The methodology used to analyse the economic consequences of policies targeting air quality in Arctic Council countries is described in this chapter. This methodology requires multiple steps, from creating plausible economic projections and changes in air pollutant emissions, to calculating concentrations of key pollutants, the biophysical impacts on health and crop yields, and the economic consequences of the policy scenarios. The scenario analysis relies on the OECD ENV-Linkages model, along with IIASA’s GAINS model and the European Commission JRC’s TM5-FASST model.
The Economic Benefits of Air Quality Improvements in Arctic Council Countries
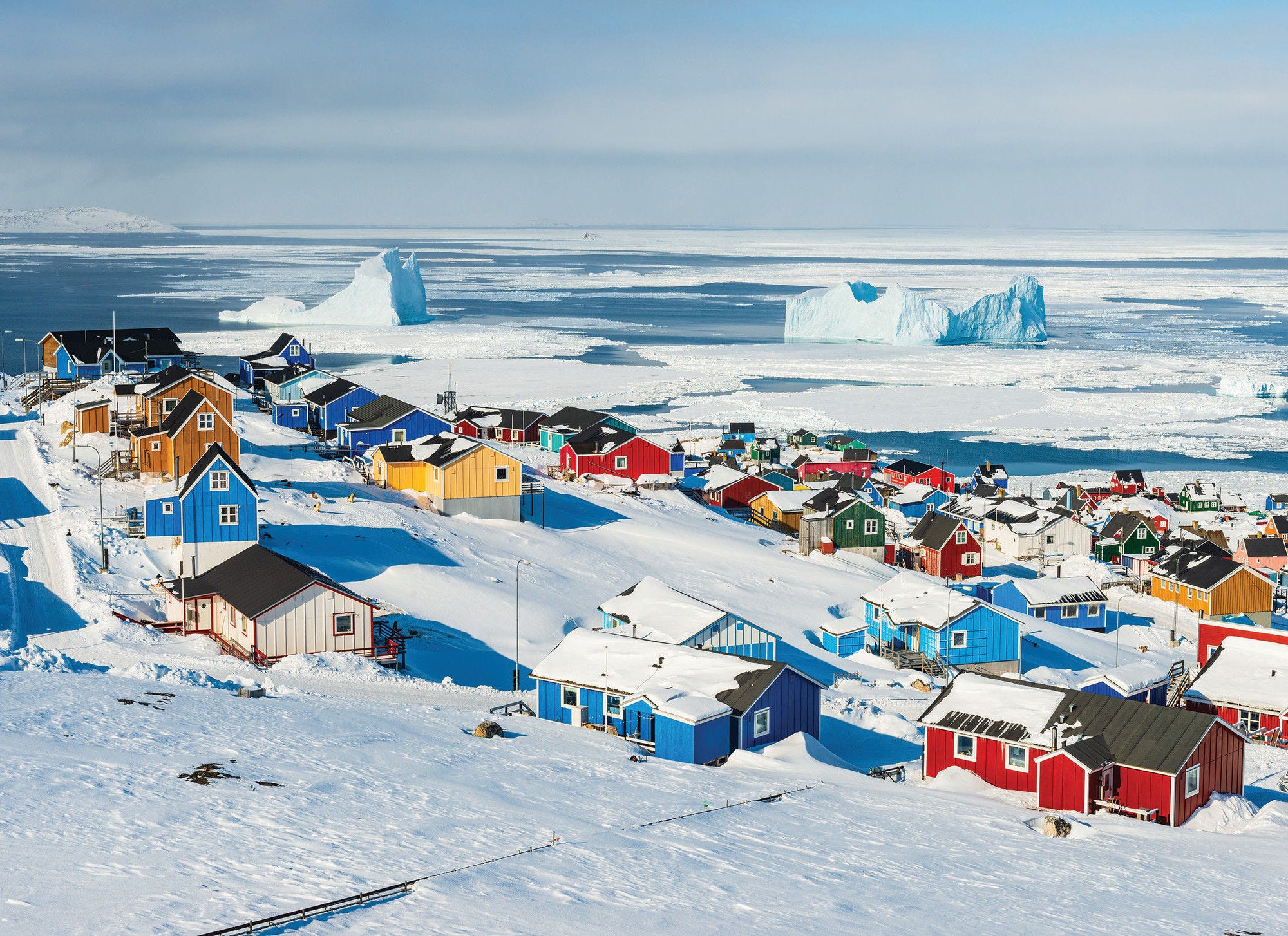
2. Description of the approach and scenarios
Abstract
2.1. Scenarios overview
This report analyses the economic consequences of policies targeting air pollution, based on a scenario analysis performed with the OECD Computable General Equilibrium (CGE) model ENV-Linkages (Chateau, Dellink and Lanzi, 2014[1]). The report compares four policy scenarios to a reference baseline scenario, all with a 2050 time horizon (Table 2.1; see also Chapter 1).
Table 2.1. Overview and regional coverage of scenarios
Scenario label |
Scenario description |
Arctic Council countries |
Observer countries |
Rest of the world |
---|---|---|---|---|
CLE |
Current Legislation (Baseline) |
CLE |
CLE |
CLE |
MTFR-AC |
Maximum Technically Feasible Reduction in Arctic Council countries only |
MTFR |
CLE |
CLE |
MTFR-AC&Obs |
Maximum Technically Feasible Reduction in Arctic Council and Observer countries |
MTFR |
MTFR |
CLE |
MTFR-Global |
Maximum Technically Feasible Reduction at the global level |
MTFR |
MTFR |
MTFR |
MTFR-SDS |
Maximum Technically Feasible Reduction and Sustainable Development Scenario at the global level |
MTFR - SDS |
MTFR – SDS |
MTFR - SDS |
The baseline scenario assumes the effective implementation of the current legislation (CLE) in the eight Arctic Council countries. The legislation considered addresses air pollution from combustion plants, industrial processes, road and non-road vehicles, shipping, agriculture, and use of solvents and paint, as well as residential emissions.
The CLE scenario is compared with four policy action scenarios that reflect the implementation of maximum technically feasible reductions (MTFR) to abate pollutant emissions. The MTFR scenario explores the extent to which emissions could be further reduced through the policy-induced application of all existing best available techniques (BATs), in addition to the implementation of current regulations.
The main policy scenario analysed in this report considers the achievement of the maximum technically-feasible emission reductions in the eight Arctic Council countries, referred to as the MTFR-AC scenario. For this scenario, data on the investments needed to reduce air pollution are provided by the GAINS model and included in ENV-Linkages (see Section 2.2) below on the modelling framework).
As emission reductions in other regions can also contribute to improving air quality in Arctic Council countries through reduced transboundary air pollution, two additional scenarios are considered: one in which MTFR technologies are also adopted in Arctic Council Observer countries (referred to as MTFR-AC&Obs), and one representing the global deployment of the MTFR technologies (MTFR-Global).
Finally, an additional scenario (MTFR-SDS) considers integrated policy action across different environmental domains, focusing on the interaction among air pollution, climate and energy transition policies. This scenario assumes that the policies of the MTFR-Global scenario are complemented by the climate and energy policies.
All scenarios are are designed based on inputs from the Greenhouse Gas and Air Pollution Interactions and Synergies (GAINS) model developed by the International Institute for Applied Systems Analysis (IIASA) (Box 2.1). The MTFR-SDS scenario reflects the climate and energy policies included in the International Energy Agency (IEA)’s Sustainable Development Scenario (SDS) presented in the 2018 World Energy Outlook (IEA, 2018[2]) (Box 2.2).
While a full analysis of the costs and benefits of the deployment of MTFR technologies is only presented for the MTFR-AC scenario, the three additional scenarios highlight the importance of wider policy action in the fight against air pollution. For these scenarios, only the welfare improvements are presented, as a full assessment of the macroeconomic consequences of these scenarios is beyond the scope of this report.
Box 2.1. The GAINS model’s emission abatement scenarios
The scenarios analysed in this report are based on inputs from IIASA’s GAINS integrated assessment model, which provides projections of air pollutants, emission reduction potentials, as well as the cost of emission reductions (Amann et al., 2011[3]; Höglund-Isaksson et al., 2020[4]; Winiwarter et al., 2018[5]; Klimont et al., 2017[6]). The emission projections provided by the GAINS model stem from projections of economic activities, and rely on specific assumptions about current environmental legislations as well as existing technical options to reduce air pollution. While GAINS offers a coherent framework to assess emission projections, its projections can differ from the ones developed by individual countries. Data quality varies across countries and, for those cases in which information is missing, the model’s default assumptions are applied. These include representative emission factors, which reflect the state of technology deployment in a country, assumptions about the shares of specific technologies to achieve a given emission standard, and sometimes also alternative sources of activity data (e.g. peer reviewed studies, international assessment reports, contacts with local experts). The latter is often the case for residential combustion of wood fuels (accounting for non-commercial fuel use), open burning of agricultural residues, and gas flaring.
The CLE scenario reflects the continuation of current legislation on energy use, energy efficiency, and climate mitigation, as described in the World Energy Outlook 2018’s New Policies Scenario (IEA, 2018[2]). In addition to these policies, the CLE scenario considers detailed inventories of national emission control legislation described thoroughly by Amman et al. (2018[7]). The policies and inventories included in the CLE scenario include all those in place in 2017. Therefore, recent zero-emission pledges and targets are not included in this scenario.
The MTFR scenario reflects efforts to reduce the emission of air pollutants through regulations, emission standards, and emission limits. Such reductions are achieved thanks to the deployment of existing best available techniques (BATs) in different sectors. In other words, the MTFR scenarios reflect the maximum mitigation potential that is possible in each sector. The GAINS model contains an inventory of technically-feasible and commercially-available measures that could cut emissions below the baseline projections, adding to the measures already in place and included in the CLE scenario. Hence, when compared to the CLE scenario, the MTFR policy scenarios entail higher emission reductions.
The MTFR scenario reflects the deployment of source- and region-specific technologies that can reduce the emissions of several pollutants. These include (1) end-of-pipe technologies such as filters, scrubbers and catalytic converters; (2) pollutant capture and recovery systems (e.g. addressing non-methane volatile organic compounds emissions from the solvent sector); (3) cleaner and more efficient solid fuels for stoves and boilers; and (4) measures to reduce ammonia and methane emissions in the agricultural sector.
The BATs included in the MTFR scenarios differ in the timing of deployment, which depends on their ease of implementation. For instance, some BATs can quickly complement existing technologies (e.g. end-of-pipe cleaning techniques), while others, which completely replace existing technologies, have a longer lifetime and require more complex changes (e.g. heating stoves replacement).
Box 2.2. The IEA’s Sustainable Development Scenario
The SDS scenario takes into account climate change mitigation and energy transition policies. The SDS scenario describes a path for the global energy sector that is aligned with the mitigation targets established by the Paris Agreement – i.e. holding the increase in global temperature to “well below 2°C” and “pursuing efforts to limit [it] to 1.5°C” (UNFCCC, 2015[8]). The policies included in the SDS scenario also allow for the energy-related goals established by the United Nations’ (UN) 2030 Agenda for Sustainable Development to be achieved. Most notably, the SDS scenario includes the policies needed to transform the energy sector in order to (1) achieve universal access to energy (SDG 7); (2) reduce the health impacts of air pollution (SDG 3); (3) tackle climate change (SDG 13); and (4) achieve universal access to clean water and sanitation (SDG 6).
The policy instruments needed to achieve these results are integrated into the SDS scenario and include carbon pricing, energy taxes and the removal of fossil fuel subsidies. All these instruments are implemented in the ENV-Linkages model. More specifically, in the SDS scenario, carbon prices increase over time, from USD 121.5 to USD 140 per tCO2 in 2040, with differences across countries and sectors. In parallel, fossil fuel subsidies are gradually removed during the same period. In addition, the share of non-fossil power sources rises to 40% in 2040, accompanied by improvements in energy efficiency.1 Energy efficiency is measured by the energy intensity of GDPs, which drops from 110 tonnes of oil equivalent (toe)/USD 1 000 in 2017 to 40 toe/USD 1 000 in 2040 at the global level (IEA, 2018[2]).
Source: (IEA, 2018[2]).
2.2. Modelling framework
This report takes a quantitative approach to assess the economic consequences of air pollution to 2050. The modelling framework is based on an impact pathway approach,2 which includes multiple steps and requires the use of different techniques and models. The methodology relies on the modelling framework used for the OECD’s CIRCLE project, as described in the OECD report “The Economic Consequences of Outdoor Air Pollution” (OECD, 2016[9]). The modelling framework used in this report links projections of (1) sectoral economic activities to (2) emissions of air pollutants, (3) concentrations of fine particulate matter and ground-level ozone, and finally to (4) the biophysical and (5) economic impacts of outdoor air pollution (Figure 2.1). The steps outlined in Figure 2.1 are repeated for each scenario outlined in Section 2.1.
This methodology relies on a suite of modelling tools. The main tool for analysing the economic consequences of air pollution in the different scenarios is the OECD computable general equilibrium model ENV-Linkages, described in Annex A (Chateau, Dellink and Lanzi, 2014[1]). This analysis is supported by results from the GAINS integrated assessment model of the International Institute for Applied Systems Analysis (IIASA), which provides projections of air pollutants, emission reduction potentials, and required investments in emission reduction technologies (Amann et al., 2011[3]; Höglund-Isaksson et al., 2020[4]; Winiwarter et al., 2018[5]; Klimont et al., 2017[6]). The global air quality source-receptor model TM5-FASST (Van Dingenen et al., 2018[10]) of the Joint Research Centre of the European Commission provides the link between emission projections and exposure to PM2.5 and ground-level ozone.
Figure 2.1. Methodological steps
The models operate at different scales while sharing the same socio-economic baseline trends. Combining these models allows the economic consequences of air pollution to be calculated at the macroeconomic level, while accounting for pollution concentrations at the local level. Specifically, economic projections are provided for 19 aggregate regions, while emission levels are available for 48 regions, and pollutant concentrations are specified at a 1-degree grid-level (i.e. about 44 km2). The five methodological steps and their models (Figure 2.1) are described in turn below:
1. The projections of sectoral economic activities are obtained with the OECD CGE model ENV‑Linkages (Chateau, Dellink and Lanzi, 2014[1]). ENV-Linkages allows for the creation of detailed projections of sectoral and regional economic activities to 2050 for 19 global regions. These projections rely on a range of key assumptions, drivers and exogenous trends, including demographic and technological developments, as described in OECD (2019[11]). The economic projections underlying the five scenarios reflect the steady economic growth characterising OECD countries and faster economic growth characterising non-OECD economies3 (OECD, 2019[11]). This difference in economic growth trends is due to the long-term convergence process of non-OECD countries towards the OECD’s average income levels, conditional on country-specific circumstances. Annex A contains more details on the ENV-Linkages model.
2. The economic activities are then used to obtain projections of air pollution emissions to 2050. This is done using the GAINS model (Höglund-Isaksson et al., 2020[4]; Winiwarter et al., 2018[5]; Klimont et al., 2017[6]; Amann et al., 2011[3]). Although this technology-based model has high spatial and sectoral resolution, this report relies on more aggregate information. Specifically, the analysis in this report uses activity-specific emissions projections for 48 countries and regions.4 The pollutants projected in this report are: black carbon, organic carbon, sulphur dioxide, nitrogen oxides, volatile organic compounds, nitrous oxide, carbon monoxide, and ammonia. Due to lack of data, the report does not cover other pollutants that affect the Arctic region, such as mercury (AMAP/UN Environment, 2019[12]) and persistent organic pollutants (POPs) (AMAP, 2016[13]).
3. Projected emissions are then used to calculate atmospheric concentrations of PM2.5 and ground-level ozone, relying on the Fast Scenario Screening Tool TM5-FASST, a global air quality source-receptor model developed by the European Commission’s Joint Research Centre (EC-JRC) (Van Dingenen et al., 2018[10]). Based on the aggregate emission projections provided by GAINS, TM5‑FASST provides grid-level concentrations of PM2.5 and ground-level ozone on a 1-degree grid. These are used as an input to calculate the country-specific biophysical impacts of air pollution. In order to provide a comprehensive assessment of air pollutant concentrations, TM5‑FASST completes emission projections with additional data on emission sources that are not covered in GAINS and ENV-Linkages. These include natural emission sources (desert dust and sea salt), as well as emissions from forest fires, which are obtained from the Coupled Model Intercomparison Project (CMIP) projections. The TM5-FASST model also provides population-weighted5 concentrations of air pollutants by country, which serve as an indicator of human exposure to air pollution.
4. Pollutant concentrations are used to calculate the biophysical impacts of air pollution on human health and agriculture. The biophysical impacts are described by a range of indicators, including mortality, hospital admissions and crop productivity losses (see Annex A). Health impacts at the country level are obtained using concentration-response functions based on the results of the Global Burden of Disease project for PM2.5 (Forouzanfar et al., 2015[14]) and ground-level ozone (Stanaway et al., 2018[15]) (see Annex B). There is evidence that some PM2.5 and ground-level ozone precursor gases such as NO2 have direct negative health effects (COMEAP, 2015[16]; EPA, 2016[17]). However, the lack of available data with global coverage reduced the possibilities to include the environmental impacts of these gases in the analysis. Finally, the agricultural impacts are calculated using the TM5-FASST model, which estimates the crop yield changes associated with ground-level ozone concentrations (Van Dingenen et al., 2018[10]; Van Dingenen et al., 2009[18]).
5. Finally, the economic consequences of air pollution policies are calculated, and distinguish between: (1) macroeconomic effects; and (2) the welfare improvements from reduced mortality and morbidity. Since this step is central to the main results on the economic consequences of air pollution policies, Section 2.3 presents additional details on the methodology used for the economic analysis.
The benefits of policy action are quantified as the difference in results between the policy scenarios and the baseline scenario.6
2.3. Quantifying the economic consequences of air pollution policies
This report quantifies the economic consequences of air pollution policies by drawing a distinction between two complementary effects (Figure 2.2):
1. Macroeconomic effects: how air pollution and air pollution policies affect economic growth.
2. Welfare improvements: the reduced mortality and incidence of illness that follow from better air quality when air pollution policies are implemented are presented in monetary values.
Figure 2.2. Schematic overview of the economic consequences of air pollution policies

Macroeconomic effects
The macroeconomic effects quantified reflect the impact of air pollution policies on the economic system as a whole, including impacts on expenditures, factor productivity, production, consumption and trade. The indicator used for the macroeconomic effect is gross domestic product, as in previous OECD work on the costs of environmental inaction (OECD, 2015[19]; OECD, 2016[9]). While GDP is not a perfect indicator of the success of environmental policies (Paltsev and Capros, 2013[20]), it is a common indicator of economic growth.7
The macroeconomic effects of air pollution policies are calculated relying on the OECD ENV-Linkages model. The model considers both the benefits from the reduced air pollution impacts, and the costs of air pollution policies based on the necessary investment in best available techniques (BATs). The overall macroeconomic effect of policy action therefore depends on the relative size of such benefits and costs.
The modelling framework takes into account both direct and indirect effects. For instance, changes in households’ health expenditures (direct costs) lead to changes in their consumption choices (indirect costs). Furthermore, in ENV-Linkages higher government spending encourages firms to increase investment, therefore leading to a positive effect on economic growth that partly offsets the initial costs.
The benefits of air pollution policies considered in ENV-Linkages result from reduced health expenditures, and improved labour and agricultural productivity (see Annex A for details). The input data to calculate these benefits are obtained by converting the biophysical impacts of air pollution (e.g. hospital admissions) into indicators that can be linked to ENV-Linkages’ variables and regional aggregation (e.g. changes in health expenditures). For instance, health expenditures are calculated by multiplying the number of hospital admissions by the unit costs attributed to a single hospital admission.
The costs of the policy scenarios are quantified in ENV-Linkages, including as inputs the estimates of the investment and expenditures (hereafter, investment) in new technologies for firms and households provided by the GAINS model. In ENV-Linkages, the deployment of cleaner technologies is set to create additional capital stock that allows less-polluting production processes for each sector and for households.8
These input data on investment are higher in the MTFR-AC scenario than in the CLE scenario, with some differences across sectors and regions (Figure 2.3). In those regions or sectors where stringent emission controls are already in place, investments associated with the adoption of the BATs are already included in the CLE scenario, rendering the difference in investment between the two scenarios very small. However, overall for Arctic Council countries, the MTFR-AC scenario sees substantial additional investments in the residential, energy and industry sectors, which have high potential for emission reductions.
Figure 2.3. Arctic Council country investment in BATs
Value in million USD, 2017 PPP exchange rates, 2050

Note: In the graph, for each bar, the sum of grey and blue colours reflects MTFR-AC levels, while blue reflects CLE levels. Scales differ for each country. MTFR-AC: Maximum Technically Feasible Reduction in Arctic Council countries only; CLE: Current Legislation (Baseline); PPP: purchasing power parity.
Source: IIASA’s GAINS model.
The differences in investment across sectors reflect the different costs and emission reduction potentials of the BATs, as well as the sectoral contribution to national emissions. Overall, two-thirds of the investment needed to achieve emission reductions under the CLE scenario are in the transport sector. This includes low-sulphur fuel for ocean ships, alongside end-of-pipe reductions that provide equivalent emission reductions in the case that high-sulphur fuel is used. The implementation of the best available techniques in the MTFR-AC scenario would require greater investments targeting the residential, energy, and agricultural sectors. In fact, about half of the additional investments under the MTFR-AC scenario are devoted to the residential sector, especially in Canada, the United States and the Nordic Arctic Council countries.9 The energy and industry sector is the second by volume of additional investments under the MTFR-AC scenario, with most of these investments projected to take place in Russia.
Welfare improvements
The welfare improvements from reduced air pollution considered in this report include reduced air pollution-related mortality, and pain and suffering caused by illness. These effects are monetised using economic valuation techniques (see Annex B for details).
The welfare costs associated with mortality are calculated using the value of a statistical life (VSL), following previous OECD work (OECD, 2014[21]). This is a long-established metric, which can be quantified by aggregating individuals’ willingness to pay (WTP) to secure a marginal reduction in mortality risk over a given timespan (Box 2.3) (OECD, 2012[22]; OECD, 2014[21]; Roy and Braathen, 2017[23]). The OECD environmental indicator database (OECD, 2020[24]) provides country-specific VSL values for adults for OECD member countries and some non-OECD economies. These VSLs, as well as values for other countries not covered by the database, are calculated using a benefit transfer methodology (Box 2.3).10
Alongside mortality, this report accounts for several morbidity impacts, including adult and childhood bronchitis, respiratory and cardiovascular illness, and asthma symptom days for children. While the healthcare costs included in the modelling analysis reflect the expenditures linked with each case of illness (e.g. the costs of medicines), the welfare costs of morbidity reflect the pain and suffering of each case of illness.
Welfare costs of morbidity impacts are monetised using previous work by the European Commission (Holland, 2014[25]), which established unit values for the welfare costs of each morbidity impact (reported in Annex B). Adjustment of morbidity welfare costs to specific countries is based on income, with the benefit transfer methodology used for mortality (Box 2.3).
Box 2.3. Valuing mortality using the value of a statistical life
One of the most common procedures to value risks to life in standard economic theory is the value of a statistical life (VSL) (OECD, 2006[26]). The VSL is derived from aggregating individuals’ willingness to pay to secure a marginal reduction in mortality risks over a given timespan.
The VSL is most commonly elicited through stated preference techniques, although revealed preferences techniques are also used. OECD (2012[22]) describes the basic process for deriving a VSL from a stated preference survey. The VSL is not the value of an identified person’s life, but rather an aggregation of individual values for small changes in risk of death (OECD, 2012[22]). As such, the total economic cost of the impact equals the VSL multiplied by the number of deaths; the economic benefit of a mitigating action becomes the same VSL multiplied by the number of lives saved (OECD, 2014[21]).
Since not all countries have a specific VSL value that they use for cost-benefit analysis, country-specific VSL values are established based on average national income, using a benefit transfer methodology (OECD, 2012[22]; OECD, 2014[21]). The key parameter in this methodology is the elasticity of income, which determines the extent to which the VSL changes according to different income levels. This approach allows for comprehensiveness and comparability across countries by using the same methodology and reference VSL. Furthermore, the VSL can be adapted over time to the income changes in the economic projections for each country. While the methodological choices and parameter values used could affect the magnitude of the results, the overall results and policy messages should not be affected. A sensitivity analysis to the values of the income elasticities is presented in OECD (2016[9]).
Mortality can also be valued using the “value of a life year lost” (VOLY). This technique calculates the number of “years of life lost” (YOLLs) from a specific risk, based on an estimated life expectancy, and then evaluates them by multiplying them by the VOLY. One issue with this technique is that the combination of counting YOLLs, rather than lives lost, means that the VOLY approach “explicitly places a lower value on reductions in mortality risk accruing to older populations with lower quality of life” (Hubbell, 2002[27]). There are also major complications in the robust estimation of YOLLs, and the extent to which existing country-specific life expectancy values can and should be used. Given the limitations of the use of VOLYs, and following OECD (2012[22]; 2014[21]), mortality is evaluated with the same VSL for all age groups in this report.
References
[7] Amann, M. et al. (2018), Progress Towards the Achievement of the EU’s Air Quality and Emissions Objectives, International Institute for Applied Systems Analysis (IIASA), Laxenburg.
[3] Amann, M. et al. (2011), “Cost-effective control of air quality and greenhouse gases in Europe: Modeling and policy applications”, Environmental Modelling & Software, Vol. 26/12, pp. 1489-1501, http://dx.doi.org/10.1016/j.envsoft.2011.07.012.
[28] Amann, M. et al. (2017), Costs, Benefits and Economic Impacts of the EU Clean Air Strategy and their Implications on Innovation and Competitiveness, International Institute for Applied Systems Analysis (IIASA), Laxenburg.
[13] AMAP (2016), AMAP Assessment 2015: Temporal Trends in Persistent Organic Pollutants in the Arctic. Arctic Monitoring and Assessment Programme (AMAP), AMAP, Tromsø.
[12] AMAP/UN Environment (2019), Technical Background Report for the Global Mercury Assessment 2018, AMAP/UN Environment, Tromsø.
[31] Chantret, F. et al. (2020), “Can better technologies avoid all air pollution damages to the global economy?”, Climatic Change, Vol. 163/3, pp. 1463-1480.
[1] Chateau, J., R. Dellink and E. Lanzi (2014), “An Overview of the OECD ENV-Linkages Model: Version 3”, OECD Environment Working Papers, No. 65, OECD Publishing, Paris, https://dx.doi.org/10.1787/5jz2qck2b2vd-en.
[16] COMEAP (2015), Statement on the evidence for the effects of nitrogen dioxide on health., COMEAP, London, https://www.gov.uk/government/publications/nitrogen-dioxide-health-effects-of-exposure.
[17] EPA (2016), Integrated Science Assessment for Oxides of Nitrogen – Health Criteria, Environmental Protection Agency, Washington DC, https://cfpub.epa.gov/ncea/isa/recordisplay.cfm?deid=310879.
[14] Forouzanfar, M. et al. (2015), “Global, regional, and national comparative risk assessment of 79 behavioural, environmental and occupational, and metabolic risks or clusters of risks in 188 countries, 1990-2013: a systematic analysis for the Global Burden of Disease Study 2013”, The Lancet, Vol. 386/10010, pp. 2287-2323, http://dx.doi.org/10.1016/S0140-6736(15)00128-2.
[32] Harmsen, M. et al. (2020), “Taking some heat off the NDCs? The limited potential of additional short-lived climate forcers’ mitigation”, Climatic change, pp. 1443–1461.
[4] Höglund-Isaksson, L. et al. (2020), “Technical potentials and costs for reducing global anthropogenic methane emissions in the 2050 timeframe –results from the GAINS model”, Environmental Research Communications, Vol. 2/2, p. 025004, http://dx.doi.org/10.1088/2515-7620/ab7457.
[25] Holland, M. (2014), Cost-benefit Analysis of Final Policy Scenarios for the EU Clean Air Package, Corresponding to IIASA TSAP Report No. 11, International Institute for Applied Systems Analysis (IIASA), Laxenburg, http://ec.europa.eu/environment/air/pdf/TSAP%20CBA.pdf (accessed on 9 March 2021).
[27] Hubbell, B. (2002), Implementing QALYs in the Analysis of Air Pollution Regulations, US Environmental Protection Agency, Washington, DC, https://www3.epa.gov/ttnecas1/workingpapers/ereqaly.pdf (accessed on 7 December 2020).
[2] IEA (2018), World Energy Outlook 2018, International Energy Agency, Paris, https://dx.doi.org/10.1787/weo-2018-en.
[6] Klimont, Z. et al. (2017), “Global anthropogenic emissions of particulate matter including black carbon”, Atmospheric Chemistry and Physics, Vol. 17/14, pp. 8681-8723, http://dx.doi.org/10.5194/acp-17-8681-2017.
[24] OECD (2020), Air quality and health: Mortality and welfare cost from exposure to air pollution (database), Statistics, OECD Environment, https://doi.org/10.1787/c14fb169-en (accessed on 3 November 2020).
[11] OECD (2019), Global Material Resources Outlook to 2060: Economic Drivers and Environmental Consequences, OECD Publishing, Paris, https://dx.doi.org/10.1787/9789264307452-en.
[9] OECD (2016), The Economic Consequences of Outdoor Air Pollution, OECD Publishing, Paris, https://dx.doi.org/10.1787/9789264257474-en.
[19] OECD (2015), The Economic Consequences of Climate Change, OECD Publishing, Paris, https://dx.doi.org/10.1787/9789264235410-en.
[21] OECD (2014), The Cost of Air Pollution: Health Impacts of Road Transport, OECD Publishing, Paris, https://dx.doi.org/10.1787/9789264210448-en.
[22] OECD (2012), Mortality Risk Valuation in Environment, Health and Transport Policies, OECD Publishing, Paris, https://dx.doi.org/10.1787/9789264130807-en.
[26] OECD (2006), Cost-Benefit Analysis and the Environment: Recent Developments, OECD Publishing, Paris, https://dx.doi.org/10.1787/9789264010055-en.
[20] Paltsev, S. and P. Capros (2013), “Cost concepts for climate change mitigation”, Climate Change Economics, Vol. 04/supp01, p. 1340003, http://dx.doi.org/10.1142/s2010007813400034.
[29] Rao, S. et al. (2016), “A multi-model assessment of the co-benefits of climate mitigation for global air quality”, Environmental Research Letters, Vol. 11/12, p. 124013, http://dx.doi.org/10.1088/1748-9326/11/12/124013.
[23] Roy, R. and N. Braathen (2017), “The rising cost of ambient air pollution thus far in the 21st century: results from the BRIICS and the OECD countries”, OECD Environment Working Papers, No. 124, OECD Publishing, Paris, https://dx.doi.org/10.1787/d1b2b844-en.
[30] Smith, S. et al. (2020), The Energy Modeling Forum (EMF)-30 study on short-lived climate forcers: introduction and overview, Springer Science and Business Media B.V., http://dx.doi.org/10.1007/s10584-020-02938-5.
[15] Stanaway, J. et al. (2018), “Global, regional, and national comparative risk assessment of 84 behavioural, environmental and occupational, and metabolic risks or clusters of risks for 195 countries and territories, 1990–2017: a systematic analysis for the Global Burden of Disease Study 2017”, The Lancet, Vol. 392/10159, pp. 1923-1994, http://dx.doi.org/10.1016/s0140-6736(18)32225-6.
[8] UNFCCC (2015), “Paris Agreement”, United Nations Framework Convention on Climate Change, (UNFCCC), New York, https://unfccc.int/sites/default/files/english_paris_agreement.pdf. (accessed on 1 December 2020).
[10] Van Dingenen, R. et al. (2018), “TM5-FASST: a global atmospheric source–receptor model for rapid impact analysis of emission changes on air quality and short-lived climate pollutants”, Atmospheric Chemistry and Physics, Vol. 18, pp. 16173-16211, https://doi.org/10.5194/acp-18-16173-2018.
[18] Van Dingenen, R. et al. (2009), “The global impact of ozone on agricultural crop yields under current and future air quality legislation”, Atmospheric Environment, Vol. 43/3, pp. 604-618, http://dx.doi.org/10.1016/j.atmosenv.2008.10.033.
[5] Winiwarter, W. et al. (2018), “Technical opportunities to reduce global anthropogenic emissions of nitrous oxide”, Environmental Research Letters, Vol. 13/1, p. 014011, http://dx.doi.org/10.1088/1748-9326/aa9ec9.
Notes
← 1. ENV-Linkages does not fully incorporate the investment costs of energy-saving technologies in non-power industries, services and agricultural sectors, due to lack of information.
← 2. Impact pathway assessments estimate environmental benefits and costs following the pathway from the sources of the environmental damage (in this case emissions of air pollutants), via changes in environmental quality (air quality), to physical impacts, before being expressed in monetary benefits and costs.
← 3. The economic projections underlying the scenarios presented in this report do not consider the current COVID-19 pandemic and its effect on economic growth.
← 4. The methodology used for create emission projections relies on the methodology used in the EU FP7 project on Low climate IMpact scenarios and the Implications of required Tight emission control Strategies (LIMITS) (Rao et al., 2016[29]), which was also used in previous OECD work (OECD, 2016[9]), and on the 30th Energy Modelling Forum (EMF30) modelling comparison exercise on the potential role of Short-Lived Climate Forcers (SLCF) mitigation in climate policy (Smith et al., 2020[30]), which also included the ENV‑Linkages model (Chantret et al., 2020[31]; Harmsen et al., 2020[32]).
← 5. The population-weighted average concentrations link population density to air pollution exposure. This indicator reflects the fact that areas with high population density imply higher exposure to air pollution, thereby capturing more accurately the exposure to air pollution in that country.
← 6. In this modelling set up, the damage from air pollution affects economic output and growth. Therefore, in principle, for each scenario, emissions, concentration levels and impacts of air pollution should be re-assessed after considering the economic feedbacks from air pollution. This additional step should be repeated until convergence is reached between all steps in the framework. However, the reductions in economic activity due to the air pollution impacts for each scenario are limited and estimated to be around 1% of emissions in the baseline scenario (OECD, 2016[9]). Therefore, the second-order effect of lower emission projections on concentrations and impacts is very small, and can be ignored in the light of the uncertainties surrounding all calculations in this report.
← 7. An alternative choice would have been to calculate the welfare impacts of the different scenarios in the ENV-Linkages model. Following previous OECD work (OECD, 2016[9]), the choice was made to highlight impacts on output and growth in the modelling analysis and to separately assess the welfare impacts of the policy scenarios with valuation techniques.
← 8. This methodology is similar to the one used in the GEM-E3 model of the European Commission (Amann et al., 2017[28]).
← 9. The Nordic Arctic Council countries are Denmark, Finland, Iceland, Norway and Sweden.
← 10. The income elasticity used for the calculations is 0.8 for high-income countries, 0.9 for middle-income countries and 1 for low-income countries. See Annex B for a thorough description of the benefit-transfer methodology and for a discussion of the elasticity values.