This chapter introduces the study and situates it in a broader context of related research. The study assesses artificial intelligence (AI) capabilities by collecting expert judgements on whether AI can carry out tests from the OECD’s Survey of Adult Skills of the Programme for International Assessment of Adult Competencies (PIAAC). It follows up an earlier study from 2016 to track changes in AI capabilities with regard to PIAAC over time. The chapter first provides an overview of past studies that assess computer capabilities and their impact on the economy. Against this background, it presents the objectives of this study and discusses potential strengths and weaknesses of the methodological approach the study uses to assess AI. The chapter concludes with an outline of the structure of this report.
Is Education Losing the Race with Technology?
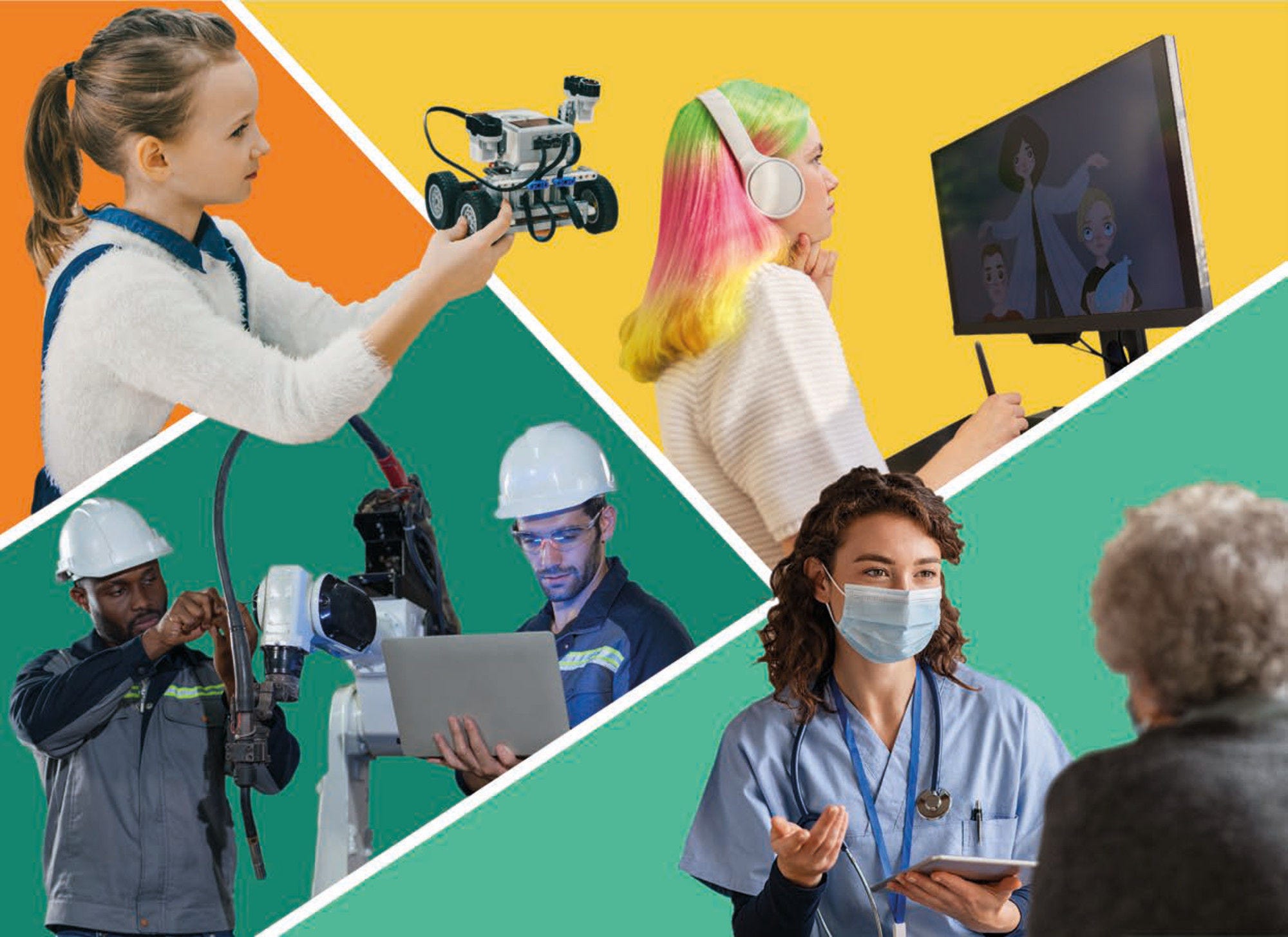
1. Setting the stage: Approaches to assessing AI’s impact
Abstract
New technologies can profoundly change the way people live and work. In the past, the steam engine, electricity and the computer transformed societies by accelerating productivity and growth and by shifting employment from agriculture to manufacturing and later to services. Today, advances in artificial intelligence (AI) and robotics are ushering in a larger and more rapid transformation. Compared to past technologies, AI and robotics can match or surpass humans in a larger number of tasks, especially those including image and speech recognition, predictions and pattern identification. This process is evolving faster than previous waves of technological progress due to steady improvements in computational power, storage capacity and algorithms.
Understanding how the capabilities of AI and robotics relate to human skills and how they develop over time is crucial for understanding the ongoing technological transformation. Knowing what AI can do compared to humans can help predict which work tasks may be automated, which skills may become obsolete and which skills may become more significant in the years ahead. This knowledge base can help develop effective labour-market policies to tackle the challenges of technological change. Moreover, it can enable policy makers to reshape education systems in ways that best prepare today’s students for the future.
In 2016, OECD carried out a study that assessed AI capabilities with respect to core human skills (Elliott, 2017[1]). This pilot study used the OECD’s Survey of Adult Skills, which is part of the Programme for International Assessment of Adult Competencies (PIAAC), as a tool to assess whether AI can carry out education tests designed for adults. The results showed that AI capabilities in literacy, numeracy and problem solving in technology-rich environments,1 as assessed by experts, resemble the performance of adults at Level 2. In OECD countries and economies, on average, more than half of the adult population perform at Level 2 or below in these domains on the PIAAC test and so would not be able to “outperform” AI (OECD, 2019[2]). This shows that many people could potentially be affected by evolving computer capabilities at their work.2
The present report follows up the pilot study, collecting expert judgements on whether computers can carry out the PIAAC literacy and numeracy tests. It shows how AI capabilities in these domains have evolved since the previous assessment. Another goal is to improve the assessment framework for eliciting expert knowledge on AI using standardised tests. The study is part of a more comprehensive ongoing project for assessing the capabilities of computers and their implications for work and education. The AI and the Future of Skills (AIFS) project at OECD’s Centre for Educational Research and Innovation (CERI) aims at developing measures of AI capabilities that are understandable, comprehensive, repeatable and policy relevant.3 For this purpose, the project uses various sources of information on AI, including expert evaluations.
PIAAC assesses the proficiency of adults aged 16-65 in three general cognitive skills – literacy, numeracy and problem solving in technology-rich environments. These skills are key determinants of individuals’ ability to participate effectively in the labour market, education and training, and social and civil life. Higher literacy proficiency, for example, is linked to higher wages, more participation in volunteer activities, higher levels of social trust, better employability and health (OECD, 2013[3]). Therefore, countries have large incentives to invest in the formation of these skills. They are associated with economic returns in the form of higher productivity and enhanced capacity for innovation. They are also linked to significant social returns such as social cohesion and civic engagement, and political and social trust.
Experts’ assessments of AI performance on the PIAAC literacy and numeracy tests provide useful information for policy making. Assessing AI capabilities in these domains is indicative of AI’s potential impact on work and life since literacy and numeracy are relevant in most social contexts and work situations. In addition, using human tests for the assessment makes it possible to compare AI and human capabilities and to draw conclusions on AI’s capacity to reproduce human skills.
This chapter draws upon extensive research in social sciences, economics and computer science to provide an overview of studies that assess computer capabilities and their impact on the economy. It then introduces the current study and its objectives. The chapter concludes with an outline of the structure of this report.
Previous studies that measure AI capabilities and impact
Most of the work on AI and robotics that is prominent in the policy discourse stems from economics and the social sciences. This literature typically focuses on AI’s potential to replace workers in the workplace and assesses its capabilities with regard to job tasks. Other strains of research from computer science and psychology analyse AI from the perspective of skills and abilities. They measure which computer capabilities are available, how they evolve over time and how they relate to human skills.
The task-based approach
Many studies in the economic literature start their analysis by looking at occupations and their task content. They analyse whether occupational tasks are susceptible to automation, typically by drawing on the judgement of computer experts. The goal is to quantify the extent to which machines can carry out occupations. This information is then linked to labour-market data to study the impact of occupations’ automatability on employment and wages. This section highlights key studies in this area.
The task-based approach originated in the seminal study of Autor, Levy and Murnane (2003[4]). This study assumes that machines can replace workers only in tasks that follow exact, routine procedures as these tasks can be easily codified. By contrast, non-routine tasks, such as those involving problem solving or social interaction, were not apt for automation because they are less explicable. The model predicts that declining prices of technology would affect the labour demand in these task domains differently. The demand for workers performing routine tasks would decrease as employers increasingly replace them with cheap machines. At the same time, more high-skilled workers will be needed for non-routine tasks emerging from the use of technology at the workplace, such as developing and operating machines.
Many studies have extended the approach of Autor, Levy and Murnane (2003[4]) to account for more recent technological advancements. The most widely cited study, Frey and Osborne (2017[5]), identifies three types of work tasks that are still hard to automate: perception and manipulation tasks, such as navigating in unstructured environments; creative intelligence tasks, such as composing music; and social intelligence tasks, such as negotiating and persuading. The authors study how these “bottleneck” tasks relate to how experts rate the automatability of 70 occupations. They use the estimated relationships to predict the probability of automation of more than 600 further occupations. The analysis relies on the Occupational Network (O*NET) database of the US Department of Labor – an occupation taxonomy that systematically links occupations to work tasks (National Center for O*NET Development, n.d.[6]). By mapping the measure of occupations’ automatability to US labour-market data, the study estimates that 47% of US employment is at high risk of automation.
Two studies supported by the OECD – Arntz, Gregory and Zierahn (2016[7]) and Nedelkoska and Quintini (2018[8]) – improve upon the methodology of Frey and Osborne’s framework. The studies include more countries in their analyses and use more fine-grained data on “bottleneck” tasks. Moreover, they estimate the measure of automatability at the level of jobs instead of occupations. This accounts for the fact that jobs within the same occupation may differ in their propensity for automation. The studies find a much smaller share of jobs prone to automation compared to Frey and Osborne (2017[5]): 9% on average across 21 OECD countries and economies as shown by Arntz et al. (2016[7]) and 14% across 32 countries and economies as shown by Nedelkoska and Quintini (2018[8]).
Assessing automation through the content of patents
Some studies draw on information from patents to measure the applicability of AI and robotics in the workplace. The study of Webb (2020[9]) scans patent descriptions for keywords such as “neural networks”, “deep learning” and “robot” to identify patents of AI and robotic technologies. It then studies the overlap between the text descriptions of such patents and the task descriptions of occupations available in O*NET. In this way, the study quantifies the exposure of occupations to these technologies. The results show that, while jobs occupied by low-skilled workers and low-wage jobs are most exposed to robotic technologies, it is the jobs of those with college degrees that are most exposed to AI. In addition, increases in occupations’ susceptibility for robotic technologies are linked to declines in employment and wages.
Squicciarini and Staccioli (2022[10]) adopted a similar approach to Webb (2020[9]). They identify patents of labour-saving robotic technologies using text-mining techniques and measure their textual proximity to occupation descriptions in ISCO08 – a standardised classification of occupations (ILO, 2012[11]). In this way, they estimate exposure of occupations to robotics. The study finds that low-skilled and blue-collar jobs, and also analytic professions, are the occupations most exposed to robotic technologies. However, there is no evidence of labour displacement, as employment shares in these occupations remain constant over time.
AI measures relying on benchmarks
In AI research, benchmarks are used to evaluate machines’ progress with regard to specific tasks and domains. A benchmark is a test dataset, on which systems perform a task or a set of tasks, and performance is rated with a standard numerical metric. This provides a common testbed for comparing different systems. Several studies connect the information from benchmarks to information on occupations to assess how evolving AI capabilities can impact the workplace.
Examples for popular benchmarks include ImageNet, a large publicly available dataset used to test systems’ ability to correctly classify images (Deng et al., 2009[12]). In the language domain, the General Language Understanding Evaluation (GLUE) benchmark tests systems on a multitude of tasks. These include predicting the sentiment of single sentences and detecting semantic similarity between the sentences in sentence pairs (Wang et al., 2018[13]). In reinforcement learning, the Arcade Learning Environment tests the ability of AI agents to maximise their performance on a defined task by testing various strategies to solving the tasks and identifying the most effective solutions (Bellemare et al., 2013[14]).
The study of Felten, Raj and Seamans (2019[15]) uses evaluation results from benchmarks to measure progress across major AI application domains, such as image and speech recognition. The authors asked gig workers on a crowdsourcing platform to rate how each AI application domain is linked to key abilities required in occupations from O*NET. By linking the AI domains to occupations, the authors assessed the extent to which occupations are exposed to AI. They assumed that occupations requiring abilities related to more rapidly advancing AI domains are more exposed to AI. The study finds that AI’s occupational impact is positively linked to wage growth, but not to employment.
Tolan et al. (2021[16]) use research output related to 328 AI benchmarks (e.g. research publications, news, blog entries) to measure the direction of AI progress (see also Martínez-Plumed et al. (2020[17])). They link these measures to tasks within occupations, obtained from labour force surveys, as well as O*NET. The link between AI progress and work tasks goes through an intermediate layer of key cognitive abilities. The latter are derived from work in psychology, animal cognition and AI, and include broad, basic capabilities, such as visual processing and navigation. Concretely, the study connects the three – AI benchmarks to cognitive abilities, abilities to work tasks, and AI benchmarks to work tasks via cognitive abilities – by drawing on expert judgement from various disciplines. The results suggest relatively high AI exposure for high-income occupations, such as medical doctors, and low AI impact on low-income occupations, such as drivers or cleaners.
Using AI-related job postings as an indicator for use of AI in firms
The demand for AI experts in firms can serve as a proxy for the use of AI in the workplace. This assumes that firms deploying AI technology also need workers with AI-related skills to operate and maintain it. Studies following this approach obtain information on firms’ skills needs from job postings.
Alekseeva et al. (2021[18]) scan job postings for AI-related skills. Both the job postings and a list of pre-defined AI skills are obtained from Burning Glass Technologies (BGT), a company that collects online vacancies daily and provides systematic information on their skill requirements. The study shows that firms with high demand for AI skills offer higher wages for both their AI and non-AI vacancies. According to the authors, this evidence supports the view that use of AI in the workplace raises the demand for complementary tasks that require advanced skills, such as project and people management tasks.
Instead of using pre-specified AI keywords, Babina and colleagues (2020[19]) estimate how frequently the skills contained in the BGT data co-occur with core AI concepts within vacancies, such as “artificial intelligence” and “machine learning”. The idea is that skills often mentioned with core AI terms are relevant for AI. In this way, the authors assess the AI-relatedness of the skill requirements of job postings. They find that firms demanding AI-related skills grow faster in terms of sales, employment and market share within the industry.
Skills-based assessment: A new approach
The impact of AI on work can also be measured by comparing the capabilities of AI to the full range of human skills required in the workplace. This comparison directly addresses the question of whether AI can replace humans in their jobs. Moreover, it provides information on the impacts of AI that go beyond the scope of current occupations. For example, it can show how occupations should be rearranged in future to better reconcile AI and human skills, and how education should evolve in response.
An AI-human comparison can be achieved by assessing AI capabilities with standardised tests developed for humans. Computer science research has already used different types of human tests for AI evaluation, including IQ tests (e.g. Liu et al., (2019[20])), school exams in mathematics (Saxton et al., 2019[21]) and science (Clark et al., 2019[22]).
Objective for the exploratory assessment of AI capabilities
This study aims at assessing AI capabilities using expert judgement on whether AI can carry out the PIAAC test. It is part of a bigger effort at the OECD to assess AI. The AIFS project aims at developing measures of AI capabilities. These are intended to help policy makers and the public to understand AI’s implications for education and work.
These measures should meet several criteria:
They should provide an accepted framework to describe AI capabilities, which shows the most important strengths and limitations of AI and highlights when AI capabilities change substantially.
As with any measures, they should be valid and reliable. In other words, they should both reflect the aspects of AI they claim to measure (validity) and provide consistent information (reliability).
Measures should be understandable for non-experts, repeatable and comprehensive, meaning they should cover all key aspects of AI. They should also be relevant to policy, helping to draw out the implications of AI for education, work and the economy.
The AIFS project draws on various sources of information about AI capabilities to develop AI measures: direct assessment of AI capabilities through benchmarks and expert judgement (OECD, 2021[23]).
Direct assessments of AI capabilities are made through benchmarks, competitions and evaluation campaigns in the AI field to track progress and evaluate systems’ performance. However, direct measures are typically only available for areas of current research and development, leaving many tasks and skills relevant for work uncovered. Moreover, direct measures are centred around state-of-the-art AI and do not assess performance on tasks that are too easy or too difficult for current systems.
Expert judgement can complement the assessment framework in areas in which information from direct measures is lacking. By filling these gaps, measures relying on expert judgement can contribute to a more comprehensive assessment of AI capabilities.
The project uses a battery of different tests to collect expert judgement on AI. As a complement to PIAAC, it uses the Programme for International Student Assessment (PISA) to measure key cognitive skills, while assessing occupation-specific skills with tests from vocational education and training. In addition, tests from the fields of animal cognition and child development will be used to assess basic low-level skills of all healthy adult humans, but which AI does not necessarily have (e.g. spatial and episodic memory) (OECD, 2021[23]).
The pilot study conducted in 2016 served as a stepping stone into the AIFS project (Elliott, 2017[1]). Expert judgement on whether AI can carry out human tests constitutes a valuable source of information for the study. Both the pilot and this follow-up intend to explore the assessment of AI capabilities with the Survey of Adult Skills using expert judgement. This new approach reveals a number of strengths:
Rating based on specific test items enables a more precise estimate of computer capabilities. Test items provide experts judging computer capabilities with precise, contextualised and granular descriptions of the task. This allows computer experts to rate potential AI performance on the task without making additional assumptions about the task requirements. This implies greater reliability across raters and greater reproducibility.
Using human tests makes it possible to compare computer to human capabilities. In particular, PIAAC enables fine-grained analyses of skill supply across different contexts, different age groups and occupations. This allows comparing AI to the average performance of particular groups of human workers. Moreover, the test offers a graduated progression from simple to complex tasks, allowing assessment and comparison of the level of proficiency of AI and humans.
Standardised tests allow tracking AI progress across time. They enable the reproducibility of the assessment – both across experts and across different time points.
Assessing AI against standardised tests provides understandable measures. Using PIAAC to describe AI capabilities provides information that is meaningful to educators and education researchers. Educators and education researchers are usually familiar with the types of skills assessed on tests like the Survey of Adult Skills. They are also familiar with the ways those skills are developed in education and potentially used at work and in daily life.
However, the assessment approach bears some challenges as well:
Overfitting is a common danger, not only with respect to using human tests on AI but also with regard to any evaluation instrument. Overfitting means that an AI system can excel on a test without being able to perform other tasks that differ only slightly from the test. This happens because AI systems are generally “narrow” or trained to perform specific tasks.
As another challenge, tests designed for humans typically take for granted skills that all humans (without severe disabilities) share, such as vision and common sense. Because such skills cannot be assumed for AI, human tests can have different implications for humans and machines. For example, the simple task to count the objects in a picture tests humans’ ability to count; for AI, it becomes a test for object recognition.
Plan of the report
This report presents the motivation, the methodological approach and the results of the assessment of AI capabilities with PIAAC. Chapter 2 provides background information on how human skills in literacy and numeracy have changed over time and how technologies processing language and solving mathematical tasks have evolved in the same period. By showing that computer capabilities develop much more rapidly than capabilities of humans in key domains, the chapter highlights the need for periodically assessing and comparing both. Chapter 3 describes the methodological approach of collecting expert judgements on whether AI can carry out the PIAAC test. Chapters 4 and 5 present the results. Chapter 4 presents the results of this follow-up study, while Chapter 5 compares these results to the results of the pilot study to track changes in the assessed AI capabilities in literacy and numeracy since 2016. Chapter 6 discusses the policy implications of evolving AI capabilities for education and work.
References
[18] Alekseeva, L. et al. (2021), “The demand for AI skills in the labor market”, Labour Economics, Vol. 71, p. 102002, https://doi.org/10.1016/j.labeco.2021.102002.
[7] Arntz, M., T. Gregory and U. Zierahn (2016), “The Risk of Automation for Jobs in OECD Countries: A Comparative Analysis”, OECD Social, Employment and Migration Working Papers, No. 189, OECD Publishing, Paris, https://doi.org/10.1787/5jlz9h56dvq7-en.
[4] Autor, D., F. Levy and R. Murnane (2003), “The Skill Content of Recent Technological Change: An Empirical Exploration”, The Quarterly Journal of Economics, Vol. 118/4, pp. 1279-1333, https://doi.org/10.1162/003355303322552801.
[19] Babina, T. et al. (2020), “Artificial Intelligence, Firm Growth, and Industry Concentration”, SSRN Electronic Journal, https://doi.org/10.2139/ssrn.3651052.
[14] Bellemare, M. et al. (2013), “The Arcade Learning Environment: An Evaluation Platform for General Agents”, Journal of Artificial Intelligence Research, Vol. 47, pp. 253-279, https://doi.org/10.1613/jair.3912.
[22] Clark, P. et al. (2019), “From ’F’ to ’A’ on the N.Y. Regents Science Exams: An Overview of the Aristo Project”.
[12] Deng, J. et al. (2009), “ImageNet: A large-scale hierarchical image database”, 2009 IEEE Conference on Computer Vision and Pattern Recognition, https://doi.org/10.1109/cvpr.2009.5206848.
[1] Elliott, S. (2017), Computers and the Future of Skill Demand, Educational Research and Innovation, OECD Publishing, Paris, https://doi.org/10.1787/9789264284395-en.
[15] Felten, E., M. Raj and R. Seamans (2019), “The Variable Impact of Artificial Intelligence on Labor: The Role of Complementary Skills and Technologies”, SSRN Electronic Journal, https://doi.org/10.2139/ssrn.3368605.
[5] Frey, C. and M. Osborne (2017), “The future of employment: How susceptible are jobs to computerisation?”, Technological Forecasting and Social Change, Vol. 114, pp. 254-280, https://doi.org/10.1016/j.techfore.2016.08.019.
[11] ILO (2012), International Standard Classification of Occupations. ISCO-08. Volume 1: Structure, group definitions and correspondence tables, International Labour Organization.
[20] Liu, Y. et al. (2019), “How Well Do Machines Perform on IQ tests: a Comparison Study on a Large-Scale Dataset”, Proceedings of the Twenty-Eighth International Joint Conference on Artificial Intelligence, https://doi.org/10.24963/ijcai.2019/846.
[17] Martínez-Plumed, F. et al. (2020), “Does AI Qualify for the Job?”, Proceedings of the AAAI/ACM Conference on AI, Ethics, and Society, https://doi.org/10.1145/3375627.3375831.
[6] National Center for O*NET Development (n.d.), O*NET 27.2 Database, https://www.onetcenter.org/database.html (accessed on 24 February 2023).
[8] Nedelkoska, L. and G. Quintini (2018), “Automation, skills use and training”, OECD Social, Employment and Migration Working Papers, No. 202, OECD Publishing, Paris, https://doi.org/10.1787/2e2f4eea-en.
[23] OECD (2021), AI and the Future of Skills, Volume 1: Capabilities and Assessments, Educational Research and Innovation, OECD Publishing, Paris, https://doi.org/10.1787/5ee71f34-en.
[25] OECD (2021), The Assessment Frameworks for Cycle 2 of the Programme for the International Assessment of Adult Competencies, OECD Skills Studies, OECD Publishing, Paris, https://doi.org/10.1787/4bc2342d-en.
[2] OECD (2019), Skills Matter: Additional Results from the Survey of Adult Skills, OECD Skills Studies, OECD Publishing, Paris, https://doi.org/10.1787/1f029d8f-en.
[3] OECD (2013), OECD Skills Outlook 2013: First Results from the Survey of Adult Skills, OECD Publishing, Paris, https://doi.org/10.1787/9789264204256-en.
[24] OECD (2012), Literacy, Numeracy and Problem Solving in Technology-Rich Environments: Framework for the OECD Survey of Adult Skills, OECD Publishing, Paris, https://doi.org/10.1787/9789264128859-en.
[21] Saxton, D. et al. (2019), “Analysing Mathematical Reasoning Abilities of Neural Models”.
[10] Squicciarini, M. and J. Staccioli (2022), “Labour-saving technologies and employment levels: Are robots really making workers redundant?”, OECD Science, Technology and Industry Policy Papers, No. 124, OECD Publishing, Paris, https://doi.org/10.1787/9ce86ca5-en.
[16] Tolan, S. et al. (2021), “Measuring the Occupational Impact of AI: Tasks, Cognitive Abilities and AI Benchmarks”, Journal of Artificial Intelligence Research, Vol. 71, pp. 191-236, https://doi.org/10.1613/jair.1.12647.
[13] Wang, A. et al. (2018), “GLUE: A Multi-Task Benchmark and Analysis Platform for Natural Language Understanding”.
[9] Webb, M. (2020), “The Impact of Artificial Intelligence on the Labor Market”, SSRN Electronic Journal, https://doi.org/10.2139/ssrn.3482150.
Notes
← 1. PIAAC defines problem solving in technology-rich environments as the ability to use “digital technology, communication tools and networks to acquire and evaluate information, communicate with others and perform practical tasks” (OECD, 2012[24]). The focus is not on “computer literacy”, but rather on the cognitive skills required in the information age. Examples include locating and evaluating information on the Internet for quality and credibility, managing personal finances using spreadsheets, statistical packages, or operating a computer.
These skills are assessed only in the First Cycle of PIAAC (2011-17), which is the focus of this report. The Second Cycle, which is under way, assesses adaptive problem solving instead. This is the ability of problem solvers to handle dynamic and changing situations, and to adapt their initial solution to new information or circumstances (OECD, 2021[25]).
← 2. Throughout this report, the term “computers” is used to refer generally to AI, robots and other types of information and communications technologies.
← 3. See https://www.oecd.org/education/ceri/future-of-skills.htm (accessed on 21 February 2023).