiling skills while the other will examine working conditions.
The “skills profiling test” will assess skills in five domains. Namely, personal, cognitive, social, technical, and management skills. For each skill area there will be 15 questions. Each question will present a statement, with individuals asked to rate how much they agree with the question on a scale of one point (strongly disagree) to five points (strongly agree). A total score for each skill category will be calculated by summing up the answers to each question, so that higher scores represent higher subjectively-rated skills.
The other test, “skills assessment in different work settings”, will work similarly but will focus on working conditions. Seven different areas will be covered, namely: physical demands, working outdoors, working indoors, working in hazardous conditions, fixed working mode (e.g. specific place, hours etc.), flexible working modes, and cognitive/intellectual demands.
Clients and counsellors will reflect jointly on the results of these tests. One feature of these tests worth noting is that they both rely on the jobseekers’ awareness, judgement and honesty when answering the questions. However, the advantage of these skills profiling tests is that both tests are easy to understand and quick to undertake with the skills test taking about 25 minutes to complete on average and the working conditions test taking around 15 minutes. At this stage the SEA has launched procurement processes only for the tests themselves, but they intend for the test to be designed in a way that API’s can transfer the information from the test into the BURVIS information system.
These two tests will utilise a modest amount of funding the SEA has available from the EU’s Recovery and Resilience Facility (RRF) in 2023 for developing skills profiling tools.
4.4.3. Latvia is looking to learn from other countries to further develop skills profiling tools
Copy link to 4.4.3. Latvia is looking to learn from other countries to further develop skills profiling toolsWhile the two tests described above can be designed and deployed quickly, Latvia is also working on developing more sophisticated skills profiling that will take longer to develop. To do this, Latvia is keenly interested in learning from and building on the experiences of other countries. At the March 2023 workshop, the SEA and MoW learned about skills profiling practices and developments in several other countries Latvia is hoping to leverage the learnings of other countries further potentially building on the digital infrastructure and assessment procedures (questions and scoring) of other countries’ platforms. For example, the SEA and MoW have been actively engaging with the French public company Pix which develops digital skills profiling and, in an excellent example of international co‑operation, Latvia is also working with the German PES to see if it is possible to leverage not only the questionnaires, but the code and digital infrastructure of Germany’s skills profiling tool. The aim is to see if this infrastructure can be modified so as to also serve the needs and purpose of Latvia. Such co‑operation would help avoid duplication of effort across European countries and potentially save Latvia much time and effort. This co‑operation relies on the efforts of other countries to share resources such as their code and questions.
Turning to synergies within Latvia, there are strong links between the needs of skills profiling at the SEA and those of the Ministry of Education, with the Ministry of Education interested in understanding the skills of the entire adult population (not only jobseekers which is the priority of the SEA). To provide complimentary approaches and synergies and avoid any unnecessary duplication, the SEA, the MoW and the Ministry of Education and Science will need to maintain close dialogue with each other.
References
[5] Desiere, S., K. Langenbucher and L. Struyven (2019), “Statistical profiling in public employment services: An international comparison”, OECD Social, Employment and Migration Working Papers, No. 224, OECD Publishing, Paris, https://doi.org/10.1787/b5e5f16e-en.
[6] European Commission (2023), Job Vacancy Statistics: National Reference Metadata in ESS Standard for Quality Reports Structure (ESQRS), https://ec.europa.eu/eurostat/cache/metadata/EN/jvs_esqrs_lv.htm (accessed on 21 July 2023).
[9] OECD (2023), A note on a technical specification proposal for a skills profiling tool, unpublished.
[1] OECD (2022), Harnessing digitalisation in Public Employment Services to connect people with jobs, OECD, Paris, https://www.oecd.org/els/emp/Harnessing_digitalisation_in_Public_Employment_Services_to_connect_people_with_jobs.pdf.
[8] OECD (2021), New ways to reach out to employers, research note, http://oecd.org/els/emp/Employers.pdf.
[3] OECD (2019), Evaluating Latvia’s Active Labour Market Policies, Connecting People with Jobs, OECD Publishing, Paris, https://doi.org/10.1787/6037200a-en.
[4] OECD (2018), Profiling tools for early identification of jobseekers who need extra support, Policy Brief on Activation Policies, OECD, Paris, http://oecd.org/els/emp/OECD-Profiling-policy-brief-DEC-18.pdf.
[2] SEA (2020), Bezdarbnieku profilēšanas metodes pilotprojekta rezultāti [Results of the pilot project on profiling the unemployed], Unpublished.
[7] SEA (n.d.), Latvia’s State Employment Agency’s (SEA) online vacancy portal (CVVP), https://cvvp.nva.gov.lv/#/pub/vakances/saraksts (accessed on 30 June 2023).
Annex 4.A. Latvia’s responses to the OECD AI survey
Copy link to Annex 4.A. Latvia’s responses to the OECD AI surveyAnnex Table 4.A.1. The SEA responses to the OECD questionnaire on digitalisation in PES
Copy link to Annex Table 4.A.1. The SEA responses to the OECD questionnaire on digitalisation in PES
Online solutions for jobseekers, people at risk of job loss, citizens |
Online solutions for employers and service providers |
User interfaces for PES staff |
---|---|---|
Functions the SEA has |
||
Apply for registration / register with the PES |
Register (post) a vacancy |
Identify potential clients proactively to support outreach – including inactive people, people leaving the prison system, etc. |
Create CVs and job application documents |
Advertise vacancies |
Profile jobseekers |
Provide information for PES on skills, education etc. |
Find suitable employees |
Map jobseeker’s distance to occupations and gaps in competencies |
Test skills |
Receive information and counselling, including via chatbots and conversation bots |
Recommender systems in career services based on analysing expected skills by employers and previous career choices of workers |
Analyse suitable career paths based on jobseeker’s interests |
Share information with PES (on hired jobseekers, filled vacancies) |
Develop and manage individual action plans |
Report job-search activities |
Apply for measures for employers (employment incentives, staff training etc.) |
Monitor clients’ job search activities |
Receive info on available services and measures and their eligibility conditions, including via chatbots |
Share information on using PES measures (wage data for employment incentives etc.) |
Monitor clients’ participation in services and measures |
Find suitable training options |
Share participant data (jobseeker data, contacts) |
Match jobseekers to vacancies |
Participate in virtual training |
Share data on jobseeker participation (no-shows, drop-outs) |
Process jobseeker applications for registration |
Apply for services and measures (including training) |
Process unemployment benefits, unemployment assistance and other benefits |
|
Apply for unemployment benefits, unemployment assistance or other income support benefits |
|
Identify entitlement for unemployment benefits, unemployment benefits and other benefits (proactively) |
Choose a provider (training provider, private provider of employment services) |
|
Monitor activities of contracted service providers |
Provide feedback on services and measures (satisfaction) |
|
Share information on suitable candidates with employers |
Find suitable vacancies |
|
|
Apply for suitable vacancies |
|
|
Functions the SEA does not have |
||
Map jobseeker’s distance to occupations and gaps in competencies |
Identify vacancies proactively – companies with high recruitment likelihood |
Target ALMPs – receive recommendations for the type of support to provide to different profiles of PES clients |
Recommender systems in career services based on analysing expected skills by employers and previous career choices of workers |
Share performance monitoring data |
Refer clients to ALMPs |
|
Apply to become a co‑operation partner / receive accreditation, certification |
Process applications for services and measures by jobseekers |
|
|
Process applications by employers, service providers |
|
|
Identify vacancies proactively regarding companies with high recruitment likelihood |
|
|
Pool vacancies from other portals (web crawl) |
Note: PES – public employment service. This table shows the functions that the IT infrastructure in in the Latvian PES (State Employment Agency) supports. Functionalities related to unemployment benefits are not shown as these are not managed by the PES in Latvia.
Source: OECD questionnaire on digitalisation in Public Employment Services to support the provision of active labour market policies launched in March 2023.
Annex 4.B. The SEA’s profiling tool
Copy link to Annex 4.B. The SEA’s profiling toolAnnex Figure 4.B.1. The SEA’s profiling tool spreadsheet
Copy link to Annex Figure 4.B.1. The SEA’s profiling tool spreadsheet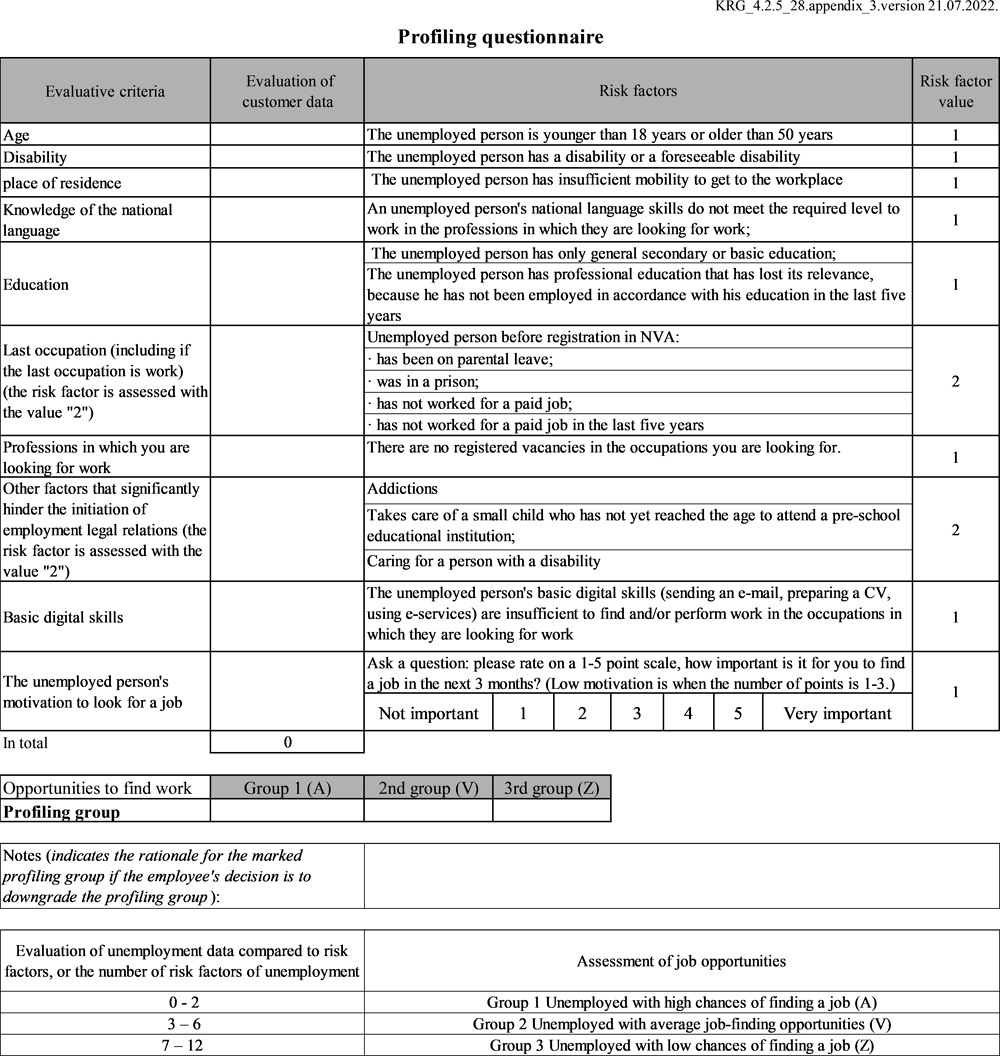
Note: This form has been machine translated from Latvian into English.
Source: Latvia’s State Employment Agency (SEA).
Notes
Copy link to Notes← 1. This requirement, while increasing the number of vacancies posted with SEA, in fact can make it more difficult to gauge the true number of job openings in practice. Employers may artificially inflate the number of job vacancies posted in order to ensure that a sufficient number of vacancies remain unfilled from the local workforce, ensuring that they are able to get a permit to employ third-country nationals.
← 2. Agenda and presentations available at: www.oecd.org/els/emp/Digital_tools_workshop07032023_Agenda_and_concept%20note.pdf.