Pierre Dillenbourg
École polytechnique fédérale de Lausanne, Switzerland
OECD Digital Education Outlook 2021
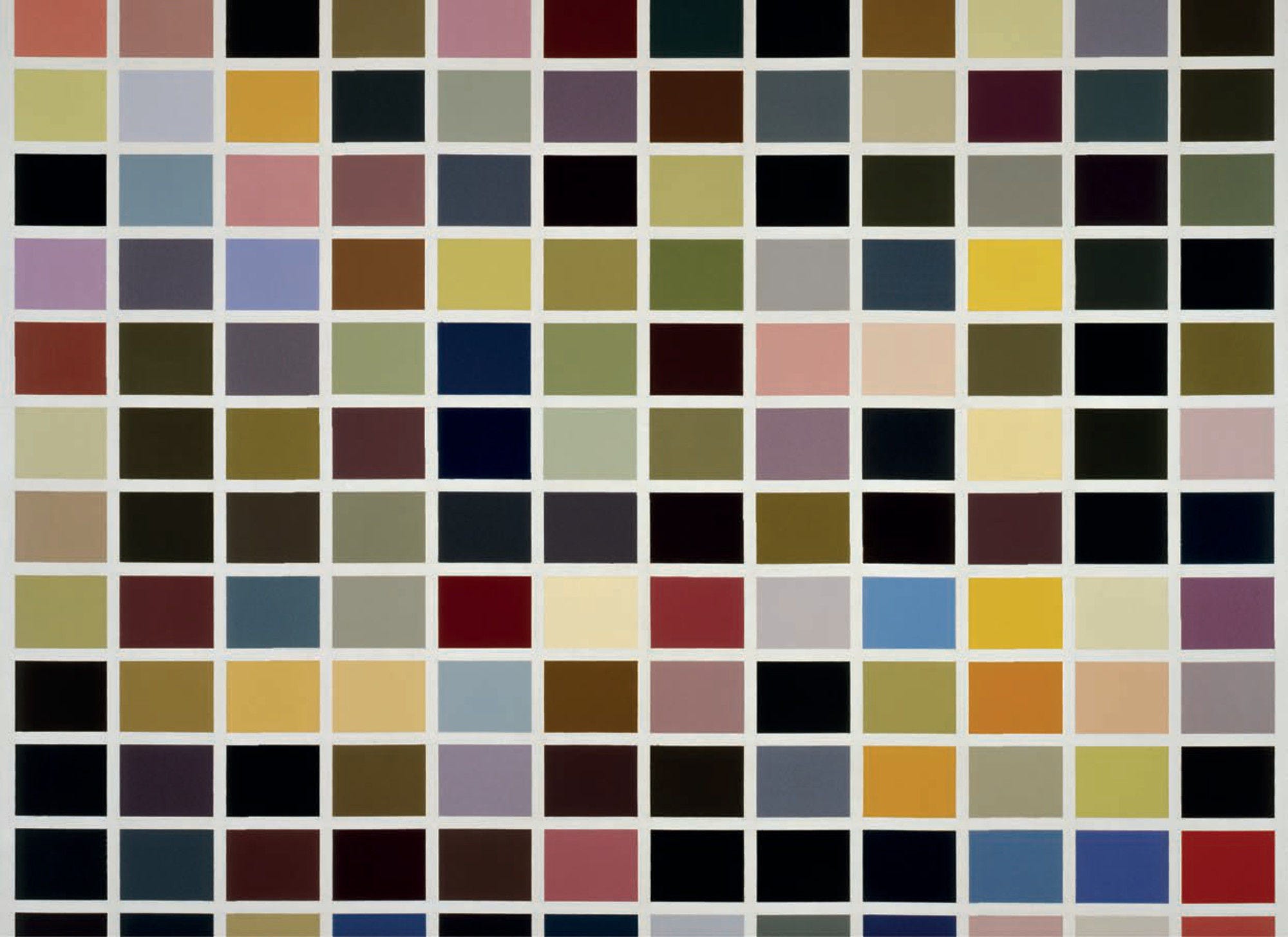
5. Classroom analytics: Zooming out from a pupil to a classroom
Abstract
The term “learning technologies” usually refers to the activities that one or a few learners perform on a digital device. How do technologies take into account the fact that a classroom has many more learners? Classroom analytics provide teachers with real-time support for class management: monitoring the engagement of learners in a class; deciding when and how to intervene in their learning activities; reusing the output from one activity in another one; forming student groups; integrating the learner’s production in a lecture; deciding when to shift to another activity; or helping teachers to regulate their own behaviour. This chapter describes the classroom itself as a digital system. It speculates on how this vision can come true, and what can already be learned from it, namely the critical role of teachers in the success of digital education.
Introduction
Learning analytics aim at modelling the learning process, i.e. how knowledge, skills and competencies are developed by learners while performing some activities. When these activities are run on computers, these models enable the software to adapt the next learning activities to the learner’s needs. What if 30 learners independently use this education software in the same classroom? Many interesting classroom events would then occur outside the software and be mostly ignored by analytics: the teacher’s interventions, discussion among peers, etc. Do students engage more with the system when the teacher passes nearby than when he/she is further away? What if the individual activity on computers is only a part of the lesson, in addition to teamwork and lectures? These activities, invisible to the software, will not be taken into account by the analytics even though they do actually matter in terms of how much learners actually learn.
This chapter broadens the scope of learning analytics, from modelling the learner’s interactions with the device to capturing anything that happens in this peculiar ecosystem called a classroom. Classroom analytics are multimodal, i.e. they collect relevant data with a variety of sensors in order to analyse conversation patterns, attention levels, body postures, etc. Some of these sensors are cameras, which immediately raises ethical issues. Balancing these risks with the potential benefits of classroom analytics is a core concern.
The benefits of classroom analytics should ideally occur in two steps. First, classroom analytics are designed to enhance the management of the classroom, for instance, by displaying a dashboard that shows the teacher which learners are struggling or that helps him/her to decide when to move on to the next activity. In the second step, improving classroom management is expected to lead to higher outcomes for the learners, as has been demonstrated by Do-Lenh et al. (2012[1]) as well Holstein, McLaren and Aleven (2018[2]). Another expected benefit of classroom analytics is to expand learning analytics to a rich diversity of learning activities such as lectures, teamwork, hands-on activities or collective discussions and even some activities outside the classroom, such as field trips. The management of such a rich pedagogical scenario, which includes individual, team and class-wide activities, some with and some without digital technology, is captured by the term “classroom orchestration”. Classroom analytics do not make decisions in place of teachers. Rather, they provide teachers with information for them to interpret as teachers, generally, are aware of context: for instance, a learner detected as poorly performing may actually be ill, helping another student or experiencing slow connectivity. There should always be a teacher in the loop; classroom analytics empowers her/him to teach more effectively.
This chapter looks at how learning analytics may transform education in the next decade. I hypothesise that a 2030 classroom will be visually similar to a 2020 classroom, just as the 2020 one is similar to the 1920 one. If a person who drove a car in 1920 resurrected in 2020, they would have difficulties driving a new car. They would neither recognise an induction cooker as a stove nor a smartphone as a telephone, but they would know when they entered a “classroom”. Education evolves slowly. Today’s teachers live in the “classroom of the future” of teachers who lived in the 1980s. Therefore, analysing how digital education has evolved over the past 40 years affords linear projections for the next 10‑20 years. Predicting that there will still be physical classrooms may sound conservative. Some would even argue classrooms will disappear. They forget an unpleasant reality: schools also fulfil a babysitting function, i.e. keeping kids busy while parents are at work. This chapter postulates that decision makers will still need to make choices about physical classrooms in 2030. Some initiatives entitled “classroom of the future” envision it as a cold space overloaded with individual screens and computers. This chapter proposes a different vision, in which classrooms remain rich social living places and not visually dominated by technological devices.
The vision: Classrooms as a digital system
Entering the classroom of tomorrow might be similar to getting into a car today. In both cases, one enters a physical space with doors, seats, windows, other people, etc., as well as many sensors, computers and actuators. Sitting in a car is much like being inside a digital system. It may sound scary but a digital system that sounds an alarm when the driver has nodded off has benefits that bypass the ethical concerns of video-monitoring the driver. Classroom systems face the same ethical trade-off.
A digital system captures (input), processes and communicates (output) information in a digital form. Since anything can be described as a system, why should we describe a classroom as a (digital) system? It emphasises the difference between the usual viewpoint which is that a classroom is a physical space where digital systems are introduced and the viewpoint whereby the classroom becomes the system.
As in any system, a classroom is composed of several subsystems and so forth. This set of subsystems can be described as a system if these subsystems collectively fulfil a function that none of them individually perform. A classroom may include several digital devices (e.g. software for mathematics, a spreadsheet, some educational robots, a teamwork platform, etc.) that each performs its specific function. Other cognitive functions are performed by the people in the room, the teacher and the learners, as well as by artefacts (e.g. a poster displaying the periodic table). The “classroom system” performs at a higher level function than these subsystems and one of these functions is classroom orchestration.
In personalised instruction systems, the input is the behaviour of learners, the function is adapting instruction for each learner and the output is the system’s next action, for instance, the feedback given or the next activity proposed. In the classroom-as-system approach, the input is the analytics collected in the classroom, the output is the information given to the teacher or to the learners, for instance, dashboards, and the function is the classroom orchestration. Holstein, McLaren and Aleven (2017[3]) observed the behaviour of teachers when their students used an intelligent tutoring system and found that teachers spent on average 47% of their time either inactive or outside the classroom. They simply felt “out of the loop”. In learning analytics, the vision is to keep one or more humans in the loop. We talk about the co-orchestration of the classroom by the teacher and the digital components of the system (Santos, 2012[4]).
How did we get there?
Looking at the evolution of learning technologies over the past 40 years, four trends led to the emergence of the concept of “classroom as a system”.
The first trend is the growing integration of pedagogical approaches that have for many years been considered as mutually exclusive. Many educational tools used in school are based on the “mastery learning” ideas (Bloom, 1968[5]): decomposing complex skills into simple ones by providing rapid feedback and incrementally practicing more complex skills. Another family of tools, called “micro-worlds” (Papert, 1987[6]), are some kind of digital sandboxes where learners acquire problem-solving skills by trial and error, reflecting constructivist theories. The same theory inspired inquiry-based learning tools, namely learning by running real or simulated experiments. “Instructionist” approaches inspired massive open online courses (MOOCs) and other environments where learners are mostly watching lectures or reading texts. These learning theories focus on individual learning, building on the ability to adapt instruction to the differences among learners. To the contrary, empirical studies revealed the benefits of collaborative learning, which gave rise to environments designed for learning in teams (Dillenbourg, Järvelä and Fischer, 2009[7]) based on social cognition theories (Vygotsky, 1964[8]). These oppositions are fading out. The human cognitive system is a social software running on an individual hardware, the brain. Why should a teacher bet on a single digital education approach when he/she can integrate several approaches where and when they are relevant?
The second trend is the growing compatibility between the technologies used in education. For many years, one could, for instance, not technically integrate a piece of software with math exercises and a video player. Web technologies contributed to the interoperability across almost any digital component. The possibility of technically integrating different tools converges with the first trend, the interest of integrating different pedagogical approaches. This evolution does not lead to the ultimate learning management system that offers all of the functions required for all learning activities, but rather to the development of ecosystems of digital tools, each having specific functions. Right now, interoperability among learning environments is still far from sufficient. Metadata standards (Duval, 2001[9]) have developed for exchanging digital contents (such as the Sharable Content Object Reference Model [SCORM] or Instructional Management System Learning Design [IMS LD]) and others, such as Learning Tools Interoperability (LTI), to foster interoperability, i.e. to exchange data about learners (Severance, Hanss and Hardin, 2010[10]). Today, the digital traces produced when learners use a tool are collected and aggregated into models specific to each tool they use. If they used multiple learning tools, no tool would have a comprehensive model of the learner. Recent projects (Mangaroska, Vesin and Giannakos, 2019[11]) aggregate data across applications in order to produce a synthetic account of the learners’ learning paths. A standard for sharing records across applications, xAPI (Bakharia et al., 2016[12]) seems to be gaining momentum. Of course, producing cross-platform analytics multiplies the risks regarding data protection.
The third trend concerns the evolution of hardware. For many years, dedicated rooms were equipped with computers, so-called “computer labs”, with rows of cumbersome boxes and vertical displays, making students hardly visible by teachers. Next, laptops started to enter genuine classrooms, then tablets and smartphones brought learning technologies to informal settings, from being seated on a sofa to walking in the forest. Nowadays, the diversity of devices that can be exploited in education has exploded with the integration of sensors and actuators in shoes, mugs, clothes, etc. – basically any object (the “Internet of Things”). Devices are becoming more present but less visible; they do not properly disappear, but are more in the background. The frontier between what is digital and what is not has been progressively blurred, as illustrated by the examples in Figure 5.1. Learners may physically manipulate tangible objects, tracked by sensors, combined with augmented reality. Physical-digital technologies expand the range of skills that can be practiced and assessed digitally, which is especially relevant for professional gestures taught in vocational education.
However, even if future classrooms become populated with digital components, they should not visually look like a NASA control room, fully packed with displays and devices. The classroom of the future may indeed appear close to being a technology-free room. Why not a room with wooden furniture and bright views of outside gardens? The more peripheral the digital tools, the less obtrusive they become for social interactions (dialogues, eye contact, etc.) and classroom orchestration.
Figure 5.1. The physical-digital blur

Note: Top left panel: Tangible interfaces enable students to interact by moving physical objects on a table. This simulation of a warehouse makes logistics principles more concrete for apprentices. Top right panel: Augmented reality combines real images of the environment with digital information displayed on a tablet or within head-mounted displays. This simulation of forces in roof structures provide future carpenters with an intuitive understanding of statics. Bottom panel: Educational robots are both physical and digital objects. This Cellulo robot provides learners with haptic feedback during various manipulative tasks.
Sources: Do-Lenh et al. (2012[1]); Cuendet et al. (2015[13]).
The fourth trend is to pay more attention to the learning activity than to the learning technology. Consider the example of educational robots used to learn how to code. Some robots may be more appropriate than others, but the extent to which children learn depends less on the robot’s features than on the activity that learners have to do with the robot. The same is true for MOOCs, augmented reality or virtual reality tools, and for any technology. The main variable of success is the ability to orchestrate rich activities in the classroom. Classroom orchestration refers to the real-time management of multiple activities under multiple constraints (Dillenbourg, 2013[14]). The multiplicity of activities refers to the integration of individual, team and class-wide activities with various digital environments as well as without any digital tool. The multiplicity of constraints emphasises the many practical aspects that shape teachers’ decision making: managing learning time, coping with learners that missed previous lessons or joined late, taking into consideration the physical space – for instance, shifting from teamwork to lectures, maintaining a reasonable level of discipline, minimising the teacher’s workload, etc. These constraints have somehow been neglected in scientific research but probably explain some difficulties in the adoption of learning technologies.
Classroom as the input
The rationale for expanding the input from usual keyboard and mouse to the whole classroom is that learner-software interaction traces only provide a partial (and limited) account of what is going on in a classroom. Even when learners are supposed to interact exclusively with a personal device, they actually often engage in “out-of-software” activities, some being on-task (e.g. asking the teacher for help), while others are off-task (e.g. chatting, surfing the web, daydreaming, etc.). Some will ask the teacher for help and conversely the teacher may intervene to nudge an inactive learner.
We propose the terms “classroom analytics” to emphasise that any event in the classroom may be captured and analysed for modelling the learning and teaching process. Holstein et al. (2017[3]) used a classroom replay tool for integrating these “out-of-software” interactions with the analytics produced by “in-software interactions”. Data can indeed be collected for any classroom activity, including those with light technologies. For instance, the so-called “clickers” or “personal response systems” aim at increasing engagement during lectures, as well as collecting data: 1) the teacher interrupts her lecture and asks a multiple-choice question; 2) learners individually select a response on a personal device; 3) their answers are collected and visualised on the teacher’s slides (output), enabling the teacher to give feedback and comment on frequent errors. Numerous variations of this scenario exist that allow for open questions, graphical questions, voting mechanisms, etc. In the peer instruction scenarios, between the individual answer phase (2) and the teacher’s feedback phase (3), students are asked to compare their answer with their neighbour’s answer and explain their choice. Fagen, Crouch and Mazur (2002[15]) collected robust evidence that this classroom scenario actually improves students’ grades on university physics exams. Box 5.1 presents another example that was piloted in Chile.
Box 5.1. Single Display Groupware: A low-cost tool to make student activity visible to teachers (Chile)
Single Display Groupware (SDG) is an example of classroom analytics that is potentially very attractive for schools in developing countries because it only requires minimal equipment. SDG allows multiple people to work and interact over a single display, with everyone controlling their own input device. One application of SDG for the classroom is “One Mouse per Child for Basic Math”, a software programme developed by researchers from Chile that only requires one PC, one projector, one mouse for the teacher and one mouse for each child participating in the activity (Alcoholado et al., 2012[16]). The programme integrates personalised learning in the classroom, which is particularly useful in contexts where a teacher has to manage a class with students at different levels.
As Figure 5.2 illustrates, the display – visible to everyone through the projector – contains individual cells, with the number of cells equal to the number of mice connected to the system. Students solve arithmetical exercises in their individual cells, moving to a new exercise when answering correctly and moving to the next difficulty level when solving multiple exercises correctly in a row. On the right of the screen, students are ranked based on the number of problems solved and difficulty level achieved. This informs them about their relative performance in the class, adding a competitive, fun element to the learning activity, and allows the teacher to identify students who are lagging behind. The teacher can freely move her cursor across the screen to help individual students, or switch the programme from practice to teaching mode to solve sample exercises and to explain math rules to the class. Results from an experimental evaluation in a primary school in Santiago using pre- and post-tests suggest that the programme has a positive impact on achievement, especially for low-achieving students.
Figure 5.2. One Mouse per Child for Basic Math

Note: Students solve math exercises in individual cells. Icons in the middle of the cells provide four types of feedback: correct, incorrect, pass to the next level, mouse inactivity. The panel on the right displays a ranking of students based on number of exercises solved and level achieved.
Source: Alcoholado et al. (2012[16]) (reproduced with permission)
Multimodal analytics (Ochoa and Worsley, 2016[17])) broaden the range of behaviours to be collected in classroom analytics. If a classroom is equipped with sensors, any gaze, gesture, body pause, stress level, etc. can be collected as input. If we consider that inside the smartphone of each students there are 15‑20 sensors already, every classroom is potentially equipped with hundreds of sensors. Ahuja et al. (2019[18]) combined microphones and cameras in classrooms in order to detect which learners raised their hand, what posture and speech behaviour they displayed, and then correlated these features with lesson effectiveness. Scholars such as Yanga et al. (2018[19]) developed algorithms to identify emotions from facial images. The input data are not only behaviours (e.g. answers asking a question), but what could be called “behavioural dust”, i.e. fragments of behaviour such as head rotation (Figure 5.3), a sigh or a gaze fixation. Taken individually, these fragments can hardly be interpreted; but aggregated over time or across learners, they eventually become meaningful. For instance, the approach taken by Raca, Kidzinski and Dillenbourg (2015[20]) was not to estimate the individual level of attention and then to average it over all students. Instead, they found a measure that could only be computed for a class: learners who pay attention to the lecture tend to rotate their head at the same time, simply because they are paying attention to a moving object, the teacher.
Figure 5.3. Estimating the average level of attention in a classroom by analysing head movements
Modelling an entire classroom is more complex than modelling interactions within a digital environment, in which correct and incorrect responses are often defined in advance. Some Bayesian Knowledge-Tracing variations integrated system-triggered instructional interventions (Lin and Chi, 2016[21]) into the model; one could also add the teacher’s interventions. The more a learning environment is complex and open, the less accurate predictions can be. This lower accuracy is, however, not a concern in a classroom situation since the computational method does not aim to take autonomous decisions, but to inform a teacher who then takes decisions. Such a system, combining artificial and human intelligence, is often referred to as “a human in the loop”. Classroom analytics want to keep a teacher in the loop.
Turning the classroom into an input device immediately raises an ethical red flag. In some projects, learners or teachers have been equipped with sensors (electroencephalography, skin conductivity, accelerometers, heart frequency, eye trackers, etc.). Placing cameras in a classroom is less intrusive, but does not comply with data protection principles. One way to be compliant would be that the system does not store images, but deletes them as soon as the relevant features have been extracted. Despite this solution, we believe that the risk of “Big Brotherisation” of schools remains high, and unacceptable in many cultures. As in many data protection debates, this risk has to be compared with the benefits, that is, the value of the output. According to Raca, Kidzinski and Dillenbourg (2015[20]), signalling to a teacher the sudden loss of attention can be useful for novice teachers or those who fail to keep their audience’s interest. However, the benefits are not always obvious. Many scholars such as Yanga et al. (2018[19]) developed algorithms to infer emotions from facial images. What should the system do if it detects a learner’s frustration? Strong negative feelings may hamper the learner’s motivation, but some moderate level of confusion may indeed motivate them to try harder (D’Mello et al., 2014[22]). Classroom input should be restricted to what can actually provide a clear added-value to learning and teaching, and based on theories that have ideally sufficient empirical evidence or very plausible theories of action.
Classroom as the output
In adaptive personalisation systems, the output of the learning analytics is usually a decision to adapt instruction to the needs of an individual learner. In classroom analytics, the output is some information given to the humans in the loop – teachers and learners – who may then take a decision. This information often takes the form of a teaching dashboard, i.e. a visualisation of the state of learners or the progress of learning in the classroom, beamed onto the classroom walls or presented in a display (usually a screen).
The design of these dashboards involves a usability challenge: providing teachers with information without increasing their cognitive load. Most dashboards developed so far are indeed overwhelming teachers with too many details. One solution is to develop “zoomable” interfaces, i.e. providing a global picture, with minimal information per learner, but allowing the teacher to get more detailed information for any learner. Moreover, the dashboard should not reduce the visual attention that teachers pay to the classroom. Various solutions have pros and cons: to show the dashboards on the classroom display provides teachers with permanent access to its information (see Figure 5.4), but the information – including personal difficulties – is also made public to the whole class; to display the dashboard on the teacher’s desktop preserves privacy but requires her to return to her desk; to display the dashboard on a tablet gives permanent and private access, but may be cumbersome; to display the dashboard on a head-up display (e.g. glasses) (Holstein et al., 2018[23]) provides both information while maintaining visual contact with learners and keeping the teachers’ hands free, but is not very natural. Other design dimensions concern the nature of the displayed data (e.g. the response contents vs. the score), the social level (e.g. individual, teams, class), etc. One design choice that matters for classroom orchestration in particular is the spatial mapping of the given information (does the position of John on the dashboard correspond to his physical location in the classroom?).
Classroom dashboards can be centralised (on a display), distributed (through several displays in the room) or ambient (just providing minimal information to teachers through distributed or centralised hints). This is another design choice that is important. Dashboards are generally centralised, but distributed dashboards also have their advantages for the orchestration of teaching and learning. Figure 5.4 shows a set of Lantern devices spread within the classroom: they constitute a distributed dashboard. Alavi and Dillenbourg (2012[24]) compared it with a centralised dashboard also visible to all, showing exactly the same information, and found that the centralised one tended to trigger competition among male students while the distributed one triggered some interactions among neighbouring teams.
Since the teacher’s visual attention is saturated by the elements he/she needs to monitor, one may exploit peripheral vision and provide teachers with an “ambient” dashboard. For instance, Tomitsch, Grechenig and Mayrhofer (2007[25]) displayed information on the ceiling. The teacher is, of course, not expected to look at the ceiling, but if the colour of the classroom ceiling suddenly darkens, she will notice it. Gellersen, Schmidt and Beigl (1999[26]) conveyed information by changing the intensity of various lights or by controlling the pumps of a table fountain. Peripheral vision does not convey precise information, such as a numerical value, but a global impression. The term “ambient computing” describes technologies that do not require focal attention but change some contextual or background components. Today, ambient computing is alien to education stakeholders, but it has a great potential to turn the entire classroom into a display. It relates to “modest computing” (Dillenbourg et al., 2011[27]), which emphasises that the design of these displays deliberately degrades the accuracy of information: if the average score of learners in the classroom is 75%, it can be conveyed by setting the colour of the wall at the back of the classroom (which teachers often face) with a nuance of blue that is not as accurate as displaying the number 75, but is permanently visible to the teacher. On the Lantern device (Figure 5.4, left panel), the teacher perceives, for instance, which team has been waiting more than another team, without knowing exactly how much. Similarly, on the Reflect table (Figure 5.4, right panel), the colour of the table area in front of each learner approximates their amount of speech, but does not provide an exact count. It may happen that a participant keeps the floor for a while simply because an overall introduction or a long explanation is required. It does also occur that some participants game the system by deliberately and meaninglessly over-speaking. In both cases, the participants are aware of the conversation that happened, they know what the table display corresponds to. This justifies the “human in the loop” approach that we previously emphasised: knowing the context allows humans to interpret the feedback (while computers could misinterpret it).
Figure 5.4.

Notes: Left panel: Regulating the assistant’s attention. During recitation sections, students communicate their progress to the teaching assistant by using a small device called a Lantern. The colour indicates which exercises they are working on, the number of LEDs indicate how long they have been working on this exercise. To ask for help, they push on the Lantern, which starts blinking slowly, then faster, revealing their waiting time. Right panel: Regulating dialogues in teamwork. A microphone array placed on the middle of the Reflect table captures the angle of the voices and thereby identifies who is speaking. The more someone speaks, the more the LEDs in front of him/her light up.
Source: Left: Alavi and Dillenbourg (2012[24]); Right: Bachour, Kaplan and Dillenbourg (2010[28])
While the previous examples rely on visual perception, Moher et al. (2010[29]) also exploited sound for a classroom simulation on seismology. Over a period of 6 weeks, 21 earthquakes were simulated in the classroom. A low-frequency rumbling sound was generated by a subwoofer and displays located in different parts of the room simulated seismographs, showing a continuously running strip chart recorder of ground vibrations. Then, during lessons dedicated to earthquakes, students analysed the seismograph waves in order to locate the earthquake epicentre inside the classroom and to mark it by hanging a Styrofoam ball from the ceiling whose colour indicated the magnitude of the earthquake. While science simulations in schools usually run inside computers, this simulation was embedded in physical space, i.e. the classroom was the output.
System functions
A digital system processes data between the input and the output, a typical example being to aggregate data over time or across learners. These processes implement the functions expected from the system. The overarching function of classroom analytics, classroom orchestration, is fulfilled by implementing some specific functions. Seven more specific functions help to understand the current possibilities offered by this approach: monitoring and intervention, data propagation, team formation, debriefing, timing transitions, teacher self-regulation and, orchestration as a whole.
Monitoring and intervention
The main function of classroom dashboards is to monitor the state of the learners in order to detect which learner is inactive or struggling, which teams do not collaborate well, which learner could help another one, etc. Why would a teacher benefit from this information when he/she can, in a glance, see what the learners located in the classroom are doing? There are several answers to this question: when the number of learners is very high; when the learners’ activities are not easily seen by the teacher (e.g. working on laptops); when the student’s activity cannot be assessed at a glance (e.g. when they are writing complex code); when direct observation is intractable (e.g. monitoring 15 teams of 2 learners); when what matters is not only the current state, but what learners have done since the lesson outset, etc. In a nutshell, the key functionality of system is to make visible what is invisible, e.g. how long a learner has been silent, how much a learner dominates his teammates in a group discussion, etc.
Figure 5.5 illustrates this principle. Four teams are using the tangible logistics simulation tools shown in Figure 5.1. The four lines in the top panel show the history warehouse layouts designed by each team, which helps the teacher to perceive their strategy. Experiments carried out by Do‑Lenh et al. (2012[1]) showed that the pairs who modified the warehouse layout without much reflection and frequently ran the simulation did not learn much. Therefore, the dashboard includes the colour bar below the history which records the frequency of the warehouse manipulations, from yellow to red (too many manipulations). Students moving plastic shelves on the table is visible, but the variations of frequency of these movements for four teams is not visible.
For this function, the data processes are aggregation and evaluation. Aggregation consists of cumulating answers or behaviours over time and over teams to provide teachers with visualisations (timelines, histograms, etc.) that restore the “at a glance” effect. Simple evaluation processes compare the aggregated data to some thresholds (e.g. more than 5 minutes’ idle time; less than 30% correct responses) or use a colour code from least to most desirable as in Figure 5.5. More sophisticated evaluation methods are, for instance, code synthesis (e.g. highlighting incorrect lines in the code written by learners) and text processing (e.g. finding similarities between texts). The goal is not to intervene in place of the teacher, but to trigger an alert for the teacher, an invitation to pay attention to someone or something. For instance, in Figure 5.5, when the colour bar includes many red periods, the teacher may pause the simulation and ask teams to predict the outcomes of the next simulation before restarting it. This triggers reflective effort. As we previously observed, teams with fewer reflection phases achieved lower learning gains.
Figure 5.5. Teaching dashboard of a logistics lesson
A plausible hypothesis is that, by supporting teachers’ monitoring and intervention for learning tasks and processes that they might otherwise not perceive, dashboards would lead to higher learning outcomes. Evidence supporting this assumption is not abundant at present. In the previous example, Do‑Lenh et al. (2012[1]) showed that using the dashboard actually led to higher learning gains, but as the dashboard was combined with other orchestration tools, they may not be due to the dashboard itself. The state of research on teaching dashboards still has to mature. Schwendimann et al. (2017[30]) analysed 55 publications on this topic; only 15 included an evaluation in authentic contexts, the majority being based on questionnaires to teachers or learners. Only four of these papers actually measured the effects on learning. A more robust piece of evidence came from an experiment with 286 middle-school students: Holstein, Mc Laren and Aleven (2018[2]) showed that head-up display dashboards actually led to better orchestration which, in turn, increased learning gains for students using an intelligent tutoring system in mathematics. It is very interesting to zoom in on the relationship between providing a dashboard and the increase of learning gains. Holstein, McLaren and Aleven (2019[31]) observed (Figure 5.6) that the use of the dashboards led teachers to change their time allocation and pay more attention to weaker students, while it was the other way around without the dashboard.
Figure 5.6. Use of a dashboard to increase learning gains

Notes: The teachers with the dashboard displayed on their glasses (red line) pay more visual attention (vertical axis) to weaker students, i.e. low pretest score (left end of the horizontal axis), than teachers without glasses (control group) or with inactive glasses (placebo group). Shaded regions indicate standard errors.
Source: Holstein, McLaren and Aleven (2019[31])
Data propagation
Another orchestration function supported by classroom dashboards and analytics is to feed an activity with the data produced into a different activity. Some examples include:
1. During the first activity, Teams A and B each invent a small math problem. In the next activity, A solves B’s problem and vice versa. The data process is simply to rotate the problem statements across the teams.
2. First, students are invited to enter the country of birth of their grandparents. In the next activity, the teacher shows a map that visualises the migration flows over two generations. The data process is to aggregate and visualise the individual data.
3. Learners collect pictures of mushrooms during a trip to the forest. In the next activity, they work in teams to classify the set of pictures collected by the class. The data process is to aggregate all of the pictures, but it could also include automatic picture annotation from existing mushroom libraries.
The list of examples is infinite and only bound by teachers’ imagination. The “data propagation” function refers to a learning situation where an activity produces objects (or data) that are processed by an operator to feed into a subsequent activity. For physical objects, the operator is physical: in the first example, Teams A and B could simply exchange a sheet of paper. For digital objects, Dillenbourg (2015[32]) proposed a taxonomy of 26 operators that connect 2 or more learning activities. This flow of data across activities, referred to as a workflow, enables rich pedagogical scenarios. It can also create some rigidity, for instance if one team drops out. One challenge is to develop flexible workflows that enable teachers to fix on-the-fly the unexpected events that inevitably populate classroom life.
The two next subsections highlight two specific cases of data propagation that are especially relevant for classroom orchestration: team formation and debriefing.
Team formation
A specific function of classroom analytics is to process the data produced by learners in one activity in order to form dynamic teams for a subsequent activity. This function can be illustrated with an often tested pedagogical scenario that scaffolds cognitive conflict between peers (Dillenbourg and Jermann, 2007[33]). It is inspired by socio-constructivist theories that predict that the interactions necessary to overcome a cognitive conflict enhance learning (Doise, Mugny and Perret-Clermont, 1975[34]). In the first activity, each student responds to an online multiple-choice questionnaire. The questions do not have right or wrong answers, but reflect different viewpoints. For each answer, the students have to write a few words justifying their choice. In the second activity, the system produces a specific dashboard, a map of opinions (Figure 5.7, left panel): every answer in the first activity has been associated with an x,y value on the map. The teacher discusses this dashboard with students, who often comment on their positions. The system forms pairs of students in a way that maximises their distance on the map; that is, it finds students whose responses reveal opposite opinions. In the third activity, pairs are asked to answer the same online questionnaire as in the first activity. The environment provides them with the answers and justifications provided individually. In the fourth activity, the teacher uses another dashboard (Figure 5.7, right panel) for the debriefing activity (see Debriefing).
Figure 5.7. Using dashboards to form dynamic teams

Notes: Left panel: The map represents the learners’ opinions and the lines indicate how individuals with different opinions have been paired. Right panel: the pie charts indicate how they change opinions between the individual and the collaborative activities, which the teacher exploits during the debriefing activity.
Source: Dillenbourg and Jermann (2007[33])
The data operator used for this function consists of maximising differences within teams. This is also the case in the example of Gijlers and De Jong (2005[35]) on learning from simulations: they form teams of individuals who previously expressed an opposite hypothesis in order to reduce the natural bias of designing experiments that confirm one’s own hypothesis.
Another pedagogical scenario could use an operator that minimises the difference among team members, e.g. making teams of learners who made the same error in the previous exercises. Group formation is an example of data propagation in which the data fed into an activity are not the object of the activity, but its social organisation.
Debriefing
The dashboard presented in Figure 5.5 includes a tool (bottom part) that enables the teacher to select two warehouse layouts designed by teams and to compare them in terms of capacity and performance. This allows the teacher to debrief the exploration activities of his/her students. Debriefing means reflecting on what has been done in order to extract concepts or principles to be taught. By comparing warehouses, the teacher will, for instance, illustrate the trade-off between capacity and performance. The dashboard on the right in Figure 5.7 is used by the teacher to push students to explain why they change opinions between the individual and collaborative phases in order to later connect their explanations to the scientific debate.
The role of debriefing activities is a critical orchestration phase in constructivist learning scenarios, based on discovery or open problem-solving activities. These approaches have been criticised by some for being unproductive per se. However, Schwartz and Bransford (1998[36]) or Kapur (2015[37]) showed that, if this phase of exploration is followed by direct instruction, the lesson is actually more effective than the opposite sequence, i.e. if direct instruction is followed by application exercises. The reason is that, during the exploration activity, learners rarely shout “eureka”. More commonly, they get some intuition, some vague ideas, on the basis of which the concepts can later be clarified. They ask themselves questions that will give meaning to the teachers’ lecture. As Bransford and Schwartz (1998[36]) put it, there is a “time for telling”, but this instruction phase has to build on what learners have done during the exploration phase. It should not be a standard lecture disconnected from their experience. This is a very demanding activity for teachers, as it includes improvisation. The “debriefing” function of classroom analytics aims to support this task by collecting the learners’ productions, comparing them, annotating them, etc., and facilitating their exploitation by the teacher.
Timing transitions
Orchestrating learning within classrooms is a very time-constrained process. Teachers permanently compare the remaining class time to the pedagogical activities to be done. In addition, orchestrating rich learning scenarios is difficult while transitioning between activities involving different social levels: individual activities, team activities or class activities, referred to as “social planes”. A typical trade-off is as follows: the teacher had planned to devote 15 minutes to an individual exercise to practice some skill and this skill is required for the next activity, conducted in teams. After 15 minutes, the teacher realises that some learners have not finished their individual exercises. If he/she decides to start the next activity anyway, these late students and their teammates will be penalised. If he/she gives late individuals five more minutes, he/she will have to reduce the time budget of the next activity, and moreover, will have a majority of students wasting time and engaging in off-task interactions. Similar constraints arise when, as in the examples given in section Team formation, individual answers need to be provided in order to make automatic group formations.
One example of classroom analytics that addresses this issue is the “time extension gain”, i.e. the percentage of additional students who would complete the activity if its duration was extended by one single time unit. On the progression chart presented in Figure 5.8, the “time extension gain” corresponds to the slope of the curve (Faucon et al., 2020[38]). When the curves become flat, it is time to move to the next activity. This chart has been used in real time for orchestrating a variety of activities in lecture theatres. Future dashboards are expected to provide more instances of similar time prediction tools, thus supporting teachers to introduce short activities without wasting time.
Figure 5.8. Progression chart of the time extension gain

Notes: When students are engaged in an activity composed of multiple steps, this dashboard display shows the percentage of students who are doing the activity (blue) and the percentage of students who have completed the activity (red). The vertical bar is the moment when the snapshot was taken. The plain lines on the left are based on observed data while the dotted lines on the right are predictions based on data collected in previous experiments. In this example, the teacher could move on after approximately 300 seconds, when about 95% of the class has completed the activity. Three additional minutes (stopping at 480 seconds) would allow virtually all students to complete the activity, but 95% of the class would have wasted time.
Source: Faucon et al. (2020[38])
Teacher self-regulation
Since the input of classroom analytics can be any event in the classroom, classroom analytics can also capture the teacher’s behaviour. So far, learning analytics have not often included the teacher in their analysis since they typically analyse behaviours in a learning environment where the teacher has no or few possibilities of intervening. On the contrary, modelling classroom processes requires modelling the teacher’s behaviour, since the teacher plays a critical role in the teaching and learning processes: how much does the teacher speak, did they distribute verbal attention to all learners, how do they decide to whom they will ask a question, did they walk across the classroom, how varied is the tone of their voice?
For instance, classroom analytics may make a teacher realise that he/she has been speaking much longer than they planned to or that he/she has been neglecting some learners. This enables real-time self‑regulation, what Schön (2017[39]) called “reflection-in-action”, which is cognitively demanding. More sophisticated analytics can also (or alternatively) be provided after the lesson, supporting “reflection-on-action” that enables the teacher to reflect later on in order to improve his/her teaching over time – a powerful form of professional development.
Prieto, Sharma and Dillenbourg (2015[40]) combined eye-tracking measures and personal questionnaires to estimate the orchestration load of teachers in a classroom. They found that high-load episodes occur when teachers provide explanations or questions to the entire class, often looking at students’ faces in an attempt to assess their progress and understanding, which confirms the relevance of dashboards that provide such information. On the other hand, low-load episodes tended to correspond to individual or small group feedback, during which the teacher often focuses on the students’ worksheets or laptops. By combining eye-tracking measures with other sensors (electroencephalogram, accelerometers, etc.), Prieto et al. (2016[41]) applied machine-learning methods (random forest and gradient-boosted decision trees) automatically characterise the ongoing learning activities. The lesson was following an orchestration graph composed of only two planes: team and class activities. In Figure 5.9, the colour represents the teacher’s activity. The algorithm identified the plane of interactions with an accuracy of 90% but was less accurate, only 67%, for identifying the teacher’s activity based on those digital observations.
Now, any observation tool designed for teacher professional development may quickly drift into a teacher control or evaluation tool. The recommendation for this other touchy ethical issue is to strive for minimalism (capturing only information that may improve teaching) and to trust regulation and education stakeholders’ self-regulation (showing data to the teachers only and not to the school principals, other people in their hierarchy, parents or students).
Figure 5.9. Progression chart of the time extension gain

Note: These simplified orchestration graphs represent the activities that actually happened during the lesson (upper panel) and those identified by the teaching analytics algorithm (lower panel).
Source: Prieto et al. (2016[41])
As for learning analytics, classroom analytics focusing on teaching are useful if they help teachers reflect on their practice in order to improve it. In another study, Prieto et al. (2017[42]) showed teachers their location in the classroom. One teacher, whose classroom locations during a class are represented in Figure 5.10, was indeed surprised to discover that she neglected the right-hand part of the classroom and offered her support mainly to the left-hand side and middle tables (when not at her desk). This behaviour is not problematic in itself: perhaps students sitting at these tables required more support than those on the right-hand side of the classroom. However, this is a good illustration of how classroom analytics can help teachers identify some characteristics or possible issues in their teaching practice – for example, if they show her that she mainly supports students who are strong academically rather than those who struggle, that she ignores her female students during science lessons, or students with minority or underprivileged backgrounds, etc.
Figure 5.10. Showing teachers their location in the classroom
Orchestration
Classroom analytics aim to facilitate the orchestration of learning activities, which is not a single function, but an umbrella concept that includes many functions. Six of them have been described in the previous subsections. Basically, classroom analytics are designed to empower teachers in the demanding task of conducting rich scenarios; with individual, classroom and class-wide activities; with or without digital tools; considering all of the practical constraints of daily classroom life. The idea of empowering teachers to better orchestrate learning in their classroom can be viewed as provocative in times when many scholars define the teacher’s role as being a facilitator or a guide on the side. Empowering teachers for a better orchestration of learning does not mean increasing lecturing time; it is about supporting them in steering rich learning scenarios, whatever their actual components. Implementing constructivist learning scenarios with 30 learners requires the teacher to feel comfortable with driving the variety of pedagogical activities they include.
Technology can help in different ways. Or not. For example, a typical mistake is to suddenly distribute a tablet to every child in a classroom. This may destroy teachers’ established orchestration habits. What should the learner do? How can the teacher get their attention? Most initiatives to massively introduce technologies in education have failed because the hardware availability was not the bottleneck. The key is to provide teachers with scenarios describing which learning activities they can ask their students to do with technology. The answer is not one or two specific activities, but scenarios that include multiple activities, with or without technology, and embrace the whole classroom life. This is the educational proposition of classroom analytics – a new way to empower teachers in their classroom.
Perspectives
This chapter presents a new vision according to which a future classroom could be viewed as a digital system. It proposed the term “classware” to describe such a digital system which captures and supports classroom processes. This is a concept for the years to come. Classrooms are not digital systems yet, and very few classroom analytics today could be described as “classware”. The chapter developed a graphical language to model-integrated pedagogical scenarios, called “orchestration graphs” (Dillenbourg, 2015[32]), as a first step to modelling the flow of data required by classroom orchestration.
Coming back to the car example – or perhaps the “connected home” idea, classrooms within schools could look apparently very much the same as today, but they could be equipped with sensors feeding learning analytics and digital tools that would not only help teachers to orchestrate rich learning scenarios during class, but would also give them feedback on their teaching in real time, and food for thought for improving their teaching, and, as a result, students’ learning outcomes. Before this new schooling model emerges, research and development on classroom analytics and on better understanding the types of dashboards that would make the display of information the most helpful to teachers has to continue. The ethical and privacy issues these developments may raise also need to be addressed.
There are several immediate implications of this vision though.
First, this vision is a thinking tool for decision makers when designing or assessing educational projects. They should favour projects that do not bet on a single pedagogical approach or a single technology. Rather than projects that focus, for instance, on teamwork but neglect the need for individual practice, they should support projects that integrate individual activities, team activities and class-wide activities into consistent pedagogical scenarios. Good development projects cannot be defined by a technology only either, e.g. “virtual reality for X” or “3D printers for Y”. The belief that technologies have intrinsic effects have been proven wrong many times. A promising school or education research project should be defined by pedagogical goals that drive the necessary sequence of classroom activities, whatever technology is supporting these activities and their orchestration at this point only. Technology can help individual learning, but it can also help and empower teachers to mix learning scenarios including activities with no technology, activities carried out with or without technology, or fully digital activities. All of those scenarios are possible, and none is intrinsically superior to the others.
Second, this proposition implies that the design of learning technologies should embed these classroom orchestration functions. Schools will fully exploit the potential of digital technologies only when teachers feel empowered and confident in using them. This will not come rapidly, but could come with the help of technology. The education technology (EdTech) market is currently structured by competition between various tools, while the integration of digital tools is the condition to build digital ecosystems – and a sustainable EdTech market.
Third, all teacher training programme courses should include some learning on digital learning technologies. Currently, they often include one or two courses on that topic, but digital technologies can and should support any type of teaching.
Finally, policy makers and other education stakeholders have to address regulatory as well as ethical issues regarding classroom analytics. Everything that can be done within a regulatory framework is not necessarily desirable. Greater collaboration between researchers in learning sciences and in data protection should occur and inform regulation and ethical practice.
The road for learning technology and classroom analytics to reach their maturity is still long. But we may see the light at the end of the tunnel much sooner than many expect.
Acknowledgements
The presented technologies were developed by many current or former lab members: Jennifer Olsen, Patrick Jermann, Stian Haklev, Louis Faucon, Luis Prieto Santos, Son Do‑Lenh, Sébastien Cuendet, Guillaume Zufferey, Hamed Alavi, Khaled Bachour and Fredéric Kaplan. The development of a research community around orchestration benefited from interactions with Frank Fischer, Miguel Nussbaum, Yannis Dimitriadis, Manu Kapur, Nikol Rummel, Vincent Aleven and Chee Kit Looi. The author would also like to thank the colleagues who reviewed this chapter (Ryan Baker and Stéphan Vincent-Lancrin).
References
[18] Ahuja, K. et al. (2019), “EduSense”, Proceedings of the ACM on Interactive, Mobile, Wearable and Ubiquitous Technologies, Vol. 3/3, pp. 1-26, https://doi.org/10.1145/3351229.
[24] Alavi, H. and P. Dillenbourg (2012), “An Ambient Awareness Tool for Supporting Supervised Collaborative Problem Solving”, IEEE Transactions on Learning Technologies, Vol. 5/3, pp. 264-274, https://doi.org/10.1109/tlt.2012.7.
[16] Alcoholado, C. et al. (2012), “One Mouse per Child: interpersonal computer for individual arithmetic practice”, Journal of Computer Assisted Learning, Vol. 28/4, pp. 295-309, https://doi.org/10.1111/j.1365-2729.2011.00438.x.
[28] Bachour, K., F. Kaplan and P. Dillenbourg (2010), “An Interactive Table for Supporting Participation Balance in Face-to-Face Collaborative Learning”, IEEE Transactions on Learning Technologies, Vol. 3/3, pp. 203-213, https://doi.org/10.1109/tlt.2010.18.
[12] Bakharia, A. et al. (2016), “Recipe for success”, Proceedings of the Sixth International Conference on Learning Analytics & Knowledge - LAK ’16, https://doi.org/10.1145/2883851.2883882.
[5] Bloom, B. (1968), “Learning for mastery: Instruction and curriculum”, Regional Education Laboratory for the Carolinas and Virginia. Topical Papers and Reprints., Vol. 1, https://files.eric.ed.gov/fulltext/ED053419.pdf.
[13] Cuendet, S. et al. (2015), “An integrated way of using a tangible user interface in a classroom”, International Journal of Computer-Supported Collaborative Learning, Vol. 10/2, pp. 183-208, https://doi.org/10.1007/s11412-015-9213-3.
[22] D’Mello, S. et al. (2014), “Confusion can be beneficial for learning”, Learning and Instruction, Vol. 29, pp. 153-170, https://doi.org/10.1016/j.learninstruc.2012.05.003.
[32] Dillenbourg, P. (2015), Orchestration Graphs, EPFL.
[14] Dillenbourg, P. (2013), “Design for classroom orchestration”, Computers & Education, Vol. 69, pp. 485-492, https://doi.org/10.1016/j.compedu.2013.04.013.
[7] Dillenbourg, P., S. Järvelä and F. Fischer (2009), “The Evolution of Research on Computer-Supported Collaborative Learning”, in Technology-Enhanced Learning, Springer Netherlands, Dordrecht, https://doi.org/10.1007/978-1-4020-9827-7_1.
[33] Dillenbourg, P. and P. Jermann (2007), “Designing Integrative Scripts”, in Scripting Computer-Supported Collaborative Learning, Springer US, Boston, MA, https://doi.org/10.1007/978-0-387-36949-5_16.
[27] Dillenbourg, P. et al. (2011), Classroom orchestration: The third circle of usability., Proceedings of the 9th Computer-Supported Collaborative Learning Conference, Hong-Kong. July 4-8, 2011.
[34] Doise, W., G. Mugny and A. Perret-Clermont (1975), “Social interaction and the development of cognitive operations”, European Journal of Social Psychology, Vol. 5/3, pp. 367-383, https://doi.org/10.1002/ejsp.2420050309.
[1] Do-Lenh, S. et al. (2012), “TinkerLamp 2.0: Designing and Evaluating Orchestration Technologies for the Classroom”, in Lecture Notes in Computer Science, 21st Century Learning for 21st Century Skills, Springer Berlin Heidelberg, Berlin, Heidelberg, https://doi.org/10.1007/978-3-642-33263-0_6.
[9] Duval, E. (2001), “Metadata standards: What, who & why”, Journal of Universal Computer Science, Vol. 7/7, pp. 591-601.
[15] Fagen, A., C. Crouch and E. Mazur (2002), “Peer Instruction: Results from a Range of Classrooms”, The Physics Teacher, Vol. 40/4, pp. 206-209, https://doi.org/10.1119/1.1474140.
[38] Faucon, L. et al. (2020), “Real-Time Prediction of Students’ Activity Progress and Completion Rates”, Journal of Learning Analytics, Vol. 7/2, pp. 18-44, https://doi.org/10.18608/jla.2020.72.2.
[26] Gellersen, H., A. Schmidt and M. Beigl (1999), “Ambient media for peripheral information display”, Personal Technologies, Vol. 3/4, pp. 199-208, https://doi.org/10.1007/bf01540553.
[35] Gijlers, H. and T. de Jong (2005), Confronting ideas in collaborative scientific discovery learning, Paper presented at AERA 2005.
[23] Holstein, K. et al. (2018), “The classroom as a dashboard”, Proceedings of the 8th International Conference on Learning Analytics and Knowledge, https://doi.org/10.1145/3170358.3170377.
[31] Holstein, K., B. McLaren and V. Aleven (2019), “Co-Designing a Real-Time Classroom Orchestration Tool to Support Teacher–AI Complementarity”, Journal of Learning Analytics, Vol. 6/2, https://doi.org/10.18608/jla.2019.62.3.
[2] Holstein, K., B. McLaren and V. Aleven (2018), “Student Learning Benefits of a Mixed-Reality Teacher Awareness Tool in AI-Enhanced Classrooms”, in Lecture Notes in Computer Science, Artificial Intelligence in Education, Springer International Publishing, Cham, https://doi.org/10.1007/978-3-319-93843-1_12.
[3] Holstein, K., B. McLaren and V. Aleven (2017), “SPACLE”, Proceedings of the Seventh International Learning Analytics & Knowledge Conference, https://doi.org/10.1145/3027385.3027450.
[37] Kapur, M. (2015), “The preparatory effects of problem solving versus problem posing on learning from instruction”, Learning and Instruction, Vol. 39, pp. 23-31, https://doi.org/10.1016/j.learninstruc.2015.05.004.
[21] Lin, C. and M. Chi (2016), “Intervention-BKT: Incorporating Instructional Interventions into Bayesian Knowledge Tracing”, in Intelligent Tutoring Systems, Lecture Notes in Computer Science, Springer International Publishing, Cham, https://doi.org/10.1007/978-3-319-39583-8_20.
[11] Mangaroska, K., B. Vesin and M. Giannakos (2019), “Cross-Platform Analytics”, Proceedings of the 9th International Conference on Learning Analytics & Knowledge, https://doi.org/10.1145/3303772.3303825.
[29] Moher, T. et al. (2010), “Spatial and temporal embedding for science inquiry: An empirical study of student learning”, Proceedings of the 9th International Conference of the Learning Sciences, Vol. 1, pp. 826-833.
[17] Ochoa, X. and M. Worsley (2016), “Editorial: Augmenting Learning Analytics with Multimodal Sensory Data”, Journal of Learning Analytics, Vol. 3/2, pp. 213-219, https://doi.org/10.18608/jla.2016.32.10.
[6] Papert, S. (1987), “Microworlds: Transforming education”, Artificial Intelligence and Education, Vol. 1: Learning Environments and Systems, pp. 79-94.
[42] Prieto, L. et al. (2017), Reflection for Action: Designing Tools to Support Teacher Reflection on Everyday Evidence, Center for Open Science, https://doi.org/10.31219/osf.io/bj2rp.
[40] Prieto, L., K. Sharma and P. Dillenbourg (2015), “Studying Teacher Orchestration Load in Technology-Enhanced Classrooms”, in Design for Teaching and Learning in a Networked World, Lecture Notes in Computer Science, Springer International Publishing, Cham, https://doi.org/10.1007/978-3-319-24258-3_20.
[41] Prieto, L. et al. (2016), “Teaching analytics”, Proceedings of the Sixth International Conference on Learning Analytics & Knowledge - LAK ’16, https://doi.org/10.1145/2883851.2883927.
[20] Raca, M., L. Kidzinski and P. Dillenbourg (2015), Translating head motion into attention-towards processing of student’s body language, Proceedings of the 8th International Conference on Educational Data Mining, https://files.eric.ed.gov/fulltext/ED560534.pdf.
[4] Santos, P. (2012), Supporting orchestration of blended CSCL scenarios in distributed learning environments, Doctoral dissertation - Universidad de Valladolid, https://doi.org/10.35376/10324/1794.
[39] Schön, D. (2017), The Reflective Practitioner: How Professionals Think In Action., Routledge.
[36] Schwartz, D. and J. Bransford (1998), “A time for telling”, Cognition and Instruction, Vol. 16/4, pp. 475-522, http://www.jstor.org/stable/3233709.
[30] Schwendimann, B. et al. (2017), “Perceiving Learning at a Glance: A Systematic Literature Review of Learning Dashboard Research”, IEEE Transactions on Learning Technologies, Vol. 10/1, pp. 30-41, https://doi.org/10.1109/tlt.2016.2599522.
[10] Severance, C., T. Hanss and J. Hardin (2010), “Ims learning tools interoperability: Enabling a mash-up approach to teaching and learning tools”, Technology, Instruction, Cognition and Learning, Vol. 7/3-4, pp. 245-262.
[25] Tomitsch, M., T. Grechenig and S. Mayrhofer (2007), “Mobility and emotional distance: exploring the ceiling as an ambient display to provide remote awareness”, 3rd IET International Conference on Intelligent Environments (IE 07), https://doi.org/10.1049/cp:20070362.
[8] Vygotsky, L. (1964), “Thought and language”, Bulletin of the Orton Society, Vol. 14/1, pp. 97-98, https://doi.org/10.1007/bf02928399.
[19] Yang, D. et al. (2018), “An Emotion Recognition Model Based on Facial Recognition in Virtual Learning Environment”, Procedia Computer Science, Vol. 125, pp. 2-10, https://doi.org/10.1016/j.procs.2017.12.003.