The second step in the methodology concerns the selection of relevant variables from micro data sources to link to the national accounts items. This chapter discusses the main types of micro data sources that may be available for this purpose and provides guidance on how to best approach the selection.
OECD Handbook on the Compilation of Household Distributional Results on Income, Consumption and Saving in Line with National Accounts Totals
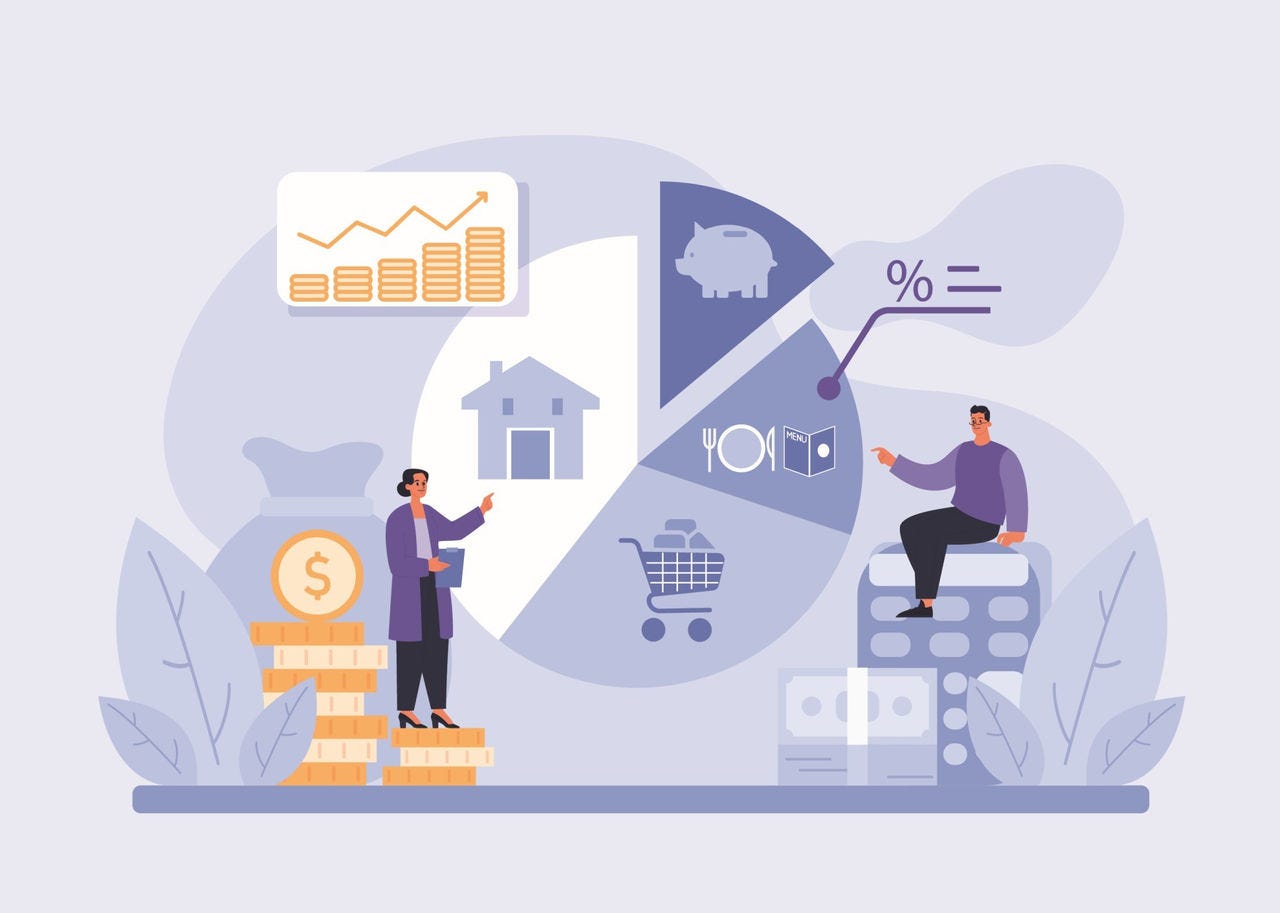
5. Determining relevant micro data sources
Abstract
5.1. The importance of selecting the appropriate items from micro data sources
Once the adjustment of the national accounts totals is performed, the methodology foresees a second step to link micro data to the various national accounts items. Whereas the national accounts totals as derived in the first step provide the benchmark for the distributional results, micro data are essential for distributing these amounts across household groups.
For the purpose of obtaining the most accurate distributional results, those micro variables should be selected that best match the national accounts items in terms of scope and definition. A targeted and structured micro data sources’ selection is meant to be a precondition for the success of deriving distributional results in line with the adjusted national accounts totals. For this purpose, it is essential to have a comprehensive overview of the available micro data sources with information on what variables are included and their specific definitions, their population coverage, and their timeliness and frequency. These characteristics are essential in selecting the best micro data source for each item.
Ideally, items can be found in the micro data sources that perfectly match the definition and coverage of the national accounts variables. However, because of different purposes, the classifications and definitions will not always perfectly match. This may require aggregating or disaggregating specific items from the micro side or the national accounts side, or making explicit adjustments in order to provide for a better horizontal match (i.e. matching micro and macro data at the level of the various income and consumption items). Furthermore, it has to be borne in mind that not all items will have a corresponding item in the micro data sources, especially those items that are specific to the System of National Accounts (SNA) (such as FISIM and investment income disbursements).
It is also important to note that the selection of the most appropriate items may concern the use of multiple data sources. This may necessitate the need to link the data across various data sources to arrive at coherent households’ results across the various items, known as vertical consistency. These issues are dealt with in the following chapters.
In order to facilitate the selection of the relevant items from micro data sources to match the national accounts items as described in Chapter 2, this chapter discusses the main types of micro data sources that may be available in countries for the compilation of distributional results consistent with national accounts totals. In general, the main data sources can be broken down into two categories, i.e. survey data and administrative data. Sections 5.2 and 5.3 discuss these two types of data sources with their main characteristics and main pros and cons. Chapters 10 and 11 provide a more detailed overview of information that may be available at the level of the detailed income and consumption items. As the available micro data sources may differ across countries, it is not possible to state which data sources should be used by default in the compilation of distributional results. Section 5.4 presents criteria on the basis of which compilers may select the best data sources depending on their national situation. To provide some practical guidance in selecting the most appropriate data source, Section 5.5 provides an overview of the micro data sources that are used by countries involved in the DNA work.
5.2. Survey data
Traditionally, surveys are an important data source for statistics. They have provided statisticians with relevant input data for the compilation of various kinds of statistics for many years. They may be used to obtain information on specific themes (e.g. the use of different transport modes or details on the expenditures by tourists) as well as on specific groups of entities (e.g. households or corporations), the setup of the survey depending on the specific purpose of the statistics.
Survey data derive from specific questionnaires including questions related to the target variables to be published in the statistics. In some cases, the questionnaire directly targets the variables that are needed in the statistics, but it may also be the case that information is requested on the basis of which the relevant variables can be derived. The information is usually collected for a sample of the population in which each sample unit (e.g. a person, a household or a corporation) is assigned a specific weight, indicating how many population units are represented by that specific sample unit, in order to be able to derive information for the total target population. The sample is the result of a design process that takes into account population characteristics (e.g. socio-demographic information) and non-response rates in order to arrive at representative results for the population as a whole. It also considers the response burden and other costs related to the survey, in order to arrive at good quality results while minimising the costs.
The main benefit of survey data is that statisticians have a large influence on the specific questions to be included in the questionnaire as well as on the sample design. Although some questionnaires are prescribed by international organisations to ensure international comparability, most of them are set up at the national level designed by the statistical authorities, taking into account country specific circumstances and needs. Another benefit is that surveys can include questions related to household as well as to individuals within the households, which broadens the scope for analysis and to group households according to alternative criteria based on characteristics of the individuals. This is often not possible with administrative data sources as they usually focus on either the individual or the household. Finally, survey data may be able to capture subjective variables (such as sentiments, opinions and perceptions) which can normally not be retrieved via administrative data sources.
The downside of survey results is that they may be affected by specific types of errors. The first concerns estimation errors which relate to the extrapolation of the survey results to the target population. They can be linked to the sample size, the representativeness of the sample and the magnitude of the non-response. The errors related to the sample size are referred to as standard sampling error, implying that the smaller the survey sample, the larger the margin of error surrounding the results, as less data underlie the ultimate estimates. The other two issues are referred to as coverage errors. These occur in the case of the sampling frame being different from the target population and in the case of selective non-response, both possibly causing bias to the overall results. It may for example be difficult to capture homeless people or the very rich, or it may be the case that some specific items are highly concentrated among a small set of households which may be difficult to properly capture via a sample survey. All these aspects may lead to margins of error surrounding the results.
A second type of errors concerns measurement errors, related to mistakes in the data reported in the surveys, either in the form of item non-response or the reporting of incorrect data. These errors may be due to misinterpretation of the questions, difficulty by respondents to recall the exact values, or deliberate misreporting. Meyer, Mok and Sullivan (2009[1]) show that a lot of statistics have to deal with measurement error and that these errors have increased over time, at least for some specific items. Especially questions on income are usually understood to be relatively sensitive and prone to higher non-response rates or larger measurement errors. Measurement errors may affect both survey and administrative data, but the impact on survey results may be larger due to the fact that these results are weighted to arrive at population totals, whereas this is usually not the case for administrative data (or to a much smaller extent).
Because of the possible impact of estimation and measurement errors, survey data are usually prone to substantial checks and edited in case of any errors, including imputations in case of unit non-response (if an entity does not respond to the survey) and item non-response (if a specific item is missing from the survey for a specific respondent).1 If the unit non-response is random, the sample weights may simply be adjusted to account for the missing entity, but in case of non-random unit non-response or item non-response, more sophisticated techniques are needed to properly correct for the missing data.2 In that regard, evidence shows that non-response is usually not random, but correlated to characteristics such as age, educational level and social status.3
In analysing the micro data and matching them with the national accounts totals, it is relevant to have more insight in the construction of the micro aggregates, i.e. broken down into the initial survey results, sample weights, and any corrections that may have been made to the micro data and the sample weights. A better understanding of the composition of the micro aggregates may provide more insight into the robustness of the results and possible margins of error surrounding the results. This may be particularly important in case of large gaps between the micro aggregates and the national accounts totals.
Another important issue that should be borne in mind when analysing differences between the micro and macro results when using survey data, is that surveys often focus on a specific point in time, which implies that the target population only includes the persons or households at that specific date. Conversely, national accounts focus on results over a certain time span, including all relevant information over that period. This means that survey data may exclude households that are included in the macro totals. For example, if the reference date is the 1st of January, the survey target population will exclude any immigrants and new-borns that will enter the national accounts population throughout the year. On the other hand, if the reference date is the 31st of December, emigrants and people that may have passed away throughout the year will not be covered in the survey results. These differences should be corrected for, before aligning the micro data to the national accounts data.
The availability of survey data sources will differ across countries. Despite the fact that many statistical offices compile similar statistics and despite several initiatives to further harmonise survey designs at the international level, countries often still have their own specific survey program, with the design of underlying surveys depending on historical considerations and country-specific issues. Notwithstanding these differences, almost all countries conduct surveys to obtain information on income and consumption, sometimes collecting data on these two topics in separate surveys, sometimes combining the two in a single survey. Moreover, some countries combine them with the collection of data on wealth.
Multipurpose surveys may be a very useful tool to collect consistent data on various topics at the household level and to analyse the relationships between these various topics. On the other hand, as explained by Cifaldi and Neri (2013[2]), combining income and consumption questions in one survey may reduce the willingness of respondents to participate to the questionnaire or hamper the quality of the survey results given the high level of detailed information which is required from respondents on both topics. Despite these caveats and the different approaches applied by countries, it is common practice to include a few recall questions on consumption within the income surveys, and vice versa, with the aim of analysing household saving (computed as income minus consumption) and of having an immediate feedback on the coherence between income and consumption information, enhancing the quality of the responses. Furthermore, it may help to link information on similar types of households across different surveys.
In addition to household surveys on income and consumption, which usually target residents of private dwellings (excluding people living in institutional households and people with no usual place of residence), relevant information may also be derived from other surveys, such as business surveys which may contain information on unincorporated enterprises, and surveys targeting specific topics such as health, housing or energy use. These may provide input for the distributional of some specific items in the work. Section 5.5 provides an overview of the various data sources that are used by countries involved in the DNA work.
5.3. Administrative data
An increasing number of statisticians is using administrative data (also often referred to as register data) in the compilation of their statistics. On the one hand, this relates to the increasing pressure for statistical offices to reduce the response burden and to cut costs related to the production of statistics. On the other hand, it is understood that the use of administrative data may solve issues of decreasing quality of survey data as observed in various countries due to lower response rates and provide the possibility to publish data at much more granular levels of detail.
Administrative data usually concern large data sets that cover the whole or a large part of the population. Hence, they are less subject to estimation errors and, as stated above, provide the opportunity to publish at very granular levels of detail. This is the main benefit of administrative data over survey data. Administrative data may still suffer from under-coverage or missing data, for example due to the fact that part of the population may fall outside the scope or due to non-reporting, but the impact will be far smaller than for sample surveys. Furthermore, the risk of non-reporting is often reduced by the use of legal sanctions to enforce compliance.
Like survey data, administrative data may suffer from measurement errors. These may relate to deliberate or accidental misreporting by respondents. In this regard, Adler and Wolfson (1988[3]) explain that high gaps between administrative data and macro totals may point to large under-reporting in tax records due to tax evasion. Fioro and D’Amuri (2006[4]) even argue that survey data may have a higher reliability than administrative data, as the latter may be affected by strategic reporting to lower the tax burden. Burkhauser et al. (2010[5]) also discuss the issue of “fiscal manipulation strategies” in which taxpayers reclassify specific types of income in order to limit their tax liabilities. Moreover, it is understood that the quality of auxiliary variables in administrative data sources (such as socio-demographic information or breakdowns of certain items) may be less reliable, as this is not related to the main purpose of the data collection. On the other hand, the legal sanctions that are often related to non- or misreporting normally ensure a high level of accuracy of the information enclosed in registers (Moore, Stinson and Welniak, 1997[6]). Moreover, part of the information may be reported by companies or financial institutions. Furthermore, it has to be borne in mind that the impact of errors, at least if they are not systematic, will be smaller than for survey data, because of the fact that the data sets usually cover the population as a whole. However, in order to avoid measurement errors in administrative data to negatively affect distributional results, it is important to carefully check the data and to correct for any errors, particularly if one targets to publish at very granular levels of detail.
Another important characteristic of administrative data is that whereas statisticians often have a large influence on the items to be included in a survey questionnaire, this is usually not the case for administrative data. As these are usually collected for administrative purposes, the setup of the data collection and the items included are usually not fully tuned to statistical needs. As a consequence, the items included in administrative data may often be based on different concepts and classifications than the ones used in micro statistics or in national accounts. Administrative records on income may for example be limited to cash-based income and may exclude certain in-kind payments. It may also be the case that certain categories include benefits that are treated differently in statistical measures, such as holding gains and losses. These differences may require re-classification of items as well as adjustments to correct for conceptual differences.
Furthermore, some of the items covered in administrative data sources may change over time as a consequence of changes in policy. For example, Burkhauser et al. (2013[7]) show how the change in the tax income base in Australia to include a more detailed breakdown of income items (e.g. dividends, capital gains, etc.) led to an overstatement of the increase in the income share held by top income groups due to the fact that the newly included income sources in the tax base were disproportionately held by them.
Finally, the unit of analysis may not always align to statistical needs. Dependent on the administrative purpose, the data set may focus on persons, households and/or other combinations of individuals. This may require adjustments in order to be able to use the administrative data for specific statistical purposes.
Most administrative data sources used by countries in the DNA work concern data sets from government agencies. Examples are population census data from statistical institutes, tax data from tax authorities, information on inbound and outbound visitors from immigration authorities, data on home ownership from land registers, and information on employment and wages from social security authorities. However, other administrative data sources may be envisaged as well, such as data from pension funds, insurance companies and large energy companies. They may provide useful information on some specific items.
In assessing the usefulness of administrative data sources, it is important that compilers obtain more information on their characteristics. In addition to the items that are included in the data source, it is important to obtain meta data on the definitions of these items, the population covered by the data source, the unit of analysis applied, the degree of under-coverage of specific household groups, and the frequency and timeliness of the data. Furthermore, it is important to know whether the data have already been checked, and if so, what corrections have been made (including imputations for missing records or missing items). The latter is important for checking the robustness of the results and assessing the margins of error for various groups of households in view of matching the data with the national accounts totals.
Looking at the national accounts items that may be covered in administrative data sources, the coverage is expected to be relatively high on the income side, with tax information possibly being available for the estimation of operating surplus from owner-occupied dwellings (as well as land register data), mixed income, compensation of employees (as well as social security data), property income (also related to information on wealth), taxes and social contributions and benefits (as well as social security data). On the other hand, information will probably be lacking for income flows between households (e.g. other current transfers) and income items specific to the SNA (e.g. investment income disbursements).
On the consumption side, the amount of available administrative data sources is expected to be lower. Information may be available on consumption of housing (from land register data), water, electricity, gas and other fuels (from data obtained from energy and water suppliers), health services (from data from health providers), purchases of vehicles (from car registries), and education (from school registries). Furthermore, “big data” sources such as credit card data, bank statements and data from special discount cards may provide relevant information for the distribution of some parts of household consumption. However, these may not provide full coverage of all consumption expenditure and may require more research on how to use them to derive reliable estimates.
In addition to directly using administrative data to match the national accounts items, they may also be used as supplementary information to check or to complete the information obtained via surveys. In that regard, it is common practice in an increasing number of countries to combine register data with survey data in compiling income statistics. This practice may improve the quality of income estimates which may be under-reported in household surveys. As mentioned in the previous section, it may also be the case that administrative data sources include information on the number of people benefiting from a certain type of income or purchasing a specific good or service. This may then be used to assess the degree of item non-response for specific items in the survey. Combining survey and administrative data may in that regard also lead to better input in matching the micro data to the national accounts totals.
5.4. Selecting the most appropriate data sources
As survey programs and the availability of and access to administrative data sources may differ across countries, it is not possible to specify upfront which data sources should be used by countries in compiling their distributional results. This will depend on the available data sources in the countries, the variables included in the data sets with their specific definitions, the population covered, the assessment of the data quality, and the timeliness and the frequency of the data. In some cases, there may only be one data source available for a specific variable, but if information is available from multiple data sources, compilers should carefully assess the available information to see which provides the best match with the national accounts items. In some cases, this may involve combining information from multiple data sources for a specific item to complement the main data source for information that may be missing or to cross-check some of the information included in the main data source.
In selecting micro data variables in relation to the national accounts totals, there are generally two approaches, i.e. the single-source approach and the multi-source approach. In the first case, all micro variables are taken from the same micro data source, whereas in the second case, multiple micro data sources are used in the process. Although using multiple data sources creates the need to link data across different data sets, it probably leads to the most useful micro data set underlying the distributional results. In that regard, a multi-source approach will often provide more and better links to the various national accounts items than a single-source approach. However, it also has to be borne in mind that selecting multiple micro data sources may in some cases lead to conflicting numbers on a same phenomenon (e.g. when information on a specific items is available from both the household survey and from tax records) as well as inconsistent estimates on inter-connected phenomena (e.g. when integrating data on income and consumption expenditure based on different surveys that may be based on different samples). This leads to a challenge to arrive at the best results, but it may also provide compilers with the possibility to cross-check and, if necessary, correct some of the micro data, of course after consultation of the micro experts.
In general, for each national accounts item, the micro variable should be selected that is regarded to provide the best basis for the distributional results in line with the national accounts total. On the one hand, this will depend on the conceptual fitness of the item with the national accounts variable and the difficulty to correct for any conceptual or classification differences. In this regard, items included in survey data may often provide a better match with the national accounts variables, although the concepts of the items covered in administrative data may often still come close (and auxiliary information may be available to correct for any conceptual differences). On the other hand, the selection of micro variables will depend on the quality of the underlying data to provide an accurate reflection of the actual distribution for this item for the target population (and the difficulty to impute for the part of the population that is missing). The latter relates to the possible impact of measurement and estimation errors on the underlying distribution in the micro data. It will often be difficult to assess the impact of measurement errors, as it would involve assessing the reliability of the reported data for the various data sets. Normally, it is possible to assess the impact of estimation errors, as it relates to the sample size in relation to the target population. In this respect, administrative data sources usually perform much better than survey data, as they cover the whole or a large part of the population, whereas survey data often rely on a sample, requiring weighting the data to arrive at population totals.
The selection of micro variables may also involve the combination of two or more data sources. Multiple data sets may for example provide reliable information on different parts of the population.4 In this regard, it is important to bear in mind that survey data may often suffer from relatively low coverage in the tails of the distribution. When relying on survey data as main data source, it may therefore be relevant to use administrative data sources to obtain a better coverage in the tails. Information as included in other data sources may also be used to cross-check information as included in the selected data source. This may be particularly relevant in case of large gaps between the micro and macro totals.
In addition to looking at the best conceptual and statistical match, it is also important to look at the timeliness and frequency of the available data sources. Some data sources may be compiled on an annual basis, whereas others may only become available every couple of years. In that case, it may be preferred to choose the annual data source as it may provide the opportunity to compile distributional results more frequently or to look at ways to combine the two data sources to arrive at reliable and consistent results on an annual basis. The latter may depend on the stability of the distributions over time and the robustness of nowcasting or interpolation techniques to derive results for the intermediate years.
Furthermore, users are mostly interested to obtain information shortly after the reference period, so also the timeliness with which the data sources may become available may be an important factor in selecting the most appropriate data sources. In that regard, survey data often suffer from substantial time lags, whereas administrative data may often become available within a short period after the reference period.
5.5. Overview of data sources currently used in compilation process
As micro statistics differ across countries it is difficult to provide a comprehensive list of surveys that may be available across countries providing information on income or consumption to be used in the compilation of distributional results in line with national accounts. However, because of the importance of selecting the appropriate micro data sources and to assist compilers in obtaining a comprehensive overview of possible data sources, this section provides an overview of the data sources that are used by countries in the DNA work.
Table 5.1 provides an overview of the micro data sources used by countries for both income and consumption items. It shows that different types of surveys and administrative data sources can be distinguished that contain relevant information on the household sector to be used as input to compile distributional results in line with national accounts. Some cover a single topic, focusing on either income or consumption, whereas others may cover both topics and, in some cases, even wealth information. Furthermore, some include detailed information on various income and/or consumption items, whereas others focus on a specific item, for example health, housing, farming or fishery.
Table 5.1. Micro data sources used by countries in their DNA work (stocktake conducted in the first half of 2022)
Country |
Name of the data source |
Nature* |
# elements where data source is used for |
Frequency** |
|||
---|---|---|---|---|---|---|---|
Income |
Consumption |
Saving |
Socio-demographic |
||||
Australia |
Survey of Income and Housing (SIH) |
S |
X |
- |
- |
X |
B |
Household Expenditure Survey (HES) |
S |
- |
X |
- |
X |
Every 6 years |
|
Census |
C |
X |
X |
- |
X |
Every 6 years |
|
Austria |
Statistics on Income and Living Conditions (SILC) |
M |
X |
X |
- |
X |
A |
Household Budget Survey (HBS) |
S |
X |
X |
- |
- |
Every 5 years |
|
Household Finance and Consumption survey (HFCS) |
S |
X |
- |
- |
- |
Every 3 years |
|
Education Expenditure Statistics |
M |
X |
- |
- |
- |
A |
|
School Statistics |
Y |
X |
- |
- |
- |
A |
|
Belgium |
Household Budget Survey (HBS) |
S |
X |
X |
- |
- |
B |
Statistics on Income and Living Conditions (SILC) |
S |
X |
X |
- |
X |
A |
|
Tax records - Belcotax/IPCAL data |
A |
X |
X |
- |
- |
A |
|
Demobel/CENSUS |
C |
- |
- |
- |
X |
A |
|
Data on government spending |
Y |
X |
X |
- |
X |
Y |
|
Belgian Health survey interview – HISIA |
S |
- |
X |
- |
X |
Every 5 years |
|
Canada |
Social Policy Simulation Database/Model (SPSDM) |
M |
X |
X |
- |
X |
O |
Canadian Income Survey (CIS) |
M |
X |
X |
- |
X |
A |
|
Survey of Household Spending (SHS) |
M |
X |
X |
- |
X |
A |
|
Annual Income Estimates for Census Families and Individuals (T1FF) |
A |
X |
X |
- |
X |
A |
|
Czech Republic |
Statistics on Income and Living Conditions (SILC) |
S |
X |
- |
- |
X |
A |
Household Budget Survey (HBS) |
S |
X |
X |
- |
X |
A |
|
Income tax return by individuals |
A |
X |
- |
- |
X |
A |
|
Population and Housing Census |
C |
- |
- |
- |
X |
Every 10 years |
|
Finland |
Statistics on Income and Living Conditions (SILC) |
S |
X |
X |
- |
X |
A |
Household Finance and Consumption survey (HFCS) |
S |
X |
- |
- |
- |
Every 3 years |
|
Household Budget Survey (HBS) |
S |
X |
X |
- |
- |
Every 5 years |
|
Tax data |
A |
X |
- |
- |
X |
A |
|
France |
Household Budget Survey (HBS) |
S |
X |
X |
X |
X |
Every 5 years |
Tax and social incomes survey |
M |
X |
- |
X |
X |
A |
|
Health data set |
M |
X |
- |
- |
X |
O |
|
Ireland |
Statistics on Income and Living Conditions (SILC) |
S |
X |
- |
- |
X |
A |
Household Budget Survey (HBS) |
S |
X |
X |
- |
- |
Every 5 years |
|
Fiscal data |
A |
X |
- |
- |
- |
A |
|
Administrative data on expenditure per student per level of education |
A |
X |
- |
- |
- |
A |
|
Israel |
Household Expenditure Survey |
S |
- |
X |
- |
- |
A |
Italy |
Statistics on Income and Living Conditions (SILC) |
M |
X |
- |
- |
X |
A |
Household Budget Survey (HBS) |
S |
- |
X |
- |
- |
A |
|
Survey on Household Income and Wealth (SHIW) |
S |
X |
- |
- |
- |
Every 2 years |
|
Ministry of Economy and Finance estimates of per capita expenditure on health |
Y |
X |
- |
- |
- |
A |
|
School Statistics |
M |
X |
- |
- |
- |
A |
|
Mexico |
Survey of household income and expenditure (ENIGH) |
S |
X |
X |
- |
X |
B |
Netherlands |
Register for Addresses and Buildings |
A |
X |
X |
- |
X |
A |
Income tax data |
A |
X |
X |
- |
X |
A |
|
Wealth tax data |
A |
X |
- |
- |
X |
A |
|
Household Budget Survey (HBS) |
S |
X |
X |
- |
X |
Every 5 years |
|
Pension Claims Statistics |
A |
X |
- |
- |
X |
A |
|
Household Finance and Consumption survey (HFCS) |
S |
X |
- |
- |
- |
Every 3 years |
|
Giving in the Netherlands panel survey |
S |
X |
- |
- |
X |
B |
|
Longitudinal Internet Studies for the Social Sciences |
S |
X |
- |
- |
X |
B |
|
Wage Register |
A |
X |
- |
- |
X |
A |
|
Insurance Healthcare Act |
A |
X |
- |
- |
X |
A |
|
Long-term Healthcare Act |
Y |
X |
- |
- |
X |
B |
|
Education enrolment registration |
A |
X |
- |
- |
X |
A |
|
Legal counsel |
A |
X |
- |
- |
X |
A |
|
Population data |
C |
X |
X |
- |
X |
A |
|
Portugal |
Statistics on Income and Living Conditions (SILC) |
S |
X |
- |
- |
X |
A |
Household Budget Survey (HBS) |
S |
- |
X |
- |
- |
Every 5 years |
|
Census |
C |
X |
- |
- |
X |
O |
|
Slovenia |
Statistics on Income and Living Conditions (SILC) |
M |
X |
X |
- |
X |
A |
Real Estate Register |
A |
X |
- |
- |
- |
A |
|
Household Budget Survey (HBS) |
S |
X |
X |
- |
- |
Every 3 years |
|
Sweden |
Income and tax statistics |
A |
X |
- |
- |
X |
A |
Statistics on Income and Living Conditions (SILC) |
S |
X |
X |
- |
X |
O (for variables used) |
|
Household Budget Survey (HBS) |
S |
X |
- |
X |
Every 5 years |
||
Distributional analysis system for income and transfers |
A |
X |
X |
- |
X |
A |
|
Property tax register |
A |
X |
X |
X |
A |
||
Vehicle register |
A |
X |
X |
X |
A |
||
United States |
Current Population Survey (ASEC) |
S |
X |
- |
- |
X |
A |
Consumer Expenditure Survey |
S |
- |
X |
- |
X |
A |
|
Statistics of Income |
Y |
X |
- |
- |
- |
A |
|
Survey of Consumer Finances |
S |
X |
- |
- |
- |
O |
|
American Community Survey |
S |
X |
- |
- |
- |
A |
|
Medial Expenditure Panel Survey (MEPS) |
A |
X |
- |
- |
- |
A |
Note: * Nature of data source: C = Census; S = Survey data; M = Combination of survey and administrative records; A = Administrative records; Y = Secondary statistics.
** Frequency: M = Monthly; Q = Quarterly; A = Annual; B = Biannual; O = Occasional.
References
[3] Adler, H. and M. Wolfson (1988), “A prototype micro-macro link for the Canadian household sector”, Review of Income and Wealth, Vol. 34/4, pp. 371-392, http://www.roiw.org/1988/371.pdf (accessed on 11 October 2017).
[12] Bricker, J. et al. (2015), “Measuring income and wealth at the top using administrative and survey data”, Finance and Economics Discussion Series, No. 2015-030, Board of Governors of the Federal Reserve System, Washington, https://doi.org/10.17016/FEDS.2015.030.
[5] Burkhauser, R. et al. (2010), “Recent Trends in Top Income Shares in the USA: Reconciling Estimates from March CPS and IRS Tax Return Data”, http://www.iariw.org/papers/2010/2dBurkhauser.pdf (accessed on 11 October 2017).
[7] Burkhauser, R., M. Hahn and R. Wilkins (2013), “Measuring top incomes using tax record data: A cautionary tale from Australia”, NBER Working Paper Series, No. 19121, http://www.nber.org/papers/w19121 (accessed on 11 October 2017).
[2] Cifaldi, G. and A. Neri (2013), “Asking income and consumption questions in the same survey: what are the risks?”, No. 908, Banca d’Italia, https://www.bancaditalia.it/pubblicazioni/temi-discussione/2013/2013-0908/en_tema_908.pdf?language_id=1 (accessed on 11 October 2017).
[10] D’Alessio, G. and I. Faiella (2002), “Non-response behaviour in the bank of Italy’s survey of household income and wealth”, No. 462, Banca d’Italia, https://www.bancaditalia.it/pubblicazioni/temi-discussione/2002/2002-0462/tema_462_02.pdf?language_id=1 (accessed on 27 October 2017).
[8] D’Alessio, G. and A. Neri (2015), “Income and wealth sample estimates consistent with macro aggregates: some experiments”, No. 272, Banca d’Italia, https://www.bancaditalia.it/pubblicazioni/qef/2015-0272/QEF_272.pdf?language_id=1 (accessed on 27 October 2017).
[4] Fiorio, C. and F. D ’Amuri (2006), “Tax evasion in Italy: an analysis using a tax-benefit microsimulation model”, http://fiorio.economia.unimi.it/res/tax_ev.pdf (accessed on 30 October 2017).
[1] Meyer, B., W. Mok and J. Sullivan (2009), “The under-reporting of transfers in household surveys: Its nature and consequences”, NBER Working Paper Series, No. 15181, http://www.nber.org/papers/w15181 (accessed on 27 October 2017).
[6] Moore, J., L. Stinson and E. Welniak (1997), “Income Measurement Error in Surveys: A Review”, http://citeseerx.ist.psu.edu/viewdoc/download?doi=10.1.1.39.6539&rep=rep1&type=pdf (accessed on 11 October 2017).
[11] Sabelhaus, J. et al. (2013), “Is the consumer expenditure survey representative by income?”, NBER Working Paper Series, No. 19589, http://www.nber.org/papers/w19589 (accessed on 27 October 2017).
[9] Törmälehto, V. (2017), “High income and affluence: Evidence from the European Union statistics on income and living conditions (EU-SILC)”, Eurostat Statistical Working papers, http://ec.europa.eu/eurostat/documents/3888793/7882117/KS-TC-16-027-EN-N.pdf (accessed on 9 October 2017).
Notes
← 1. In some cases, information on the number of persons or households receiving a certain income type or purchasing a specific good or service is used for this purpose. This type of information may be available from other (mostly administrative) data sources and may be confronted with the number of persons or households reporting a number for specific items in the survey to assess the possible degree of item non-response. Dependent on the setup of the data source, it may also provide insight which records may need to be edited.
← 2. For example, D’Alessio and Neri (2015[8]) discuss post-stratification in which the socio-demographic composition of the sample is aligned with known distributions from the census or other statistics. Administrative data may also be used for post-stratification in case it includes socio-demographic information. Furthermore, Törmälehto (2017[9]) explains that register data may be used to calibrate survey weights in order to reduce the estimation error in the top tail, although it has to be borne in mind that the top tail may include more heterogeneous groups of households for which it may be more difficult to correct for non-response by simply adjusting the sample weights.
← 3. D’Alessio and Faiella (2002[10]) show that non-response is often more frequent among higher income and wealthier households. Furthermore, Sabelhaus et al. (2013[11]) show that high income households are likely to be underrepresented in the consumer expenditure survey in the United States. The latter issue has been addressed by applying non-interview adjustment factors to the results based on fiscal data.
← 4. For example, Bricker et al. (2015[12]) explain that for income data, surveys may often represent a good instrument to collect information on low-income earners since they are not limited by any fiscal thresholds as tax registers are (e.g. households with low income that are not requested to pay taxes are not recorded in tax statistics, or income components that are not subject to taxation by law), whereas tax records are assumed to provide better estimates of top income shares, since in general surveys are characterised by under-representation of very high income households.