OECD Digital Education Outlook 2021
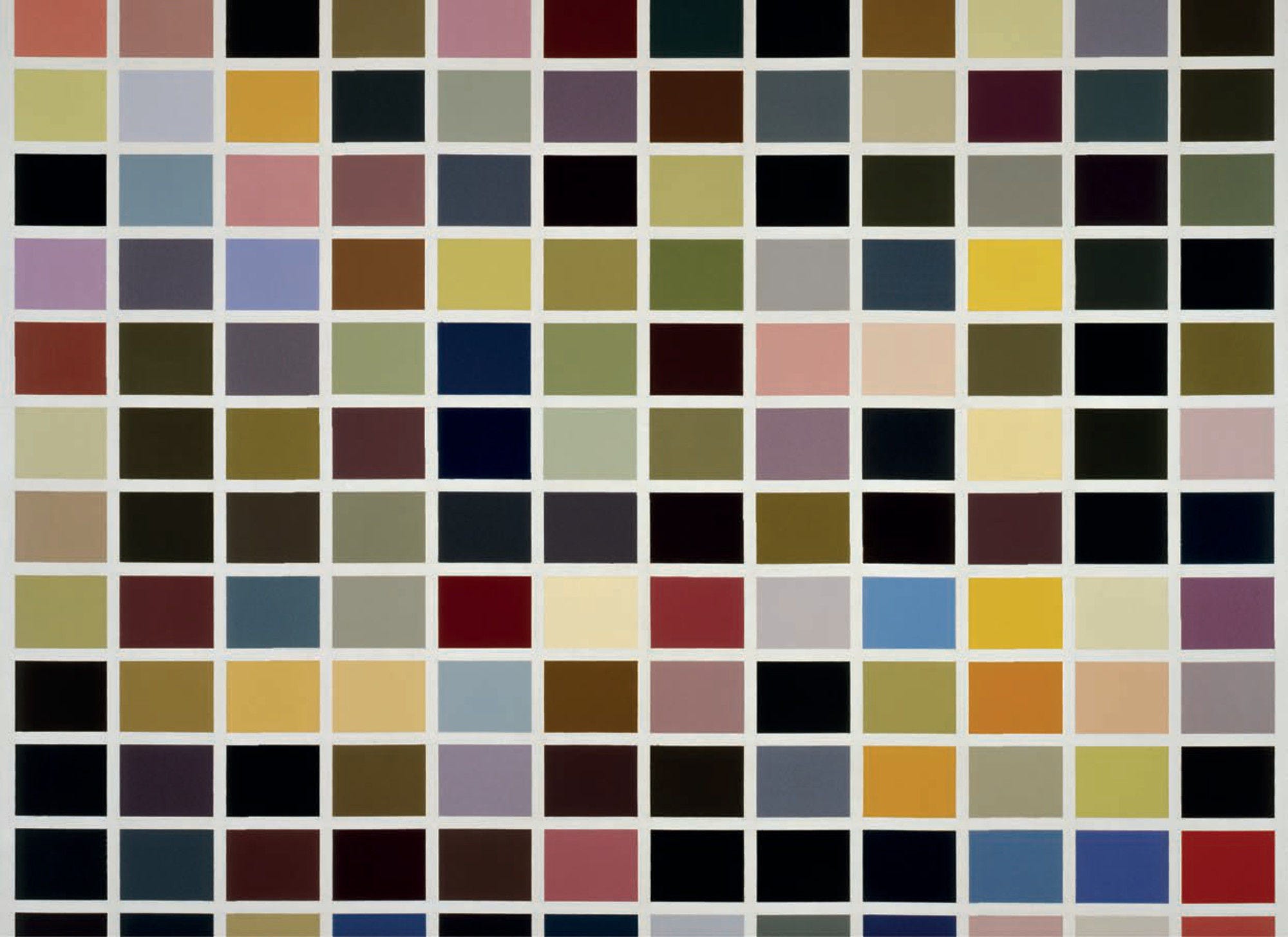
8. Learning analytics for school and system management
Dirk Ifenthaler
University of Mannheim, Germany and Curtin University, Australia
Introduction
With the increase in the amount of educational data, improved data storage and handling, and advances in computing and related analytics tools and algorithms, educational organisations are starting to embrace learning analytics. Learning analytics assess, elicit and analyse static and dynamic information about learners and learning environments for real-time modelling, prediction and optimisation of learning processes and environments, as well as educational decision making in an organisation (Ifenthaler, 2015[1]). To integrate learning analytics systems into educational organisations, actionable frameworks and adoption models are required (Buckingham Shum and McKay, 2018[2]; Dyckhoff et al., 2012[3]). However, these models may vary in different countries and organisations or within an individual organisation (Klasen and Ifenthaler, 2019[4]).
The potential benefits of learning analytics for educational organisations have been a topic of discussion for the past decade (Pistilli and Arnold, 2010[5]). However, there remains a lack of systematic and holistic adoption of learning analytics by organisations (Gašević et al., 2019[6]). The degree of adoption of learning analytics within an organisation is a measure of the number of stakeholders who use it or who have altered their practice because of it (Colvin et al., 2015[7]). For learning analytics to spread to more organisations, there needs to be communication between potential actors, who then follow a decision-making process as to whether to adopt or ignore it (Kotter, 2007[8]; Rogers, 1962[9]). Influencers who wish to promote the adoption of learning analytics must ensure and secure open communication channels and encourage people to start and stay on the journey of decision making from awareness to action (Colvin et al., 2015[7]; Drachsler and Greller, 2016[10]; Ifenthaler and Gibson, 2020[11]).
Higher education organisations have shown interest in adopting learning analytics but it is not yet a major priority (Ifenthaler and Yau, 2019[12]; Tsai and Gašević, 2017[13]; Lester et al., 2017[14]). Some have begun experimenting with dashboards for students and teachers but this is far from organisational transformation (Siemens, Dawson and Lynch, 2014[15]; Viberg et al., 2018[16]).
While studies on the adoption of learning analytics in higher education exist, the implementation of learning analytics in K-12 schools remains scarce (Andresen, 2017[17]; Gander, 2020[18]). There are two schools of thought on the usefulness of learning analytics for K-12 schools. Agasisti and Bowers (2017[19]) outline the importance of educational data and analytics for policy making, managing institutions, and classroom learning and teaching. However, Sergis and Sampson (2016[20]) argue that K-12 schools may not benefit as much from learning analytics as higher education organisations. K-12 schools require a holistic, multilevel analytics framework with several layers of data to produce sufficiently granular feedback to the school leadership and other stakeholders within the school while school analytics capture, analyse, and exploit organisation-wide educational data, allowing school leaders to monitor and (partially) influence their organisation’s developments in order to better meet the needs of the students, teachers, parents, and external policy mandates. Therefore, Sergis and Sampson (2016[20]) propose a school analytics framework that includes elements on a micro layer (learning process monitoring, learning process evaluation, learner performance monitoring, learner performance evaluation), on a meso-layer (curriculum planning, teaching staff management, teaching staff professional development), and on a macro layer (district stakeholder accountability, infrastructural resource management, financial resource management, learner data management). However, research on how state and local policies can leverage data analytics for school improvement is scarce (Jimerson and Childs, 2017[21]).
This chapter focusses on organisational benefits from learning analytics and the challenges of adopting learning analytics in educational organisations. Three case examples provide insights into how organisations have been successful in adopting learning analytics and producing organisational benefits or overcoming organisational hurdles. The conclusion presents guidelines for policy makers, researchers and educational organisations adopting learning analytics and ends with a set of open questions to be addressed in future research and practice.
Organisational benefits from learning analytics
Current learning analytics research and practice in Australia, the United Kingdom and the United States is proving its value in addressing issues related to successful studying and identification of students at risk (Sclater and Mullan, 2017[22]), and monitoring and improving organisational capabilities (Ifenthaler, Yau and Mah, 2019[23]).
Governance (mega-level) facilitates cross-organisational analytics by incorporating data from all levels of learning analytics initiatives. Based on common data standards and open data schemas, rich datasets enable the identification and validation of patterns within and across organisations and therefore provide valuable insights for informing educational policy making. Examples on the government level include performance compressions across institutions including benchmarking and helping to inform policy development and resource allocations across school districts, states or countries. In crises such as the COVID-19 pandemic, learning analytics at the governance level may enable rapid response and coordination of expert support for institutions in need.
At the macro-level, organisation-wide analytics enable a better understanding of learner cohorts to optimise processes. These include allocating critical resources to do things like reduce dropout rates, and increase retention and success rates. It can be used to support school admission processes and predict school performance (based on individual student performance). Other applications support transitions between educational systems such as entry into higher education or job-seeking processes. The meso- and micro-level provide analytics insights within organisations and will not be further discussed in this contribution (Ifenthaler and Widanapathirana, 2014[24]).
An essential prerequisite of learning analytics benefits is knowing what the data and analytics is being used for. These can be broken down to: (1) summative and descriptive – detailed insights after completion of a learning phase (e.g., study period, semester, final degree) often compared against previously defined reference points or benchmarks; (2) formative and (near) real-time, which uses on-going information for improving processes through direct interventions on-the-fly; (3) predictive and prescriptive, which forecast the probability of outcomes in order to plan for future interventions, strategies, and actions. Table 8.1 provides examples at the governance and organisational level for all data and analytics perspectives (Ifenthaler, 2015[1]). These benefits can be mapped to different data profiles (e.g., student profile, learning profile, curriculum profile) including various analytics indicators (e.g., trace data, demographic background, course characteristics). Yau and Ifenthaler (2020[25]) provide an in-depth analysis of analytics indicators for specific learning analytics benefits.
Table 8.1. Example of benefits from learning analytics for the governance and organisation level
Data and analytics perspective |
|||
Summative/Descriptive |
Formative/Real-time |
Predictive/Prescriptive |
|
Governance |
• Apply cross-institutional comparisons • Develop benchmarks • Inform policy making • Inform quality assurance processes |
• Increase productivity • Apply rapid response to critical incidents • Analyse performance • Provide expert help |
• Model impact of organisational decision making • Plan for change management |
Organisation |
• Analyse educational processes • Optimise resource allocation • Meet institutional standards • Compare units across programs and entities |
• Monitor educational processes • Evaluate educational resources • Track enrolments • Analyse churn • Involve alumni |
• Forecast educational processes • Project attrition • Model retention rates • Identify gaps |
Source: Ifenthaler (2015[1]).
Three examples
Though there is rich research on the organisational benefits of learning analytics, the implementation of organisation-wide analytics systems is scarce (Buckingham Shum and McKay, 2018[2]). The following three case examples showcase how learning analytics can impact school and system management.
Case example 1: Adoption of learning analytics at the University of Wollongong, Australia
The University of Wollongong, Australia, faced the challenge of adopting learning analytics at the organisational level of the Deputy Vice-Chancellor (Academic) while finding ways to integrate different disciplinary learning and teaching cultures and demonstrating its value to students and teaching staff (Heath and Leinonen, 2016[26]). The university began by considering the existing tools and resources that could support learning analytics. Despite a mature data warehouse infrastructure, it was necessary to invest in additional support staff to focus on analytics, big data, and statistics. The University of Wollongong chose the ‘blossoming’ adoption of learning analytics – as opposed to a phased up-scaling adoption, which usually begins with a prototype, and is followed by up-scaling before reaching the stage of fully implemented learning analytics (Ferguson et al., 2014[27]). Faculties varied quite widely in the degree to which they could carry out a ‘blossoming’ form of adoption. This added additional complexity to the organisational change process. In addition to technical capabilities, a survey was used to collect students’ views about learning analytics, including their preferences for functionalities, intervention strategies, and perceptions of privacy. Two governance committees were then formed: (a) the Learning Analytics Governance Committee, focussing on adopting learning analytics and (b) the Ethical Use of Data Advisory Group, focussing on student privacy and ethical issues regarding educational data (Heath and Leinonen, 2016[26]).
To conclude, four salient points shall be taken into consideration for a successful organisation-wide adoption (Heath and Leinonen, 2016[26]): (1) use common technological infrastructure such as a data warehouse; (2) involve students in all stages of the adoption process; (3) engage early adopters and establish communities of practice;
(4) institute governance frameworks focussing on learning analytics strategy, data privacy, and ethics.
Case example 2: Teachers’ Diagnostic Support System (TDSS) (Stuttgart, Germany)
Developed by researchers at the University Hohenheim in Stuttgart (Germany), the Teachers’ Diagnostic Support System (TDSS) helps teachers adapt their teaching practices to the variety of student needs in the classroom. The stakeholders are teachers and students. The TDSS’ collection and analysis of data are of particular interest. The TDSS allows data collection on (1) students’ personal characteristics (e.g. domain-specific knowledge and competencies, emotional-motivational characteristics), (2) descriptions of instructional characteristics (e.g. characteristics of the learning content), and (3) students’ learning experiences and learning progress (e.g. situational interest in the subject matter, actual knowledge about the topic) (Kärner, Warwas and Schumann, 2020[28]). Figure 8.1 provides an overview on the TDSS, which is a client–server-based software that is optimised for mobile devices.
TDSS allows the teacher to retrieve and analyse data during and after instruction to inform their teaching practice on-the-fly as well as prepare learning materials and future lessons. Micro-management through learning analytics may be expanded for cross-curricular teaching activities and school-wide diagnostic purposes.
Source: Kärner, Warwas and Schumann (2020[28])
Case example 3: The LAPS project using machine-learning techniques for early student support at the Stuttgart Media University, Germany
The LAPS (Learning analytics for exams and study success) project was developed to identify students at risk of failing their studies. It was implemented at the Stuttgart Media University, Germany in 2014. The purpose of LAPS was to create an evidence-based discussion with academic staff and students at an early stage in their studies. Ethical issues of privacy, voluntariness, self-determination, self-responsibility, respect of individuality, confidentiality and anonymity are essential to the project. LAPS data, which is updated each semester, is used to produce a list of participating students’ critical study progressions. It can identify more than 200 individual risk characteristics (see Figure 8.2) as well as study progressions with a high potential (Hinkelmann and Jordine, 2019[29]). In addition, LAPS is used for quality assurance, providing information about specific programmes, lectures and student cohorts. LAPS provides information on number of enrolled students, drop-outs, successful study progressions, average risk possibility, minimum/average/maximum student age, gender distribution, average grade of the university entrance qualification, and retreats from examinations for different programmes.
Source: Hinkelmann and Jordine (2019[29])
The LAPS lectures insights allow detailed analysis for each semester of lectures and provides access to distribution of grades, number of successful examinations, average grade, number of retreats, number of registrations. The LAPS cohorts view allows comparison of the distribution of students’ obtained ECTS (European Credit Transfer System) credits per semester. It also identifies possible structural problems when students do not achieve the required ECTS credits (Hinkelmann and Jordine, 2019[29]).
Learning analytics’ challenges for organisations
The three case examples above show some possible benefits of learning analytics in triggering organisational change, and supporting teachers and students within the classroom or during their studies. There are many other possible benefits but also implementation challenges. How can educational organisations invest limited resources so as to achieve maximum benefits? Tsai and Gašević (2017[13]) point out several challenges for organisations implementing learning analytics initiatives:
insufficient leadership in planning and monitoring learning analytics implementation and the organisational change process;
uneven understanding of and commitment to the initiative by stakeholders, namely, administrative, technical, and teaching staff;
a lack of pedagogical concepts and general awareness about the organisation’s learning culture driving the expected benefits for learning and teaching;
insufficient professional training for teaching staff, student service staff, technical staff and the like on the benefits and limitations of learning analytics or its technical infrastructure;
insufficient rigorous empirical evidence on the effectiveness of learning analytics to support organisational decision making;
not enough policies, regulations, and codes of practice on privacy and ethics in learning analytics.
Leitner, Ebner and Ebner (2019[30]) recommend a framework of seven categories for driving organisation-wide learning analytics initiatives. These include: (1) defining how students, educators, researchers and administrators will gain from learning analytics; (2) stakeholders having access to actionable information on dashboards;
transparent communication with all stakeholders and ensuring of policies – particularly, privacy – that align with the organisation’s core principles and others such as the EU’s General Data Protection Regulation; (4) setting up and managing an information technology (IT) infrastructure that supports the requirements of the learning analytics initiative. Such advanced IT infrastructures may be provided through organisation-owned services or external service providers; (5) a scalable development and operation of learning analytics functions that the organisation can monitor and evaluate; (6) implementing a code of conduct and (7) procedures on an ethics of learning analytics that adapts to different cultural contexts.
Drachsler and Greller (2016[10]) have compiled an eight-point DELICATE checklist to facilitate trusted implementation of learning analytics which includes many of the points in Leitner, Ebner and Ebner’s’s framework (2019[30]). To these, they add the need to legitimise or provide reasons for the right to obtain data, secure consent through a contract with the data subjects, anonymise data and subjects, and ensure that external stakeholders adhere to national guidelines.
A change management strategy begins with a readiness assessment. The initial step consists of identifying the to-be-achieved benefits of learning analytics. The educational organisation then carries out an in-depth review of existing practices, procedures, and capabilities (see Case Example 1). The ensuing strategy thus lays out which benefits and specific learning analytics features are included as well as which infrastructure is required to successfully implement learning analytics.
A readiness assessment is also conducted using standardised instruments. Data on organisational readiness such as existing policy and data protection regulation is collected with a specific focus on the organisation’s technical readiness (e.g. data warehouse, system integration) and the staff’s level of educational data literacy (Schumacher, Klasen and Ifenthaler, 2019[32]).
Source: Ifenthaler (2020[31])
After the readiness assessment, any resulting implementation strategy should encompass monitoring and evaluating the learning analytics with regard to predefined and measurable KPIs (Key Performance Indicators). More importantly, the return on investment, defined as the expected gains (returns) per unit of cost (investment) needs to be monitored closely (Psacharopoulos, 2014[33]). These may be monetary returns, but also other gains, such as student retention, staff improvement (see Case Example 2) and student satisfaction (Gibson et al., 2018[34]).
In sum, a change management strategy could be guided by the following principles (Ifenthaler, 2020[31]; Leitner, Ebner and Ebner, 2019[30]; Tsai and Gašević, 2017[13]):
definition of the learning analytics vision and objectives (e.g. using the abovementioned benefits matrix) and align them with the organisations mission and learning culture;
identification of organisational, political, or technological factors that will affect the implementation;
involvement and continuous information of all stakeholders including students, teachers, administrators, etc.;
development of (and continuously update) a strategic plan focussing on short-term and long-term wins, including a needs and risk analysis as well as a clear timeline outlining responsibilities of involved stakeholders;
allocation of resources and identification of expertise (inside and outside of the organisation) for achieving the learning analytics objectives;
undertaking of a robust formative and summative evaluation of the learning analytics initiative to further refine the overall implementation and organisational change process.
Moving forward with learning analytics
Many learning analytics initiatives either follow an action research-based (Argyris and Schon, 1974[35]) or a design-based research approach (Huang, Spector and Yang, 2019[36]). For example, at the macro-level, learning analytics insights help staff target retention initiatives (see Case Example 3). At the meso-level, data reports enable improved teaching practices (see Case Example 2). At the micro-level, ‘at-risk’ student lists are provided to student support staff, enabling them to triage and prompt individual students to take corrective action (see Case Example 3) or to cope with student heterogeneity in classrooms (see Case Example 2).
Despite these benefits, implementing learning analytics in educational organisations is often a paradoxical exercise. A research organisation that is contemplating learning analytics may have world-class experts in data science, information systems, management, educational leadership and learning science. These experts may even be contributing to the development of robust learning analytics systems. But that does not necessarily mean they have clear insights into the “political” dimension of what is required to implement learning analytics within their organisation. Or, even if they have expertise in organisation development, perhaps their organisation’s administration is not interested in it. Further, as bureaucracy takes over, these experts may not be interested in facilitating the change processes required.
In order to overcome such organisational barriers, Buckingham Shum and McKay (2018[2]) suggest the following:
The institution’s IT services develops and implements the learning analytics system as it already oversees the learning management system, data warehouse, student information system, etc. In this approach, however, the academic faculty is unlikely to guide an evidence-centred development and implementation of the learning analytics system. (b) Faculty members conduct evidence-centred research and development, and they use their findings to drive the implementation of a learning analytics system. (c) An autonomous entity of the higher education institution – well connected to faculty and administration – drives implementation, requiring partnership among all stakeholders. This innovative approach combines the strengths of the other two mentioned above.
In a systematic review that included over 6 000 studies on learning analytics over the past six years, Ifenthaler and Yau (2020[37]) indicate that greater adoption of organisation-wide learning analytics systems is needed (or at least research about it). Establishing standards may speed organisational take-up. While standards for data models and data collection such as xAPI (Experience API) exist (Kevan and Ryan, 2016[38]), standards for indicators, visualisations, and design guidelines that would make learning analytics pedagogically effective are lacking (Seufert et al., 2019[39]; Yau and Ifenthaler, 2020[25]). This is something learning analytics research and development needs to address. This may be accomplished with the following guidelines (Ifenthaler, Mah and Yau, 2019[40]):
develop flexible systems that can adapt to the needs of individual organisations, i.e., their learning culture, requirements of specific study programmes, student and teacher preferences, technical and administrative specifications;
define requirements for data and algorithms;
involve all stakeholders in developing a learning analytics strategy and implementation;
establish organisational, technological and pedagogical structures and process for the application of learning analytics systems as well as providing support for all involved stakeholders for a sustainable operation;
inform all stakeholders with regard to ethical issues and data privacy regulations including professional learning opportunities (e.g. educational data literacy);
build a robust process for ensuring the validity and veracity of the system, data, algorithms and interventions;
fund research on learning analytics;
constitute local, regional and national learning analytics committees including stakeholders from science, economics and politics with a focus on adequate development and implementation (and accreditation) of learning analytics systems.
Conclusion
Learning analytics draw on an eclectic set of methodologies and data to provide summative, real-time and predictive insights for improving learning, teaching, organisational efficiency and decision making (Lockyer, Heathcote and Dawson, 2013[41]; Long and Siemens, 2011[42]). While there is a great amount of attention on the ability of learning analytics to predict possible student failure, this has been for individual isolated courses rather than educational organisations in general (Gašević, Dawson and Siemens, 2015[43]). Additionally, not all learning analytics seem to be effective for learning and teaching, as demonstrated in Dawson et al. (2017[44]). The adoption of learning analytics in educational organisations requires capabilities not yet fully developed (Ifenthaler, 2017[45]). There have not been any wide-scale organisational implementation of learning analytics and therefore no empirical evidence that learning analytics improves the performance of educational organisations. International perspectives on adoption models (Nouri et al., 2019[46]) as well as on policy recommendations (Ifenthaler and Yau, 2019[12]; Tsai et al., 2018[47]) may help push this forward.
There are also important questions: Who owns the data that is available to teachers and learners? What data should be made available, and what data should remain private? Who analyses it and what is the data analysed for? What can teachers do with the data? What feedback and monitoring of learning might students expect from learning analytics? How can techno-led or -enabled assessments be used fairly and what are the risks associated with data use for assessing students’ achievements?
While considering the benefits of learning analytics for school and system management, the following developments may be implemented:
Learning analytics can be used to develop specialised curricula, aligned with job market demand, to better prepare students for their future careers. Examples of job market intelligence for supporting learning at the workplace already exist (Berg, Branka and Kismihók, 2018[48]). This may be only a small step away.
Learning analytics can facilitate course management and redesign of learning materials for flexible learning opportunities (Gosper and Ifenthaler, 2014[49]). This may also include adaptive and on-demand professional learning for educators.
Learning analytics applications can support a plethora of administrative tasks (Bowers et al., 2019[50]). Examples include budgeting, purchasing and procurement activities as well as facilities management. In addition, human resources management may better support the demands of individual educators. These systems can support flexible teaching and learning in or out of schools in the most economical way. They can help manage a variety of different curricular specialisations, class sizes, teaching staff, technologies and general demands of the learning environment regarding aspects such as rooms or even furniture.
Student applications and enrolment processes will best support student needs, and enable the school and teaching staff to micro-adapt the learning environment to students. Adaptive translation applications will support schools in communicating with parents and dealing with sick or absent students.
Policy makers are asked to develop and align policy that will encourage adoption of learning analytics for school and system management. This needs to be based on rigorous research findings, which are currently lacking. Research and development of trusted and effective learning analytics for school and system management should be encouraged.
In sum, policy makers, researchers and practitioners (Ifenthaler et al., In progress[51]) may consider the following strategies and actions:
Evidence-based practice led by analytics: Researchers need to gather more evidence from the use of learning analytics in order to develop systems that positively impact learning. Policy makers can then develop learning analytics policies that focus on leadership, professional learning, enabling mechanisms, and data governance with added confidence.
Promote the adoption of learning analytics: A readiness for cultural change sets the stage for acceptance and adoption, and helps guide the development of standards, principles and procedures by policy makers. These actions also address the challenge of updating principles and policies by engaging the impacted communities in the continual process of adapting and improving the organisational response to change.
Inform and guide data services providers and users: Trustworthy, ethical learning analytics practices are supported by policy mechanisms such as standards, accreditation processes, audits and evidence-based recommendations informed by practice. Researchers play a critical role here in promoting sustainability and scalability of policy and practice, for example, by producing the knowledge needed to effectively embed analytics and provide just-in-time data services that support good decision making focussed on learning. This strategy of wisely balancing investment in data services as well as users supports both the supply and demand sides of the flow of information, which accelerates adoption and positive change.
Impact learning via analytics tools: the priority for learning analytics should be optimising learning to achieve a more equitable and effective educational system and only, secondarily, accountability, testing, organisational change or financial efficiency. All stakeholders, including practitioners, researchers and policy makers, need new levels of data literacy to use this new tool and make use of the information learning analytics reveals.
The role of vendors in analytics solutions, adoption and implementation of analytics systems: There is a growing supply of commercial vendors of these systems for school management. Vendors, such as BrightBytes (https://www.brightbytes.net), develop solutions and show evidence in self-funded studies concerning the benefits of implemented learning analytics systems. Researchers should critically consider these commercial solutions but seek rigorous independent evidence of benefits. Policy makers may take stock of existing commercial solutions and their growing adoption in educational organisations.
References
Agasisti, T. and A. Bowers (2017), “Data analytics and decision-making in education: towards the educational data scientist [19] as a key actor in schools and higher education institutions”, in Johnes, G. et al. (eds.), Handbook of contemporary education economics, Edward Elgar Publishing, Cheltenham, UK.
Andresen, B. (2017), “Learning analytics for formative purposes”, in Tatnall, A. and M. Webb (eds.), Tomorrow’s Learning: Involving Everyone. Learning with and about Technologies and Computing. WCCE 2017. IFIP Advances in Information and Communication Technology, Springer, Cham.
[17]
Argyris, C. and D. Schon (1974), Theory in practice: Increasing professional effectiveness, Jossey-Bass, San Francisco, CA. [35]
Berg, A., J. Branka and G. Kismihók (2018), “Combining learning analytics with job market intelligence to support learning at the workplace”, in Ifenthaler, D. (ed.), Digital workplace learning. Bridging formal and informal learning with digital technologies, Springer, Cham.
Bowers, A. et al. (2019), Education leadership data analytics (ELDA): A white paper report on the 2018 ELDA Summit,
Teachers College, Columbia University.
Buckingham Shum, S. and T. McKay (2018), “Architecting for learning analytics. Innovating for sustainable impact”,
EDUCAUSE Review, Vol. 53/2, pp. 25-37.
Colvin, C. et al. (2015), Student retention and learning analytics: A snapshot of Australian practices and a framework for advancement, Australian Government Office for Learning and Teaching, Canberra, ACT.
[48]
[50]
[2]
[7]
Dawson, S. et al. (2017), “From prediction to impact: Evaluation of a learning analytics retention program”, in Molenaar, I., X. [44]
Ochoa and S. Dawson (eds.), Proceedings of the seventh international learning analytics & knowledge conference, ACM, New York, NY.
Drachsler, H. and W. Greller (2016), Privacy and analytics - it’s a DELICATE issue. A checklist for trusted learning analytics.
Dyckhoff, A. et al. (2012), “Design and implementation of a learning analytics toolkit for teachers”, Educational Technology & Society, Vol. 15/3, pp. 58-76.
Ferguson, R. et al. (2014), Setting learning analytics in context: Overcoming the barriers to large-scale adoption,
http://dx.doi.org/10.1145/2567574.2567592.
Gander, T. (2020), “Learning analytics in secondary schools”, in Peters, M. and R. Heraud (eds.), Encyclopedia of educational innovation, Springer, Singapore.
[10]
[3]
[27]
[18]
Gašević, D., S. Dawson and G. Siemens (2015), “Let’s not forget: Learning analytics are about learning”, TechTrends, Vol. 59/1, pp. 64-71, http://dx.doi.org/10.1007/s11528-014-0822-x.
Gašević, D. et al. (2019), “How do we start? An approach to learning analytics adoption in higher education”, International Journal of Information and Educational Technology, Vol. 36/4, pp. 342-353, http://dx.doi.org/10.1108/IJILT-02-2019-0024.
Gibson, D. et al. (2018), Return on investment in higher education retention: Systematic focus on actionable information from data analytics.
Gosper, M. and D. Ifenthaler (2014), “Curriculum design for the twenty-first Century”, in Gosper, M. and D. Ifenthaler (eds.), Curriculum models for the 21st Century. Using learning technologies in higher education, Springer, New York, NY.
Heath, J. and E. Leinonen (2016), “An institution wide approach to learning analytics”, in Anderson, M. and C. Gavan (eds.), Developing effective educational experiences through learning analytics, IGI Global, Hershey, PA.
Hinkelmann, M. and T. Jordine (2019), “The LAPS project: using machine learning techniques for early student support”, in Ifenthaler, D., J. Yau and D. Mah (eds.), Utilizing learning analytics to support study success, Springer, Cham.
Huang, R., J. Spector and J. Yang (2019), “Design-based research”, in Huang, R., J. Spector and J. Yang (eds.), Educational technology. A primer for the 21st century, Springer, Singapore.
Ifenthaler, D. (2020), “Change management for learning analytics”, in Pinkwart, N. and S. Liu (eds.), Artificial intelligence supported educational technologies, Springer, Cham.
Ifenthaler, D. (2017), “Are Higher Education Institutions Prepared for Learning Analytics?”, TechTrends, Vol. 61/4, pp. 366-371, http://dx.doi.org/10.1007/s11528-016-0154-0.
Ifenthaler, D. (2015), “Learning analytics”, in Spector, J. (ed.), The SAGE encyclopedia of educational technology, Sage, Thousand Oaks, CA.
[43]
[6]
[34]
[49]
[26]
[29]
[36]
[31]
[45]
[1]
Ifenthaler, D. and D. Gibson (2020), Adoption of data analytics in higher education learning and teaching, Springer, Cham. [11]
Ifenthaler, D. et al. (In progress), “Putting learning back into learning analytics: actions for policy makers, researchers, and [51]
practitioners”, Educational Technology Research and Development.
Ifenthaler, D., D. Mah and J. Yau (2019), “Utilising learning analytics for study success. Reflections on current empirical findings”, in Ifenthaler, D., J. Yau and D. Mah (eds.), Utilizing learning analytics to support study success, Springer, Cham.
Ifenthaler, D. and C. Widanapathirana (2014), “Development and Validation of a Learning Analytics Framework: Two Case Studies Using Support Vector Machines”, Technology, Knowledge and Learning, Vol. 19/1-2, pp. 221-240, http://dx.doi.org/10.1007/s10758-014-9226-4.
Ifenthaler, D. and J. Yau (2020), “Utilising learning analytics to support study success in higher education: a systematic review”, Educational Technology Research and Development, Vol. 68/4, pp. 1961-1990,
http://dx.doi.org/10.1007/s11423-020-09788-z.
[40]
[24]
[37]
Ifenthaler, D. and J. Yau (2019), “Higher Education Stakeholders’ Views on Learning Analytics Policy Recommendations for [12]
Supporting Study Success”, International Journal of Learning Analytics and Artificial Intelligence for Education (iJAI), Vol. 1/1, p. 28, http://dx.doi.org/10.3991/ijai.v1i1.10978.
Ifenthaler, D., J. Yau and D. Mah (2019), Utilizing learning analytics to support study success, Springer, New York, NY. [23]
Jimerson, J. and J. Childs (2017), “Signal and symbol: how state and local policies address data-informed practice”,
Educational Policy, Vol. 31/5, pp. 584-614.
Kärner, T., J. Warwas and S. Schumann (2020), “A Learning Analytics Approach to Address Heterogeneity in the Classroom: The Teachers’ Diagnostic Support System”, Technology, Knowledge and Learning, http://dx.doi.org/10.1007/s10758-020-09448-4.
Kevan, J. and P. Ryan (2016), “Experience API: Flexible, Decentralized and Activity-Centric Data Collection”, Technology, Knowledge and Learning, Vol. 21/1, pp. 143-149, http://dx.doi.org/10.1007/s10758-015-9260-x.
Klasen, D. and D. Ifenthaler (2019), “Implementing learning analytics into existing higher education legacy systems”, in Ifenthaler, D., J. Yau and D. Mah (eds.), Utilizing learning analytics to support study success, Springer, New York, NY.
[21]
[28]
[38]
[4]
Kotter, J. (2007), “Leading change: Why transformation efforts fail”, Havard Business Review, Vol. January, pp. 96-103. [8]
Leitner, P., M. Ebner and M. Ebner (2019), “Learning analytics challenges to overcome in higher education institutions”, in Ifenthaler, D., J. Yau and D. Mah (eds.), Utilizing learning analytics to support study success, Springer, Cham.
[30]
Lester, J. et al. (2017), Learning analytics in higher education, Wiley, Malden, MA. [14]
Lockyer, L., E. Heathcote and S. Dawson (2013), “Informing Pedagogical Action”, American Behavioral Scientist,
Vol. 57/10, pp. 1439-1459, http://dx.doi.org/10.1177/0002764213479367.
Long, P. and G. Siemens (2011), “Penetrating the fog: Analytics in learning and education”, EDUCAUSE Review, Vol. 46/5, pp. 31-40.
Nouri, J. et al. (2019), “Efforts in Europe for Data-Driven Improvement of Education – A Review of Learning Analytics Research in Seven Countries”, International Journal of Learning Analytics and Artificial Intelligence for Education (iJAI), Vol. 1/1, p. 8, http://dx.doi.org/10.3991/ijai.v1i1.11053.
Pistilli, M. and K. Arnold (2010), “Purdue Signals: Mining real-time academic data to enhance student success”, About campus: Enriching the student learning experience, Vol. 15/3, pp. 22-24.
Psacharopoulos, G. (2014), “The returns to investment in higher education”, in Menon, M., D. Terkla and P. Gibbs (eds.),
Using data to improve higher education. Global perspectives on higher education, Sense Publishers, Rotterdam.
[41]
[42]
[46]
[5]
[33]
Rogers, E. (1962), Diffusion of innovations, Free Press of Glencoe, New York, NY. [9]
Schumacher, C., D. Klasen and D. Ifenthaler (2019), “Implementation of a learning analytics system in a productive higher education environment”, in Khine, M. (ed.), Emerging trends in learning analytics, Brill, Leiden‚ NL.
[32]
Sclater, N. and J. Mullan (2017), Learning analytics and student success – assessing the evidence, JISC, Bristol. [22]
Sergis, S. and D. Sampson (2016), “School analytics: a framework for supporting school complexity leadership”, in Spector, [20]
J. et al. (eds.), Competencies in teaching, learning and educational leadership in the digital age, Springer, Cham.
Seufert, S. et al. (2019), “A pedagogical perspective on big data and learning analytics: a conceptual model for digital learning support”, Technology, Knowledge and Learning, Vol. 24/4, pp. 599-619,
http://dx.doi.org/10.1007/s10758-019-09399-5.
Siemens, G., S. Dawson and G. Lynch (2014), Improving the quality and productivity of the higher education sector - Policy and strategy for systems-level deployment of learning analytics. Canberra, Australia: Office of Learning and Teaching, Australian Government, http://solaresearch.org/Policy_Strategy_Analytics.pdf.
Tsai, Y. and D. Gašević (2017), Learning analytics in higher education - challenges and policies: A review of eight learning analytics policies.
[39]
[15]
[13]
Tsai, Y. et al. (2018), “The SHEILA Framework: Informing Institutional Strategies and Policy Processes of Learning Analytics”, [47]
Journal of Learning Analytics, Vol. 5/3, http://dx.doi.org/10.18608/jla.2018.53.2.
Viberg, O. et al. (2018), “The current landscape of learning analytics in higher education”, Computers in Human Behavior, Vol. 89, pp. 98-110, http://dx.doi.org/10.1016/j.chb.2018.07.027.
Yau, J. and D. Ifenthaler (2020), “Reflections on Different Learning Analytics Indicators for Supporting Study Success”, International Journal of Learning Analytics and Artificial Intelligence for Education (iJAI), Vol. 2/2, p. 4, http://dx.doi.org/10.3991/ijai.v2i2.15639.
[16]
[25]