The State Employment Agency in Latvia (SEA) needs to modernise its digital backbone to better support jobseekers, persons at risk of unemployment and employers in Latvia. Key priorities for the SEA are to develop a comprehensive digitalisation strategy to establish the objectives and relevant frameworks, and develop a dedicated analytics system consisting of a data warehouse and a Business Intelligence tool. In addition, the SEA should consider to gradually improve the design and implementation of the jobseeker profiling tool, continue co‑operating with other organisations to find feasible solutions for skills profiling, and move towards competency-based job matching. While the SEA does not have an urgent need to fundamentally change or replace its main operational IT system in the near future, some fine‑tuning will be necessary, particularly concerning system and data security, and data quality management.
Modernising Latvia’s Public Employment Service through Digitalisation
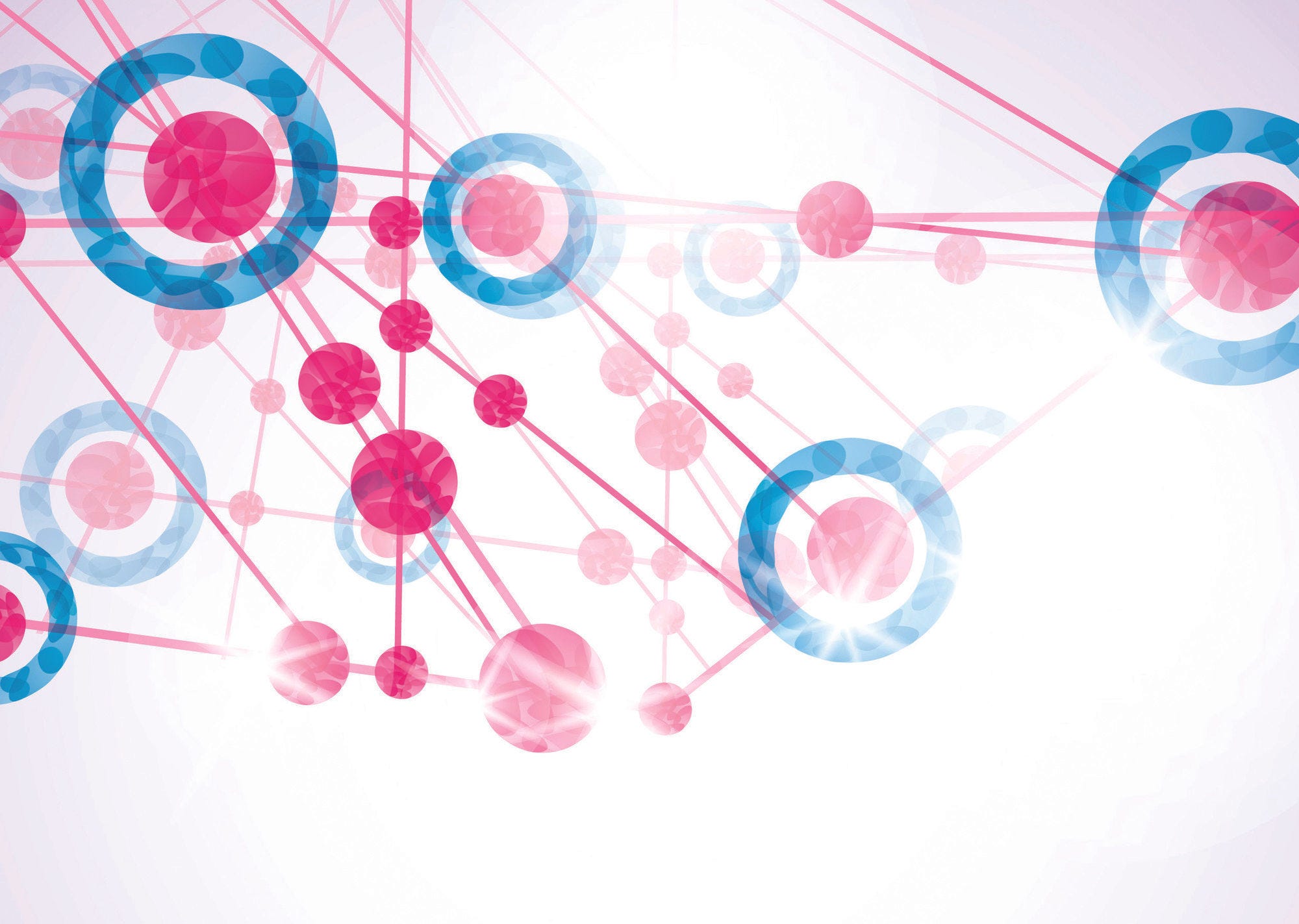
6. Detailed recommendations for modernising the State Employment Agency
Abstract
6.1. Introduction
This chapter provides detailed recommendations to support the State Employment Agency (SEA) to modernise its digital backbone and help the SEA in its role to help jobseekers, persons at risk of unemployment and employers in Latvia. This chapter, building on the assessments in Chapters 2 to 5 and complementing the high-level recommendations made in Chapter 1, makes detailed in-depth technical recommendations for improvements in the overall IT infrastructure of the SEA, as well as specific digital tools within the broader system, particularly the jobseeker profiling, skills profiling and job matching tools.
Overall, the SEA does not have an urgent need to fundamentally change or replace its main operational IT system in the near future, although some fine‑tuning to the system should be feasibly made, particularly concerning system and data security, and data quality management. Key priorities for the SEA are to develop a comprehensive digitalisation strategy to establish the objectives and relevant frameworks, and start the development of an advanced data analytics system consisting of a data warehouse and a Business Intelligence (BI) tool. In addition, the SEA should consider to gradually improve the design and implementation of the jobseeker profiling tool, continue co‑operating with other organisations to find feasible solutions for skills profiling, and move towards competency-based job matching.
6.2. Capacity of the system of active labour market policies in Latvia
Empirical evidence from counterfactual impact evaluations have demonstrated that Latvia’s ALMPs help people become employed, but Latvia’s low funding levels for ALMPs limits their reach. Past impact evaluations, including one conducted by the OECD, indicate that training and (private sector) wage subsidy programmes are effective at placing most groups of jobseekers into employment. These evaluations have employed counterfactual impact evaluation techniques to account for the counterfactual (unobserved) outcomes that individuals would have experienced if they had not participated in ALMPs. This approach allows to identify the effects that can be directly attributed to programme participation. For almost all groups of participants, the training and (private sector) wage subsidy programmes that were examined were found to improve participants’ labour market outcomes, such as employment probability and earnings (although the magnitude of the effects did vary across programmes as well as groups of participants). However, Latvia’s spending on ALMPs remains much lower than that of other OECD countries, with funding remaining largely reliant on EU funds (which from past experience have sometimes been associated with difficulties in implementation). Increasing ALMP funding from the national budget could thus expand the reach of ALMPs and improve labour market outcomes for more jobseekers, as well as people at risk of job loss.
Continuing to regularly conduct impact evaluations and further strengthening the evaluation activities, including on the effectiveness of more intensive and skilled counsellor-based support, could also help strengthen the business case for increasing ALMP funding. For example, several studies in other OECD and EU countries have shown a positive effect of increasing the intensity of counselling, especially in situations where jobseekers are otherwise not subject to frequent counselling in practice (Schiprowski, 2020[1]; Crépon et al., 2013[2]; Glover, 2019[3]; OECD, forthcoming[4]). However, the effects of PES actions and ALMPs can vary across the design and implementation of the specific intervention, the economic and labour market context, as well as different groups of jobseekers (or even across different types of counsellors implementing the intervention, or the level of counsellor skills and qualification). Rigorous impact evaluations could thus help establish the extent to which different interventions are (cost) effective and provide an empirical justification for hiring more (skilled) staff in the SEA, upskilling the SEA staff and supporting them with better (digital) counselling tools, as well as making the business case for higher wages, and/or increasing spending on other ALMPs.
More broadly, increasing the wages of the SEA staff – possibly in parallel with a strengthened monitoring and accountability framework – could also address some of the challenges discussed in this report. In particular, many of the IT challenges require experts who may command higher wages in the private sector, with departures of qualified staff further compounding the challenges for those who remain. Even if large components of the IT development and operations are contracted-out to external providers, having suitable numbers of competent staff in-house is important even if their role is limited to managing the contracts with the external providers. To the extent feasible within the public sector wage system, introducing performance‑based pay could help introduce some financial incentives for performance while attracting a wider array of staff. This should be done within a broader increase in the budget available for paying PES counsellors (so that their average wages would increase with such a reform).
6.3. Digitalisation strategy and resources
Digitalisation is addressed extensively in the SEA’s general strategy for 2021‑23, rightfully recognising the SEA’s IT infrastructure as a key prerequisite to achieve the set objectives, and increasing the effectiveness, efficiency, accessibility and user-friendliness of the SEA’s services.
6.3.1. A dedicated digitalisation strategy would pave a clear pathway to modernisation
The SEA could consider developing a dedicated digitalisation strategy to address the aspirations and challenges more comprehensively and paving a more systematic pathway for modernisation, going beyond the few key digitalisation projects highlighted in the general strategy of the SEA. According to recent information collected by the OECD from most OECD and EU countries, about a quarter of PES systems in the OECD and EU countries have a dedicated digitalisation strategy (Brioscu et al., Forthcoming[5]). Another quarter has included a digitalisation component in the general PES strategy similarly to the SEA, while half of the PES do not follow a strategy in their digitalisation projects or use only related national strategies, concepts and guidelines that involve all organisations in the public sector or beyond.
The digitalisation strategy should declare the overall objectives and principles for the digital transformation, helping to prioritise any related investment and providing a clear framework for digitalisation. For example, Australia has articulated four key shifts in its Digital Business strategy for employment services: i) simpler access, ii) consistent experience, iii) data driven services, and iv) modern, connect and digital – all of this to be delivered by leveraging modern platforms and approaches. Austria has laid out in its PES digitalisation strategy a roadmap for five to eight years, aiming to fundamentally renew PES digital backbone technically and functionally, starting with the placement and online services. The Swedish PES has both a Digital transformation strategy and a Digital strategy to comprehensively lay down the relevant objectives and principles, and the PES system in Korea has a thorough digital strategy to holistically address their digitalisation and data management journey (see Box 6.1).
Box 6.1. Strategies for PES digitalisation in Korea and Sweden
A strategy for a “digital first” approach in Sweden
The Swedish public employment service (PES) has a comprehensive digital strategy. The main principles of the digital strategy are to: provide a digital ecosystem, provide data security, have data readily available, and to achieve value for customers, including through a “digital first” set up.
The digital strategy aims to achieve more efficient matching of jobseekers with vacancies and an improved and more productive working environment at the PES. The Swedish PES hopes that the strategy will provide an efficient internal development process that supports a cohesive delivery to customers.
A comprehensive “digital first” approach is a key part of the strategy. “Digital first” concerns everyone at the PES, and includes more digital meetings, and more digital solutions for PES customers to meet their needs. Other design principles beyond the “digital first” approach include a customer and solutions focus, developing tools for accessibility and inclusion, delivering fast, adopting data-driven insights, and maintaining transparency.
Better informing PES customers in Korea
Korea also has a digital strategy for PES. The objectives of the digital strategy include increasing the use of employment services, making sure clients are better informed about employment services, and better providing labour market information to customers.
Korea hopes to achieve these objectives using several methods. This includes making sure that employment services are delivered in different ways so that customers can choose the method that suits them best. Korea will also ensure information on employment services is clear and comprehensive, and that the information only needs to be collected once from customers (once‑only data collection principle to be achieved via data exchange across administrative registers).
Korea recognises that different customers need different support. To tailor support, Korea plans to make better use of data and artificial intelligence. It also aims to target support depending on the customer’s age and stage in life. Furthermore, Korea is implementing counselling support that is tailored to jobseekers’ competencies and skills.
Further into the future, Korea will provide a “one‑stop” online employment service for individuals and companies called Employent24. Employment24 will provide “next-generation” digital employment services, that combine information on jobs, vocational training, and grants. It will also provide information on services available to employers including on training and wage subsidies for employees. Career development information will be provided to jobseekers through JobCare, a platform for comprehensive resources for career management.
Source: OECD questionnaire on digitalisation in Public Employment Services to support the provision of active labour market policies launched in March 2023; OECD (forthcoming[6]), Strengthening Active Labour Market Policies in Korea; OECD (2023[7]), “Addressing the legal and IT challenges of data exchange to support contracted-out employment services in Sweden,” www.oecd.org/els/emp/Sweden_Challenges_Data_Exchange.pdf.
6.3.2. The digitalisation strategy needs to propose a route to achieve sustainable budget and mechanisms to ensure cost-effective investments in digitalisation
Moreover, the SEA should think through how to achieve sustainable financial and human resources for IT developments while developing the digital strategy. Continuing with limited and project-based resources poses serious risks for a more sustainable modernisation and comprehensive digital approaches, enables only a patchwork of adaptions and functionalities, as well as forces more often to opt for a scaled-down versions than the ideal. Nevertheless, even in the context of a more viable digitalisation budget, bringing in additional project-based resources (e.g. EU funding) and co‑operating with other Latvian and international organisations would remain important to maximise the resources available for the digital transformation. Thus, the mechanisms to attract additional funding and build partnerships should be addressed in the SEA digital strategy.
The SEA digitalisation strategy should also address how to maximise the value‑added of its new and updated digital solutions, i.e. to maximise the effectiveness and efficiencies that these solutions incur functionality wise, as well as to ensure take‑up and avoid staff resistance to allow the positive effects to materialise. The SEA should develop systematic approaches to involve end-users throughout the development processes to increase value‑added of the new digital solutions. The end-users should be involved already in the stage of developing the concepts and not only in the testing stage when the main functionalities have been already developed, only to identify bugs (OECD, 2022[8]). The involvement of the SEA staff as one group of end-users should be particularly prioritised and most feasible to systematically involve, while the ways to involve other user groups (employers, jobseekers, partners) in the development of the SEA online services and incorporate their views and feedback should be established as well.
The two‑way communication between the development side (the business unit in the SEA that “owns” the digital solution and the IT unit) and the users (SEA staff, jobseekers, employers, partners) need to continue even once the solution is deployed. Feedback from users supports continuous improvement and fine‑tuning of the digital solutions. Communication to users involving guidelines and user support channels further ensures an appropriate use and take‑up of the digital tool by decreasing the hurdles and resistance to use it and helping to raise awareness and understanding how it functions and how to incorporate it seamlessly in the work processes.
The digitalisation strategy should also envisage a monitoring and evaluation framework for digital tools in the SEA to understand the performance of its digital solutions, helping to fine‑tune and adjust functionalities and user experience, as well as find alternative solutions for those tools that do not perform and cannot be easily fixed. In addition to monitoring qualitative feedback from users and quantitative indicators like take‑up rates and user numbers, it is important to evaluate the effects of the digital tools on the SEA outcomes and the cost-effectiveness of these tools. Being able to show how the digital solutions help jobseekers, people at risk of job loss and employers, as well as the benefits that investments in digitalisation incur would enable the SEA to better communicate its solutions to the end-users, and make the business case for further sustainable funding in digitalisation. For example, an evaluation of a digital tool for job counsellors in Spain showed that this tool enabled counsellors to make better recommendations on job search and jobseekers to find better jobs quicker, and thus enabled the Spanish PES to encourage the use of this tool among job counsellors (OECD, 2022[8]).
6.3.3. Considering the development frameworks, processes and methodologies in the digitalisation strategy would ensure its comprehensiveness
The SEA’s digital strategy should also address the mechanisms to manage the risks associated with digital tools, such as issues concerning ethics, trustworthiness, accountability, transparency, fairness, data protection and system security. These risks are likely to amplify if AI algorithms would be harnessed to enhance digital tools (Brioscu et al., Forthcoming[5]), but can be present even in simpler digital solutions. For example, PES that are more advanced in digitalisation and adopting AI, such as Belgium (Flanders), France and the Netherlands (Pôle Emploi, 2022[9]; Scheerlinck, 2020[10]; Scheerlinck, 2023[11]), have often set up dedicated frameworks and committees to ensure new digital developments to not pose undesired risks, particularly in terms of ethics and biases.
Developing a digitalisation strategy provides an opportunity for the SEA to comprehensively reconsider its IT development approaches and processes more generally, including in terms of the development methodology. While modernising the development methodology for the tools and systems already in production might not be feasible or sensible, modern agile development methodologies could be considered for future developments. In more particular, Section 6.6.3 proposes adopting DevSecOps development methodology in the SEA.
Furthermore, the SEA needs to ensure sufficient capacity of its development partners. The SEA needs to analyse options for changing its procurement approaches to receive competitive proposals for the IT projects and ensuring development partner’s commitment and available capacity in the contracts. Also more modular systems facilitate working with several development partners (see Section 6.4.1). The digital strategy could include the pathway to achieve sufficient external capacity for developments to ensure that the milestones of the SEA digital transformation could be achieved.
The next sections discuss the different aspects that could be addressed to modernise and strengthen the SEA’s digital backbone, and which could feed into the SEA’s new digitalisation strategy to be developed in co‑operation with the OECD and DG REFORM within the context of the same project as the current report. The strategic goals and milestones set in the digitalisation strategy should be accompanied by necessary actions, responsibilities and a monitoring framework either in the strategy itself or an accompanying action plan to ensure the implementation of the digitalisation strategy.
6.4. The operational IT system
6.4.1. A more modular architecture of the main IT system would facilitate development processes
The main operational IT system in the SEA, BURVIS, is implemented as a monolith. A monolith is more likely to break down (have bugs) during upgrading and deploying new features than a more modular system with well-defined interactions among its modules. Thus, the current solution is somewhat fragile, which also causes hesitancy to use automatic deployment processes.
In case of a modular architecture, deploying one module (only the one undergoing the change) at a time would reduce risks associated with automatic deployment. It would also speed up the development process and adding new features, as new releases via automatic deployment could take place more frequently.
Having a more modular architecture together with automatic testing and deployment would also facilitate working with more than one development company. This would mitigate the capacity issues that can arise when working with a single provider as experienced by the SEA over the years when co‑operating with the current development partner, UNISO.
A modular architecture would speed up development, as well as enable experimental development, e.g. buying specific features and even complete systems and integrating these with the existing solution using well-defined internal and external Application Programming Interfaces (APIs).
Nevertheless, changing the architecture of an existing monolith to separate modules requires considerable resources and time. Hence, developing new digital solutions that could be more easily implemented as new modules, is a more clear-cut way forward for the SEA. This approach has been also initiated with the development of the new tool to profile jobseeker skills in the SEA (see more in Section 6.9).
6.4.2. Introducing network-level segmentation would improve system security
The production environment and the two testing environments in the SEA are not fully separated as these share the same non-segmented network. A network allows traffic flow between nodes (physical servers, virtual machines, applications, database servers etc.). Firewalls and other restrictive technologies apply usually on the boundaries of a network, but not within a network. While firewalls can be also inside networks, this is in practice rather uncommon. Thus, a network is a prime target for hacking. If a network is compromised (broken into), it often allows for “lateral movement”, i.e. gaining access to other nodes within that network (Figure 6.1). For example, hacking into one server within a network may provide easy access to other servers in the same network.
The SEA should separate the three environments using network level segmentation. Segmentation would divide a computer network into smaller parts and thus improve network performance and security. Establishing several networks or subnets is not any more expensive or difficult like in the past when networks were a physical entity of cables and devices, as today networks are mostly virtual. At a minimum, all production resources should be isolated from anything else, such as development and testing resources. Separating development and testing networks from the production network is particularly important as these networks do not contain real data and need to be accessed by more developers, and hence have often more relaxed access policies. Separating development and testing networks from the production network thus disables the lateral movement path from development to production and reduces risks.
Figure 6.1. A single network is more vulnerable against malicious actors
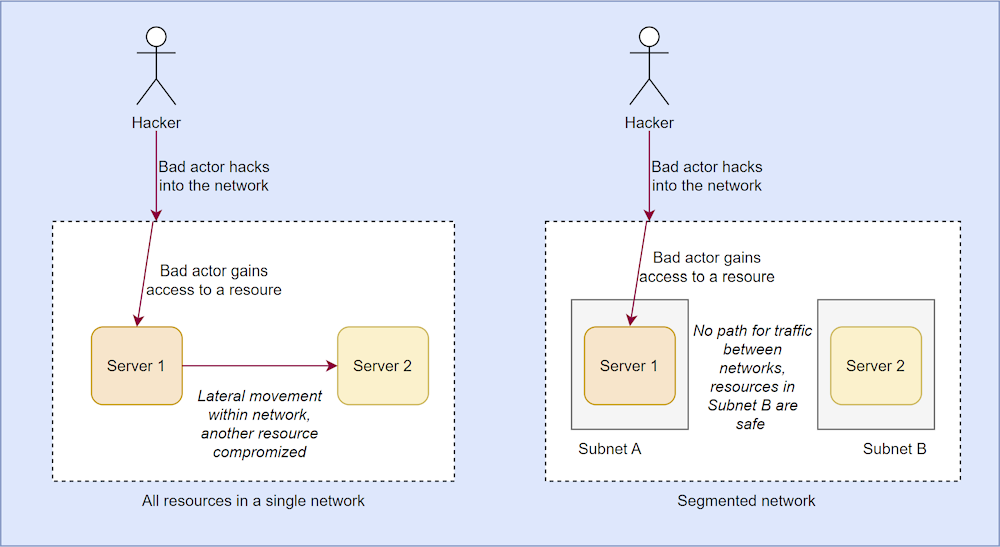
Source: Author’s work.
6.4.3. Monitoring system performance and security should take place near real-time
System monitoring in the SEA takes place by going through logs after an incident has happened. In such an approach, issues in the system are hardly detected (near) real-time, which can be particularly problematic regarding system security related incidences.
The SEA should consider implementing a dedicated monitoring tool that offers near real-time information on the state and behaviour of the system. Such tools are typically presenting the key monitoring indicators on a dashboard with a possibility to drill down into details on specific errors or performance incidences (such as a sudden increase in response times of the system). Several products are available on the market for such monitoring tools, such as Splunk and Azure Application Insights (Figure 6.2). Adopting a tool offered by the market would require some work to implement it in the specific system, although basic monitoring capabilities can be achieved already with only small adjustments of the tool (adding an instrumentation key and some provided scripts).
Figure 6.2. A dedicated monitoring tool offers near real-time information on the state and behaviour of the system
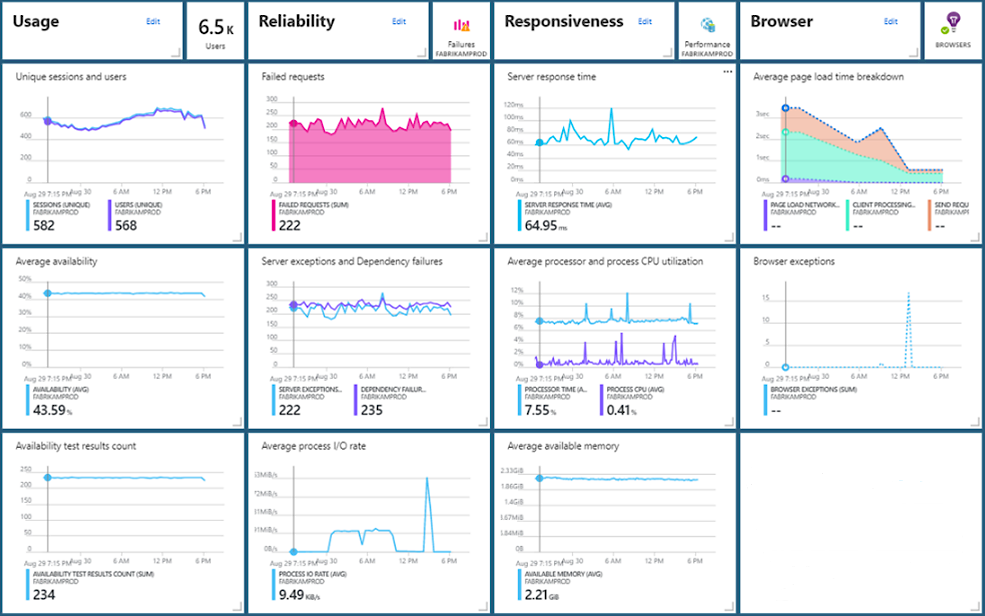
Source: Author’s work.
6.4.4. The technology stack needs updating
The technology stack of the SEA includes some technologies that are somewhat dated, as for example Java 8 is used for the interface to external registers via the VRAA access point and PostgreSQL 13 for database technology. Using old versions of any software, including Java, exposes the system to security risks because not all security fixes are provided for older versions. Although Java 8 is currently still supported, it needs to be upgraded by the SEA’s development partner at least to Java 11 soon, before the support ends. Although such an upgrade would require some investment, pushing it to the future will only make it more expensive altogether.
The SEA IT system uses many external libraries (collections of resources in software development, such as configuration data, documentation, help data, message templates, pre‑written codes, value or type specifications etc.), yet the SEA does not have a complete list of libraries used for BURVIS development. It is very important to manage the life cycle of libraries, including making sure that no up-to-date versions are used. Routinely upgrading to the newest versions as soon as these become available does not require investments per se, but just more systematic processes.
Upgrading any part of the digital infrastructure, including software platforms and libraries requires comprehensive testing of the system. To facilitate such comprehensive testing, the SEA needs automatic testing systems with sets of pre‑defined cases of high testing coverage and sufficient test environments, because any change in codes can introduce bugs.
6.4.5. Deployment should be automatic
The SEA deploys software manually (hands-on processes and individual control at each step, instead of automatic tools and scripts), which has several downsides. Manual deployment is slower, prevents frequent releases, and is more error prone than automatic processes contrary to common belief. Automatic systems perform processes as they have been instructed to do, whereas humans can get tired and make errors when performing repetitive tasks.
Automatic deployment also facilitates rolling back in case a deployed version does not work as intended, because automatic tools create a procedure for undoing the changes. As the SEA uses VMware for the virtualisation of the servers, also VMware images could be used for rollback, although these are less granular and thus the process can be less effective.
Furthermore, automatic deployment would remove the need for developers to access the SEA production environment and thus increase system and data security. In addition, the fewer people can access the production systems, the less likely are errors due to manual changes in the production systems.
The automatic deployment should be also used for database changes, e.g. when creating new or adjusting existing tables. Automatic deployment would decrease the issues with database changes by removing the possibility for human error in the process, such as typos in table/field/index or other names.
Automatic and comprehensive system-level testing is extremely important for automatic deployment in addition to unit-level testing. A new version should be deployed first in the test environment, thoroughly tested, and deployed in the production environment only once all detected errors are fixed.
6.4.6. A cloud solution could be considered in the future
The current solution of having external data centres to host the SEA servers and the SEA itself maintaining the virtual layer has been sufficient for the SEA’s current needs. In case in the future more scalability would become desirable (e.g. the number of simultaneous users increases and fluctuates), a public cloud-based solution could be considered instead.
The cloud can offer many benefits
A migration to the cloud can offer several advantages, for example advanced system security. The security level of the major public clouds is on a very high level, much higher than most organisations can afford on their own. Public clouds have typically 24/7 staffed locations with experts monitoring the state of the environment constantly. The public cloud data centres are generally built with several layers of redundancy, i.e. duplicated (or even more than that) power sources, network connections and computing and storage hardware.
Although the security of public clouds is often questioned, it is important to differentiate between the security of the cloud solution itself and the customer workloads (computing resources and tasks to run an application or service) within the cloud. The cloud itself is the responsibility of the cloud provider, who need to take care of securing their environments (and all the major cloud providers have so far succeeded in that). Ensuring security of the customer workloads within the cloud are the customer’s (in this case the SEA’s) responsibility. While the cloud itself does not itself ensure the security of customer workloads, it does offer numerous additional possibilities to secure the applications and services.
A cloud solution can offer cost savings, particularly considering possible indirect costs. For example, a smaller application like BURVIS may not be cheaper to host in the cloud per se. However, adding the time it takes to build and maintain servers in physical centres, the total costs of using a cloud solution might be lower. In addition, cost savings are more common for workloads that have highly fluctuating usage, for example mobile applications. In such cases, hosting the application requires very high capacity during the peak loads. Contrary to having a solution of a limited number of physical servers, capacity can be easily downscaled and even adjusted automatically in the cloud. Nevertheless, such cost savings are likely only low in the case of BURVIS, as the application profile is rather stable and scaling for cost savings not as relevant.
A cloud solution can offer also more possibilities for new technologies, as these are currently developed largely in the cloud. For example, the AI technology is almost exclusively available via cloud computing. Also, many new security technologies have emerged first in the public cloud. The SEA should consider a cloud-based solution in case desiring to implement such new technologies or functionalities in the future.
Understanding the implications of moving to the cloud
Moving to the cloud might seem like losing control over the hosting environment as becoming not able to fix issues directly and having to wait for the cloud provider to address these issues. However, these concerns are not generally valid as the cloud providers have staff available for 24/7 and are much more likely to be able to fix any problems than most organisations by themselves even during nights and weekends. As such, instead of losing control, the sift to the cloud could be rather seen as outsourcing a better service.
It is important to understand that the risk landscape changes when moving to the cloud, as risk management becomes shared between the customer (SEA) and the cloud provider. The cloud provider would provide the framework and tooling and secure the hosting environment, but the SEA would be still fully in charge of configuring and handling its applications and data. Furthermore, the cloud can be a major target for attackers, since these host a high volume of assets. While the IT systems of a smaller organisations like the SEA might not draw hackers’ attention, being a part of larger system (the cloud) can be more likely under constant hacking and other malicious activities. While the cloud provider aims to take care of the associated risks, the SEA would need to consider the implications of this context and be prepared to adjust its approaches.
Moving to the cloud requires new skills and knowledge. While many technologies are easily transferrable to the cloud, some technologies and processes need to be adjusted, and some additional technologies become available. For example, PaaS services (Platform-as-a-Service, sometimes called “serverless computing”) in the cloud means a rather different way of accessing servers or networks in an organisation’s our own environments.
6.4.7. The SEA should deploy a modern API management tool
Some of the interfaces for data exchange in the SEA IT system enable direct access to the BURVIS database. However, such direct database access also reveals the database structure of the BURVIS system to the external parties and creates direct coupling, thus making the external system dependent on the internal details of the BURVIS database. These features make the maintenance of BURVIS harder than necessary as changes to the database structure would affect the access of the external parties.
Deploying a modern API management tool would enable the SEA to create an abstraction layer between BURVIS and external systems (Figure 6.3). Such a tool would also provide additional useful functionalities, such as access control (to manage which parties can access), throttling (managing how many users can access at a given time, preventing attacks), key management (creating, sharing, storing and exchanging access keys), handling errors and monitoring data exchange processes. These functionalities would contribute to a better management of data security risks. The SEA should analyse the feasibility of deploying a modern API management tool, such as Apigee, MuleSoft, Azure API Management or Dell Boomi, which are reasonably priced and easy to deploy, as well as often cloud-based.
Figure 6.3. An API management tool should control access to BURVIS
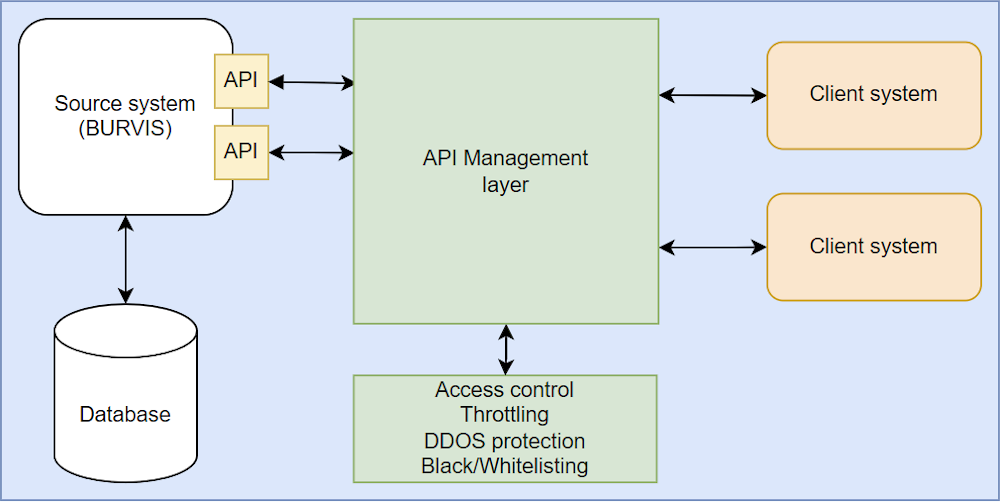
Note: API – Application Programming Interface. DDOS – a distributed denial-of-service attack.
Source: Author’s work.
6.5. Data management
Although the digital infrastructure of the SEA enables it to collect rich data to support its business processes, data management processes and mechanisms are currently quite limited. Although the SEA does not need a complete overhaul of data management, many smaller steps should be taken to improve data management and make the associated processes more efficient.
6.5.1. The SEA should improve the system to analyse and improve data quality
Data quality affects all aspects of the SEA operations and digital systems, from using data to deliver services to jobseekers and employers to producing statistics and analysis and sharing data with external partners for their purposes. Yet, BURVIS application has only limited data quality checks, and data quality issues are mostly managed manually.
The SEA needs to define an official process to manage data quality issues to have clarity on how to address any identified data quality concerns. Currently, the SEA has a semi‑informal system to address data quality issues as staff in the statistics unit aim to identify data issues using personalised data in case they have capacity for this task, and counsellors in local offices make the necessary changes to the data, which are then logged in the SEA’s audit database. As the SEA deals with personally identifiable information, only staff members with the relevant authorisation should be allowed to edit the data, including in case of data quality issues. In addition, any such edit needs to be logged for a proper audit trail separately from application logs that might be stored for a shorter duration. A systematic process to address data quality issues also facilitates improving the digital infrastructure more generally as it creates a continuous feedback loop between users and developers.
A prerequisite for a system of data quality management is an agreed data quality standard. The SEA needs to define target levels of and acceptable variation in data quality. Aiming for 100% in data quality throughout the system can get very costly, and hence it is necessary to identify which parts of the data are the most critical and need the highest quality. The priorities for data quality can differ between operational and analytical needs and thus the different needs should be discussed and agreed on involving all relevant data users.
All sets of data in the SEA’s databases need to have clearly defined ownerships to facilitate setting the data quality standards, define when data can be considered “correct”, and have clarity on who is responsible and has the final say with regards to decisions on these data more generally. The SEA has not currently defined clear ownerships, apart from all data being the property of the SEA. However, such shared responsibility is not recommended, as it tends to lead to situations where nobody might take responsibility to handle some issues assuming that someone else is handling these.
The SEA needs to implement system-wide controls over input data that enable a singular point of validation of each data element. The SEA’s system is designed and built over a longer period and receives data from numerous sources (user input, APIs, integrations with other systems). In such a case, it is likely that similar data (e.g. jobseekers’ addresses) are received through different mechanisms, and all these different mechanisms check for data validity separately. This can lead to problems, especially when the data validity rules change and the changes are not implemented in all sources from which data are received, leading to potentially erroneous data. Therefore, the system should entail a singular point of validating each data element, which can be done by implementing internal APIs for saving data (as well as provide data to other parties from the system). In such set-up, each route for incoming data calls the same internal API which validates the data always the same way regardless of the data source. This validation should also be used in the user interface layer of BURVIS to help job counsellors input data correctly. Implementing such functionality can be done in steps, one internal API at a time and then moving the input routes to use that API.
The SEA could implement an automated system to analyse data quality, enabling to monitor the data in the system and report any issues detected, as well as potentially automatically fix some types of issues. Such automation would help avoid human errors, as well as additional manual work. Automatically fixing data quality issues could be feasible in case duplicate entries and incorrect data formats. For other types of data quality issues, implementing alerts to indicate errors and suspicious data might be more beneficial. An automatic alerting system can have a much broader coverage in terms of potential issues than automatic corrections as it is not a destructive function.
6.5.2. Data retention periods need to be analysed
The SEA logs currently all clicks, keystrokes and transactions in its digital systems in detail and has not so far deleted or archived any historic log data. The current system creates very detailed log by logging clicks and keystrokes in addition to transactions. This practice yields a very large and continuously growing log database, which is becoming problematic.
The SEA needs to analyse its logging practices and define retention periods for its different datasets. For example, application logs might not provide much value after a few months, while audit trails might need to be kept even long after the application itself has been decommissioned. Furthermore, the SEA needs to analyse which log data need to contain personal information, while all other data should be pseudonymised.
The General Data Protection Regulation (GDPR) is one of the key instruments to help assess the data management practices, including regarding retention periods of log and other data. For example, GDPR regulates retention periods of personally identifiable information after the customer relation has ended. Hence, if a jobseeker is no longer using the SEA services, they have the right to have their data deleted from the SEA’ databases as their data is no longer required by the system. Thus, the SEA should have a mechanism to remove the user information in these cases from its databases completely, which should strictly speaking also apply to log data.
In addition, although a log can be used as a debugging tool, it should not be the primary tool for the debugging purpose. More suitable approaches for debugging include for example application monitoring described in Section 6.4.3.
6.6. System security
A system could be deemed secure only by comprehensively and continuously monitoring and testing the system and all its components. Maintaining security requires a proper design and implementation of the technical components and processes, but also continuous effort to face the existing and emerging security concerns.
6.6.1. Access control could be tightened
The SEA manages access rights centrally using Active Directory, adding new staff members to appropriate groups of access rights and thus ensuring a secure implementation of access control. However, also external developers are granted access to the SEA production environment in addition to staff. In total too many people have access to the production environment, also because the deployment process is still manual.
The process of granting access to IT systems and allocating persons to access groups should be carefully designed, executed with discipline, and generate audit trail. Access rights should be never given ad hoc to the production systems or any other systems that contain production data. Also, developers should have no access to the production environments, but only to the development, test, and quality assurance environments. Moving to a fully automated deployment process would make it possible to reduce or even eliminate the developer access to the production environment, as their tasks could be automated.
Only very few administrators in the SEA should have access to the production environment, and their actions should be rigorously logged. Besides administrators, also the actions of all other people with privileged access (users with higher rights than regular users) should be logged, and the logs audited regularly to reduce insider risk and to ensure that privileged access rights are being used properly.
6.6.2. Continuous penetration testing is needed to face constantly evolving vulnerabilities
Any system’s security can only be proven by security testing, especially penetration testing (authorised simulated attacks performed on a computer system to evaluate its security, also known as pentests or ethical hacking).1 The SEA performs penetration testing once a year, which is not frequently enough as new vulnerabilities emerge at least monthly, if not weekly. Hackers – malicious actors – do their own research and development work, and continuously find and invent new methods to break (into) systems. From testing a system today against all knows vulnerabilities, we can only conclude that the system is secure for today, while we have no way of knowing what new vulnerabilities emerge next week or month.
Thus, the SEA needs to test its systems against the new discovered vulnerabilities more frequently, potentially using automated testing. Automated penetration testing is available as a service from many companies, and the SEA could consider subscribing to such a service.
Continuous testing results should be logged and audited to make sure that testing is a part of a continuous improvement process and the issues found are fixed. Auditing should take place after each round of testing if feasible.
6.6.3. DevSecOps to integrate security seamlessly into the development cycle
Some of the mistakes that humans make can be easy for computers to detect. For example, a piece of code or a library can be insecure due to human errors, making the system vulnerable for malicious actors. Automatic code scanning can be used to detect known security problems and vulnerabilities in codes used in the systems, as well as already before these are deployed.
One possible approach to implement automatic code scanning already from the start of developing process, is to adopt DevSecOps (short for development, security, operations) development methodology. DevSecOps is an increasingly popular practice in application security as it introduces security already from the start in the software development cycle, i.e. it automates the integration of security into the initial design (first codes) through to testing and deployment, as well as into monitoring a published product. Automation enabled by DevSecOps ensures that security issues are not forgotten or skipped in any of the steps of development and that these issues are addressed early on, when these are easier and less expensive to fix. DevSecOps could be ideally used in combination with regular vulnerability scanning described in Subsection 6.6.2.
Automation in the context of DevSecOps means adding scanning functionality into CI/CD pipelines (Continuous Integration / Continuous Deployment – a process by which software is automatically processed from source code format to a testable application), and even in source control2 pull/merge process (the developer can create a pull request when finishing a piece of work, triggering automated system(s) to merge the new code into the existing code base, potentially in turn triggering the CI/CD pipeline). Whenever code is pulled to a repository, it is scanned by an automatic tool. Whenever a build is created (a compilation of software source code from human-readable format to machine‑readable), in test, development or production environment, it is automatically scanned. By placing release gates (a decision to continue the process or not based on set criteria) that depend on the results of the scanning, it can be ensured that no vulnerabilities make their way into production.3
6.6.4. Comprehensive security processes need to support the technical components of digital systems
Although security has technical components, it revolves mainly around processes, such as risk management, vulnerability monitoring, access monitoring, disaster recovery, logging and informing. These processes aim to establish an understanding of the current level of security, compare it to the required level of security, and identify and manage any exceptions correctly.
To manage risks, the SEA needs to document and assess the relevant risks periodically, as well as compare the risk assessments over time to see if some risks are becoming more prominent and need graver actions. Risk management means understanding the risks and their potential impact. No system is without risks and each of the risks has a cost to mitigate it. A completely risk-free environment is almost always too costly to achieve. Thus, some risks always remain, which need to be understood and managed. Typical examples are insider risks – it is extremely difficult to design a system that does not have administrators with privileged access, and with such access it is easy to steal or destroy data. This risk is often accepted, as building bullet-proof controls to mitigate it can be very expensive.
Vulnerability monitoring in the SEA needs to be a continuous process as vulnerabilities change over time. For example, a third-party library could be deemed safe today, but next week a new type of vulnerability is found in it. Doing vulnerability assessment only once when releasing the software, this change in the status of the library would not be recorded.
Also access monitoring and auditing access rights needs to be a continuous process. Typical mistakes in managing access are to forget to close accounts when people leave an organisation or close access of external partners or developers after their access is no longer needed. Only by having a strict and continuous audit across all accounts it is possible to manage associated risks well.
Disaster recovery process is part of security, as well as operative processes. Disasters (such as loss of data, loss of system functionality, denial of service etc.) can be the result of data breaches, in which case the recovery process could be initiated by the security team, instead of the operative team. The process needs to be always the same and all parties are working together to prevent further damage. It is common for disaster recovery processes to be too vague and not controlled enough, which can mean that after recovery unexpected amounts of data might be lost, or the data that did get recovered were not required. The disaster recovery process should be detailed and explicit, so that it can be followed in a very stressful situation and by people who might not have previous experience in disaster recovery.
Informing relevant officials and administrations, as well as customers of breaches and suspected breaches is an important part of security communication. While poor information can send a negative signal, a clear message together with decisive actions will send a signal that the situation is under control and being handled. Furthermore, all suspected breaches should be logged thoroughly and separately from the operational system, so that these issues and their handling can be examined later to feed into continuous learning and improvement process.
6.7. The digital backbone for data analytics
The data analytics solution in the SEA relies on a copy of the operational database of BURVIS, which does not have the flexibility to better adjust data for the needs of analytics or enrich the database with additional data sources. The statistics team in the SEA is able to use only limited and inflexible tools to query and process data, making the production of statistics and analysis mostly a manual process that exhibits various data protection concerns.
6.7.1. Introducing a data warehouse solution could greatly benefit data analytics
A data warehouse is a central repository containing structured and semi-structured data from one or more data sources to facilitate data analytics in an organisation, particularly supporting and automating the production of regular statistics, reports and data analysis. PES systems in 74% of OECD and EU countries have set up a data warehouse to facilitate data analytics (OECD questionnaire on digitalisation in Public Employment Services to support the provision of active labour market policies launched in March 2023; the share of countries calculated based on those countries that responded to the question). Most of the data warehouses in PES include data from different internal registers on jobseekers, ALMPs and benefits, while some of these receive data from external registers (above all employment, social security, revenue, benefit, disability and immigration data) and even national statistics offices (Estonia, Germany, Poland, Spain).
For example, Poland has set up the Central Analytical and Reporting System (Centralny System Analityczno-Raportowy, CeSAR) that includes data from the internal registers of the PES system (data on registered unemployed people and jobseekers, services and measures provided to individuals and organisations, job offers, employment agencies and training institutions, electronic services of PES, etc.). In addition, CeSAR includes unemployment and demographic data from the Central Statistical Office, as well as data from external administrative registers on disabilities, social assistance, family benefits, alimonies, family support, foster care and childcare services.
Furthermore, 13% of PES (Australia, Belgium (Flanders and Wallonia), Korea and Sweden) have set up a data lake in addition to or instead of a data warehouse. Data lakes are used for storage of various data that are not in standardised format and may contain data errors, i.e. the data are not prepared to fit data analytics like in data warehouses, as data do not have to go through the data processing stage beforehand. A data lake can be a separate environment for data discovery where the users can test different new queries, analyse new datasets etc. Nevertheless, the priority for the SEA would be to set up a data warehouse to automate and improve the regular data analytics, while the needs for an additional data lake solution could be analysed in the future.
A separate data warehouse would maintain the benefits to the operational system
Considering the volume of BURVIS database, SEA should adopt a separate data warehouse for analytical purposes. Adopting a data warehouse solution facilitates data analytics and producing statistics, as well as maintains the same benefits to the operational system as the current solution of having a back-up copy for data analytics.
Similarly to having a separate copy of the BURVIS database, a data warehouse would technically de‑couple the operational and analytical databases. This means that neither of the databases can affect the performance of the other due to service interruptions or update installations on systems or underlying hosting layer. When the analytical and operational databases are separated, both can be updated and maintained without affecting the other one, giving much more freedom for the required maintenance tasks. In addition, a complete de‑coupling ensures that the performance of the operational system would not be affected by an abnormally high load on analytics database, for example due to end-of-month or end-of-year reporting needs (and vice versa). This is currently achieved at the SEA by having the analytical database to be a copy of the operational database on a separate server, and these benefits would remain when adopting a data warehouse solution.
Even when the SEA would build a data warehouse, the current BURVIS database copy could have the purpose of testing the database backup. In case loading the copy of the BURVIS production database fails, it would signal that disaster recovery would also be endangered. Such testing is often overlooked in many IT systems, as backups are simply taken and archived but not thoroughly tested on a regular basis to make sure these processes actually work. In addition, the current BURVIS database copy can be used to cater for some simple reporting needs within the BURVIS application also in the future, and to cater for reporting needs while a data warehouse is being developed.
A data warehouse would enable to better meet the data protection needs
As analysts and statisticians should generally not process personally identifiable data. A data warehouse could potentially contain only anonymised or pseudonymised data, and thus help the SEA better comply with data protection regulation, such as the GDPR, when processing data.
Anonymisation / pseudonymisation for data analytics could take please in the ETL/ELT phase (Extract-Transform-Load / Extract-Load-Transform). ETL is the more common approach to move data to a data warehouse and, in this case, data are extracted from the source (e.g. an operational database), then transformed while being moved, i.e. before loading the data to the data warehouse. ELT is a newer approach, in which data are extracted from the source system, loaded to the data warehouse, and transformed only later. A data transformation could be automated after loading in ELT (in a separate data staging area in the data warehouse), or even only when data are queried. ELT can be more efficient than ETL, particularly for larger data volumes, but can be more complex to set up and maintain.
A data warehouse enables to implement a data model meeting the data analytics needs
With a fully separate data warehouse and ETL/ELT process, data models in the operational database and in the data warehouse can be better designed to serve specific purposes. When the analytical needs are being catered to using the data directly from the operational database, the data model is not likely fully suitable for analytical needs and the analytics side has to accept some compromises on the data model it works on.
The data structure and content in the data warehouse does not need to follow exactly the same administrative logic as in the operational database. For example, the operational database might need to include information on the date of application from the jobseeker to be registered as unemployed, the date of the decision by the SEA regarding the application, the content of the decision (e.g. to grant the unemployment status or not), as well as all other administrative dates and actions related to this application and decision (e.g. cancellations, revokes etc). On the analytics side, potentially only the valid date of the start of registered unemployment regarding this application might be relevant. Similarly, other data relevant for administrative processes might not be relevant for analytics, or can be simplified or aggregated. Often, some of the more commonly needed variables are pre‑calculated in the data warehouse to enable shorter query times, while the same variables are not relevant for the operational needs and should not be stored in the operational database.
In addition, other data transformation processes might be relevant to prepare operational data fit for data analytics in the data warehouse, such as:
Quality control;
Classifying (in case data are not classified correctly);
Coding (in case data stored in a long text format);
Cleaning;
Processing duplicates;
Transforming into suitable formats for analytical and statistical purposes;
Imputing missing values;
Linking the data from different sources (see next Subsection).
A data warehouse enables linking data from different sources
Contrary to using an operational database or its copy for data analytics, a data warehouse solution enables bringing in additional data sources, including additional metadata and pre‑calculated aggregate data. Thus, the data readily available for statistics and analysis are richer, extending the scope of knowledge that can be generated using the data warehouse, and facilitating the production of statistics using (linked) data.
To make additional data available in the data warehouse, separate ETL/ELT processes can be set up for this purpose. The modern ETL/ELT tools enable extracting data from different types of data sources, such as data stored in (Excel) files, (operational) databases or the web. Also, APIs can be implemented to obtain external data, for example metadata directly for the data warehouse. Furthermore, additional datasets can be either linked and stored together with the main (BURVIS) data or kept in a separate dataset within the data warehouse, and only linked in a background process when a relevant query is launched. These design choices can have different outcomes in terms of ease of implementation, speed, efficiency and compliance with data protection legislation, and should be thus decided case by case.
A data warehouse offers a channel to share data with external partners
A central data warehouse can contain many different data marts to serve specific purposes, typically focused on a particular subject and thus containing only limited essential data. A data mart could include only statistical information on the subject if this would serve the purpose. Thus, it is possible to share specific data marts to make some data securely available to specific user groups, partners or other external stakeholders, without a possibility of leaking data not meant to be shared. Publishing specific data marts is a secure approach, because even in case a data mart is breached it would not contain any other information, and thus the scope of a potential breach is limited.
The solution to share data with third parties should be designed together with the overall design of the data warehouse. Designing the data sharing approach early on helps avoid the solution evolving over time ad hoc to a complex and chaotic set of methods that will be difficult to maintain.
When sharing data marts with third parties, an API approach is preferable over direct access to the database as it allows for a better access control. APIs including web service technology is a common and standardised way to share data, which has also functionalities to transform data, as well as to share metadata. Using web services, the system owners can define and implement what data to share and how fast these can be accessed. Web services can use alternative secure authentication methods and support logging functionality, and thus allow the data owner to monitor the users’ activity even by each individual user (which users use which data, etc.). However, not all users and third parties will be able to use an API, and hence database access or even secure file uploads may be needed in some cases. Nevertheless, these options should be avoided whenever possible. In addition to lower access control, secure file sharing lacks possibilities to monitor the use of the files and data.
The architecture of a data warehouse can be developed further as new needs arise
The architecture of a data warehouse could be arranged in many different ways. Thus, the design of the architecture should take into account all relevant needs, while being as simple as feasible (Figure 6.4). The architecture of a data warehouse can be usually easily expanded when new needs arise. For example, new metadata storage or new data marts can be easily added to the architecture, provided the data warehouse is designed with as little constraints as possible.
Figure 6.4. A potential architecture of the first version of a data warehouse in the SEA
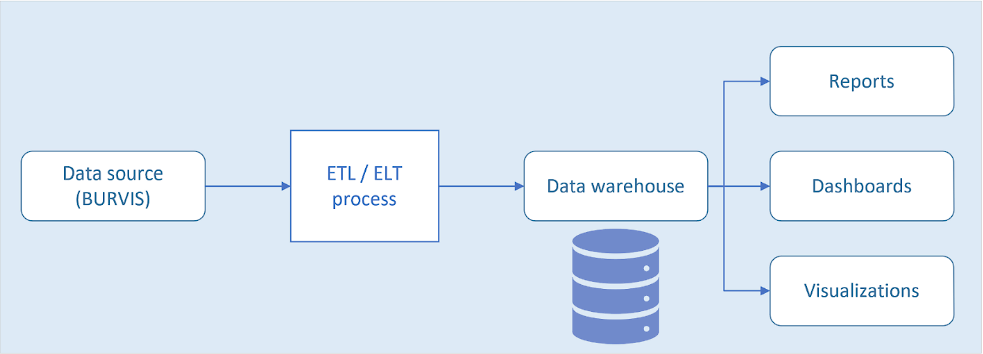
Note: ETL/ELT phase – Extract-Transform-Load / Extract-Load-Transform.
Source: Author’s work.
The simplest architecture of a data warehouse could be simply a separate database that is created through an ETL/ELT process. Such an architecture would already allow the data to be shaped to be more suitable for analytical and statistics purposes, and it would not get overwritten by a database copy.
The simple architecture of a data warehouse can be easily extended to cater for more complex needs, such as to contain multiple sources of data, additional metadata and aggregate data, and even data marts, without breaking the fundamental architectural approach (Figure 6.5).
Figure 6.5. The SEA data warehouse could be developed to cover different data sources in the future
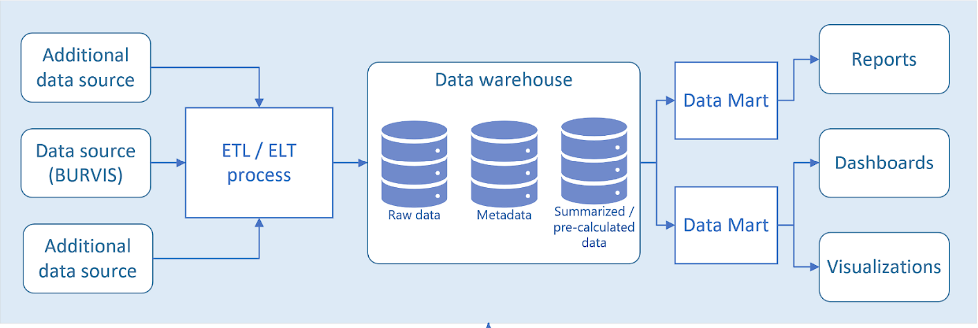
Note: ETL/ELT phase – Extract-Transform-Load / Extract-Load-Transform.
Source: Author’s work.
The SEA could investigate whether it could be sensible and feasible to build in the public cloud. The cloud offers scalability in a way that self-hosted environments cannot easily do. However, the public cloud solution can be more expensive if the usage of the data warehouse is constant.
6.7.2. Automating data analytics using Business Intelligence tools
Business Intelligence (BI) tools facilitate data analytics and generating knowledge, supporting thus the production of statistics and reports, performance management, monitoring and evaluation frameworks, knowledge‑based decision making for operative and strategic management and any other data analytics-driven function in an organisation. BI tools enable to quickly analyse and visualise large volumes of structured and sometimes unstructured data for a quantitatively assess performance and identify trends.
PES systems in 76% of OECD and EU countries have adopted BI tools to produce statistics, analyses and support data analytics more generally (OECD questionnaire on digitalisation in Public Employment Services to support the provision of active labour market policies launched in March 2023; the share of countries calculated based on those countries that responded to the question). Many different BI tools available on the market have been adopted by PES across OECD and EU countries, such as Tableau, Qlik Sense, Microsoft Power BI, Oracle Business Intelligence, SAP BusinessObjects, IBM Cognos Analytics and MicroStrategy. Nevertheless, some of the PES have developed their data analytics already some time ago and the solutions are outdated for current needs, some have not yet implemented visualisation or otherwise not harnessed the full potential that BI solutions can offer. Thus, continuous effort to improve data analytics systems is on-going in many PES.
Business Intelligence tools can support any data analytics-driven function in the SEA
BI tools would enable the SEA statistics department to automate the production and visualisation of all regular statistics, and implement ad hoc queries flexibly. These tools enable setting up pre‑defined queries that can produce aggregate or individual level results, with the help of a developer. In addition, the SEA statisticians can define new queries themselves flexibly based on the variables made available via the BI tool without needing to use SQL.
In addition, other units in the SEA like the management and regional offices could have key information automatically available for them within a BI tool in an easily understandable and flexible format that can be used without having data analytics skills, i.e. dashboards. Dashboards offer an interactive visual representation of key data that can be tailored to the user (Figure 6.6). A user of the BI tool can access several dashboards to cover various needs, potentially with a “drill down” functionality to gain further insights on specific indicator by clicking on it. Via visualisation, dashboards enable to quickly identify trends, rankings, outliers or other anomalies in data and take action.
Figure 6.6. A dashboard in the Business Intelligence tool can cover key indicators relevant for the specific user
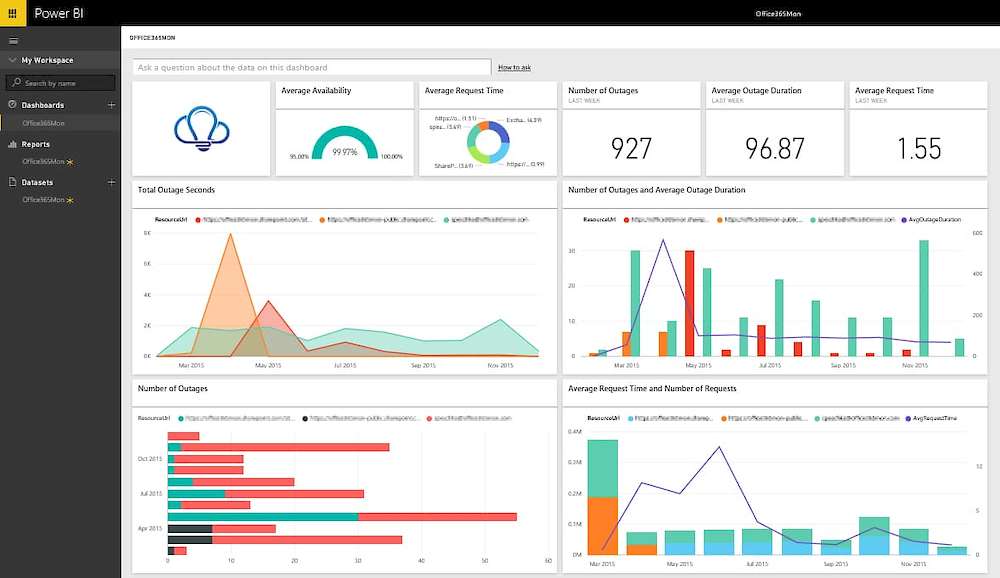
Source: Author’s work.
BI tools also support producing automatic reports that can combine tables, figures and text into a single document, for example for monthly or annual reporting. Contrary to dashboards, such reports are generally not interactive to enable sharing and printing these externally from the BI tool environment.
Adopting a modern BI technology offers many benefits
BI technology has developed significantly over the past years to enable automatising any routine data processing. Modern BI tools have many advantages over any older tools serving similar purposes:
1. Modern BI tools allow using data from different sources simultaneously, including the data warehouse, databases of classifications and code lists, as well as directly from the operational database.
2. The newer solutions allow automated visualisation of data, including on geographical maps, thus allowing spatial data analyses. In most cases, there is no need to use Geographic Information System (GIS) servers to visualise such maps, which makes these solutions cost-effective and reduces the number of tools needed to produce the outcomes.
3. The newer BI tools have in-memory calculation capabilities making them much more efficient (the user gets the query results much faster). This minimises the need to optimise SQL queries, thus reducing the need for database developers.
4. BI tools enable to authorise/restrict access to specific datasets and reports by user groups as well as by specific users. In addition, it is possible to manage user rights by which actions they are allowed to take (such as only to see built-in reports and queries, or also build reports and queries and build data cubes). This means that the same tool can be used also beyond the statistics department in the SEA, such as the SEA management, regional offices or even external users.
5. The modern BI tools enable also to apply data protection better. The user access to data can be restricted to anonymised data only, even in case the underlying query uses personal data. Furthermore, the query could for example allow only displaying aggregate results of otherwise sensitive micro data. Also, a query can link personal data from different data sources using personal identification codes, but display data for the user as anonymised data or as aggregate data.
All commonly used modern BI tools like Tableau, Qlik Sense or Microsoft Power BI have similar relevant functionalities, such as data visualisation, dashboards and AI assisted analytics, and are thus likely to meet the SEA’s needs. Nevertheless, the SEA should map its needs for a data analytics tool and compare these with what the tools offered on the market could support. In addition, technical suitability should be taken into account when choosing an appropriate BI tool, although this is not likely to be an issue considering that BURVIS database is based on PostgreSQL, which is supported by all mainstream BI tools. Operating costs are another factor when choosing a tool but should not be the driving one – a tool that is cheaper to run is a waste of money if it does not meet the SEA’s needs and requirements.
A Business Intelligence tool can be the technical solution to publish data and statistics
In addition to meeting the SEA’s internal needs to facilitate data analytics, a BI tool could be used to disseminate data and statistics in a user-friendly and flexible way. Adopting a modern tool to disseminate statistics, preferably the same solution as for in-house data analytics, helps the SEA in:
Consolidating the statistical outputs and channels into one solution.
Increasing user-friendliness of the format used for disseminating statistics.
Incorporating dynamic statistical tables (solutions ensuring flexibility and user-friendliness for the external user of statistics). The user could customise the tables, export data (Excel, CSV, XML, etc.), draw charts, save, and manage personal queries (save favourite customised queries).
Enabling disseminating metadata simultaneously with the data.
Enabling incorporating an easy application for quickly finding, comparing and sharing data through charts, maps, tables and related publications. This enables users to get a fast overview of more crucial indicators, as well as supports well users with lower analytical skills through easy and user-friendly visualisation. This can support for example higher level policy makers who have only limited time to find the most relevant facts.
Enabling developing application programming interfaces (APIs) that provide access to datasets in the catalogue of the SEA’s public datasets. The APIs (available in different machine‑readable formats, such as JSON and XML formats) could allow the user to query the data in several ways, using parameters to specify their request so that they can create innovative software applications which use the SEA’s datasets.
Enabling providing some of the SEA’s datasets as open data (pending on content of the data, i.e. the statistics unit and the data protection experts need to discuss and identify which parts of the datasets could be published as open data).
A Business Intelligence tool could be linked to the copy of the operational database for some preliminary feasibility testing
A BI tool can use data from different data sources, such as operational databases, data warehouses and publicly disseminated datasets in the web, and do that even simultaneously. For the SEA, the key data would need to come from the BURVIS database, whether directly from the operational database, its copy or a data warehouse that contains BURVIS data. Although these different options would be technically possible, a solution where a BI tool is connected to a data warehouse that contains BURVIS data is strongly recommended due to the different benefits that a data warehouse offers as explained in Section 6.7.1. In addition to the key benefits of not affecting the performance of the operating system and having data fit for analytical needs, a BI tool connected to a data warehouse means that less or even no adjustments are needed within the BI tool when changes are implemented in the BURVIS operational database. If a BI tool would be connected directly to the operational database and something needs to be changed in the operational system, queries and dashboards can stop functioning or produce wrong results, and additional work will be required to fix them.
However, the SEA can connect its BI tool directly to the operational database or its copy in the very initial stages of adopting such a tool. In this way, the SEA can quickly set up some first key queries and dashboards with minimal investments, the data would be up-to-date, and the SEA would quickly acquire some knowledge and skills on fully developing its data analytics system. Nevertheless, the SEA needs to simultaneously start setting up a data warehouse, to gradually avoid dependency between the operational system and the BI system, improve the performance and usefulness of the BI tool, and increase the feasibility to link additional (external) data with BURVIS data for analytical purposes.
6.8. Jobseeker profiling tool
Jobseeker profiling tools can be used to place clients into different service streams or flag key obstacles to employment. The SEA currently employs a basic profiling tool to categorise jobseekers into three groups, based on their proximity to the labour market. This tool, however, merely counts the number of risk factors each individual has, such as age, employment history, and care responsibilities, rather than using more sophisticated methods such as regression analysis or machine learning adopted by some OECD or EU Member States. Additionally, the SEA’s profiling process is manual and lacks IT support. Data from the SEA’s databases and external sources are not automatically integrated into the profiling, requiring manual collection and processing by counsellors.
The profiling tool’s categorisation of jobseekers into three “employability” risk groups has shown some success in aligning with the intended risk groups (as shown in Chapter 5). The profiling scores very broadly correspond with the relative risks of participants remaining in unemployment, with half of participants classified in each of the three groups (from most to least job-ready) exiting unemployment within 6, 8 and 10 months, respectively. In addition, jobseekers in the low employability group tend to participate more in ALMPs designed for those with low employability. This includes a higher likelihood of their involvement in ALMPs that focus on addressing health and integration barriers.
On the other hand, the relatively coarse method of categorising jobseekers used by the SEA’s profiling tool, which simply counts risk factors, has considerable scope for refinement. While all profiling tools invariably misclassify some individuals, the rate at which the SEA’s current tool does so is arguably quite high: for example, almost three in five (58.7%) jobseekers classified as least job-ready exit unemployment within a year. This lack of accuracy reflects the fact that a relatively simple method of counting risk factors (even with a rudimentary weighting, with risk factors such as having two children counting for double) cannot account for the nuanced and complex factors affecting unemployment duration. More sophisticated approaches, especially those that combine survey-based data with administrative data and employ statistical or AI-based profiling techniques, can dramatically improve the accuracy of the profiling model’s predictions.
The targeting of individuals who receive services from the SEA could be improved (see Chapter 5). Jobseekers with high employability are more likely to participate in ALMPs designed for jobseekers with either low or medium employability.
6.8.1. Profiling could be used to help counsellors focus on those clients most in need of support
One of the key challenges facing the SEA is how to provide timely and necessary support – including counselling or other ALMPs, such as training – for those who would benefit from it the most. Latvia’s current strategy of mandating meetings for all jobseekers within the first month of registration may entail a trade‑off, with job-ready and motivated individuals receiving counselling that would be better targeted towards less job-ready individuals. Thereafter, meetings are in principle scheduled every one to two months, although for clients such as the long-term unemployed, who are not considered labour market ready (and are referred to municipality services), the interval can be up to six months.
In order to better target support, the profiling tool could be used to identify jobseekers who are likely to quickly become employed and may not require an immediate meeting with counsellors within the first 30 days of becoming unemployed. Analysis of the unemployment registry data for Latvia suggests that sizable shares of jobseekers exit unemployment relatively quickly and that a significant minority of jobseekers have at most one barrier to employment.
A sensible approach for the SEA would be to use the results of the profiling tool to push back the initial counsellor meeting for carefully selected groups of clients. This could include those who are assessed to be likely to exit unemployment quickly, who do not express a desire to meet with an unemployment counsellor, and who do not meet any additional rule‑based criteria set by the SEA (for example, all first-time jobseekers could automatically be required to meet with a counsellor at the SEA within the first month of registering). Estimates from the profiling exercise could be used to identify, for example, individuals with at least a 75% probability of exiting unemployment within three months, and then exempt them from having an initial in-person meeting for the first three months. If the date of such a meeting were announced well in advance, this could have the additional effect of stimulating some jobseekers to become employed before the meeting: mandated participation in ALMPs has been shown to have small but statistically-significant effects on the probability of individuals becoming employed through “threat effects” (Filges and Hansen, 2017[12]).
6.8.2. In the short term, several changes could be made to the current profiling tool
Several relatively simple changes could be made to the existing profiling approach in the SEA in the short term. These changes would not require significant investments into the IT infrastructure and may thus be more feasible in the short term compared to the more comprehensive changes suggested in the next section.
The profiling questionnaire should be administered in a way so that detailed data on each of the reported individual-level risk factors from the profiling tool – the inputs to the calculations – can be retained, ideally in the BURVIS database. Currently, only the summary classification of an individual into one of the three main categories from the profiling tool is retained (even this is provided without the date an individual was profiled). This makes it difficult to monitor and evaluate the effectiveness of the profiling tool. Retaining such detailed information could be facilitated by administering the profiling questionnaire via a (secure) online survey (ideally a user interface integrated into the SEA’s online tools), with the individual responses shared with the SEA counsellors (ideally via BURVIS). For jobseekers who may have difficulty filling out an online survey themselves – most prominently, those who do not register as unemployed with the SEA online – the SEA counsellors could input this information at their first in-person meeting. This would be similar to an approach recently trialled in Australia (DEWR, 2021[13]), which was generally found to be more time‑efficient and easy to use – although a small minority of jobseekers reported difficulty in completing the questionnaire Box 6.3. Administering the survey online could also be useful for using the profiling results to determine the date of the first meeting with the SEA counsellors (as discussed further below).
Box 6.2. Australia’s online trial of its profiling questionnaire
Australia has been using a statistical profiling model since the early 1990s. The current system, the Job Seeker Classification Instrument (JSCI), has been in place since 1998. The JSCI, which is currently under the responsibility of the Department of Employment and Workplace Relations (DEWR), originally collected data through interviews at the time of a jobseeker’s initial income support claim. Those interviews are undertaken by Services Australia (the primary service delivery agency for the Australian Government) or an employment services provider. Any significant changes in a claimant’s circumstances necessitate a reassessment of their JSCI score.
In 2018, Australia started a trial to move this data collection online, resulting in the Jobseeker Snapshot (JSS). Fully integrated into DEWR’s the Online Employment Services platform since April 2020, the JSS essentially gathers the same information as the earlier interview-based system, albeit with some adjustments in question wording and order to suit the online format, plus additional questions around whether the jobseeker has the appropriate access or ability to self-manage in online services. Those unable to complete the JSS online can still provide information via telephone or in-person interviews.
The JSCI model has undergone re‑estimation with each update of the mainstream employment services programme. For instance, it was recalibrated in 2015 with the introduction of Jobactive, which replaced the Job Services Australia program, as noted by the Department of Education, Skills, and Employment in 2021. This ongoing refinement ensures that the model stays relevant and effective in assessing jobseekers’ needs, and circumstances and level of labour market advantage.
Sources: DEWR (2021[13]), Online Job Seeker Classification Instrument Trial Evaluation Report, www.dewr.gov.au/download/11093/online-job-seeker-classification-instrument-trial-evaluation-report/21320/document/pdf; Langenbucher and Vodopivec (2022[14]), “Paying for results: Contracting out employment services through outcome‑based payment schemes in OECD countries”, https://doi.org/10.1787/c6392a59-en.
The profiling questionnaire could be revised to incorporate questions that have been scientifically cross-validated via rigorous empirical analyses. The factors currently in the profiling model are important in determining how long an individual may remain unemployed. However, they may also contain important omissions and the relative weighting would ideally be subject to a rigorous process to empirically ascertain their relative importance. Several countries have adopted survey-based profiling questionnaires which could be conceivably applied, with sensible modifications in the case of Latvia. Two of these stand out in terms of the degree to which they have been validated and tweaked (for details on the specific questions, see Annex 6.A):
“Work Profiler 2.0”, a questionnaire with 18 key factors used by the Dutch PES (Wijnhoven et al., 2023[15]). Some of the key dimensions covered in this questionnaire relate to aspects such as age, duration of employment in the last job, views on returning to work, desired profession, household position, duration of unemployment benefits, industry experience, job search behaviour, and self-perceived health (see Annex Box 6.A.1). This is an updated version of a questionnaire which had been previously used by the Dutch PES, “Work Profiler 1.0” that contained 11 factors and which itself had been selected, in multiple steps, from a list of 550 potential factors.
A questionnaire with roughly 10 questions each for jobseekers and caseworkers used in Denmark (Bodilsen, Albeck Nielsen and Rosholm, 2023[16]). This questionnaire has been shown to be especially accurate at predicting unemployment for the long-term unemployed, although conceivably it could be useful for other target groups as well. Furthermore, it is arguably broadly generalisable to other countries – a subset of the questions have been adopted by several PES offices to profile jobseekers in Sweden.
Implementing a revised questionnaire for profiling jobseekers in Latvia would also require assigning weights to each of the questions to calculate a profiling score. This would ideally be done by piloting the survey with a group of jobseekers, tracking their labour market outcomes over the following year and then developing a suitable set of weights based on these outcomes (using e.g. a logistic regression). A simpler, but less accurate, alternative would be to use the weights calculated in other countries and apply them to Latvia (for example, Wijnhoven et al. (2023[15]) list regression coefficients that could be applied, with some modifications, to the Latvian case). In the longer term, a new statistical profiling tool combining survey and administrative data could be designed and implemented.
In the longer term, the SEA should aim at adopting a more sophisticated profiling tool based on the best practice examples of other OECD countries. Such a tool could be implemented as part of a broader overhaul of the digital counselling tools within the SEA. To facilitate the work of counsellors, it could automatically incorporate information from administrative sources in addition to subjective, survey-based sources. Although a recent study from Denmark (Bodilsen, Albeck Nielsen and Rosholm, 2023[16]) found only marginal gains from adding administrative data into a well-designed questionnaire, using administrative data where possible would make the profiling process more efficient for jobseekers and counsellors. The tool would ideally go beyond merely classifying jobseeker employability – it would provide detailed insights into factors influencing a client’s score and suggest specific actions to enhance a client’s employability through, for example, participation in training (a topic also discussed in Section 6.9).
Such a profiling tool could adopt one of two broad methodological approaches, either based on traditional statistical techniques or newer ones based on AI. Although AI-based models can offer better accuracy than traditional jobseeker profiling tools, the precise degree to which their predictions are better is an unresolved question subject to ongoing research (Gallagher and Griffin, 2023[17]; Andonovikj et al., 2024[18]; Boškoski et al., 2021[19]; Bodilsen, Albeck Nielsen and Rosholm, 2023[16]). In contrast to most traditional statistical techniques, where the contribution of the tool to explaining an individual’s profiling score is relatively straightforward, AI-based models often adopt a more “black box” approach where the underlying reasons for a classification can be more difficult to understand. However, some AI-based approaches can generate decision rules which are more straightforward to understand (Gabrikova, Svabova and Kramarova, 2023[20]) and the contribution of individual factors can still be measured in AI-based approaches (Andonovikj et al., 2024[18]). While the potential for introducing bias in the profiling algorithm is not unique to AI-based approaches, their potential for exacerbating such biases is greater given this greater difficulty in understanding the underlying factors explaining the model: a study of the AI-based tool used in Flanders (Belgium) by Desiere and Struyven (2020[21]) showed that increased accuracy for the entire population of jobseekers came at the cost of increased misclassification of immigrants as more difficult to employ (see Box 6.3 for a description of this tool). Regardless of the approach taken, modelling decisions can have important implications on the assessed importance of different factors used to calculate the profiling score (Bach et al., 2023[22]).
Box 6.3. VDAB’s “Job opportunity” AI-based profiling model
Since October 2018, the PES of Flanders (Belgium), VDAB, has been using its “Job opportunity” AI-based profiling tool to help guide the provision of services to clients. “Job Opportunity” employs a random forest model – a type of machine‑learning technique – to predict the likelihood of an individual securing a job within six months. This model utilises data gathered from jobseekers upon their registration with VDAB and the completion of their online profiles. The data inputs into the model include:
Demographic information: This includes residence, age, education, vocational skills and competencies, previous work experiences, and periods of unemployment.
Work preferences: Details such as desired occupation, industry, or location.
Behavioural indicators: These are inferred from the jobseekers’ activities on the VDAB’s My Career platform, including logins, profile updates, and interactions with job listings.
Both jobseekers and caseworkers can update this information anytime, ensuring the data feeding the prediction model remains current, as the model and profiling scores are updated daily. The model translates its predictions into a colour-coded system to prioritise customer support. Specifically, the following colour categories are generated:
Red: under 35% probability of finding employment within six months.
Orange: 35‑49.9% probability.
Yellow: 50‑64.9% probability.
Green: at least 65% probability.
Black: profiling score cannot be calculated because of lack of data (this may be due to certain exemptions, like being enrolled in a training program, or incomplete online profiles, indicating a need for guidance in profile completion).
In the service line, these colours are used to prioritise interactions with customers, with black and red files being addressed first, followed by orange, and green if remaining capacity. Caseworkers only receive the group classification of a jobseeker, not individual scores, to prevent bias in decision-making. They use this information, along with a phone interview and their professional judgment, to determine the support a jobseeker needs. VDAB has a goal to contact and assess all new jobseekers within six weeks of their registration.
Source: VDAB (2021[23]), AI & Advanced Analytics @ VDAB, https://web-archive.oecd.org/2020-11-24/570855-AI_presentation_VDAB.pdf; in addition to bilateral discussions.
Given these trade‑offs involved and taking into account Latvia’s specific circumstances, a profiling tool adopting an established statistical technique may be the more sensible approach. The AI-based tools are the subject of considerable ongoing research, including into their potential for introducing bias. Furthermore, many of these more advanced solutions require the capacity to adopt AI solutions and regularly update them based on changing conditions (this may be a particularly important consideration in the case of AI-based models, whose greater accuracy may disproportionally be due to how they incorporate period-specific factors). Such considerations have led the Swedish PES, Arbetsförmedlingen, to adopt a new profiling model based on a survival analysis model, an established statistical technique commonly used in the medical sciences (Arbetsförmedlingen, 2023[24]). This new model has relatively high accuracy and was introduced specifically to provide more accurate predictions for individuals at different points in their unemployment spell (the previous model was used for profiling jobseekers throughout their unemployment spell, even though it was designed specifically to predict unemployment duration only for newly-registered unemployed). The precise choice of model by the SEA in the long-term should also take into account how it would fit in with a broader strategy of jobseekers’ customer journey, as well as its adoption of new digital counselling tools.
6.8.3. The involvement of the SEA counsellors and the broader public in the process of designing and implementing any new profiling tools should be carefully considered
Experience of PES in implementing new profiling tools underscores the importance of securing widespread support when such new tools are used to guide decisions on which services are provided to specific clients. The experience of Austria, which piloted a new statistical profiling model in 2019, serves as an instructive example (OECD, 2020[25]). Despite thorough and detailed external communication, a vigorous public debate arose about the ethics of “machine decision making” and data protection. This debate centred on four concerns: First, the apprehension that an automated algorithm, instead of a caseworker through personal consultations, would determine services for jobseekers. Second, the fear of potential discrimination by such statistical profiling, especially against women and individuals with disabilities. Third, concerns that identifying long-term unemployed or those at risk of becoming so could lead to reduced services for them. Fourth, there was criticism regarding the opacity in both the new system’s implementation and the profiling tool. These experiences highlight the importance of involving many stakeholders at different stages of the design and implementation process.
6.9. Skills profiling tool
As of end of 2023, the SEA is in the process of digitising short questionnaires to get a general understanding of jobseekers’ skills and preferences concerning working conditions to support the SEA’s job matching and career counselling services. Following this, SEA looks to implement more sophisticated skills profiling tools to support counsellors to better and more quickly understand their clients, and to help jobseekers gain self-knowledge of their skills and interests, as well as explore ideas for occupations they should seek. Furthermore, the skills profiling results are expected to contribute to a better overall profiling of jobseekers’ needs for support (Section 6.10). A better skills profiling would be relevant also for people at risk of job loss to target training, as well as for the working-age population more generally to better manage adult learning in co‑operation with the Ministry of Education.
To minimise the costs to design and adopt more advanced approaches to skills profiling, it is sensible for Latvia to learn from other countries and organisations about their solutions, and potentially even aim to use one of the available solutions after some adjustments if feasible. The SEA and MoW have already started a dialogue with France and Germany to seek synergies across countries in co‑operation with the OECD and DG REFORM in the context of the same project as the current report.
6.9.1. Skills profiling tools can serve different purposes
A range of different approaches exist in other countries concerning profiling jobseekers’ skills, reflecting a diverse variety of purposes. Thus, it is crucial to first carefully define the purpose of skills profiling for the SEA and then match the right tool to the right task. While some tools can serve a variety of purposes – with perhaps some primary and other secondary purposes – it is important to identify the main purpose of the tool early in the design process so that the other design choices are made to best serve this purpose.
For example, skills profiling tools can:
Provide insights and self-knowledge to jobseekers on their own skills, interests, and personality. This is done (on the topics assessed) by essentially all skills profiling tools when the results are displayed.
Support jobseekers to explore occupations and jobs that potentially match their skills and interests, as is done with the OECD’s Skill Profiling Tool and Germany’s New Plan (see Box 6.4). Also Austria’s Berufskompass shows professions that map to jobseeker’s interests, provides insight into their personality, and includes career recommendations for the types of occupations ranked as most suitable for them (AMS, 2022[26]). These suggestions come with links allowing the client to read more about each occupation. Links are also provided to all training courses available through the PES. Finally, a feedback box allows the PES to understand what works well and what are the challenges jobseekers have with Berufskompass. In 2023, the Austrian PES adopted additionally a more modern AI-based solution Berufsinfomat to advise jobseekers in career and training pathways, using information on 2 500 occupations and training courses and chatGPT (AMS, 2023[27]).
Match jobseekers to appropriate training, which may be hosted externally to the tool (e.g. Germany’s New Plan) or within the online environment of the tool (e.g. Pix in France and Ikanos in Spain (Basque region), which provide training for digital skills).
Support employment counsellors and career counsellors to provide better job counselling and career management services and refer their clients to appropriate training and education programmes. This could be in a variety of ways including through helping employment counsellors understand their clients or by suggesting suitable occupations, job search strategies, upskilling and reskilling possibilities or vacancies. For example, counsellors in the Slovenian PES (use the EU Skills Profiling Tool for Third Country Nationals in the beginning of the counselling process in order to set employment goals based on the migrant’s previous experiences and skills, which are not always linked to the migrant’s formal qualifications (European Commission, 2023[28]). This EU tool aims to map and document skills to provide support for further assessment (including career guidance), as well as identifying training needs and suitable employment. It is based on users self-identified skills across a range of domains, including languages, literacy, numeracy, digital skills, professional skills, driving skills, as well as questions about a person’s education and training. As the skills are self-assessed, it is not suitable as an authentication or recognition tool.
Support PES management and policy makers by providing an overall picture of the skills of PES clients (i.e. information on skills supply on the labour market), e.g. like OECD’s Education and Skills Online (see Section 6.9.3).
Accredit jobseekers with a certificate, so they can demonstrate their skills to potential employers (e.g. on their CVs). Examples of tools with this purpose include specialist tools to assess digital skills, such as Pix and Ikanos. The Netherlands National Centre for Recognition of Prior Learning (EVC) and the Dutch PES collaborate to match jobs seekers who have undertaken a recognition of prior learning assessment with jobs. Their tool supports an in-depth process lasting three to six months to allow individuals to be recognised for skills they have gained without formal recognition, such as by learning on the job, so that they can demonstrate these skills to future employees (EVC, 2023[29]).
As an input into the jobseeker profiling approach, such as an input into statistical profiling tools that calculate jobseekers’ distance to the labour market based on the experiences of similar jobseekers in the past (OECD, forthcoming[4]; Wijnhoven et al., 2023[15]), see also Section 6.10.
Skills profiling tools are of course not the only tools and processes that support these purposes. For example, some tools use past outcomes of similar clients to help search for appropriate ALMPs (particularly training) or job opportunities (e.g. Spain’s Send@ tool (OECD, 2022[8]), and also a feature of Germany’s New Plan) to support career counselling services. Other tools, such as VDAB’s Jobnet, use a plethora of information to automatically suggest vacancies to jobseekers (OECD, 2021[30]). Another type of tools profile not the skills of workers, but the skill demands of employers. For example, the Danish PES uses AI to estimate firms’ demand for skills based on online vacancy postings (The Danish Agency for Labour Market and Recruitment, 2021[31]) to guide career counselling services and target training measures. More broadly, Skills Assessment and Anticipation exercises should support career counselling and targeting training measures and need to be implemented complementary to skills profiling tools. For example, the Estonian PES assesses jobseeker skills during the first meeting with the jobseeker, including assessing their digital skills using the International Digital Skills Framework. Referrals to training take into account the skills profiling results, as well as forecasted skill needs from the Skills Assessment and Anticipation exercises, both in terms of short-term (internally conducted Occupational Barometer), and long-term forecasts (an in-depth assessment exercise involving a wide range of stakeholders in Estonia), see OECD (2021[32]; 2021[33]; 2023[34]).
Box 6.4. Skills profiling to help jobseekers explore occupations and jobs
The OECD’s Skills Profiling Tool
The purpose of the OECD’s Skills Profiling Tool (SPT) is to highlight users’ skills, so they can explore suitable occupations (OECD, 2022[35]). The SPT differs from other OECD skills assessment projects in that the SPT focuses on providing advice to individual users, as opposed to OECD initiatives like the Programme for International Student Assessment (PISA) and the Programme for the International Assessment of Adult Competencies (PIAAC) that aim to provide policy makers and others with cross-country information on the skills and competencies of teenagers and adults respectively. Linked to the PIAAC initiative, the Education & Skills Online is another OECD assessment tool designed to provide individual-level results on literacy, numeracy and problem solving in technology-rich environments, that will be further developed into a more modular tool that could be used to profile the skills of jobseekers, people at risk of job loss, as well as adult population more generally (see Section 6.9.3).
Launched in 2022, the SPT aims to capture both hard and soft skills. Hard skills are measured by asking users about their experiences (e.g. with cooking, cleaning, caregiving etc.) and how often they perform certain tasks (e.g. read books, read the news, write code, make charts and tables, etc.). Soft skills are measured by a personality test. A report is then generated with a score in each of seven skill domains: literacy, numeracy, digital skills, customer and personal service, time management, motivation and commitment, and creative thinking. Based on O*NET data (O*NET, 2023[36]) on the skills required in many different occupations (using the International Standard Classification (ISCO) grouping), the tool suggests the occupations that best match the user’s needs.
The SPT is available free of charge to anyone interested. It has been piloted in five Latin American countries and some are currently using it for some of their (largely underprivileged) clients.
“New Plan” – a German PES tool with three pillars: Test, inspire, and search
New Plan is a digital tool of the German PES that helps jobseekers find “their true talents and strengths” and is also useful for career counsellors to understand their clients (European Commission, 2021[37]). The tool consists of three pillars, one of which focuses on the measurement of skills while the other two help jobseekers consider different occupations and trainings. The three pillars are:
Test: Modular tests on different topics and competencies.
Inspire: Makes tailored suggestions for career changes based on a jobseeker’s employment history matched to the experiences of others with similar profiles drawing from a database of millions of job-to-job transitions.
Search: Allows jobseekers to search for further training opportunities.
The Test pillar features a modular design. Different tests are separated into four categories depending on purpose: development opportunities (looks at suitable professions), further education (assesses suitable training opportunities), activities (assesses quickly attainable alternative occupations), and quick competency checks (assesses selected competencies, a few minutes per competence) (Bundesagentur für Arbeit, 2022[38]).
New Plan development began in late 2019 and the first version was made available in December 2020. It is part of Germany’s Lifelong Career Guidance initiative and is provided free of charge to all interested and has so far served around 500 000 people. Career counsellors at the PES report it is useful in their work, while the German PES stresses that the tool is designed to supplement the work of counsellors and not replace them.
Source: OECD (2022[35]), The OECD Skills profiling tool, http://oecd-skillsprofilingtool.org/home (accessed on 26 February 2023); O*NET (O*NET, 2023[36]), O*NET Online, www.onetonline.org (accessed on 26 February 2023); European Commission (2021[37]), New Plan: A self-assessment tool for orientation and counselling, https://ec.europa.eu/social/BlobServlet?docId=24836&langId=en; Bundesagentur für Arbeit (2022[38]), Möglichkeiten testen [test possibilities], http://web.arbeitsagentur.de/sete/testuebersicht/ (accessed on 26 February 2023).
6.9.2. Further design choices need to serve the tools’ purpose and its integration into the SEA’s processes
Once the main objective of skills profiling has been decided, SEA needs to define the scope of the profiling, particularly in terms of the types of skills that are relevant to cover in the tool. An advantage of a broad test is that it can potentially be useful for a wide range of jobseekers. An advantage of a narrowly focused test is that while it may not be suitable for many jobseekers it may provide a more accurate in-depth assessment of a particular skill which may be better suited to understanding the level of training that matches a client’s needs or for providing accreditation to demonstrate this skill to an employer. As such, some tests developed so far are very broad, measuring a wide range of skills (e.g. Germany’s New Plan or the OECD’s Skill Profiling Tool which look at both hard and soft skills). Other tools focus more narrowly only on certain skills (e.g. Pix and Ikanos, which focus on digital skills, Box 6.5).
Box 6.5. Profiling digital skills
Pix – French non-profit organisation providing digital skills testing, certification, and training
Pix is a French based non-profit public organisation that provides an online platform to test digital skills (Pix, 2023[39]). The tool allows users to test across five domains (information and data, communication and collaboration, content creation, protection and security, and digital environment) covering 16 skills assessed at six different levels. Tutorials are then provided that help students improve based on their current level. Originally founded in France, Pix has now been used by schools and companies in 30 countries, with 6 million active users and over 2 million certifications taken.
The test includes examples of “real life” situations and goes beyond simple multiple‑choice questions. Moreover, as the test is a knowledge‑based measure of performance (as opposed to self-described competency level), the tests can be used as a means of certification. Indeed, to help students demonstrate their skills to employers, a certificate is generated upon passing a test that users can place on their CVs (OECD, 2020[40]).
A number of tools use the EU’s DigComp framework to assess digital skills
In addition to the French example of Pix, many other tools have been developed to support the profiling of digital skills. These include the digital skills tests Mydigiskills by the All Digital association (All Digital, 2021[41]), as well as skills tests provided by Europass – the European Union’s set of tools to help with CVs, cover letters, and finding jobs. Both Mydigiskills and Europass’ digital skills test uses the European Digital Competence Framework for Citizens (DigComp) (European Commission, 2023[42]). This framework encompasses five areas – information and data literacy, communication and collaboration, digital content creation, safety, problem solving. The Europass test includes a mix of self-assessment (where uses rate their own view of their skills) and knowledge‑based questions with an objective answer (e.g. true/false and multi-choice questions).
Ikanos developed in Spain (Basque region) is another test using the DigComp framework (Ikanos, 2023[43]). However, in addition to hosting a digital skills test, the Ikanos platform is notable for providing an entire ecosystem of digital learning. This ecosystem consists of a 5‑step-model: “discover” (enables individuals to test their skills), “audit” (presents profiles of these skills), “analyse” (helps set objectives), “guide” (provides a resource catalogue,) “learning” (provides an e‑learning environment to improve digital skills), and “evidence” (provides certification in approved testing centres, which can then be used as evidence of skill acquisition to employers).
Source: Pix (2023[39]), Pix, http://pix.fr (accessed on 26 February 2023); OECD (2020[40]), The potential of online learning for adults: Early lessons from the COVID‑19 crisis, https://doi.org/10.1787/ee040002-en; All Digital (2021[41]), mydigiskills, http://mydigiskills.eu/ (accessed on 26 February 2023); European Commission (2023[42]), Test your digital skills!, https://europa.eu/europass/digitalskills (accessed on 26 February 2023).
If the accuracy of skills profiling is highly relevant for the objective of the tool (e.g. for certification, accreditation, assigning the right level for a training group), knowledge‑based tests could be part of skills profiling. Such tools ask jobseekers questions aiming to test their knowledge, such as via true/false questions, multiple‑choice questions, or even simulation of tasks (as is done with Pix’s assessment of digital skills).
Self-reported tests are a more subjective measure of skills, where jobseekers self-report their own level of skill. Such tests can be easier to design as the questions can be quite straightforward, but they are not suitable as a means of accreditation, and moreover clients may not always be well placed to understand their own skills or may have a different sense of scale in mind when thinking of different skill levels. In between self-reported and knowledge‑based tests, some tools, like the OECD’s Skill Profiling Tool, ask users how often they engage in certain tasks, and approximate the skill level based on how frequently a person engages in tasks that require this skill.
As it might be more relevant for the SEA to cover different types of skills in the profiling tool, a more modular approach rather than a single test will be likely more suitable. An advantage of a single test is that it can be useful to all jobseekers and clients are not overwhelmed with the number of choices of test. However, the advantage of a modular test is that clients need to take only some of the tests (not all of them). This can be particularly useful to provide different tests to different jobseekers (e.g. those with backgrounds in different occupations) and then assess the skills relevant to the jobseeker in an in-depth way. A modular approach also means that new modules assessing different skill areas can be added as new features when the skills profiling tool is updated.
Furthermore, the technological solution of a skills profiling tool could further increase user experience. For example, the Flemish PES in Belgium, VDAB, uses an orientation test Orient 2.0 to help understand the self-described interests of jobseekers and how these interests map to professions (VDAB, 2023[44]). While this test measures jobseekers’ interests rather than their skills, it shows how AI can be used to shorten questionnaires and dramatically save time for both clients and counsellors. Orient 2.0 asks a series of simple questions for self-declared interest (thumbs up or down) in many professions, skills and tasks. Upon completion the results show a list of professions ranked by how well they match client’s interests. Previously VDAB used a test with over 100 questions that required manual assessment by its labour market experts (Radix, 2020[45]). A new test makes use of machine learning and artificial intelligence (AI) to adapt the questions asked of clients based on their previous responses (allowing the average number of questions to fall from 114 to 58 questions) and then automatically compile the results (saving counsellor time). This reduced the time taken to complete the test by nearly 80% from an average of 45 minutes to 10 minutes.
6.9.3. Skills and Education Online could be a suitable option for Latvia
Education and Skills Online (E&S Online) is a web-based tool developed by the OECD to assess adults’ cognitive skills, particularly literacy, numeracy and problem solving in technology rich environments (OECD, 2023[46]). E&S Online provides individual-level results that can be benchmarked against the national and international results of PIAAC.
E&S Online can have many different uses cases, such as government organisations aiming to get insights on the skill level and training needs of the workforce or training providers to assess adult numeracy and literacy to better place participants in specific courses and evaluate their progress. Nevertheless, the current design of this assessment tool is quite time‑consuming for the participants as the core modules take 65 minutes and need to be completed in one sitting, and the entire test about 120 minutes, with the optional modules having the possibility to be taken at a later point in time. Thus, the present set-up of E&S Online is not very well suited for PES to get quick insights of jobseekers’ different relevant skills to provide them job search and career management services and refer them to appropriate reskilling and upskilling options (OECD, 2019[47]).
To meet the increased and diversified needs of labour and education authorities and related stakeholders across the OECD countries to profile and assess adult skills, the OECD is aiming to redesign E&S Online. The redesigned assessment tool will be made more flexible to tailor it to the objectives of the organisations benefitting from the tool. Different versions of the tool will cater to different purposes from providing quick assessments for individuals to reporting representative statistical overviews of a population to organisations. The more modular design will aim to enhance user experience and value‑added, as well as enable covering additional skill sets relevant for the different labour markets (Figure 6.7). This may include adding optional modules on skill use at work, digital skills, and financial literacy amongst others. Further adjustments involve for example the funding model and security set-ups to increase the availability of the tool across countries. The launch of the adjusted version of E&S Online is planned to take place by the end of 2025.
Figure 6.7. A more modular approach of Education and Skills Online will increase the user friendliness and flexibility for both institutional and individual users
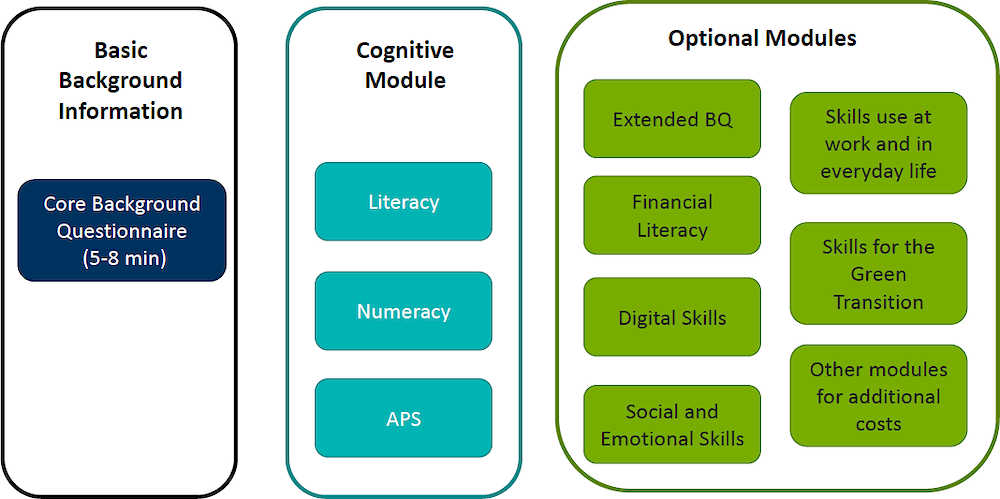
Notes: BQ – Background questionnaire.
Source: OECD (2023[48]) “A Personalised Skills Assessment Tool: Education and Skills Online Version 2.” Information webinar on 14 December 2023.”
Adopting E&S Online would be a viable option for Latvia to be able to profile the skills of jobseekers, people at risk of job loss and the adult population more generally. The adjusted set-up will be more feasibly integrated to the SEA’s processes to understand their clients’ needs for support, and the additional modules will be likely able to cover the key skills needed on the Latvian labour market (e.g. digital skills). Furthermore, the modernised E&S Online tool that will be better aligned with the innovations introduced recently in PIACC, has the potential to become a recognised international standard to profile adult skills, thus enabling Latvia to better compare itself with other countries. In addition, adopting E&S Online would not require an in-depth knowledge in the SEA on designing the technology nor the content of the tests for skill assessments, thus eliminating such potential barriers that could arise in case of in-house development. Nevertheless, adopting E&S Online in Latvia will need some financial resources from Latvia to contribute to the development and maintenance costs together with other participating countries. The development of optional modules, such as digital skills, will incur further costs.
6.10. Job matching tool
The SEA considers job mediation and matching services rightfully to be some of its key responsibilities, and has developed dedicated digital solutions for these purposes. The SEA counsellors access functionalities to match jobseekers and vacancies within the BURVIS interfaces, thus being able to recommend vacancies to apply to during the jobseeker counselling sessions, as well as recommending suitable candidates for employers. In addition, jobseekers and employers can use digital matching services independently in the SEA’s CV and vacancy matching portal (CVVP). Although the matching algorithm used in the SEA’s digital infrastructure is transparent and straightforward for the different user groups and thus caters to their needs, further improvements in these digital solutions could lead to better possibilities to match labour supply and demand in Latvia.
6.10.1. Job mediation and matching services are key for PES
Mediating jobs and matching jobseekers and vacancies are some of the core tasks of PES across OECD countries, aiming at facilitating labour market functioning and reducing frictions. Job mediation and matching services in PES have a clear dual objective of supporting both jobseekers and employers simultaneously. In addition to contributing to a better alignment between labour supply and demand and thus decreasing mismatches, job matching services can decrease job search duration and costs for jobseekers simultaneously with reducing vacancy durations and costs to search for employees on the side of employers, hence potentially even encouraging job creation (Pissarides, 2000[49]; Kircher, 2020[50]).
As job mediation and matching services are central, PES across OECD and EU countries have developed digital support for such services (OECD questionnaire on digitalisation in Public Employment Services to support the provision of active labour market policies launched in March 2023; the share of countries calculated based on those countries that responded to the question). Nevertheless, the level of availability, effectiveness, sophistication and user experience of such digital solutions varies significantly across countries. For example, the Romanian PES enables employers to publish their vacancies on a dedicated website, but the platform does not enable to match vacancies and jobseekers or even filter the vacancies by different criteria other than job location. The criteria to filter matching vacancies is also for instance very limited for jobseekers in Greece and Hungary. Furthermore, the full details on the vacancies have been so far available for the jobseekers in Greece only when visiting a local employment office due to the concern of digital tools replacing the need for counsellors – the situation is, however, likely to change in the near future due to a major on-going reform in the Greek PES advancing both the digital backbone and the work processes (OECD, 2024[51]). At the other end of the spectrum are PES that have already a long experience in continuously improving their digital infrastructure to support job mediation and matching services, offering advanced digital solutions for the PES staff, jobseekers and employers (e.g. Belgium (Flanders), Estonia, France, Korea, the Netherlands).
In total, digital solutions to enable jobseekers to independently search for matching vacancies are available in 93% of OECD and EU countries, digital solutions to enable employers to independently search for staff in 88% of countries, and digital solutions to enable PES counsellors to match jobseekers and employers (and consequently support either of the labour market stakeholders) in 80% of countries. Enabling employers to directly contact jobseekers is less often implemented than the other way around due to more complications in making personal data available rather than employer data (i.e. explicit consent form jobseekers is needed), as well as the more traditional approach of jobseekers having to apply for the vacancies. Some countries have opted for having digital matching services available for jobseekers and/or employers rather than PES counsellors, because employment services are largely contracted out (e.g. Australia), job mediation services are the responsibilities of sub-national authorities (e.g. Spain), or the PES operating model assumes that the labour market partners have self-efficacy to use digital tools independently, enabling employment counsellors to focus on other tasks (e.g. Sweden).
Regardless of the exact institutional set-up and operating model of the PES, digital job mediation and matching services can contribute to more effective and efficient service delivery. For example, the online platform Job Market in Finland (Box 6.6) has been evaluated to increase efficiency of matching services, i.e. shorter vacancy durations and higher mediated vacancy numbers (Räisänen, 2023[52]; Räisänen, 2022[53]). Other job matching platforms and related digital tools have been evaluated for example to increase firm hiring rates (Horton, 2017[54]), improve job search strategies and increase the number of job interviews for jobseekers (Belot, Kircher and Muller, 2022[55]), and increase job finding rates (Barbanchon, Hensvik and Rathelot, 2023[56]; Behaghel et al., 2022[57]). Nevertheless, the emergence and magnitude of positive effects, as well as the possibility for negative effects (e.g. distorting competition for certain types of vacancies (Altmann et al., 2022[58])) depends on the design and implementation of the digital job matching tool. More tailored vacancy recommendations for jobseekers could minimise potential negative spillover effects (Barbanchon, Hensvik and Rathelot, 2023[56]) and a job matching algorithm that takes jobseeker preferences and labour market situation more into account can increase the positive effects (Alfonso-Naya et al., 2021[59]).
Box 6.6. Finland’s job matching tool uses AI to automatically identify and code skills
Finland’s public employment service (PES) maintains a database of about 224 000 jobseeker profiles, about 10% (24 000) of which are active, alongside around 20 000 job vacancies. To support matching vacancies to jobseekers, Finland’s PES uses a sophisticated job matching tool, Job Market, which incorporates the use of artificial intelligence (AI).
AI is used in several ways within the tool, aiming to improve useability and attach skills to jobseeker profiles, employer vacancies, and training courses, which can later be used to match jobseekers with vacancies as well as training. The AI-based algorithm allows for comprehensive identification of skills regardless of how they are worded or indeed the language they are written in with the tool compatible with Finnish, Swedish, and English. By allowing jobseekers and vacancies to be searched for and matched on the basis of skills rather than occupation alone the tool promotes occupational mobility.
To support linking jobseeker’s profiles to skills an AI system analyses a user’s profile and then a “competency recommender” suggests to them skills and occupations, which the user can then confirm. These skills are coded using the European Skills, Competencies, Qualifications, and Occupations (ESCO) classification. This process is easier for users than scrolling through the thousands of skills in ESCO. A similar AI process takes the text from a job description (so-called “free‑text”) and codes the skills associated with this text. Likewise, this is done for the descriptions of training and education opportunities available for jobseekers. A key difficulty is identifying the relevant parts of a job description. For example, a job description may include a generic description of the company or details on the application process, neither of which is relevant text in determining the skills of a job. Different AI (e.g. machine learning and natural language processing) models are used to identify relevant text. Other algorithms help to code some skills as “required” and others as “optional” (especially relating to language skills).
A key part of the tool’s natural language processing capabilities involves using an externally trained Large Language Model (LLM) to process text. LLM’s have made rapid progress in recent years and many different models are available. The LLM is then adapted to the matching tool’s use case through a process known as “fine‑tuning”, where the model is trained on PES specific text. Data are also used on each ESCO skill description available in the substantive ESCO metadata. Such models can then compare the metadata descriptions of a skill with the jobseeker profile, or job vacancy, to understand if the skill is present in the job description.
Evaluating the effects of Job Market is difficult, partly because the technology is available to all jobseekers and employers (so there is no obvious control group) and because other interventions have been rolled out at similar times, such as the Nordic Employment Service Model in 2022, that may affect matching. Due to these constrains an evaluation of Job Market noted its findings were inconclusive but suggestive of a relatively modest effect on improving matching efficiency (Räisänen, 2023[52]).
Source: Hirsimäki (2023[60]), A platform for job matching using AI: Job Market Finland, Digital tools for matching jobseekers and vacancies: international workshop, www.oecd.org/els/emp/presentation_job_matching_Finland.pdf; Räisänen (2023[52]), How to approach the ex ante employment effects of the Job Market matching platform?, www.oecd.org/els/emp/Session3_Job_Market_Matching_Platform.pdf.
6.10.2. The SEA could improve its job matching tool by strengthening competency-based matching
The SEA’s rules-based job matching algorithm in CVVP and BURVIS uses simple and straightforward filters to allow jobseekers, employers and counsellors to match jobseekers and vacancies. The main filters focus on occupations (desired occupations, experience in occupations), geographic location, working conditions (wage, full time/part time), and a few hard skills (driving licences, language skills and digital skills). In addition, the SEA introduced the European Union’s employment, skills, competences, qualifications and occupations (ESCO) taxonomy in 2021 regarding both the vacancies and jobseeker CVs. Nevertheless, as ESCO classification is extensive and detailed and thus complex for jobseekers and employers to use, job matching has remained to use only limited occupations, skills and qualifications.
Strengthening the component of competencies and skills in the SEA’s matching algorithm could improve the performance of the job matching tool. Focusing on competencies rather than occupations would enable jobseekers to identify matching jobs that otherwise they would have not thought to be qualified for, as well as employers to discover a larger pool of suitable staff. Focusing on competencies is particularly important in the context of changing labour market structure and needs, for example to identify suitable candidates for bottleneck or new emerging occupations (that lack jobseekers that have previously worked on these occupations), as well as provide alternatives for jobseekers that have previously worked on occupations that are no longer needed on the labour market in as large volumes. Evaluations of related digital tools that make recommendations on alternative occupations considering jobseeker skillsets or focusing on occupations of increasing importance have shown to affect jobseekers’ job search behaviour, and lead to more stable employment and higher earnings (Belot, Kircher and Muller, 2022[61]; Altmann et al., 2022[58]; Charleer, Gutiérrez and Verbert, 2019[62]).
Moving towards a more competency-based matching algorithm could be implemented gradually in the SEA. As the first priority, the SEA could introduce those competencies in the job matching tool that are considered to be of key importance on the Latvian labour market. For example, the job matching tool used in the French PES includes three sections regarding skills and competencies: i) automatically proposed skills associated with specific occupations (occupation of the vacancy on the employer side, desired and previous occupations on the jobseeker side) according to the skills taxonomy with the possibility to add additional skills, ii) other hard skills like language skills and driving licences, iii) 16 soft skills that are considered to be the most important for the French labour market (Box 6.7).
Box 6.7. France uses competency-based matching to move beyond occupation-based matching
The French public employment service, Pôle Emploi, focuses on competency-based matching to help match jobseekers and employers. This system allows, and indeed encourages, jobseekers to consider switching to different occupations where they have acquired the requisite skills from their previous roles, and for employers to consider workers with experience outside the advertised vacancies’ roles.
A key part of the matching system is a skills repository. This is a public database, based on 4 million job vacancies and co‑operation with employer organisations. It features 532 detailed descriptions of jobs, listing the skills, knowledge required and working conditions. This database helps to suggest and tag skills based on a worker’s or vacancy’s occupation.
As in some other countries, such as Finland, Pôle Emploi’s tool automatically suggests skills and occupations to jobseekers based on their CVs and other information available in the jobseeker profile. Similar automation helps apply skills to job vacancies. The skills available include not only technical skills but 16 soft skills that can be added to a jobseeker’s profile. Jobseekers are then suggested vacancies where they match at least 50% of the vacancy’s skills, though they can also choose to search more broadly for vacancies (such as searching by occupation). Likewise, employers can see which jobseekers have a good match with their advertised vacancies or they can search more broadly for candidates on Pôle Emploi’s website, and even choose to receive alerts when new candidates register with the skills they are searching for.
Source: Duretz (2023[63]), Pôle emploi: Competency based job matching, Digital tools for matching jobseekers and vacancies: International workshop, www.oecd.org/els/emp/presentation_job_matching_France.pdf.
As the SEA plans to gradually introduce different skill sets in its skills profiling tools (see Section 6.9), the same competencies could be simultaneously introduced in the matching algorithm. Including accredited skills in the SEA’s job matching tool could further increase its performance. Recent evidence indicates that credibly assessing jobseeker skills and competencies and making these assessments available for jobseekers and potential employers can have significant positive effects on jobseekers’ job search success both in terms of employment and wage (Carranza et al., 2022[64]). Nevertheless, the SEA should aim to also include those key skills and competencies in its matching algorithm that are not yet implemented in the skills profiling tool or that will not be part of the skills profiling tool due to the nature of the skills, to ensure all relevant competencies would be covered and thus contribute to the overall performance of the tool.
It is important to cover the key competencies and skills in a job matching tool systematically to avoid biased and thus unusable matching results. Although algorithms to map competencies without specific skill taxonomies have been proposed in computer sciences (e.g. Schlippe and Bothmer (2023[65])), competency-based job matching tools in PES aim to ensure systematic approaches by using dedicated skills taxonomies like ESCO, O*NET or ROME (Répertoire Opérationnel des Métiers et des Emplois). PES in the EU are increasingly using the ESCO classification as the main skills taxonomy due to the obligation to use this classification also for the job mediation across EU countries (European Employment Services job mediation EURES). As such, the SEA should link the competencies to be introduced in its job matching algorithm to the ESCO taxonomy, including those competencies that are being mapped in the skills profiling tool.
6.10.3. User experience and effectiveness of the SEA’s job matching tool could be further enhanced by AI
Similarly to the SEA, other PES have faced difficulties in adopting the ESCO taxonomy within their digital solutions in a user-friendly manner. As the ESCO taxonomy is complex, jobseekers and employers have difficulties in selecting the relevant competencies, leading to errors in the selections or missing the competencies altogether.
To overcome the difficulties in using the ESCO or other similar taxonomies, PES are increasingly harnessing AI algorithms to facilitate users to quickly identify the relevant competencies or even identify the competencies for them. Such an approach can increase user-friendliness of the process to identify skills, as well as ensure a more comprehensive skill detection by supporting jobseekers to identify skills they had not realised they possessed. For example, the Luxembourg PES has developed an algorithm using Natural Language Processing (NLP) to automatically classify vacancies according to the ESCO taxonomy, that is currently applied for statistical purposes, but will be used in job matching algorithms in the future ( (Baer, 2023[66]), Box 6.8). The NLP-based algorithm in the online platform Job Market in Finland is already extracting competencies from CVs, job application documents and vacancy texts using the ESCO taxonomy (Job Market Finland, 2023[67]; Hirsimäki, 2023[60]). Also the Israeli PES is using AI in a similar fashion, i.e. to extract competencies according to the ESCO taxonomy using jobseeker CVs as inputs (Brioscu et al., Forthcoming[5]). The Estonian PES is testing how to facilitate using ESCO taxonomy for the job matching tool by harnessing ChatGPT and thus aiming to avoid developing a new NLP-based algorithm. While the latter approach is not likely to have as high performance and integrated solution as in the aforementioned countries, it can be a lot less costly way to improve the job matching tool.
Box 6.8. Luxembourg is developing a new matching tool
Luxembourg is in the process of developing a new tool to support the Luxembourg public employment service (PES), ADEM, to match jobseekers with vacancies. Currently, ADEM uses a tool which is only available for counsellors to use and is not available to jobseekers or employers. The current tool is also a simple rules-based tool and does not use any artificial intelligence (AI) or machine learning or support matching of jobseekers to training.
The new tool will offer many improvements. It will be available to both counsellors and jobseekers, which could increase convenience for jobseekers while also potentially saving time for counsellors. In fact, the tool will be available to all jobseekers, not only those that are registered with ADEM, further increasing the tool’s value. The tool will offer skill-based matching and make use of AI to help identify and tag the skills of both vacancies and jobseekers and encode these skills to the European Skills, Competencies, Qualifications, and Occupations (ESCO) classification. The AI uses different Natural Language Processing (NLP) approaches to detect skills in vacancies and jobseekers’ profiles. In addition to matching jobseekers and vacancies, the tool will also support matching of jobseekers to training that may be beneficial for them.
To support the tool’s, development ADEM is leveraging the expertise and experiences of others, noting the importance of working with other countries and outside providers. ADEM is working with the Flanders’ PES, VDAB, through the support of the EU PES Network’s Mutual Assistance Project, to bring in the perspectives of a PES with an advanced matching tool. To develop the tool, some work is done in-house, with one data scientist working part time on skills encoding. However, Luxembourg will contract out the development of the tool itself to bring in the additionally necessary expertise.
While the new tool is still in the development and planning process, ADEM has already learned many important lessons. They have already made progress with the skills tagging and note that the ESCO resources and metadata (with the detailed descriptions of each skill) have been very helpful. In just a few months they have found that the skills tagged by the algorithm are indeed accurate reflections of job vacancies, although they note that the algorithm does struggle to identify all the skills relevant to a vacancy, and most especially the algorithm misses less technical “generic” skills. To ensure the accuracy of the algorithm, ADEM invests in “in-depth” validation of the results, which ADEM notes is time consuming but crucial.
Source: Baer (2023[66]), Algorithms to facilitate job matching in Luxembourg, Digital tools for matching jobseekers and vacancies: international workshop, https://www.oecd.org/els/emp/presentation_job_matching_Lux.pdf.
AI algorithms can also be used to improve the performance of job matching tools in other ways, such as to improve matching quality. Above all, AI algorithms can better take into account jobseeker interests (including using the information on jobseeker behaviour while using the matching tool, i.e. click data) and the labour market dynamics, make the digital tools more intuitive, and thus make more tailored and personalised recommendations for both jobseekers and employers (Brioscu et al., Forthcoming[5]). As a result, job matching algorithms enhanced by AI can have more positive effects on the labour market (Barbanchon, Hensvik and Rathelot, 2023[56]; Alfonso-Naya et al., 2021[59]). AI has been introduced into the job matching algorithms for example by the PES in Belgium (Flanders, Box 6.9), Canada, Finland, Korea and Mexico.
Box 6.9. The Flemish public employment service, VDAB, uses several sophisticated tools to support matching
The public employment service of Flanders, VDAB, invests heavily in digitisation, machine learning and artificial intelligence (AI). Indeed, VDAB has an “innovation lab” of 30 people in 2022 including Data Scientists, Data Engineers, Project Managers, Business Solutions Professionals and “DevOps” employees. Such investment has yielded several sophisticated tools to support matching jobseekers with vacancies.
VDAB has developed Jobbereik (job reach) and Talent API to match jobseekers and vacancies. Jobbereik includes a graphical interface to visualise how well the skills of a jobseeker map to specific occupations. The tool is available to both jobseekers and their counsellors. Talent API is a job matching tool that recommends vacancies to jobseekers using sophisticated AI that analyses vacancies, jobseeker profiles (location, previous work experience and skills), and even past vacancy-browsing on the website to find the most relevant vacancies for a jobseeker. As described in Section 6.9.2. VDAB also uses another tool, Orient, to understand jobseekers’ preferences and map these to occupations and corresponding vacancies.
The above‑mentioned digital tools for jobseekers and counsellors are in turn supported by background tools that code vacancies and job profiles with occupations and skills in a consistent way. Such tools include the “competency-extractor” to tag competencies from CVs and vacancies and the “occupation finder” to code correctly the occupation of a vacancy. These tools make use of sophisticated classification for skills (The Competent Standards) and occupations (the VDAB Skills framework).
Source: OECD (2021[30]), New ways to reach out to employers, http://oecd.org/els/emp/Employers.pdf; VDAB (2021[23]), AI & Advanced Analytics @ VDAB, https://web-archive.oecd.org/2020-11-24/570855-AI_presentation_VDAB.pdf; Broecke (2023[68]), Artificial intelligence and labour market matching, https://doi.org/10.1787/2b440821‑en; Brioscu, Lauringson, Saint-Martin and Xenogiani (Forthcoming[5]), “New dawn for public employment services: Service delivery in the age of artificial intelligence”.
However, introducing AI into matching algorithms means that the design, testing and monitoring of the job matching tool needs to be even more careful and thorough. AI use can further increase risks associated with digital tools in general, such as issues concerning data protection, potential biases and discrimination, trustworthiness, transparency, caseworker oversight and ethicality (OECD, 2022[69]; Allhutter et al., 2020[70]). For example, the PES in Belgium (Flanders) has used an AI-based job matching tool and another job matching tool without the AI components simultaneously, to give jobseekers the opportunity to choose between the enhanced tool and the simpler and transparent tool. Furthermore, the design of job matching tools needs to specifically consider managing the risks of distorting labour market functioning, increasing the competition between jobseekers, and locking certain groups in the society into lower quality jobs (OECD, forthcoming[4]).
Enhancing the SEA’s job matching tool with AI technology could address several of the issues associated with the current solution, such as helping to strengthen competency-based matching and fully integrate ESCO taxonomy, perform higher quality and more personalised matches, provide better overview of possible career choices for the jobseekers, as well as make the tool more intuitive and user-friendly. Nevertheless, developing more advanced technologies can be time‑consuming and costly, while some advancements in the performance of the job matching tool could be achieved without AI adoption. In the long-run, the SEA should aim at adopting an AI-based job matching tool that uses competencies, jobseeker interests and labour market information to match jobseekers and vacancies, potentially in co‑operation with other PES to avoid duplicating efforts and use the resources efficiently. Smaller adaptions to increase the tool performance could be done already in the near future, such as allocating more server capacity to increase the speed of providing matching results, adding key competencies in the matching algorithm, displaying full matches and near matches for the users in a comprehensible way, as well as enhancing user experience within the tool design more generally.
6.10.4. The vacancy posting process could be streamlined and automated
The SEA already achieves good vacancy coverage indicating no major problems in the share of vacancies collected. Indeed, about 80% of vacancies posted in Latvia are available through the SEA’s CVVP platform (see Chapter 4). To increase the number of vacancies to mediate to the jobseekers, some PES (such as in the Netherlands and Austria see Box 4.1 in Chapter 4) collect vacancies using techniques like web scrapping and automatic connections (APIs) to other vacancy databases. The SEA could consider such techniques, particularly APIs and agreements with other large vacancy websites, which are likely easier to implement than web scraping techniques. However, given the SEA already reports large coverage of vacancies, such tools may not be necessary or worth the cost and so are a lower priority than other investments.
Some other improvements to streamline the collection of vacancy postings may, however, be warranted. Notably the uploading of vacancies in the CVVP portal is often a manual process, with many vacancies submitted via e‑mail or telephone and then manually added to BURVIS by counsellors. The SEA reports that this is often the preference of employers rather than employers directly submitting them through the CVVP. Indeed, regular contact between counsellors and employers is positive and can help foster good relations, which are crucial for a PES. However, these manual processes naturally take counsellor’s time, and such time could be put to other uses (including fostering good relations with employers in other ways). Moreover, some advanced systems in other countries (such as in the French PES) rely on automatic processing and tagging of data as a first step to more sophisticated algorithms.
The SEA should continue to provide manual uploading of vacancies as a valuable service to those employers who need it, but it should seek to better understand why employers are not uploading them through the CVVP themselves. If it is because employers are simply not aware of the online services available for them, they should be informed. Quite possibly it is because the interface is cumbersome, not well understood or otherwise not user friendly for employers. If this is the case, the SEA should identify the design issues and improve the CVVP for employers, so that they find it easier and more helpful to use. This can save employers’ and counsellors’ time while ensuring a consistent format for data uploading. Such consistent data helps support the matching process and search features within the CVVP and indeed would be helpful if more advanced features (such as automatic skills and competency tagging) was adopted as discussed in Sections 6.10.2 and 6.10.3 above.
6.11. Further possibilities to enhance the SEA services to jobseekers, people at risk of job loss and employers via digitalisation
The previous sections of this report focused on the overall digital infrastructure of the SEA and the key PES-specific digital tools – jobseeker profiling, skills profiling and job matching – to advise the SEA in modernising its digital backbone and thus better support the Latvian labour market.
Although not in the centre of attention of this report and a key priority for the SEA, the current chapter and Chapter 4 make a few references to some of the other PES-specific digital solutions that have been developed in other countries. These include for example digital tools for career management, e.g. recommender tools for job search, training options and career pathways going beyond matching the jobseeker with a specific vacancy but rather taking a more holistic approach. Other solutions help with the vacancy pool to mediate for the jobseekers including digital solutions that detect vacancies available in other platforms outside the SEA, as well as identifying employers with a potential for upcoming vacancies (i.e. before the vacancy is even advertised). Another set of digital tools can support employers more directly, for example by helping to redesign and reconstruct job postings to better identify and attract possible candidates, which can be particularly important for bottleneck occupations. Furthermore, PES in other countries are continuously looking for opportunities to facilitate their work processes and back-office tasks with advanced technologies that are not necessarily PES-specific in nature, such as AI-generated automatic email responses (see more in Brioscu et al. (Forthcoming[5])).
Many of the recent advanced digital solutions to increase PES effectiveness, efficiency and user-friendliness in other countries have required considerable capacity in-house to manage these modernisation projects and resources and skills to develop or outsource these developments. Although the SEA has relatively tight capacity and budget to undertake similar projects, some advancements could be more feasible in co‑operation with other PES, taking advantage of the recent fast developments in AI technologies (particularly in Large Language Models and generative AI), as well as for example fine‑tuning the user experience in the current solutions and feasible technologies.
In conclusion, the SEA should focus on the recommendations made in the current report as a priority for modernising its digital backbone. The key first steps of the modernisation will be developing a comprehensive digitalisation strategy to establish the objectives and relevant frameworks, implement the more feasible fine‑tuning related to the operational IT system, start the development of an advanced data analytics system consisting of a data warehouse and a BI tool, gradually improve the design and implementation of the jobseeker profiling tool, continue co‑operating with other organisations to find feasible solutions for skills profiling, and move towards competency-based job matching. Additional improvements and new digital tools learning from the recent good examples of other countries discussed above could be considered for the future and addressed in the digitalisation strategy accordingly.
References
[59] Alfonso-Naya, V. et al. (2021), “Designing labor market recommender systems: the importance of job seeker preferences and competition”, Working Paper.
[41] All Digital (2021), mydigiskills, http://mydigiskills.eu/ (accessed on 26 February 2023).
[70] Allhutter, D. et al. (2020), “Algorithmic Profiling of Job Seekers in Austria: How Austerity Politics Are Made Effective”, Frontiers in Big Data, Vol. 3/5.
[58] Altmann, S. et al. (2022), “The Direct and Indirect Effects of Online Job Search Advice”, IZA Discussion Papers 15830, Institute of Labor Economics (IZA).
[27] AMS (2023), Berufsinfomat, https://www.ams.at/arbeitsuchende/aus-und-weiterbildung/berufsinformationen/berufsinformation/berufsinfomat?open=berufsinfomat (accessed on 4 January 2024).
[26] AMS (2022), Berufskompass, AMS, http://berufskompass.at/berufskompass/ (accessed on 26 February 2023).
[18] Andonovikj, V. et al. (2024), “Survival analysis as semi-supervised multi-target regression for time-to-employment prediction using oblique predictive clustering trees”, Expert Systems with Applications, Vol. 235, p. 121246, https://doi.org/10.1016/j.eswa.2023.121246.
[24] Arbetsförmedlingen (2023), Arbetsförmedlingens nya modell för bedömning av arbetssökandes stödbehov [Arbetsförmedlingen’s new model for the assessment of jobseekers’ support needs], https://arbetsformedlingen.se/download/18.6546760718a1c207b931ed0/1694504706728/arbetsformedlingens-nya-bedomningsmodell.pdf.
[22] Bach, R. et al. (2023), “The impact of modeling decisions in statistical profiling”, Data & Policy, Vol. 5, https://doi.org/10.1017/dap.2023.29.
[66] Baer, I. (2023), Algorithms to facilitate job matching in Luxembourg, Digital tools for matching jobseekers and vacancies: international workshop, https://www.oecd.org/els/emp/presentation_job_matching_Lux.pdf.
[56] Barbanchon, T., L. Hensvik and R. Rathelot (2023), “How can AI improve search and matching? Evidence from 59 million personalized job recommendations”, Working paper, http://rolandrathelot.com/wp-content/uploads/LeBarbanchon_Hensvik_Rathelot_Jobbtips_20230722.pdf.
[57] Behaghel, L. et al. (2022), “Encouraging and Directing Job Search: Direct and Spillover Effects in a Large Scale Experiment”, Banque de France Working Papers, https://publications.banque-france.fr/sites/default/files/medias/documents/wp900.pdf.
[61] Belot, M., P. Kircher and P. Muller (2022), Do the Long-Term Unemployed Benefit from Automated Occupational Advice during Online Job Search?, Institute of Labor Economics (IZA), Bonn, http://hdl.handle.net/10419/263668.
[55] Belot, M., P. Kircher and P. Muller (2022), “How Wage Announcements Affect Job Search— A Field Experiment”, American Economic Journal: Macroeconomics, Vol. 14/4, pp. 1-67, https://doi.org/10.1257/mac.20200116.
[16] Bodilsen, S., S. Albeck Nielsen and M. Rosholm (2023), “Measuring Employment Readiness for Hard-to-Place Individuals”, SSRN Electronic Journal, https://doi.org/10.2139/ssrn.4646348.
[19] Boškoski, P. et al. (2021), “Variational Bayes survival analysis for unemployment modelling”, Knowledge-Based Systems, Vol. 229, p. 107335, https://doi.org/10.1016/j.knosys.2021.107335.
[5] Brioscu, A. et al. (Forthcoming), “New dawn for public employment services: Service delivery in the age of artificial intelligence”.
[68] Broecke, S. (2023), “Artificial intelligence and labour market matching”, OECD Social, Employment and Migration Working Papers, No. 284, OECD Publishing, Paris, https://doi.org/10.1787/2b440821-en.
[38] Bundesagentur für Arbeit (2022), Möglichkeiten testen [test possibilities], http://web.arbeitsagentur.de/sete/testuebersicht/ (accessed on 26 February 2023).
[64] Carranza, E. et al. (2022), “Job Search and Hiring with Limited Information about Workseekers’ Skills”, American Economic Review, https://doi.org/10.1257/aer.20200961.
[62] Charleer, S., F. Gutiérrez and K. Verbert (2019), Supporting Job Mediator and Job Seeker through an Actionable Dashboard.
[2] Crépon, B. et al. (2013), “Do Labor Market Policies have Displacement Effects? Evidence from a Clustered Randomized Experiment *”, The Quarterly Journal of Economics, Vol. 128/2, pp. 531-580, https://doi.org/10.1093/qje/qjt001.
[21] Desiere, S. and L. Struyven (2020), “Using Artificial Intelligence to classify Jobseekers: The Accuracy-Equity Trade-off”, Journal of Social Policy, Vol. 50/2, pp. 367-385, https://doi.org/10.1017/s0047279420000203.
[13] DEWR (2021), Online Job Seeker Classification Instrument Trial Evaluation Report, https://www.dewr.gov.au/download/11093/online-job-seeker-classification-instrument-trial-evaluation-report/21320/document/pdf.
[63] Duretz, B. (2023), Pôle emploi: Competency based job matching, Digital tools for matching jobseekers and vacancies: international workshop, https://www.oecd.org/els/emp/presentation_job_matching_France.pdf.
[28] European Commission (2023), EU Skills profiling tool for third country nationals, http://ec.europa.eu/social/main.jsp?catId=1412&langId=en (accessed on 26 February 2023).
[42] European Commission (2023), Test your digital skills!, https://europa.eu/europass/digitalskills (accessed on 26 February 2023).
[37] European Commission (2021), New Plan: A self-assessment tool for orientation and counselling, European Commission, https://ec.europa.eu/social/BlobServlet?docId=24836&langId=en.
[29] EVC (2023), EVC, http://nationaal-kenniscentrum-evc.nl (accessed on 6 February 2023).
[12] Filges, T. and A. Hansen (2017), “The Threat Effect Of Active Labor Market Programs: A Systematic Review”, Journal of Economic Surveys, Vol. 31/1, pp. 58-78.
[20] Gabrikova, B., L. Svabova and K. Kramarova (2023), “Machine Learning Ensemble Modelling for Predicting Unemployment Duration”, Applied Sciences, Vol. 13/18, p. 10146, https://doi.org/10.3390/app131810146.
[17] Gallagher, P. and R. Griffin (2023), “(in) Accuracy in Algorithmic Profiling of the Unemployed – An Exploratory Review of Reporting Standards”, Social Policy and Society, pp. 1-14, https://doi.org/10.1017/s1474746423000428.
[3] Glover, D. (2019), “Job Search and Intermediation under Discrimination: Evidence from Terrorist Attacks in France”, Chaire Securisation des Parcours Professionels Working Paper, https://www.chaire-securisation.fr/SharedFiles/48_Glover2019.pdf.
[60] Hirsimäki, T. (2023), A platform for job matching using AI: Job Market Finland, Digital tools for matching jobseekers and vacancies: international workshop, https://www.oecd.org/els/emp/presentation_job_matching_Finland.pdf.
[54] Horton, J. (2017), “The Effects of Algorithmic Labor Market Recommendations: Evidence from a Field Experiment”, Journal of Labor Economics, Vol. 35/2, pp. 345-385, https://doi.org/10.1086/689213.
[43] Ikanos (2023), Ikanos, http://ikanos.eus (accessed on 26 February 2023).
[67] Job Market Finland (2023), How the matching works, https://tyomarkkinatori.fi/en/info/instructions-and-frequently-asked-questions/how-the-matching-works.
[50] Kircher, P. (2020), “Search design and online job search – new avenues for applied and experimental research”, Labour Economics, Vol. 64, p. 101820, https://doi.org/10.1016/j.labeco.2020.101820.
[14] Langenbucher, K. and M. Vodopivec (2022), “Paying for results: Contracting out employment services through outcome-based payment schemes in OECD countries”, OECD Social, Employment and Migration Working Papers, No. 267, OECD Publishing, Paris, https://doi.org/10.1787/c6392a59-en.
[36] O*NET (2023), O*NET Online, http://www.onetonline.org/ (accessed on 26 February 2023).
[51] OECD (2024), Impact Evaluation of Training and Wage Subsidies for the Unemployed in Greece, Connecting People with Jobs, OECD Publishing, Paris, https://doi.org/10.1787/4b908517-en.
[48] OECD (2023), A Personalised Skills Assessment Tool: Education and Skills Online Version 2. Information webinar on 14 December 2023..
[7] OECD (2023), Addressing the legal and IT challenges of data exchange to support contracted-out employment services in Sweden, https://www.oecd.org/els/emp/Sweden_Challenges_Data_Exchange.pdf.
[46] OECD (2023), Education & Skills Online Assessment, https://www.oecd.org/skills/ESonline-assessment/abouteducationskillsonline/.
[34] OECD (2023), Evaluation of Active Labour Market Policies in Finland, Connecting People with Jobs, OECD Publishing, Paris, https://doi.org/10.1787/115b186e-en.
[69] OECD (2022), Harnessing digitalisation in Public Employment Services to connect people with jobs, OECD Publishing, Paris, https://www.oecd.org/els/emp/Harnessing_digitalisation_in_Public_Employment_Services_to_connect_people_with_jobs.pdf.
[8] OECD (2022), Impact evaluation of the digital tool for employment counsellors in Spain: SEND@, OECD Publishing, Paris, https://www.oecd.org/els/emp/FinalReport-EvaluationOfSEND.pdf.
[35] OECD (2022), The OECD Skills profiling tool, http://oecd-skillsprofilingtool.org/home (accessed on 26 February 2023).
[33] OECD (2021), Improving the Provision of Active Labour Market Policies in Estonia, Connecting People with Jobs, OECD Publishing, Paris, https://doi.org/10.1787/31f72c5b-en.
[30] OECD (2021), New ways to reach out to employers, OECD, http://oecd.org/els/emp/Employers.pdf.
[32] OECD (2021), OECD Employment Outlook 2021: Navigating the COVID-19 Crisis and Recovery, OECD Publishing, https://doi.org/10.1787/5a700c4b-en.
[25] OECD (2020), Statistical profiling tools for effective targeting and tailoring of services to jobseekers in Slovenia – Workshop Summary, https://www.oecd.org/els/emp/Statistical_profiling_Slovenia.pdf.
[40] OECD (2020), “The potential of online learning for adults: Early lessons from the COVID-19 crisis”, OECD Policy Responses to Coronavirus (COVID-19), OECD Publishing, Paris, https://doi.org/10.1787/ee040002-en.
[47] OECD (2019), Strengthening Active Labour Market Policies in Italy, Connecting People with Jobs, OECD Publishing, Paris, https://doi.org/10.1787/160a3c28-en.
[4] OECD (forthcoming), Handbook on labour market transformations, Edward Elgar.
[6] OECD (forthcoming), Strengthening active labour market policies in Korea.
[39] Pix (2023), Pix, http://pix.fr (accessed on 26 February 2023).
[9] Pôle Emploi (2022), Pôle emploi se dote d’une charte pour une utilisation éthique de l’intelligence artificielle, Communiqué de presse, https://www.pole-emploi.org/accueil/communiques/pole-emploi-se-dote-dune-charte-pour-une-utilisation-ethique-de-lintelligence-artificielle.html?type=article.
[49] Press, T. (ed.) (2000), Equilibrium Unemployment Theory, Second Edition, MIT Press Books.
[45] Radix (2020), Radix and VDAB reinvent orientation test with Artificial Intelligence, Radix, http://radix.ai/cases/flemish-orientation-test-faster-and-more-accessible-than-ever-thanks-to-collaboration-between-radix-and-vdab/ (accessed on 26 February 2023).
[52] Räisänen, H. (2023), How to approach the ex ante employment effects of the Job Market matching platform?, https://www.oecd.org/els/emp/Session3_Job_Market_Matching_Platform.pdf.
[53] Räisänen, H. (2022), “Miten Työmarkkinatorin työllisyysvaikutusta voidaan arvioida?”, Työpoliittinen aikakauskirja, Vol. 1, https://julkaisut.valtioneuvosto.fi/bitstream/handle/10024/163904/TAK_1_2022_web.pdf?sequence=1&isAllowed=y.
[11] Scheerlinck, K. (2023), Ethical Board of VDAB and its role in AI Governance, https://www.oecd-events.org/ai-wips-2023/session/8d837eb4-cbbc-ed11-9f73-6045bd8890e4.
[10] Scheerlinck, K. (2020), AI & Advanced Analytics @ VDAB, https://www.oecd.org/els/emp/AI_presentation_VDAB.pdf.
[1] Schiprowski, A. (2020), “The Role of Caseworkers in Unemployment Insurance: Evidence from Unplanned Absences”, Journal of Labor Economics, Vol. 38/4, pp. 1189-1225, https://doi.org/10.1086/706092.
[65] Schlippe, T. and K. Bothmer (2023), “An AI-Based Recommendation System for Employers, Job Seekers and Educational Institutions”, International Journal of Advanced Corporate Learning, Vol. 16/1, https://doi.org/10.3991/ijac.v16i1.34779.
[31] The Danish Agency for Labour Market and Recruitment (2021), The skills tool, http://oecd.org/els/emp/PES-Digital-Oct2021-Denmark.pdf.
[44] VDAB (2023), Orient, http://orientatie.vdab.be/ (accessed on 26 February 2023).
[23] VDAB (2021), AI & Advanced Analytics @ VDAB, International Online Workshop: Introducing New Digital Tools for PES Counsellors, https://web-archive.oecd.org/2020-11-24/570855-AI_presentation_VDAB.pdf.
[15] Wijnhoven, M. et al. (2023), “The Work Profiler: Revision and maintenance of a profiling tool for the recently unemployed in the Netherlands”, International Social Security Review, Vol. 76/2, pp. 109-134, https://doi.org/10.1111/issr.12327.
Annex 6.A. Examples of profiling questions
Annex Box 6.A.1. The new profiling tool used by the Dutch PES included 18 factors
Questions included in the “Work Profiler 2.0” used by UWV
1. Age
(up to 25 years, 25‑40, 40‑60, 60+)
2. Years employed in last job
0‑0.5 year
0.5‑1 year
1‑2 years
2‑5 years
5‑10 years
10‑50 years
3. Views on return to work
(Eager to return, hesitant, uncertain)
4. Desired profession
Economic-administrative (reference category)
Agricultural
Computer
(Para)medical
Public order and safety
Educational
Socio-cultural
Technical and industrial
Transportation
Care and service
5. Position within the household
Lives alone (reference category)
Married/lives together, no children living under the same roof
Male married/lives together, youngest child under the same roof is 0‑6 years old
Female married/lives together, youngest child under the same roof is 0‑6 years old
Married/lives together, youngest child under the same roof is ≥ 7 years
Single parent
Other
6. Maximum duration of unemployment benefits
Longer than 12 months: no (reference category)
Longer than 12 months: yes
7. Industry prior to unemployment benefit
Finance (reference category)
Construction
Health
Industry
Agriculture, fishing, food
Other
Other companies and other professions
Transportation
Employment agencies
Stores and wholesale
8. Job search behaviour concerning direct contact with potential employers
(Regularly contacts employers, seldom contacts employers)
9. Perceived health
(Good, fair, poor)
10. Balance of the advantages and disadvantages of not-working
As many advantages as disadvantages (reference category)
More disadvantages than advantages
More advantages than disadvantages
11. WIA 35‑min (disability assessment)
No
Yes
12. Problems understanding Dutch
No problems
Some problems
A lot of problems/does not understand Dutch
13. Readiness to accept a fulltime job
(Ready, not ready, conditional)
14. General work ability
(High, moderate, low)
15. Number of hours per week capable of work
(20 hours, 40 hours, 60 hours)
16. Level of education
Low
Middle
High
17. Readiness to get work with irregular working hours
(Willing, unwilling, conditional)
18. Income from work besides the unemployment benefit
No
Yes
Source: Wijnhoven et al. (2023[15]), The Work Profiler: Revision and maintenance of a profiling tool for the recently unemployed in the Netherlands, https://doi.org/10.1111/issr.12327.
Annex Box 6.A.2. A Danish profiling tool incorporates questions for both jobseekers and counsellors
Questions in the Employment Readiness Indicator Questionnaire(ERIQ)
Questions to Clients
1. Are you aware of what type of work you would like to perform?
2. How do you feel about initiating contact with people whom you do not know?
3. How good are you at collaborating with others?
4. Do you have the support of family and friends when you need help?
5. Do you have the personal energy in your everyday life to focus on getting a job?
6. In general, how would you rate your (physical and mental) health in terms of being able to hold a job?
7. Do you think your skills can be used in a workplace?
8. Do you think you are able to carry out work at a workplace?
9. Do you know what to do in order to improve your job opportunities?
10. How do you search for a job?
Questions to Caseworkers
1. Does the client have a realistic understanding of where in the labour market, his/her competencies can be applied?
2. To what degree does the client act with determination in terms of obtaining a job?
3. How do you assess the client’s ability to seek and initiate dialogue with others?
4. How good is the client in discussing about him/herself and his/her relevant competencies?
5. How do you assess the client’s ability to co‑operate with others?
6. How do you assess the client’s ability to receive and understand instructions about a task?
7. How do you assess the client’s ability to concentrate on a task without being distracted?
8. To what extent does the client have a social network that provides support for entering the labour market?
9. To what extent is the client able to master his/her own life at the same time as focusing on obtaining a job?
10. To what extent is the client able to master any (physical and mental) health problems?
11. Do you believe that the client will get a job within the next year?
Source: Bodilsen, Albeck Nielsen and Rosholm (2023[16]), “Measuring employment readiness for hard-to-place individuals,”
https://doi.org/10.2139/ssrn.4646348.
Notes
← 1. Penetration testing is a process in which a system is hacked on purpose – an actor is attempting to penetrate through the defences of the system. This is often done as automated tasks, complemented with expertise of specialists of the security field. It is used to find potential weaknesses in the application that malicious actors could exploit. If such weaknesses are found in penetration testing, they can be fixed before a malicious actor can break in.
← 2. A storage system of programming code that allows for versioning and multiple developers working together on the same code.
← 3. For example, a source code pull request can have a gate where the pull request is denied if there are any high impact vulnerabilities found (for example vulnerabilities known to allow attackers access to system data). This means that a developer cannot contribute code that is known to have a high-risk vulnerability but is required to fix the issue, before the code is allowed. Another gate could be release to production, stating, that there cannot be any medium or high impact vulnerabilities. This would prevent a malicious actor that has somehow gained access to source control, from implementing a vulnerability into production.