This chapter estimates the non-exhaust PM emission factors from electric vehicles and compares these factors with those of internal combustion engine vehicles. Assuming lightweight EVs (i.e. with battery packs enabling a driving range of about 100 miles), the report finds that EVs emit an estimated 11-13% less non-exhaust PM2.5 and 18-19% less PM10 than ICEVs. Assuming that EV models are heavier (with battery packs enabling a driving range of 300 miles or higher), however, the report finds that they reduce PM10 by only 4-7% and increase PM2.5 by 3-8% relative to conventional vehicles. Additional simulations indicate that the uptake of electric vehicles will lead to very marginal decreases in total PM emissions from road traffic in future years. In scenarios where electric vehicles comprise 4% and 8% of the vehicle stock in 2030, their penetration reduces PM emissions by 0.3%-0.8% relative to current levels.
Non-exhaust Particulate Emissions from Road Transport
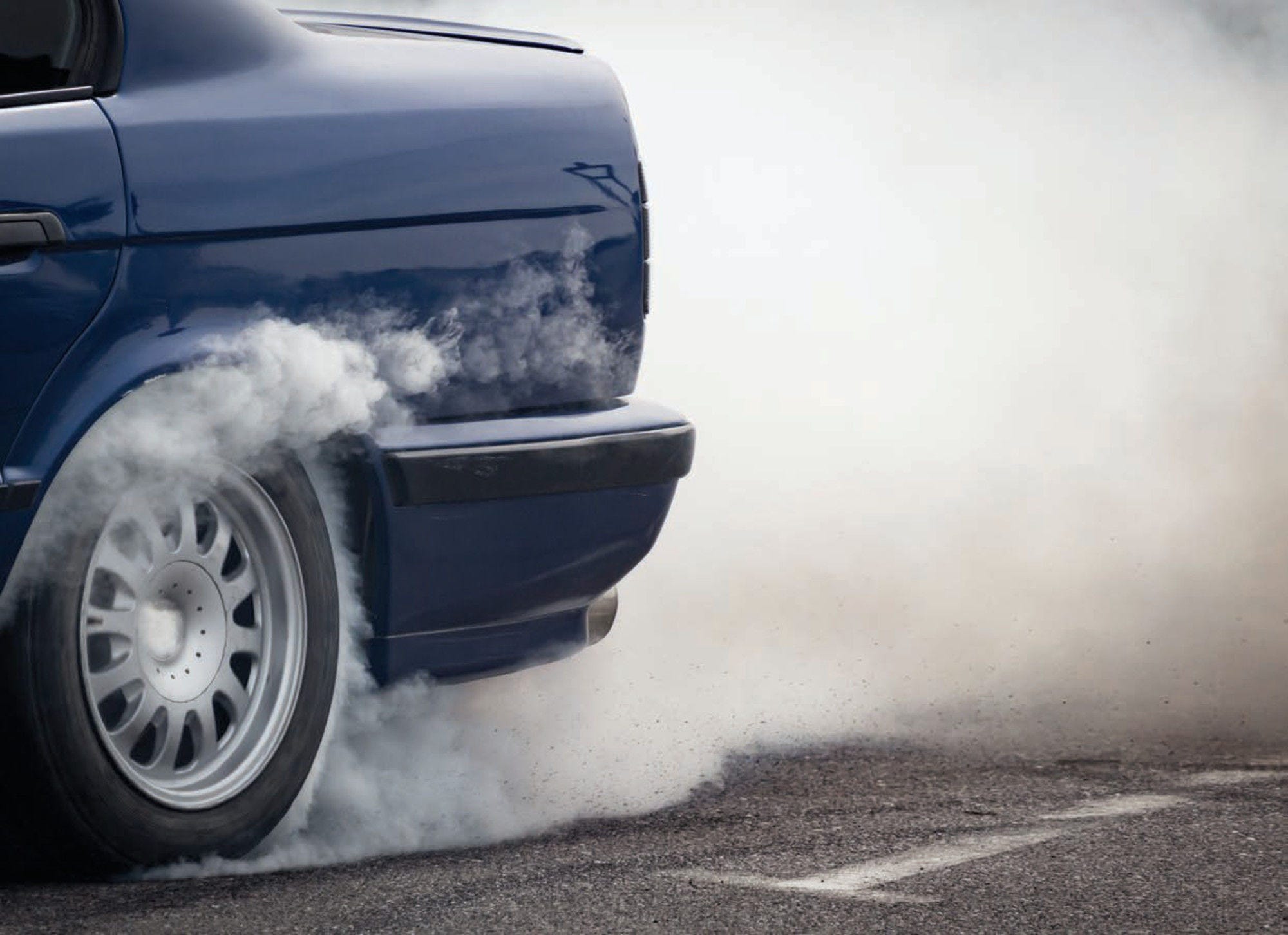
3. The implications of electric vehicle uptake for non-exhaust emissions
Abstract
The direct and indirect implications of vehicular emissions on the environment and human health indicate that current transportation systems based on the use of conventional vehicles are unsustainable from social, environmental and economic perspectives. Electric vehicles are widely regarded as a solution to many of the negative impacts of their conventional counterparts. Given their potential to reduce local air pollution and greenhouse gas emissions, consumers, businesses, and governments are increasingly supportive of electric vehicles (Requia et al., 2018[1]), which has led to rising shares of new vehicle sales around the world.
According to the IEA Global EV Outlook 2018 (IEA, 2018[2]), sales of new electric vehicles, including battery electric vehicles (BEVs), plug-in hybrid electric vehicles (PHEVs) and fuel-cell electric vehicles (FCEVs) passed 1 million units in 2017, 54% more than in 2016. More than half of new electric vehicles were sold in China, where their market share amounted to 2.2% in 2017. Twice as many cars were sold in China as in the United States, the second-largest electric car market.
Growth in the EV market has been driven by technological improvements that improve performance and reduce costs, as well as by policy support. To the extent that technological improvements are widely available, differences in EV uptake across markets is best explained by differing degrees of policy support in place in these markets. The largest EV markets by volume (China) and sales share (Norway), for example, are both characterised by highly supportive policy environments. This is true for light-duty vehicles (LDVs) as well as for buses and two-wheelers.
Looking to the future, strong policy signals in favour of EVs include electric car mandates in China and California, as well as the European Union’s recent proposal on carbon dioxide emissions standards for 2030 (IEA, 2018[3]). Electrification targets announced by a number of countries and major cities worldwide also point to continued growth in EV uptake in the coming years. The IEA (2018[3]) foresees two possible scenarios of EV penetration worldwide. In the New Policies Scenario, which takes into account existing and announced policies, the number of electric light-duty vehicles on the road reaches 125 million by 2030. Should policy ambitions continue to rise to meet climate goals and other sustainability targets, as in the EV30@30 Scenario, then the number of electric LDVs on the road could be as high as 220 million in 2030, consisting of 130 million battery electric and 90 million plug-in hybrid vehicles, respectively.
3.1. Evidence on the impact of electric vehicle use on particulate emissions
3.1.1. Impacts on particulate emissions levels
Since BEVs do not emit tailpipe emissions, the adoption of these vehicles is generally viewed as a highly effective measure for improving air quality. Although increasing the market share of EVs is an important part of achieving environmental goals, their implications for non-exhaust emissions remain less well-understood than their implications for exhaust emissions.
In a recent review, Requia et al. (2018[1]) assessed 4734 studies on the impact of a shift to greater EV use. Of the 65 studies that fulfilled the inclusion criteria for the review, the authors concluded that while the benefits of EVs with respect to exhaust emissions of a number of air pollutants are well-established, less evidence exists regarding the impact of EV use on PM emissions: these impacts appear to be particularly dependent on context.1 Since Requia et al. (2018[1]), PM10 and PM2.5 emissions have been studied in 11 papers 16 papers, respectively. Some studies find that EVs offer moderate potential for reductions in PM emissions.2
In Ireland, Alam et al., (2018[4]) addressed the co-benefits of climate change mitigation policies to reduce the air pollution (PM2.5) and climate change (CO2) impacts of passenger cars, using a scenario-based approach, disaggregating road traffic PM2.5 in exhaust and brake, tyre and road abrasion. The results revealed that CO2 emissions continuously decreased in the projection period, however, reductions of PM2.5 reversed from the year 2028 due to increases in the non-exhaust component of PM2.5 emissions. Under the two alternative scenarios, a 9-15% reduction in PM2.5 could be achieved by 2035. The analysis suggests that non-exhaust PM2.5 was found to have a larger share of total emissions (as much as 34 times that of exhaust emissions) in 2035 in a scenario in which passenger cars with alternative drivetrains comprised a major part of the vehicle fleet. In the Yangtze River Delta region in China, Ke et al. (2017[5]) estimated that a scenario with 20% of private light-duty passenger vehicles and 80% commercial passenger vehicles electrified with BEV could reduce average total PM2.5 concentrations by 0.4 to 1.1 μg/m3.
3.1.2. Determinants of PM emissions impacts
Vehicle weight
Heavier vehicles require greater amounts of energy for acceleration and deceleration, implying greater wear rate of brakes and tyres (Carslaw, 2006[6]). The recent UK government Survey “Call for Evidence on non-exhaust emissions” acknowledged that data on tyre wear from EVs was lacking or not publically available. Nonetheless, many respondents assumed that increased EV weight would lead to increased tyre wear, and possibly higher particulate emissions, although no direct evidence of this was provided.
Van Zeebroek and De Ceuster (2013[7]) compared the weight (mass in running order) of 20 battery electric passenger cars to the average weight of conventional vehicles in the same vehicle segment, finding that EVs are 22% heavier than the average of their market segments. However, comparing EVs to the average passenger car in a category is complicated by a number of issues (see also Timmers and Achten (2018[8])). First, there are various classification systems available. This study used the EURO market segment classification, but vehicles can also be classified using the US EPA Size Class or EURO NCAP Class, which would produce different results. Second, EVs do not always fit well into a specific class. Finally, and most importantly, the vehicles in any vehicle class will vary significantly even within the class. They will have a range of dimensions, engine sizes, and features that renders isolating the impact of weight differences on PM emissions problematic.
Another method of comparing the weight of EVs to ICEVs is by comparing EVs with their equivalent conventional models. This avoids classification problems and ensures that the vehicles are as similar as possible. Timmers and Achten (2016[9]) used this method to compare the mass in running order of nine EVs and their ICEV counterparts. On average, they found the difference in weight to be 24% and assume that road and tyre wear increase linearly with vehicle weight.
The most appropriate comparison would be between vehicles that share identical specifications in all respects except for their drivetrains. However, EVs and ICEVs often have different specifications in terms of materials used, range, top speed, trailer load, types of tyres, and brakes, among others. Moreover, the increased weight due to the addition of battery pack depends on a number of characteristics (battery density, efficiency and km range) which vary from one segment to another and are also expected to evolve in the future. Finally, there is no evidence that two vehicles with the same size and shape but different weights have different emission factors for road dust resuspension.
Hooftman et al. (2016[10]) assumed that BEVs had 10% higher emissions from tyre wear due to their increased weight and tyre type, but they do not specify the assumptions underlying this estimation. Other studies have assumed no difference in non-exhaust emissions between BEVs and ICEVs (Requia et al., 2018[1]; Soret, Guevara and Baldasano, 2014[11]; Huo et al., 2015[12]).
Regenerative braking
Regenerative braking systems (RBS) are energy recovery mechanisms that slow a vehicle by converting its kinetic energy into a form which can be either used immediately or stored until needed. In these systems, the electric motor uses the vehicle's momentum to recover energy that would be otherwise lost to the brake discs as heat. This contrasts with friction braking systems, where the excess kinetic energy is converted to heat by friction in the brakes. In addition to improving the overall efficiency of the vehicle, RBS can greatly extend the life of the braking system, as its parts do not wear as quickly.
On hybrid and electric vehicles, pressing the brake pedal has very different effects, as the strategy followed is mostly oriented to maximising the amount of energy recuperated through regenerative braking, i.e. using the electric circuits in reverse to transform kinetic energy into deceleration. The amount of regenerative energy that a system can produce is strictly related to the maximum power of the installed electric motor(s) and electronics, and to the capacity of the battery to receive the energy without degrading. Above a certain battery size, the energy of a braking event can be recovered, except in the case this happens with the battery still fully charged. It is therefore safe to assume, that fully electric vehicles have extremely low brake emissions (the pads are used mainly to keep the vehicle at a standstill). Hybrid vehicles also have relatively low brake wear emissions, proportional to the level of hybridisation (micro hybrids show practically no advantage from this point of view, since the added mass is likely to compensate for the reduced energy to be dissipated). Vice versa, for RBS-equipped vehicles with large batteries, vehicle mass has a low to negligible influence on brake wear.
Several estimates of the impact of RBS on brake wear PM emissions exist in literature. The Platform for Electro-Mobility (2016[13]) claims that RBS reduce brake wear by 25-50%. Van Zeebroek and De Ceuster (2013[7]) assume that regenerative braking should reduce the PM emissions associated with brake wear by 50%. Timmers and Achten (2016[9]) assume a zero brake wear emissions from RBS-equipped vehicles, and Barlow (2014[14]) also suggests that regenerative braking produces virtually no brake wear. Hooftman et al. (2016[10]) state that EVs require about two-thirds (66%) less braking activity than ICEVs due to RBS. Their analysis is based on the service times of brake pads on Teslas, BMW i3s, and Nissan Leafs, which demonstrates that on average, the brake pads of these EVs last roughly two-thirds longer than those on diesel/petrol vehicles. They note that this outweighs the additional wear due to the vehicle’s mass. Ligterink et al. (2014[15]) assume regenerative braking reduces wear by up to 95%. Del Duce et al. (2016[16]) report that brake wear emissions fall by 80% for EVs, based on a report by Althaus and Gauch (2010[17]). Nopmongcol et al. (2017[18]) estimated a 25% reduction of brake wear.
Road dust resuspension
Out of many studies evaluating the relative environmental burden of BEVs and ICEVs, only a handful have analysed PM10 (Kantor et al., 2010[19]; Huo et al., 2013[20]; Nichols, Kockelman and Reiter, 2015[21]; Huo et al., 2015[12]; Peng et al., 2018[22]; Nopmongcol et al., 2017[18]; Wu and Zhang, 2017[23]; Hooftman et al., 2016[10]; Timmers and Achten, 2016[9]), and of these, only six considered non-exhaust emissions. Road dust resuspension was included in only three studies. As evidenced in Section 2, road dust resuspension is currently understood to be the main source of non-exhaust PM10 emissions for road vehicles. This is confirmed both by emission inventories (Pachón et al., 2018[24]; Secretaria del Medio Ambiente de la Ciudad de Mexico, 2018[25]; DICTUC, 2007[26]; U.S. Environmental Protection Agency, 2019[27]), which consider primary particulates, as well as by source apportionment studies (Padoan and Amato, 2018[28]) which assess secondary PM from vehicle exhaust emissions.
Given its importance as a source of non-exhaust PM10 emissions, road dust resuspension should be taken into account in vehicle emissions assessments. As evidenced by the literature inventoried in this report, non-exhaust emissions from road dust resuspension have been neglected in most studies to date (Van Zeebroeck and De Ceuster, 2013[7]; Hooftman et al., 2016[10]; Requia et al., 2018[29]). An exception is Soret et al. (2014[11]), who include an emission factor for resuspension of 88 mg/vkm, adjusted for rainfall but invariant to vehicle class. Timmers and Achten (2016[9]) have also corrected a base road dust EF of 40 mg/vkm used for ICEV, increasing it by 24% on the assumption of a linear relationship between vehicle weight and resuspension emissions. As already seen in Section 2, a relationship between vehicle weight and road dust emission factor is questionable as only aerodynamic features (size and shape) should affect resuspension from paved roads.
Differences in findings are driven by differences in PM fractions of primary and secondary emissions, the emission processes under consideration, as well as the methodologies used for their estimation. A review of this literature reveals a number of factors that determine the amount of particulate matter emitted by BEVs vs. ICEVs.
Secondary aerosols
Secondary aerosols (SA) are formed in the atmosphere by gas-particle conversion processes such as nucleation, condensation and heterogeneous chemical reactions (Ziemann and Atkinson, 2012[30]; Zhang et al., 2007[31]; Carlton, Wiedinmyer and Kroll, 2009[32]). Secondary aerosols are therefore not included in emission inventory and their contribution can only be estimated through PM chemical speciation (offline or online) or by air quality modelling. Only eight studies accounted for secondary PM from ICEVs when comparing their environmental burden with that of BEVs, using air quality modelling (Nopmongcol et al., 2017[18]; Ke et al., 2017[5]; Razeghi et al., 2016[33]; Tobollik et al., 2016[34]; Tessum, Hill and Marshall, 2014[35]; Li et al., 2016[36]; Soret, Guevara and Baldasano, 2014[11]).
Air quality models still have difficulties, however, in reproducing observed particulate matter (PM) concentration levels. This is mostly due to a poor representation of the organic aerosols fractions—primary and notably secondary—limiting the capability to assess the full air quality impact of ICEVs. Despite the substantial number of studies conducted during the last decades, the source apportionment of the secondary organic aerosols (SOA) fraction remains difficult, due to the complexity of the physicochemical processes involved. The selection and use of appropriate approaches are a major challenge for the atmospheric science community.
3.2. Calculating primary and secondary PM from exhaust
Primary PM emissions
For ICEVs, EURO 6-temp emission factors for primary exhaust emissions of PM10 and PM2.5 are taken from (Ntziachristos and Samaras, 2018[37]), which is a widely used reference for emission reporting in EU.
Secondary inorganic aerosol (SIA) formation
While natural sources (seas and volcanoes) dominate SIA at a global scale, SIA at the urban scale are mainly due to anthropogenic (combustion) sources. Given the low-sulphur content of current fuels used in road transport in most regions of the world (CCAC, 2016[38]), the road traffic contribution to SIA is generally comprised of NH4NO3 (ammonium nitrate) formation from NOx (vehicles) and NH3 (mainly from agriculture) emissions. This report provides a range of estimates of SIAs from exhaust emissions using the LOTOS-EUROS source apportionment tool applied to European countries (as has been done for the Netherlands in Hendriks et al. (2013[39])). LOTOS-EUROS is a CTM model incorporating a “labelling” tool, which involves tracking specific emissions from the source to the receptor, thus allowing for source apportionment analysis of secondary aerosols. The main advantage of this approach with respect to receptor models like PMF, is that the full (modelled) NH4NO3 is apportioned, while PMF is typically unable to do so. Source apportionment analysis was performed for European countries over one year (2018), which provided nitrate and elemental carbon (EC) concentrations (µg/m3) from LDV for each country. This allows for the estimation of a range of gas-to-particle conversion rates over a quite heterogeneous geographical domain, i.e. covering different climatic conditions and anthropogenic source types. The range for SIA emission factors was calculated as:
where NO3i/ECi is the ratio between nitrate and elemental carbon due to LDV emissions for ith country, NOxj/NOxfleet is the ratio between the NOx emission factor for the jth vehicle type (e.g. diesel EURO6-temp) and the average NOx emission factor for the whole EU fleet, estimated based on Ntziachristos and Samaras (2018[37]) for emission factors, CARB (2020[40]) for age composition and OICA (2020[41]) for fuels/classes distribution. The constant 1.29 is the molecular mass ratio between ammonium nitrate and nitrate.
Secondary organic aerosols (SOA) formation
Similarly to SIA, SOA are known to account for a significant fraction of airborne PM, with considerable impacts on air quality. The contribution of SOA to organic aerosols (OA) reaches up to 80% under certain atmospheric conditions (Carlton, Wiedinmyer and Kroll, 2009[32]). Most organic aerosols (OA) in urban and rural atmospheres are speculated to be secondary in nature but their exact chemical composition remains uncertain (Shrivastava et al., 2007[42]; Zhao et al., 2013[43]). In contrast with SIA, SOA are formed from both biogenic (i.e. naturally occurring) and anthropogenic gaseous emission sources, even at the urban scale (Griffin et al., 1999[44]; Vidhi and Shrivastava, 2018[45]). Several methodologies currently used assess SOA levels and composition, including the elemental carbon (EC) tracer method, chemical mass balance method (CMB), SOA tracer method, radiocarbon (14C) measurement and positive matrix factorization (PMF). Another group of studies uses simulation chambers in which SOA formation is reproduced under controlled conditions (Platt et al., 2017[46]).
In this report, we compiled the chemical factor profile of 26 PMF analyses carried out in the US in which authors were able to separate between emissions from gasoline LDVs and diesel HDVs (e.g. (Kim and Hopke, 2004[47]; Kim et al., 2003[48]; Kim, Hopke and Edgerton, 2004[49]; Wang et al., 2012[50]; Zhao and Hopke, 2006[51]; Gildemeister, Hopke and Kim, 2007[52]; Lee and Hopke, 2006[53]). We then applied the EC tracer method, applying an average primary OC/EC ratio of 3.7 for gasoline and 0.5 for diesel, respectively, considering the age distribution of US fleet in 2000 and ratios of organic matter to elemental carbon (OM/EC ratios) from Ntziachristos and Samaras (2018[37]). Compiling these factor profiles, we found median values for secondary-to-primary organic carbon (SOC/POC) ratios of 0 (i.e. no formation of SOC) for diesel and 0.35 for gasoline emissions, respectively. We then multiplied the SOC by a factor of 1.5, following Aiken et al. (2008[54]), in order to convert SOC to SOA.
Estimates of SIA and SOA assume that the area of study as representative of a global situation, which is not likely given that secondary aerosol formation does not depend linearly on gaseous precursors and is heavily affected by local conditions such as air temperature, relative humidity and ambient concentrations of various precursors. There are limitations to each approach. SIA could be underestimated given that only modelled nitrate is apportioned and that secondary sulphate is not included (relevant for countries using high-sulphur diesel (CCAC, 2016[38])). SOA estimates are affected by errors from a possible inaccurate apportionment of OC. Moreover, in the United States, diesel is used almost exclusively by HDVs which may have a different OM/EC ratio than LDVs (the category used in (Ntziachristos and Samaras (2018[37])). We also assume SOC/POC ratios to be same between EURO6 vehicles and the pre-2000 fleet.
3.3. Calculating primary PM from non-exhaust sources
This section develops a methodological approach to compare non-exhaust emissions between battery electric and internal combustion vehicles. The comparison is performed for three vehicle categories: passenger cars (PCs), sport utility vehicles (SUVs) and light commercial vans (LCVs). These categories correspond to the following European Nomenclature for Reporting (NFR) categories: “Medium cars,” “Large-SUV-Executive,” and “Light Commercial Vehicles < 3.5 t (LCV).” Assumptions regarding vehicle weights follow those estimated by the Argonne National Laboratory from updated vehicle specifications for the GREET Vehicle-Cycle model (Argonne National Laboratory, 2019[55]).
The benchmark ICEVs considered are diesel and gasoline EURO6d-temp vehicles. Estimates of UFP particle numbers are not addressed given the scarcity of data and the considerable uncertainty regarding EF estimates from non-exhaust processes (Amato, 2018[56]). Additional assumptions used in the calculation of non-exhaust emission factors are reviewed in the subsequent sections.
Internal combustion engine vehicles
For ICEVs, base emission factors for primary non-exhaust emissions of PM10 and PM2.5 for brake wear, tyre wear and road wear are taken from Ntziachristos & Boulter (2016[57]), which is a widely used reference for emission reporting in the EU.3 PM10 emission factors for road dust resuspension are calculated as the median of the lower bounds of observed ranges in the literature for light duty vehicles, corresponding to 0.009 g/vkm for LDV. Because the LDV category includes PCs, SUVs and LCVs, we performed a least squares fit model, using fleet-averaged emission factor data from two studies in Barcelona and Zurich (Amato et al., 2012[58]; Bukowiecki et al., 2010[59]) and assuming that the distribution of these vehicle types in these locations is similar to that in the metropolitan area of Milan (ACI Automobile Club d’Italia, 2019[60]).
When applied to current assumptions regarding each vehicle type, this analysis yields road dust EFs of 0.0083, 0.0099, and 0.0113 g/vkm for PC, SUV and LCV respectively. All of these values are significantly lower than the 0.040 g/vkm used by Timmers and Achten (2016[9]) or the 0.088 g/vkm used by Soret et al. (2014[11]). The reason for this more conservative choice is driven by the large variability observed in the literature, which reflects the large number of factors influencing EFs (vehicle speed, weight and road dust loading among others, see Section 2). Given that the highest values were found where additional sources of road dust are present (e.g. dust sources on the roadside, road shoulders, sanding/salting and use of studded tyres), road dust loadings appear to be most responsible for the variability in EFs in the literature. The estimate of non-exhaust EF used in this analysis can therefore be considered a lower bound, as it does not take into account additional sources of road dust emissions that are likely to be present in certain regions.
We base the PM2.5 component of road dust emissions on the PM10 estimate via the application of a mean PM2.5/PM10 ratio of 0.34 (standard deviation of 0.27) that characterises road dust contributions, as found in the literature (Chan et al 1999; (Achilleos et al., 2016[61]; Chan et al., 2008[62]; Cheng et al., 2015[63]; Srimuruganandam and Shiva Nagendra, 2012[64]; Gummeneni et al., 2011[65]; Guttikunda et al., 2013[66]; Perrone et al., 2012[67]; Almeida et al., 2005[68]; Amato et al., 2009[69]; Amato et al., 2014[70]).
Battery electric vehicles
Emission factors for BEVs are calculated based on evidence about the quantitative relationships between various vehicle characteristics and the PM emissions generated by brake, tyre, and road wear, and road dust resuspension. As described in Section 1, vehicle weight is the main determinant of wear emissions. In a first step therefore, quantitative relationships between vehicle weight and each non-exhaust emissions generation process are estimated based on the available literature. Emission factors are then calculated by applying the assumed weight of BEVs in each vehicle category to these estimated relationships (see Table 3.1 and Table 3.2)
A lack of robust quantitative evidence characterises the literature concerning the relationship between brake wear and vehicle weight. This relationship was therefore estimated using existing estimates of brake wear emission factors for medium-sized cars and LCVs, assuming their respective mean weights according to the GREET model (Argonne National Laboratory, 2019[55]).
The relationship between vehicle weight and tyre wear is estimated using a range of values found in the literature. Emission factors are first estimated using a linear relationship, similar to (Chen and Prathaban, 2013[256]; Aatmeeyata, Kaul and Sharma, 2009[257]; Wang et al., 2017[258]; Li et al., 2012[259]; Simons, 2016[260]). They are also estimated using a non-linear relationship based on results from Salminen (2014[261]) and Ngeno and Mohammadi (2015). In the absence of a functional form proposed, a power-law function is used, following the approach for brake wear as described in Table 3.1 and Table 3.2
No robust relationships for road wear emission factors as a function of ve hicle weight were found in the literature. Although a power law of 4 has been proposed for HDV loads (ACEA, 2015), applying this relationship to LDVs is not recommended. Following EMEP/EEA, all vehicle classes are therefore assumed to have the same road wear emission factors. For road dust resuspension, the OLS model described in Table 3.1 and Table 3.2 estimate 19% and 36% higher road dust EFs for SUV and LCV categories relative to PCs, likely due to their larger size.
Table 3.1 Estimation methods used in the calculation of PM2.5 non-exhaust emissions for BEVs
Source |
Estimation Method |
|
---|---|---|
Brake wear |
0.25 * 2x10-7 * Weightj 1.290 Model estimated using EF values for PC and LDV from Ntziachristos and Boulter (2016[57]) (0.0029 g/km and 0.0046 g/km, respectively) and weights for vehicle classes j from (Argonne National Laboratory, 2019[55]). A 75% reduction in brake wear from RBS is also assumed. |
|
Tyre wear |
Model 1 3x10-7 Weightj 1.326 Power law model estimated using EF values for PC and LDV from Ntziachristos and Boulter (2016[57]) (0.0064 g/km and 0.0101 g/km, respectively) and weights for vehicle classes j from (Argonne National Laboratory, 2019[55]). |
Model 2 For PCs and SUVs: 0.0031 * Weightj For LCVs: 0.0035 * Weightj Linear model estimated using EFs from Ntziachristos and Boulter (2016[57]) and weights for vehicle classes j from (Argonne National Laboratory, 2019[55]). |
Road wear |
N/A Values are taken from Ntziachristos and Boulter (2016[57]) (0.0041 for all vehicle classes) |
|
Road dust resuspension |
0.34 * EFjPM10 A ratio of PM2.5/PM10 emissions from road dust is applied to EFjPM10, the PM10 EF from road dust suspension for vehicle class j (last row in Table 3.1), according to the mean found in the literature (Chan et al 1999; Achilleos et al 2016; Wahlin et al 2006; Cheng et al 2015; Srimuruganandam and Shiva Nagendra 2012a and 2012b; Gummeneni et al 2011; Guttikunda et al., 2013; Perrone at al., 2012; Almeida et al., 2005; Amato et al., 2009b and 2014a). |
Note: Base EFs are assumed the same for diesel and gasoline-fuelled vehicles. Tables 3.5, 3.7, and 3.9 in (Ntziachristos and Boulter, 2016[57]) contain estimates of the mass fraction of TSP (gPM/gTSP) and Tables 3.4, 3.6, and 3.8 in (Ntziachristos and Boulter, 2016[57]) contain estimates of TSP emission factors (gTSP/vkm). See Table 3.3 for weight assumptions.
Table 3.2 Estimation methods used in the calculation of PM10 non-exhaust emissions for BEVs
Source |
Estimation Method |
|
---|---|---|
Brake wear |
0.25 × 6x10-7 × Weightj 1.290 Model is estimated using EF values for PC and LDV from Ntziachristos and Boulter (2016[57]) (0.0074 and 0.0015 g/km, respectively) and weights for vehicle classes j from (Argonne National Laboratory, 2019[55]). A 75% reduction in brake wear from RBS is also assumed following the mean of the estimates in the literature. |
|
Tyre wear |
Model 1 4x10-7 Weightj 1.326 Power law model estimated using EF values for PC and LDV from Ntziachristos and Boulter (2016[57]) (0.0064 and 0.0101 g/km, respectively) and weights for vehicle classes j from (Argonne National Laboratory, 2019[55]). |
Model 2 For PCs and SUVs: 0.0044 * Weightj For LCVs: 0.0049 * Weightj Linear model estimated using EFs from Ntziachristos and Boulter (2016[57]) and weights for vehicle classes j from (Argonne National Laboratory, 2019[55]). |
Road wear |
N/A Values are taken from Ntziachristos and Boulter (2016[57]) for ICEV vehicles (0.0075 for all vehicle classes) |
|
Road dust resuspension |
Final EFs are calculated for each class based on an optimisation tool that minimises the difference between observed total LDV emissions per day at three locations (Bukowiecki et al., 2010[59]; Amato et al., 2012[71]) and the sum of emissions per day from different vehicles categories, assuming a distribution of PCs, SUVs, and LCVs according to vehicle stock estimates in Milan (ACI Automobile Club d’Italia, 2019[60]). |
Note: Base EFs are assumed to be the same for diesel and gasoline-fuelled vehicles. Tables 3.5, 3.7, and 3.9 in (Ntziachristos and Boulter, 2016[57]) contain estimates of the mass fraction of TSP (gPM/gTSP) and Tables 3.4, 3.6, and 3.8 in (Ntziachristos and Boulter, 2016[57]) contain estimates of TSP emission factors (gTSP/vkm). See Table 3.3 below for weight assumptions.
Table 3.3 Vehicle weight assumptions (kg)
Vehicle class |
ICEV |
BEV 100 |
Difference |
BEV 300 |
Difference |
---|---|---|---|---|---|
PC |
1453 |
1517 |
+4 % |
1949 |
+34 % |
SUV |
1775 |
1866 |
+5 % |
2437 |
+37 % |
LCV |
2051 |
2185 |
+6 % |
2904 |
+41 % |
The extra weight of BEVs with respect to ICEVs, relevant for the calculation of brake and tyre wear emission factors, is based on updated vehicle specifications for the GREET Vehicle-Cycle model developed by the Argonne National Laboratory (2019[55]) . Both BEVs with a 100-mile range (BEV 100) and a 300-mile range (BEV 300) are considered in the analysis. BEVs with a longer range are heavier due to the weight of the battery. Lighter weight BEVs with a shorter range are between 4% and 6% heavier than ICEVs, while heavier BEVs are between 34 and 41% heavier than ICEVs across vehicle classes. Other estimates in the literature have placed the difference within this range, at around 20-25% (Van Zeebroeck and De Ceuster, 2013[7]; Timmers and Achten, 2016[9]).
3.4. Particulate matter emitted by conventional and electric vehicles
3.4.1. Total PM emission factors
Estimations of exhaust and non-exhaust emission factors for passenger cars (PCs), SUVs, and LCVs are listed in Table 3.4 and Table 3.5. Between 95 and 98% of primary PM10 emissions come from non-exhaust sources and between 74 and 96% of total (primary + secondary) PM10 are emitted by EURO 6-temp ICEV. These shares are similarly high for PM2.5: 88-96% of primary PM2.5 emissions come from non-exhaust sources and 65-93% of total PM2.5 are emitted by ICEVs. The non-exhaust share of total PM is generally lower for diesel ICEVs than gasoline since the contribution of diesel to secondary aerosols is higher than that of gasoline. There are small differences between vehicle categories, with 90.6% of PM10 and 85% of PM2.5 from ICEV PCs originating from non-exhaust sources, 93% and 88% for SUVs and 95% and 91% for LCVs.
Table 3.4 PM2.5 emission factors across EURO-6-temp and BEV vehicle classes (g/vkm)
PC |
SUV |
LCV |
|||||||||||||
---|---|---|---|---|---|---|---|---|---|---|---|---|---|---|---|
Diesel |
Gasoline |
BEV 100 |
BEV 300 |
Diesel |
Gasoline |
BEV 100 |
BEV 300 |
Diesel |
Gasoline |
BEV 100 |
BEV 300 |
||||
Non-exhaust |
low |
0.0121 |
0.0121 |
0.0100 |
0.0115 |
0.0133 |
0.0133 |
0.0113 |
0.0135 |
0.0165 |
0.0165 |
0.0134 |
0.0164 |
||
high |
0.0165 |
0.0165 |
0.0147 |
0.0169 |
0.0193 |
0.0193 |
0.0174 |
0.0206 |
0.0226 |
0.0226 |
0.0200 |
0.0241 |
|||
Exhaust (total)
|
low |
0.0020 |
0.0017 |
0.0020 |
0.0017 |
0.0013 |
0.0013 |
||||||||
high |
0.0088 |
0.0026 |
0.0088 |
0.0026 |
0.0071 |
0.0020 |
|||||||||
Primary |
0.0015 |
0.0016 |
0.0015 |
0.0016 |
0.0009 |
0.0012 |
|||||||||
Secondary |
|||||||||||||||
SOA |
0.0003 |
0.0003 |
0.0002 |
||||||||||||
SIA |
low |
0.0005 |
0.0001 |
0.0005 |
0.0001 |
0.0004 |
0.0001 |
||||||||
high |
0.0073 |
0.0010 |
0.0073 |
0.0010 |
0.0062 |
0.0008 |
|||||||||
Total PM
|
low |
0.0141 |
0.0137 |
0.0100 |
0.0115 |
0.0153 |
0.0150 |
0.0113 |
0.0135 |
0.0178 |
0.0178 |
0.0134 |
0.0164 |
||
high |
0.0253 |
0.0192 |
0.0147 |
0.0169 |
0.0281 |
0.0219 |
0.0174 |
0.0206 |
0.0297 |
0.0246 |
0.0200 |
0.0241 |
|||
Percent non-exhaust |
low |
85.7% |
87.8% |
86.9% |
88.9% |
92.6% |
92.9% |
||||||||
high |
65.3% |
86.3% |
68.7% |
88.2% |
76.1% |
91.9% |
Table 3.5 PM10 emission factors across EURO 6-temp ICEV and BEV vehicle classes (g/vkm)
PC |
SUV |
LCV |
||||||||||||
---|---|---|---|---|---|---|---|---|---|---|---|---|---|---|
Diesel |
Gasoline |
BEV 100 |
BEV 300 |
Diesel |
Gasoline |
BEV 100 |
BEV 300 |
Diesel |
Gasoline |
BEV 100 |
BEV 300 |
|||
Non-exhaust PM |
low |
0.0296 |
0.0296 |
0.0243 |
0.0270 |
0.0346 |
0.0346 |
0.0281 |
0.0317 |
0.0404 |
0.0404 |
0.0326 |
0.0376 |
|
high |
0.0296 |
0.0296 |
0.0244 |
0.0276 |
0.0349 |
0.0349 |
0.0286 |
0.0333 |
0.0404 |
0.0404 |
0.0326 |
0.0388 |
||
Exhaust PM (total) |
low |
0.0033 |
0.0018 |
0.0033 |
0.0018 |
0.0024 |
0.0014 |
|||||||
high |
0.0103 |
0.0028 |
0.0103 |
0.0028 |
0.0084 |
0.0022 |
||||||||
Primary |
0.0015 |
0.0016 |
0.0015 |
0.0016 |
0.0009 |
0.0012 |
||||||||
Secondary |
0.0003 |
0.0003 |
0.0002 |
|||||||||||
SOA |
||||||||||||||
SIA |
low |
0.0018 |
0.0002 |
0.0018 |
0.0002 |
0.0015 |
0.0002 |
|||||||
high |
0.0088 |
0.0012 |
0.0088 |
0.0012 |
0.0075 |
0.0010 |
||||||||
Total PM |
low |
0.0328 |
0.0317 |
0.0243 |
0.0270 |
0.0378 |
0.0367 |
0.0281 |
0.0317 |
0.0428 |
0.0420 |
0.0326 |
0.0376 |
|
high |
0.0399 |
0.0327 |
0.0244 |
0.0276 |
0.0452 |
0.0379 |
0.0286 |
0.0333 |
0.0488 |
0.0428 |
0.0326 |
0.0388 |
||
Percent non-exhaust |
90.1% |
93.4% |
91.4% |
94.3% |
94.4% |
96.2% |
||||||||
74.1% |
90.6% |
77.2% |
92.0% |
82.8% |
94.5% |
3.4.2. Non-exhaust PM emission factors
Estimated PM2.5 and PM10 emission factors from non-exhaust sources are reported in Tables 3.4 and 3.5, respectively. Estimates indicate that both lighter weight and heavier weight BEVs emit less PM10 than their ICEV counterparts. However, this is not the case for PM2.5. Electric vehicles with a longer range, and therefore a higher weight, emit more PM2.5 than ICEV vehicles across all vehicle classes depicts these results graphically and Table 3.8 shows the relative differences in particulate matter emitted between BEVs and gasoline-fuelled ICEVs for each vehicle class.
Table 3.6 PM2.5 emission factors from non-exhaust sources across ICEV and BEV vehicle classes (g/vkm)
PC |
SUV |
LCV |
||||||||
---|---|---|---|---|---|---|---|---|---|---|
ICEV |
BEV 100 |
BEV 300 |
ICEV |
BEV 100 |
BEV 300 |
ICEV |
BEV 100 |
BEV 300 |
||
Brake wear |
0.0029 |
0.0006 |
0.0009 |
0.0031 |
0.0008 |
0.0012 |
0.0046 |
0.0010 |
0.0015 |
|
Tyre wear |
low |
0.0045 |
0.0047 |
0.0060 |
0.0055 |
0.0058 |
0.0075 |
0.0071 |
0.0076 |
0.0100 |
high |
0.0045 |
0.0050 |
0.0069 |
0.0061 |
0.0065 |
0.0093 |
0.0071 |
0.0080 |
0.0117 |
|
Road wear |
0.0041 |
0.0041 |
0.0041 |
0.0041 |
0.0041 |
0.0041 |
0.0041 |
0.0041 |
0.0041 |
|
Road dust |
0.0028 |
0.0028 |
0.0028 |
0.0034 |
0.0034 |
0.0034 |
0.0038 |
0.0038 |
0.0038 |
|
Total non-exhaust |
low |
0.0121 |
0.0100 |
0.0115 |
0.0133 |
0.0113 |
0.0135 |
0.0165 |
0.0134 |
0.0164 |
high |
0.0165 |
0.0147 |
0.0169 |
0.0193 |
0.0174 |
0.0206 |
0.0226 |
0.0200 |
0.0241 |
Note: Emission factors for BEV100 and BEV300 are calculated using the weight estimates for BEVs with a range of 100 and 300 miles, respectively (Argonne National Laboratory, 2019[55]). The amount of PM from non-exhaust sources produced by gasoline and diesel vehicles are assumed to be the same.
Source: Authors’ calculations, see Table 3.1
Table 3.7 PM10 emission factors from non-exhaust sources across ICEV and BEV vehicle classes (g/vkm)
PC |
SUV |
LCV |
||||||||
---|---|---|---|---|---|---|---|---|---|---|
|
|
ICEV |
BEV 100 |
BEV 300 |
ICEV |
BEV 100 |
BEV 300 |
ICEV |
BEV 100 |
BEV 300 |
Brake wear |
0.0074 |
0.0019 |
0.0026 |
0.0093 |
0.0025 |
0.0035 |
0.0115 |
0.0030 |
0.0044 |
|
Tyre wear |
low |
0.0064 |
0.0066 |
0.0086 |
0.0078 |
0.0082 |
0.0108 |
0.0101 |
0.0107 |
0.0144 |
high |
0.0064 |
0.0067 |
0.0092 |
0.0081 |
0.0087 |
0.0124 |
0.0101 |
0.0108 |
0.0156 |
|
Road wear |
0.0075 |
0.0075 |
0.0075 |
0.0075 |
0.0075 |
0.0075 |
0.0075 |
0.0075 |
0.0075 |
|
Road dust |
0.0083 |
0.0083 |
0.0083 |
0.0099 |
0.0099 |
0.0099 |
0.0113 |
0.0113 |
0.0113 |
|
Total non-exhaust |
low |
0.0296 |
0.0243 |
0.0270 |
0.0346 |
0.0281 |
0.0317 |
0.0404 |
0.0326 |
0.0376 |
high |
0.0296 |
0.0244 |
0.0276 |
0.0349 |
0.0286 |
0.0333 |
0.0404 |
0.0326 |
0.0388 |
Note: Emission factors for BEV100 and BEV300 are calculated using the weight estimates for BEVs with a range of 100 and 300 miles, respectively (Argonne National Laboratory, 2019[55]). The amount of PM from non-exhaust sources produced by gasoline and diesel vehicles is assumed to be the same.
Source: Authors’ calculations, see Table 3.2.
Figure 3.1. ICEV and BEV non-exhaust PM emission factors by vehicle class
PM2.5 (left) and PM10 (right)

Note: Emission factors for BEV 100 and BEV 300 are calculated using the conventional weight estimates for a BEV with a range of 100 and 300 miles, respectively (Argonne National Laboratory, 2019).
Source: Authors’ calculations, see Table 3.1 and Table 3.2.
Table 3.8 Net change in total non-exhaust emission factors of BEVs relative to gasoline ICEVs (percentage)
PC |
SUV |
LCV |
||
---|---|---|---|---|
PM2.5 |
BEV 100 |
-12.8 |
-11.2 |
-13.3 |
BEV 300 |
+2.6 |
+7.5 |
+7.8 |
|
PM10 |
BEV 100 |
-17.8 |
-18.0 |
-19.3 |
BEV 300 |
-6.5 |
-4.5 |
-5.5 |
Results show that reductions in non-exhaust emission factors are greater for lighter weight BEVs than for heavier weight BEVs. Indeed, BEV 100 vehicles are estimated to emit 11-19% less non-exhaust emissions than their conventional gasoline-fuelled counterparts across all vehicle classes. However, this is not the case for BEV 300 vehicles. Heavier EVs lead to marginal reductions in PM10 emissions of 4.5-6.5%, and increase PM2.5 emissions across all vehicle classes by 2.6-7.8%.
As shown in Figure 3.2, the relative importance of the sources of non-exhaust emissions varies by drivetrain and vehicle class. Road dust resuspension is the greatest source of PM10 emissions in all vehicle categories, representing 28% and 30-35% of total PM10 emissions from non-exhaust for ICEVs and BEVs, respectively. Road wear represents 19-25% of PM10 emissions for ICEVs and 19-31% for BEVs. Tyre wear represents 22-25% of PM10 from non-exhaust sources for ICEV vehicles, and 27-40% for BEVs. The greatest difference in composition across ICEVs and BEVs is the portion of non-exhaust emissions that come from brake wear. For ICEVs, 25-28% of non-exhaust PM10 emissions are due to brake wear, whereas for BEVs, only 8-11% of total non-exhaust PM10 emissions are due to brake wear.
While road dust makes up a significantly smaller proportion of non-exhaust PM2.5 emissions, the same general patterns hold for changes in the composition of non-exhaust emissions across ICEV and BEV vehicle categories for PM2.5. Road dust comprises an estimated 20% of total non-exhaust PM2.5 emissions for ICEVs, and 18-23% for BEVs. Road wear is responsible for 21-28% of non-exhaust PM2.5 for ICEVs and 19-32% for BEVs. Tyre wear represents 40-56% of non-exhaust PM2.5 emissions for BEVs. Finally, brake wear represents 19-23% of PM2.5 non-exhaust emissions from BEVs.
Moving from BEV100 vehicles to BEV300 vehicles shifts the composition of non-exhaust emissions toward tyre wear, especially for PM2.5 emissions, the majority of which are generated by tyre wear for all vehicle classes and drivetrains. Brake wear decreases significantly in BEVs relative to ICEVs due to regenerative braking systems.
Figure 3.2. Relative share of non-exhaust emission factors for ICEV and BEV vehicle classes (percent)
PM2.5 (top) and PM10 (bottom)

Note: Calculated on the basis of tyre wear emission factors estimated according to Model 2 as described in Tables 3.1 and 3.2. The relative shares of non-exhaust emission sources are similar for gasoline and diesel vehicles.
Source: Author’s calculations.
3.4.3. Projections of particulate emissions from non-exhaust sources
On the basis of the emission factor estimates above4 and projections for global vehicle stocks, this section presents estimates of non-exhaust emissions rates per kilometre travelled by the global vehicle fleet (tonnes/km) and annual non-exhaust emissions between 2017 and 2030. In 2017, the global vehicle fleet emitted an estimated 38.1 tonnes of PM10 per kilometre travelled, or 571,881 tonnes, assuming an annual mileage of 15,000 km/vehicle. Projected emissions are estimated for two scenarios based on different assumptions about electric vehicle uptake following the previous penetration scenarios of 4% and 8% uptake in 2030, similar to those projected by IEA’s New Policies Scenario and EV30@30 Scenario (IEA, 2019).
In both scenarios, increasing travel demand causes non-exhaust emissions to rise substantially from 2017 to 2030. However, the reductions in PM emissions made possible by a doubling of electric vehicle uptake over this period are slight. The amount of non-exhaust emissions emitted per kilometre by the global vehicle fleet in 2030 is estimated to be 58.1 tonnes PM10 with a 4% uptake of electric vehicles and 57.2 tonnes PM10 with an uptake of 8%. This amounts to 871,500 and 858,000 tonnes of total PM10, respectively.
Results are even less significant for PM2.5 emissions: global emissions in 2017 are estimated to amount to 18.5 tonnes of PM2.5 emitted per kilometre travelled by the global vehicle fleet, or 277,500 tonnes in total. Emissions are projected to rise to 28.3 tonnes per kilometre with an EV uptake of 4%, amounting to 424,500 tonnes globally. Assuming a doubling of EV uptake leads to PM2.5 emissions of 28.0 tonnes per kilometre or 420,000 tonnes globally.
Figure 3.3. Emission rate estimates for the year 2017 and projected 2030 under alternative EV uptake scenarios

Source: Author’s calculations. Projections of non-exhaust emissions are made on the basis of the emission factors estimated above and on projections for vehicle stocks. Emission factors from road wear are assumed to be unchanging from 2017 to 2030. Global vehicle fleet projections are based on those used by the IEA, and the electric vehicle penetration scenarios of 4% and 8% uptake are based on the New Policies Scenario and EV30@30 scenarios developed by the IEA (2019[72]). The annual distance travelled by each vehicle is assumed to be 15,000 km.
Other data available also permits an evaluation of the impact of BEV uptake in two specific cities: Milan, Italy, and Santiago, Chile. Following the previous projection, BEV uptake scenarios of 4% and 8% are used. Assuming that current EV technologies will not change until 2030 (e.g. battery weight), these simulations also indicate a minimal impact of BEV uptake on total particulate emissions from non-exhaust sources. In Milan (based on emission inventories from INEMAR and AMAT for 2014), an uptake of 4% and 8% leads to reductions of 0.4% and 0.8% for PM10 emissions and 0.3% and 0.7% for PM2.5 emissions. In Santiago, high road dust emission factors and a lower percentage of diesel cars in the vehicle fleet lead to a negligible reduction of non-exhaust PM emissions with the uptake of BEVs (≤0.1%).
3.4.4. Influencing factors and implications for policy
Relative to conventional gasoline-fuelled vehicles, electric vehicles emit 11-19% less PM10. Reductions in PM2.5 emissions are only observed for lightweight BEVs, which generate 11.2-13.3% less PM2.5 from non-exhaust sources. Heavier BEVs, in contrast, generate 2.6-7.8% more PM2.5 than their conventional counterparts. Moreover, projections of global PM emissions rates from non-exhaust sources show that emissions are set to increase, even when considering a relatively high level of electric vehicle penetration by that year.
For EVs, the most important source of PM2.5 emissions is by tyre wear, and the most important sources of PM10 are more evenly spread between tyre, road wear and road dust resuspension. For both drivetrain types, tyre wear increases with vehicle weight. The share of non-exhaust emissions from brake wear falls sharply for EVs relative to ICEVs due to regenerative braking. Uncertainty regarding emission factors remains, however, due to a lack of experimental data on various sources of wear, and the estimations reported here rely on a number of assumptions based on the available evidence (see Tables 3.1 and 3.2).
A number of other factors beyond those considered in the reported estimates could also affect the amount of particulate matter emitted by EVs in real world driving conditions. The higher fuel economy of BEVs may, for example, have a rebound effect of increased travel demand, which could further undermine projected PM emissions reductions, especially if it draws travel demand away from non-motorised modes. EV use can also contribute to traffic congestion. While congestion is known to increase exhaust emissions, its impact on non-exhaust emissions is not clear given that a higher braking frequency (i.e. higher brake wear) could be offset by lower average speed (i.e. lower tyre and road wear and lower resuspension). If, could also reduce the benefits of EVs with respect to PM emissions, as heavier BEVs are estimated to emit more PM2.5 than their conventional counterparts.
Given that PM, and PM2.5 in particular, is the most harmful pollutant for public health, it is clear that the widespread adoption of EVs will not eliminate air pollution concerns due to road traffic and that policies addressing non-exhaust emissions are warranted. The body of literature is too limited to assess whether the change in composition of the PM emissions generated from ICEVs vs. EVs has implications for the risk of mortality and morbidity associated with exposure to these emissions. More research is needed on the relative risk associated with each traffic source and PM component, as well as possible non-linearities in health impacts at different PM exposure levels.
References
[61] Achilleos, S. et al. (2016), “Spatial variability of fine and coarse particle composition and sources in Cyprus”, Atmospheric Research, Vol. 169, pp. 255-270, http://dx.doi.org/10.1016/j.atmosres.2015.10.005.
[60] ACI Automobile Club d’Italia (2019), Stock vehicles in the metropolitan area of Milan.
[54] Aiken, A. et al. (2008), “O/C and OM/OC ratios of primary, secondary, and ambient organic aerosols with high-resolution time-of-flight aerosol mass spectrometry”, Environmental Science and Technology, Vol. 42/12, pp. 4478-4485, http://dx.doi.org/10.1021/es703009q.
[4] Alam, M. et al. (2018), “Analysing the Co-Benefits of transport fleet and fuel policies in reducing PM2.5 and CO2 emissions”, Journal of Cleaner Production, Vol. 172, pp. 623-634, http://dx.doi.org/10.1016/j.jclepro.2017.10.169.
[68] Almeida, S. et al. (2005), “Source apportionment of fine and coarse particulate matter in a sub-urban area at the Western European Coast”, Atmospheric Environment, Vol. 39/17, pp. 3127-3138, http://dx.doi.org/10.1016/j.atmosenv.2005.01.048.
[17] Althaus, H., M. Gauch and Empa (2010), “Comparative Life Cycle Assessment in Mobility (in Germany)”, p. 162, https://www.empa.ch/documents/56122/458579/LCA-Mobilitaetsvergleich_Bericht.pdf/824aec56-3393-439e-8bc9-d1a365041e4d.
[56] Amato, F. (ed.) (2018), Non-exhaust emissions: an urban air quality problem for public health ; impact and mitigation measures, Elsevier, SAN DIEGO, https://www.elsevier.com/books/non-exhaust-emissions/amato/978-0-12-811770-5.
[70] Amato, F. et al. (2014), “Trends of road dust emissions contributions on ambient air particulate levels at rural, urban and industrial sites in southern Spain”, Atmospheric Chemistry and Physics, Vol. 14/7, pp. 3533-3544, http://dx.doi.org/10.5194/acp-14-3533-2014.
[58] Amato, F. et al. (2012), “Emission factors from road dust resuspension in a Mediterranean freeway”, Atmospheric Environment, Vol. 61, http://dx.doi.org/10.1016/j.atmosenv.2012.07.065.
[71] Amato, F. et al. (2012), “Emission factors from road dust resuspension in a Mediterranean freeway”, Atmospheric Environment, Vol. 61, pp. 580-587, http://dx.doi.org/10.1016/j.atmosenv.2012.07.065.
[69] Amato, F. et al. (2009), “Spatial and chemical patterns of PM10 in road dust deposited in urban environment”, Atmospheric Environment, Vol. 43/9, pp. 1650-1659, http://dx.doi.org/10.1016/j.atmosenv.2008.12.009.
[55] Argonne National Laboratory (2019), GREET vehicle-cycle model Series 2, https://greet.es.anl.gov (accessed on 17 December 2019).
[14] Barlow, T. (2014), “Briefing paper on non-exhaust particulate emissions from road transport”, Transport Research Laboratory.
[59] Bukowiecki, N. et al. (2010), “PM10 emission factors for non-exhaust particles generated by road traffic in an urban street canyon and along a freeway in Switzerland”, Atmospheric Environment, Vol. 44/19, pp. 2330-2340, http://dx.doi.org/10.1016/j.atmosenv.2010.03.039.
[40] California Air Resources Board (2020), Fleet Database, https://arb.ca.gov/emfac/fleet-db (accessed on 23 April 2020).
[32] Carlton, A., C. Wiedinmyer and J. Kroll (2009), “A review of Secondary organic aerosol (SOA) formation from isoprene”, Atmospheric Chemistry and Physics, Vol. 9/14, pp. 4987-5005, http://dx.doi.org/10.5194/acp-9-4987-2009.
[6] Carslaw, D. (2006), “New Directions: A heavy burden for heavy vehicles: Increasing vehicle weight and air pollution”, Atmospheric Environment, Vol. 40/8, pp. 1561-1562, http://dx.doi.org/10.1016/j.atmosenv.2005.11.039.
[38] CCAC (2016), Cleaning up the Global On-road Diesel Fleet, Climate and Clean Air Coalition, UNEP.
[62] Chan, Y. et al. (2008), “Apportionment of sources of fine and coarse particles in four major Australian cities by positive matrix factorisation”, Atmospheric Environment, Vol. 42/2, pp. 374-389, http://dx.doi.org/10.1016/j.atmosenv.2007.09.030.
[63] Cheng, Y. et al. (2015), “PM2.5 and PM10-2.5 chemical composition and source apportionment near a Hong Kong roadway”, Particuology, Vol. 18, pp. 96-104, http://dx.doi.org/10.1016/j.partic.2013.10.003.
[16] Del Duce, A., M. Gauch and H. Althaus (2016), “Electric passenger car transport and passenger car life cycle inventories in ecoinvent version 3”, International Journal of Life Cycle Assessment, Vol. 21/9, pp. 1314-1326, http://dx.doi.org/10.1007/s11367-014-0792-4.
[26] DICTUC (2007), Actualización del Inventario de Emisiones de Contaminantes Atmosféricos en la Región Metropolitana: Escenario 2010, Report to the Comisión Nacional del Medio Ambiente Región Metropolitana, Santiago, Chile, https://www.cepal.org/ilpes/noticias/paginas/3/36023/DICTUC2007-Inventario2010.pdf (accessed on 20 May 2019).
[13] Electro-mobility, P. (2016), “Briefing: Non-Exhaust Emissions of Electric Cars”, http://dx.doi.org/10.1016/j.atmosenv.2016.03.017.
[52] Gildemeister, A., P. Hopke and E. Kim (2007), “Sources of fine urban particulate matter in Detroit, MI”, Chemosphere, Vol. 69/7, pp. 1064-1074, http://dx.doi.org/10.1016/j.chemosphere.2007.04.027.
[44] Griffin, R. et al. (1999), “Organic aerosol formation from the oxidation of biogenic hydrocarbons”, Journal of Geophysical Research Atmospheres, Vol. 104/D3, pp. 3555-3567, http://dx.doi.org/10.1029/1998JD100049.
[65] Gummeneni, S. et al. (2011), “Source apportionment of particulate matter in the ambient air of Hyderabad city, India”, Atmospheric Research, Vol. 101/3, pp. 752-764, http://dx.doi.org/10.1016/j.atmosres.2011.05.002.
[66] Guttikunda, S. et al. (2013), “Receptor model-based source apportionment of particulate pollution in Hyderabad, India”, Environmental Monitoring and Assessment, Vol. 185/7, pp. 5585-5593, http://dx.doi.org/10.1007/s10661-012-2969-2.
[39] Hendriks, C. et al. (2013), “The origin of ambient particulate matter concentrations in the Netherlands”, Atmospheric Environment, Vol. 69, pp. 289-303, http://dx.doi.org/10.1016/j.atmosenv.2012.12.017.
[10] Hooftman, N. et al. (2016), “Environmental analysis of petrol, diesel and electric passenger cars in a Belgian urban setting”, Energies, Vol. 9/2, pp. 1-24, http://dx.doi.org/10.3390/en9020084.
[12] Huo, H. et al. (2015), “Life-cycle assessment of greenhouse gas and air emissions of electric vehicles: A comparison between China and the U.S.”, Atmospheric Environment, Vol. 108, pp. 107-116, http://dx.doi.org/10.1016/j.atmosenv.2015.02.073.
[20] Huo, H. et al. (2013), “Climate and environmental effects of electric vehicles versus compressed natural gas vehicles in China: A life-cycle analysis at provincial level”, Environmental Science and Technology, Vol. 47/3, pp. 1711-1718, http://dx.doi.org/10.1021/es303352x.
[72] IEA (2019), Global EV Outlook 2019: Scaling-up the transition to electric mobility, IEA, Paris, https://webstore.iea.org/download/direct/2807?fileName=Global_EV_Outlook_2019.pdf (accessed on 17 June 2019).
[2] IEA (2018), Global EV Outlook 2018: Towards Cross-Modal Electrification, IEA, Paris, https://doi.org/10.1787/9789264302365-en (accessed on 18 June 2018).
[3] IEA (2018), Global EV Outlook 2018: Towards cross-modal electrification, International Energy Agency, Paris, https://dx.doi.org/10.1787/9789264302365-en.
[19] Kantor, I. et al. (2010), “Air quality and environmental impacts of alternative vehicle technologies in Ontario, Canada”, International Journal of Hydrogen Energy, Vol. 35/10, pp. 5145-5153, http://dx.doi.org/10.1016/j.ijhydene.2009.08.071.
[5] Ke, W. et al. (2017), “Well-to-wheels energy consumption and emissions of electric vehicles: Mid-term implications from real-world features and air pollution control progress”, Applied Energy, Vol. 188, pp. 367-377, http://dx.doi.org/10.1016/j.apenergy.2016.12.011.
[47] Kim, E. and P. Hopke (2004), “Improving source identification of fine particles in a rural northeastern U.S. area utilizing temperature-resolved carbon fractions”, Journal of Geophysical Research D: Atmospheres, Vol. 109/9, http://dx.doi.org/10.1029/2003JD004199.
[49] Kim, E., P. Hopke and E. Edgerton (2004), “Improving source identification of Atlanta aerosol using temperature resolved carbon fractions in positive matrix factorization”, Atmospheric Environment, Vol. 38/20, pp. 3349-3362, http://dx.doi.org/10.1016/j.atmosenv.2004.03.012.
[48] Kim, E. et al. (2003), “Incorporation of parametric factors into multilinear receptor model studies of Atlanta aerosol”, Atmospheric Environment, Vol. 37/36, pp. 5009-5021, http://dx.doi.org/10.1016/j.atmosenv.2003.08.035.
[53] Lee, J. and P. Hopke (2006), “Apportioning sources of PM2.5 in St. Louis, MO using speciation trends network data”, Atmospheric Environment, Vol. 40/SUPPL. 2, pp. 360-377, http://dx.doi.org/10.1016/j.atmosenv.2005.11.074.
[15] Ligterink, N., U. Stelwagen and J. Kuenen (2014), Emission factors for alternative drivelines and alternative fuels, http://dx.doi.org/10.13140/RG.2.1.2655.4483.
[36] Li, N. et al. (2016), “Potential impacts of electric vehicles on air quality in Taiwan”, Science of the Total Environment, Vol. 566-567, pp. 919-928, http://dx.doi.org/10.1016/j.scitotenv.2016.05.105.
[21] Nichols, B., K. Kockelman and M. Reiter (2015), “Air quality impacts of electric vehicle adoption in Texas”, Transportation Research Part D: Transport and Environment, Vol. 34, pp. 208-218, http://dx.doi.org/10.1016/j.trd.2014.10.016.
[18] Nopmongcol, U. et al. (2017), “Air Quality Impacts of Electrifying Vehicles and Equipment Across the United States”, Environmental Science and Technology, Vol. 51/5, pp. 2830-2837, http://dx.doi.org/10.1021/acs.est.6b04868.
[57] Ntziachristos, L. and P. Boulter (2016), “1.A.3.b.vi Road transport: Automobile tyre and brake wear; 1.A.3.b.vii Road transport: Automobile road abrasion”, in EMEP/EEA air pollutant emission inventory guidebook 2016, Publications Office of the European Union, Luxembourg.
[37] Ntziachristos, L. and Z. Samaras (2018), “1.A.3.b.i, 1.A.3.b.ii, 1.A.3.b.iii, 1.A.3.b.iv Passenger cars, light commercial trucks, heavy-duty vehicles including buses and motor cycles”, in EMEP/EEA air pollutant emission inventory guidebook 2016 – Update Jul. 2018, https://www.eea.europa.eu/publications/emep-eea-guidebook-2016/part-b-sectoral-guidance-chapters/1-energy/1-a-combustion/1-a-3-b-i.
[41] OICA (2020), Production Statistics, http://www.oica.net/production-statistics/ (accessed on 23 April 2020).
[24] Pachón, J. et al. (2018), “Development and evaluation of a comprehensive atmospheric emission inventory for air quality modeling in the megacity of Bogotá”, Atmosphere, Vol. 9/2, http://dx.doi.org/10.3390/atmos9020049.
[28] Padoan, E. and F. Amato (2018), “Vehicle Non-Exhaust Emissions: Impact on Air Quality”, in Amato, F. (ed.), Non-Exhaust Emissions: An Urban Air Quality Problem for Public Health; Impact and Mitigation Measures, Academic Press, London, UK, http://dx.doi.org/10.1016/B978-0-12-811770-5.00002-9.
[22] Peng, W. et al. (2018), “Potential co-benefits of electrification for air quality, health, and CO 2 mitigation in 2030 China”, Applied Energy, Vol. 218/October 2017, pp. 511-519, http://dx.doi.org/10.1016/j.apenergy.2018.02.048.
[67] Perrone, M. et al. (2012), “Sources of high PM2.5 concentrations in Milan, Northern Italy: Molecular marker data and CMB modelling”, Science of the Total Environment, Vol. 414, pp. 343-355, http://dx.doi.org/10.1016/j.scitotenv.2011.11.026.
[46] Platt, S. et al. (2017), “Gasoline cars produce more carbonaceous particulate matter than modern filter-equipped diesel cars”, Scientific Reports, Vol. 7/1, http://dx.doi.org/10.1038/s41598-017-03714-9.
[33] Razeghi, G. et al. (2016), “Episodic air quality impacts of plug-in electric vehicles”, Atmospheric Environment, Vol. 137, pp. 90-100, http://dx.doi.org/10.1016/j.atmosenv.2016.04.031.
[1] Requia, W. et al. (2018), “How clean are electric vehicles? Evidence-based review of the effects of electric mobility on air pollutants, greenhouse gas emissions and human health”, Atmospheric Environment, Vol. 185/May, pp. 64-77, http://dx.doi.org/10.1016/j.atmosenv.2018.04.040.
[29] Requia, W. et al. (2018), “How clean are electric vehicles? Evidence-based review of the effects of electric mobility on air pollutants, greenhouse gas emissions and human health”, Atmospheric Environment, Vol. 185, pp. 64-77, http://dx.doi.org/10.1016/J.ATMOSENV.2018.04.040.
[25] Secretaria del Medio Ambiente de la Ciudad de Mexico (2018), “Inventario de Emisiones de la Ciudad de Mexico 2016”, p. 111, http://www.sedema.cdmx.gob.mx.
[42] Shrivastava, M. et al. (2007), “Sources of organic aerosol: Positive matrix factorization of molecular marker data and comparison of results from different source apportionment models”, Atmospheric Environment, Vol. 41/40, pp. 9353-9369, http://dx.doi.org/10.1016/j.atmosenv.2007.09.016.
[11] Soret, A., M. Guevara and J. Baldasano (2014), “The potential impacts of electric vehicles on air quality in the urban areas of Barcelona and Madrid (Spain)”, Atmospheric Environment, Vol. 99, pp. 51-63, http://dx.doi.org/10.1016/j.atmosenv.2014.09.048.
[64] Srimuruganandam, B. and S. Shiva Nagendra (2012), “Application of positive matrix factorization in characterization of PM<inf>10</inf>and PM<inf>2.5</inf>emission sources at urban roadside”, Chemosphere, Vol. 88/1, http://dx.doi.org/10.1016/j.chemosphere.2012.02.083.
[35] Tessum, C., J. Hill and J. Marshall (2014), “Life cycle air quality impacts of conventional and alternative light-duty transportation in the United States”, Proceedings of the National Academy of Sciences, Vol. 111/52, pp. 18490-18495, http://dx.doi.org/10.1073/pnas.1406853111.
[8] Timmers, V. and P. Achten (2018), “Non-Exhaust PM Emissions From Battery Electric Vehicles”, in Non-Exhaust Emissions, http://dx.doi.org/10.1016/b978-0-12-811770-5.00012-1.
[9] Timmers, V. and P. Achten (2016), “Non-exhaust PM emissions from electric vehicles”, Atmospheric Environment, Vol. 134, pp. 10-17, http://dx.doi.org/10.1016/j.atmosenv.2016.03.017.
[34] Tobollik, M. et al. (2016), “Health impact assessment of transport policies in Rotterdam: Decrease of total traffic and increase of electric car use”, Environmental Research, Vol. 146, pp. 350-358, http://dx.doi.org/10.1016/j.envres.2016.01.014.
[27] U.S. Environmental Protection Agency (2019), National Emissions Inventory (NEI), https://www.epa.gov/air-emissions-inventories/national-emissions-inventory-nei (accessed on 19 May 2019).
[7] Van Zeebroeck, B. and G. De Ceuster (2013), Elektrische auto zorgt voor evenveel fijn stof als conventionele auto.
[45] Vidhi, R. and P. Shrivastava (2018), “A review of electric vehicle lifecycle emissions and policy recommendations to increase EV penetration in India”, Energies, Vol. 11/3, http://dx.doi.org/10.3390/en11030483.
[50] Wang, Y. et al. (2012), “Multiple-year black carbon measurements and source apportionment using Delta-C in Rochester, New York”, Journal of the Air and Waste Management Association, Vol. 62/8, pp. 880-887, http://dx.doi.org/10.1080/10962247.2012.671792.
[23] Wu, Y. and L. Zhang (2017), “Can the development of electric vehicles reduce the emission of air pollutants and greenhouse gases in developing countries?”, Transportation Research Part D: Transport and Environment, Vol. 51, pp. 129-145, http://dx.doi.org/10.1016/j.trd.2016.12.007.
[31] Zhang, Q. et al. (2007), “Ubiquity and dominance of oxygenated species in organic aerosols in anthropogenically-influenced Northern Hemisphere midlatitudes”, Geophysical Research Letters, Vol. 34/13, http://dx.doi.org/10.1029/2007GL029979.
[51] Zhao, W. and P. Hopke (2006), Source investigation for ambient PM 2.5 in Indianapolis, IN, http://dx.doi.org/10.1080/02786820500380297.
[43] Zhao, Y. et al. (2013), “Sources of organic aerosol investigated using organic compounds as tracers measured during CalNex in Bakersfield”, Journal of Geophysical Research Atmospheres, Vol. 118/19, pp. 11388-11398, http://dx.doi.org/10.1002/jgrd.50825.
[30] Ziemann, P. and R. Atkinson (2012), Kinetics, products, and mechanisms of secondary organic aerosol formation, http://dx.doi.org/10.1039/c2cs35122f.
Notes
← 1. The vast majority of studies have focused on CO2 emissions (51 papers), followed by NOx (32 papers), VOCs (18 papers), SO2 (17 papers), and CO (15 papers).
← 2. A number of studies have evaluated the impact of EV uptake on well-to-wheel and lifecycle PM emissions (Peng et al., 2018[22]; Kantor et al., 2010[19]; Tessum, Hill and Marshall, 2014[35]; Huo et al., 2015[12]). This report focuses on particulate emissions during a vehicle’s use phase, i.e. only the particulate matter emitted when the vehicle is being driven.
← 3. These estimates are characterised by uncertainty on the order of ±50% (Ntziachristos and Boulter, 2016[57]). Ongoing activities by the UN GRPE-PMP (Particle Measurement Program) and the Horizon 2020 EU-funded LOWBRASYS project, as well as the California Air Resources Board, will soon produce updated estimates for brake wear emission factors.
← 4. Assuming an uptake of BEV300 vehicles and an average annual mileage of 15,000 km per vehicle.