To complement the policy simulations, a choice experiment was developed to understand farmers’ preferences for different attributes of agri-environmental payments, including practice-based and results-based payment mechanisms. This chapter draws on experiments undertaken in 2021 with farmers across three OECD countries (Finland, Netherlands and Sweden) to understand the role of payment design and farmer characteristics on participation in different agri-environmental payment schemes.
Making Agri-Environmental Payments More Cost Effective
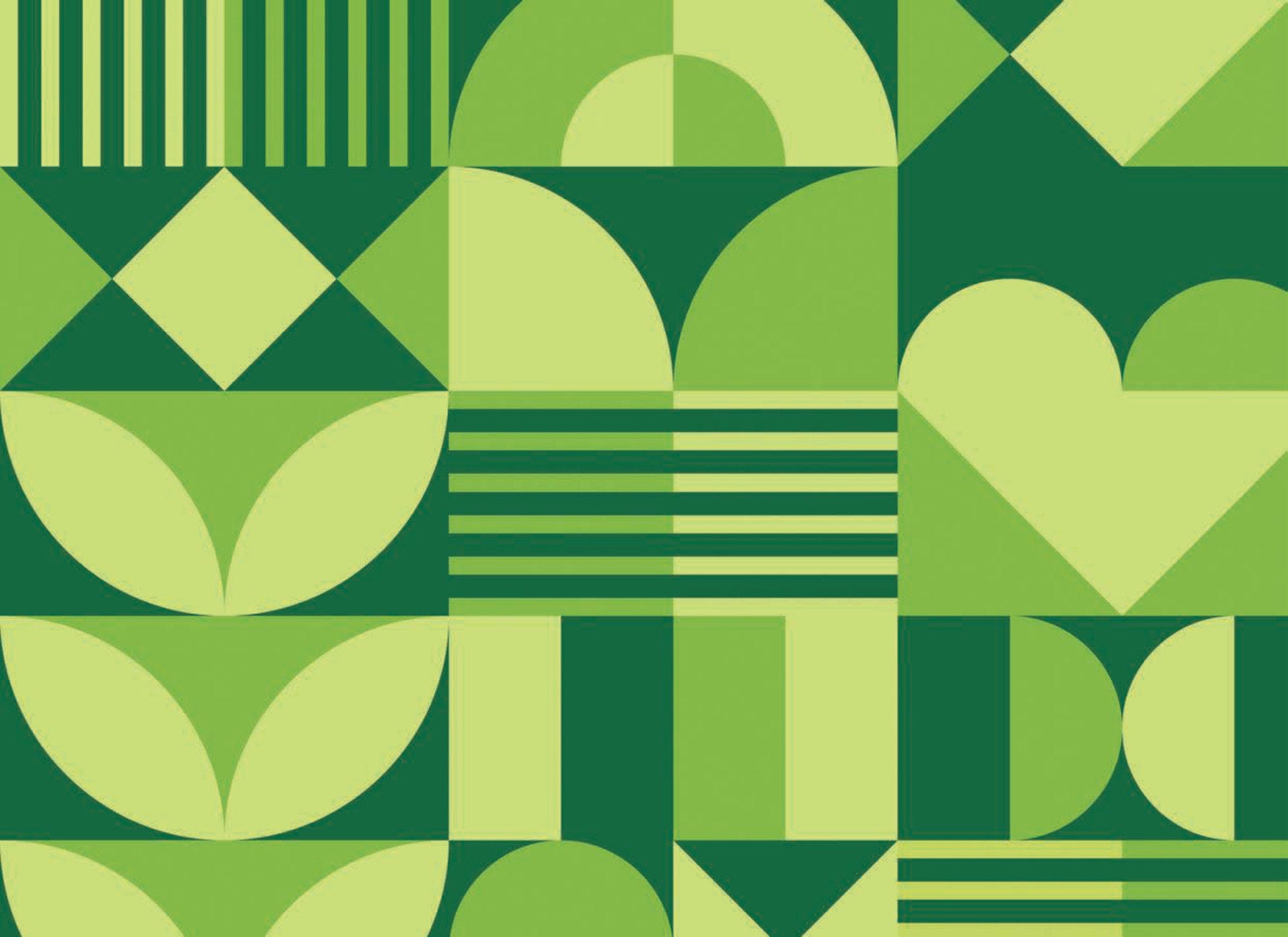
4. Choice experiment survey
Abstract
4.1. Eliciting farmers’ preferences on agri-environmental payment schemes to increase their participation
Tackling the environmental challenges associated with agriculture requires improvements in the current set of instruments available to policy makers. One such instrument, agri-environmental payment schemes, are voluntary programmes that pay farmers for the adoption of environmental practices. Despite their popularity, there is mounting evidence that many these schemes underperform against their environmental objectives.
Voluntary agri‐environmental contracts that offer fixed payment rates suffer from two major problems. First, farmers who face the lowest costs of compliance with environmental requirements are more likely to enter the programme; in cases where the programme pays some farmers for doing nothing differently from what they would have done in the absence of payment, adverse selection may induce large windfall effects (Chabé-Ferret and Subervie (2013[1]). Second, those farmers with the highest costs of compliance are less likely to enter the programme, although they may be precisely those whose engagement would make the greatest contribution to the programme’s effectiveness (Kuhfuss et al., 2015[2]). Despite these well-known shortcomings, there is limited evidence on how payment design features could be changed to improve the targeting of beneficiaries and including the best mix of practices or results upon which payments should be conditioned.
Since agri-environmental payment mechanisms rely on the voluntary participation of farmers to achieve their environmental outcomes, it is important to understand how and why farmers choose to participate in these mechanisms. This is particularly the case when considering the introduction of new agri-environmental policy mechanisms. As noted in Part I, policy makers have expressed an interest in exploring the potential of performance-based or results-based payment schemes to improve the cost-effectiveness of agri-environmental payment schemes. Gauging the preference of farmers for specific payment designs can help anticipate their possible adoption and effects.
The purpose of Part II is to offer such evidence, and explore how different types of farmers in selected countries respond to specific agri-environmental payment designs: practice-based schemes, where payments are based on adoption of specific practices; results-based payments,1 which link payments to measurable results; and hybrid payments, under which payment depends on both implementing specific practices and achieving specific results. The report is based on a choice experiment conducted with farmers, using a survey instrument that can be adapted to other country and policy contexts.
Choice experiments belong to the set of ex ante methods and have been applied to numerous issues in a variety of research fields (Holmes, Adamowicz and Carlsson, 2017[3]).2 They are stated preferences methods (surveys) that aim to reveal information about individual (in this case, farmers) choices and preferences, and are grounded in consumer and microeconomic theory (Hanley, Mourato and Wright, 2001[4]). Essentially, choice experiments consist of presenting individuals with a range of choices, and asking the individuals to trade-off different levels of attributes of interest.3
This method has been increasingly used in research. A review of the literature found 55 choice experiment studies focusing on agriculture (Annex 4.C). Most of the studies tested farmers’ willingness to accept contracts that pay for implementing specific practices. The literature is scant on analysis of willingness to accept contracts that pay for achieving results. The literature reviewed for this report found only one pure results-based contract and a few hybrid schemes that usually had no straightforward linkages between payment levels and the performance attribute.
The choice experiment presented in this part of the report studies policy design elements for multiple environmental objectives. While different design features can be tested in policy simulations (as undertaken in the first part of the report), this approach cannot account for certain real-world aspects, including behavioural characteristics of farmers, which are generally difficult to model. The experiment provides valuable evidence which, together with the simulations, yields richer insights into practical design considerations for agri-environmental payment schemes. The proposed choice experiment aimed to determine how farmer and farm characteristics (e.g. gender, age, farm size) and behavioural aspects (e.g. risk aversion, attitudes towards the environment) impacted the choice of different agri-environmental contracts and determine farmer’s willingness to accept a given contract.
The choice experiment was implemented in three volunteer countries: Finland, the Netherlands and Sweden, but the survey instrument developed for this project could be adapted and applied to other countries interested in the design of practice-based, hybrid and results-based agri-environmental payments.
In April 2020, Argentina and Finland conducted focus groups to obtain feedback from experts and farmers on the first version of the survey. The Netherlands conducted focus groups in June 2020. In light of this feedback and interactions with country experts, the OECD drafted a pilot version of the survey. The survey was originally drafted in English and was translated by participating countries into Dutch, Finnish, Spanish and Swedish and coded in LimeSurvey by a contracted expert.
In January 2021, the pilot survey was completed in Argentina, Canada, Finland and Sweden. Based on the responses from the pilot survey, the survey was adjusted and implemented in March 2021 in the Netherlands, Sweden and Finland. Each country drew on a representative sample of farmers and used incentives to increase participation in the survey. Canada and Argentina were ultimately unable to participate in the final survey due to logistical complications.
Following standard choice experiment design, the survey has four sections, i) introduction, ii) contextual questions, iii) choice exercise, and iv) behavioural questions. There are two main versions of the survey, one for arable farming (general field cropping of cereals, oilseed and protein crops) and one for mixed farming (grazing bovines or sheep and crop production) to represent the two categories of farmers that would be targeted by the potential agri-environmental schemes.4
4.2. Introduction to the survey
The introduction explains the purpose of the survey, presents general instructions on how to respond to the survey, and the use of data collected, and also includes a point of contact to clarify questions about the survey and a consent form. The introduction highlights that the answers to the survey will directly contribute to informing the design of agri-environmental payment schemes in the future.
4.2.1. Contextual questions
This section contains questions about the participant, farm characteristics and experience with agri-environmental schemes. Data gained from these questions are primarily used i) to assess the representativeness of the sample, ii) to select the survey type (arable or mixed), and iii) to serve as explanatory variables for explaining variation seen in the choice exercise.
4.2.2. Choice exercise
This section is the core of the survey. It starts with a description of the hypothetical agri-environmental policy schemes, includes instructions on how to complete the choice exercise and contains the choice exercise itself (more detail below). Since the main objective of the choice exercise is to elicit farmers’ preferences for practice-based, results-based and hybrid agri-environmental contracts for achieving water quality, climate change mitigation and biodiversity objectives, the hypothetical agri-environmental policy schemes sub-section explains how the different hypothetical contracts work, including how they would be monitored and enforced.
Schemes based on the adoption of specific practices offer an annual payment for five years and pay for the adoption of some or all of the following.
Both arable and mixed
A reduction of nitrogen fertiliser application (including mineral and manure sources) by 20% on all cultivated fields – compared to average use over the three years preceding enrolment.
The establishment of three-meter-wide buffer strips along all main ditches and water courses in all cultivated fields.
The establishment of cover (or catch) crops on 10% of cultivated fields.
The reduction in pesticide use by 20% in all cultivated fields – compared to average use over three years preceding enrolment
Only arable
The establishment of green fallow on 10% of arable farm acreage.
The establishment and management of enlarged (2-meter-wide) field edges (e.g. margins, stone walls or hedged) on at least 30% of cultivated fields.
Only mixed
The application of all manure by injection instead of broadcasting (whole farm).
The management of permanent grassland to favour biodiversity, by imposing a stocking rate between xxx (to be defined by countries) and yyy (to be defined by countries) livestock units per hectare and ensuring the removal of all brushwood.
Environmental results-based schemes offer an annual payment for five years if the following results are achieved.
Climate change mitigation. Reduction (no requirement, 25% or 50%) of greenhouse gases net emissions relative to the estimated net emissions before the start of the contract, estimated from the use of nitrogen fertilisers (mineral and manure) and fuel, and cultivation practices (ploughing, cover crops, etc.) that impact the amount of carbon stored in soils.
Biodiversity. Increase in the number of flowering and vascular plant species (no requirement, 5 flowering plants species, 10 flowering plants species) present on 10% of arable land (for arable version) / on permanent grasslands (for mixed version).
Water quality. Reduction (no requirement, 25% or 50%) of the run-off of nutrients, pesticides and sediments at the edge of field, estimated based on the slope, soil type, and recorded cultivation practices (cover crops, buffer strips, fertiliser use, etc.), relative to the estimated runoff before the start of the contract.
The survey states that under environmental results-based schemes, farmers are free to adopt any practices that they consider most suitable to achieve those results. Note that the biodiversity objective is purely based on measured results, while the water quality and climate change mitigation objectives are based on modelled/simulated results.
Hybrid schemes offer two annual payments for five years; one for the adoption of specific practices and an additional payment contingent on the achievement of environmental results. In this scheme, the menu of environmental results comes from only results-based schemes and the menu of practices comes from only practice-based schemes.
The survey also notes that, under all schemes, participants would be asked to report on the adoption of practices and/or achievement of environmental results at the end of each year; that all reports would be reviewed each year; and that 5% of farms would be selected for field controls.
Figure 4.1 shows an example of the choice cards included in the survey; for each choice card, responding farmers have to choose one option among three, considering a range of requirements and the resulting payment. A total of 36 choice cards were generated as the minimum number needed to generate efficient (low variance) parameter estimates. The cards were organised in six blocks (six blocks each containing six choice cards) and each farmer was presented with only one block (six choice cards).
Figure 4.1. Example of choice card
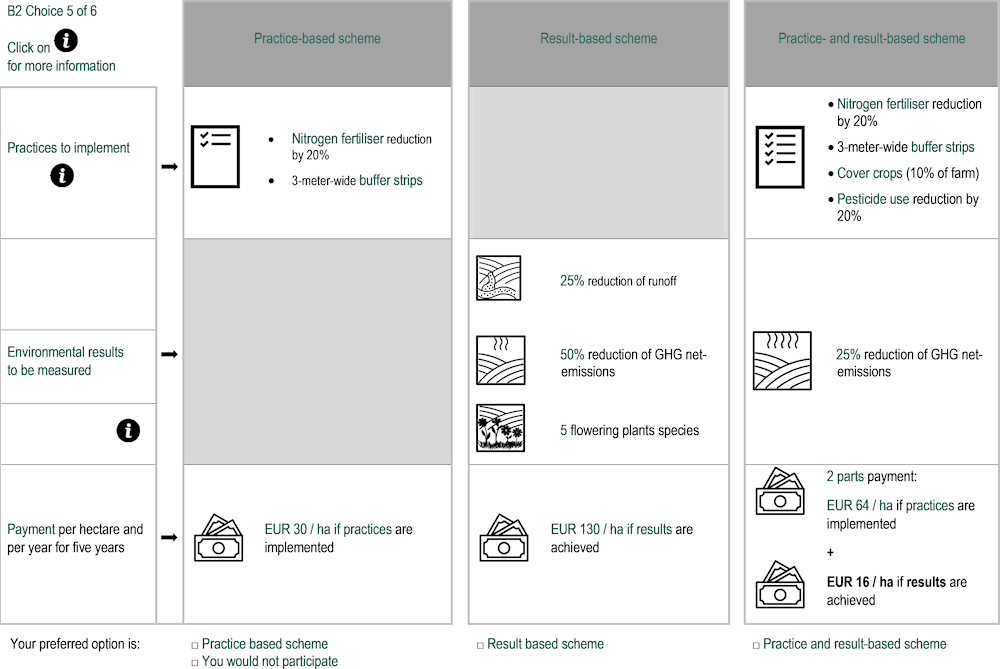
4.2.3. Attitudinal or behavioural questions
This section includes questions about participant attitudes or behaviours that are relevant to the choice exercise, such as their views on the importance of improving agricultural sustainability, attitudes towards participating in policy measures, and beliefs about the value or likelihood of co-ordinated actions, etc. As shown in the literature review, behavioural aspects such as dispositional factors (personality, risk tolerance and environmental concerns), social factors (social norms and expectations) and cognitive factors (ability to understand costs and benefits of their actions and the way programmes work) are important for farmers’ willingness to enrol in agri-environmental schemes. These questions typically appear after the choice exercise, so that responses to the choice exercise are not affected by these questions. The final survey includes twelve behavioural questions.
The related behavioural factors identified in the literature review that are relevant for understanding farmers’ motivations to enrol and participate into agri-environmental schemes include:
Questions to identify unreliable responses / protest answers (Cognitive)
Value belief norm model. This model is used for predicting behaviour and can be broken down into sub-factors that affect intentions: social norms, attitudes, values, ecological worldview, awareness of consequences and ascription of responsibility (Dispositional and Social)
Perceived efficacy. Perceptions of the environmental efficacy of adopting best management practices (Cognitive)
Theory of planned behaviour. Theory used for predicting behavioural intentions; it can be broken down into three factors: social norms, attitudes, and perceived behavioural control (Dispositional and Social)
Risk attitudes and risk perception (Dispositional)
Time preferences (Dispositional and Social)
Shifting baseline theory. Perceptions of environmental change, perceived need for action (Dispositional and Social)
Trust and uncertainty (Dispositional and Social).
4.3. Results and conclusions
4.3.1. Sample and farmer characteristics
The sample consisted of 731 complete submitted answers to the questionnaire (Table 4.1), including 270 responses for the mixed farming version of the questionnaire and 461 for the arable version of the questionnaire. In terms of participation per country, 357 farmers completed the questionnaire in Finland, 230 in Sweden and 144 in the Netherlands.
Table 4.1. Respondents
Specialisation |
Finland |
Sweden |
Netherlands |
Total |
---|---|---|---|---|
Mixed farming |
90 |
158 |
22 |
270 |
Specialised in crop production |
267 |
72 |
122 |
461 |
Total |
357 |
230 |
144 |
731 |
4.3.2. Respondents’ socio-demographic and farm characteristics
The average farm size within the sample was 160 hectares, including 70.8 hectares of arable land. On average, farmers owned 75 hectares of land; 74.4% of respondents had another source of income than the farm revenue for their household, and the farm income for those who had another source of income represented 36.7% of household income on average.
The sample was largely composed of males (89.7%) over 55 years old (49.9%). Most respondents had specialised education in agriculture, from a high school diploma (14.9%) to a university degree in agriculture (12.7%), as well as vocational training in agriculture (24.1%). 58.2% were full-time farmers, while 36.7% were part-time farmers (see Annex Table 4.D.2 and Annex Table 4.D.3 for more details on farmer characteristics).
4.3.3. Respondents’ behavioural characteristics
Farmers in the sample varied in their tolerance for risk (from risk averse to farmers who were more willing to take risks), but the majority tended to make choices now to influence outcomes farther into the future. Generally, farmers’ responses regarding their willingness to adopt new farming practices and willingness to take risks were evenly distributed, from strongly disagree to strongly agree. In contrast, only a minority of farmers displayed preferences for the present, while most tended to be more long-term oriented in their decision making. Roughly 70% of farmers stated that they made farming decisions to achieve outcomes that may not result for many years Annex Figure 4.C.1 presents these results in more detail).
With regards to farmers’ attitudes towards the environment, most farmers in the sample were satisfied with the environment around their farms and acknowledged the threat of global warming, but disagreed about the degree to which they felt responsible for either local or global environmental issues such as global warming. While most respondents believed that farmers in their area were willing to adopt (or had already adopted) farming practices to improve environmental outcomes, as an indicator of the social norm, about 30% were agnostic about whether other farmers would be willing to adopt such practices (Annex Figure 4.C.2 details on attitudes towards environmental issues.)
Finally, about half of the respondents had low levels of confidence in the ability to monitor the environmental improvements that would be achieved through the schemes proposed. There were large variations in respondents’ perceptions of their ability to adopt the proposed practices and their ability to achieve the environmental results, as well as the potential effects of these on farm profitability. Most farmers would not adopt the proposed practices or work to achieve the environmental objectives presented in the questionnaire in the absence of any payment, and most were in favour of more government spending and agri-environmental payments. A majority of farmers found the survey complex and mentioned having to ignore some of the options’ characteristics in order to be able to make choices on the cards.5 Annex Figure 4.C.3 provides more detail on farmers’ perception of the choice experiment.
4.3.4. Contract characteristics
As explained in Section 2, respondents were presented six choice cards with three contract options: practice-based, results-based and hybrid. In each of these choice cards they had to choose between one contract and the option of not participating in any of the offered schemes. The contracts varied by the nature of the attributes and levels of the particular attributes (Table 4.2).
In the following analysis, farmers’ choices are explained first by the scheme characteristics, and then by individuals’ characteristics, including behavioural factors.
Table 4.2. Attributes and their levels presented on choice cards
Attributes and constants |
Levels and associated variables |
---|---|
Alternative specific constants (ASC): Constants capturing the baseline preference for alternative scheme designs |
ASC No participation: constant for the alternative not to participate (reference) ASC Practice-based: constant for practice-based alternatives ASC Hybrid: constant for hybrid alternatives ASC Results-based: constant for results-based alternatives |
A : Practice-based requirements from list below |
Level 1: First 2 practices to be implemented (reference level) Level 2: First 4 practices to be implemented Level 3: All 3 practices to be implemented |
B: Water quality objectives: reduction of nutrient, pesticide and sediment runoff (measured at the edge of field) |
Level 0: Payment will not depend on water quality results (reference level) Level 1: 25% reduction of runoff Level 2: 50% reduction of runoff |
C: Climate Change (CC) mitigation: Reduction of GHG net-emissions from farm (GHG emissions minus soils carbon sequestration) |
Level 0: Payment will not depend on climate change mitigation results (reference level) Level 1: 25% reduction of GHG net-emissions Level 2: 50% reduction of GHG net-emissions |
D: Biodiversity objectives: Number of specified flowering plant species present on 10% of the farm acreage |
Level 0: Payment will not depend on biodiversity results (reference level) Level 1: 5 flowering plant species Level 2: 10 flowering plant species |
E: Share of payment conditioned on results |
0 % (in practice-based alternatives only) 10, 20, 30, 50, 70, 90 % (in hybrid alternatives only) 100 % (in results-based alternatives only) |
F: Payment in euros per hectare and per year |
0: In the option “I would not participate” 30, 80, 130, 180, 230, 280 |
4.3.5. Farmers’ preferences for AES designs
Overall preferences
Roughly one-third of farmers preferred not to participate in any of the schemes based on the characteristics presented. Farmers selected practice-based schemes more often than hybrid and results-based schemes (Table 4.3).
Table 4.3. Share of choices for each alternative scheme design on the choice cards, overall, per farm specialisation and per country, percentage
% |
All |
Arable farmers |
Mixed farmers |
Finland |
Sweden |
Netherlands |
---|---|---|---|---|---|---|
Practice- based |
26.93 |
26.68 |
27.35 |
30.63 |
25.36 |
20.25 |
Hybrid |
20.59 |
19.67 |
22.16 |
22.88 |
21.45 |
13.54 |
Results-based |
16.39 |
15.4 |
18.09 |
16.15 |
18.91 |
12.96 |
Would not participate |
36.09 |
38.25 |
32.41 |
30.35 |
34.28 |
53.24 |
A conditional logit model was conducted to understand which attributes of the schemes had the most weight in these choices.6 The model estimates provide information on the importance of the attributes, and their levels, in farmers’ decision making. Table 4.4 presents the marginal Willingness-to-accept (WTA) of farmers or the monetary amount that farmers would need to receive in order to accept the corresponding attribute, for each of the contract characteristics. Higher values of WTA indicate that farmers have a stronger aversion to that attribute.
Table 4.4. Overall farmer preferences for AES designs: Results from the conditional logit model and associated estimates of farmers’ willingness to accept
Conditional Logit Model |
WTA estimates |
|||||
---|---|---|---|---|---|---|
Estimate |
Robust standard errors1 |
Estimate (EUR) |
Robust standard errors |
|||
ASC practice-based |
-1.097 |
*** |
0.095 |
182.2 |
*** |
14.4 |
ASC hybrid |
-0.861 |
*** |
0.129 |
142.9 |
*** |
21.7 |
ASC results-based |
-1.483 |
*** |
0.113 |
246.2 |
*** |
19.1 |
Practices level 2 (ref 1) |
-0.187 |
*** |
0.064 |
31.1 |
*** |
10.4 |
Practices level 3 (ref 1) |
-0.313 |
*** |
0.067 |
52.0 |
*** |
10.9 |
Water level 1 (ref 0) |
-0.271 |
*** |
0.084 |
45.0 |
*** |
13.4 |
Water level 2 (ref 0) |
-0.333 |
*** |
0.058 |
55.4 |
*** |
9.2 |
CC level 1 (ref 0) |
-0.247 |
*** |
0.063 |
41.0 |
*** |
10.2 |
CC level 2 (ref 0) |
-0.286 |
*** |
0.070 |
47.6 |
*** |
11.4 |
Biodiversity 1 (ref 0) |
-0.029 |
0.064 |
4.9 |
10.7 |
||
Biodiversity 2 (ref 0) |
-0.053 |
0.070 |
8.8 |
11.5 |
||
Share conditioned on result (%) |
-0.003 |
** |
0.001 |
0.4 |
** |
0.2 |
Payment level (€/ha/year) |
0.006 |
*** |
0.000 |
|||
Number of respondents |
731 |
|||||
Number of observations |
4386 |
|||||
AIC |
11 326.35 |
|||||
BIC |
11 409.37 |
|||||
LL |
-5 650.18 |
1. Robust Standard Errors computed using the sandwich estimator (R, Apollo package).
*: robust p-value < 0.10; **: robust p-value < 0.05, ***: robust p-value <0.01.
The three model constants, ASC practice-based, ASC hybrid and ASC results-based capture respectively farmers’ preferences for joining a practice-based scheme with a requirement to adopt the first two practices of the menu of options, for joining a hypothetical hybrid scheme including only a requirement to adopt the first two practices of the menu of options but without accounting for the result requirements, and for joining a results-based scheme, without accounting for the result requirements. The negative signs of the constants reflect the cost of joining such schemes, which are not associated with the other attributes included in the model. These costs also include all costs associated with unobserved preferences of farmers for the three-scheme designs. Note that these constants are not directly comparable. For comparison of farmers’ relative preferences for alternative scheme designs, see Table 4.5, Table 4.6and Table 4.7 which include all dimensions of scheme designs.
Table 4.5. Farmers' estimated Willingness to Accept for practice-based AES
Level of requirements |
Mean WTA (EUR/ha/year) |
---|---|
Level 1: First 2 practices |
182.2 |
Level 2: First 4 practices |
213.3 |
Level 3: All 6 practices |
234.2 |
Table 4.6. Farmers' estimated Willingness to Accept for results-based AES
Level of requirements |
Mean WTA (EUR/ha/year) |
---|---|
Schemes with only 1 environmental objective |
|
Biodiversity only |
246.2 |
Water 1 only |
291.2 |
CC1 only |
287.2 |
Water 2 only |
301.6 |
CC2 only |
293.8 |
Schemes with 2 environmental objectives |
|
Biodiversity and CC1 |
291.2 |
Biodiversity and Water 1 |
287.2 |
Biodiversity and CC2 |
301.6 |
Biodiversity and water 2 |
293.8 |
Schemes with 3 environmental objectives |
|
Biodiversity, water 1 and CC1 |
332.2 |
Biodiversity, water 1 and CC2 |
338.8 |
Biodiversity, water 2 and CC1 |
342.6 |
Biodiversity, water 2 and CC2 |
349.2 |
Table 4.7. Farmers' estimated Willingness to Accept for Hybrid AES
Level of requirement |
Mean WTA (EUR/ha/year) |
|||||
---|---|---|---|---|---|---|
Share of payment conditioned on results |
||||||
10% |
20% |
30% |
50% |
70% |
90% |
|
First 2 practices |
||||||
First 2 practices, biodiversity |
146.9 |
150.9 |
154.9 |
162.9 |
170.9 |
178.9 |
First 2 practices, biodiversity, water 1 |
191.9 |
195.9 |
199.9 |
207.9 |
215.9 |
223.9 |
First 2 practices, biodiversity, water 2 |
202.3 |
206.3 |
210.3 |
218.3 |
226.3 |
234.3 |
First 2 practices, biodiversity, CC 1 |
187.9 |
191.9 |
195.9 |
203.9 |
211.9 |
219.9 |
First 2 practices, biodiversity, CC 2 |
194.5 |
198.5 |
202.5 |
210.5 |
218.5 |
226.5 |
First 2 practices, biodiversity, water 1, CC 1 |
232.9 |
236.9 |
240.9 |
248.9 |
256.9 |
264.9 |
First 2 practices, biodiversity, water 1, CC 2 |
239.5 |
243.5 |
247.5 |
255.5 |
263.5 |
271.5 |
First 2 practices, biodiversity, water 2, CC 1 |
243.3 |
247.3 |
251.3 |
259.3 |
267.3 |
275.3 |
First 2 practices, biodiversity, water 2, CC 2 |
249.9 |
253.9 |
257.9 |
265.9 |
273.9 |
281.9 |
First 4 practices |
||||||
First 2 practices, biodiversity |
178 |
182 |
186 |
194 |
202 |
210 |
First 2 practices, biodiversity, water 1 |
223 |
227 |
231 |
239 |
247 |
255 |
first 2 practices, biodiversity, water 2 |
233.4 |
237.4 |
241.4 |
249.4 |
257.4 |
265.4 |
First 2 practices, biodiversity, CC 1 |
219 |
223 |
227 |
235 |
243 |
251 |
First 2 practices, biodiversity, CC 2 |
225.6 |
229.6 |
233.6 |
241.6 |
249.6 |
257.6 |
First 4 practices, biodiversity, water 1, CC1 |
264 |
268 |
272 |
280 |
288 |
296 |
First 4 practices, biodiversity, water 1, CC 2 |
270.6 |
274.6 |
278.6 |
286.6 |
294.6 |
302.6 |
First 4 practices, biodiversity, water 2, CC 1 |
274.4 |
278.4 |
282.4 |
290.4 |
298.4 |
306.4 |
First 4 practices, biodiversity, water 2, CC2 |
281 |
285 |
289 |
297 |
305 |
313 |
All 6 practices |
||||||
All 6 practices, biodiversity |
198.9 |
202.9 |
206.9 |
214.9 |
222.9 |
230.9 |
All 6 practices, biodiversity, water 1 |
243.9 |
247.9 |
251.9 |
259.9 |
267.9 |
275.9 |
All 6 practices, biodiversity, water 2 |
254.3 |
258.3 |
262.3 |
270.3 |
278.3 |
286.3 |
All 6 practices, biodiversity, CC 1 |
239.9 |
243.9 |
247.9 |
255.9 |
263.9 |
271.9 |
All 6 practices, biodiversity, CC 2 |
246.5 |
250.5 |
254.5 |
262.5 |
270.5 |
278.5 |
All 6 practices, biodiversity, water 1, CC1 |
284.9 |
288.9 |
292.9 |
300.9 |
308.9 |
316.9 |
All 6 practices, biodiversity, water 1, CC 2 |
291.5 |
295.5 |
299.5 |
307.5 |
315.5 |
323.5 |
All 6 practices, biodiversity, water 2, CC 1 |
295.3 |
299.3 |
303.3 |
311.3 |
319.3 |
327.3 |
All 6 practices, biodiversity, water 2, CC2 |
301.9 |
305.9 |
309.9 |
317.9 |
325.9 |
333.9 |
Note: The red line in Table 3.7 indicates the threshold from which practices-based schemes are preferred over hybrid schemes.
Farmers preferred to be asked to implement fewer practices with lower levels of environmental results. For example, in practice-based contracts, requirement for farmers to adopt the first four practices was significantly less likely to be chosen than those requiring adoption of only two practices. Similarly, a contract with a requirement to adopt all six practices was even less likely to be chosen. The additional payment associated with having to adopt the first four practices instead of the first two practices in practice-based and hybrid schemes is estimated at EUR 31/ha to keep participation rates constant, while farmers would require an additional EUR 52/ha to adopt all six practices instead of only the first two.
Participation rates were responsive to increased water quality measures and GHG emissions reductions, but not to biodiversity outcomes. Increasing stringency for reaching water quality outcomes resulted in significantly fewer farmers choosing a results-based or hybrid scheme for a given payment level. A 50% reduction in nutrient runoff had a stronger negative effect on choosing a results-based contract than a requirement for a reduction of 25% in nutrient runoff. Similarly, farmers had negative preferences for stronger climate change (CC) objectives in results-based and hybrid schemes. Together, these two attributes are associated with higher WTA values: that is, for farmers to accept to join an AES (hybrid or results-based) requiring them to reduce their runoff by 25%, rather than a similar contract without water quality related objectives, farmers would require an additional payment of about EUR 45/ha, rising to EUR 55.4 for a 50% reduction. For farmers to commit to a 25% reduction in GHG net-emissions from the farm, relative to a similar contract with no objective related to GHG net-emissions, would require an additional payment of EUR 41/ha, rising to EUR 47.6 for a commitment to reduce emissions by 50%.7 In contrast, objectives related to biodiversity do not appear to be a deterrent to farmers’ participation and choice of an option. That is, farmers’ choice of an AES did not seem to be impacted by the presence of biodiversity results-based objectives.
Finally, the share of the payment based on results had a negative effect on their choice of a hybrid AES: the higher the share dependent upon the achievement of specific results, the less likely farmers were to choose this option. Farmers would require an additional EUR 0.40/ha for a one percentage point increase in the share (e.g. EUR 4/ha for 10% of the payment conditioned on results, EUR 8/ha for 20%, EUR 12/ha for 30% and up to EUR 36 per hectare if 90% if the payment was conditioned on results).8
The total payment for alternative schemes are presented in Table 4.5 for practice-based AES, Table 4.6 for results-based AES, and Table 4.7 for hybrid schemes. The red line in Table 4.7 indicates the threshold from which practices-based schemes are preferred over hybrid schemes.
Results show that hybrid schemes are always preferred to practice-based schemes with the same level of requirement when only biodiversity objectives are included in the hybrid schemes (first rows of the three level of requirement blocks in Table 4.7). This result holds despite the fact that such hybrid payment schemes include practice-based requirements based on biodiversity objectives and a share of payment conditioned on results. However, as soon as water quality protection and climate change mitigation objectives are added to the hybrid scheme requirements, pure practice-based schemes are preferred to hybrid schemes with the same requirements in terms of practices to be implemented.
We also note that hybrid schemes are always preferred to results-based schemes with the same environmental objectives.
Heterogeneity by country and farm specialisation
In terms of cross-country and cross-specialisation comparisons, the relatively small sample size means that the results should be interpreted with caution. Nevertheless, the relative preferences of farmers for AES designs were similar between the countries studied, and consistent with the overall analysis presented above Annex Table 4.D.1 and Annex Table 4.D.3. While farmers in the Netherlands, and in Sweden to a lesser extent, displayed higher WTA values than Finnish farmers, the sample sizes in the Netherlands and Sweden were small.
The share of payments conditioned on results mattered for mixed farmers, while arable farmers did not appear to be significantly deterred by the share of results-based payment in their choice of AES. Similarly, having to adopt four practices of the list of practices, instead of only the first two, did not appear to have a significant effect on arable farmers’ choices, whereas it did have a significant negative effect for mixed farmers. For example, arable farmers were not deterred by having to establish cover (or catch) crops on 10% of their arable land and having to reduce their pesticide use by 20% while mixed farmers were.
The results (Table 4.8) also show that arable farmers tended to require higher payment levels than mixed farmers to participate in equivalent AES, but the additional payments associated with higher levels of requirements were higher for mixed farmers than for arable farmers (with the exception of the second level of water quality objectives).
Table 4.8. Willingness to accept estimates per farm specialisation
WTA estimates (Delta Method) |
Pooled |
Arable |
Mixed farming |
||||||
---|---|---|---|---|---|---|---|---|---|
Based on Conditional Logit |
Estimate (EUR) |
Robust Standard Errors |
Estimate (EUR) |
Robust Standard Errors |
Estimate (EUR) |
Robust Standard Errors |
|||
ASC practice-based |
182.2 |
*** |
14.4 |
200.6 |
*** |
18.24 |
149.9 |
*** |
23.17 |
ASC hybrid |
142.9 |
*** |
21.7 |
166.6 |
*** |
27.79 |
99.4 |
*** |
34.73 |
ASC results-based |
246.2 |
*** |
19.1 |
258.3 |
*** |
24.75 |
221.4 |
*** |
29.9 |
Practices 2 (ref 1) |
31.1 |
*** |
10.4 |
18.9 |
13.36 |
50.2 |
*** |
16.33 |
|
Practices 3 (ref 1) |
52.0 |
*** |
10.9 |
43.6 |
*** |
13.4 |
65.5 |
*** |
18.66 |
Water 1 (ref 0) |
45.0 |
*** |
13.4 |
36.8 |
** |
16.79 |
59.3 |
*** |
22.37 |
Water 2 (ref 0) |
55.4 |
*** |
9.2 |
74.2 |
*** |
11.9 |
30.9 |
** |
14.56 |
CC 1 (ref 0) |
41.0 |
*** |
10.2 |
52.7 |
*** |
12.84 |
25.4 |
17.09 |
|
CC2 (ref 0) |
47.6 |
*** |
11.4 |
59.4 |
*** |
14.63 |
28.8 |
18.31 |
|
Biodiversity 1 (ref 0) |
4.9 |
10.7 |
9.1 |
13.78 |
0.6 |
17.23 |
|||
Biodiversity 2 (ref 0) |
8.8 |
11.5 |
-4.4 |
13.87 |
29.9 |
19.94 |
|||
Share conditioned on result |
0.4 |
** |
0.2 |
0.2 |
0.2248 |
0.7 |
** |
0.2739 |
Note: *: p-value < 0.10; **: p-value < 0.05, ***: p-value <0.01.
The role of behavioural factors in explaining farmers’ preferences
A latent class analysis was conducted to determine which farmer characteristics explained preferences for contract attributes. First, clusters of farmers were generated to reduce the number of socioeconomic and behavioural characteristics as explanatory variables to class membership. All clusters were independent from each other, meaning that a respondent could belong to cluster 1 in terms of their farm characteristics, while they may belong to cluster 2 or 3 based on their own individual characteristics. The details of the process are explained in Annex 4.E.
The latent class analysis shows that preferences for AES design were strongly associated with behavioural characteristics and environmental preferences, but not with farm and farmers’ characteristics. Based on the clusters, two classes of farmers based on behavioural characteristics explain most of the differences between farmers’ WTA:
Class 1. Includes a majority of respondents (63.35%) who displayed a more positive attitude towards AESs, as shown by the positive sign of the constants for the three designs of AESs. Farmers belonging to class 1 displayed the expected preferences for all scheme characteristics: the higher the requirements in terms of practices and results, the less likely they were to join, and the higher the share of payment conditioned upon results, the less likely they were to choose hybrid AES. These farmers tended to give relatively more value to the longer-term consequences of their actions; to perceive a need for environmental change; and to be more aware of the effects of farming on the environment, while also feeling more responsible for environmental issues than farmers belonging to class 2. This result aligns well with the Theory of Planned Behaviour and the Value-Belief-Norm models, in which awareness of consequences, ascription of responsibility and perceived behavioural control are described as key factors shaping intentions of behavioural change. Finnish farmers were more likely than farmers from the Netherlands to belong to this class. Farmers who reported more positive feedback to the survey were also more likely to belong to class 1. They were also on average more confident in their ability to adopt the practices or achieve the results required by the proposed schemes.
Class 2. Farmers that were more risk averse (behavioural cluster 1) were more likely to belong to class 2, and therefore to prefer not to join AES. These farmers tended to express a lower perceived need for environmental change; less awareness of the consequences of farming on the environment; and lower levels of ascription of responsibility and behavioural control over environmental quality. This can explain the relatively stronger negative effect of all AES requirements on their choices to join an AES and their overall negative perception of AES, whatever their design. Farmers who found the survey too complex, found that the payments were too low, gave lower levels of credibility to the survey and provided less consistent answers were more likely to belong to this class. These farmers also stated being less confident in their ability to adopt the practices or to achieve the objectives set in the proposed AESs, while fearing that these would put their farm profitability at risk. Finally, they also tended to have lower levels of trust in the monitoring of the proposed AESs. Class 2 of farmers comprised 36.7% of the sample. They were likely to increase the average WTA in the conditional logit analysis.
Table 4.9 presents WTA estimates for these two classes of farmers, based on their individual characteristics (age, education, status), behavioural characteristics (risk, time, and environmental attitudes), and their responses to follow-up questions.9
Farmers belonging to class 2 showed strong negative preferences towards all three AES designs, while farmers belonging to class 1 preferred to adopt any AES rather than not participating, as demonstrated by the significant and positive values of the three Alternative Specific Constants (ASC). The relative preferences between the three designs, practice-based, results-based or hybrid schemes, everything else constant, remained the same however, with both classes preferring hybrid schemes over practice-based schemes and over results-based schemes.
Table 4.9. WTA estimates for farmers’ behavioural classes
Based on Conditional Logit |
Estimate (EUR) |
Robust standard errors |
Estimate (EUR) |
Robust standard errors |
||
---|---|---|---|---|---|---|
ASC practice-based |
-130.3 |
*** |
34.69 |
383.2 |
*** |
43.79 |
ASC hybrid |
-174.8 |
*** |
37.2 |
343 |
*** |
72.49 |
ASC results-based |
-60.1 |
|
32.0 |
377.7 |
*** |
53.02 |
Practices 2 (ref 1) |
35.6 |
*** |
11.0 |
113.9 |
*** |
33.34 |
Practices 3 (ref 1) |
53.7 |
*** |
11.6 |
185.9 |
*** |
39.7 |
Water 1 (ref 0) |
45.5 |
*** |
13.8 |
69.17 |
|
72.53 |
Water 2 (ref 0) |
41.4 |
*** |
9.4 |
98.05 |
*** |
28.35 |
CC 1 (ref 0) |
29.3 |
** |
10.4 |
98.04 |
*** |
29.75 |
CC2 (ref 0) |
41.0 |
*** |
11.7 |
98.51 |
*** |
30.85 |
Biodiversity 1 (ref 0) |
12.6 |
|
10.7 |
-4.421 |
28.34 |
|
Biodiversity 2 (ref 0) |
17.4 |
|
13.2 |
23.59 |
31.69 |
|
Share conditioned on result |
0.4 |
** |
0.2 |
1.084 |
|
1.161 |
Note: *: p-value < 0.10; **: p-value < 0.05, ***: p-value <0.01.
4.4. Conclusions
In contrast to the cost-effectiveness analysis performed based on policy simulations, the choice experiment shows that farmers always tended to prefer hybrid contracts (payment depends on both implementing practices and achieving results), or practice-based schemes over results-based contracts. The references between practice-based and hybrid schemes depended on the level of results-based requirement included in the hybrid schemes. For low levels of results-based objectives (biodiversity only), hybrid schemes were preferred over practice-based schemes including the same practice-based requirements. When introducing requirements to reduce nutrient runoff to improve water quality and/or requirement to reduce net GHG emissions to mitigate climate change in hybrid schemes, practices-based schemes were always preferred over hybrid schemes including the same practice-based requirements. These preferences were stable across farm type (arable and mixed) and farmer behavioural characteristics.
The results from the conditional logit estimation reveal several notable findings. First, farmers preferred less complicated to more complicated contracts. The average willingness to accept contracts with a low level of environmental requirements for practice-based schemes (i.e. adopting two practices) is EUR 182/ha/year. The average willingness to accept for adopting a hybrid scheme with the lowest level of environmental requirements (adopting two practices and achieving biodiversity objectives) varies from EUR 147/ha/year if 10% of the payment in conditioned upon results being achieved up to EUR 179/ha/year if 90% of the payment is conditioned upon results. In the case of results-based schemes, the average amount farmers would require to adopt a contract with the lowest level of environmental requirements (i.e. biodiversity objectives only) is EUR 246/ha/year. Those estimates increased with the level of environmental requirements. For example, in the case of practice-based schemes, relative to the option with two practices, the payment level would need to increase by 17% for contracts requiring the adoption of four practices and by 28% for practice-based contracts requiring the adoption of six practices.
Second, as expected, stricter environmental requirements would require higher payments to incentivise farmers to join the scheme. For example, in the case of hybrid payments and relative to “First 2 practices, Biodiversity and 10% share of payment conditioned on the results”, a requirement to reduce nutrient runoff by 25% or 50% would require an increase in payment by EUR 45/ha (31%) or an increase in payment by EUR 55.4/ha (38%), respectively. The additional payment needed to convince farmers to sign up into contracts that required farmers to reduce net GHG emissions by 25% or 50% are EUR 41/ha and EUR 48/ha, respectively.
Third, for hybrid contracts, which combine elements of both the results-based and practice-based schemes, the greater the share of the payment conditioned on achieving results, the higher the payment required by farmers. Farmers require an additional payment of EUR 0.4/ha for each percentage increase in the payment share based on achieving results (e.g. EUR 4/ha for 10% of the payment conditioned on results, EUR 8/ha for 20%, EUR 12/ha for 30% and up to EUR 36/ha if 90% of the payment is conditioned on results).
Fourth, arable farmers tended to show greater willingness to accept levels in order to participate in AES contracts, regardless of contract type (hybrid, results-based or practice-based). For hybrid contracts, the offered payment (EUR 167/ha) needed to be 68% higher for arable farms than the payment needed for mixed farmers to participate (EUR 99/ha).
Finally, one advantage of choice experiments is that they can identify the role that behavioural factors play in farmers’ willingness to accept AES contracts. Using a latent class model estimation, we show that farmers that were more risk averse, who expressed a lower need for environmental change, and were less aware of the consequences of farming on the environment tended to have higher WTA. Strikingly, payments needed to be twice as large as the average payment for those farmers to participate in AES contracts. This premium is consistent with previous work that argues that results-based schemes expose farmers to a higher uncertainty of the amount of payment and therefore necessitate a risk premium. An analysis of the potential effectiveness of a Payment-by-Results approach to the delivery of environmental public goods and services supplied by Agri-Environment Schemes (Schwartz, 2008[5]; Andeltová, 2018[6]; Zabel and Roe, 2009[7]), and that concern for the environmental is likely to positively affect enrolment in agri-environmental schemes (Toma and Mathijs, 2007[8]; Best, 2010[9]; Läpple and Rensburg, 2011[10]);
References
[6] Andeltová (2018), Andeltová (2018), Action-based and outcome-based payments for environmental services : An experimental auction for tree planting contracts in Kenya..
[9] Best, H. (2010), “Environmental concern and the adoption of organic agriculture”, Society and Natural Resources, https://doi.org/10.1080/08941920802178206.
[1] Chabé-Ferret, S. and J. Subervie (2013), “How much green for the buck? Estimating additional and windfall effects of French agro-environmental schemes by DID-matching”, Journal of Environmental Economics and Management, Vol. 65/1, pp. 12-27.
[13] Daly, A., S. Hess and G. de Jong (2012), “Calculating errors for measures derived from choice modelling estimates”, Transportation Research Part B, Vol. 46, pp. 333-341.
[4] Hanley, N., S. Mourato and R. Wright (2001), “Choice modelling approaches: a superior alternative for environmental valuation?”, Journal of Economic Surveys, Vol. 15/3, pp. 435-462, https://onlinelibrary.wiley.com/doi/pdf/10.1111/1467-6419.00145 (accessed on 14 May 2019).
[12] Hess, S. and D. Palma (2019), “Apollo: a flexible, powerful and customisable freeware package for choice model estimation and application”, Journal of Choice Modelling, Vol. 32/September 2019.
[3] Holmes, T., W. Adamowicz and F. Carlsson (2017), “Choice Experiments”, in A Primer on Nonmarket Valuation, The Economics of Non-Market Goods and Resources, Springer Netherlands, Dordrecht, https://doi.org/10.1007/978-94-007-7104-8_5.
[2] Kuhfuss, L. et al. (2015), “Nudging farmers to enrol land into agri-environmental schemes: the role of a collective bonus”, European Review of Agricultural Economics, Vol. 43/4, pp. 609-636, https://doi.org/10.1093/erae/jbv031.
[10] Läpple, D. and V. Rensburg (2011), “Adoption of organic farming: Are there differences between early and late adoption?”, Ecological Economics, Vol. 70/7.
[14] Makles, A. (2012), “STATA Tip 110: How to get the optimal k-means cluster solution”, The STATA Journal, Vol. 12/2, pp. 347-351.
[5] Schwartz, G. (2008), An analysis of the potential effectiveness of a payment-by-results approach to the delivery of environmental public goods and services supplied by Agri-Environment Schemes.
[8] Toma and Mathijs (2007), “Environmental risk perception, environmental concern and propensity to participate in organic farming programmes”, Journal of Environmental Management.
[7] Zabel, A. and B. Roe (2009), “Optimal design of pro-conservation incentives”, Ecological Economics, Vol. 69/1, pp. 126-134.
[11] Zarembka, P. (ed.) (1974), Conditional Logit Analysis of Qualitative Choice Behaviour, Academic Press, New York.
Annex 4.A. Summary of focus groups and pilot survey
Focus groups
Focus groups are meetings organised by a chair, usually someone co-ordinating the survey, to obtain feedback on the survey from participants from the pool of potential survey respondents. They are particularly helpful to gain information on how to improve surveys along a number of dimensions. One is to improve the consequentiality of the survey (i.e. the survey conveys that respondents’ answers have real consequences influencing policy design). Focus groups help to determine the best language to be used to convey to respondents that their answers have real consequence. The second is to improve the comprehension of the experiment by participants (i.e. do supported activities make sense? Are choices credible and achievable? Are payments too high or low?). Clearly explaining the process of the experiment itself is an essential component for farmers to understand how to make choices about their preferred options. Finally, the third is to improve the information provided about the potential agri-environmental schemes so that farmers make informed choices about the potential options.
Ideally, focus groups should be held physically to facilitate the interaction among participants. Due to the effects of the COVID-19 pandemic, in Argentina, Finland and the Netherlands, the focus groups were organised online and were successful at delivering feedback.
In Finland, five farmers specialised in different crops and with different farm sizes, participated in the focus group. In Argentina there were six farmers, some of which were tenants and others were landowners and also with different specialisations. In the Netherlands, five farmers that specialised in mixed farming (both crops and livestock) participated in the focus groups.
The focus groups provided essential feedback about the comprehension of the survey, the information provided about the practices and the design of the choice cards. Notably, farmers were particularly concerned with how some of the instructions and measurement of the practices were communicated. As a result, the description of the choice experiment and of the individual practices were revised to take into account the concerns which significantly improved comprehension. Further, the way that the schemes and certain practices were described was changed significantly as farmers perceived that the questionnaire was too normative and in favour of results-based schemes.
Pilot survey
A pilot survey based on the current design of the questionnaire and choice cards was launched in the beginning of September 2020 and completed in January 2021. Argentina, Canada, Finland, and Sweden participated in the pilot survey. The pilot survey served both to test the overall questionnaire design and to calibrate the payments and parameters for the choice card experiment.
In general, conducting pilots is instrumental in the efficient design of choice experiments which provide more precise model estimates (even with relatively small sample sizes). To generate an efficient design, prior values are needed for the model parameters (the weights of each attribute in respondents’ choices). Pilots are the best instruments for obtaining reliable prior values. The pilot also permits to the test the full questionnaire with farmers.
In collaboration with the partnering institution in each participating country, a translated version of the survey was input into LimeSurvey in which they sent out the link to the survey along with an explanation about the process, an explanation of anonymity, the goals of the survey and a suggested time in which to complete it. In each country, the survey was sent to over 100 farmers with the goal to have at least 20 completed responses of the survey in each country. To have representation of both crop cultivation farmers and those with a mix between crops and livestock, each country except for Argentina that focused on crop farms, sent the survey to an equal number of farmers in each group. All responses were anonymous, and the surveys were stored on a secured OECD server.
The data collection began in November 2020 and continued through January 2021. The data collection took longer than expected due to the relatively low response rate of the survey. While this delayed data collection, it was clear that monetary incentives would be needed to both increase the response rate and the speed of the data collection. As a result, monetary incentives will be included in the launch of the final survey in March 2021.
Overall, the survey was accessed by 1 212 individuals from Sweden, Finland, Argentina, and Canada. Over 76% (n=932) of the respondents did not pass the landing page of the survey, so did not start answering it. To address this issue, the length of the introduction on the first page was reduced and checks were in place to ensure that there are no errors in moving forward past the first page. Of the remaining 280 participants, 88 were screened out through the screening question, showing that the screening is working well, and only mixed farmers and arable farmers were kept in the survey. There was further dropout throughout the survey.
Due to the complexity of the choice cards and the payment schemes, the survey includes comprehension questions. This was another point in which farmers dropped out of the survey (~14 individuals). It is reassuring that a large share of respondents (80%) provided the correct answer to these quiz questions, showing that the explanations provided were clear. Results from the initial analysis suggested that the questions to ensure that farmers understand the structure of payments under the different alternatives are expanded. These will be included in the final survey.
Data collection
Data collection for the choice experiment survey was conducted in partnership with the respective partner organisations in Finland, the Netherlands, and Sweden. The finalised survey instrument was adapted to each country context and was implemented on LimeSurvey. In each country, the survey invitation was sent by email to a large sample of representative farmers. The email informed farmers about the purpose of the survey and the role it may play in policy making. Monetary incentives were used to increase response rates, and they varied by country in the value and type. In Finland and Sweden, farmers were informed that they would be entered into a lottery to win a prize (roughly EUR 50) if they completed the survey. In the Netherlands, farmers each received a small amount for their participation.
Data collection occurred during May and June 2021. We obtained a total of 731 submitted answers to the questionnaire, including 270 responses for the mixed farming version of the questionnaire and 461 for the arable version of the questionnaire. In terms of participation per country, 357 farmers completed the questionnaire in Finland, 230 from Sweden, and 144 from the Netherlands.
Annex 4.B. Methodological framework
General methodological framework for the analysis of discrete choice data
The Conditional Logit Model
Respondents were asked to choose their preferred contract alternative on a series of six choice cards in the survey. We analyse this choice data using first a Multinomial Logit (McFadden, 1974[11]), which is based on the Random Utility Theory. Under this framework, the utility provided by an alternative contract i to individual n, Ui,n, can be decomposed in a deterministic and observable element Vi,n, and a random term, , as follows:
The observable part is a linear function of the characteristics of the contract (its attributes). On each choice card, farmers were presented with 3 different alternative contracts, each having different characteristics. We call Xi the vector of K attributes k of a contract i presented to farmers on a choice card. The observable part of the utility function is therefore defined as:
With the weight of alternative i’s attribute k on utility. These betas are the parameters of interest that we aim to estimate.
In the context of the current choice experiment with labelled alternatives, Vi is a function of different attributes depending on the alternative that is being considered:
In a practices-based alternative, the observed part of the utility is a function of the practices to be implemented (), the level of payment ( and a constant reflecting the general preferences for practices-based schemes that not reflected in the attributes presented on the choice card (, called the Alternative Specific Constant ASC). Therefore, the utility associated with a practice-based alternative is defined as:
In a results-based alternative, the observed part of the utility is a function of the results to be achieved in terms of water quality (), climate change mitigation () and biodiversity (), and the level of payment ( and a constant reflecting the general preferences for results-based schemes that not reflected in the attributes presented on the choice card ()results-based:10
In a hybrid scheme alternative, the observed part of the utility is a function of both the practices to be implemented (), and the results to be achieved in terms of water quality (), climate change mitigation () and biodiversity (), the share of payment conditioned upon results (, the level of payment () and a constant reflecting the general preferences for results-based schemes that not reflected in the attributes presented on the choice card (:
We work under the assumption that, on a choice card, a farmer n will choose the alternative i that would provide them with the highest level of utility, so that the probability that an individual n chooses alternative i, over alternative j on choice card Ct is:
Assuming that the random term is Identically and Independently Distributed (IID) following a Gumble distribution, we obtain the conditional logit model (McFadden, 1974[11]), with the probability of individual n choosing alternative i over J other alternatives on a choice card defined as:
The beta parameters of the model are estimated using maximum likelihood.
The Latent Class Model
When introducing a discrete distribution of betas in the population we obtain the latent class model, which identifies classes of respondents that share the same preferences (same betas). Conditioned on belonging to class s, the probability of individual n choosing alternative i is defined as in the conditional logit model by:
The unconditional probability of choice over T choices is therefore defined as:
With Mn,s the probability that individual n belongs to class s.
This probability itself is estimated using a Multinomial logit model and depends on individuals’ characteristics (vector Zn) as follows:
With αs the vector parameters representing the weights of individual characteristics Zn in the probability of class membership.
In the next section we present how these two modelling approaches (conditional logit and latent class models) were successively implemented in the analysis of the choice experiment data.
Overview of the analysis and model specifications
Data validation: before proceeding to the analysis, the range of responses for each variable was checked and compared to expected values and the coherence of responses was verified for the values entered for the farm acreage and repartition between arable land, grassland and other type of land. We identified one respondent with responses outside the range of expected values to all questions and a systematic choice of “I would not participate” on the choice cards. This respondent was therefore considered as a protest respondent and their responses considered as unreliable and was dropped for the analysis. All other respondents were kept in the dataset. Responses to the current usage of fertilisers were also inconsistent between respondents and were not included in the analysis.
The first analysis of farmers’ choices and preferences for alternative AES designs was performed using a conditional logit model on the overall data, and then per country and per farm specialisation. All these models share the same specifications and account for the panel structure of the data (each respondent making a series of six choices, the six choices of a single respondent are not independent): robust standard errors, t-ratios and associated p-values are computed using the ‘sandwich’ estimator, which corrects standard errors accordingly. The Apollo software in R (Hess and Palma, 2019[12]) was used for the choice modelling. Farmers’ Willingness to Accept (WTA) were estimated based on the conditional logit models results, as the ratio of the parameter associated over the attribute of interest and the price parameter, through the Delta method using the same package on R. For a full description of the Delta method and how it can be used to estimate WTA, please refer to (Daly, Hess and de Jong, 2012[13])
We then proceeded to a clustering of all respondents based on their socio demographic, farm and behavioural characteristics as well as their baseline agri-environmental characteristics on Stata. We used a K-means partitioning, based on the Dice similarity coefficient for clusters based on binary variables, and the Gower coefficient for clusters relying on a mix of continuous and binary variables. The number of clusters for each clustering exercise was determined using a combination of the following criteria: the within sum of squares for different numbers of clusters (2 to 20) (Makles, 2012[14]) and the ability to generate clusters of reasonable size with distinct and significant different characteristics that are coherent, while keeping the number of clusters reasonably low as these need to be usable in the latent class analysis.
Using the cluster membership as explanatory variables for the different classes of respondents, we performed a latent class analysis with the Apollo software in R (Hess and Palma, 2019[12]) in order to identification of groups of farmers with homogeneous characteristics and preferences.
Annex 4.C. Descriptive statistics and variable definitions
Annex Table 4.C.1. Descriptive statistics: Farm characteristics
Descriptives for farm characteristics |
||||
---|---|---|---|---|
Variable |
Nb of observations |
Mean (SD) |
Min |
Max |
Total Farm Land (ha) |
723 |
160.1 (266.3) |
1.0 |
3 500.0 |
Including: |
||||
Arable Land (ha) |
705 |
70.8 (106.7) |
0.0 |
1 760.0 |
Permanent Grassland (ha) |
671 |
10.3 (26.8) |
0.0 |
350.0 |
Average Field Size (ha) |
721 |
6.8 (16.2) |
0.0 |
200.0 |
Livestock (for mixed farming systems): |
||||
Dairy cattle (livestock units) |
92 |
53.1 (65) |
0.0 |
425.0 |
Beef cattle (livestock units) |
137 |
44.9 (71.5) |
0.0 |
550 137.0 |
Sheep (livestock units) |
94 |
56.8 (100.7) |
0.0 |
500.0 |
Other livestock (nb) |
98 |
1825.4 (16174.6) |
0.0 |
160 000.0 |
Distance closest grazing field (km) |
258 |
0.2 (0.8) |
0.0 |
10.0 |
Distance most distant grazing field (km) |
258 |
5.4 (10) |
0.0 |
70.0 |
Distance most of grazing fields (km) |
251 |
3.3 (18.8) |
0.0 |
216.0 |
Land tenure: Owned (ha) |
698 |
74.9 (206.2) |
0.0 |
4 550.0 |
Land tenure: Leased (ha) |
640 |
33.2 (62.2) |
0.0 |
850.0 |
Land tenure: Commons (ha) |
487 |
15.1 (50.2) |
0.0 |
500.0 |
Workforce on farm: Full-time workforce |
572 |
1.4 (1.6) |
0.0 |
18.0 |
Part-time workforce |
547 |
1.4 (1.7) |
0.0 |
20.0 |
Share farm income in household income (%) |
528 |
36.7 (27.6) |
0.0 |
100.0 |
Annex Table 4.C.2. Descriptive statistics: Farm characteristics (continued)
Further descriptives for farm characteristics |
|||
---|---|---|---|
Frequency |
Percentage |
Cumulative |
|
Other source of income |
|||
No |
184 |
25.6 |
25.6 |
Yes |
534 |
74.4 |
100 |
Organic farming |
|||
No |
611 |
83.6 |
83.6 |
Yes |
120 |
16.4 |
100 |
Annex Table 4.C.3. Descriptive statistics: Socio-demographic characteristics
Descriptives for socio-demographic characteristics |
|||
---|---|---|---|
Variable |
Frequency |
Percentage |
Cumulative |
Gender |
|||
Female |
62.0 |
8.6 |
8.6 |
Male |
650.0 |
89.7 |
98.3 |
Prefer not to say |
10.0 |
1.4 |
99.7 |
Other |
3.0 |
0.4 |
100.0 |
Age |
|||
18 - 34 |
39.0 |
5.4 |
5.4 |
35 - 44 |
116.0 |
16.0 |
21.4 |
45 - 54 |
193.0 |
26.7 |
48.1 |
55 - 64 |
219.0 |
30.3 |
78.3 |
> 65 |
142.0 |
19.6 |
97.9 |
Prefer not to say |
15.0 |
2.1 |
100.0 |
Education |
|||
None |
2.0 |
0.3 |
0.3 |
Minimum compulsory |
27.0 |
3.7 |
4.0 |
High school diploma in agriculture |
108.0 |
14.9 |
18.9 |
High school diploma not in agriculture |
78.0 |
10.8 |
29.7 |
Vocational training in agriculture |
174.0 |
24.1 |
53.8 |
Vocational training not in agriculture |
98.0 |
13.6 |
67.4 |
University degree in agriculture |
92.0 |
12.7 |
80.1 |
University degree not in agriculture |
100.0 |
13.8 |
93.9 |
Other |
44.0 |
6.1 |
100.0 |
Farmer status |
|||
Full-time farmer |
419.0 |
58.2 |
58.2 |
Part-time farmer |
264.0 |
36.7 |
95.5 |
Farm employee |
4.0 |
0.6 |
96.1 |
Other |
33.0 |
4.6 |
100.0 |
Annex Figure 4.C.1. Risk and time attitudes
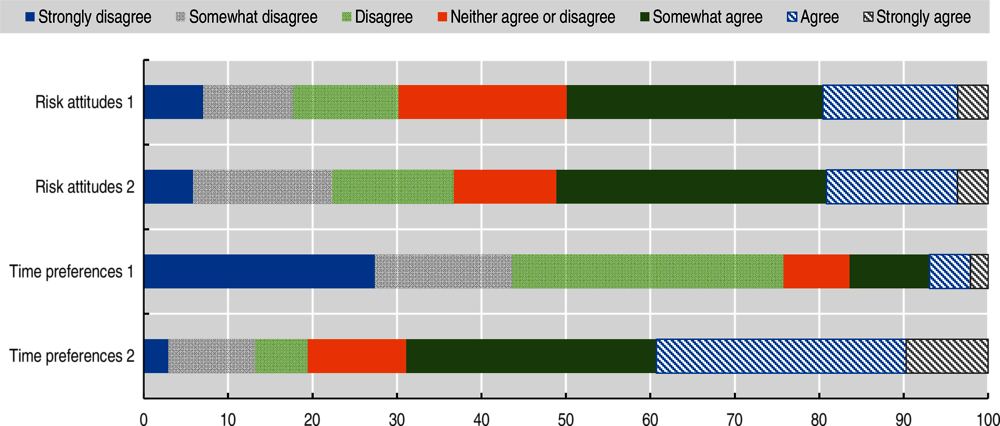
Annex Table 4.C.4. Statements used to elicit risk attitudes and time preferences
Risk attitudes 1 |
I am willing to adopt new farming practices that may or may not pay off in the future |
Risk attitudes 2 |
I am generally willing to take risks when managing my farm |
Time preferences 1 |
My farm management decisions are only influenced by the consequences of my actions within the current farming season and not beyond |
Time preferences 2 |
Often, I manage my farm in order to achieve outcomes that may not result for many years |
Annex Figure 4.C.2. Awareness of and ascription of responsibility for environmental issues
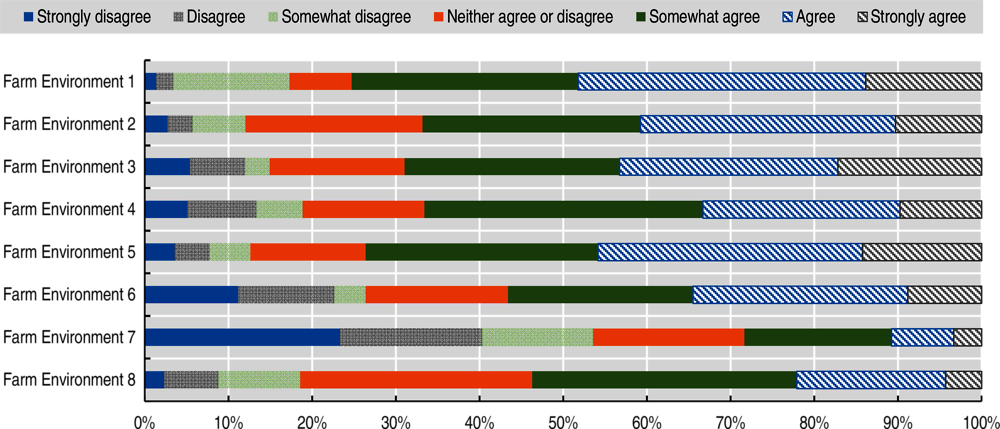
Annex Table 4.C.5. Statements used to elicit attitudes towards the environment
Farm environment 1 |
The environment around me is doing okay (shifting baseline theory – perception of environmental change) |
Farm environment 2 |
The environment around me has gotten better over the past five years (shifting baseline theory – perception of environmental change) |
Farm environment 3 |
Global warming is a serious threat (perception on environmental issues) |
Farm environment 4 |
Farming practices directly impact the health of the environment (awareness of consequences) |
Farm environment 5 |
Farming using environmentally friendly practices can improve the health of the environment (awareness of consequences) |
Farm environment 6 |
I feel responsible for local environmental issues (ascription of responsibility) |
Farm environment 7 |
I feel responsible for international or global environmental issues (e.g. global warming) (ascription of responsibility) |
Farm environment 8 |
The farmers in my area are willing to adopt (or have already adopted) farming practices to improve environmental outcomes (social norms) |
Annex Figure 4.C.3. Responses to survey follow-up questions
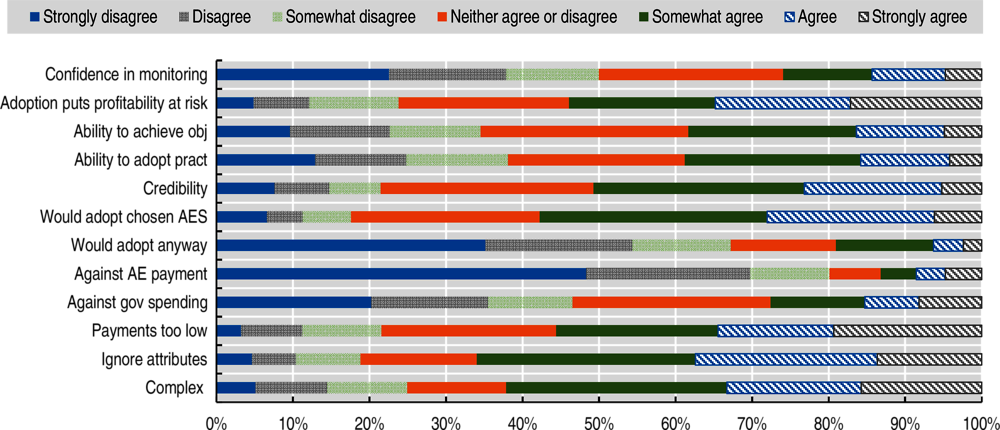
Annex 4.D. Model extensions and detailed tables
To complement the main analysis, we analyse separately the choices made by farmers from each participating country and for each farm specialisation (mixed farmers or arable farmers).
Annex Table 4.D.1. Cross country comparison of farmers’ preferences for AES designs: Results from conditional logit models
Conditional Logit Model |
Finland |
Sweden |
Netherlands |
||||||
---|---|---|---|---|---|---|---|---|---|
|
Estimate |
|
Robust Standard Errors |
Estimate |
|
Robust Standard Errors |
Estimate |
|
Robust Standard Errors |
ASC practice-based |
-1.011 |
*** |
0.139 |
-0.967 |
*** |
0.166 |
-1.447 |
*** |
0.220 |
ASC hybrid |
-0.624 |
*** |
0.181 |
-0.872 |
*** |
0.232 |
-1.383 |
*** |
0.321 |
ASC results-based |
-1.413 |
*** |
0.162 |
-1.398 |
*** |
0.207 |
-1.644 |
*** |
0.244 |
Practices 2 (ref 1) |
-0.147 |
0.090 |
-0.324 |
*** |
0.114 |
-0.022 |
0.159 |
||
Practices 3 (ref 1) |
-0.291 |
*** |
0.092 |
-0.279 |
** |
0.125 |
-0.461 |
*** |
0.166 |
Water 1 (ref 0) |
-0.400 |
*** |
0.117 |
-0.259 |
* |
0.155 |
0.125 |
0.194 |
|
Water 2 (ref 0) |
-0.416 |
*** |
0.085 |
-0.207 |
** |
0.099 |
-0.334 |
** |
0.135 |
CC 1 (ref 0) |
-0.352 |
*** |
0.094 |
-0.112 |
0.103 |
-0.207 |
0.145 |
||
CC 2 (ref 0) |
-0.318 |
*** |
0.097 |
-0.245 |
** |
0.125 |
-0.337 |
* |
0.189 |
Biodiversity 1 (ref 0) |
-0.177 |
* |
0.091 |
0.217 |
** |
0.109 |
-0.190 |
0.169 |
|
Biodiversity 2 (ref 0) |
-0.093 |
0.098 |
0.062 |
0.128 |
-0.218 |
0.164 |
|||
Share conditioned on result |
-0.003 |
** |
0.001 |
-0.002 |
0.002 |
-0.002 |
0.003 |
||
Payment level |
0.007 |
*** |
0.001 |
0.005 |
*** |
0.001 |
0.004 |
*** |
0.001 |
Number of respondents |
357 |
230 |
144 |
||||||
Number of observations |
2142 |
1380 |
864 |
||||||
AIC |
5481.22 |
5481.22 |
2043.05 |
||||||
BIC |
5554.93 |
5554.93 |
2104.95 |
||||||
LL |
-2727.611 |
-1814.703 |
-1008.523 |
*: robust p-value < 0.10; **: robust p-value < 0.05, ***: robust p-value <0.01
Note that a Likelihood Ratio Test shows that the difference between the coefficents associated with 2 levels of the CC attribute (CC1 and CC2) in Finland is not significantly different from 0 (p-value = 0.729), similarly a LR test shows that the difference between Practices 2 and 3 in Sweden is not significantly different from 0 (p-value = 0.671), neither are Water 1 and Water 2 in Sweden (p-value =0.729).
Annex Table 4.D.2. Estimated willingness to accept per country
WTA estimates (Delta Method) |
Finland |
Sweden |
Netherlands |
||||||
---|---|---|---|---|---|---|---|---|---|
UR |
Estimate (EUR) |
Robust Standard Errors |
Estimate (EUR) |
Robust Standard Errors |
Estimate (EUR) |
Robust Standard Errors |
|||
ASC practice-based |
137.2 |
*** |
16.9 |
181.3 |
*** |
29.0 |
368.4 |
*** |
67.7 |
ASC hybrid |
84.7 |
*** |
24.7 |
163.8 |
*** |
44.0 |
352.0 |
*** |
102.2 |
ASC results-based |
191.7 |
*** |
22.2 |
262.2 |
*** |
38.0 |
418.3 |
*** |
85.3 |
Practices 2 (ref 1) |
19.9 |
12.1 |
60.9 |
*** |
19.9 |
5.5 |
40.2 |
||
Practices 3 (ref 1) |
39.5 |
*** |
12.2 |
52.3 |
** |
22.9 |
117.3 |
*** |
42.2 |
Water 1 (ref 0) |
54.3 |
*** |
15.1 |
48.6 |
* |
28.3 |
-31.9 |
52.7 |
|
Water 2 (ref 0) |
56.5 |
*** |
11.0 |
38.9 |
** |
18.5 |
85.0 |
*** |
30.3 |
CC 1 (ref 0) |
47.8 |
*** |
12.7 |
21.0 |
19.0 |
52.6 |
35.5 |
||
CC2 (ref 0) |
43.2 |
*** |
12.9 |
46.1 |
** |
23.0 |
85.9 |
* |
46.6 |
Biodiversity 1 (ref 0) |
24.1 |
* |
12.5 |
-40.8 |
** |
20.6 |
48.5 |
43.5 |
|
Biodiversity 2 (ref 0) |
12.6 |
13.2 |
-11.6 |
24.2 |
55.4 |
40.9 |
|||
Share conditioned on result |
0.5 |
** |
0.2 |
0.4 |
0.4 |
0.5 |
0.7 |
Note: *: p-value < 0.10; **: p-value < 0.05, ***: p-value <0.01.
Annex Table 4.D.3. Comparison of farmers’ preferences for AES designs by farm specialisation: Results from conditional logit models
Conditional Logit Model |
Pooled |
Arable |
Mixed farming |
||||||
---|---|---|---|---|---|---|---|---|---|
|
Estimate |
|
Robust standard errors |
Estimate |
|
Robust standard errors |
Estimate |
|
Robust standard errors |
ASC practice-based |
-1.097 |
*** |
0.095 |
-1.212 |
*** |
0.122 |
-0.904 |
*** |
0.152 |
ASC hybrid |
-0.861 |
*** |
0.129 |
-1.007 |
*** |
0.164 |
-0.600 |
*** |
0.208 |
ASC results-based |
-1.483 |
*** |
0.113 |
-1.560 |
*** |
0.147 |
-1.336 |
*** |
0.178 |
Practices 2 (ref 1) |
-0.187 |
*** |
0.064 |
-0.114 |
0.081 |
-0.303 |
*** |
0.105 |
|
Practices 3 (ref 1) |
-0.313 |
*** |
0.067 |
-0.264 |
*** |
0.082 |
-0.395 |
*** |
0.116 |
Water 1 (ref 0) |
-0.271 |
*** |
0.084 |
-0.222 |
** |
0.106 |
-0.358 |
** |
0.141 |
Water 2 (ref 0) |
-0.333 |
*** |
0.058 |
-0.448 |
*** |
0.075 |
-0.186 |
** |
0.090 |
CC 1 (ref 0) |
-0.247 |
*** |
0.063 |
-0.319 |
*** |
0.077 |
-0.153 |
0.106 |
|
CC2 (ref 0) |
-0.286 |
*** |
0.070 |
-0.359 |
*** |
0.089 |
-0.174 |
0.113 |
|
Biodiversity 1 (ref 0) |
-0.029 |
0.064 |
-0.055 |
0.083 |
-0.003 |
0.104 |
|||
Biodiversity 2 (ref 0) |
-0.053 |
0.070 |
0.027 |
0.084 |
-0.180 |
0.122 |
|||
Share conditioned on result |
-0.003 |
** |
0.001 |
-0.001 |
0.001 |
-0.004 |
** |
0.002 |
|
Payment level |
0.006 |
*** |
0.000 |
0.006 |
*** |
0.000 |
0.006 |
*** |
0.001 |
Number of respondents |
731 |
461 |
270 |
||||||
Number of observations |
4386 |
2766 |
2766 |
||||||
AIC |
11326.35 |
7053.31 |
4266.18 |
||||||
BIC |
11409.37 |
7130.34 |
4336.26 |
||||||
LL |
-5650.176 |
-3513.654 |
-2120.092 |
Note: *: robust p-value < 0.10; **: robust p-value < 0.05, ***: robust p-value <0.01.
In order to confirm the robustness of the results obtained under alternative specifications of the utility functions, the models were ran using a single ASC constant capture farmers’ preferences for joining a scheme that are not captured by the attributes included on the choice cards. The share attribute was coded as continuous in the hybrid alternative and the share attribute in the other two design options is considered as two additional independent variables: either 0% (practice-based) or 100% (results-based), to avoid a discontinuous jump in WTA for the share attribute at approximatively 0 and approximatively 100. Therefore, the variable `share’ as a continuous variable in the utility function associated with hybrid schemes would only apply for a range from 10% to 90% (which was the range used within the hybrid schemes). The observable parts of the utility functions are now defined as:
The results obtained using this specification are presented in Annex Table 4.D.4, which yields the following values of WTA in Annex Table 4.D.5.
Annex Table 4.D.4. Results of the Conditional logit model under a specification with 1 single ASC and the share attribute is specific to each scheme design
30/03/2022 |
|
n |
731 |
LL |
-5649.8 |
||
---|---|---|---|---|---|---|---|
MNL |
|
obs |
4386 |
Adjust. R2 |
0.0685 |
||
|
|
|
|
AIC |
11327.6 |
||
|
|
|
|
BIC |
11417.01 |
||
Conditional Logit Model |
|||||||
Estimate |
Rob.std.err. |
Rob.t-ratio(0) |
Rob.p-val(0) |
||||
|
|||||||
ASC |
-0.909 |
*** |
0.142 |
-6.394 |
0.000 |
||
Practices 1 |
0.063 |
|
0.074 |
0.861 |
0.389 |
||
Practices 2 |
-0.166 |
** |
0.070 |
-2.392 |
0.017 |
||
Practices 3 |
-0.294 |
*** |
0.068 |
-4.310 |
0.000 |
||
Water 1 (ref 0) |
-0.264 |
*** |
0.085 |
-3.113 |
0.002 |
||
Water 2 (ref 0) |
-0.326 |
*** |
0.060 |
-5.390 |
0.000 |
||
CC 1 (ref 0) |
-0.239 |
*** |
0.064 |
-3.752 |
0.000 |
||
CC2 (ref 0) |
-0.276 |
*** |
0.073 |
-3.802 |
0.000 |
||
Biodiversity 1 (ref 0) |
-0.022 |
|
0.063 |
-0.343 |
0.732 |
||
Biodiversity 2 (ref 0) |
-0.042 |
|
0.070 |
-0.603 |
0.547 |
||
All payments based on practice |
-0.214 |
* |
0.121 |
-1.770 |
0.077 |
||
Share conditioned on result [10 to 90%] |
-0.002 |
** |
0.001 |
-2.297 |
0.022 |
||
All payments based on results |
-0.582 |
*** |
0.124 |
-4.682 |
0.000 |
||
Payment level |
0.006 |
*** |
0.000 |
16.510 |
0.000 |
Annex Table 4.D.5. WTA estimates derived from Annex Table 4.D.4
WTA estimates (Delta Method) |
|
|
||||||
---|---|---|---|---|---|---|---|---|
|
Estimate (EUR) |
|
Rob.std.err. |
Rob.t-ratio(0) |
Rob.p-val(0) |
|||
ASC |
152.30 |
*** |
25.47 |
5.98 |
0.000 |
|||
Practice 1 |
Not significantly different from 0 |
|||||||
Practice 2 |
27.85 |
** |
11.27 |
2.471 |
0.014 |
|||
Practice 3 |
49.29 |
*** |
11.03 |
4.469 |
0.000 |
|||
Water 1 (ref 0) |
44.16 |
*** |
13.55 |
3.26 |
0.001 |
|||
Water 2 (ref 0) |
54.53 |
*** |
9.578 |
5.694 |
0.000 |
|||
CC 1 (ref 0) |
40.01 |
*** |
10.38 |
3.854 |
0.000 |
|||
CC2 (ref 0) |
46.31 |
*** |
11.79 |
3.927 |
0.000 |
|||
Biodiversity 1 (ref 0) |
3.60 |
10.52 |
0.3423 |
0.732 |
||||
Biodiversity 2 (ref 0) |
7.07 |
11.66 |
0.6065 |
0.544 |
||||
All payments based on practice |
35.89 |
* |
19.79 |
1.814 |
0.070 |
|||
Share conditioned on result [10 to 90%] |
0.41 |
** |
0.1756 |
2.339 |
0.020 |
|||
All payments based on results |
97.55 |
*** |
19.96 |
4.888 |
0.000 |
The WTA for alternative scheme designs are then computed, accounting for all their characteristics, to compare the results with our initial findings. These WTA values are obtained by summing up the marginal WTA values for each of the attributes and the ASC reported in Annex Table 4.D.5. The results show that the WTA results under this new specification are very close to the initial specification and, most importantly, all the report’s interpretation based on relative preferences hold.
Practice-based schemes
Level of requirements |
Initial analysis Mean WTA (EUR/ha/year) |
Analysis using specification MNL Mean WTA (EUR/ha/year) |
---|---|---|
Level 1: First 2 practices |
181.7 |
188.19 |
Level 2: First 4 practices |
212.8 |
216.04 |
Level 3: All 6 practices |
233.7 |
237.48 |
Results-based schemes
Level of requirements |
Initial analysis Mean WTA (EUR4/ha/year) |
Analysis using specification MNL Mean WTA (EUR/ha/year) |
---|---|---|
Biodiversity only |
245.8 |
249.85 |
Water 1 and CC1 |
331.7 |
334.02 |
Water 2 and CC2 |
348.7 |
350.69 |
Hybrid schemes
Level of requirement |
Mean WTA (EUR 4/ha/year) |
|||||
---|---|---|---|---|---|---|
20% of payment conditioned on results |
50% of payment conditioned on results |
80% of payment conditioned on result |
||||
Initial spec. |
US2 spec |
Initial spec. |
US2 spec |
Initial spec. |
US2 spec |
|
First 2 practices + Biodiversity 1 |
151.3 |
160.5 |
163.8 |
172.8 |
176.3 |
185.1 |
First 4 practices + Water 1 and CC1 |
268.2 |
272.5 |
280.8 |
284.9 |
293.3 |
297.2 |
First 4 practices + Water 2 and CC2 |
285.2 |
289.2 |
297.7 |
301.5 |
310.3 |
313.8 |
All 6 practices + Water 1 and CC1 |
289.2 |
294.0 |
301.7 |
306.3 |
314.2 |
318.6 |
All 6 practices + Water 2 and CC2 |
306.1 |
310.6 |
318.7 |
323.0 |
331.2 |
335.3 |
Annex 4.E. Heterogeneity in farmer preferences (clusters)
Clustering farmers according to their characteristics
As a first step before the latent class analysis, with the objective to reduce the number of variables to be included as explanatory variables to the class membership, we generate several clustering of respondents: described below. Note that all clustering are independent from each other, meaning that a respondent could belong to cluster 1 in terms of their farm characteristics, while they may belong to cluster 2 or 3 based on their own individual characteristics.
The first clustering separates farmers according to their farm’s characteristics: farmers are separated in three clusters, which characteristics are presented in Table A E.1. Note that farm specialisation (mixed farming or arable farming) is not a determinant of cluster membership despite being included for the clustering.
Annex Table 4.E.1. Description of three clusters of farm characteristics
Clusters of farm characteristics |
Mean (SD) |
Bartlett’s test |
||
---|---|---|---|---|
Variables |
Cluster 1 N=162 |
Cluster 2 N=243 |
Cluster 3 N=263 |
|
Total land (ha) |
198.4 (381.1) |
175.6 (185.6) |
133.6 (264.7) |
*** |
Arable land (ha) |
89.7 (149.4) |
91.8 (92.0) |
41.8 (84.1) |
*** |
Specialisation (% in mixed farming) |
33.3 (47.3) |
42.4 (49.5) |
37.3 (48.4) |
|
Share of land owned (%) |
54.0 (48.5) |
75.7 (309.6) |
57.0 (44.1) |
*** |
Other income (%) |
100.0 (0.0) |
30.9 (46.3) |
100.0 (0) |
*** |
Share farm income in household (%) |
52.1 (9.5) |
95.1 (8.4) |
14.5 (10.7) |
*** |
Note: *: p-value < 0.10; **: p-value < 0.05, ***: p-value <0.01
Cluster 1 (Farm 1, n=162): Largest farms (198 hectares on average, including 89.7 ha of arable land), about half of the farmland in ownership, with all farms having another source of income available for the household, and the income from the farm representing about half of the household income (52.1% in average)
Cluster 2 (Farm 2, n=243): Medium farms (175.6 hectares on average, including 91.8 ha of arable land), with in average about three-quarters of the farmland in ownership, with only a third of farms having another source of income available for the household than the farm revenues, therefore the income from the farm representing the largest share of the household income (95.1% in average)
Cluster 3 (Farm 3, n=263): Smallest farms (133.6 hectares on average, including 41.8 ha of arable land), with in average slightly more than half of the farmland in ownership, with most of the household income coming from other sources of income (only 14.5% of the household income being generated by the farm activity on average).
The second clustering separates farmers according to their socio-demographic characteristics: farmers are separated in three clusters (Annex Table 4.E.2).
Annex Table 4.E.2. Description of three clusters of farmers characteristics
Clusters of farmers’ characteristics |
Mean (SD) |
Bartlett’s test |
||
---|---|---|---|---|
Variables (%) |
Cluster 1 N=219 |
Cluster 2 N=229 |
Cluster 3 N=270 |
|
Gender |
||||
Female |
11.0 (31.3) |
6.1 (24.0) |
8.9 (28.5) |
*** |
Age |
||||
18 - 34 |
10.0 (30.1) |
0.0 (0.0) |
6.30 (24.3) |
*** |
35 - 44 |
30.6 (46.2) |
0.0 (0.0) |
17.8 (38.3) |
*** |
45 - 54 |
55.3 (49.8) |
0.0 (0.0) |
26.7 (44.3) |
* |
55 - 64 |
0.0 (0.0) |
67.2 (47.0) |
24.1 (42.8) |
|
> 64 |
0.0 (0.0) |
32.8 (47.0) |
23.3 (42.4) |
|
Education |
||||
None |
0.4 (6.8) |
0.0 (0.0) |
0.4 (6.1) |
|
Minimum compulsory |
2.7 (16.4) |
4.8 (21.4) |
3.7 (18.9) |
*** |
High school diploma in agriculture |
18.7 (39.1) |
21.4 (41.1) |
6.7 (25.0) |
*** |
High school diploma not in agriculture |
8.7 (28.2) |
12.7 (33.3) |
11.1 (31.5) |
** |
Vocational training in agriculture |
27.4 (44.7) |
29.3 (45.6) |
16.7 (37.3) |
*** |
Vocational training not in agriculture |
10.5 (30.7) |
10.5 (30.7) |
17.8 (38.3) |
*** |
University degree in agriculture |
19.2 (39.5) |
10.0 (30.1) |
10.0 (30.1) |
*** |
University degree not in agriculture |
7.76 (26.8) |
5.2 (22.3) |
26.3 (44.1) |
*** |
Other |
4.6 (20.9) |
6.1 (24.0) |
7.4 (26.2) |
*** |
Farmer Status |
||||
Full-time farmer |
95.4 (20.9) |
90.8 (28.9) |
0.0 (0.0) |
*** |
Part-time farmer |
0.0 (0.0) |
0.0 (0.0) |
97.8 (14.8) |
*** |
Farm employee |
1.3 (11.7) |
0.4 (6.6) |
0.0 (0.0) |
*** |
Other |
3.2 (17.6) |
8.7 (28.3) |
2.2 (14.8) |
*** |
Note: *: p-value < 0.10; **: p-value < 0.05, ***: p-value <0.01
Cluster 1 (Farmer 1, n=219): Relatively more female farmers (11%), younger farmers, with a larger share having a university degree in agriculture, mostly full time farmers
Cluster 2 (Farmer 2, n=229): Relatively fewer female farmers (6%), older farmers, with a larger share having the minimum compulsory education, a high school diploma or a vocational training in agriculture, mostly full time farmers
Cluster 3 (Farmer 3, n=270): Average share of female farmers (8.6%), average age similar to the overall sample, with a larger share with a university degree not in agriculture or a vocational training not in agriculture, part-time farmers
The third clustering separates farmers according to their current agri-environmental practices: farmers are separated in three clusters (Annex Table 4.E.3).
Annex Table 4.E.3. Description of 3 clusters of current agri-environmental practices
Clusters of current AE Practices |
Mean (SD) |
Bartlett’s test |
||
---|---|---|---|---|
Variables |
Cluster 1 N=166 |
Cluster 2 N=340 |
Cluster 3 N=148 |
|
Field size (ha) |
8.7 (19.8) |
5.5 (14.3) |
7.9 (17.6) |
*** |
Manure injection (ref: does not use manure injection) |
42.8 (49.6) |
20.9 (40.7) |
30.4 (46.2) |
*** |
Cover crops |
1.9 (0.8) |
0.1 (0.3) |
1.1 (0.9) |
*** |
Green fallow |
14.3 (22.3) |
5.39 (12.4) |
5.5 (14.4) |
*** |
Landscape |
0.4 (0.8) |
0.6 (0.9) |
2.1 (0.9) |
|
Buffer |
0.5 (0.8) |
0.8 (1.0) |
2.3 (0.9) |
** |
Currently engaged in an AES (%) |
65.7 (47.6) |
80.9 (39.4) |
81.8 (38.8) |
*** |
Never participated in an AES (%) |
27.1 (44.6) |
7.4 (26.1) |
10.1 (30.3) |
*** |
Organic farming |
16.9 (37.6) |
12.9 (33.6) |
26.4 (44.2) |
*** |
Note: *: p-value < 0.10; **: p-value < 0.05, ***: p-value <0.01
Cluster 1 (AEpract 1, n=166): Large fields, larger share injecting manure (43%), more use of cover crops, larger share of arable land in green fallow (14%), but scarcer presence of buffer strips next to water courses, relatively fewer currently engaged in an AES (66%), and larger share who have never participated in an AES (27%),
Cluster 2 (AEpract 2, n= 340): Small fields, smaller share injecting manure (21%), lowest use of cover crops, small share of arable land in green fallow (5%), medium presence of buffer strips next to water courses, most engaged in an AES (81%), few organic farmers (12%)
Cluster 3 (AEpract 3, n=148): Medium fields, medium share injecting manure (30%), medium use of cover crops, small share of arable land in green fallow (5.5%), but largest presence of buffer strip next to water courses (majority or all fields), most engaged in an AES (82%), largest share of organic farmers (26%).
The fourth clustering separated farmers according to their behavioural (time and risk) and environmental preferences: farmers are separated in two clusters (Annex Table 4.E.4).
Annex Table 4.E.4. Description of 2 clusters of behavioural characteristics of farmers
Clusters of behavioural characteristics |
Mean (SD) |
Bartlett’s test |
T-test |
|
---|---|---|---|---|
Variables |
Cluster 1 N= 254 |
Cluster 2 N=448 |
|
|
Time |
||||
Time preferences 1 |
-0.9 (1.6) |
-1.6 (1.5) |
*** |
|
Time preferences 2 |
0.4 (1.5) |
1.1 (1.4) |
*** |
|
Risk |
||||
Risk attitudes 1 |
-0.5 (1.5) |
0.6 (1.5) |
*** |
|
Risk attitudes 2 |
-0.4 (1.6) |
0.4 (1.5) |
*** |
|
Environment |
||||
Farm environment 1 |
1.7 (1.2) |
1.2 (1.3) |
*** |
|
Farm environment 2 |
1.0 (1.4) |
1.0 (1.3) |
||
Farm environment 3 |
-0.3 (1.6) |
1.5 (1.3) |
*** |
*** |
Farm environment 4 |
-0.4 (1.5) |
1.1 (1.3) |
*** |
*** |
Farm environment 5 |
0.1 (1.5) |
1.5 (1.2) |
*** |
*** |
Farm environment 6 |
-1.3 (1.5) |
1.2 (1.3) |
** |
*** |
Farm environment 7 |
-2.2 (1.0) |
0.1 (1.5) |
*** |
*** |
Farm environment 8 |
0.1 (1.4) |
0.8 (1.2) |
* |
*** |
Note: *: p-value < 0.10; **: p-value < 0.05, ***: p-value <0.01
Cluster 1 (Behaviour 1, n= 254): Strongest preferences for present (/ present biais), more risk averse, lower perceived need for environmental action, less awareness of consequences and perceived control, lower sense of responsibility over local and global environmental issues
Cluster 2 (Behaviour 2, n= 448): Longer term thinking, less risk averse, perceive more need for environmental action, show more awareness of consequences of farming on the environment, more awareness of consequences (Farming using environmentally friendly practices can improve the health of the environment), and sense of responsibility for local and global environmental issues
And finally, the fifth clustering separates farmers according to their answers to the follow up questions, measuring their attitudes towards the questionnaire itself: farmers are allocated to one of two clusters.
Annex Table 4.E.5. Testing for cluster differences
Clusters of follow up questions |
Mean (SD) |
Bartlett’s test |
t-test |
||
---|---|---|---|---|---|
Variables |
Cluster 1 N=298 |
Cluster 2 N=386 |
|
||
Complex |
1.0 (1.8) |
0.4 (1.6) |
** |
*** |
|
Ignore attributes |
1.1 (1.7) |
0.6 (1.5) |
** |
*** |
|
Payments too low |
1.5 (1.5) |
0.1 (1.5) |
*** |
||
Against government spending |
-0.3 (2.0) |
-0.7 (1.7) |
*** |
*** |
|
Against AE payment |
-1.9 (1.7) |
-1.6 (1.8) |
** |
||
Would adopt anyway |
-2.1 (1.4) |
-0.7 (1.7) |
** |
*** |
|
Would adopt chosen AES |
-0.3 (1.6) |
1.2 (1.0) |
*** |
*** |
|
Credibility |
-0.5 (1.6) |
1.0 (1.1) |
*** |
*** |
|
Ability to adopt practice |
-1.5 (1.4) |
0.8 (1.1) |
*** |
*** |
|
Ability to achieve objective |
-1.1 (1.5) |
0.8 (1.1) |
*** |
*** |
|
Adoption puts profitability at risk |
1.6 (1.5) |
-0.1 (1.4) |
*** |
||
Confidence in monitoring |
-1.5 (1.7) |
-0.02 (1.6) |
*** |
Note: *: p-value < 0.10; **: p-value < 0.05, ***: p-value <0.01.
Cluster 1 (Followup 1, n= 298): Find the survey more complex, are more likely to have ignores attributes in their choices, slightly more to be against more government spending, less likely to adopt the proposed measures without payment, provide less genuine answers (i.e. state they would adopt the chosen AES), gave less credibility to the survey, feel less able to adopt the practices or to achieve the results presented on the choice cards 🡺 protesters
Cluster 2 (Followup 2, n=386): The symmetric of cluster 1.
Each farmer is allocated to one of the clusters for each category of variables and we use the cluster allocation to describe the class membership in the latent class model below.
Annex Table 4.E.6. Results of the latent class model, with two distinct classes of farmers in terms of AES preferences
Latent class model |
Class 1 (63.35%) |
Class 2 (36.65%) |
||||||||
---|---|---|---|---|---|---|---|---|---|---|
2 classes |
Estimate |
Rob.std.err. |
Rob.t-ratio(0) |
Rob.p-val(0) |
Estimate |
Rob.std.err. |
Rob.t-ratio(0) |
Rob.p-val(0) |
||
Preferences |
|
|
|
|
|
|
|
|
|
|
ASC practice-based |
0.925 |
*** |
0.195 |
4.732 |
0.000 |
-3.456 |
*** |
0.283 |
-12.209 |
0.000 |
ASC hybrid |
1.240 |
*** |
0.214 |
5.802 |
0.000 |
-3.094 |
*** |
0.545 |
-5.672 |
0.000 |
ASC results-based |
0.427 |
** |
0.208 |
2.049 |
0.040 |
-3.406 |
*** |
0.400 |
-8.526 |
0.000 |
Practices 2 (ref 1) |
-0.253 |
*** |
0.084 |
-3.013 |
0.003 |
-1.027 |
*** |
0.270 |
-3.807 |
0.000 |
Practices 3 (ref 1) |
-0.381 |
*** |
0.090 |
-4.243 |
0.000 |
-1.677 |
*** |
0.321 |
-5.226 |
0.000 |
Water 1 (ref 0) |
-0.323 |
*** |
0.106 |
-3.037 |
0.002 |
-0.624 |
0.673 |
-0.927 |
0.354 |
|
Water 2 (ref 0) |
-0.293 |
*** |
0.069 |
-4.228 |
0.000 |
-0.884 |
*** |
0.264 |
-3.347 |
0.001 |
CC 1 (ref 0) |
-0.208 |
*** |
0.077 |
-2.708 |
0.007 |
-0.884 |
*** |
0.256 |
-3.458 |
0.001 |
CC2 (ref 0) |
-0.291 |
*** |
0.086 |
-3.383 |
0.001 |
-0.888 |
*** |
0.279 |
-3.187 |
0.001 |
Biodiversity 1 (ref 0) |
-0.089 |
0.077 |
-1.161 |
0.246 |
0.040 |
0.255 |
0.156 |
0.876 |
||
Biodiversity 2 (ref 0) |
-0.123 |
0.097 |
-1.266 |
0.206 |
-0.213 |
0.285 |
-0.748 |
0.455 |
||
Share conditioned on result |
-0.003 |
** |
0.001 |
-2.417 |
0.016 |
-0.010 |
0.010 |
-0.935 |
0.350 |
|
Payment level |
0.007 |
*** |
0.001 |
12.293 |
0.000 |
0.009 |
*** |
0.001 |
8.166 |
0.000 |
Class membership |
|
|
|
|
|
|
|
|
|
|
Constant |
- |
|
- |
- |
- |
-1.396 |
*** |
0.277 |
-5.041 |
0.000 |
Farmer cluster 2 (ref cluster 1) |
- |
|
- |
- |
- |
-0.207 |
0.255 |
-0.812 |
0.417 |
|
Farmer cluster 3 (ref cluster 1) |
- |
|
- |
- |
- |
0.385 |
0.256 |
1.503 |
0.133 |
|
Behaviour cluster 2 (ref cluster 1) |
- |
|
- |
- |
- |
0.625 |
*** |
0.221 |
2.834 |
0.005 |
Follow up cluster 2 (ref cluster 1) |
- |
|
- |
- |
- |
2.020 |
*** |
0.209 |
9.644 |
0.000 |
Finland (ref Netherlands) |
- |
|
- |
- |
- |
0.812 |
*** |
0.266 |
3.056 |
0.002 |
Sweden (ref Netherlands) |
- |
|
- |
- |
- |
0.388 |
0.283 |
1.370 |
0.171 |
Note: *: robust p-value < 0.10; **: robust p-value < 0.05, ***: robust p-value <0.01. Number of observations=3960; Log-likelihood=-3967.93; AIC=8002; BIC=8209.
Annex Table 4.E.7. Summary statistics describing the two classes of the latent class model
|
Class 1 |
Class 2 |
||
---|---|---|---|---|
Mean |
sd |
Mean |
sd |
|
Total land area (ha) |
166.71 |
319.91 |
146.83 |
147.78 |
Arable land area (ha) |
66.24 |
108.47 |
81.47 |
106.92 |
Mixed farmers (ref: arable) (0/1) |
.39 |
0.49 |
.34 |
0.47 |
Share of land owned (1=100%) |
.68 |
2.43 |
.57 |
0.51 |
Other income available for household (0/1) |
.80 |
0.40 |
.64 |
0.48 |
Share of farm income in household income (%) |
47.28 |
35.31 |
63.82 |
35.91 |
Female (ref: male) (0/1) |
.10 |
0.29 |
.06 |
0.24 |
Age1: 18-34 |
.05 |
0.22 |
.06 |
0.24 |
Age2: 35-44 |
.19 |
0.39 |
.13 |
0.34 |
Age3: 45-54 |
.27 |
0.45 |
.27 |
0.45 |
Age4: 55-64 |
.32 |
0.47 |
.28 |
0.45 |
Age5: >65 |
.15 |
0.36 |
.22 |
0.41 |
Status: Farm employee (0/1) |
.01 |
0.08 |
0 |
0.06 |
Status: Full-time farmer (0/1) |
.5 |
0.50 |
.7 |
0.46 |
Status: Other (0/1) |
.05 |
0.22 |
.05 |
0.21 |
Status: Part-time farmer (0/1) |
.44 |
0.50 |
.25 |
0.44 |
Field size - average (ha) |
5.56 |
12.46 |
8.78 |
20.10 |
Use of manure injection (0/1) |
.23 |
0.42 |
.38 |
0.49 |
Use of cover crops** |
.79 |
0.95 |
.82 |
0.97 |
Area in green fallow (ha) |
8.45 |
17.03 |
6.71 |
14.90 |
Landscape** |
.9 |
1.08 |
.79 |
1.04 |
Buffer** |
1.16 |
1.15 |
.93 |
1.14 |
Currently in AES (0/1) |
.86 |
0.35 |
.64 |
0.48 |
Never been in AES (0/1) |
.06 |
0.25 |
.22 |
0.42 |
Organic (0/1) |
.22 |
0.42 |
.08 |
0.28 |
Time preferences 1* |
-1.39 |
1.56 |
-1.37 |
1.64 |
Time preferences 2* |
.95 |
1.41 |
.71 |
1.59 |
Risk attitudes 1* |
.43 |
1.46 |
-.32 |
1.64 |
Risk attitudes 2* |
.16 |
1.58 |
-.02 |
1.59 |
Farm environment 1* |
1.19 |
1.36 |
1.72 |
1.17 |
Farm environment 2* |
.92 |
1.38 |
1.1 |
1.42 |
Farm environment 3* |
1.05 |
1.57 |
.44 |
1.73 |
Farm environment 4* |
.81 |
1.50 |
.17 |
1.60 |
Farm environment 5* |
1.33 |
1.31 |
.36 |
1.60 |
Farm environment 6* |
.54 |
1.73 |
-.11 |
1.88 |
Farm environment 7* |
-.53 |
1.73 |
-1.15 |
1.73 |
Farm environment 8* |
.62 |
1.30 |
.34 |
1.32 |
Survey too complex* |
.45 |
1.54 |
.95 |
1.92 |
Ignored attributes* |
.8 |
1.44 |
.84 |
1.81 |
Payments were too low* |
.29 |
1.49 |
1.51 |
1.62 |
Against gov. spending* |
-.75 |
1.73 |
-.15 |
1.98 |
Against AE payments* |
-1.72 |
1.75 |
-1.74 |
1.68 |
Would adopt anyways* |
-1.14 |
1.63 |
-1.61 |
1.75 |
Would adopt chosen AES* |
1.03 |
1.14 |
-.2 |
1.73 |
Credibility * |
.81 |
1.28 |
-.44 |
1.68 |
Ability to adopt practices* |
.39 |
1.48 |
-1.12 |
1.61 |
Ability to achieve objectives* |
.39 |
1.46 |
-.84 |
1.64 |
Adoption puts profitability at risk* |
.23 |
1.56 |
1.42 |
1.65 |
Confidence in monitoring* |
-.28 |
1.70 |
-1.34 |
1.75 |
Notes
For statements “Farm environment” 1 to 8 see Table A C.5 for definitions.
For “Risk” and “Time” statements see Table A C.4 for definitions.
* Likert scale from -2 “Strongly disagree” to +2 “Strongly agree”
** Scale: 0: "None of the cultivated fields (less than 10% of arable land)",
1: "A minority of cultivated fields (between 10% and 49% of arable land)", 2: "A majority of cultivated fields (between 50 and 90% of arable land)", 3: “All cultivated fields (more than 90% of arable land)".
In bold: Variables included in clusters that significantly explain class membership.
Notes
← 1. In this chapter, results-based payments are based on modelled rather than measured environmental results for nutrient runoff and GHG emissions, given the fact that these are non-point source pollution, hence extremely difficult and costly to measure at field parcel level directly. In contrast, payments related to the biodiversity objective is based on measurable results
← 2. Other commonly-used methods include contingent valuations and best-worst scaling. According to Holmes, Adamowicz and Carlsson (2017, p. 134[3]), choice experiments (CEs) offer a number of advantages over other methods, and after consideration of other possible methods, the overall objectives of this project, and the available resources, the choice experiment method was selected.
← 3. In the context of this project, “attribute” refers to a specific policy design element: examples include environmental policy objectives, contract length, contract flexibility, payment basis, payment level, beneficiary eligibility conditions, monitoring and compliance design (e.g. monitoring frequency and type, penalties/sanctions, etc.), services provided (e.g. technical assistance). Level refers to the value(s) of the attribute that are tested in the experiment. For example, levels for the attribute of contract length could be 5, 10 and 20 years.
← 4. Additional details on the methods are shown in Annex 4.F.
← 5. Note that the results presented in this report hold when dropping individuals who answered that they strongly agreed with statements: (i) the survey was too complex or (ii) they had to ignore some of the attributes. However, the willingness to accept for the alternative specific constants (ASC practice-based, ASC hybrid and ASC results-based) are lower when dropping the individuals who thought the choices were too complex or stated they ignored some attributes, meaning that the overall willingness to accept of farmers for the different schemes are lower in this case. Importantly, the relative preferences between scheme designs and between attributes remain stable.
← 6. All models were estimated using the Apollo software on R (Hess and Palma, 2019[12]) (see also Hess and Palma, Apollo version 0.01, www.ApolloChoiceModelling.com.). A mixed logit model was also used, yielding similar results.
← 7. It is interesting to note that the increase in farmers’ marginal willingness to accept for both water quality improvement and climate change mitigation requirements is not linear, with a relatively lower payment requirements to go from a 25% emissions reduction to a 50% reduction than what is required on average for the first 25% of reduction.
← 8. Note that, in hybrid schemes, the share of payments conditioned on results on the choice cards presented to farmers only took values between 10% and 90%, so that the results are to be interpreted only within this range in hybrid schemes.
← 9. A first latent class model was estimated using all clusters to explain class membership. This leads to a loss of 1044 observations (174 respondents) due to missing values in the respondents’ characteristics. Farm characteristics and current AE practices were not significantly explaining class membership, so we removed these variables from the latent class model to increase the number of observations. The results are similar whether the clusters of farm characteristics and AE practices are included or not, so we only present the results of the model excluding them from the analysis (Annex Table 5.E.7), reducing the loss of observations to 426 observations (71 respondents) not included in the latent class analysis.
← 10. Note: An alternative specification for the utility functions of the three scheme designs were used to check the robustness of results. The results are presented in Annex 5.D and show that the results hold.