What is specific and unique about data that fuels such economic and social change? This chapter describes what data are, how they can be characterised and how these characteristics challenge the measurement of their value, as well as their relationship with digital technologies. In so doing, it clarifies a policy debate that is complicated by a lack of a common conceptual understanding of data. The chapter concludes by outlining the cross-cutting policy objectives and tensions at the heart of data governance policy making and the potential role of technological and organisational approaches.
Going Digital to Advance Data Governance for Growth and Well-being
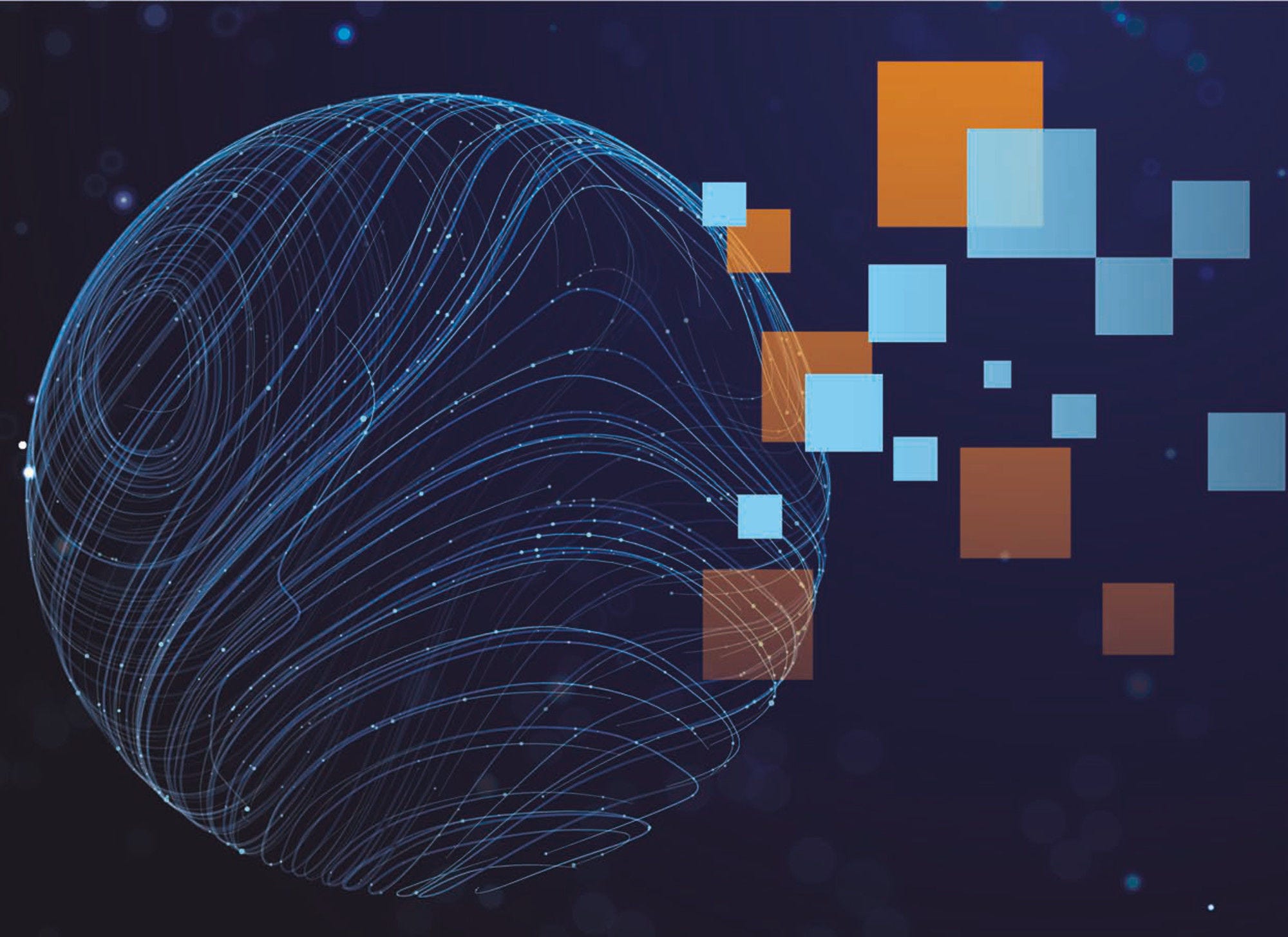
3. Understanding data: Key characteristics, technological developments and policy challenges
Abstract
3.1. Key characteristics of data
Data are intangible, meaning they do not have a physical or financial embodiment. Like other intangible assets, like software, they are viewed as an asset because they are a source of economic value, can provide future economic returns and are usually under the effective control of an economic actor (Haskel and Westlake, 2018[1]). Data are also non-rival (Jones and Tonetti, 2020[2]). It is impossible for two people to drive the same car at the same time, or for a single drop of oil to be used in two different cars. However, unlike cars or oil, data are theoretically infinitely usable. Data can be used productively and continuously, including simultaneously by multiple actors or machines, without reducing the amount of data available to other actors or applications.
Some non-rival goods are also non-excludable, meaning that access to the good cannot be easily restricted. Take the example of a lighthouse: it would be nearly impossible for a lighthouse keeper to restrict the light to guide some boats and not others. Excludability is a spectrum, however. Thus, use of data can be restricted through a variety of means, including intellectual property rights, data protection regimes or encryption (Ostrom, 2010[3]). Data are not inherently non-excludable, although data that are made available or circulated e.g. on the Internet, cannot easily be controlled, and their use may not be easily restricted.
Data are subject to a range of externalities, which is to say that data sharing and use can generate wider benefits and costs for those who may not be directly implicated in the transaction. Pollution is a classic example of a negative externality: the negative effect of air pollution on society is not usually captured in the private cost to car owners or oil- or car-producers. Data and their sharing and use can be subject to negative externalities. For example, when an individual chooses to share data on line, this could be used to gain information about others who did not consent to the data being shared, and who are not compensated for what might constitute a loss of privacy (Acemoglu et al., 2020[4]; Coyle et al., 2020[5]).
However, data can also exhibit positive externalities, often due to synergies. With synergies, value emerges from comparison, aggregation or processing for information; individual data points often have little to no value. For example, knowing the location of one coronavirus patient may be of little use. However, combining this data point with the location of many other positive patients contributes to a more accurate map of the spread of COVID-19. Importantly, the benefits of this increased accuracy e.g. through better public health management, also flow to those people who did not share their data. A key aspect of the economic and social benefits of data come from such positive spillovers from data’s productive use. Chapter 2 examines the benefits of data use for economies and societies, but these gains likely pale in comparison to the potential social benefits from enabling data locked in organisations to be shared more widely.
Externalities can be a source of market failure, indicating that private incentives may not deliver the full range of economic and social benefits possible from greater investment, sharing and use of data. For example, firms may not have an incentive to share data if they cannot capture the benefits of this sharing. There may also be an over-production or collection of some kinds of data, and underproduction of data in contexts with few commercial opportunities (Coyle et al., 2020[5]). In turn, market failure provides a rationale for government intervention (see Chapter 4).
When data are used as a factor of production, they can exhibit increasing returns to scale. This means the output from the use of data increases by a larger proportion than an increase in data volume.1 A virtuous cycle can emerge where data analysis yields data-driven insights, and the use of such learnings increases product quality and/or scope of operations such that more data are generated. The benefits of this cycle of value creation could be amplified across many actors and across the global economy because of data’s non-rival characteristics. However, realising these benefits depends on access to data, as well as significant complementary investments and capacities, like skills or computing (OECD, 2022[6]).
Although data’s value can often be a function of their scale, and data are easily generated en masse and automatically by digital technologies, data are highly heterogenous. As the technical means to record information expands, data can relate to any observable phenomenon. Policy frameworks often recognise this heterogeneity by identifying different settings for data with different information content or different origins. For example, weather data are usually treated differently in policy frameworks to health data. The latter are usually both personal in nature and subject to ethical standards like medical confidentiality (OECD, 2019[7]).
Data are often co-produced, meaning they are often the result of interactions between many different actors and/or machines. This can mean that many actors can have multiple and overlapping claims to data. Through their choices to share or use data, individuals or organisations might create positive or negative externalities for each other. In addition, the non-rival nature of data and their ability to easily flow and be exploited in multiple applications can challenge conventional notions of ownership (OECD, 2022[8]).
3.2. Understanding the value of data
These characteristics mean that the value of data can be hard to measure. The value of data often relates to their content, namely the information embodied in data after processing, and the context in which the data are collected, stored and used (Mitchell, Ker and Lesher, 2021[9]). Because they can be used in many current and future applications, data may also have considerable option value. This means that incentives exist to collect and store data even without any immediate plans for their use. Similarly, the lack of defined ownership or property rights, and the heterogeneity and other characteristics of data, pose challenges to the emergence of data markets. This is especially true for large-scale, multilateral markets in which data are exchanged under standardised terms (OECD, 2022[10]).
As a result, market statistics can provide an illustrative, but limited, picture of the value of data. For example, while the vast majority of firms do not trade data, firms may indirectly monetise data by selling data-driven services like targeted advertising. The market capitalisation of such firms, or their reported revenues from the sale of data-intensive services, or international trade in such services, can shed light on the value of data. However, data-driven services combine data with other inputs, including digital technologies like AI. This makes it difficult to isolate the value of data. Moreover, classifications in statistical frameworks do not generally help delineate data-driven services, or the firms that provide them. Such estimates would also fail to capture the value of data for the firms that collect data and use them for their internal operations (OECD, 2022[10]).
Nevertheless, data clearly have value as an input into productive activities for organisations. Moreover, better capture of that value in macroeconomic statistics through the System of National Accounts (SNA) would help enable better and more comparable estimates of investments in, and the stock of, data across economies.
With other international institutions, the OECD is working to explicitly incorporate business production and use of data into the planned 2025 update of the SNA statistical framework. In view of the issues with market-based valuations of data, a consensus has emerged on the use of the “sum-of-cost” approach. This measures the value of data and data assets based on the incurred costs of production, like compensation of employees and use of fixed capital.
Many practical challenges persist for the sum-of-cost approach. These include understanding which costs to include, identifying assumptions that should inform estimates of costs and avoiding overlap with the costs included in estimates of other intangible assets, Developing guidance on these issues is a key part of the work of the OECD and the international statistical community for the next update of the SNA (OECD, 2022[10]).
National statistical institutes across the OECD have developed experimental estimates for the value of data assets using the sum-of-cost approach, although with varying underlying assumptions. These initial estimates are sizeable: annual investment in total data assets was between 2.2% and 2.9% of total value added in Australia (2016), 1.4% and 1.9% in Canada (2018), 2.4% and 3.0% in the Netherlands (2017) and 0.8% in the United States (2020). Estimates by academia, based on a broader definition of data assets, range between 3.8% and 6.6% of the market sector’s value added in selected EU countries. Nevertheless, empirical studies suggest other parts of the SNA already capture these expenditures. This implies that implementation of these efforts in macroeconomic statistics will help better separate and understand the value of data and data assets. However, these efforts will have limited effect on macroeconomic statistics like gross domestic product and productivity (OECD, 2022[10]).
3.3. Data and digital technologies are fundamentally linked
Recorded information could theoretically be in any format, including analogue formats like paper, or emerging quantum forms like qubits. However, policy efforts have largely focused on the governance of digital data, namely information stored by a computer in binary format. Unlike some other inputs into production, like air or water, digital data are not naturally occurring or managed. Instead, digital data depend on digital technologies for their generation, collection, storage, transfer and use.
The emergence of vast amounts of digital data stems from the deployment of broadband networks. These enable more networked users and machines in an increasing number of contexts, sectors and applications to generate and transmit digital data. The proliferation and increasing frequency of use of connected devices like the smartphone enables the observation and recording of a range of primary data. It also permits the collection of inferred or secondary data, such as with respect to user behaviour in the digital environment or previous searching or purchasing patterns.
These digital data are put to productive use through the use of digital technologies like AI and data analytics. Increasingly statistical and probabilistic AI systems use data to “train” models to make accurate predictions, recommendations or decisions (OECD, 2019[11]). In turn, such data processing can enable the extraction of valuable insights from datasets, which may have otherwise remained unexploited. The magnitude of possible opportunities and risks of data collection has increased as a result of advances in the capacities of these digital technologies.
More generally, when organisations use data in their activities and operations, they often adopt digital technologies in the process of generating, collecting and storing data, in addition to analysing them. Cloud computing is a digital technology that enables scalable access to computing resources, including software, storage and processing infrastructures. Current cloud computing business models often involve the transfer of data to data centres that centralise computing services and resources. Across the OECD, the uptake of data analytics appears complementary to the uptake of cloud computing (OECD, 2022[6]). This highlights that cloud computing may enable more actors, including SMEs, to use data.
Data governance policies should seek to consider the digital technologies that underpin the generation, collection, transfer and productive use of data. For example, a current concern of data governance involves regulations that mandate that some kinds of data are processed, or at least one copy is stored, within a given jurisdiction. But evolutions in digital technologies, including the virtualisation of broadband networks and the increasing bandwidth requirements of the Internet of Things, may mean that more data are processed and stored at the “edge” of the network, including within intelligent devices themselves. This could reduce the need for data to cross borders in some applications (OECD, 2022[12]).
3.4. Coping with the new data-driven reality
With many policies pre-dating the data-driven era, existing institutions, organisations and legal frameworks must adjust to the growing importance of data and the shifts in their use. Many policy frameworks have struggled to keep up with a shifting technological landscape. Meanwhile, institutions have been slow to collect, share and use data to their full potential.
Privacy enforcement authorities increasingly acknowledge the difficulties with safeguarding privacy in the digital age. A recent review of the OECD Guidelines on the Protection of Privacy and Transborder Flows of Personal Data (hereafter the OECD Privacy Guidelines) (OECD, 2021[13]) acknowledged that technological advances are expanding the methods and ease with which individuals may be identified by their data or re-identified from apparently anonymised data. The rapid pace of such advances means that data that are considered private today may not be private tomorrow (OECD, 2022[14]). For example, in 2019, all but four of the surveyed privacy enforcement authorities noted that technological developments were the main challenge to regulatory frameworks. Most frequently, they cited big data analytics and AI as the greatest risk (see Figure 3.1).
Figure 3.1. Emerging technologies that pose the main challenges for privacy and personal data protection
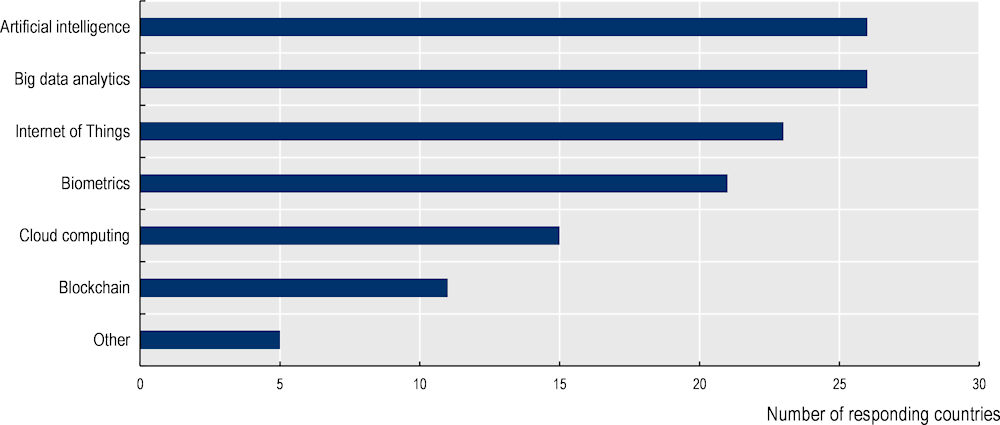
Source: OECD (2021[13]), Report on the Implementation of the Recommendation of the Council Concerning Guidelines Governing the Protection of Privacy and Transborder Flows of Personal Data, https://one.oecd.org/document/C(2021)42/en/pdf.
Figure 3.2. OECD Open, Useful and Re-usable data (OURdata) Index: 2019
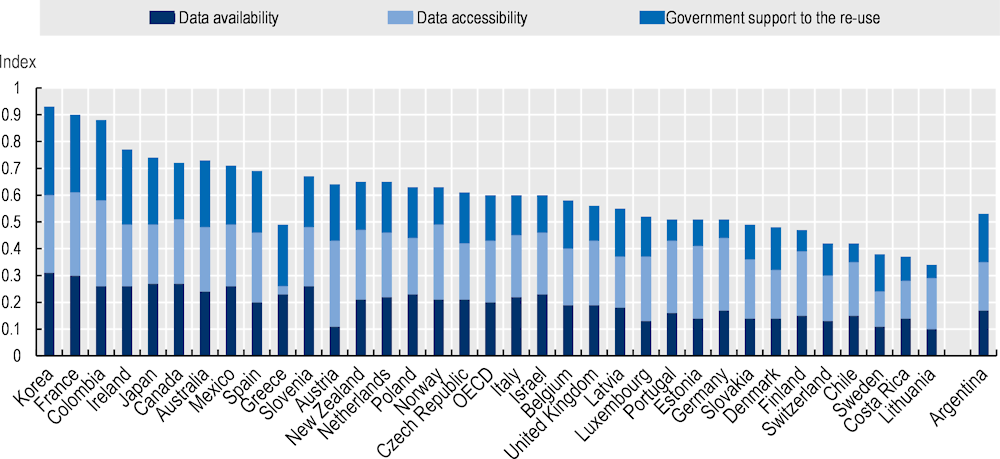
Note: Data are not available for Hungary, Iceland, Türkiye and the United States. Data for Costa Rica were collected from the IDB-OECD Open Government Data Survey 2018.
Source: OECD (2020[20]), "Open, Useful and Re-usable data (OURdata) Index: 2019", https://doi.org/10.1787/45f6de2d-en.
Governments are among the largest producers and consumers of data, and making these data available can be a cornerstone of innovation for other actors (OECD, 2015[15]; 2019[16]). In particular, governments can aim to make data more available through stronger open data agendas. Potential exists to further enable access to public data. For example, the quantity of available datasets only slightly increased from 2017 to 2019 (OECD, 2019[17]).
Of policy measures to foster and enhance access to data, most (65%) still focus on public sector data (OECD, 2019[18]). In this regard, open government data has been a priority for countries for over a decade to support business innovation, social value creation and government transparency. In 2018, as many as 8 out of 10 OECD countries had a strategy for open government data, and 9 out of 10 had requirements for public-sector organisations to publish open data in a machine-readable format (OECD, 2020[19]). Also, results from the 2019 Open, Useful and Re-usable data (OURdata Index) show that “the OECD average increased from 0.54 in 2017 to 0.60 in 2019, indicating a greater general maturity of open data policies at the central level” (OECD, 2020[20]).
Box 3.1. Few countries had the real-time data they needed when the pandemic hit
The lack of timely data is one of the key areas of concern for policy makers combatting the COVID-19 pandemic. Figure 3.3 shows that just before the pandemic hit, few countries had real-time data about key parts of the health care system. Denmark, Estonia, Korea and Latvia stood out for having a short time lapse, of one week or less, between when a data record was first created and when it was included in the national dataset used for analysis across all or most key national health datasets. The main barriers cited by countries related to legal restrictions or policy barriers to data sharing among policy authorities or undertaking data linkages. Since the pandemic, several countries have acted to improve timely information for decision making (see section 2.4).
Figure 3.3. Real-time data available to governments as the COVID-19 pandemic hit
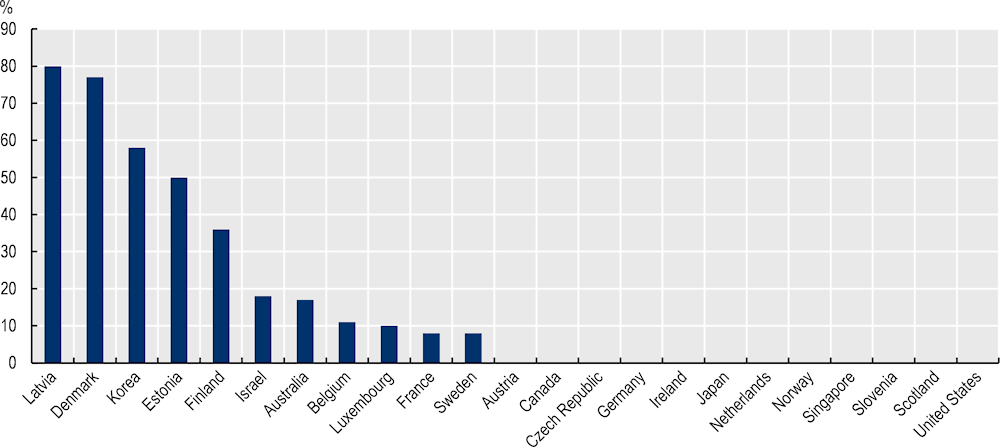
Source: Oderkirk (2021[23]), “Survey results: National health data infrastructure and governance”, https://doi.org/10.1787/55d24b5d-en.
In contrast, only 1 out of 10 OECD countries had a dedicated, comprehensive public sector data strategy covering a broader spectrum of data access and sharing arrangements, and their enablers. Examples of such strategies include Ireland’s Public Service Data Strategy 2019-23 (Ireland DPER, 2019[21]) and the Danish government’s Basic Data Strategy, published as early as 2012 (Local Government Denmark, 2012[22]). Few countries have policy initiatives to facilitate data sharing within the private sector, although sharing and re-use of private sector data is a frequently cited challenge (OECD, 2019[18]).
Similarly, governments have been slow to realise the benefits of data in informing better policies and decision making. For example, although governments invested in health data and governance in response to COVID-19 (section 2.4), the pandemic revealed the weakness of data collection and sharing frameworks (see Box 3.1).
In a globalised and digital economy, enabling cross-border flows of data remains essential for many individuals, firms and organisations. However, data crossing jurisdictional boundaries can raise concerns about the application and enforcement of domestic policies. Governments are increasingly placing conditions on cross-border data flows. This results in a complex landscape for many actors, including regulators. For example, in 2019, privacy enforcement authorities most often noted “uncertainty regarding legal privacy regimes” and “incompatibility of legal regimes” as the main challenges to transborder data flows (OECD, 2021[13]). Despite this uncertainty, recent OECD work highlights that many frameworks show a degree of commonalities, convergence or complementarities. These can be used to foster a foundation of trust for data flows (Casalini, López González and Nemoto, 2021[24]) (see Box 3.2).
Box 3.2. Data free flows with “trust”: An essential component of the global economy
Data have become integral to international trade, and cross-border data flows are necessary for enabling social interactions, facilitating trade in digital products, co‑ordinating production along global value chains and enabling firms to access global markets. However, despite the international relevance of data, countries make policies at the national level. This includes policies that aim to protect privacy, digital security, national security and other public policy interests. These data governance policies can nonetheless have international impacts as international firms are forced to comply with multiple, intersecting policy frameworks. Such a patchwork can carry a risk of international fragmentation in the trade of data and digital products across borders. This fragmented landscape also shifts frequently, as new arrangements proliferate and others are struck down. This, in turn, contributes to regulatory uncertainty that may preclude the ability of firms to make long-term plans and investments.
The challenge for policy makers is promoting international discussions that create a global and interoperable digital environment where data can move across borders with trust. Despite some differences between countries, there remain areas of complementarity, convergence and commonality among national approaches to cross-border data flows. This indicates the potential for architectures that seek to combine the benefits of data flows and the achievement of public policy objectives. A promising approach focuses on interoperability of privacy regimes, understood as the “ability of different privacy and data protection regimes, or legal frameworks, to work together at multiple levels through policy and practical arrangements and thereby bridge any differences in approaches and systems of privacy and personal data protection to facilitate transborder flows of personal data”.
Sources: OECD (2022[14]), “Fostering cross-border data flows with trust”, https://doi.org/10.1787/139b32ad-en; Robinson, Kizawa and Ronchi (2021[25]), “Interoperability of privacy and data protection frameworks”, https://doi.org/10.1787/64923d53-en.
3.5. Cross-cutting policy objectives and tensions and promising approaches
Data governance is characterised by overlapping policy tensions and objectives, and different intersections across policy domains. These overlaps call for whole-of-government approaches and new organisational and technical mechanisms to data governance. In particular, data intermediaries and privacy-enhancing technologies show promise in navigating the new data governance landscape.
The Going Digital Guide to Data Governance Policy Making (OECD, 2022[26]) introduces a conceptual foundation of policy objectives and tensions related to data governance that apply across policy domains and a checklist to put it into practice. These policy tensions result from the underlying characteristics of data (section 3.1), their benefits (section 2.1) and potential risks (section 2.3):
1. Balancing data openness and control while maximising trust: Data can be characterised by different levels of openness on a spectrum ranging from full and unrestricted openness to arrangements that condition or limit the access, sharing, transfer and/or use of data to specific users, destinations or use cases. Higher levels of openness imply an increased ability to reap the benefits of data. However, they are often associated with a higher degree of risk, often linked to loss of control.
2. Managing overlapping and potentially conflicting interests and regulations related to data: Multiple stakeholders are often involved in data generation and may have competing or overlapping rights, interests or legal control over these data. Data governance structures may need to evolve to accommodate these interests or enable them to be prioritised.
3. Incentivising investments in data and their effective re-use: Investments are often required to generate, collect and use data. However, the original data holder may not have an incentive to make such investments or share data if the benefits of data use accrue to other actors or society.
These tensions cut across policy domains and need to be addressed holistically. For example, the increasing importance of data collected from or about individuals calls for cross-regulatory co‑operation between privacy enforcement authorities (and frameworks) and other regulators, such as competition authorities. As outlined in section 2.2, some firms may be in a better position to collect and generate data, and this position may be self-reinforcing. Where a firm’s control of non-replicable datasets can inhibit competition and distort the level playing field, authorities may feel compelled to intervene in the market to enhance access to data. Yet, when the data in question are personal and under the purview of data protection and privacy laws, it may conflict with privacy frameworks to provide third parties with access to such data (OECD, 2022[6]).
On the other hand, data protection requirements may be interpreted as distorting competition if equivalent transfers of personal data are possible within large incumbents i.e. between subsidiaries but not between independently owned businesses (CMA/ICO, 2021[27]). Some OECD jurisdictions have interpreted the personal data collection practices of firms, and the resulting reduction in privacy, as an abuse of market power and a violation of competition law (OECD, 2022[28]; 2020[29]).
The relationship between competition and privacy frameworks highlights several of the cross-cutting policy opportunities and tensions outlined above. Namely, privacy considerations can be conceptualised as a legal compulsion to keep data more closed and under the effective control of relatively few users. Meanwhile, some efforts to increase competition involve opening access to data. Efforts to mediate this conflict include data portability measures, which enable controlled data transfers between parties upon request (OECD, 2021[30]).
However, firms may not be incentivised to invest in collecting and using data if the expected returns to such investments are reduced by legally compelled data sharing. Similarly, effective sharing of data to enable their wider use relies on technical standards and interoperability of data across use cases, which is a sub-dimension of openness. This relationship underscores that formerly disparate policy frameworks now require a whole-of-government approach and cross-regulatory co‑operation to address data governance challenges, adapt policies and realise the potential of data in the digital age.
New organisational and technical approaches may complement traditional policy making to address some of the tensions. “Data intermediaries” – “service providers that facilitate data access and sharing under commercial or non-commercial agreements between data holders, data producers, and/or users” (OECD, 2021[31]) – are expected to play a key role in the data ecosystem. Added-value services provided by data intermediaries could include data processing services (including data aggregation), payment and clearing services, as well as legal services including the provision of standard licence and certification schemes (OECD, 2019[18]; forthcoming[32]).
Privacy-enhancing technologies (PETs) also show promise to enable wider re-use of data while managing potential risks to privacy and security. PETs enable data to be processed without disclosing their inputs, or preventing their (re-)identification. As such, they might fundamentally alter the way organisations gather, access and process data, including personal data.
In general, PETs include data accountability tools, data obfuscation tools, encrypted data processing tools and distributed analytics that enable more control for data subjects. PETs are increasingly cited in a variety of policy domains as a means of moving closer to the goal of “privacy by design” (OECD, forthcoming[33]). However, developments in data analytics and AI combined with the increasing volume and variety of available data sets and the capacity to link these different data sets have altered the technical landscape. Essentially, these changes have made it easier to infer and relate seemingly non-personal or anonymised data to an identified or identifiable entity (OECD, 2022[12]).
References
[4] Acemoglu, D. et al. (2020), “Too much data: Prices and inefficiencies in data markets”, NBER Working Paper, No. 26296, https://www.nber.org/papers/w26296.
[34] Bajari, P. et al. (2019), “The impact of big data on firm performance: An empirical investigation”, AEA Papers and Proceedings, No. 109, https://www.aeaweb.org/articles?id=10.1257/pandp.20191000.
[24] Casalini, F., J. López González and T. Nemoto (2021), “Mapping commonalities in regulatory approaches to cross-border data transfers”, OECD Trade Policy Papers, No. 248, OECD Publishing, Paris, https://doi.org/10.1787/ca9f974e-en.
[27] CMA/ICO (2021), “Competition and data protection in digital markets: A joint statement between the the CMA and the ICO”, Competition & Markets Authority and Information Commissioner’s Office, London, https://ico.org.uk/media/about-the-ico/documents/2619797/cma-ico-public-statement-20210518.pdf.
[5] Coyle, D. et al. (2020), The Value of Data – Policy Implications, Bennett Institute for Public Policy, Cambridge in partnership with the Open Data Institute, https://www.bennettinstitute.cam.ac.uk/publications/value-data-policy-implications/.
[1] Haskel, J. and S. Westlake (2018), Capitalism without Capital: The Rise of the Intangible Economy, Princeton University Press, Princeton.
[35] Iansiti, M. (2021), “The value of data and its impact on competition”, Harvard Business School NOM Unit Working Paper, No. 22-002, https://doi.org/10.2139/ssrn.3890387.
[21] Ireland DPER (2019), Public Service Data Strategy 2019-2023, Department of Public Expendiure and Reform, Ireland, https://www.gov.ie/en/publication/1d6bc7-public-service-data-strategy-2019-2023/.
[2] Jones, C. and C. Tonetti (2020), “Nonrivalry and the economics of data”, American Economic Review, Vol. 9, pp. 2018-2058, https://doi.org/10.1257/aer.20191330.
[22] Local Government Denmark (2012), Good Basic Data For Everyone - A Driver for Growth and Efficiency, https://en.digst.dk/media/18773/good-basic-data-for-everyone-a-driver-for-growth-and-efficiency.pdf.
[9] Mitchell, J., D. Ker and M. Lesher (2021), “Measuring the economic value of data”, OECD Going Digital Toolkit Notes, No. 20, https://doi.org/10.1787/f46b3691-en.
[23] Oderkirk, J. (2021), “Survey results: National health data infrastructure and governance”, OECD Health Working Papers, No. 127, OECD Publishing, Paris, https://doi.org/10.1787/55d24b5d-en.
[12] OECD (2022), “Data in an evolving technological landscape: The case of connected and automated vehicles”, OECD Digital Economy Papers, No. 346, OECD Publishing, Paris, https://doi.org/10.1787/ec7d2f6b-en.
[6] OECD (2022), “Data shaping firms and markets”, OECD Digital Economy Papers, No. 344, OECD Publishing, Paris, https://doi.org/10.1787/7b1a2d70-en.
[14] OECD (2022), “Fostering cross-border data flows with trust”, OECD Digital Economy Policy Papers, No. 343, OECD Publishing, Paris, https://doi.org/10.1787/139b32ad-en.
[26] OECD (2022), Going Digital Guide to Data Governance Policy Making, OECD Publishing, Paris, https://doi.org/10.1787/40d53904-en.
[10] OECD (2022), “Measuring the value of data and data flows”, OECD Digital Economy Papers, OECD Publishing, Paris, https://doi.org/10.1787/923230a6-en.
[28] OECD (2022), OECD Handbook on Competition Policy in the Digital Age, OECD, Paris, https://www.oecd.org/daf/competition/oecd-handbook-on-competition-policy-in-the-digital-age.pdf.
[8] OECD (2022), “Responding to societal challenges with data: Access, sharing, stewardship and control”, OECD Digital Economy Papers, No. 342, OECD Publishing, Paris, https://doi.org/10.1787/2182ce9f-en.
[30] OECD (2021), “Mapping data portability initiatives, opportunities and challenges”, OECD Digital Economy Papers, No. 321, OECD Publishing, Paris, https://doi.org/10.1787/a6edfab2-en.
[31] OECD (2021), Recommendation of the Council on Enhancing Access to and Sharing of Data, OECD/LEGAL/0463, OECD, Paris, https://legalinstruments.oecd.org/en/instruments/OECD-LEGAL-0463.
[13] OECD (2021), Report on the Implementation of the Recommendation of the Council Concerning Guidelines Governing the Protection of Privacy and Transborder Flows of Personal Data, OECD, Paris, https://one.oecd.org/document/C(2021)42/en/pdf.
[29] OECD (2020), “Consumer data rights and competition”, OECD Competition Background Note, OECD, Paris, http://www.oecd.org/daf/competition/consumer-data-rights-and-competition.htm.
[19] OECD (2020), “Digital Government Index: 2019 results”, OECD Public Governance Policy Papers, No. 03, OECD Publishing, Paris, https://doi.org/10.1787/4de9f5bb-en.
[20] OECD (2020), “Open, Useful and Re-usable data (OURdata) Index: 2019”, OECD Public Governance Policy Papers, No. 01, OECD Publishing, Paris, https://doi.org/10.1787/45f6de2d-en.
[11] OECD (2019), Artificial Intelligence in Society, OECD Publishing, Paris, https://doi.org/10.1787/eedfee77-en.
[18] OECD (2019), Enhancing Access to and Sharing of Data: Reconciling Risks and Benefits for Data Re-use across Societies, OECD Publishing, Paris, https://doi.org/10.1787/276aaca8-en.
[16] OECD (2019), Going Digital: Shaping Policies, Improving Lives, OECD Publishing, Paris, https://doi.org/10.1787/9789264312012-en.
[17] OECD (2019), Government at a Glance 2019, OECD Publishing, Paris, https://doi.org/10.1787/8ccf5c38-en.
[7] OECD (2019), Recommendation of the Council on Health Data Governance, OECD/LEGAL/0433, OECD, Paris, https://legalinstruments.oecd.org/en/instruments/OECD-LEGAL-0433.
[15] OECD (2015), Data-Driven Innovation: Big Data for Growth and Well-Being, OECD Publishing, Paris, https://doi.org/10.1787/9789264229358-en.
[32] OECD (forthcoming), Companion document to the OECD Council Recommendation on Enhancing Access to and Sharing of Data, OECD Publishing, Paris.
[33] OECD (forthcoming), “Emerging privacy enhancing technologies: Maturity, opportunities and challenges”, OECD Digital Economy Papers, OECD Publishing, Paris.
[3] Ostrom, E. (2010), “Beyond markets and states: Polycentric governance of complex economic systems”, American Economic Review, Vol. 100/3, pp. 641-672, https://pubs.aeaweb.org/doi/pdfplus/10.1257/aer.100.3.641.
[25] Robinson, L., K. Kizawa and E. Ronchi (2021), “Interoperability of privacy and data protection frameworks”, Going Digital Toolkit Note, No. 21, OECD Publishing, Paris, https://doi.org/10.1787/64923d53-en.
Note
← 1. Data used in a single application might exhibit diminishing returns to scale over time. Additional data can have a large initial effect on model precision, but the effect may decrease with increasing sample size (Iansiti, 2021[35]). For example, Bajari et al. (2019[34]) find that increasing data sample size over time improves the accuracy of forecasts but at a diminishing rate.