This chapter analyses how digitalisation modifies the skills needed and tasks performed by workers. Technology can replace workers in routine tasks that are easy to automate and complement workers in more cognitively demanding tasks that require creativity and problem solving. To investigate these links between digitalisation and workers’ skills and tasks, this chapter uses a new set of empirical analyses based on the Survey of Adult Skills (PIAAC). To successfully navigate the transition to a digital world of work and thrive in it – workers need not only digital skills but also a broad mix of skills, including cognitive and socio-emotional skills. In technology-related occupations, advanced digital skills are necessary. The chapter also assesses the extent to which digital transformation may affect countries and occupations that are lagging behind.
OECD Skills Outlook 2019
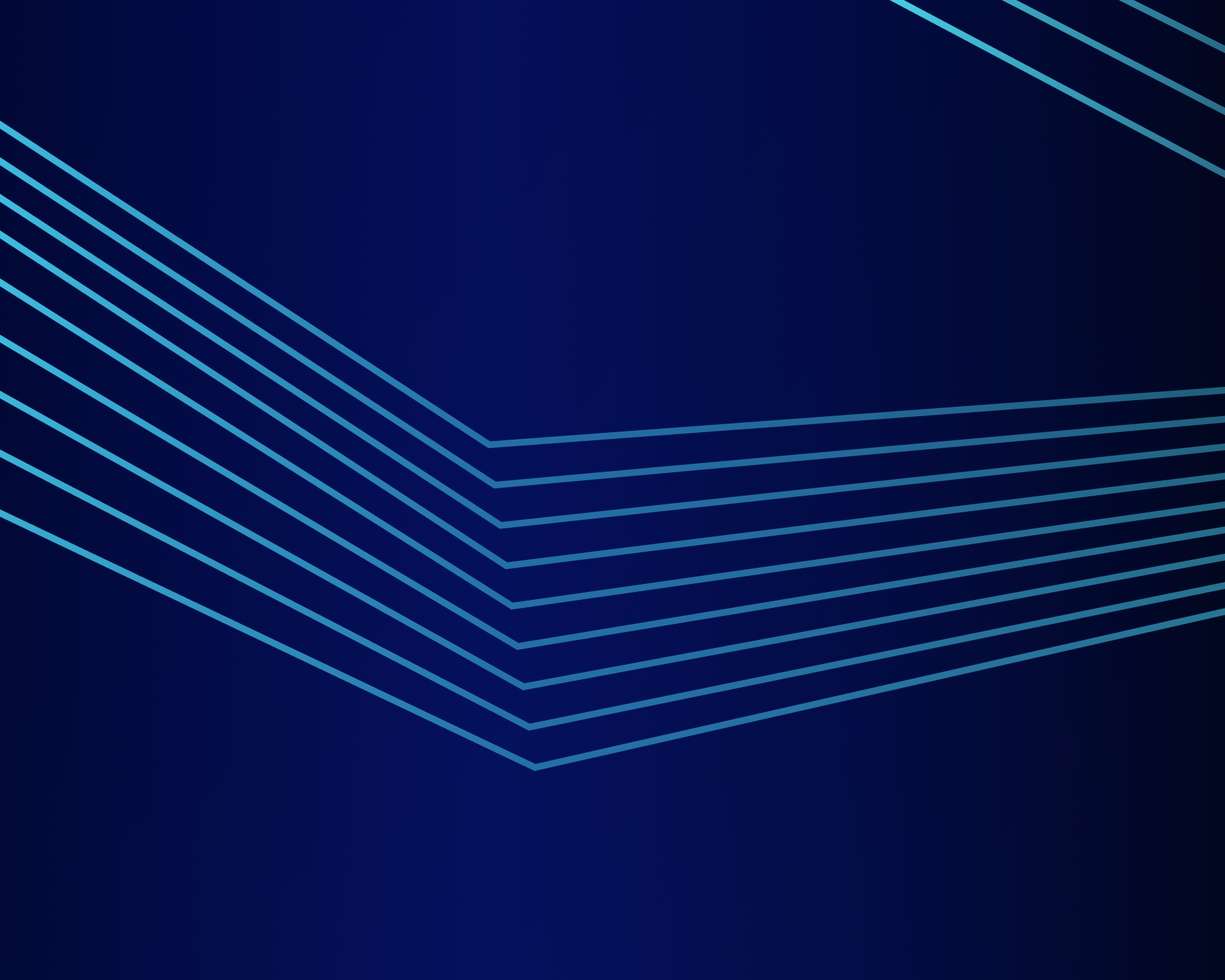
Chapter 2. A digital world of work: Transformations of occupations and the implications for skills needs
Abstract
The statistical data for Israel are supplied by and under the responsibility of the relevant Israeli authorities. The use of such data by the OECD is without prejudice to the status of the Golan Heights, East Jerusalem and Israeli settlements in the West Bank under the terms of international law.
Digitalisation profoundly changes the world of work: what people do on the job, how and where they work, the skills they need to remain in employment in a changing world, and the type of career progression they may have. Digital transformation involves many technologies and therefore changes the labour market in many different ways. The use of the Internet by most workers, the possibility of automating an increasing range of tasks and the opportunity to supply skills through online platforms have different implications for workers and require different policy approaches. The effects of digital transformation go beyond manufacturing sectors and traditional high-tech sectors and require much more than ensuring that all or some workers have information and communication technology (ICT) skills.
This chapter examines how digital transformation affects the world of work, with a specific focus on skills. It analyses how new technologies change i) skills requirements, and ii) the use and development of skills. A large part of the analysis has been carried out at the level of occupations, which appears to be the most relevant level for examining the implications of the digital transformation for skills needs, and in turn, for skills-related policies.
Digital transformation changes occupations in three major ways that have implications for skills demand and supply, and for workers:
New technologies transform occupations. Some tasks are automated, others are done differently as technology complements workers in their tasks. Overall, the tasks carried out in each occupation change and therefore, the demand for skills also changes. These changes affect most workers who have to adapt their skills sets.
New technologies make some occupations less needed in the economy. In some occupations, most tasks may be automatable, rendering these occupations more likely to disappear in the future. Workers in these occupations may need to change occupations to remain in employment, which would require them to adapt their skills sets and perhaps acquire new skills and knowledge. While assessments of the risk of automation vary, along with the number of jobs that may disappear, policies are needed that prepare workers for this risk.
New technologies create new occupations and new ways to supply skills to the market. New occupations develop that directly involve new technologies (e.g. big data specialists). Preferences for well-being and leisure may change, leading to the expansion of other occupations (e.g. sport coaches). Online platforms lead to major changes in some sectors but also facilitate self-employment for a broader range of workers. People need to have the skills to benefit from these new work opportunities.
Overall, to make the most of digital transformation, policies need to ensure that workers have and develop the skills to both adapt to changes within occupations and navigate between occupations. In other words, people need to have the skills to be resilient and mobile.
This chapter discusses the transformations of occupations and their implications for skills needs. Chapter 3 investigates how training policies can facilitate mobility between occupations.
The main findings of this chapter are:
There are many facets to digital transformation, from the development of robots to the platform economy. They affect workers differently depending on the country, sector and firm they work in. The adoption of new technologies leads to both substitution and complementarity effects. Technology replaces workers in the performance of some tasks that can be automated, such as routine tasks (substitution). Workers use technology, such as ICT tools, to perform their tasks differently and perhaps more efficiently (complementarity).
Workers need to have the right mix of skills to successfully navigate the transition to the digital world of work, and thrive in it. This mix of skills includes, first, strong general cognitive skills, such as literacy, numeracy and basic ICT skills. It also includes analytical skills and a range of complementary skills such as problem solving, creative and critical thinking, communication skills and a strong ability to continue learning. Working in growing occupations linked to new technologies requires advanced ICT skills such as coding skills.
This chapter provides a range of evidence supporting the need for a mix of skills, and not only for digital skills, to work in a digital environment:
Workers in more digital work environments tend to perform more frequently all the tasks considered in the analysis – managing and communicating, accountancy and selling, and tasks involving advanced numeracy skills. Workers more exposed to digitalisation also make a greater use of general cognitive skills and perform more tasks at work that are related to reading, writing and numeracy.
Workers in growing occupations exposed to technology (e.g. software and application developers, database professionals, and ICT support technicians) perform sets of tasks and have levels of skills that are similar to those of workers in other occupations requiring similar education levels, except that they also need advanced ICT skills such as coding.
In sectors that are more digital intensive, skills get a wage premium compared with other sectors, but this premium is as big for ICT skills as for numeracy skills or management and communication skills.
Workers are more likely to maintain their skills in workplaces more exposed to digitalisation. Comparing workers with similar characteristics but differing in terms of the digitalisation of their work environments suggests digital environments help workers to retain problem-solving skills in technology-rich environments while non-digital environments may lead to skill obsolescence. Digital transformation affects sectors and firms differently. It may exacerbate the skills gap and inequalities between workers in sectors and firms (mainly large firms) at the forefront of technology adoption and those in sectors and firms lagging behind.
Online labour platforms may give workers opportunities to find jobs they would not have had otherwise, but flows have been asymmetric. The United States has been the major hiring country and Asia (South and Southeast Asia) a major provider of services. Social protection and labour rights of workers using these platforms need to be respected. It is important to ensure that i) workers in the “gig economy” also benefit from training opportunities and ii) firms looking for skills through online labour platforms do not consequently neglect investment in training for employees. The development of online labour platforms may require rethinking the allocation of responsibility for workers’ training between platforms, employers and employees.
Countries will continue to face significant labour market changes that have implications for skills. Among countries covered by the Survey of Adult Skills (PIAAC), a group of countries (Chile, Greece and Turkey) are lagging behind, suggesting their workers are likely to face major changes to their jobs’ task-content in the coming years, in particular a substantially greater use of technology, and may not have the skills required to face this transformation. Conversely, other countries (Denmark, Sweden and the Netherlands) are ahead in the digital transformation of the workplace, with most of their workers intensively using ICTs on the job and predominantly performing non-routine tasks.
Low-skilled occupations such as services and sales workers, plant machine operators, or elementary occupations exhibit considerable variation among workers in their exposure to digitalisation, according to the two considered indicators. In some of these occupations, machines may take over the performance of routine tasks. In other occupations, workers who do not currently use computers may be required to start using them. The nature of these low-skilled occupations may therefore change. Workers in these occupations will have to adapt their skills mix to changing needs. In contrast, for high-skilled occupations such as managers, professionals or technicians, the exposure to digitalisation differs significantly less from worker to worker, suggesting these occupations will probably experience fewer changes, unless unforeseen technological disruptions emerge.
To thrive in the digital economy, workers will need more than just ICT skills. They will also need complementary skills, ranging from good literacy and numeracy skills to the socio-emotional skills required to work collaboratively and flexibly. As a result, a first policy implication is to ensure all individuals acquire a good level of skills proficiency in initial education so they can develop these skills further over their lifetime as well as learn new skills along the way. Reducing the share of young people leaving education with low basic skills becomes increasingly important in a digital world of work. In addition, vocational education and training programmes need to continue to develop general cognitive skills in addition to job-related specific skills. University programmes need to enable students to develop a broader set of skills, including social and emotional skills, in addition to skills related to their fields of study.
Digital transformation brings with it a crucial need for high-quality and accessible training as skills needs change rapidly. Chapter 3 explores these questions in greater depth.
Recent changes in the world of work
Digital transformation affects most workers in OECD countries. Ubiquitous digital devices, connectivity, software and data are profoundly changing the organisation of production, the organisation within firms, what people do on the job and the way they work. An ever-growing share of people will have to work with technology.
In 2015, 57% of European Union workers regularly used a computer or smartphone at work, a surge from 36% only 10 years earlier (Eurofound, 2017[1]). The Survey of Adult Skills (PIAAC), which was administered in 2012 or 2015 depending on countries, also shows that a majority of workers were using a computer (Figure 2.1). However, it shows that large differences existed between countries in the share of workers using the Internet, email and software.
Figure 2.1. Use of computers, the Internet and software at work
Sources: OECD calculations based on OECD (2012[1]) and OECD (2015[2]), Survey of Adult Skills (PIAAC), www.oecd.org/skills/piaac/publicdataandanalysis.
Workers increasingly use technology on the job. Even those who do not use technology may find the nature of their work changing as tasks are increasingly automated. Digitalisation also creates risks that jobs will disappear. Progress in artificial intelligence and machine learning generates possibilities to automate parts of the production process by creating intelligent machines that work, learn and react like humans. The deployment of industrial robots has transformed production processes, particularly in the manufacturing sector, attracting a lot of attention from the media and the public. There are large variations among countries in the use of robots, however, reflecting differences in the adoption of technology (Figure 2.2).
Digital transformation has many facets, which are difficult to summarise in a single indicator. It entails investing in and developing the most advanced “digital” tools, embedding them in production with the help of workers with appropriate skills, and using them when dealing with clients and suppliers (Calvino et al., 2018[3]). When the multidimensional nature of digital transformation is taken into account, it becomes clear that the uptake of digital technologies also differs substantially across sectors (Figure 2.3). Advanced adopters include not only the ICT sector, as one might expect, but also some service sectors such as finance and insurance. In addition, while most firms in the OECD now have access to broadband networks, there are large differences among them in the use of more advanced digital technologies, in particular by SMEs (Andrews, Nicoletti and Timiliotis, 2018[4]).
Figure 2.2. Top robot-intensive economies and BRICS
Note: Robot use collected by the International Federation of Robotics (IFR) is measured as the number of robots purchased by a given country/industry. Robot stock is constructed by taking the initial IFR stock starting value, then adding to it the purchases of robots from subsequent years with a 10% annual depreciation rate. The graph covers all manufacturing, mining and utilities sectors. Data for the following countries is extrapolated for the years 2014 and 2015 due to the lack of data: Australia, Chile, Estonia, Finland, Greece, Iceland, Ireland, Latvia, Lithuania, New Zealand, Norway and Slovenia. Due to lack of available data, the OECD average excludes Canada, Israel, Luxembourg and Mexico. The EU28 average excludes Cyprus and Luxembourg.
Source: OECD (2017[5]), OECD Science, Technology and Industry Scoreboard 2017: The digital transformation, http://dx.doi.org/10.1787/9789264268821-en.
The emergence of online platforms also profoundly changes the world of work. Companies such as Amazon, Facebook, Google and Uber have created online structures that enable a wide range of work activities. Those platforms are diverse in nature and therefore affect labour markets differently. Google is used by many workers to look for information that was more difficult to access in the past. Some platforms (Upwork, Uber) enable anybody to offer their skills in the labour market, often outside standard labour contracts and in addition to other labour activities. People use other platforms to signal skills and create networks (LinkedIn, Facebook). Job-matching sites such as LinkedIn and Monster are changing the way people look for work and the way companies identify and recruit talent.
Digital platforms lead to the reorganisation of a wide range of markets, labour relations and contracts, and ultimately of value creation and capture (Kenney and Zysman, 2016[6]). For instance, Uber has deeply changed the organisation of the taxi industry. The rise of the platform economy raises questions about online workers’ social protection and rights, as well as their ability to access and pay for continuing education and training. If the share of workers whose main activity is performed on online platforms increased, the relationship between workers and employers would become more tenuous, reducing employers’ involvement in training policies. At the same time, most workers can access online training platforms that provide courses for free (Chapter 4).
Figure 2.3. Dispersion of sectors in each considered dimension of digitalisation
Note: All underlying indicators are expressed as sectoral intensities. For each indicator, the sectoral values are averages across countries and years. These values are then standardised relative to the mean, such that the resulting series by indicator have mean zero and standard deviation 1.
Source: OECD (2017[5]), OECD Science, Technology and Industry Scoreboard 2017: The digital transformation, http://dx.doi.org/10.1787/9789264268821-en.
Substitution and complementarity between technology and skills: understanding the effects
Technology enables workers to perform some tasks more effectively, complementing their skills. For instance, managers use computer software to organise the work of their team, monitor outcomes, and enhance their collective productivity. Scanners substantially increase cashiers’ speed and efficiency. While technology can increase the productivity of both high-skilled and low-skilled workers, it generally favours skilled over unskilled labour by increasing its relative productivity (Acemoglu, 1998[7]; Autor, Katz and Krueger, 1998[8]). New information technologies tend to complement skilled labour, an effect known as skill-biased technological change.
Technology also replaces workers. Computers, software and robots can do routine tasks, such as those involving manual activities (assembling, packing and mail sorting) and those that can be accomplished by following well-defined explicit rules (Autor, Levy and Murnane, 2003[9]).
Overall, digitalisation affects workers in two main ways:
A complementarity effect: Technology enables workers to do tasks – such as searching for information or communicating with colleagues or clients – differently and perhaps more efficiently. An increasing share of workers doing problem-solving and communication tasks use ICT tools on the job.
A substitution effect: Technology replaces workers in the performance of some tasks that can be automated. As routine tasks are easier to automate, the substitution effect decreases the routine intensity of jobs.
With digitalisation, workers perform more non-routine, ICT-intensive tasks, and fewer non-routine tasks with no use of technology, as illustrated in Figure 2.4 by the move from (A) to (B). Workers can be complemented by computers in performing routine tasks, though it is likely that these tasks will eventually be wholly automated. Increasingly, computers complement workers in non-routine tasks, while some routine tasks that do not involve ICTs continue to be performed by workers, for instance those involving face-to-face contact. Overall, workers then tend to perform more non-routine, ICT-intensive tasks or routine, non-ICT intensive tasks, as illustrated by the move from (A) to (C).
Figure 2.4. The impact of digitalisation on tasks performed on the job: A framework
Digitalisation of work changes skills requirements and skills development. As some tasks are automated (substitution effect), some skills are needed less while others become more important. As workers use technology (complementarity effect), they work differently, which affects the way they develop their skills. There is a continuous, self-reinforcing dynamic between workers’ skills and tasks performed (Heckman and Corbin, 2016[10]; Cavounidis and Lang, 2017[11]). Technology is modifying the sets of tasks that workers perform, and complementing their unique skillsets – but not substituting humans totally (Box 2.1).
Box 2.1. Substitution and complementarity between digitalisation, tasks and skills: Some illustrative examples
Though these are still early days for some of the most advanced technology, such as artificial intelligence (AI), examples ranging from low-skilled to high-skilled occupations suggest that technology has led to both substitution and complementarity effects but is not replacing humans entirely. Rather it is modifying the task composition of workers, and reinforcing and complementing their unique skillset.
Warehouse and production chain workers
Robots have been adopted in many industries, but the most automated systems still tend to lack flexibility. Modern automobile plants, for example, employ industrial robots to install windshields on new vehicles as they move through the assembly line. Yet windshield replacement companies employ technicians rather than robots to change broken windshields. Amazon has automated part of its warehouse and robots handle routine tasks such as moving shelves. However, the firm continues to employ many workers to locate and handle merchandise, and label and ship goods. In many situations, automation is not suitable for a flexible environment, so workers’ greater adaptability gives them a comparative advantage over robots.
Cashiers
Since the introduction of self-checkout machines, the role of cashiers has been moving towards assisting customers. Their role can now consist in helping customers properly use the self-checkout machines as well as monitor that the machines are functioning correctly (Andrews, 2009[12]). Cashiers are also redeployed within the store to other tasks such as customer service and sales. However, shops generally continue to offer traditional cashier-staffed checkouts alongside self-checkouts because some people prefer to interact with a human being than with a machine. This societal value attached to human relationships gives workers a new type of comparative advantage over machines, even in low-skilled occupations that technically could be automated. For similar reasons, it is likely that jobs such as nursing or caring for the elderly will remain labour-intensive though these are so far less easily automatable.
Bank tellers
In the United States, the number of automated teller machines has more than quadrupled between 1990 and the 2010s (Bessen, 2016[13]). However, this expansion has not resulted in a decline in the number of bank tellers. Instead, the automation of routine cash-handling tasks has modified the role of bank tellers, who now focus predominantly on fostering customer relationships. Bank tellers are expected to be salespersons rather than checkout clerks (Autor, 2015[14]). The emergence of automated teller machines has changed the content of bank tellers’ jobs. Technology substitutes these workers in performing routine tasks, and complements them in the performance of non-routine tasks.
Journalists
Like many professions, journalism has been radically transformed by digitalisation (Örnebring, 2010[15]). In addition to compelling newspapers and magazines to reinvent their business models, technology has altered the practice of journalists themselves. In recent years, sophisticated AI software has been used by newsrooms to automate the writing of stories, to identify breaking news from eyewitnesses, as well as to help journalists in their data-driven reporting (Carlson, 2015[16]). For example, the Los Angeles Times uses a computer program named Quakebot to automatically report on earthquakes, Reuters employs News Tracer to give an edge to its journalists in identifying breaking news, and The Washington Post’s Heliograf has been autonomously writing articles since the 2016 Rio Olympics (Underwood, 2017[17]). Automated journalism is still in its infancy, yet case studies suggest human journalists and “robot journalists” can complement each other: robots can easily report on a multitude of simple events (sports games, polling data, crime, etc.), while humans can focus on creative and detailed stories (Van Dalen, 2012[18]).
Translators
Machine translation has existed for decades. Over the past half-decade, however, advances in various fields of AI, and in particular in artificial neural networks, have vastly improved the accuracy of translating services (Gideon, 2016[19]; Larousserie, 2017[20]). For example, the AI-enabled version of Google Translate launched in late 2016 was able to improve overnight roughly as much as the previous version had over its entire existence (Gideon, 2016[19]). These monumental achievements are likely to significantly disrupt the work of translators, perhaps for the better. As software becomes more precise, translators will be able to rely on it for the first draft and focus on using the special language skills that software lacks (at least for the moment) to improve the style or flow of the translation, identify idioms and translating them accordingly, recognise humour or sarcasm, and ensure it will be understood accordingly.
Lawyers
Some of the tasks performed by lawyers are already being replaced or facilitated by machines (Williams, 2016[21]). Kira, a machine learning-enabled program that analyses legal contracts, “reduces the lawyer time required for contract review by 20 percent to 60 percent”, according to the chief executive of the company that produces it (Lohr, 2017[22]). Another program called Ross Intelligence, a legal application of IBM’s Watson, can answer legal questions with a two-page memo overnight (Lohr, 2017[22]; Remus and Levy, 2016[23]). As with translators, human lawyers improve upon the machine’s first draft. Using data on lawyer time usage in 2014 in large law firms, one study estimated that if all existing technologies were immediately adopted by law firms, the number of hours worked would be reduced by 13% (Remus and Levy, 2016[23]). A more realistic adoption period of five years decreases that number to 2.5% annually. These estimates are small because many tasks undertaken by lawyers require either technical, unstructured knowledge (legal writing, document management) or interpersonal and emotional skills (advising clients, court appearances). The time savings from technology will likely be accompanied by only minor labour savings. Greater productivity from the use of AI-enabled technologies may in fact expand demand for legal advice as costs fall.
Radiologists
Computer-aided diagnosis (CAD) “has become a part of the routine clinical work for detection of breast cancer on mammograms at many screening sites and hospitals in the United States,” (Shiraishi et al., 2011[24]). CAD does not replace radiologists or dermatologists in detecting lesions and assessing the extent of the disease, but it does give them a second opinion with which to improve and adjust their initial diagnosis (Deepa and Aruna Devi, 2011[25]; Doi, 2007[26]). This assistance is becoming substantially more reliable with the increasing availability of large medical datasets. On average, a full-time dermatologist might see about 200 000 cases over her career. By contrast, an algorithm based on artificial neural networks built by Stanford researchers could analyse nearly 130 000 cases in three months (Mukherjee, 2017[27]). This algorithm was found to perform on a par with expert dermatologists in detecting skin cancer (Esteva et al., 2017[28]). Algorithms will not be replacing oncologists in the near future, however. Doctors will continue to be needed to understand the conclusions of the program, to evaluate potential causes for the diagnosis, and to interact with patients. Moreover, ethical considerations surrounding the exclusive use of technology cannot be brushed aside easily: who would become liable in the case of an incorrect diagnosis by the machine? The better performance of machines in some diagnoses will likely improve the judgement of radiologists rather than replace them.
Sources: Andrews, C. (2009[12]), 'Do-It-Yourself': Self-checkouts, Supermarkets, and the Self-Service Trend in American Business, https://drum.lib.umd.edu/handle/1903/9593?show=full (accessed on 03 November 2017); Bessen, J. (2016[13]), “How computer automation affects ocupations: Technology, jobs, and skills”, http://dx.doi.org/10.2139/ssrn.2690435; Autor, D. (2015[14]), “Why are there still so many jobs? The history and future of workplace automation”, http://dx.doi.org/10.1257/jep.29.3.3; Örnebring, H. (2010[15]), “Technology and journalism‑as‑labour: Historical perspectives”, http://dx.doi.org/10.1177/1464884909350644; Carlson, M. (2015[16]), “The robotic reporter”, http://dx.doi.org/10.1080/21670811.2014.976412; Underwood, C. (2017[17]), Automated Journalism - AI Applications at New York Times, Reuters, and Other Media Giants, https://www.techemergence.com/automated‑journalism‑applications/ (accessed on 11 December 2017); Van Dalen, A. (2012[18]), “The algorithms behind the headlines”, http://dx.doi.org/10.1080/17512786.2012.667268; Gideon, L. (2016[19]), The Great A.I. Awakening, http://dx.doi.org/10.1002/cne.21974; Larousserie, D. (2017[20]), La traduction dopée par l’intelligence artificielle, http://www.lemonde.fr/sciences/article/2017/11/27/la‑traduction‑dopee‑par‑l‑intelligence‑artificielle_5221041_1650684.html?xtmc=la_traduction_dopee_par_l_intelligence_artificielle&xtcr=1 (accessed on 12 December 2017); Williams, M. (2016[21]), “'Moneyball for lawyers’: How technology will change the practice of law”, https://www.lawsocietysa.asn.au/bulletin/BULL_Moneyball_for_lawyers.PDF (accessed on 13 December 2017); Lohr, S. (2017[22]), A.I. Is Doing Legal Work. But It Won’t Replace Lawyers, Yet, https://www.nytimes.com/2017/03/19/technology/lawyers-artificial-intelligence.html (accessed on 12 December 2017); Remus, D. and F. Levy (2016[23]), “Can robots be lawyers? Computers, lawyers, and the practice of law”, http://dx.doi.org/10.2139/ssrn.2701092; Shiraishi, J. et al. (2011[24]), “Computer‑aided diagnosis and artificial intelligence in clinical imaging”, http://dx.doi.org/10.1053/J.SEMNUCLMED.2011.06.004; Deepa, S. and B. Aruna Devi (2011[25]), “A survey on artificial intelligence approaches for medical image classification”, http://52.172.159.94/index.php/indjst/article/view/30291/26223 (accessed on 14 December 2017); Doi, K. (2007[26]), “Computer-aided diagnosis in medical imaging: Historical review, current status and future potential”, http://dx.doi.org/10.1016/j.compmedimag.2007.02.002; Mukherjee, S. (2017[27]), A.I. Versus M.D., https://www.newyorker.com/magazine/2017/04/03/ai-versus-md (accessed on 14 December 2017); Esteva, A. et al. (2017[28]), “Dermatologist-level classification of skin cancer with deep neural networks”, http://dx.doi.org/10.1038/nature21056.
The way technology enters the workplace is influenced by workers’ skills, managerial practices, and several other firm- and industry-specific factors that determine the organisation of work and production, and the capacity to change. Policies that affect the organisation of production play a key role in shaping the adoption of new technologies (Milgrom et al., 1990[29]). It is also important to adopt technology as part of an overall organisation package (Brynjolfsson, Renshaw and Van Alstyne, 1997[30]). Organisational structure, skills and competition have all been suggested as the factors that play the most important role in the adoption of technology (Gallego, Gutierrez and Lee, 2015[31]; Andrews, Nicoletti and Timiliotis, 2018[32])
Policies also have an important influence on the extent to which technology will predominantly substitute or complement workers’ skills, and thereby on the impact of digital transformation on employment levels and growth. Investments that complement technology itself include those in skills, in organisational change, in new processes and business models, and also in the intellectual assets that help create value from the new technologies (OECD, 2018[33]).
Up to now, there has been no consensus on the overall impact of digital transformation on employment, because it is difficult to account for the multifaceted aspects of digital transformation and because jobs will be both created and destroyed (Box 2.2). However, there is mounting evidence that it results in considerable restructuring of the labour market, thereby affecting the distribution of jobs, wages and income (OECD, 2018[33]). High-skilled workers have tended to benefit more from technological change as they have skills that complement technology in undertaking non-routine tasks, such as problem solving, or creative and complex communications activities. As a result, over the past two decades the share of employment in high-skilled jobs (and to some extent in low-skilled jobs) has increased in most OECD countries, while the share of employment in middle-skilled jobs has decreased.
In the long term, however, low-skilled workers are most likely to bear the costs of digital transformation. While some high skilled tasks also face a risk of automation, low-skilled workers look most likely to lose their jobs, face increased competition for jobs from middle-skilled workers, are least likely to be able to adapt to new technologies and working practices, and are also least likely to benefit from the new opportunities that arise as a result of digital transformation.
Disparities exist within countries as well as between them, as new jobs appear in places other than where they have been lost. Regions are not equally prepared to face the impact of digital transformation. In countries with important regional differences in skill endowments, fast-growing high-tech firms that create jobs are more likely to be concentrated in high-skilled regions. Low-skilled workers face bigger difficulties finding work in these regions, as they have to compete with more highly skilled workers. These geographic inequalities may exacerbate overall inequalities.
Box 2.2. Assessing the impact of digital transformation on employment
Digital transformation has many facets and involves a range of technologies that affect skills needs and jobs differently. Measuring the impact of digital transformation on employment is difficult. Most studies have focused on automation, which can come not only from the application of AI but also from other types of technologies not necessarily linked to digital transformation. Conversely, AI goes beyond automation as it aims to create intelligent machines that work and react like humans (via machine learning). Digital transformation also includes other aspects – such as online labour platforms and big data analytics – that can have different labour market effects from those coming from the increased automation of tasks.
As a result, the evidence on the net impact of robots and other computer-assisted technologies on employment is mixed (OECD, 2016[34]). For example, though there is a clear direct labour substitution channel, the productivity gains they induce may also expand jobs in the firm or enable the emergence of new productive activities, thereby creating jobs. When these positive effects of digitalisation on employment and wages are factored in, the adoption of robots has been found to lead to a net destruction of jobs in the United States (Acemoglu and Restrepo, 2017[35]) while evidence gathered from several countries points to job losses only for low-skilled workers (Graetz and Michaels, 2018[36]). According to a broader measure of technological change (total factor productivity growth), even when employment falls within an industry as industry-specific productivity increases, that drop is more than offset by positive spillovers to other industries (Autor and Salomons, 2018[37]). Using patent grant texts to identify innovation in the field of automation showed that automation boosted total employment as job growth in the service sector compensated for a fall in manufacturing employment (Mann and Puttmann, 2017[38]).
Sources: OECD (2016[34]), “ICTs and Jobs: Complements or Substitutes?”, http://dx.doi.org/10.1787/5jlwnklzplhg‑en; Acemoglu, D. and P. Restrepo (2017[35]), “Robots and jobs: Evidence from US labor markets”, https://www.nber.org/papers/w23285.ack (accessed on 03 November 2017); Graetz, G. and G. Michaels (2018[36]), “Robots at work”, http://dx.doi.org/10.1162/rest_a_00754; Autor, D. and A. Salomons (2018[37]), “Is automation labor‑displacing? Productivity growth, employment, and the labor share”, https://www.brookings.edu/wp‑content/uploads/2018/03/1_autorsalomons.pdf (accessed on 04 July 2018); Mann, K. and L. Puttmann (2017[38]), “Benign effects of automation: New evidence from patent texts”, http://dx.doi.org/10.2139/ssrn.2959584.
Changing skills needs on the job
This and subsequent sections use the Survey of Adult Skills (PIAAC) to analyse how digitalisation changes skills needs on the job. They go beyond the usual distinction between routine and non-routine jobs in order to understand how digitalisation affects skills needs by changing the frequency and combination of tasks performed on the job.
Using PIAAC to assess changes in skills needs at the workplace
PIAAC provides a broad range of information about adults’ skills and the tasks they perform on the job, which can be analysed using the framework in Figure 2.4. This information can be used to build two indicators of exposure to digitalisation in the workplace (Box 2.3).
The intensity of ICT use at work indicator captures how technology complements workers in accomplishing their tasks. It is computed from the frequency with which workers perform a range of tasks using a computer and the Internet, such as reading and writing emails, or using software or a programming language (Grundke et al., 2017[39]). The non-routine intensity of jobs indicator captures how technology substitutes workers in their routine tasks. It is calculated using questions about workers’ freedom to change or choose the sequence of their tasks, the way they work, and how they plan and organise their work, which can approximate the performance of non-routine tasks (Marcolin, Miroudot and Squicciarini, 2016[40]).
Identifying the routine content of tasks performed by individuals is challenging as this is mostly unobservable and therefore has to rely on a proxy. PIAAC has information on workers’ degree of independence in planning and organising their activities and time as well as their freedom in deciding what to do on the job and in what sequence, which gives an indirect measure of the routine content of tasks performed at an individual level. The analysis also uses the following information (Box 2.3):
Skills indicators that reflect the frequency with which tasks are performed that involve specific skills (so-called task-based skills indicators): management and communication skills, accountancy and selling skills, and advanced numeracy skills (Table 2.1).
A measure of “readiness to learn” based on self-assessment information.
Cognitive skills assessed through tests in three domains: literacy, numeracy and problem solving in technology-rich environments (interchangeably referred to as problem solving or computer skills).
PIAAC, which was administered between 2012 and 2015 depending on the country, cannot capture all aspects of the digitalisation of the world of work. In particular, it does not provide information on new forms of work (e.g. Uber drivers, Deliveroo riders, TaskRabbit handypersons) or on how workers can use and market their skills through platforms and online, or even the new jobs that have emerged since (e.g. social media marketing). These issues are discussed later in the chapter. Moreover, as the survey only includes one point in time for each country, it cannot provide a straightforward indication of how digitalisation changes the world of work over time.
Box 2.3. Methodology: Indicators of digitalisation and other indicators considered in the analysis
The Survey of Adult Skills (PIAAC) tests the cognitive skills of adults along three dimensions: literacy, numeracy and problem solving in technology-rich environments. In addition, the survey measures how often people perform several tasks, including reading, writing, numeracy, ICTs and problem solving, thus partially matching the cognitive skills assessed through the tests. It also includes information on how often workers perform other tasks, such as those related to management, communication, organisation and planning, and physical work. Workers also report on their attitudes towards learning, trust, health and other issues.
Non-routine intensity
The routine/non-routine intensity of jobs is computed following the methodology proposed by Marcolin, Miroudot and Squicciarini (2016[40]). The indicator builds on four questions that capture the extent to which one’s job is codifiable and sequentiable: 1) “To what extent can you choose or change the sequence of your tasks?” (sequentiability); 2) “To what extent can you choose or change how you do your work?” (flexibility); 3) “How often does your current job involve planning your own activities?” (planning); and 4) “How often does your current job involve organising your own time?” (self-organisation).
Answers to each question range from 1 to 5 (using a Likert scale of integer values with 1 corresponding to “Not at all” or “Never” and 5 to “To a very high extent” and “Every day” depending on the question). To ensure that an individual answering “Not at all” or “Never” to each question has a score of 0, 1 is subtracted from the answer values (such that they range from 0 to 4). The index of routine/non-routine intensity is calculated as an average of the answers to these four questions. It is close to 0 when the job is routine-intensive and to 1 when the job is non-routine intensive.
ICT intensity at work, and other task-based skills indicators
The ICT intensity of jobs and other task-based skills indicators used in this study were developed in work by Grundke et al. (2017[39]). That study summarised 57 questions of the Survey into six factors that are called task-based skills as they capture the types of tasks workers perform on the job and hence presumably the skills they may develop. These six task-based skills are (Table 2.1):
1. ICT intensity. This indicator describes tasks associated with ICT use, from reading and writing emails to using word-processing or spreadsheet software, or a programming language. It is used as one of the indicators of digital exposure.
2. Self-organisational skills. This indicator includes questions on the extent of flexibility at work and sequentiability of tasks. As some of these questions are used in the non-routine intensity index, this factor is excluded from the analysis.
3. Management and communication skills. This indicator gathers a diverse set of items, from teaching people to planning others’ activities. All these activities involve communicating with and managing other people, whether they be co-workers or not.
4. Accountancy and selling skills. This indicator includes questions on the performance of tasks such as reading financial statements, calculating costs or budgets, using a calculator, and selling products or services.
5. Advanced numeracy skills. This indicator includes questions on the performance of numeric tasks such as using simple algebra or formulas, or using advanced maths or statistics, thus corresponding to tasks that are more complex and less specific than those included in the previous indicator.
6. Readiness to learn. This indicator consists of items designed to measure this dimension, such as relating new ideas to real life or enjoying learning new things.
Each task-based skill has a score ranging from 0 to 1 and is calculated at the individual level. A higher score is associated with a higher frequency of performing the underlying tasks on the job. Comparing intensities between workers is subject to the limitation that moving from “Never” to “Less than once a month” does not imply the same change as moving from “At least once a week but not every day” to “Every day”.
Sources: Marcolin, L., S. Miroudot and M. Squicciarini (2016[40]), “The Routine Content Of Occupations: New Cross‑Country Measures Based On PIAAC”, http://dx.doi.org/10.1787/5jm0mq86fljg‑en; Grundke, R. et al. (2017[39]); “Skills and global value chains: A characterisation”, http://dx.doi.org/10.1787/cdb5de9b-en.
Table 2.1. Indicators of skills involved in the performance of tasks (task-based skills)
List of items from the Survey of Adult Skills (PIAAC) included in task-based skills indicators
Task-based skills indicators |
Items included in the construction of the indicator |
---|---|
ICT Skills |
Frequency of: Excel use; programming language use; transactions through the Internet (banking, selling/buying); email use; simple Internet use; word use; real-time discussions through ICT computer; reading letters, emails, memos; writing letters, emails, memos; and working physically over long periods. Level of computer use required for the job. |
Readiness to learn |
I like to get to the bottom of difficult things; If I don't understand something, I look for additional information to make it clearer; When I come across something new, I try to relate it to what I already know; When I hear or read about new ideas, I try to relate them to real-life situations to which they might apply; I like learning new things; I like to figure out how different ideas fit together. |
Managing and communication |
Frequency of: negotiating with people (outside or inside the firm or organisation); planning activities of others; instructing and teaching people; advising people; persuading or influencing others. |
Self-organisation |
Extent of own planning of: the task sequences; style of work; speed of work; working hours. |
Accountancy and selling |
Frequency of: reading financial invoices, bills etc.; calculate prices, costs, budget; using a calculator; client interaction selling a product or a service. |
Advanced Numeracy |
Frequency of: preparing charts and tables; use simple algebra and formulas; use complex algebra and statistics. |
Sources: Grundke, R. et al. (2017[39]); “Skills and global value chains: A characterisation”, http://dx.doi.org/10.1787/cdb5de9b-en, based on OECD (2012[1]) and OECD (2015[2]), Survey of Adult Skills (PIAAC), www.oecd.org/skills/piaac/publicdataandanalysis.
How digitalisation changes jobs’ task-content and workers’ skills needs
Analysing the task composition of workers who are in the same group of occupations (1‑digit ISCO-08) but whose digital exposure differs may indicate how digitalisation changes the task-content of jobs. However, workers decide to work in digital or less digital environments depending on their skill level. In addition, the data used does not follow workers over time. As a result, this analysis cannot provide causal estimates of the effect of digitalisation on the range of tasks performed on the job and workers’ skills.
Skills specific to tasks performed on the job
For a given group of occupations, the analysis here assumed that workers in the most digital work environments are those with a non-routine intensity of tasks whose use of ICTs at work is above the median of their group of occupations. Conversely, workers performing more routine tasks and fewer ICT-related tasks than the median of their group of occupations are classified as working in the least digital environments.
Adults working in a digital workplace perform all types of tasks considered in the analysis more frequently than workers in a workplace least exposed to digitalisation. That is, they perform more tasks involving management and communication, accountancy and selling, and advanced numeracy skills (Figure 2.5). This is the case for all groups of occupations considered in the analysis, from elementary to managerial occupations, though for the least skilled jobs there is no increase in advanced numeracy tasks. In more digital environments, managers appear to perform mainly more tasks involving advanced numeracy skills. Workers in elementary occupations perform more tasks involving management and communicating, accountancy and selling skills, while clerks face an increase in the performance of all tasks to the same extent.
Figure 2.5. Job-related skill intensities and digital exposure
Note: Each bar displays, for each 1-digit occupation, the difference (not percentage change) in task intensity between the median worker in a most digital environment (above median of the 1-digit occupation in both non-routine and ICT intensities) and the median worker in a least digital work environment (below median of the 1-digit occupation in both non-routine and ICT intensities). For example, the median manager’s task-content in a most digital work environment is 0.14 more intensive in management and communication tasks, 0.15 more intensive in accountancy and selling tasks, and 0.39 more intensive in advanced numeracy tasks. The construction of the non-routine and ICT intensity and task-based skills indicators is explained in Box 2.3.
Sources: OECD calculations based on OECD (2012[1]) and OECD (2015[2]), Survey of Adult Skills (PIAAC), www.oecd.org/skills/piaac/publicdataandanalysis.
This result, that a range of skills are more frequently involved in an occupation more exposed to digitalisation, holds even when the effect of exposure to digitalisation is separated from other effects by taking into account country, industry and occupation characteristics, as well as age, educational attainment and literacy skills (Figure 2.6).
To put this difference into perspective, if the ICT intensity of workers’ professional environment were to increase from the median to the level of the current 75th percentile, they would, on average, perform communication tasks just over 40% more intensively, accountancy tasks close to 60% more intensively, and more advanced numeracy tasks over 50% more intensively. Likewise, when assessing differences with respect to non-routine intensity, if the non-routine intensity of workers’ environment were to increase from the median to the 75th percentile, they would, on average, perform communication tasks almost 40% more intensively, accountancy tasks close to 30% more intensively and more advanced numeracy tasks 10% more intensively.
Workers who have more flexibility to plan their work and who make greater use of ICTs perform these specific non-routine tasks more frequently. They mainly do more tasks involving communication and management skills and to some extent more tasks involving accountancy and selling skills. They also perform more tasks involving advanced numeracy, but to a lesser extent, because some less complex tasks involving advanced numeracy skills can be routine cognitive tasks. ICTs tend to be used more mainly for tasks involving accountancy and selling skills, and advanced numeracy skills, and to a lesser extent for tasks involving communication and management skills.
Figure 2.6. Relationship between job-related skills and digital exposure
Notes: Each bar displays the expected percentage increase in three task-based skills from an increase in the intensity of ICT use at work or in non-routine intensity from the 50th to the 75th percentile. In particular, each task-based skills indicator (management and communication, accountancy and selling, and advanced numeracy) is regressed on the two indicators of digitalisation (ICT and non-routine intensity) and a group of control variables that include occupation, industry, education, age, country, and assessed literacy skills. The error bars represent the 95% confidence interval.
Occupations and industries are included as 1-digit codes of the 2008 International Standard Classification of Occupations (ISCO-08) and 1-digit codes of the International Standard Industrial Classification (ISIC) Rev. 4, respectively. Education is included as six categories from the 1997 International Standard Classification of Education (ISCED). The construction of the non-routine and ICT intensity indicators is explained in Box 2.3.
Sources: OECD calculations based on OECD (2012[1]) and OECD (2015[2]), Survey of Adult Skills (PIAAC), www.oecd.org/skills/piaac/publicdataandanalysis.
General cognitive skills
Understanding how digitalisation may affect the use of major cognitive skills learned in school, such as reading, writing and numeracy, can help policy makers to improve education policies.
Workers in jobs where ICT use and non-routine tasks are more intensive perform tasks involving reading, writing and numeracy more often (Figure 2.7). Technology complements workers in the performance of tasks like reading and writing emails or using a computer for numeracy tasks. By facilitating the execution of some of these tasks, technology can help workers develop their skills in this area. In addition, as workers perform non-routine tasks more frequently, general cognitive skills are more needed.
Figure 2.7. General cognitive skill intensities and digital exposure
Note: Each bar displays, for each 1-digit occupation, the difference (not percentage change) in cognitive task intensities between the median worker in a most digital environment (above median of the 1-digit occupation in both non-routine and ICT intensities) and the median worker in a least digital work environment (below median of the 1-digit occupation in both non-routine and ICT intensities). For example, the median manager’s cognitive skill use in a most digital work environment is 0.20 more intensive in reading at work, 0.15 more intensive in writing at work, and 0.27 more intensive in numeracy at work. The construction of the non-routine and ICT intensity indicators is explained in Box 2.3.
Sources: OECD calculations based on OECD (2012[1]) and OECD (2015[2]), Survey of Adult Skills (PIAAC), www.oecd.org/skills/piaac/publicdataandanalysis.
The importance of this complementarity effect can be assessed by comparing how reading, writing and numeracy skills are used by workers with similar observable characteristics (country, industry, education, age, and assessed literacy skills) and in the same occupation but whose intensity of ICT use is different (Figure 2.8). When the work environment becomes more intensive in non-routine tasks, workers also tend to perform more tasks involving cognitive skills but to a lesser extent than when the use of ICTs increases. The fact that the non-routine intensity of jobs can only be indirectly measured through a range of questions related to the degree of freedom to organise tasks performed may explain why it seems to be less closely related to tasks performed on the job than the use of ICT, which can be captured precisely.
Figure 2.8. Relationship between the use of general cognitive skills and digital exposure
Note: Each bar displays the expected percentage increase in three cognitive skills from an increase in the intensity of ICT use at work or in non-routine intensity from the 50th to the 75th percentile. The same econometric procedure as explained in Figure 2.6’s notes is used. The error bars represent the 95% confidence interval.
Sources: OECD calculations based on OECD (2012[1]) and OECD (2015[2]), Survey of Adult Skills (PIAAC), www.oecd.org/skills/piaac/publicdataandanalysis.
Overall, these results suggest that there are large complementarities between technology and workers. In more digital workplaces, workers make a greater use of cognitive skills in addition to a heightened use of management and communications skills, accountancy and selling skills, and advanced numeracy skills. When workers gain more freedom to plan and organise their work and their work becomes less intensive in routine tasks, they tend to make greater use of communication and management skills, suggesting that social and emotional skills also become more important, as supported by other recent analyses (Deming, 2017[41]).
Learning by doing: The development of skills in a digital workplace
Workers in a digital environment are more likely to maintain or improve the skills they developed during their studies or in past professional experiences, because digitalisation widens the variety of tasks they perform. Conversely, workers who perform specific and technical tasks at work may see the cognitive skills they do not use gradually erode over time and may not develop the social and emotional skills needed. Differences in the task complexity of occupations lead to different learning opportunities (Yamaguchi, 2012[42]). When the value of some skills decreases due to digitalisation, workers invest more in those skills that are more demanded (Cavounidis and Lang, 2017[11]).
How skills evolve with experience
As PIAAC includes only one point in time for each individual, it is not possible to directly observe how skills evolve with experience. However, it is possible to compare the skills of workers who have worked for their employer for the same number of years but whose work environment differs in digital intensity. Such an analysis sheds light on how skills develop with experience, depending on the degree of digitalisation of the workplace.
The analysis includes only individuals who have been working for their current employer for at most 20 years, to increase the likelihood that those whose environment is currently digital also worked in a relatively digital environment when they joined their firm. Self-employed workers were excluded. Crucially, the analysis assumes that the future of individuals who have been with their employers for one year, for example, is proxied by individuals who have been with their employer for longer.
The exposure of the workplace to digitalisation seems to be linked to the development of skills (Figure 2.9). In terms of average problem-solving skills – the interaction between the capacity to use ICT tools and skills to solve problems – the difference between workers in digital and non-digital environments increases with years of experience in that environment. Workers in digital environments are, on average, initially more skilled (in terms of problem-solving skills) than workers in non-digital environments, as evidenced by the difference between the two lines at zero years of experience (i.e. workers who have been with their current employer for less than a year).
The difference in skills development seems to be driven by skill obsolescence of workers in non-digital environments rather than by upskilling of digitally exposed workers. This could be explained by the fact that workers in digital environments do not necessarily use complex ICT tools. They tend to use simple software (e.g. Excel, Word) very frequently, preventing skill degradation, while a high proportion of workers in non-digital environments do not use any ICTs (18% have no computer experience) leading to rapid decreases in problem-solving skills in technology-rich environments. The depletion of one’s proficiency in a particular skill will be greatly affected by the frequency with which one uses the skill in question. ICT skills appear to be particularly subject to obsolescence due to rapid changes both in hardware (smartphones, tablets) and software (more advanced programs, new features) (Cedefop, 2012[43]).
However, these results cannot be interpreted as showing that increasing the digital intensity of workplaces improves skills development. Individuals with better skills and a stronger capacity to adapt are more likely to choose to work in a digital workplace. Likewise, less skilled individuals with a weaker capacity to adapt are more likely to choose to work in a workplace less exposed to digitalisation. To limit this bias (but not fully remove it), several factors that influence workers’ choices in terms of workplaces can be accounted for.
The resulting analysis shows that each additional year of experience in a digital work environment is associated, on average, with a 2.2-point increase in workers’ problem-solving skills relative to working in a non-digital environment (Figure 2.10).
Figure 2.9. Problem-solving skills development and digital exposure – Descriptive analysis
Notes: The top panel shows, for each number of years of experience with current employer, the average level of problem-solving skills in technology-rich environments for workers in digital and non-digital work environments. For example, the average level of problem-solving skills in technology-rich environments score of workers who work in a digital environment and have been with their current employer for five years is around 290 while it is around 160 for workers in a non-digital environment. Digital work environments are defined as being above the overall median in both ICT and non-routine intensities. Conversely, non-digital work environments are defined as being below the overall median in both ICT and non-routine intensities. Workers without any computer experience and those who failed the ICT core assessment are assigned a score of 0. Self-employed individuals are excluded from the sample. Both lines represent linear trendlines.
The bottom panel shows the difference, for each number of years of experience with current employer, between the average level of problem-solving skills in technology-rich environments of workers in a digital environment and that of workers in a non-digital work environment. The line represents a linear trendline.
Sources: OECD calculations based on OECD (2012[1]) and OECD (2015[2]), Survey of Adult Skills (PIAAC), www.oecd.org/skills/piaac/publicdataandanalysis.
Figure 2.10. Problem-solving skills development and digital exposure – Econometric analysis
Notes: Each bar displays the expected increase in problem solving in technology-rich environments (PSTRE) score following one year of experience in a digital work environment relative to a non-digital one. Digital work environments are defined as those with a greater ICT and non-routine intensities than the overall median while non-digital environments have lower ICT and non-routine intensities than the overall median. Individuals without any computer experience and those who failed the ICT core assessment are assigned a PSTRE score of 0. To obtain the “No controls” estimate, the PSTRE score of individuals is regressed on an interaction between a digital environment dummy and years of experience with current employer, age, age-squared, years of experience with current employer, a digital environment dummy and country fixed effects. The “Controls” estimate includes occupation and industry fixed effects as well as other variables (education level, literacy and numeracy scores and the readiness to learn).
Occupations and industries are included as 1-digit codes of the 2008 International Standard Classification of Occupations and 1-digit codes of the International Standard Industrial Classification Rev. 4, respectively. The sample is restricted to individuals with at most 20 years of experience with their current employer and self-employed individuals are excluded, though the results remain unchanged if these restrictions are loosened. The error bars represent the 95% confidence interval.
Source: OECD calculations based on OECD (2012[1]) and OECD (2015[2]), Survey of Adult Skills (PIAAC), www.oecd.org/skills/piaac/publicdataandanalysis.
Learning at work and readiness to learn
Workers in digital environments may have greater incentives, preferences and opportunities to develop their skills. As they tend to perform a greater variety of tasks, they are more likely to learn new work-related tools and methods during their careers. Workers who use ICTs more and perform non-routine tasks more often – the two aspects of digitalisation considered in the analysis – tend to learn more from co-workers, learn more by doing and keep up to date more (Figure 2.11). Digitalisation may facilitate the learning process by easing communication within and among teams. As technology changes rapidly, digitalisation also forces workers to keep up to date.
The readiness to learn new things may be one of the most important skills to develop in a rapidly changing working environment. For instance, firms like Google declare they look for “learning animals” while others say they want “people who are intellectually curious” (The Economist, 2017[44]). Readiness to learn or curiosity, just like other skills, can be shaped through education and experience.
Figure 2.11. Relationship between likelihood of learning at work and digital exposure
Note: Each bar displays the expected percentage increase in the likelihood of learning from various sources at least once a week from an increase in the intensity of ICT use at work or in non-routine intensity from the 50th to the 75th percentile. The exact same econometric procedure as explained in Figure 2.6’s notes is employed. The error bars correspond to associated 95% confidence interval.
Sources: OECD calculations based on OECD (2012[1]) and OECD (2015[2]), Survey of Adult Skills (PIAAC), www.oecd.org/skills/piaac/publicdataandanalysis.
New job opportunities and the implications for skills
New technologies bring many new opportunities for workers, including new or newly growing occupations, and new ways to supply skills to the market. As new products and services emerge, alongside new modes of production and business models, jobs develop not only within high-tech sectors but also outside them. Technologies such as online platforms enable new forms of entrepreneurial activity. These can benefit workers in small businesses, self-employed occupations and others who want to develop activities in addition to their main job. These new ways of working lead to concerns, however, about such workers’ social protection. Understanding the type of skills required by these new opportunities is important to ensure that education and training policies help workers and future cohorts of workers benefit from these opportunities.
Skills demanded in growing occupations and growing sectors
Digitalisation creates jobs that did not exist in the past. Examples include applications and systems developers, cloud computing specialists, transport network engineers, medical device consultants, data analysts, and electrical engineers for smart grids. These jobs require workers with a good knowledge of new technologies who are able to complement this knowledge with their own skills, such as communication, problem solving and creativity. Increasingly, workers will have to use technologies on the job to amplify their own skills.
The Survey of Adult Skills (PIAAC) does not capture very recent emerging occupations but does include some occupations that may grow due to digitalisation. Workers in these growing IT occupations can be compared with workers in similar occupations and those who have similar education levels to uncover the skills requirements that seem to differentiate growing occupations from their peers.
Three growing occupations are analysed:
Software and applications developers and analysts. With the ubiquity of personal computers, smartphones and tablets, software and application development often ranks high in people’s perception of jobs that have a future. Software and applications developers and analysts include systems analysts, web and multimedia developers, and application programmers.
Database and network professionals. As the amount of data stored by companies and governments has inflated exponentially, so has the need for skills to maintain, secure and facilitate access to this data. Database and network professionals include database designers and administrators as well as computer network professionals. They are likely to be increasingly sought after.
ICT operations and user support technicians. The widespread use of technology (both hardware and software) in numerous areas of life, and especially at work, increases the need for maintenance, installation and assistance to users (whether clients or simply workers in the same firm). ICT operations and user support technicians are key to ensuring the good functioning and appropriate use of technologies within firms and organizations.
The cognitive skills and task-based skills as well as the frequency of programming of workers in these occupations can be compared with those of workers in similar occupations (other professionals for the first two occupations and technicians for the third) who are not ICT specialists and have a tertiary degree.
Workers in the three growing occupations have slightly higher proficiency in literacy, numeracy and problem-solving skills in technology-rich environments than their peers. They perform ICT tasks slightly more frequently and management and communication tasks less frequently. The biggest difference between the two groups is the frequency of programming: over 70% of software and applications developers, 40% of database and network professionals and 20% of ICT technicians report using a programming language at least once a week, while only about 5% of other professionals or technicians report the same intensity (Figure 2.12).
This simple analysis based on specific examples suggests that growing IT occupations require advanced ICT skills coupled with other general cognitive skills and social and emotional skills.
Figure 2.12. Skills mix required in some growing occupations
Note: Software and applications developers and analysts and database and network professionals are compared with professionals who are not ICT professionals and who have a tertiary degree. ICT operations and user support technicians are compared with technicians and associate professionals who are not information and communications technicians and who have a tertiary degree. The share of workers who score at least Level 2 in problem-solving skills in technology-rich environments is calculated from the sample excluding workers who opted out of the computer-based assessment, those for whom there is no observation as well as those from France, Italy and Spain. The construction of the task-based skills indicators is explained in Box 2.3.
Sources: OECD calculations based on OECD (2012[1]) and OECD (2015[2]), Survey of Adult Skills (PIAAC), www.oecd.org/skills/piaac/publicdataandanalysis.
Digital transformation has different effects not only on occupations but also on sectors. As higher wages may reflect relative skills shortages, comparing the wage returns to skills in digital-intensive and less digital-intensive sectors can help explain which skills are more in demand because of digital transformation. In all sectors, wage returns to ICT skills are twice as big as those related to numeracy skills, whereas management and communication skills are rewarded as much as numeracy skills (Figure 2.13). When the many facets of digital transformation are taken into account, skills receive a wage premium in sectors that are more digital intensive. This premium is as big for ICT skills as for numeracy skills or management and communication skills. These findings also provide evidence that ICT skills but also general cognitive skills (such as numeracy) and non-cognitive skills (management and communication) involved in tasks performed on the job are particularly important in digital-intensive sectors.
Figure 2.13. Labour market returns to skills in digital-intensive industries and in less digital‑intensive ones
Note: Each bar displays the percentage change in hourly wages for a standard deviation increase in various skills, for both less digital-intensive and more digital-intensive industries. For example, a one standard deviation increase in ICT skills is expected, on average, to yield a 10% and 12% hourly wage increase in less digital-intensive and digital-intensive industries respectively. Digital-intensive sectors are defined according to a range of indicators capturing the multidimensionality of digital transformation: ICT tangible and intangible investment, purchases of ICT goods and services, robot use, revenues from online sales and ICT specialists. The sectors ranking above the median sector by the joint distribution of these indicators are defined as digital intensive. The construction of the task-based skills indicators is explained in Box 2.3. Shaded bars indicate that the result is insignificant at the 5% level.
Source: OECD (2017[5]), OECD Science, Technology and Industry Scoreboard 2017: The digital transformation, http://dx.doi.org/10.1787/9789264268821-en.
Online platforms
Online labour platforms have proliferated. They enable anybody to supply labour and skills to the market, in addition to or instead of a standard job. Two major types of platforms exist (Oyer, 2018[45]). In the first group, platforms mediate on-site and in-person services by assigning a provider of services to a buyer of those services, generally for a given price of the service. Examples include Uber, Deliveroo and TaskRabbit. In a second group, freelancing platforms act as a marketplace where sellers of services can connect to buyers of those services from everywhere, with prices negotiated between the parties. Examples include Upwork and Freelancer.
Through these platforms, hiring managers can connect with millions of freelancers around the world (Corporaal and Lehdonvirta, 2017[46]). Small to large firms are using these platforms to access skills and flexible labour. First studies providing data on digital labour platforms are now available (Kässi and Lehdonvirta, 2018[47]; Horton, Kerr and Stanton, 2017[48]). They show that flows have an asymmetric North-South nature. The United States is the major hiring country and South Asia and Southeast Asia are the major providers of services. Except in the United States, flows within countries are limited.
Online labour platforms are mainly used for software development and technology, then for creative and multimedia activities, and then for writing and translation, with differences between countries (Figure 2.14).
Workers using these platforms typically have specific contracts such as contracts with “very short hours” or “on-call” work, including “zero-hours” contracts (with no guaranteed minimum hours). As a result, these platforms are expected to lead to an increase in the share of workers on non-standard forms of contract and more specifically with atypical contracts that are not the same as traditional temporary contracts. There are no comparable data on the extent of these atypical forms of work. In the United Kingdom, 2.5% of employees were on zero-hours contracts at the end of 2015 (ILO, 2016[49]). In the United States, 1.7% of workers worked “on call” in May 2017 and 6.9% were employed as independent contractors, down from 7.4% in February 2005, the last time the survey was taken (U.S. Bureau of Labor Statistics, 2018[50]). Overall, it is not clear at this stage whether these platforms are simply replacing traditional intermediaries in the labour market with digital ones or whether they will lead to large expansions of self-employment and non-standard forms of work.
It is still unclear how these new forms of work will affect workers’ skills development and participation in training. The development of online labour platforms exacerbates competition between workers around the world. In particular, it enables firms in OECD countries to call on workers elsewhere with a variety of skill levels, possibly at the expense of investing in the skills of their employees. Evidence suggests that firms using workers on call tend to have a larger share of temporary contracts than other firms and to provide less training (ILO, 2016[49]). Overall, the responsibility of training might shift from firms to individual workers, because firms can look for skills through online labour platforms rather than train their employees and because employees who supply their skills online cannot expect employers to invest in their skills development. At the same time, as work and value creation may become more dispersed with employers playing a smaller role, platform owners increasingly centralise the transactions and may capture their value (Kenney and Zysman, 2016[51]). This raises the question of whether platforms have a responsibility to develop workers’ skills.
Figure 2.14. Main skills demanded by OECD countries on the online labour market
Note: Each bar displays employer countries’ share of projects/tasks posted on online labour platforms between January and July 2018 by the occupation of project/task. For example, for projects/tasks posted online by employers based in Chile, over 50% of these were related to software development and technology and 20% to creative and multimedia. The Online Labour Index is based on tracking all projects and tasks posted on the five largest English-language platforms, which account for at least 70% of all traffic to online labour platforms. The occupation classification builds on that used by Upwork.com (Kässi and Lehdonvirta, 2018[52]).
Source: Online Labour Index in Kässi, O. and V. Lehdonvirta (2018[47]), Online Labour Index: Measuring the Online Gig Economy for Policy and Research, https://mpra.ub.uni-muenchen.de/86627/ (accessed on 20 June 2018).
Within the OECD, online labour platforms may enable workers who can supply services online to find jobs they would not have had otherwise. Therefore, regulations should not prevent these developments. These workers should be covered by social protection and employment policies, however, and have the same incentives and opportunities to participate in training as other workers, while not creating unnecessary new administrative and fiscal burdens. Chapter 6 discusses these aspects further.
The knowns and unknowns about future demand for skills
Some aspects of future demand for skills are predicable. Countries, firms, industries and occupations will progressively adopt existing technologies, albeit at different speeds. The way these technologies have changed demand for and use of skills for countries, industries and firms at the frontier of technology adoption gives an idea of the changes that other countries and occupations may face as they catch up. Other aspects of future demand for skills are much more difficult to predict, because they will depend on future technological developments. They are likely to affect initially countries and occupations that tend to adopt new technology earliest.
The known: catching up effects among countries and occupations
Digitalisation has affected countries, industries and occupations unevenly (Figure 2.4), in ways that may indicate how occupations and, in turn, countries may be affected by digitalisation in the near future.
Variations across countries
Countries have adopted digital tools in the workplace at different speeds (Figure 2.15). A group of countries, including Denmark, the Netherlands, Singapore and Sweden, seems to be well advanced in the digitalisation process, with workers predominantly performing non-routine tasks and using computers intensively. At the other end of the spectrum, Chile, Greece and Turkey are lagging far behind with respect to ICT use at work. The position of countries is not the same for all occupations, but overall, the same countries tend to be well advanced with digitalisation or lag behind.
Figure 2.15. Countries’ exposure to digitalisation
Note: Each dot represents a country’s median non-routine and ICT intensities across all workers. The construction of the non-routine and ICT intensity indicators is explained in Box 2.3.
Sources: OECD calculations based on OECD (2012[1]) and OECD (2015[2]), Survey of Adult Skills (PIAAC), www.oecd.org/skills/piaac/publicdataandanalysis.
Countries that lag behind may soon catch up with frontier countries. Yet, differences between countries’ digital exposure may also reflect differences in their industrial or occupational structures, such as large differences within a country in digital exposure of occupations or industries. It is possible to assess the extent to which various factors explain the variation in digital exposure between individuals (Figure 2.16). Differences between individuals’ intensity of ICT use at work are predominantly explained by differences in the nature of occupations (34%) and by differences in their proficiency in computer skills and literacy (14%), while countries (2%), industries (6%) and firm size (2%) play a minor role. The conclusions are very similar for explaining differences in non-routine intensity, though the factors explain significantly less of its variance. The most important factor that explains differences between workers’ exposure to digitalisation is the occupation.
Figure 2.16. The contribution of countries and other factors to the variance of digital exposure
Notes: Results obtained using regression-based decompositions proposed by Fields (2003[53]) with one model estimated for each indicator of digital exposure. The height of the bar corresponds to the total R-squared of the full regression model. The subcomponents show the contribution of each factor to the total R-squared. For example, workers’ skills explain 14% of the total variance in ICT intensity while their occupation explains 34%.
Occupation and industry are included as 1-digit codes of the 2008 International Standard Classification of Occupations and 1-digit codes of the International Standard Industrial Classification Rev. 4, respectively. Skills proficiency corresponds to literacy proficiency and level of problem solving in technology-rich environments. The construction of the non-routine and ICT intensity indicators is explained in Box 2.3.
Sources: OECD calculations based on OECD (2012[1]) and OECD (2015[2]), Survey of Adult Skills (PIAAC), www.oecd.org/skills/piaac/publicdataandanalysis.
Variations across and within occupations
Occupations differ in their (median) exposure to digitalisation, mostly in their intensity of ICT use but also in their non-routine intensity (Figure 2.17). Unsurprisingly, the most skilled occupations, such as managers and professionals, involve more intensive use of ICT and higher non-routine intensity than less skilled ones. This is consistent with the finding that technology complements high-skilled workers and increases their productivity. Though these occupations are the least prone to substantial changes, new technologies may expand the possibilities of automation, pushing these occupations towards even less routine intensity and greater ICT use.
Conversely, occupations with low ICT use and high routine intensity, such as elementary occupations and plant and machine operators, are most likely to evolve in the near future as automation, industrial robots, computers and artificial intelligence replace workers in performing routine tasks. As a result, adults in these occupations will be increasingly required to perform non-routine tasks and tasks involving ICTs or socio-emotional skills for which they hold a comparative advantage relative to computers. Therefore, it is particularly important to ensure these workers are equipped with the appropriate skill set to adapt to these changes.
Figure 2.17. Occupations’ exposure to digitalisation
Note: Each dot represents a 1-digit occupation’s median non-routine and ICT intensities across all workers of that group of occupations in all countries. The construction of the non-routine and ICT intensity indicators is explained in Box 2.3.
Sources: OECD calculations based on OECD (2012[1]) and OECD (2015[2]), Survey of Adult Skills (PIAAC), www.oecd.org/skills/piaac/publicdataandanalysis.
However, even within occupations there is considerable variation in task structure (Spitz‐Oener, 2006[54]). Within the same occupation (1-digit), there are large differences between workers of all countries in their use of ICTs and performance of non-routine tasks (Figure 2.18). There are generally smaller variations within high-skilled occupations than within low-skilled ones. This is particularly the case for the non-routine intensity of tasks performed by workers: while there is little variation between occupations for the 75th percentile, low-skilled occupations have a much lower 25th percentile than high-skilled ones. These variations help explain how digitalisation might alter the daily task-content of workers in the near future. Three major scenarios emerge:
In occupations with the largest proportion of routine, such as elementary occupations and plant and machine operators, workers differ the most in terms of their non-routine intensity and to a lesser extent in terms of their use of ICTs. Workers in these occupations might be mainly affected by a substitution effect, with machines taking over the performance of routine tasks.
Within some occupations, the variation in the use of ICTs is bigger than in non-routine task intensity, with a large share of workers not using computers at all, such as craft and trade workers, and service and market sale workers. The biggest change for some of these workers might be the introduction and development of technology at their workplace. These workers are also likely to be strongly affected by automation, especially those with high non-routine intensities.
Finally, skilled occupations such as managers and professionals display small variation in both dimensions, suggesting that the impact of digitalisation on workers in these occupations might be limited, at least in the short term. Other occupations, such as clerks and technicians, show slightly larger variations in these two dimensions, indicating that workers in these occupations will be affected by both a substitution and a complementarity effect.
Figure 2.18. Variation in digital exposure within the same occupation
Note: For each 1-digit occupation, the figure displays the 25th, 50th (median) and 75th percentile of the distribution of the non-routine and ICT intensity indicators across all workers of that group of occupations in all countries. For example, a manager at the 25th percentile has an ICT intensity around 0.7 while it is over 0.85 for a manager at the 75th percentile. The construction of the non-routine and ICT intensity indicators is explained in Box 2.3.
Sources: OECD calculations based on OECD (2012[1]) and OECD (2015[2]), Survey of Adult Skills (PIAAC), www.oecd.org/skills/piaac/publicdataandanalysis.
The unknown: The technology frontier
Countries and occupations at the frontier of technology adoption are also likely to face important changes as new technologies develop. However, there are large uncertainties about the technological capabilities required and the extent to which non-routine tasks could be automated.
Artificial intelligence (AI) is one of the main technologies expected to change the world of work. AI has already several applications today in the transport and health sectors (OECD, 2017[55]). In its current state-of-the-art uses, however, AI is said to be “applied” or “narrow” – designed to accomplish a specific problem-solving or reasoning task. In a hypothetical Artificial General Intelligence scenario, autonomous machines would become capable of general intelligent action, like a human being, including generalising and abstracting learning across different cognitive functions. There are large uncertainties about the time frame for the generalisation of AI that partly depend on advancement of machine learning.
AI may eventually affect a wider range of firms and industries. So far, AI has been mostly adopted by tech firms such as Amazon, Google, and Microsoft, as well as by some start-ups (OECD, 2017[55]). For most firms, AI is still too expensive or too complex to adopt, as they lack the necessary skills. Machine-learning tools based in the cloud are bringing AI to a broader audience, however. These and other technological tools are poised to change the world of work, according to experts (Box 2.4).
Box 2.4. Breakthrough technologies in 2018
Each year, the Massachusetts Institute of Technology lists ten breakthrough technologies (or ranges of technologies) that have not reached widespread use but may have a profound effect on people’s lives. In the 2018 list, technologies that could change the world of work include:
3-D metal printing
While this technology is not new, it has remained in the domain of a small group of experts using prototypes and mostly printing objects from plastics. Now the technology has become cheap and easy enough to be integrated in the manufacturing of products. If widely adopted, it could change production, limiting the need for large inventories and making it cheaper to customise products to individual needs.
AI for everybody
For most firms, AI is still too expensive or too complex. Cloud-based AI, which is making the technology cheaper and easier to use, could significantly expand the use of AI by firms and industries. Up to now, AI is used mostly in the tech industry. Other sectors such as medicine, manufacturing, and energy can be transformed if they adopt the technology.
Generative adversarial networks: Advancement in machine learning
The issue of making machines learn how to teach themselves is at the core of much research in the domain of AI. “Generative adversarial networks” are considered a big advance in this direction. The idea is to build on the interactions of two AI systems to achieve an outcome without human intervention. The technology could create ultra-realistic images or sounds that cannot be dissociated from those coming from the real world, for instance. Such technology enlarges the use of AI by making machines less reliant on humans.
Babel-fish earbuds
This technology translates foreign languages in real time. It now works for a large number of languages and is easy to use. In an increasingly global world, language is still a barrier to communication. At work, this technology can give another boost to the globalisation of production.
Source: MIT (2018[56]), 10 Breakthrough Technologies 2018, https://www.technologyreview.com/lists/technologies/2018/ (accessed on 30 August 2018).
While it is important to understand how technology developments may affect the world of work and skills needs, the technical feasibility of automating a larger range of tasks will not automatically translate into automation of jobs. It will continue to change what workers do on the job, however. Technical feasibility is only one of the factors shaping the future of work. Other major factors include: i) the performance and reliability of future technologies; ii) the cost of technology deployment and adoption relative to the cost of workers and other costs of production; iii) managers and employees’ skills and their capacity to work with new technologies. Policies can influence all of these factors.
Summary
Digital transformation includes many facets that profoundly change labour markets. The debate about digitalisation often focuses on jobs at risk of automation and risks that technology will displace workers. In fact, workers in almost all jobs are seeing or will see changes in their work because of digital transformation.
These changes create both challenges and opportunities for workers. Technology can enrich the content of occupations by allowing workers increasingly to focus on non-routine tasks, such as problem solving, creative activities and more complex communications while developing their digital skills by using new technologies. It can also enable workers around the world to bring their skills to the market much more easily through labour market platforms, boosting opportunities for entrepreneurship. On the other hand, workers who lack the necessary skills may find it hard to adapt to change or benefit from new opportunities, and may be left behind.
Because technologies spread to industries, firms and occupations at different paces, workers are affected differently. Workers who are less exposed to digitalisation may be less exposed to job-loss risks in the short term but do not develop the skills they may need to work with new technologies in the longer term. Education and training policies have an important role to play in ensuring that digital transformation does not reinforce inequalities by dividing workers into those who are ICT savvy and those who lag behind.
Education and training policies need to ensure that workers have the right mix of skills to successfully navigate the transition to the digital world of work, and thrive in it. This chapter shows that digital skills are not the same thing as skills for a digital world of work. Even workers in fast-growing, technology-exposed occupations, who need to have advanced ICT skills such as coding, also need strong general cognitive skills, analytical skills and problem-solving skills, as well as social and emotional skills, communication skills and the ability to manage stress and change. Workers in less technologically advanced occupations need to have similar mix of skills but with less advanced ICT skills.
This chapter sheds light on the importance of co-ordinating education and training policies with other policies, an issue discussed further in other chapters. The emergence of labour market platforms raises the issue of the social protection of workers participating in these platforms. Another conclusion of this chapter is the importance for education and training policies to be more forward-looking and to be co‑ordinated with the technological trajectory planned by countries. In particular, countries lagging behind in the adoption of new technologies need policies to develop the workforce’s skills, co‑ordinated with investment in digital infrastructure and research and innovation policies.
References
[7] Acemoglu, D. (1998), “Why do new technologies complement skills? Directed technical change and wage inequality”, The Quarterly Journal of Economics, Vol. 113/4, pp. 1055-1089, http://dx.doi.org/10.1162/003355398555838.
[35] Acemoglu, D. and P. Restrepo (2017), “Robots and jobs: Evidence from US labor markets”, NBER Working Paper, No. 23285, National Bureau of Economic Research, Cambridge, MA, https://www.nber.org/papers/w23285.ack (accessed on 3 November 2017).
[12] Andrews, C. (2009), ’Do-It-Yourself’: Self-checkouts, Supermarkets, and the Self-Service Trend in American Business, University of Maryland, https://drum.lib.umd.edu/handle/1903/9593?show=full (accessed on 3 November 2017).
[4] Andrews, D., G. Nicoletti and C. Timiliotis (2018), “Digital technology diffusion: A matter of capabilities, incentives or both?”, OECD Economics Department Working Papers, No. 1476, OECD Publishing, Paris, https://dx.doi.org/10.1787/7c542c16-en.
[32] Andrews, D., G. Nicoletti and C. Timiliotis (2018), “Going digital: What determines technology diffusion among firms?”, Economics Department Economic Policy Committee, No. ECO/CPE/WP1(2018)8, OECD, Paris, https://one.oecd.org/document/ECO/CPE/WP1(2018)8/en/pdf (accessed on 16 April 2018).
[14] Autor, D. (2015), “Why are there still so many jobs? The history and future of workplace automation”, Journal of Economic Perspectives, Vol. 29/3, pp. 3-30, http://dx.doi.org/10.1257/jep.29.3.3.
[8] Autor, D., L. Katz and A. Krueger (1998), “Computing inequality: Have computers changed the labor market?”, The Quarterly Journal of Economics, Vol. 113/4, pp. 1169-1213, http://dx.doi.org/10.1162/003355398555874.
[9] Autor, D., F. Levy and R. Murnane (2003), “The skill content of recent technological change: An empirical exploration”, The Quarterly Journal of Economics, Vol. 118/4, pp. 1279-1333, http://dx.doi.org/10.1162/003355303322552801.
[37] Autor, D. and A. Salomons (2018), “Is automation labor-displacing? Productivity growth, employment, and the labor share”, Brooking Papers on Economic Activity, Conference Drafts, https://www.brookings.edu/wp-content/uploads/2018/03/1_autorsalomons.pdf (accessed on 4 July 2018).
[13] Bessen, J. (2016), “How computer automation affects ocupations: Technology, jobs, and skills”, Law & Economics Working Paper, No. 15-49, Boston University School of Law, Boston, MA, http://dx.doi.org/10.2139/ssrn.2690435.
[30] Brynjolfsson, E., A. Renshaw and M. Van Alstyne (1997), “The matrix of change: A tool for business process reengineering”, Sloan Management Review, pp. 37-54, https://www.researchgate.net/publication/23738801_The_Matrix_of_Change_A_Tool_for_Business_Process_Reengineering (accessed on 13 July 2018).
[3] Calvino, F. et al. (2018), “A taxonomy of digital intensive sectors”, OECD Science, Technology and Industry Working Papers, No. 2018/14, OECD Publishing, Paris, http://dx.doi.org/10.1787/f404736a-en.
[16] Carlson, M. (2015), “The robotic reporter”, Digital Journalism, Vol. 3/3, pp. 416-431, http://dx.doi.org/10.1080/21670811.2014.976412.
[11] Cavounidis, C. and K. Lang (2017), “Ben-Porath meets Lazear: Lifetime skill investment and occupation choice with multiple skills”, NBER Working Paper, No. 23367, National Bureau of Economic Research, Cambridge, MA, http://dx.doi.org/10.3386/w23367.
[43] Cedefop (2012), “Preventing skill obsolescence”, No. 9070 EN, Briefing note, Cedefop, http://www.cedefop.europa.eu/files/9070_en.pdf (accessed on 23 July 2018).
[46] Corporaal, G. and V. Lehdonvirta (2017), Platform Sourcing How Fortune 500 Firms Are Adopting Online Freelancing Platforms, Oxford Internet Institute, https://www.oii.ox.ac.uk/publications/platform-sourcing (accessed on 20 June 2018).
[25] Deepa, S. and B. Aruna Devi (2011), “A survey on artificial intelligence approaches for medical image classification”, Indian Journal of Science and Technology, Vol. 4/11, pp. 1583-1595, http://52.172.159.94/index.php/indjst/article/view/30291/26223 (accessed on 14 December 2017).
[41] Deming, D. (2017), “The growing importance of social skills in the labor market”, The Quarterly Journal of Economics, Vol. 132/4, pp. 1593-1640, http://dx.doi.org/10.1093/qje/qjx022.
[26] Doi, K. (2007), “Computer-aided diagnosis in medical imaging: Historical review, current status and future potential”, Computerized Medical Imaging and Graphics, Vol. 31/4-5, pp. 198-211, http://dx.doi.org/10.1016/j.compmedimag.2007.02.002.
[28] Esteva, A. et al. (2017), “Dermatologist-level classification of skin cancer with deep neural networks”, Nature, Vol. 542/7639, pp. 115-118, http://dx.doi.org/10.1038/nature21056.
[53] Fields, G. (2003), “Regression-based decompositions: A new tool for managerial decision-making”, http://citeseerx.ist.psu.edu/viewdoc/download?doi=10.1.1.494.9450&rep=rep1&type=pdf (accessed on 22 November 2018).
[31] Gallego, J., L. Gutierrez and S. Lee (2015), “A firm-level analysis of ICT adoption in an emerging economy: Evidence from the Colombian manufacturing industries”, Industrial and Corporate Change, Vol. 24/1, pp. 191-221, http://dx.doi.org/10.1093/icc/dtu009.
[19] Gideon, L. (2016), The Great A.I. Awakening, The New York Times Magazine, http://dx.doi.org/10.1002/cne.21974.
[36] Graetz, G. and G. Michaels (2018), “Robots at work”, The Review of Economics and Statistics, http://dx.doi.org/10.1162/rest_a_00754.
[39] Grundke, R. et al. (2017), “Skills and global value chains: A characterisation”, OECD Science, Technology and Industry Working Papers, No. 2017/05, http://dx.doi.org/10.1787/cdb5de9b-en.
[10] Heckman, J. and C. Corbin (2016), “Capabilities and skills”, Journal of Human Development and Capabilities, Vol. 17/3, pp. 342-359, http://dx.doi.org/10.1080/19452829.2016.1200541.
[48] Horton, J., W. Kerr and C. Stanton (2017), “Digital labor markets and global talent flows”, NBER Working Paper, No. 23398, National Bureau of Economic Research, http://www.nber.org/papers/w23398 (accessed on 28 August 2018).
[49] ILO (2016), Non-standard Employment Around the World: Understanding Challenges, Shaping Prospects, International Labour Organization, http://www.ifrro.org (accessed on 29 August 2018).
[47] Kässi, O. and V. Lehdonvirta (2018), “Online labour index: Measuring the online gig economy for policy and research”, Technological Forecasting and Social Change, Vol. 137, pp. 241-248, http://dx.doi.org/10.1016/J.TECHFORE.2018.07.056.
[52] Kässi, O. and V. Lehdonvirta (2018), “Online labour index: Measuring the online gig economy for policy and research”, Technological Forecasting and Social Change, Vol. 137, pp. 241-248, http://dx.doi.org/10.1016/J.TECHFORE.2018.07.056.
[6] Kenney, M. and J. Zysman (2016), “The rise of the platform economy”, Issues in Science and Technology, Vol. Spring 2016, http://www.brie.berkeley.edu/wp-content/uploads/2015/02/Kenney-Zysman-The-Rise-of-the-Platform-Economy-Spring-2016-ISTx.pdf (accessed on 2 July 2018).
[51] Kenney, M. and J. Zysman (2016), “What is the future of work? Understanding the platform economy and computation-intensive automation”, No. 2016-9, Berkeley Roundtable on the International Economy, http://www.brie.berkeley.edu/wp-content/uploads/2015/01/BRIE-WP-2016-9.pdf (accessed on 2 July 2018).
[20] Larousserie, D. (2017), La traduction dopée par l’intelligence artificielle, Le Monde, http://www.lemonde.fr/sciences/article/2017/11/27/la-traduction-dopee-par-l-intelligence-artificielle_5221041_1650684.html?xtmc=la_traduction_dopee_par_l_intelligence_artificielle&xtcr=1 (accessed on 12 December 2017).
[22] Lohr, S. (2017), A.I. Is Doing Legal Work. But It Won’t Replace Lawyers, Yet, The New York Times, https://www.nytimes.com/2017/03/19/technology/lawyers-artificial-intelligence.html (accessed on 12 December 2017).
[38] Mann, K. and L. Puttmann (2017), “Benign effects of automation: New evidence from patent texts”, SSRN Electronic Journal, http://dx.doi.org/10.2139/ssrn.2959584.
[40] Marcolin, L., S. Miroudot and M. Squicciarini (2016), “The Routine Content Of Occupations: New Cross-Country Measures Based On PIAAC”, OECD Trade Policy Papers, No. 188, OECD Publishing, Paris, http://dx.doi.org/10.1787/5jm0mq86fljg-en.
[29] Milgrom, P. et al. (1990), “The economics of modern manufacturing: Technology, strategy, and organization”, American Economic Review, Vol. 80/3, pp. 511-28, https://econpapers.repec.org/article/aeaaecrev/v_3a80_3ay_3a1990_3ai_3a3_3ap_3a511-28.htm (accessed on 13 July 2018).
[56] MIT (2018), 10 Breakthrough Technologies 2018, MIT Technology Review, https://www.technologyreview.com/lists/technologies/2018/ (accessed on 30 August 2018).
[27] Mukherjee, S. (2017), A.I. Versus M.D., The New Yorker, https://www.newyorker.com/magazine/2017/04/03/ai-versus-md (accessed on 14 December 2017).
[33] OECD (2018), Going Digital in a Multilateral World, OECD, Paris, http://www.oecd.org/mcm/documents/C-MIN-2018-6-EN.pdf.
[55] OECD (2017), OECD Digital Economy Outlook 2017, OECD Publishing, Paris, http://dx.doi.org/10.1787/9789264276284-en.
[5] OECD (2017), OECD Science, Technology and Industry Scoreboard 2017: The digital transformation, OECD Publishing, Paris, http://dx.doi.org/10.1787/9789264268821-en.
[34] OECD (2016), “ICTs and Jobs: Complements or Substitutes?”, OECD Digital Economy Papers, No. 259, OECD Publishing, Paris, http://dx.doi.org/10.1787/5jlwnklzplhg-en.
[2] OECD (2015), Survey of Adult Skills, Programme for the International Assessment of Adult Competencies (PIAAC), OECD, Paris, http://www.oecd.org/skills/piaac/publicdataandanalysis.
[1] OECD (2012), Survey of Adult Skills, Programme for the International Assessment of Adult Competencies (PIAAC), OECD, Paris, http://www.oecd.org/skills/piaac/publicdataandanalysis.
[15] Örnebring, H. (2010), “Technology and journalism-as-labour: Historical perspectives”, Journalism, Vol. 11/1, pp. 57-74, http://dx.doi.org/10.1177/1464884909350644.
[45] Oyer, P. (2018), The Independent Workforce in America, Upwork, https://s3-us-west-1.amazonaws.com/adquiro-content-prod/documents/paul_oyer_the_independent_workforce_in_america.pdf (accessed on 20 June 2018).
[23] Remus, D. and F. Levy (2016), “Can robots be lawyers? Computers, lawyers, and the practice of law”, http://dx.doi.org/10.2139/ssrn.2701092.
[24] Shiraishi, J. et al. (2011), “Computer-aided diagnosis and artificial intelligence in clinical imaging”, Seminars in Nuclear Medicine, Vol. 41/6, pp. 449-462, http://dx.doi.org/10.1053/J.SEMNUCLMED.2011.06.004.
[54] Spitz‐Oener, A. (2006), “Technical change, job tasks, and rising educational demands: Looking outside the wage structure”, Journal of Labor Economics, Vol. 24/2, pp. 235-270, http://dx.doi.org/10.1086/499972.
[44] The Economist (2017), What Employers Can Do to Encourage Their Workers to Retrain, The Economist, https://www.economist.com/news/special-report/21714171-companies-are-embracing-learning-core-skill-what-employers-can-do-encourage-their (accessed on 3 November 2017).
[50] U.S. Bureau of Labor Statistics (2018), Contingent and Alternative Employment Arrangements, U.S. Bureau of Labor Statistics, Department of Labor, http://www.bls.gov/cps (accessed on 29 August 2018).
[17] Underwood, C. (2017), Automated Journalism - AI Applications at New York Times, Reuters, and Other Media Giants, Techemergence, https://www.techemergence.com/automated-journalism-applications/ (accessed on 11 December 2017).
[18] Van Dalen, A. (2012), “The algorithms behind the headlines”, Journalism Practice, Vol. 6/5--6, pp. 648-658, http://dx.doi.org/10.1080/17512786.2012.667268.
[21] Williams, M. (2016), “’Moneyball for lawyers’: How technology will change the practice of law”, Vol. 38/5, pp. 14-15, https://www.lawsocietysa.asn.au/bulletin/BULL_Moneyball_for_lawyers.PDF (accessed on 13 December 2017).
[42] Yamaguchi, S. (2012), “Tasks and heterogeneous human capital”, Journal of Labor Economics, Vol. 30/1, pp. 1-53, http://dx.doi.org/10.1086/662066.