The gender gaps on the Estonia labour market remain stubbornly persistent and have not noticeably decreased over the past two decades. This chapter assesses past economic gains from increases in female employment and includes projections of potential economic growth under a range of different labour market scenarios for men and women in Estonia. Section 7.2 considers the labour market position of Estonian men and women and discusses gains that have been made from increasing female employment in Estonia and selected OECD countries. Section 7.3 provides two separate assessments of the potential economic gains from closing gender gaps: i) a cross-country assessment of the economic gains from partially or fully closing gender gaps in labour force participation and working hours by 2050 in Estonia and selected OECD countries, and ii) an analysis of country-specific scenarios on potential economic output.
The Economic Case for More Gender Equality in Estonia
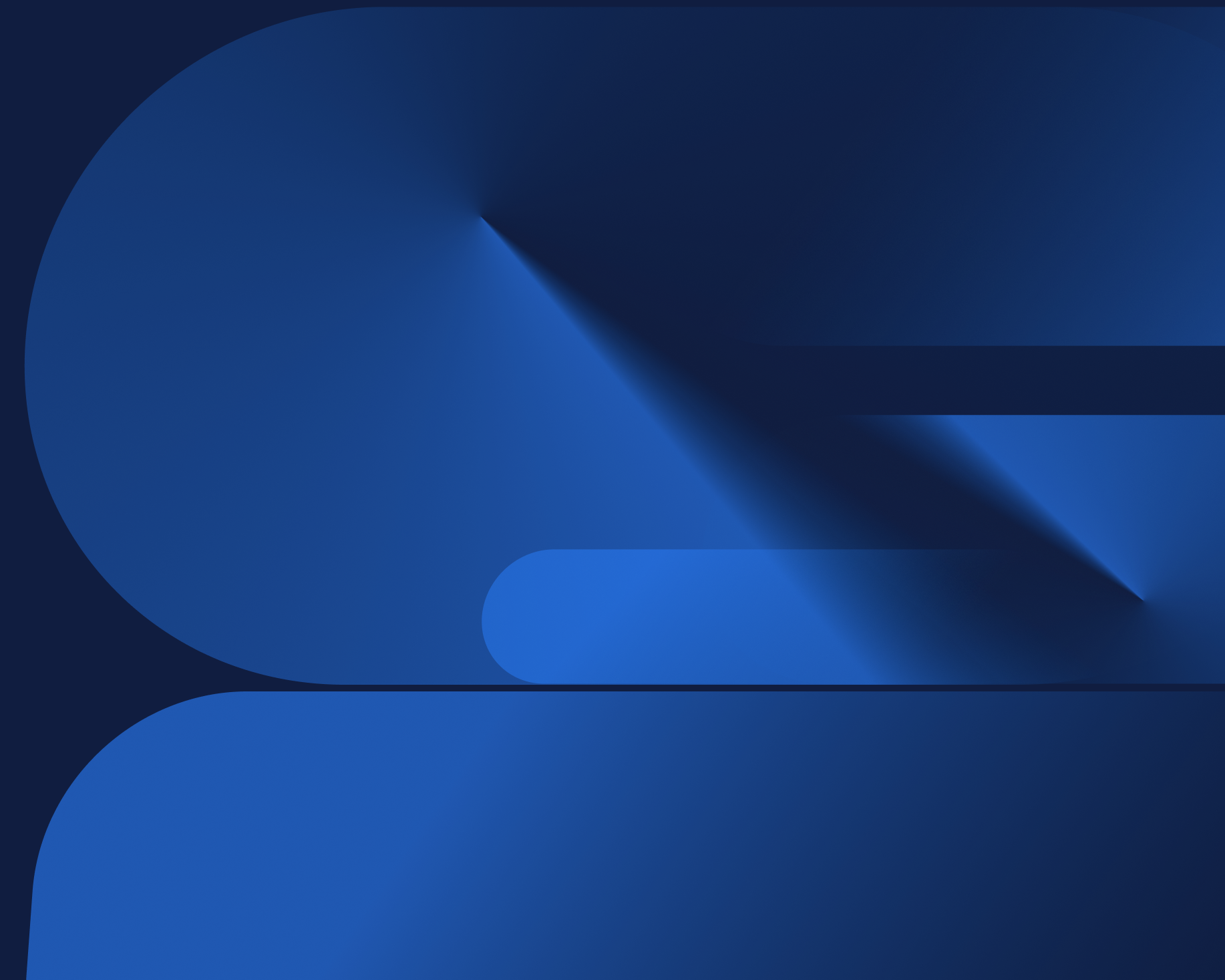
7. Gender equality pays in Estonia
Abstract
7.1. Introduction and main findings
Women’s employment is an important contributor to economic performance across countries. The persistence of unequal chances of labour market success for men and women does not only go against the moral imperative of gender equality on the labour market and in society as a whole, it also entails significant economic costs by excluding talents from the labour market and by preventing many among those employed from realising their full productive potential.
Women’s labour input and the utilisation of their talent, boost national output considerably, and increasing labour market participation of women across the OECD has therefore been an important driver of economic growth over past decades (see e.g. OECD (2018[1])). But how important have changes in women’s employment outcomes been for Estonia? To answer this question, this chapter looks at employment trends for men and women in Estonia and selected neighbouring countries over the past 20 years, and based on the changes in these outcomes, estimates their relative contribution to the growth in economic output. Subsequently, the chapter looks at different future labour force scenarios for men and women and how these could potentially affect economic development in the years to come.
The chapter relies on two frameworks two measure the importance women’s employment on past economic output and the potential for additional future growth. The first framework uses growth accounting – a procedure used to identify and decompose the sources of past economic growth. With this, it is possible to account for the contribution increases of women’s labour market engagement had for economic growth over the past 20 years, which has been quite substantial for Estonia. The second framework uses the OECD long-term growth model to estimate the impact of greater gender equality on the labour market for future economic output. The exercise draws on hypothetical scenarios of convergence between men’s and women’s employment rates, working hours, productivity and life expectancy, and reports the deviations from the baseline in terms of GDP per capita growth.
7.2. Women’s employment and past economic growth
7.2.1. The labour market outcomes of Estonian men and women over the past 20 years
The previous chapters have shown that gender gaps on the Estonian labour market remain stubbornly persistent. Even though the gaps in employment rates and working hours are comparatively small in relation to the OECD average, the past 20 years have not shown a substantial reduction of gender gaps in employment rates and working hours. For example, based on National Accounts data estimates, 75% of Estonian men and 69% of Estonian women aged 15‑64 were employed in 2019 (see Figure 7.1). For women, this is slightly above the OECD average, though the gap between men and women in Estonia is hardly different from where it stood in 2000 (7 percentage points). While men’s employment rates are generally higher than women’s employment rates across the OECD, some other countries have seen a convergence of the two. In Germany, for example, the gender gap in employment rates was halved over the past two decades (from 16 to 8 percentage points).
Despite generally stronger decreases in men’s working hours, the total hours worked by male employees are still higher than those of female employees across all countries (see Figure 7.2). However, in Estonia, the difference between male and female working hours is noticeably smaller than for the OECD and EU average – while Estonian women worked for about 1 829 hours per year in 2019, men worked only 121 hours more (1950 hours). Despite being small, this gap has not closed over the past 20 years, contrary to some other countries. For example, the large working hour’s gap in Iceland shrunk from 653 hours in 2000, to 309 hours in 2019. Over the same period, women’s working hours increased in Lithuania, Norway and Sweden.
Figure 7.1. Gender employment gaps have narrowed in many countries, but not in Estonia
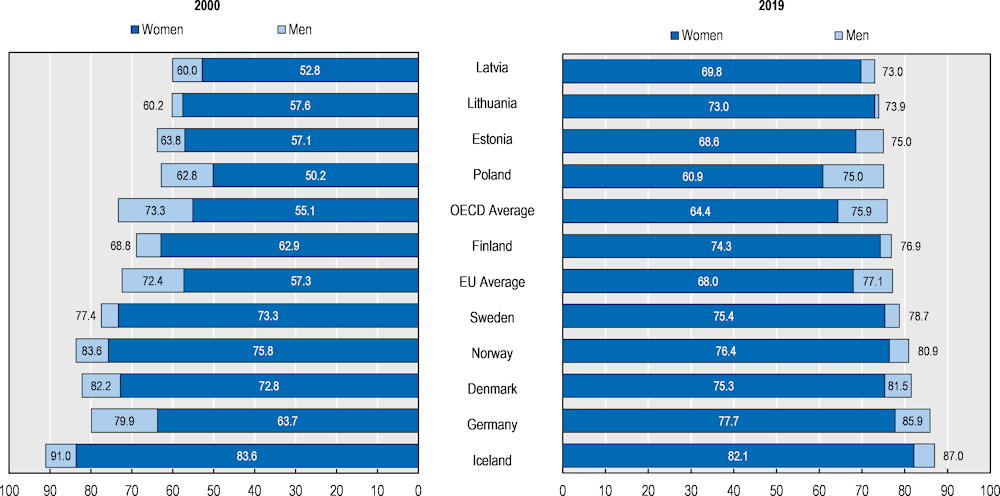
Note: The figure is based on the National Accounts data, which provide cross-country macroeconomic indicators on economic output and input factors. Employment input in the National accounts may differ from Labour Force Survey estimates – for various coverage, definitional and methodological reasons (see e.g. Ward, Zinni and Marianna (2018[2])). The OECD and EU averages only include countries for which the necessary data for the growth accounting exercise is fully available between 2000 and 2019. The OECD average here does not include Australia, Chile, Colombia, Costa Rica, the Czech Republic, Japan, Korea, Luxembourg, Mexico, New Zealand, and Türkiye. The EU average does not include EU countries that do not belong to the OECD, Luxembourg, and the Czech Republic. See Annex 7.B for more detail.
Source: OECD estimates based on data from the OECD National Accounts Database, http://www.oecd.org/std/na/ and the OECD Employment Database, http://www.oecd.org/employment/emp/onlineoecdemploymentdatabase.htm.
Figure 7.2. The decline in men’s working hours outpaced that of women, but less so in Estonia
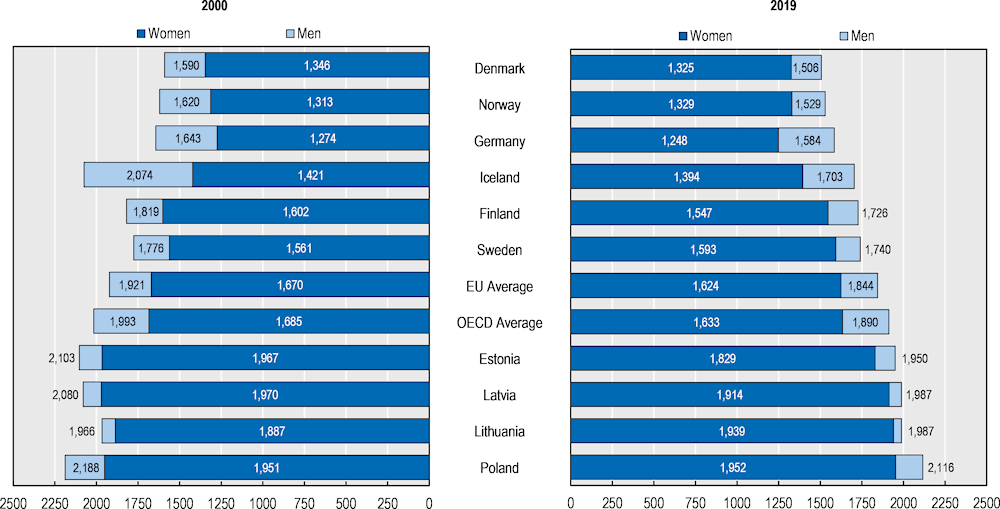
Note: The figure is based on the National Accounts data, see notes to Figure 7.1. See Annex 7.B for more detail.
Source: OECD estimates based on data from the OECD National Accounts Database, http://www.oecd.org/std/na/ and the OECD Employment Database, http://www.oecd.org/employment/emp/onlineoecdemploymentdatabase.htm.
Accounting for the link between women’s employment and past economic growth
To what extent have changes in women’s labour input contributed to past economic growth? To answer this question, this section draws together historical time‑series on macroeconomic performance and women’s employment, and combines them with a growth accounting exercise (for an overview see Box 7.1). In this exercise, growth in GDP per capita is decomposed into parts that can be accounted for by changes in: labour productivity, the working-age share of the population, as well as male and female headcount employment and total annual hours worked by men and women aged 15 to 64 (see Annex 7.B for more on methods and detail).
Some limits to this exercise are important to state at the outset. For instance, the estimates shown here do not account for the impact of any changes in female paid work on unpaid work and household production, which has considerable, though not monetarily remunerated, economic value in itself (see e.g. OECD (2021[3])). As a result, the estimates may overstate the boost to actual living standards enjoyed by households that follows an increase in women’s employment. Estimates also do not account for any gender differences in productivity, and implicitly assume that one additional hour of labour input produces the same output regardless of sex, age, education, and/or any other factor. The growth accounting exercise is also predominantly based on National Accounts data (OECD, 2021[4]), which are not always fully consistent with estimates from Labour Force Surveys – for various coverage, definitional and methodological reasons (see e.g. Ward, Zinni and Marianna (2018[2])).
Increases in female employment outcomes across the OECD have been an important driver of economic growth over the 20 years between 2000 and 2019. Figure 7.3 and Annex Table 7.A.1 summarise results from the growth accounting exercise for Estonia and selected neighbouring OECD countries, by presenting the average annual growth in GDP per capita (in percentage) and the percentage point contribution of each included component. With output increasing about 3.93% on average per year, Estonia has enjoyed considerable economic growth over this period, well above the OECD total (1.20%) and comparable to its direct neighbours in the Baltic countries (Latvia and Lithuania).
While increases in labour productivity had the greatest impact on economic growth across the OECD overall (+1.20 percentage points), increases in female employment have been important as well (+0.28 percentage points). Overall, women’s employment has been particularly important in countries with generally higher average annual growth, such as Estonia’s Baltic neighbours Latvia (+0.72 percentage points) and Lithuania (+0.61 percentage points). Despite seeing much more modest growth, Finland and Germany also benefitted considerably from increases in women’s employment (+0.40 p.p. and +0.47 percentage points, respectively), predominately driven by large increase in the female employment rate. In the other Nordic countries, much of the increase in female employment rates were realised before 2000, and are thus less important for the time‑frame considered here (see OECD (2018[1])).
With particularly stable gender employment gaps in Estonia however, the economic growth of the past 20 years has been driven more by changes in male employment (+0.57 percentage points) than by changes in female employment (+0.40 percentage points). The main reason for the weaker contribution of female than male employment on economic growth is the change in the female working-age population. To illustrate this, Annex Table 7.A.2 further decomposes the gender-disaggregated employment components of the growth accounting exercise into a part attributable to changes in the male and female employment rates and a part attributable to changes in their share of the working-age population. In Estonia, the female share of the working-age population has been shrinking between 2000 and 2019, such that the population aged between 15 and 64 is now made up of equal parts men and women. The decline in the female working-age share relative to that of men dampened the positive contribution of female employment rates alone, which sum up to 0.49 percentage points of annual growth (the “raw” effect of employment rates), by 0.09 percentage points.
Box 7.1. Measuring the contribution of changes in women’s employment to economic growth
The analysis in this section is based on growth accounting – a procedure used to identify and decompose the sources of past economic growth. A detailed overview of the procedure is given in Annex 7.B, but the basic idea is to use information on observed trends in macroeconomic indicators to split growth into its main components parts – that is, under standard macroeconomic theory, into labour, capital, and total factor productivity (Solow, 1956[5]). Data permitting, the contribution of each of these main components can then be further decomposed into their sub-components. For example, the contribution of labour input to growth can be split between changes in employment in the different sectors of the economy, while the contribution of capital can be divided between ICT-related capital (e.g. computer hardware, telecommunications equipment, and computer software) and non-ICT capital (more traditional forms of capital, like machinery and transport equipment).
In this report, the focus is on the contribution of labour input to economic growth and, in particular, on the contribution of women’s labour input. Growth accounting is used to identify and separate the contribution of labour from the other two main components – capital and total factor productivity, from here on labelled together as “labour productivity” – and then to decompose the contribution of labour input itself. Labour input is, in this instance, separated into three parts: changes in the size of the working-age population, the employment rate, and average hours worked per employed person. The contributions of changes in both the employment rate and average working hours are decomposed by gender and, where possible, by age group too. These two factors are where the main interest lies as they provide information on the extent to which changes in men’s and women’s employment rates and working hours have contributed to economic growth.
Note: See OECD (2018[1]) for a previous growth accounting exercise that focussed on the Nordic countries.
Except for Denmark, declines in the female working-age share are present in all other presented countries, and thus dampen the positive contribution of raw female employment increases, though this effect is weaker than in Estonia (Annex Table 7.A.2). These small changes in the gender-composition of the working-age population can, for example, in part be attributed to the moderate convergence of life expectancy for men and women for most age groups across the OECD (see e.g. OECD Health Database or Raleigh (2019[6])).
For Estonia and most of its neighbours, the total working hours of men and women in have been decreasing since 2000 (see Figure 7.2). In these countries, changes in working hours generally had a dampening effect on economic growth as the total labour input per worker diminished. In most countries, the reduction of men’s working hours was greater than for women’s working hours. In Germany and Lithuania, increases in female working hours had a positive effect on the average annual GDP per capita growth. Across the OECD, changes in men’s working hours reduced average annual growth by 0.32 percentage points, while changes in women’s working hours increased growth by 0.03 percentage points per year.
The development in Estonia stands in contrast to its neighbours and the OECD overall. Here, the changes in women’s working hours (‑0.26 percentage points) have reduced economic growth stronger than changes in men’s working hours (‑0.16 percentage points), while the “raw” decrease in hours worked per year was very similar for men and women (see Figure 7.2). Similar to the employment component discussed above, this can mainly be attributed to the decrease of women’s share among the working-age population, which decrease women’s share of the employed labour force – even as the employment rates of men and women grew at a similar pace. A further decomposition of the gender-disaggregated working hours, therefore, shows that as the female share of all employed in the Estonian labour force has decreased between 2000 and 2019, it reduced average growth by 0.07 percentage points per year (see Annex Table 7.A.3). As a result, the female share of the working hours component in the growth accounting exercise shrunk between 2000 and 2019, increasing the negative effect of the “raw” decrease in female hours worked (‑0.19 percentage points) further. With the decrease in the female employed labour force, the male share of the employed labour force increased and thus the stronger “raw” effect of the reduction of male working hours (‑0.23 percentage points) is dampened somewhat. An opposite pattern can be observed in Germany, where the contribution of the raw decrease in female working hours on average annual economic growth was negative (‑0.04 percentage points), but the strong increase in the female share of the employed labour force makes the economic contribution of female working hours positive (+0.09 percentage points).
Figure 7.3. Gains in female employment have been an important driver of GDP growth (2000‑19)
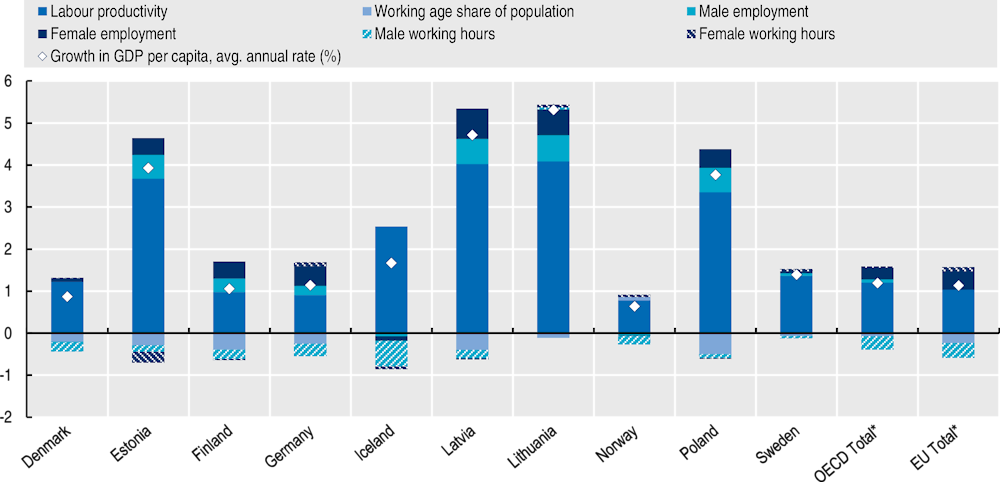
Note: Estimates based on the decomposition of national accounts data using labour force survey estimates. See notes to Figure 5.1 for OECD and EU totals. See Annex 7.B for more detail.
Source: OECD estimates based on data from the OECD National Accounts Database, http://www.oecd.org/std/na/ and the OECD Employment Database, http://www.oecd.org/employment/emp/onlineoecdemploymentdatabase.htm.
7.3. Closing gender employment gaps and their potential impact on economic growth
The following sections of this chapter look to the future and aim to project how much Estonia could gain from closing remaining gender gaps in employment outcomes. It concentrates first on the potential economic gains from partially or fully closing gender gaps in labour force participation (LFP) rates and working hours for Estonia and selected OECD countries. In a second step, the chapter zooms in on Estonia in detail by considering potential economic effects of weakening some of the underlying gender norms that drive gender gaps on the countries labour market (e.g. differences in unpaid work and educational choices), as well as the considerable difference in life expectancy and healthy life years between men and women.
The potential impact of a narrowing of the gender gaps in labour market outcomes works primarily through an increase in labour input, through rising women’s aggregate working hours or growing the female labour force. More equal gender norms work predominately through increased access to more productive jobs for women, following more equal sharing of unpaid work at home and less gender stereotyping in educational choices, as well as increased female bargaining power and reduced bias and discrimination by employers. These changes also have spill-over effects to other employment outcomes, as increased access to more productive jobs could increase wages among women, which in turn could attract more women to enter the labour force.
The projections presented in this chapter follow simplified calculations based on the OECD Long-Term Model (Guillemette and Turner, 2018[7]; 2021[8]) and the OECD’s in-house labour force projection models. They take the OECD’s standard baseline projections until 2050 for both the size of the labour force between ages 15 and 74 and economic output and adjust estimates following a range of scenarios about future labour market development. These scenarios are based on a given set of assumptions about changes in the gender gaps in LFP rates, working hours, life expectancy as well as hourly wages and labour productivity. A detailed methodology that underlies the projections is available in Annex 7.B.
Similar to the growth accounting exercise above, these projections have clear limitations. For example, the resulting estimates are mechanical only and assume that any changes in LFP rates or working hours do not interact with factors outside of the model or have any indirect effects beyond its scope. In addition, only the detailed scenarios assume differences in male and female productivity. For technical reasons, the measures and units used here differ slightly from those used in the growth accounting exercise – while the earlier results included the 15‑64 year‑old age group, the estimates here are based on the 15‑74 year‑old age group. This is done to help ensure compatibility with the inputs used for the OECD’s standard long-term growth models and because the participation of over‑65 workers is likely only to increase in importance in future decades (OECD, 2020[9]; Geppert et al., 2019[10]). The outputs of the OECD’s in-house labour force projection models used here also project LFP rates, rather than employment rates. As a result, all economic production is presented as potential economic output (potential GDP per capita), which refers to economic output at full-employment of all members of the labour force.
In addition, the labour market projections for LFP rates and working hours used in this exercise are based on Labour Force Survey data, in contrast to the National Accounts data used on the growth accounting framework. As mentioned above, these sources are not always perfectly consistent with each other, but both are necessary for the respective exercises presented here. As a result, some of the presented figures presented in the dedicated sections may differ from each other. For example, the LFP rates based on the National Accounts data tend to be slightly higher than those based on Labour Force Surveys (Ward, Zinni and Marianna, 2018[2]).
7.3.1. Closing gender gaps in labour market outcomes: basic scenarios
The basic growth scenarios model changes in the gender gaps in labour force participation and working hours for Estonia and selected OECD countries, including Latvia and Lithuania and Nordic countries (Denmark, Finland, Iceland, Norway and Sweden), as well as Germany and Poland. These scenarios offer a cross-country perspective of the potential for additional economic growth that a closing of the present gaps may hold by 2050. The assumptions in this set-up are straightforward: The necessary adjustment for gender gaps in LFP and working hours to close is assumed to occur linearly between the projection start year (2020) and two target years (2035 and 2050). The basic scenarios do not say anything about the mechanism underlying the narrowing of gender gaps in the labour market. The section that zooms in on Estonia below has a closer look at potential drivers of narrowing gender gaps.
Closing gender gaps in labour force participation holds growth potential
In an initial step, the basic scenarios are modelled following slightly adapted and extended prescriptions of the G20 Brisbane declaration of closing the gender gap in employment 25% by 2025 – a benchmark target for international progress on gender equality in labour participation established by the G20 in 2014 (ILO, IMF, OECD and World Bank Group, 2014[11]). In the adapted target for this projection exercise, starting from the year 2020, this goal is pushed to 2035 and complemented with a target that closes 50% of the gap by 2050 in Scenario A, as well as a set of more ambitious goals in Scenario B:
Baseline: The baseline scenario, where LFP rates of men and women (aged 15‑74) are estimated using the OECD’s standard dynamic age‑cohort model, which projects participation rates (by gender and five‑year age groups) based on current (2016‑20) rates of labour market entry and exit. This scenario services as the reference or business-as-usual scenario.
Scenario A: gender LFP gaps reduced by 25% by 2035 and by 50% in 2050. In this scenario, within each five‑year age group, the LFP rate of the gender group with the highest probability of being in the labour force is held at the baseline and the LFP rate of the gender group with the lowest probability of being in the labour force is projected so that the gender LFP gap falls by 25% by 2035, and 50% by 2050 in each five‑year age group relative to the baseline.
Scenario B: gender employment gaps reduced by 50% by 2035 and by 100% in 2050. In this scenario, within each five‑year age group, the LFP rate of the gender group with the highest probability of being in the labour force is held at the baseline and the LFP rate of the gender group with the lowest probability of being in the labour force is projected so that the gender LFP gap falls by 50% by 2035, and 100% (i.e. is fully closed) by 2050 in each five‑year age group relative to the baseline.
Figure 7.4 shows how the aggregate labour force participation rate could evolve in Estonia and selected other OECD countries under each of the scenarios across the projection period between 2020 and 2050.
Over the early 2000s and well into the 2010s, the LFP rates in Estonia and Latvia rose between 8 and 10 percentage points, as both men and women increased their labour force participation over this period. This pattern is broadly similar to Germany but contrasts with the developments of potential employment in the Nordic countries, where over the same period Denmark, Finland and Norway experienced a decline in LFP rates while these stagnated in Iceland and Sweden. In Latvia and Lithuania, LFP-rates dropped sharply with the financial crisis at the end of the 2000s, but they have since increased to a level comparable with LFP-rates in Estonia. The increase of labour force participation in the three Baltic countries over the 2000‑19 period outpaced the rise in labour force participation across the EU and the OECD on average.
The baseline labour force projections from 2020 until 2050 point to rather stable LFP rates for Estonia, but also for Iceland and Sweden, as well as to a limited degree in the OECD overall. Some countries are projected to experience small decreases in LFP-rates well into the 2030s, which are then followed by a slight increase towards 2050 (e.g. Germany, Latvia and Lithuania). In all three of these countries, the LFP rate levels are projected to fall only slightly below 2020 levels by the year 2050. Poland, which already has the lowest LFP-rates among the presented countries, may see their labour force participation fall by a further 5 percentage points by 2050.
One major driver of these labour force trends for all countries are the projected demographic changes over the coming decades. As populations age, a lower population-share will be of working-age (age 15‑64), and thus, even a constant LFP-rate at each age will eventually lead to a decrease of aggregate labour supply in a given country as labour force participation usually declines for those beyond 65 years of age. In Estonia for example, about 64% of the population was aged 15‑64 in 2020, while demographic projections estimate a fall to 59.5% by 2050. Similar developments can be observed in virtually all countries presented here.
Figure 7.4. Closing gender gaps in labour force participation: basic scenarios
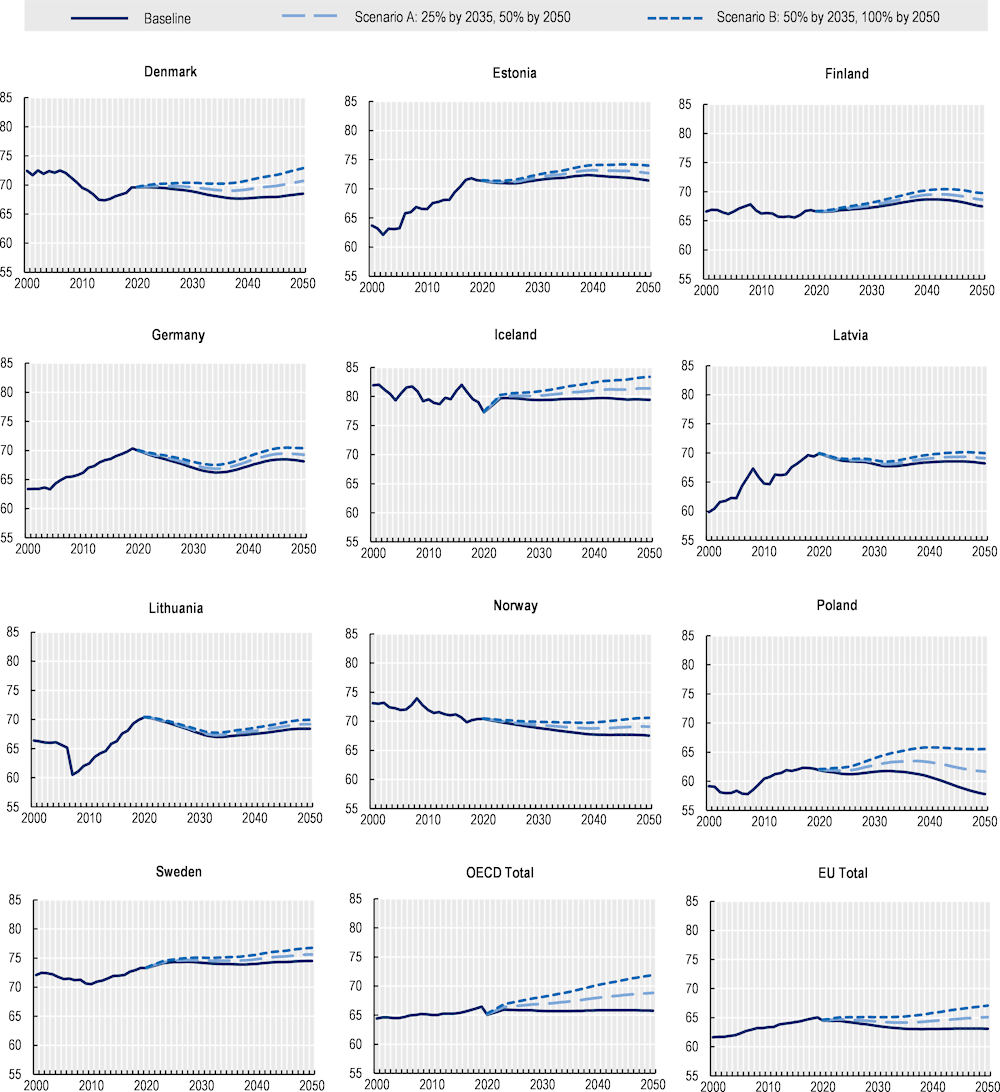
Note: Data based on Labour Force Surveys and OECD in-house labour force projections, see Annex 7.B. The OECD and EU totals are the weighted total across all OECD and EU member countries. For the OECD total, data for Türkiye is missing.
Source: OECD estimates based on OECD population data, https://stats.oecd.org/index.aspx?DataSetCode=POP_PROJ, the OECD Employment Database, http://www.oecd.org/employment/emp/onlineoecdemploymentdatabase.htm, and Employment Projections,.
At the same time, low fertility rates in the past affect how many people enter the labour force a couple of decades later. This negatively affects LFP-rates over time. In Estonia (1.85) and Latvia (1.75), average total fertility rates since the latter half of the 20th century (1950‑2015) have been below the TFR in Lithuania (2.01) (Manakov, Suvorkov and Stanaitis, 2017[12]). While more recent figures in these countries- 1.66 in Estonia as well as 1.61 in Latvia and Lithuania in 2019 – coincide with or fall slightly above the OECD total (1.61), they are well below levels that can offset population ageing and therefore result in a shrinking workforce and LFP rate (OECD Family Database). However, in some cases, recent trends in labour participation also play a role, especially those among young people in education. In Estonia, for example, labour force participation rates for young people in their early 20s (20‑24 year‑olds) has fallen in recent years, partly because they are staying longer in education and thus enter the labour force at a later age.
Closing gender LFP gaps and boosting female employment can reduce these expected declines in the overall LFP rate, or spur on additional increments to projected LFP-rate increases (Figure 7.4). As growing labour input increases economic production, rising female labour force participation towards male participation will increase total economic output. Figure 7.5 and Annex Table 7.A.4 show that the annual potential GDP per capita growth is projected to increase when gender gaps in labour force participation narrow and effects are particularly pronounced when the gender gap is assumed to be closed fully by 2050 (Scenario B).
Figure 7.5. Closing gender gaps in labour force participation will spur economic growth
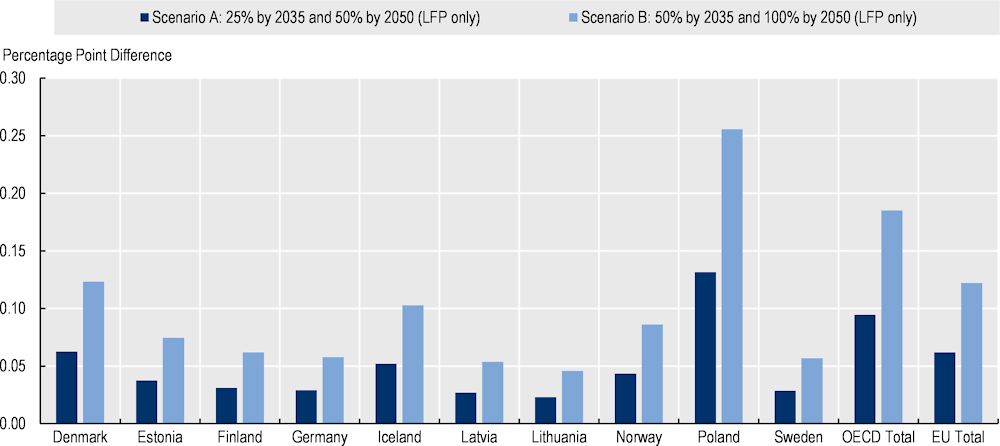
Note: See Annex 7.B for a description of the methods and data used. The OECD and EU totals are the weighted total across all OECD and EU member countries. For the OECD total, data for Türkiye is missing.
Source: OECD estimates based on OECD population data, https://stats.oecd.org/index.aspx?DataSetCode=POP_PROJ, the OECD Employment Database, http://www.oecd.org/employment/emp/onlineoecdemploymentdatabase.htm, and Employment Projections; and the OECD Long-Term Growth Model, https://doi.org/10.1787/b4f4e03e-en.
However, the magnitude of the potential increase in labour force participation varies across countries. The size of the effect naturally depends on the initial size of the gender employment gap. As such, countries with small differences in men’s and women’s labour force participation rates at baseline only record a limited effect. For example, Lithuania could expect 0.02 percentage points additional growth in potential GDP per capita per year in Scenario A and 0.05 percentage points in Scenario B. Estonia, has a slightly larger gender LFP gap at baseline, thus it could expect 0.04 percentage points or 0.07 percentage points of additional growth per year under the respective scenarios. This is noticeably smaller than the OECD (0.09 percentage points and 0.19 percentage points, respectively) and the EU total (0.06 percentage points and 0.12 percentage points, respectively). Of the countries presented, Poland could expect the largest increases in annual GDP growth, reaching up 0.13 and 0.26 percentage points in the respective scenarios. Despite lower gender LFP gaps in 2019, Denmark and Iceland see a smaller baseline convergence of men’s and women’s labour force participation rates until 2050 than Germany, where the gender gap in the LFP is projected to almost half over this period. Thus, the scenarios that close gender LFP gaps in Germany have a lower effect on economic growth relative to baseline than Denmark and Iceland. The accumulated boost for the Estonian potential GDP per capita ranges from 1.2% in Scenario A to 2.3% of additional potential output per capita in Scenario B in 2050.
Closing gender gaps in working hours would have limited effect on growth in the three Baltic countries
This section considers a narrowing of the working hours gap between men and women in combination with a narrowing of gender gaps in labour force participation (see Annex 7.B for details). The following working hours scenarios are considered first:
Scenario C: gender working hour gaps reduced by 25% by 2035 and by 50% in 2050. In this scenario, within each five‑year age group, the weekly working hours of the gender group with the highest hours are held at the baseline and the weekly working hours of the gender group with the lowest hours are projected to increase so that the gender working hour gap falls by 25% by 2035, and 50% by 2050 in each five‑year age group relative to the baseline.
Scenario D: gender working hour gaps reduced by 50% by 2035 and by 100% in 2050. In this scenario, within each five‑year age group, the weekly working hours of the gender group with the highest hours are held at the baseline and the weekly working hours of the gender group with the lowest hours are projected to increase so that the gender working hour gap falls by 50% by 2035, and 100% (i.e. is fully closed) by 2050 in each five‑year age group relative to the baseline.
Figure 7.6 shows the average weekly working hours fell or remained broadly stable until 2020 for all selected countries. The baseline scenario assumed that average hours of work remain stable within each 5‑year age group (see Annex 7.B). Any dynamics that are visible after 2020 are thus fully driven by demographic changes, but remain marginal in all cases.
While most Nordic countries have small gender gaps in labour force participation rate relative to the OECD total (see above), there are larger gaps between men and women in terms of working hours in some of these countries. For example, in 2020 Icelandic men worked 42.1 hours per week, while Icelandic women worked for only 34.6 hours. At the same time, men worked an average of 41.3 hours and women 35.1 hours per week across the OECD. In contrast, the Baltic countries have particularly small gender gaps in working hours. In Estonia, for example, men worked an average of 39.1 hours per week, while women did so for 36.7 hours. As a result, the Baltic countries see very limited changes in average weekly working hours when closing the gender gap working hours, while the Nordics and the OECD overall exhibit more noticeable changes under the different scenarios.
One of the reasons for the differences between Baltic and Nordic countries is the substantially lower incidence of part-time work among women in Estonia, Latvia and Lithuania (Riekhoff, Krutova and Nätti, 2019[13]). While 13% of Estonian women work part-time, 24% of Danish women did so in 2020, with comparable patterns across the other Baltic and Nordic countries (OECD, 2021[14]). In conjunction with generally shorter work weeks (e.g. 37 hours in Denmark), average working hours in the Nordic countries – except for Iceland – are thus well below the Baltic countries, yet relatively close to the OECD total (36.4 hours). The largest effects on potential labour force participation in terms of persons and hours are projected for Germany, where part-time work is more common among women than in Nordic countries (OECD Employment Database).
Figure 7.6. Closing gender gaps in working hours: basic scenarios
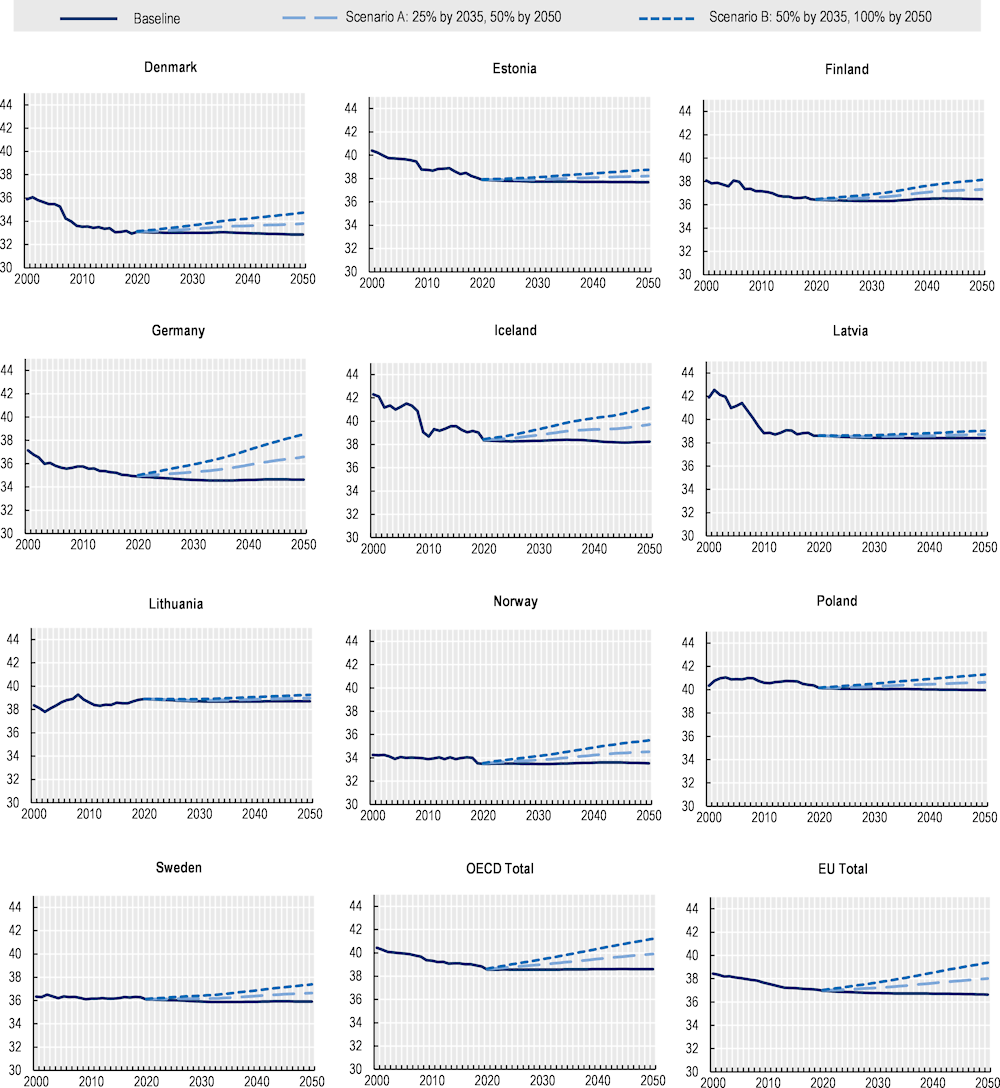
Note: Data based on Labour Force Surveys and OECD in-house labour force projections, see Annex 7.B. The OECD and EU totals are the weighted total across all OECD and EU member countries For the OECD total, data for Türkiye is missing.
Source: OECD estimates based on OECD population data, https://stats.oecd.org/index.aspx?DataSetCode=POP_PROJ and the OECD Employment Database, http://www.oecd.org/employment/emp/onlineoecdemploymentdatabase.htm, and Employment projections.
In view of the limited effects of closing gender gaps in working hours in Baltic countries, the associated projected changes to average annual potential GDP per capita between 2020 and 2050 are also relatively small (Figure 7.7 and Annex Table 7.A.4). While the OECD may grow by additional 0.07 percentage points per year in Scenario C and 0.14 percentage points in Scenario D, this would only be 0.03 percentage points and 0.06 percentage points for Estonia, respectively. In contrast, the Nordic countries and Germany could potentially benefit much more from a narrowing of the gender gap in working hours. Germany, for example, would see additional 0.12 percentage points of annual growth when closing the working hour gap by half, and 0.23 percentage points when closing it completely by 2050. The latter would correspond to a total accumulated difference in the potential GDP per capita of 7.4% in 2050, while for Estonia it would be much smaller with 1.9%.
Figure 7.7. Closing gender gaps in working hours shows similar growth potential
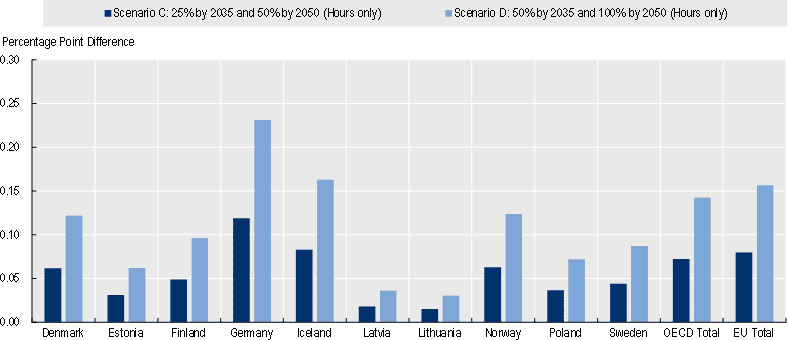
Note: See notes for Figure 7.5.
Source: OECD estimates based on OECD population data, https://stats.oecd.org/index.aspx?DataSetCode=POP_PROJ, the OECD Employment Database, http://www.oecd.org/employment/emp/onlineoecdemploymentdatabase.htmv, and Employment Projections; and the OECD Long-Term Growth Model, https://doi.org/10.1787/b4f4e03e-en.
Combining a narrowing of gender LFP gaps with a simultaneous narrowing of the gaps in working hours can be expected to further stimulate economic growth. Two additional scenarios are considered here:
Scenario E: gender LFP and working hour gaps reduced by 25% by 2035 and by 50% in 2050. This scenario follows the approaches of Scenario A and Scenario C, so that the gender employment and working hour gaps fall by 25% by 2035, and 50% by 2050 relative to the baseline.
Scenario F: gender LFP and working hour gaps reduced by 50% by 2035 and by 100% in 2050. This scenario follows the approaches of Scenario B and Scenario D, so that the gender employment and working hour gaps fall by 50% by 2035, and 100% (i.e. are fully closed) by 2050 relative to the baseline.
Across the OECD, annual potential GDP per capita would grow an additional 0.17 percentage points (Scenario E) or 0.34 percentage points (Scenario F), respectively (see Figure 7.8 and Annex Table 7.A.4). While overall somewhat smaller than in the Nordics and Germany, where the effects are strongly driven by the closing of the working hours gap, Estonia could see the largest additional growth in potential GDP per capita among the Baltic states. Closing half of the gender LFP and working hours gap by 2050 would add 0.07 percentage points of additional annual growth relative to the baseline projections, and closing both altogether over this period would add additional 0.14 percentage points of annual GDP per capita growth. This would increase potential GDP overall by 2.1% (Scenario E) or 4.3% (Scenario F) in 2050.
Figure 7.8. Closing LFP and working hours gaps simultaneously would boost GDP strongly
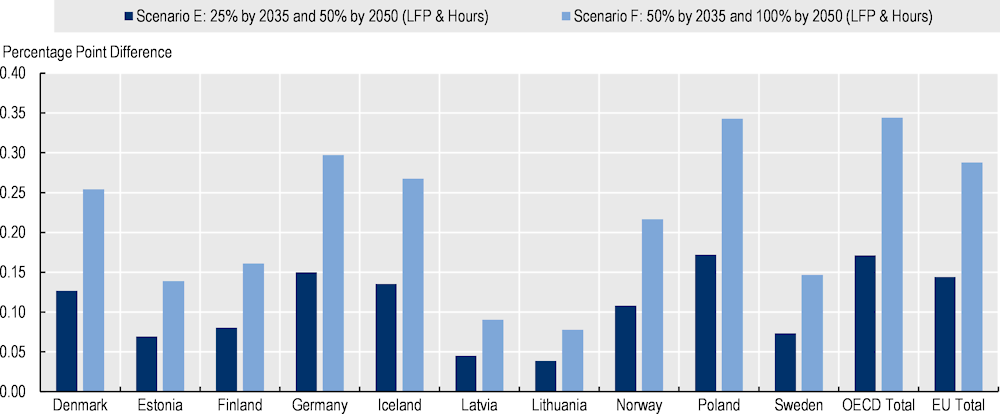
Note: See notes for Figure 7.5.
Source: OECD estimates based on OECD population data, https://stats.oecd.org/index.aspx?DataSetCode=POP_PROJ, the OECD Employment Database, http://www.oecd.org/employment/emp/onlineoecdemploymentdatabase.htmv, and Employment Projections; and the OECD Long-Term Growth Model, https://doi.org/10.1787/b4f4e03e-en.
7.3.2. Zooming in on Estonia: Unpaid work, educational sorting and life expectancy
This section considers more detailed scenarios in order to illustrate the dynamics that could lead to more gender equality in the Estonian labour market. Rather than an international comparison, these scenarios zoom in on Estonia in isolation to reflect the country-specific context that can differ markedly from other OECD countries (e.g. concerning the gender LFP gap among older workers). In contrast to the basic scenarios above, where gender LFP- and working hours gaps converge over time to a certain level, the detailed scenarios generally model a one‑off permanent change in labour force participation, working hours, life expectancy or productivity at different ages that transmit through the population as it ages.
Below, three different scenarios are considered: more equal sorting of boys and girls into different fields of study; less sexist prejudice on the labour market and less gender stereotyping within households and families; and, a convergence of (healthy) life expectancy for older populations. Details on the exact mechanisms of the scenarios are given in each of the respective sections.
The scenarios presented here are stylised and only serve as illustrative examples of what could happen if action regarding the issues were undertaken. The model dynamics and parameters chosen may not fully reflect “real-world practice”, but nonetheless reflect real issues as identified in the previous chapters. Again, the scenarios illustrate the potential gains the Estonian labour market and economy may reap, when closing gender gaps in labour force participation.
Educational sorting: More women into STEM fields
A substantial part of the gender differences in labour market outcomes can be linked to differential labour market sorting between men and women (Card, Cardoso and Kline, 2016[15]; Masso, Meriküll and Vahter, 2020[16]). Differential enrolment across educational fields and tracks is often one of the drivers behind differences in sorting across occupations and industries. For example, women often tend to enrol more in educational fields with lower earnings potential and to a larger extent sort into occupations with lower earnings (Sloane, Hurst and Black, 2020[17]; Couppié, Dupray and Moullet, 2014[18]).
Gendered segregation is particularly strong in STEM programmes (Science, Technology, Engineering and Mathematics) and may have a dampening effect on the overall productivity of women workers in the economy. Like in many other countries, Estonian boys and young men are more likely to be enrolled in, and graduate from, STEM programmes, while Estonian girls and young women graduate more from EHW programmes (Education, Health and Welfare). In 2018, for example, 47% of male graduates were previously enrolled in STEM programmes, while it was only 21% of female graduates. At the same time, 9% of male and 26% of female graduates were previously enrolled in EHW fields (OECD, 2019[19]). As wages are generally higher and differences between male and female wages lower, a persistent underrepresentation of women and girls in STEM tracks prevents the closing of the gender wage gap. At the same time, a shortage of workers in STEM sectors could mean that women’s productive potential may not be fully exploited, potentially reducing overall economic output (Breda et al., 2021[20]).
Less gender stereotyping in education and life‑long learning, for example by increasing students’ exposure to female role models, could help increase the share of women in STEM fields, and improve women’s access to more productive jobs (see e.g. Breda et al. (2021[20])). As a result, women’s average economic productivity should increase, along with their wages and the aggregate economic output. A better allocation of women’s talent across occupations is, for example, an important factor for economic growth in the United States (Hsieh et al., 2019[21]).
The educational sorting scenario thus assumes a change of the gender gap in enrolment and graduation rates, which leads to a closing of the gender productivity gap among typical graduates (age 15‑24 year‑olds), approximated by the wage gap between men and women at different ages (more details in Annex 7.B). This reduces the productivity gap between men and women over time and increases overall productivity in Estonia. At the same time, the labour force participation and working hours of both men and women follow the baseline projections of the previous section.
Table 7.1 shows the effects this scenario could have on the Estonian economy. If enrolment and graduation rates are more equal among men and women, and the gap in productivity of the jobs held by men and women graduating subsequently closes from 2020 onwards, the annual potential GDP per capita growth is projected to increase by 0.30 percentage points until 2050. This would increase Estonian potential GDP per capita by about 9.63% in 2050.
Unpaid work and leave‑taking: Weakening traditional gender norms in families
Persistent gender norms and a traditional division of paid and unpaid work can have substantial effects on women’s opportunities in the labour market. For example, the career interruptions related to taking maternity and/or parental leave limit the labour supply and productivity of female employees with young children. As a result, mothers career paths are negatively affected in the medium to longer term (see e.g. Kleven et. al (2019[22]; 2019[23]), and for Estonia, Masso et. al (2020[16])). At the same time, women, and in particular mothers, take on a majority of the childcare work over the years following childbirth, along with a majority of other unpaid housework (OECD, 2021[3]; 2017[24]).
Table 7.1. Growth scenarios for Estonia
Estimated difference in economic growth relative to the baseline, different gender gap scenarios
Scenario |
Detail |
Age |
Difference in projected potential GDP, 2050, % |
Difference in the average annual rate of growth in potential GDP, 2020‑50, percentage points |
Difference in projected potential GDP per capita, 2050, % |
Difference in the average annual rate of growth in potential GDP per capita, 2020‑50, percentage points |
---|---|---|---|---|---|---|
Educational sorting |
Productivity gap closure (100%) |
15‑24 |
9.63 |
0.30 |
9.63 |
0.30 |
Unpaid work and leave‑taking |
LFP & HRS gap closure (100%) + productivity gap closure (50%) |
25‑49 |
10.30 |
0.33 |
10.30 |
0.33 |
Life expectancy |
LFP gap closure (100%) + life expectancy gap closure (100%) |
50+ |
0.96 |
0.03 |
‑3.10 |
‑0.10 |
Female labour force participation only |
Convergence to Iceland’s female LFP |
15‑74 |
5.97 |
0.19 |
5.97 |
0.19 |
Note: The first two scenarios assume no changes in the population development, whereas the male population increase in the last scenario. Therefore, potential GDP and potential GDP per capita are similar in the first two scenarios and differ in the last scenario (as the population base increases). See Annex 7.B for a description of the method and data used. LFP: Labour force participation rate; HRS: Working hours.
Source: OECD estimates based on OECD population data, https://stats.oecd.org/index.aspx?DataSetCode=POP_PROJ and Eurostat Population Projections, https://ec.europa.eu/eurostat/web/population-demography/population-projections/database, the OECD Employment Database, http://www.oecd.org/employment/emp/onlineoecdemploymentdatabase.htm and Employment Projections,; the OECD Long-Term Growth Model, https://doi.org/10.1787/b4f4e03e-en, and the Eurostat Structure of Earnings Survey, https://ec.europa.eu/eurostat/cache/metadata/en/earn_ses2014_esms.htm.
The norms around unpaid work and parental leave‑taking in Estonian families have remained rigid. For example, when it comes to taking leave to care for very young children, Estonian mothers are still about 11 times more likely to use publicly administered parental leave than fathers (OECD Family Database). Estonian women also spend 55% more of their time on unpaid activities in and around the household than men (OECD Time‑Use Database). These patterns limit the time Estonian women can spend in paid work and inhibits their possibilities to advance in the labour market, with negative implications for pay, particularly in jobs with inflexible hours, as well as for their human capital accumulation and productivity (Goldin, 2014[25]).
A consequence of the unequal division of unpaid work is that women can face additional effects that limit their access to better paid and more productive jobs. Employers may engage in bias and discriminatory behaviour towards women, regarding hiring and career advancement, as they may assume that women will be less committed to their job due to their greater share of unpaid work (Ciminelli, Schwellnus and Stadler, 2021[26]). More productive firms may discriminate more against women at the hiring stage than less productive firms, as the chance that a female job seeker exceeds the necessary productivity level is lower the higher the firms own productivity is.
Women’s greater share of unpaid work also makes their labour market more monopsonist than for men (e.g. women may be more likely to exclude workplaces that involve a long commute from their job search/exit options (Le Barbanchon, Rathelot and Roulet, 2020[27])). This pattern implies that employers have a stronger wage‑setting power over women than over men, regardless of their prejudice/stereotype. In other words, due to their greater share of unpaid work, women cannot bargain their wages as assertively as men do, meaning that they are more likely to be paid below their productivity potential.
More equal gender norms provide a boost to women’s productivity as they reduce the factors limiting labour force participation and access to more productive jobs, see e.g. Farré and González (2019[28]) and Druedahl, Ejrnæs and Jørgensen (2019[29]). Outsourcing unpaid work to household service providers will increase employment in this sector, which is predominantly female, as well as increase the labour supply of women previously engaged in unpaid work at home (OECD, 2021[3]).
In practice, the gender norms scenario assumes an equal sharing of unpaid work in the household and an equal sharing of parental leave‑taking. This is assumed to lead to a closing of the baseline LFP and working hours gap for men and women aged between 25 and 39. At the same time, this scenario assumes productivity increases among women, in a way that it closes the gender productivity gap by 50% over the same age group. As shown in Table 7.1, more equal sharing of unpaid work and leave‑related career breaks could increase annual potential GDP per capita growth by 0.33 percentage points until 2050. This would raise the Estonian potential GDP per capita in 2050 by approximately 10.30%.
Life expectancy: Longer lives for men
Health problems can significantly impair the quality of life, but they also may reduce the ability to work and engage on the labour market. Such health problems become more prevalent as individuals age and the health status of the workers thus becomes an important determinant of labour market attachment at older ages (OECD, 2019[30]). In Estonia, the life expectancy, as well as the expectancy of disability-free lives, are noticeably different for men and women (see also Chapter 2). New-born males are expected to live for about 53 years without activity-imitating disabilities, while newborn females can do so for 56 years (Statistics Estonia, 2019[31]). In the middle of their labour market career at age 40, women are also expected to live for roughly 44 additional years, which is about 8 years longer than for Estonian men aged 40 (OECD Health Database). For men, these figures are particularly low relative to other OECD countries and can have important implications for their late and extended work lives.
In sharp contrast to other OECD countries, the gender employment gap in Estonia reverses among older workers (55‑74). For example, while the probability of labour force participation for women aged 60 to 64 is almost 18 percentage points lower than that of their male counterparts OECD-wide in the labour-force projection model for 2020, Estonian women of this age are nearly 7 percentage points more likely than men to be employed. This latter result is consistent with the significantly lower number of healthy life years of Estonian men.
Fostering healthier lives, particularly for men, could significantly increase the quality of life as well as the ability to engage in paid employment towards the end of their life. As such, it may not only increase life satisfaction, but also the aggregate LFP rate of men and overall economic output in Estonia. The life expectancy scenario assumes that the life expectancy gap for men and women aged 50+ closes, particularly by lowering men’s probability of dying at each age to women’s probability of dying at the same age. This should come with an increase of the healthy life years of men and their possibility to engage in paid employment so that the gender LFP gap for men and women aged 50+ closes in full, while the working hours gap is unaffected and follows the baseline scenario. An increase in life expectancy increases total economic output but also increases the elderly population. As a result, the effect on GDP per capita is ambiguous, as it depends on the relative size of the effects on the population size and economic output.
Table 7.1 shows that increased life expectancy indeed has a twofold effect. While the longer lives of men increase the total population base, it does so by predominately increasing the population among ages with comparatively low LFP rates. As a result, the increase of the population weighs stronger than the additional economic output, thus reducing potential GDP per capita in 2050 by 3.10%. However, on an aggregate basis, the total potential economic output in 2050 is increased by 0.96% through more old-age labour force participation. One of the reasons for the relatively weak effect of closing the LFP gap among older workers is that the OECD baseline projections already assume a convergence of labour force participation among older ages. Among the 55‑69 year‑olds, for example, the gender gaps in labour force participation practically close already in 2023, based on the recent labour market entry and exit rates and demographic developments that define the baseline projections.
Increasing female employment only: Reaching Iceland’s labour force participation
The previous scenarios have all assumed that more gender equality on the labour market and at home closes labour market gaps between men and women, by assuming that the lowest of labour force participation rates for men and women in each age group converge to the highest LFP in the same age group. As such, wherever female LFP is lower than the male LFP in a specific age group, it cannot increase above male levels even though more gender equality could potentially spur even larger gains in the engagement on the labour market. At the same time, even though more gender equality could further increase labour force participation of older women, the projections only increase male LFP for those aged 55 and above and leave female LFP at older ages entirely unaffected.
For this reason, the last scenario presented in this chapter considers an increase in female LFP rates in isolation, without being bound by or affecting male LFP at all. Here, Iceland serves as the benchmark for women’s engagement on the labour market. In Iceland, female LFP rates for those aged 15‑74 are by far the highest in the OECD. At the same time, while women’s usual working hours and GDP per capita differ somewhat from Estonia, they do so to a lesser extent than other countries with notably higher female LFP than Estonia. As such, the labour force participation of women across all age groups is allowed to increase to the levels of Iceland, without being bound by male LFP.
Table 7.1 shows that such a scenario would boost GDP and GDP per capita by about 6% in 2060 relative to the baseline scenario that does not assume any divergence from the LFP projections. This corresponds to an additional 0.19 percentage points of average annual growth between 2020 and 2050. This is more than twice as large than a closure of gender labour force participation gaps alone and is driven by particularly strong increases in LFP for younger women (aged 15‑39) and those aged 60 to 69. While the former is mainly driven by the overall high rates if female LFP at the commencement of labour market careers and throughout prime childbearing ages, the latter is increasing particularly because changes in female LFP rates are not bound by the low labour market engagement of older males in Estonia.
References
[33] Azevedo, J. et al. (2013), “Is Labor Income Responsible for Poverty Reduction? A Decomposition Approach”, World Bank Policy Research Working Papers, No. 6414, https://doi.org/10.1596/1813-9450-6414.
[34] Bargain, O., K. Orsini and A. Peichl (2014), “Comparing Labor Supply Elasticities in Europe and the United States”, Journal of Human Resources, Vol. 49/3, pp. 723-838, https://doi.org/10.3368/jhr.49.3.723.
[20] Breda, T. et al. (2021), “Do Female Role Models Reduce the Gender Gap in Science? Evidence from French High Schools”, Working Paper, https://drive.google.com/file/d/1-J8RTPdDG_kHW_KY3JpSbOd6kU9Ie6fh/view.
[15] Card, D., A. Cardoso and P. Kline (2016), “Bargaining, Sorting, and the Gender Wage Gap: Quantifying the Impact of Firms on the Relative Pay of Women”, The Quarterly Journal of Economics, Vol. 131/2, pp. 633-686, https://doi.org/10.1093/qje/qjv038.
[26] Ciminelli, G., C. Schwellnus and B. Stadler (2021), “Sticky floors or glass ceilings? The role of human capital, working time flexibility and discrimination in the gender wage gap”, OECD Economics Department Working Papers, Organisation for Economic Co-Operation and Development (OECD), https://doi.org/10.1787/02ef3235-en.
[18] Couppié, T., A. Dupray and S. Moullet (2014), “Education-based occupational segregation and the gender wage gap: evidence from France”, International Journal of Manpower, Vol. 35/3, pp. 368-391, https://doi.org/10.1108/ijm-09-2012-0143.
[29] Druedahl, J., M. Ejrnæs and T. Jørgensen (2019), “Earmarked paternity leave and the relative income within couples”, Economics Letters, Vol. 180, pp. 85-88, https://doi.org/10.1016/j.econlet.2019.04.018.
[28] Farré, L. and L. González (2019), “Does paternity leave reduce fertility?”, Journal of Public Economics, Vol. 172, pp. 52-66, https://doi.org/10.1016/j.jpubeco.2018.12.002.
[10] Geppert, C. et al. (2019), “Labour supply of older people in advanced economies: the impact of changes to statutory retirement ages”, OECD Economics Department Working Papers, No. 1554, OECD Publishing, Paris, https://doi.org/10.1787/b9f8d292-en.
[25] Goldin, C. (2014), “A Grand Gender Convergence: Its Last Chapter”, American Economic Review, Vol. 104/4, p. 1091, https://doi.org/10.1257/aer.104.4.1091.
[8] Guillemette, Y. and D. Turner (2021), “The long game: Fiscal outlooks to 2060 underline need for structural reform”, OECD Economic Policy Papers, No. 29, OECD Publishing, Paris, https://doi.org/10.1787/a112307e-en.
[7] Guillemette, Y. and D. Turner (2018), The Long View: Scenarios for the World Economy to 2060, https://doi.org/10.1787/2226583X.
[21] Hsieh, C. et al. (2019), “The Allocation of Talent and U.S. Economic Growth”, Econometrica, Vol. 87/5, pp. 1439-1474, https://doi.org/10.3982/ecta11427.
[11] ILO, IMF, OECD and World Bank Group (2014), Achieving stronger growth by promoting a more gender-balanced economy, https://www.oecd.org/g20/topics/employment-and-social-policy/ILO-IMF-OECD-WBG-Achieving-stronger-growth-by-promoting-a-more-gender-balanced-economy-G20.pdf.
[23] Kleven, H. et al. (2019), “Child Penalties across Countries: Evidence and Explanations”, AEA Papers and Proceedings, Vol. 109, pp. 122-126, https://doi.org/10.1257/pandp.20191078.
[22] Kleven, H., C. Landais and J. Søgaard (2019), “Children and Gender Inequality: Evidence from Denmark”, American Economic Journal: Applied Economics, Vol. 11/4, pp. 181-209, https://doi.org/10.1257/app.20180010.
[27] Le Barbanchon, T., R. Rathelot and A. Roulet (2020), “Gender Differences in Job Search: Trading off commute against wage”, The Quarterly Journal of Economics, Vol. 136/1, pp. 381-426, https://doi.org/10.1093/qje/qjaa033.
[12] Manakov, A., P. Suvorkov and S. Stanaitis (2017), “Economic Development of the Baltic and Nordic Countries: Characteristics of Еconomic Models”, Baltic Region, Vol. 9/1, pp. 41-54, https://doi.org/10.5922/2079-8555-2017-1-5.
[16] Masso, J., J. Meriküll and P. Vahter (2020), “The Role of Firms in the Gender Wage Gap”, SSRN 3532955, https://papers.ssrn.com/sol3/papers.cfm?abstract_id=3532955.
[3] OECD (2021), Bringing Household Services Out of the Shadows: Formalising Non-Care Work in and around the House, OECD Publishing, Paris, https://doi.org/10.1787/fbea8f6e-en.
[4] OECD (2021), “National Accounts at a Glance”, OECD National Accounts Statistics (database), https://doi.org/10.1787/data-00369-en (accessed on 8 October 2021).
[14] OECD (2021), Part-time employment rate (indicator), https://doi.org/10.1787/f2ad596c-en (accessed on 30 July 2021).
[9] OECD (2020), Promoting an Age-Inclusive Workforce: Living, Learning and Earning Longer, OECD Publishing, Paris, https://doi.org/10.1787/59752153-en.
[19] OECD (2019), Education at a Glance 2019: OECD Indicators, OECD Publishing, Paris, https://doi.org/10.1787/f8d7880d-en.
[30] OECD (2019), Working Better with Age, Ageing and Employment Policies, OECD Publishing, Paris, https://doi.org/10.1787/c4d4f66a-en.
[1] OECD (2018), Is the Last Mile the Longest? Economic Gains from Gender Equality in Nordic Countries, OECD Publishing, Paris, https://doi.org/10.1787/9789264300040-en.
[24] OECD (2017), The Pursuit of Gender Equality: An Uphill Battle, OECD Publishing, Paris, https://doi.org/10.1787/9789264281318-en.
[6] Raleigh, V. (2019), “Trends in life expectancy in EU and other OECD countries : Why are improvements slowing?”, OECD Health Working Papers, No. 108, OECD Publishing, Paris, https://doi.org/10.1787/223159ab-en.
[13] Riekhoff, A., O. Krutova and J. Nätti (2019), “Working-hour Trends in the Nordic Countries: Convergence or Divergence?”, Nordic Journal of Working Life Studies, https://doi.org/10.18291/njwls.v9i3.116056.
[32] Shorrocks, A. (2013), “Decomposition procedures for distributional analysis: a unified framework based on the Shapley value”, The Journal of Economic Inequality, Vol. 11/1, pp. 99-126, https://doi.org/10.1007/s10888-011-9214-z.
[17] Sloane, C., E. Hurst and D. Black (2020), “A Cross-Cohort Analysis of Human Capital Specialization and the College Gender Wage Gap”, University of Chicago, Becker Friedman Institute for Economics Working Paper, Vol. 2019/121, https://bfi.uchicago.edu/working-paper/a-cross-cohort-analysis-of-human-capital-specialization-and-the-college-gender-wage-gap/.
[5] Solow, R. (1956), “A Contribution to the Theory of Economic Growth”, The Quarterly Journal of Economics, Vol. 70/1, p. 65, https://doi.org/10.2307/1884513.
[31] Statistics Estonia (2019), Living longer, but health is worse, https://www.stat.ee/en/uudised/news-release-2019-105.
[2] Ward, A., M. Zinni and P. Marianna (2018), “International productivity gaps: Are labour input measures comparable?”, OECD Statistics Working Papers, No. 2018/12, OECD Publishing, Paris, https://doi.org/10.1787/5b43c728-en.
Annex 7.A. Additional tables and figures
Annex Table 7.A.1. Decomposition of the drivers of GDP per capita growth (2000‑19)
|
GDP per capita growth, avg. annual rate (%) |
Percentage point contribution of main components |
|||||
---|---|---|---|---|---|---|---|
Labour productivity |
Working age share of population |
Male employment |
Female employment |
Male working hours |
Female working hours |
||
Denmark |
0.87 |
1.22 |
‑0.21 |
‑0.02 |
0.08 |
‑0.22 |
0.01 |
Estonia |
3.93 |
3.67 |
‑0.30 |
0.57 |
0.40 |
‑0.16 |
‑0.26 |
Finland |
1.05 |
0.97 |
‑0.40 |
0.32 |
0.40 |
‑0.21 |
‑0.03 |
Germany |
1.14 |
0.90 |
‑0.25 |
0.22 |
0.47 |
‑0.29 |
0.09 |
Iceland |
1.67 |
2.53 |
‑0.01 |
‑0.06 |
‑0.11 |
‑0.61 |
‑0.07 |
Latvia |
4.72 |
4.02 |
‑0.41 |
0.60 |
0.72 |
‑0.20 |
‑0.02 |
Lithuania |
5.32 |
4.08 |
‑0.11 |
0.63 |
0.61 |
0.05 |
0.06 |
Norway |
0.64 |
0.77 |
0.08 |
‑0.06 |
‑0.01 |
‑0.20 |
0.05 |
Poland |
3.77 |
3.35 |
‑0.50 |
0.58 |
0.45 |
‑0.09 |
‑0.01 |
Sweden |
1.39 |
1.36 |
‑0.07 |
0.07 |
0.05 |
‑0.06 |
0.04 |
OECD Total |
1.20 |
1.20 |
‑0.07 |
0.08 |
0.28 |
‑0.32 |
0.03 |
EU Total |
1.14 |
1.03 |
‑0.25 |
0.16 |
0.44 |
‑0.34 |
0.09 |
Note: Estimates based on the decomposition of national accounts data using labour force survey estimates. The OECD and EU totals only include countries for which the necessary data for the growth accounting exercise is fully available between 2000 and 2019. The OECD total here does not include Australia, Chile, Colombia, Costa Rica, the Czech Republic, Japan, Korea, Luxembourg, Mexico, New Zealand, and Türkiye. The EU total does not included Luxembourg and the Czech Republic. See Annex 7.B for more detail.
Source: OECD estimates based on data from the OECD National Accounts Database http://www.oecd.org/std/na/ and the OECD Employment Database, http://www.oecd.org/employment/emp/onlineoecdemploymentdatabase.htm.
Annex Table 7.A.2. Decomposition of the employment component
|
Decomposition of the contribution of the employment-to-population rate (percentage points) |
|||||
---|---|---|---|---|---|---|
Men |
Women |
|||||
Employment |
Share of the whole working age population |
Employment rate |
Employment |
Share of the whole working age population |
Employment rate |
|
Denmark |
‑0.02 |
0.00 |
‑0.02 |
0.08 |
0.00 |
0.08 |
Estonia |
0.57 |
0.10 |
0.47 |
0.40 |
‑0.09 |
0.49 |
Finland |
0.32 |
0.02 |
0.31 |
0.40 |
‑0.02 |
0.42 |
Germany |
0.22 |
0.01 |
0.21 |
0.47 |
‑0.01 |
0.48 |
Iceland |
‑0.06 |
0.07 |
‑0.13 |
‑0.11 |
‑0.06 |
‑0.05 |
Latvia |
0.60 |
0.05 |
0.55 |
0.72 |
‑0.05 |
0.77 |
Lithuania |
0.63 |
0.06 |
0.57 |
0.61 |
‑0.06 |
0.67 |
Norway |
‑0.06 |
0.03 |
‑0.09 |
‑0.01 |
‑0.03 |
0.02 |
Poland |
0.58 |
0.04 |
0.54 |
0.45 |
‑0.03 |
0.48 |
Sweden |
0.07 |
0.02 |
0.05 |
0.05 |
‑0.02 |
0.07 |
OECD Total |
0.08 |
0.01 |
0.07 |
0.28 |
‑0.01 |
0.28 |
EU Total |
0.16 |
0.00 |
0.15 |
0.44 |
0.00 |
0.45 |
Note: See notes for Annex Table 7.A.1. See Annex 7.B for more detail.
Source: OECD estimates based on data from the OECD National Accounts Database http://www.oecd.org/std/na/ and the OECD Employment Database, http://www.oecd.org/employment/emp/onlineoecdemploymentdatabase.htm.
Annex Table 7.A.3. Decomposition of the working hours component
|
Decomposition of the contribution of average working hours (percentage points) |
|||||
---|---|---|---|---|---|---|
Men |
Women |
|||||
Working hours |
Share of all employed |
Hours worked per employed |
Working hours |
Share of all employed |
Hours worked per employed |
|
Denmark |
‑0.22 |
‑0.06 |
‑0.16 |
0.01 |
0.05 |
‑0.04 |
Estonia |
‑0.16 |
0.07 |
‑0.23 |
‑0.26 |
‑0.07 |
‑0.19 |
Finland |
‑0.21 |
‑0.06 |
‑0.15 |
‑0.03 |
0.05 |
‑0.08 |
Germany |
‑0.29 |
‑0.17 |
‑0.12 |
0.09 |
0.13 |
‑0.04 |
Iceland |
‑0.61 |
0.03 |
‑0.64 |
‑0.07 |
‑0.02 |
‑0.04 |
Latvia |
‑0.20 |
‑0.07 |
‑0.13 |
‑0.02 |
0.06 |
‑0.08 |
Lithuania |
0.05 |
0.02 |
0.03 |
0.06 |
‑0.02 |
0.08 |
Norway |
‑0.20 |
‑0.02 |
‑0.18 |
0.05 |
0.02 |
0.03 |
Poland |
‑0.09 |
0.01 |
‑0.11 |
‑0.01 |
‑0.01 |
0.00 |
Sweden |
‑0.06 |
0.00 |
‑0.06 |
0.04 |
0.00 |
0.05 |
OECD Total |
‑0.32 |
‑0.13 |
‑0.19 |
0.03 |
0.11 |
‑0.08 |
EU Total |
‑0.34 |
‑0.19 |
‑0.15 |
0.09 |
0.16 |
‑0.07 |
Note: See notes for Annex Table 7.A.1. See Annex 7.B for more detail.
Source: OECD estimates based on data from the OECD National Accounts Database http://www.oecd.org/std/na/ and the OECD Employment Database, http://www.oecd.org/employment/emp/onlineoecdemploymentdatabase.htm.
Annex Table 7.A.4. Further gains in growth from closing gender gaps in labour force participation
|
Difference in the average annual rate of growth in potential GDP per capita, 2020‑50, scenario vs. baseline, percentage points |
|||||
---|---|---|---|---|---|---|
Scenario: |
A |
B |
C |
D |
E |
F |
LFP |
25x35, 50x50 |
50x35, 100x50 |
Baseline |
Baseline |
25x35, 50x50 |
50x35, 100x50 |
Hours |
Baseline |
Baseline |
25x35, 50x50 |
50x35, 100x50 |
25x35, 50x50 |
50x35, 100x50 |
Denmark |
0.06 |
0.12 |
0.06 |
0.12 |
0.13 |
0.25 |
Estonia |
0.04 |
0.07 |
0.03 |
0.06 |
0.07 |
0.14 |
Finland |
0.03 |
0.06 |
0.05 |
0.10 |
0.08 |
0.16 |
Germany |
0.03 |
0.06 |
0.12 |
0.23 |
0.15 |
0.30 |
Iceland |
0.05 |
0.10 |
0.08 |
0.16 |
0.14 |
0.27 |
Latvia |
0.03 |
0.05 |
0.02 |
0.04 |
0.05 |
0.09 |
Lithuania |
0.02 |
0.05 |
0.02 |
0.03 |
0.04 |
0.08 |
Norway |
0.04 |
0.09 |
0.06 |
0.12 |
0.11 |
0.22 |
Poland |
0.13 |
0.26 |
0.04 |
0.07 |
0.17 |
0.34 |
Sweden |
0.03 |
0.06 |
0.04 |
0.09 |
0.07 |
0.15 |
OECD Total |
0.09 |
0.19 |
0.07 |
0.14 |
0.17 |
0.34 |
EU Total |
0.06 |
0.12 |
0.08 |
0.16 |
0.14 |
0.29 |
Note: See Annex 7.B for a description of the method and data used. The OECD and EU totals are the weighted total across all OECD and EU member countries For the OECD total, data for Türkiye is missing. LFP: Labour force participation; HRS: Working hours.
Source: OECD estimates based on OECD population data, https://stats.oecd.org/index.aspx?DataSetCode=POP_PROJ, the OECD Employment Database and Employment Projections, http://www.oecd.org/employment/emp/onlineoecdemploymentdatabase.htm; and the OECD Long-Term Growth Model, https://doi.org/10.1787/b4f4e03e-en.
Annex 7.B. Methodology
This annex provides detail on the methods and data used in this chapter. It covers the theoretical background as well as the data and estimation procedure for both the growth accounting exercise and the growth projection scenarios.
Growth accounting
The first analysis is based on growth accounting – a procedure used to identify and decompose the sources of economic growth. Using macroeconomic time‑series, the basic principle of growth accounting is to split economic growth into its main components parts under standard macroeconomic theory: labour, capital, and total factor productivity (Solow, 1956[5]). Data permitting, the contribution of each of these main components can then be further decomposed into sub-components, as required.
The starting point for the growth accounting exercise is a standard Cobb-Douglas production function with constant returns to scale. Total economic output, measured by Gross Domestic Product (GDP), can be expressed using the following multiplicative function:
where Y is GDP, K is physical capital, A is technological progress, Q is human capital per person employed, E is headcount employment, H is total hours worked (and therefore, H/E hours worked per person employed), and α is the labour share of output.
After some manipulations, this can be re‑written as:
and output per person, measured by GDP per capita, can be expressed as:
where P stands for the population and WP for the working-age population, Y/P is GDP per capita, K/Y is the capital-to‑output ratio, WP/P is the working-age share of the population, and E/WP is the employment-to-working-age‑population ratio or employment rate. In this particular model, employment is limited to those of working-age (15‑64 year‑olds) only. This is to allow for better modelling of how shifts in the working-age share of the population contribute to output.
GDP per capita can be considered as the product of labour utilisation (covering the working-age share of the population, the employment-to-working-age‑population ratio, and hours worked per person employed), and labour productivity or output per hour worked, covering all other factors (physical capital, technological progress, and human capital):
where:
Labour utilisation itself can be split into components. The focus here is on disaggregating the employment rate (E/WP) and hours worked per person employed (H/E) by gender and, where possible, age group, as expressed by the following two additive functions:
and
Lastly, the model shown in equation 4 can be re‑written in growth rates to give changes in economic output over time, as expressed by the following additive function:
where the g is the growth rate for the given factor, and with growth rates for the employment rate (g[E/WP]) and hours worked per person employed (g[H/E]) themselves calculated as
and
It is equations 8‑10 that form the core of the growth accounting exercise.
Estimation itself is conducted using the “Shapley decomposition” (Shorrocks, 2013[32]) – a procedure initially used in the inequalities literature to identify the contribution of different income sources to income inequality, but more recently it has also been applied elsewhere as, for instance, the decomposition of the drivers of poverty reduction (Azevedo et al., 2013[33]). Shapley decomposition runs through all possible sequences (effectively, combinations) of a given function to isolate the contribution of a given component – in this case, the contributions of growth in labour productivity (Y/(E.H)), growth in the working-age share of the population (WP/P), growth in the employment rate (E/WP), and growth in average hours worked per person employed (H.E). It has the advantage of producing additive estimates (so that, in this case, growth in GDP per capita is estimated as the sum of growth in the various components) and, at least when the number of components is fairly low, is also simple to calculate. A detailed overview of the Shapley decomposition method itself can be found in Shorrocks (2013[32]).
The estimates are produced in two stages. First, growth in GDP per capita is decomposed into each of its main components – as shown in equation 8 above, growth in labour productivity (Y/(E.H)), in the working-age share of the population (WP/P), in the employment rate (E/WP), and in average hours worked per person employed (H.E). Second, the contributions of growth in both the employment-to-population rate (E/WP) and average hours worked per person employed (H.E) are then themselves decomposed by gender and, where possible, by age group too, as shown in equations 9 and 10. It is these last two decompositions (of the employment rate and average working hours) that are the focus here as they provide information on the extent to which changes in men’s and women’s employment rates and working hours have contributed to economic growth. This procedure is run separately for each country.
The data used for the growth accounting exercise are based on a combination of official macroeconomic data from national accounts databases and employment and working hours estimates from labour force surveys. Data for the first stage are taken from the OECD National Accounts Database. The data series used include Gross Domestic Product (GDP), total population, total employment, and average working hours per person employed (see Annex Table 7.B.1 for a summary). On occasion, important series are missing in the OECD database. Where this is the case, values are imputed or interpolated using information from alternative national accounts databases, such as those published by national statistical offices.
Annex Table 7.B.1. Summary of data series used in growth accounting
Series |
Details |
Main source |
Additional sources |
---|---|---|---|
GDP (expenditure approach) |
Constant prices, constant PPPs, OECD base year (USD 2015) |
OECD National Accounts Database |
|
Total population |
National concept, 1000s |
OECD National Accounts Database |
|
Total employment |
Domestic concept, 1000s |
OECD National Accounts Database |
OECD Employment Database |
Hours worked for total employment |
Domestic concept, hours, millions |
OECD National Accounts Database |
OECD Employment Database |
Population by age and gender |
1000s |
OECD Employment Database |
|
Employment by age and gender |
1000s |
OECD Employment Database |
|
Average usual weekly working hours by age and gender |
Total employment, total declared employment |
OECD Employment Database |
Statistics Finland, Statistics Sweden |
The second stage of the growth accounting exercise requires data on population, employment, and working hours that are disaggregated by age and gender. Because national accounts databases do not typically disaggregate information by age or gender, these data are estimated using information from labour force surveys – in short, the overall national accounts series on population, employment and hours are split and “allocated” across the various gender- and age groups according to the distribution of the given series provided by labour force survey data. The labour force survey estimates for employment and working hours are re‑scaled before this “allocation” so that the aggregated estimates for the working-age population match exactly those from the national accounts series.
The labour force survey data used for this second stage are taken primarily from the OECD Employment Database. Where data are missing, values are imputed using information from alternative sources such as national statistical offices or are estimated by trending the nearest observation back or forward using alternative but similar series (see Annex Table 7.B.2). For a lack of sufficiently comparable data, the OECD average and totals presented in the results figures and tables do not include Australia, Chile, Colombia, Costa Rica, the Czech Republic, Japan, Korea, Luxembourg, Mexico, New Zealand and Türkiye; while the EU average and total does not include the Czech Republic, Luxembourg and EU-countries that do not belong to the OECD.
Annex Table 7.B.2. Country-specific notes for the data series used in the growth accounting
Series |
Country |
Details |
---|---|---|
Total employment |
Iceland |
Data missing in the OECD National Accounts Database for years before 2008. Data for years before 2008 are estimated by trending backwards using alternative data from the Labour Force Survey (total labour force). |
Hours worked for total employment |
Iceland |
Data missing in the OECD National Accounts Database for years before 2008. Data for years before 2008 are estimated by trending backwards using alternative data from the Labour Force Survey (average annual hours worked per worker/year and total employment). |
Average usual weekly working hours by age and gender |
Finland |
Data missing in the OECD Employment Database for years before 2001. Data for years before 2001 are estimated by trending backwards using alternative data on working hours (average annual hours actually worked) provided by Statistics Finland. |
Sweden |
Data missing in the OECD Employment Database for years before 2002. Data for years before 2002 are estimated by trending backwards using alternative data on average usual weekly working hours provided by Statistics Sweden. |
Forward-looking growth projections
The economic growth scenarios are based on the OECD Long-Term Model, which projects economic outcomes until 2060, following a range of projections on productivity, employment and population development (Guillemette and Turner, 2018[7]; 2021[8]). Production of these estimates themselves takes place in two stages. First, estimates of the size of the labour force and overall average working hours are produced under different scenarios by applying the assumed labour participation (LFP) rates and working hours to the OECD’s in-house labour force projection model. For the various hypothetical “gender gap” scenarios, participation rates and, where needed, working hours for the relevant gender and age groups are adjusted so that they meet the given assumed gender gap targets by the given target year.
Second, estimates of potential GDP per capita and annual growth of the potential GDP per capita under each scenario are produced by combining the labour force and working hours estimates with a modified version of the long-term growth models presented by the OECD in OECD Economic Outlook No. 109 (see Guillemette and Turner (2021[8]) for detail). The theoretical foundation for the long-term growth models is similar to that outlined for the growth accounting exercise above. The models estimate potential GDP based on a range of long-term growth determinants and their long-term dynamics within the given country as well as on convergence patterns between countries across the projection period (here, 2020 to 2050).
The potential economic output () in the model is based on a simple Cobb-Douglas production function with constant returns to scale featuring physical capital (K), trend potential employment (N) as production factors, hours worked (), plus trend labour efficiency per hour worked (E). Trend employment, hours worked and labour efficiency enter separately for men () and women () and each age group :
Where α denotes the labour share and is fixed at 0.67. Trend employment and labour efficiency for gender and age group are defined as follows:
Where and are the employment rate and productivity factor for gender and age group (in the initial basic scenarios no gender differences in productivity are assumed). In addition, is the baseline trend labour efficiency per worker and are the average hours worked per worker at baseline. Throughout the calculations of the baseline model and the scenarios, working-age is defined as 15 to 74. All computations in this model are based on separate input for sex-specific 5‑year age groups, even if a specific input is not available on such a disaggregation. In the following, we describe the model inputs in some more detail (see also Annex Table 7.B.3).
The capital stock is based on the baseline output of the OECD Long-Term Model and taken as an exogenous input up until the start year of scenario modelling. As changes in potential trend employment generally also necessitate changes in the capital stock, K is assumed to grow at the same rate as aggregate trend employment and average trend labour efficiency so that the capital-to‑output ratio is stable in both baseline and scenario outcomes. This abstracts somewhat from a dynamic adjustment of the capital stock as modelled in the OECD Long-Term Model, but the impact on model outcomes is, at most, marginal. Likewise, trend labour efficiency is based on the baseline output of the OECD Long-Term Model, but taken as fully exogenous.
Annex Table 7.B.3. Summary of data series used in growth projections
Input |
Details |
Main source |
Additional sources |
---|---|---|---|
Physical capital (K) |
Adjusted for stable capital-to‑output ratio stable from 2020. |
OECD Long-Term Model |
|
Trend labour efficiency (E) |
Taken as exogenous from OECD Long-Term Model. For detailed scenarios, gender differences are proxies by raw gender wage gaps for Estonia from Eurostat Structure of Earnings Survey (2014). |
OECD Long-Term Model |
Eurostat Structure of Earnings Survey |
Trend employment (N) |
Projection of future labour participation by gender and five‑year age group using current rates of labour market entry and exit. |
OECD Long-Term Employment Projections |
Eurostat Population Projections and Population Statistics |
Hours worked (H) |
No projections are available. Thus, from 2020, fixed at the 2019 level within each 5‑year age group. |
OECD Employment Database |
Trend employment is based on long-run projections on employment rates and population. These baseline demographics use Eurostat Population Projections from 2020 to 2050 as well as Eurostat’s historical Population Statistics. Labour force participation rates are based on an OECD dynamic age‑cohort model that, under baseline conditions, projects future labour participation by gender and five‑year age‑group using current rates of labour market entry and exit. Hours worked per worker are based on the average usual weekly hours worked on the main job for total declared employment in the OECD Employment Database, and extrapolated to hours worked per worker per year. In the absence of future projections of the hours worked, the latest data point is kept fixed and projected into the future (i.e. it is assumed that hours worked to stay the same until 2050).
Basic scenarios
Potential GDP per capita under each scenario is estimated by adjusting projections from these long-term growth models according to the assumed change (relative to the baseline) in the overall labour force participation rate and the assumed change (relative to the baseline) in overall average usual weekly working hours. The adjustment under these scenarios for employment and working hours is assumed to occur linearly between the projection start year (2020) and the target year (2035 and 2050). As introduced above, the basic scenarios follow slightly adapted and extended prescriptions of the G20 Brisbane declaration of closing the gender gap in employment by 25% by 2025. This is goal extended to 2035 and at a 50% closure target for 2050 in Scenario A and add a more ambitious goal in Scenario B, while similar targets for working hours are treated in Scenario C and Scenario D. Combined scenarios on LFP rates and working hours are treated in Scenario E and Scenario F. In each of these scenarios, the LFP rate and the weekly worked hours for each age group and country converge to the highest level for each age group and country so that the target closure of the baseline gaps is reached by 2035 and 2050 (see details for each scenario in Chapter 5).
As mentioned above, the capital stock is adjusted so that the capital-to‑output ratio is stable. Changes and developments in other production factors – such as population trends and labour efficiency – are only considered in the detailed scenarios (see below). The resulting estimates are aggregated across both genders and all five‑year age groups to produce estimates of the size of the overall labour force (15‑74 year‑olds) and overall average working hours. Due to a lack of sufficient data on working hours, projections for Türkiye are missing from the OECD average.
Projections used in these scenarios are simply mechanical. In other words, they assume that any changes in labour force participation rates or weekly working hours do not interact with, or have any indirect effects on, other labour inputs or any other production factors (except physical capital growing to ensure a stable capital-to‑output ratio). It is possible, for example, that changes in labour force participation rates and weekly working hours among, say, parenting-age women (25‑54 year‑olds) could lead to changes in participation and/or hours among older workers if, for instance, grandparents or older friends and relatives are used as substitute carers for children. If any such indirect effects occur, the impact of changes in patterns of paid work on the overall labour supply may differ from those estimated here. It should also be noted that the projections do not explicitly factor in any possible effects of changes in patterns of paid work on household production. Again, to the extent that changes in male or female labour supply lead to changes in household production or shifts between measured and unmeasured economic activity, the estimates shown here may not fully capture the effects of a change in patterns of paid work on economic output. All of the potential effects of more equal sharing of unpaid work come from an exogenous shock in the detailed scenarios (see below).
Lastly, the measures and units used for these forward-looking projections differ slightly from those used in the growth accounting exercise. Specifically, while the latter concentrates on employment rates and a 15‑64 year‑old age group, here estimates are based on labour force participation rates (i.e. the employed plus unemployed population) and a 15‑74 year‑old age group. This is to help ensure compatibility with the inputs used for the OECD’s standard long-term growth models – which use the age‑group 15‑74 as their core input (Guillemette and Turner, 2018[7]; 2021[8]) – and because the participation of over‑65 workers is likely to increase in importance in future decades. As a result, all economic production is presented as potential economic output (potential GDP per capita).
Detailed scenarios
In contrast to the basic scenarios above, where gender LFP- and working hours gaps converge over time to a certain level, the detailed scenarios generally model a shock to labour force participation, working hours, life expectancy or productivity at different ages. Over time, these shocks transition to older age groups as the population groups subjected to the shocks age (i.e. the shock is assumed to be permanent). For example, assuming a shock that closes the gender LFP gap by 50% at ages 15‑29 in 2020, some people of this group will have turned 30 by 2021. As the shock persists, the gender LFP gap of 30‑year‑olds will remain closed by 50% relative to the baseline. In the absence of more detailed age groupings, it is therefore assumed that the gender LFP gap in the 30‑34 age group closes by 50% for a fifth of this group in 2021, then for two‑fifths a year later, reaching a closing by 50% for all members of this age group by 2025. In 2026, this cycle continues, and the gender LFP gap closes for one‑fifth of all 35‑39 year‑olds and so on. With this approach, shocks on either LFP rates or working hours slowly transition throughout the population over time.
The detailed scenarios consider gender differences in economic productivity, proxied by the relative difference in male and female wages across all age. While this measure is imperfect, productivity has been previously linked to the overall wage gap between men and women (e.g. Card, Cardoso and Kline (2016[15]) and Masso, Meriküll and Vahter (2020[16])). This does not assume that men and women have different productive potential, but rather that men and women have different access to the most productive jobs, resulting from a variety of reasons related to gender norms as present on the Estonian labour market (see below). In practice, the approach is based on the raw wage gender wage for different age groups in the Eurostat Structure of Earning Survey 2014, i.e. the gender wage gap between all men and women without controlling for individual and job characteristics. As these wage gaps were only available for 10‑year age groups, each 5‑year age group modelled in the growth projections is assigned the wage gap of the 10‑year age group they are contained in (i.e. the wage gap of 40‑49 year‑olds is assigned to those aged 40‑44 and 45‑49). As no gender wage gap beyond age 60 is available, these age groups are assigned the wage gaps of the closest age group (i.e. 50‑59 year‑olds).
Closing productivity and/or wage gaps would also affect labour force participation rates, as the incentives to enter the labour market increase with earnings. To link changes in productivity and wages with labour supply, the own-wage elasticities of labour supply as estimated by Bargain, Orsini and Peichl (2014[34]) are used. The own-wage elasticity is an estimate that is indicative of the relative change in labour supply following a change in wages, based on historical labour market data. For Estonia, the own-wage elasticity estimated by Bargain, Orsini and Peichl (2014[34]) only come disaggregated by gender and marital status. Thus, the projections assign a weighted average of the elasticity for single and married women by age group (based on single/married shares by age group for 2011 from Eurostat) and increase their LFP rate according to the increase in productivity/wages. Any of these effects are entering the projections before the employment rate shocks. Here, the projections also abstract from the own-wage elasticity concerning working hours as they are typically negligible (Bargain, Orsini and Peichl, 2014[34]).
With gender gaps in productivity, it is assumed that more equal gender norms decrease the gender wage and gender productivity gaps over time. By closing the gap in gender norms, the scenarios assume a lower prevalence of sexist prejudice and gender stereotyping, as well as a more equal sharing of unpaid work between men and women. Thinking in a between firm/within-firm framework, several channels should, under reasonable assumptions, lead to productivity increases among women (and potential decreases among men). A more equal sharing of unpaid work, would increase women’s bargaining power and human capital accumulation within firms, decrease their tendency to sort into less productive firms, as well as decrease discrimination against women across firms (more productive firms discriminating against them out of fear they will be less committed to their job due to their family responsibilities) and within firms (women being discriminated against in access to high managerial positions).
Raw wage gaps are linked to gender differences in productivity and are closed in a similar fashion as in the other scenarios (shock and transition over time). As such, assuming a shock closing the gender productivity gap by 50% for 30‑34 year‑olds, the gender productivity gap for the 35‑39 year‑olds will close some years later. Given equation , a closing of the gender gap in productivity increases overall labour productivity per worker and hour worked, and in turn increases economic output.
The life expectancy scenario assumes a reduction in the gender health gap by increasing in life expectancy for men. Current life expectancy is substantially lower for men than for women. In 2020, older women have a higher labour force participation rate than their male peers and this scenario assumes that the gender employment gap among older workers closes following increases in life expectancy and healthy life years. Based on Eurostat migration, death and fertility projections starting from 2020 (for earlier years it takes actual population figures), the scenario then introduces a shock to the male death probability for each age group 50+ to close the gap to the female equivalent, starting from 2020. It follows that the population of men increases somewhat.