This chapter considers the role of people and communities in developing and adopting artificial intelligence (AI) technologies. It reveals a significant polarisation of attitudes among adults in OECD countries about the overall impact of AI on people in the next two decades. The demand for professionals with the skills to develop, adapt and maintain AI systems grew between 2019 and 2022 but remained very small overall. Such demand differs across countries and economic sectors. Employers advertising positions for professionals with AI skills generally demand a mix of technical, socio-emotional and foundational skills. Despite increasing ethical concerns over the development and use of AI, virtually no vacancy in 2022 explicitly prioritised ethical aspects or responsible AI development and use. The chapter concludes by considering risk preferences and attitudes towards failure among adults and youth, two attitudes and dispositions which will guide the integration of AI in workplaces and everyday decision making.
OECD Skills Outlook 2023
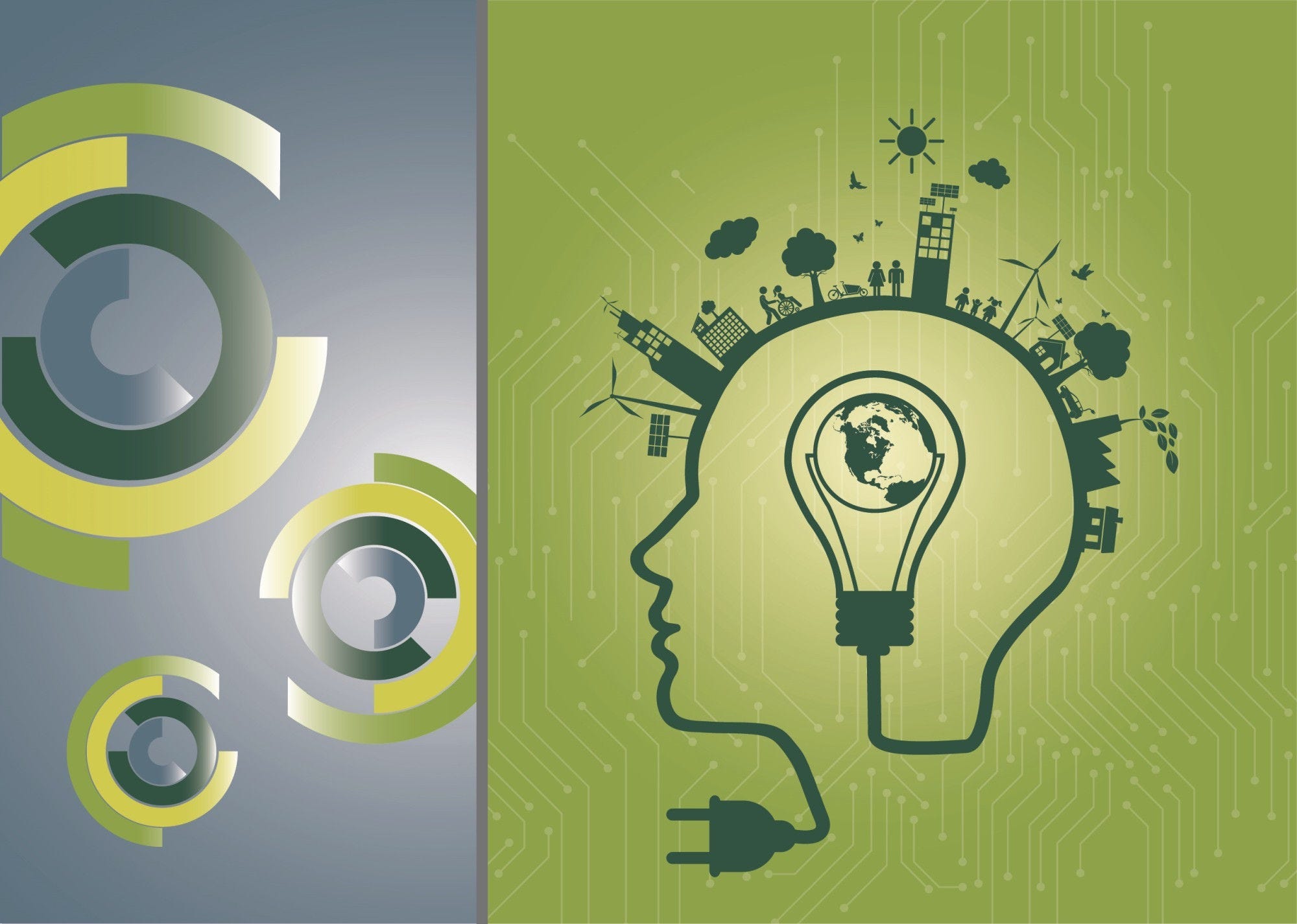
8. The human behind the machine: Skills and attitudes towards the future of artificial intelligence
Abstract
Key messages
Artificial intelligence (AI) and robotics have the potential to profoundly impact industries and occupations that rely on data and information, automating a wide range of tasks currently performed by humans. Many of today’s political and social tensions arising in response to the automation of tasks revolve around the questions of whether technologies will substitute or complement workers, give rise to better or worse labour market conditions, and ultimately whether they will be associated with an increase or a decrease in labour market opportunities.
This chapter considers the skills humans will need to make the most of technological change. In particular, it explores the demand for workers involved in the development and deployment of AI and the skills demanded of them, including technical skills, socio-emotional and foundational skills, as well as skills related to ethical decision making. The chapter also considers the set of attitudes and dispositions that may shape the readiness of individuals to benefit from large-scale deployment of AI systems.
Key empirical findings include:
Across OECD countries, 35% of adults in 2021 reported worrying that AI would mostly harm people in the next two decades, whereas 42% believed it would mostly help people.
Perceptions about the long-term effects of AI on societies differed according to gender, education level and experience with discrimination. For example, on average across OECD countries in 2021, 32% of men but 38% of women indicated that they worried that the long-term impact of AI would be mostly harmful.
The demand for professionals working in AI development and deployment increased markedly between 2019 and 2022. On average, the share of online vacancies requiring AI skills increased by 33% in 14 countries with available data. In the United States, for example, the country with the highest share of online vacancies requiring AI skills, the increase has been around 22%. However, in a few countries, such as Austria and Sweden, no increase over time has been observed.
The demand for professionals to fill positions requiring AI-related skills is highly concentrated, both in terms of sectors and in terms of occupations. The majority of job postings in the sample requiring AI skills were for positions referring to Professional Activities (25%), Information and Communications Technology (ICT) (24%), and Manufacturing (13%).
Employers looking for AI talent require a mix of technical, socio-emotional and foundational skills. Among the top ten AI employers and other AI firms, the skill profiles demanded do not vary substantially, with programming languages accounting for many of the most demanded technical skills, especially Python. This is followed by competencies in Computer and Data Science.
Only a small minority of firms advertising AI positions mentioned ethics and responsible AI in job adverts for professionals with AI skills. Despite large increases between 2019 and 2022, in 2022, in the majority of countries with available data, less than 1% of AI positions advertised mentioned such keywords.
Large sections of OECD populations hold negative attitudes towards risk and uncertainty and have high levels of fear of failure. This is more the case among women and girls: for example, 64% of men but 72% of women reported that they associate the word “risk” with danger rather than opportunity. Further, 45% of 15-year-old boys but 61% of girls indicate that failing makes them doubt their plans for the future.
8.1. Introduction
In today’s world, data and information are at the core of many economic activities, technological advances, and social exchanges. The increasing reliance on data analytics and the analysis and sharing of information for economic and social decisions, thanks to the development and use of AI, a category of models that make statistical predictions by identifying patterns in existing data, is significantly impacting individuals and societies.
Since AI plays a key role in the use of information and decision making in modern economies and societies, it is crucial to understand the human element behind advances in AI. The continuous evolution of AI systems brings with it different skills needed by AI workers to develop, adapt, and maintain AI applications. Across countries, differences in AI penetration mean that the demand for AI workers and the demand for specific AI-related skills may vary, too. An extensive review of the literature on the skills required in the development, adaptation and maintenance of AI systems and how the demand for professionals with such skills varies across countries, industries and occupations is available in the following technical working paper, which was developed to support the preparation of this report: Borgonovi et al. (2023[1]). Readers interested in the extensive set of analyses and indicators as well as the underlying methodology can consult this working paper.
The emergence of generative AI models has further enhanced the capabilities of AI systems with potentially far-reaching consequences for labour markets and societies more widely. For example, AI could automate tasks previously performed by humans, in particular, outperforming existing workers on tasks that rely on identifying patterns in large data infrastructures, leading to job displacement in certain industries. Early indications suggest that generative AI applications could be positively associated with increases in workers’ performance and productivity (OECD, 2023[2]). It could also improve quality of life by enhancing, for example, medical diagnostics and increase efficiency and productivity by automating repetitive tasks, which, however, might also exacerbate existing inequalities.
Over the past few decades, education and training systems have evolved to ensure that individuals can solve non-routine tasks. This transformation was driven by previous waves of technological progress, which emphasised the significance of problem solving alongside technology in the workplace (Autor, Levy and Murnane, 2003[3]; Ikenaga and Kambayashi, 2016[4]; Spitz‐Oener, 2006[5]). However, contrary to previous technological advances, AI is a general-purpose technology that has the potential to solve a wide range of non-routine problems, thus posing new demands on education and training systems (Brynjolfsson, Rock and Syverson, 2017[6]). The emergence of AI systems has the potential to significantly reshape the future demand for skills as algorithms and machines become increasingly capable of reliably performing non-routine tasks (Georgieff and Hyee, 2021[7]) and performing at human levels or surpassing human levels in some fields, such as science and reading (OECD, 2023[8]; 2023[9]). On the one hand, technology may obviate the need for humans to perform certain tasks. On the other hand, technologies may complement humans, requiring workers to learn to work effectively with new technologies (Arntz, Gregory and Zierahn, 2016[10]; Georgieff and Hyee, 2021[7]) as some tasks, but not all, will be affected by automation (Bessen, 2016[11]; Lassébie and Quintini, 2022[12]). The way in which AI will affect workers’ employment opportunities and job quality will also depend on their occupations. In particular, because AI is a prediction technology in occupations such as managerial occupations in which decisions encompass a judgement-related component, as well as a prediction component, AI adoption might require workers to improve skills complementary to AI, such as managerial decision skills, and skills related to risk management and ethics (Agrawal, Gans and Goldfarb, 2022[13]; OECD, 2023[2]).
Many of today’s political and social tensions arising in response to the automation of tasks previously carried out by humans in labour markets revolve around questions of whether digital technologies will substitute or complement workers, whether they will give rise to better or worse labour market conditions, and ultimately whether they will be associated with an increase or a decrease in labour market opportunities. In addition to the direct implications that AI and digital technologies have for productivity and growth, a crucial aspect of addressing these questions lies in understanding the tasks various technologies can undertake and the skills humans must acquire to effectively harness the benefits of technological advancements (OECD, 2021[14]; Violante, 2008[15]; OECD, forthcoming[16]).
This chapter identifies the human element behind advances in the development and application of AI by analysing skill requirements for individuals engaged in AI development and adaptation across 14 OECD countries – Australia, Austria, Belgium, Canada, France, Germany, Italy, the Netherlands, New Zealand, Spain, Sweden, Switzerland, the United Kingdom and the United States – during the period from 2019 to 2022. Using information derived from vacancies posted on line by prospective employers of AI professionals, the chapter considers differences in the demand for AI professionals – i.e. workers with skills needed to develop, adapt and maintain AI systems – in different sectors, and the range of skills required of AI professionals in general and by large “AI employers” in particular. The chapter also considers to what extent vacancies advertising positions for individuals with AI skills mention aspects related to ethics and responsible use of AI, elements of growing importance given advances in AI adoption and deployment. For the purposes of this Skills Outlook AI skills refer to the skills that are needed to develop, maintain and adapt AI systems and not the broader set of skills that are needed to work with and alongside AI. The chapter further considers some of the attitudes, beliefs and dispositions that may shape how general populations will respond to AI adoption and determine the success of integrating AI systems in labour markets and societies.
8.2. Perceptions about AI differ by country and socio-economic characteristics
AI-powered systems can process large amounts of data, identify patterns and make predictions faster and more accurately than humans, leading to increased efficiency and productivity in various industries. Furthermore, because AI systems can analyse vast amounts of data in a short timeframe and reveal patterns in ways that would be impossible for humans to identify, they can provide valuable insights to support decision-making processes in contexts characterised by large data infrastructures. This could be the case in areas such as healthcare, finance and scientific research, where AI can assist in diagnosing diseases, identifying investment opportunities or accelerating the discovery of new drugs. Finally, AI could provide individualised input to individuals based on their needs and preferences.
On average, across OECD countries, 35% of adults in 2021 reported worrying that artificial intelligence in the next two decades would “mostly harm”, and 42% believed it would “mostly help”, with the rest having no opinion, declining to answer or indicating that AI would neither help nor harm (Figure 8.1). However, countries differ significantly in terms of perceptions of AI. For example, in Portugal, over 50% of individuals worried that AI would mostly harm people in the next 20 years, whereas in Korea, Japan and Iceland, less than 20% did. Similarly, in Korea, Finland and Japan, over 60% of people reported believing that AI will mostly help people; in Chile, Croatia, Portugal and Colombia, less than 30% did. Across OECD countries, as many as 18% of adults indicated not having an opinion on the likely impact of AI, and in Iceland, as many as 35% of adults reported the same. In contrast, in Korea and Italy, only 9% indicated not having an opinion.
Figure 8.1. Adults’ perception of the long-term impact of AI in selected countries, 2021
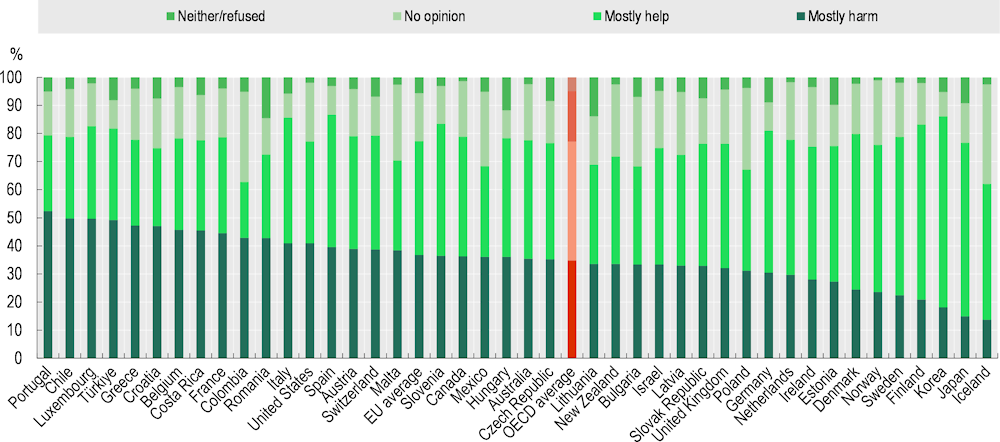
Note: The figure shows the percentage of adults who respond to the following question: “Artificial intelligence will help or harm people in the next 20 years”, with “mostly harm”, “mostly help”, “no opinion”, “neither/refused”. Countries are sorted in descending order of the percentage of those answering “mostly harm”.
Source: World Risk Poll (2021[17]), World Risk Poll 2021: A Digital World - Perceptions of Risk from AI and Misuse of Personal Data, https://wrp.lrfoundation.org.uk/data-resources/.
When considering only individuals expressing an opinion on AI’s likely impact on people in the next two decades, on average across OECD countries, the percentage of those who believe that AI would mostly help was 7 percentage points higher than those who worried that AI would mostly harm (Figure 8.2). This suggests that, on average, across OECD countries in the general population in 2021, there were more optimists than pessimists concerning the overall impact of AI. At the same time, countries differed greatly in whether local populations generally had an optimistic or pessimistic view of AI. For example, in Portugal, there was a difference of 25 percentage points in the share of adults reporting that they believed AI would mostly harm and those who thought AI would mostly help people in the next two decades. Similarly, this difference was 23 percentage points in Colombia and 21 percentage points in Chile. By contrast, in Korea, there was a difference of 50 percentage points in the share of adults reporting that they believed AI would mostly help and those reporting that AI would mostly harm people in the next 20 years. In Finland, this difference was 41 percentage points; in Japan, it was 47 percentage points.
Figure 8.2. Difference between positive and negative associations with AI in selected countries, 2021
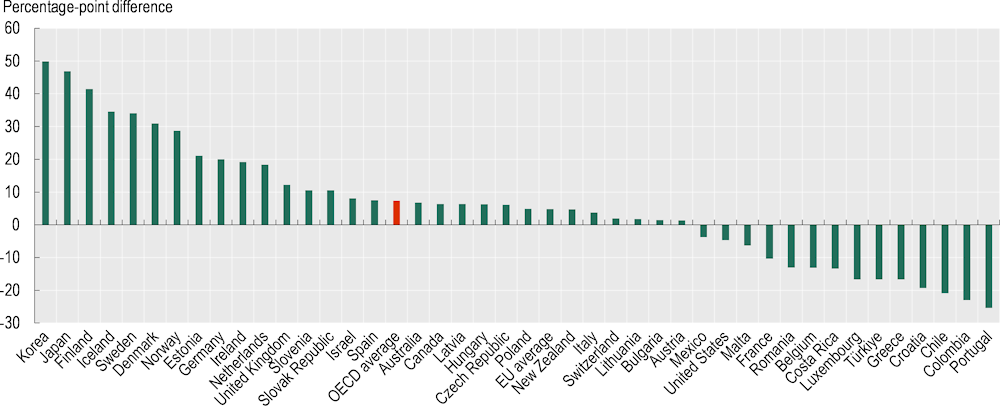
Note: The figure shows the percentage-point difference of adults who responded “mostly helpful” and “mostly harmful” to the following question, “Artificial intelligence will help or harm people in the next 20 years?”
Source: World Risk Poll (2021[17]), World Risk Poll 2021: A Digital World - Perceptions of Risk from AI and Misuse of Personal Data, https://wrp.lrfoundation.org.uk/data-resources/.
Perceptions about the long-term effects of AI on societies differ according to individuals’ socio-economic profiles and experiences of discrimination. In particular, women, individuals without tertiary qualifications and individuals who had faced discrimination due to the colour of their skin, religion, nationality/ethnic group, gender or disability were more likely to report worrying that AI would have a harmful long-term impact. For example, on average, across OECD countries in 2021, 32% of men but 38% of women indicated that they worried that the long-term impact of AI would be mostly harmful, whereas 49% of men and 36% of women indicated they believe AI would be mostly helpful in the long term (Figure 8.3). Similarly, 28% of tertiary-level graduates and 37% of individuals without tertiary-level qualifications worried that the long-term effects of AI would be mostly harmful, and 55% of tertiary-level graduates and 39% of individuals without tertiary-level qualifications indicated they believed that the effects of AI would be mostly helpful. Finally, whereas a similar number of individuals with and without a past experience of discrimination expected AI to have positive long-term effects, 32% of individuals with and 27% without a past experience of discrimination expected AI to have harmful long-term effects.
Figure 8.3. Adults’ perception of the long-term impact of AI, by gender, discrimination and education, 2021
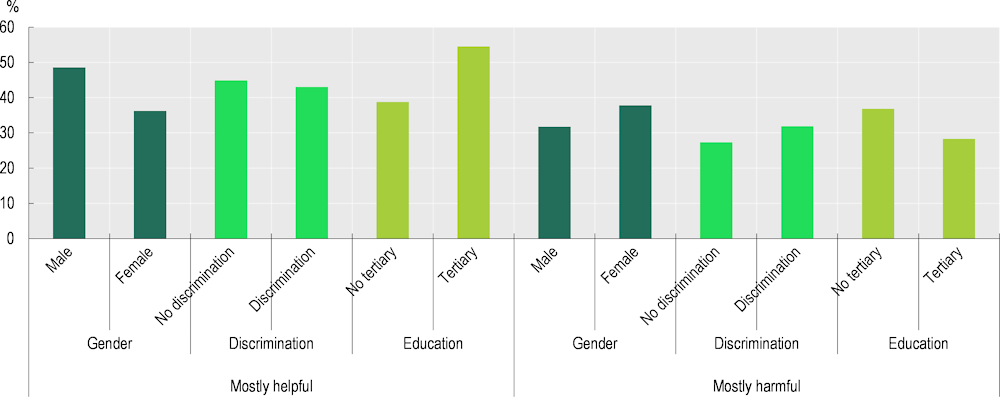
Note: The figure shows the percentage of adults who perceive the long-term impact of AI as “mostly helpful” or “mostly harmful” by gender, discrimination (individuals who faced discrimination due to the colour of their skin, religion, nationality/ethnic group, gender or a disability) and education (tertiary versus non-tertiary).
Source: World Risk Poll (2021[17]), World Risk Poll 2021: A Digital World - Perceptions of Risk from AI and Misuse of Personal Data, https://wrp.lrfoundation.org.uk/data-resources/.
Data presented on general populations refer to attitudes about the expected overall impact of AI on people. Recent work on the likelihood that employees in manufacturing and finance firms indicated higher levels of optimism about the role of AI in the labour market than those expressed by the general population on the overall expected impact of AI: 19% of workers in finance and 14% in manufacturing said that they were very or extremely worried about job loss in the next ten years, while 46% and 50% were not worried at all (Lane, Williams and Broecke, 2023[18]). To note that the survey was administered before the advent of generative AI and attitudes may have shifted since then, both with respect to the overall impact of AI and the impact of AI on the workplace. In fact, a survey conducted in August 2023 in the United States indicated that over two thirds of adults reported being concerned about AI displacing workers with around a third of respondents reporting being very worried (American Federation of Labor and Congress of Industrial Organizations (AFL-CIO), 2023[19]).
8.3. The demand for and the skills of workers responsible for AI development, adaptation and maintenance
The debate on the consequences of AI development and adoption generally considers machines and humans as separate and conflicting entities, even though AI systems are developed, deployed and used by humans and perform tasks driven by human decision making. For example, the lack of interpretability of the outputs of AI systems does not arise from AI systems acquiring decision-making abilities or free will. Rather, it is a result of the limited information capacity of human cognition in relation to the level of complexity of the steps and processes AI systems engage in to produce output. This complexity is driven by the interaction between the algorithms AI systems are built on and the amount of data used by the systems to generate predictions. Large language models at the basis of now widely known AI systems such as ChatGPT were developed by humans and only become useful when they are put to use by humans to solve their questions and challenges. What differs whenever AI is used is that decision making is shifted in time and space: decisions over the course of action taken by AI systems are not made when and where such systems encounter a problem to solve but, rather, when and where AI developers defined the parameters in the system.
Because AI systems are the product of humans, appreciating the capabilities and potential of AI for societies and labour markets starts with a better understanding of the role humans play in creating new AI systems or adapting AI systems to the unique needs of different end users. AI professionals, in fact, determine how AI systems collect, store and use data to make predictions ranging from cancer detection (Bi et al., 2019[20]) to providing instantaneous translation between different languages (Borgonovi, Hervé and Seitz, 2023[21]). Yet little is known about the demand for workers with skills to develop and adapt AI systems in different countries, how this demand has changed – and is changing – over time, and whether it differs across sectors and occupations.
Moreover, exploring potential variations in the skill sets required by different employers utilising AI can assist in uncovering the underlying factors contributing to disparities in technology diffusion, productivity and economic growth. Recent evidence has, in fact, documented increasing productivity gaps between the best-performing firms and the rest of the business population (Andrews, Criscuolo and Gal, 2016[22]), reflecting a slowdown in technology diffusion. Skills are key for such diffusion processes, especially in the current stages of digital transformation. Recent OECD work has explored the characteristics of firms that adopt AI (Calvino et al., 2022[23]; Dernis et al., 2023[24]) as well as the connections between AI usage and productivity (Calvino and Fontanelli, 2023[25]). This research has underscored substantial heterogeneity based on factors such as firm size, age and sector. Importantly, it has also highlighted the crucial role of complementary assets, particularly skills, for both AI adoption and the productivity benefits experienced by AI users. In this context, understanding the specific skill sets sought by different types of AI employers can enhance understanding of the foundations on which their performance hinges. This knowledge can inform policies aimed at promoting an inclusive digital transformation.
The existing literature on the AI workforce and its participants is limited and primarily relies on evidence from online job postings by employers. This reliance stems from the lack of dedicated categories in official employment statistics to accurately capture individuals involved in developing and utilising AI systems. Although estimates of the number of people engaged in developing and using AI tools and systems vary (Acemoglu et al., 2022[26]; Alekseeva et al., 2021[27]; Green and Lamby, 2023[28]; Samek, Squicciarini and Cammeraat, 2021[29]), they all suggest that few individuals worldwide are involved in the development and use of AI technologies.
Previous efforts to map the demand for workers that could advance the development and use of AI systems focused on English-speaking countries and exploited different algorithms as well as AI-related keywords to identify AI-related skills and jobs in online job postings (Alekseeva et al., 2020[30]; Babina et al., 2020[31]; Manca, 2023[32]; Samek, Squicciarini and Cammeraat, 2021[29]; Squicciarini and Nachtigall, 2021[33]). More recently, Green and Lamby (2023[28]) widened the scope, providing estimates of the size and characteristics of the AI workforce across OECD countries. In particular, Green and Lamby (2023[28]) highlighted how the AI workforce currently lacks diversity, being disproportionally male and having high levels of educational qualifications.
8.3.1. The demand for AI professionals
Few vacancies posted on line require skills to develop, maintain and adapt AI systems
Figure 8.4 shows how the share of online vacancies advertising positions requiring AI skills changed between 2019 and 2022. On average, across countries in the sample, the share of online vacancies requiring AI skills increased from 0.30% in 2019 to 0.40% in 2022 [estimates are in line with other related studies, such as Green and Lamby (2023[28])]. These results represent trends up to 2022 before the generative AI chatbot ChatGPT was first introduced. However, evidence from the United States indicates that despite a very large growth of generative AI between December 2022 and September 2023, generative AI remains small in proportion to the whole AI market. In 2022, there were 519 job postings calling for generative AI. Between December 2022 and September 2023 there were 10 113 generative AI postings in the United States, an increase of 1.848%. However generative AI postings represented only 0.026% of all AI postings advertised between December 2022 and September 2023 (Lightcast, 2023[34]).
Figure 8.4. Share of online vacancies requiring AI skills in selected countries, 2019 and 2022
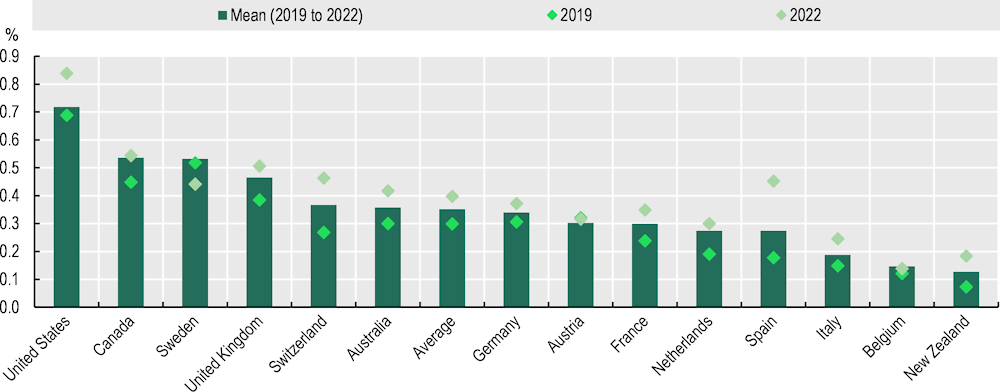
Note: The figure shows the percentage of online vacancies advertising positions requiring AI skills by country. This corresponds to the total number of online vacancies requiring AI skills relative to all vacancies advertised in a country. Vacancies requiring AI skills are vacancies in which at least two generic AI skills or at least one AI-specific skill were required (see Borgonovi et al. (2023[1]) on generic and specific skills). Countries are sorted in descending order by the highest average share across 2019 to 2022 of vacancies requiring AI skills. Average refers to the average across countries with available data.
Source: Calculations based on Lightcast (2022[35]), Lightcast™, https://lightcast.io/ (accessed December 2022), in Borgonovi et al. (2023[1]), ”Emerging trends in AI skill demand across 14 OECD countries”, https://doi.org/10.1787/7c691b9a-en.
The share of online vacancies that require AI-related skills and their evolution over time vary across the 14 OECD countries with available data. In 2019, the share of positions requiring AI skills ranged between 0.07% in New Zealand to 0.69% in the United States, while in 2022, the share of positions requiring AI skills ranged between 0.14% in Belgium to 0.84% in the United States. While initial levels differed across countries, in most countries, the share of overall vacancies requiring AI-related skills increased between 2019 and 2022, except for Austria and Sweden. At the same time, the share of all online vacancies requiring AI skills did not exceed 1%, not even in the most recent year under analysis. These estimates do not shed light on the actual stock of workers with AI-related skills in the labour market but, rather, the evolution of the demand for workers who would be required to use AI skills in their jobs. As such, they suggest that only a few occupations require the specialised skills necessary to develop and use AI systems.
Over time, the diffusion of AI skills, measured as the increase in the share of online vacancies requiring AI skills, varied across countries. On average, across countries in the sample, the share of online vacancies requiring AI skills increased by 33%. In some countries, the diffusion of AI skills is well beyond this average increase. For example, in Spain and New Zealand, countries with initially relatively low shares of online vacancies requiring AI skills, shares increased by 155% and 150%, respectively, between 2019 and 2022. Spain ranked 11th out of 14 countries when considering the share of positions requiring AI skills in 2019 but ranked 5th by 2022. New Zealand ranked last in 2019 and second to last in 2022. The increase over time has been more modest for countries that started with relatively high shares of online vacancies requiring AI skills in 2019. In the United States, for example, the country with the highest share of online vacancies requiring AI skills, the increase has been around 22%. In a few countries, such as Sweden and Austria, no increase over time has been observed.
Figure 8.5. Top three sectors in which online vacancies requiring AI skills are advertised in selected countries, 2019-22
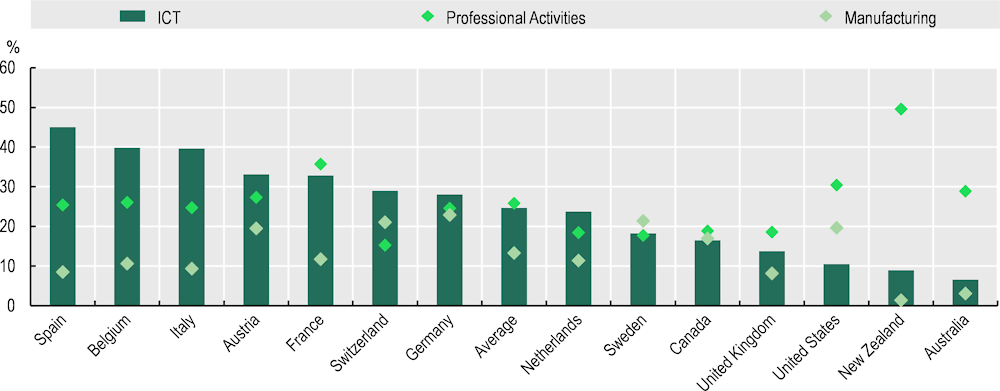
Note: The figure shows the percentage of online vacancies advertising positions requiring AI skills (reported in this figure for the top three industries). This corresponds to the total number of online vacancies advertising positions requiring AI skills in a given industry sector in a country relative to all positions requiring AI skills across all industries in a given country. Countries are sorted in descending order of the highest average share of vacancies requiring AI skills in ICT. The top three industries are based on the highest share of positions requiring AI skills across countries and years. Positions requiring AI skills are positions in which at least two generic AI skills or at least one AI-specific skill were required (see Borgonovi et al. (2023[1]) on generic and specific skills). Borgonovi et al. (2023[1]) detail how the harmonisation of sectoral information across European countries and English-speaking countries was conducted, given differences in the taxonomies used by Lightcast in the compilation of data for English-speaking and countries in Europe. Average refers to the average across countries with available data.
Source: Calculations based on Lightcast (2022[35]), Lightcast™, https://lightcast.io/ (accessed December 2022), in Borgonovi et al. (2023[1]), ”Emerging trends in AI skill demand across 14 OECD countries”, https://doi.org/10.1787/7c691b9a-en.
The share of online vacancies that require AI-related skills and their evolution over time also vary across sectors of the economy. In particular, the share of postings requiring AI skills over the 2019‑22 period was considerably higher in “Professional Activities”, “ICT” and “Manufacturing” than in other sectors, such as “Accommodation and Food”, “Agriculture”, and “Transportation”, where virtually no postings required AI skills (Borgonovi et al., 2023[1]). Moreover, sectors with very low shares of AI-related job postings in 2019 exhibited little change over time. In contrast, sectors such as Manufacturing, ICT and Professional Activities, for which the shares were already comparatively high in 2019, exhibited substantial increases over time. Figure 8.5 indicates the share of online vacancies for positions requiring AI skills posted in ICT, Manufacturing, and Professional Activities, the three industry sectors that rank highest in demand across all countries and years over the 2019‑22 period.
The average share of positions requiring AI skills across English-speaking and European countries in the sample was 25% for Professional Activities, 24% for ICT and 13% for Manufacturing. The country with the highest share of vacancies requiring AI skills in Professional Activities was New Zealand (50%), followed by France (36%) and the United States (30%). Among postings requiring AI skills in the ICT sector, shares were highest in Spain (45%), Belgium (40%) and Italy (40%). For Manufacturing, shares were highest in Germany (23%), Sweden (21%) and Switzerland (21%). Within countries, the relative share of positions requiring AI skills can differ substantially between sectors, likely reflecting specialisation differences.
The skills most requested in online vacancies for AI professionals
While the previous analyses focused on the distribution of positions requiring AI skills across countries, sectors and occupations, this section focuses on the type of skills required by employers when looking for AI talent to hire. To categorise positions into those that require AI skills versus those that do not, a list of 211 pre-defined AI keywords was used. In order to meaningfully describe the skills required in AI-related postings, these skills were further condensed into meaningful clusters of skills. These clusters were determined based on industry expert assessment of skills common in these topic areas or implementations of AI. Skills were grouped into the following seven skill clusters: “Artificial Intelligence”, “Autonomous Driving”, “Machine Learning”, “Natural Language Processing”, “Neural Networks”, “Robotics” and “Visual Image Recognition” (Table 8.1 provides some examples of the set of skills in each of the seven skill clusters).
Table 8.1. Examples of skill sets in the seven AI-related skill clusters
Artificial Intelligence |
Autonomous Driving |
Machine Learning |
Natural Language Processing |
Neural Networks |
Robotics |
Visual Image Recognition |
---|---|---|---|---|---|---|
Baidu |
Advanced Driver Assistance Systems |
Supervised Learning |
Text Mining |
TensorFlow |
Cognitive Robotics |
3D Reconstruction |
Intelligent Control |
Autonomous System |
Test Datasets |
Tokenization |
Pybrain |
Motion Planning |
Activity Recognition |
Weka |
Path Finding |
Training Datasets |
Voice Interaction |
Long Short-Term Memory (LSTM) |
Robot Framework |
Computer Vision |
OpenAI Gym |
Remote Sensing |
Vowpal Wabbit |
Voice User Interface |
Keras (Neural Network Library) |
Nvidia Jetson |
Machine Vision |
Watson Studio |
OpenCV |
Xgboost |
Word2Vec Models |
Long Short-Term Memory (LSTM) |
Servomotor |
Eye Tracking |
Note: The table provides a selected list of skills for each of the seven skill groups.
Source: Calculations based on Lightcast (2022[35]), Lightcast™, https://lightcast.io/ (accessed December 2022), in Borgonovi et al. (2023[1]), ”Emerging trends in AI skill demand across 14 OECD countries”, https://doi.org/10.1787/7c691b9a-en.
Figure 8.6 shows the evolution of online vacancies advertising positions requiring AI skills by skill cluster between 2019 and 2022. In 2022, the skill cluster mentioned most frequently in postings was Machine Learning. Machine Learning was required in more than 0.25% of all vacancies posted on line. Among postings requiring AI skills, which account for around 0.35% of total online vacancies in 2022 (Figure 8.4), almost 35% require Machine Learning skills (Figure 8.7). Artificial Intelligence is the second most frequently requested skill cluster, with around 0.16% of postings requesting such skills in 2022, almost 21% of postings among those requiring AI skills (Figure 8.7). The remaining skill clusters are demanded in less than 0.1% of all vacancies posted on line, with Robotics and Autonomous Driving being the skills clusters requested least frequently (0.04% and 0.06%). One reason these skills may be the least in demand is that they are highly industry- and country-specific.
Figure 8.6. Trend in the demand for AI workers by skill cluster, 2019-22
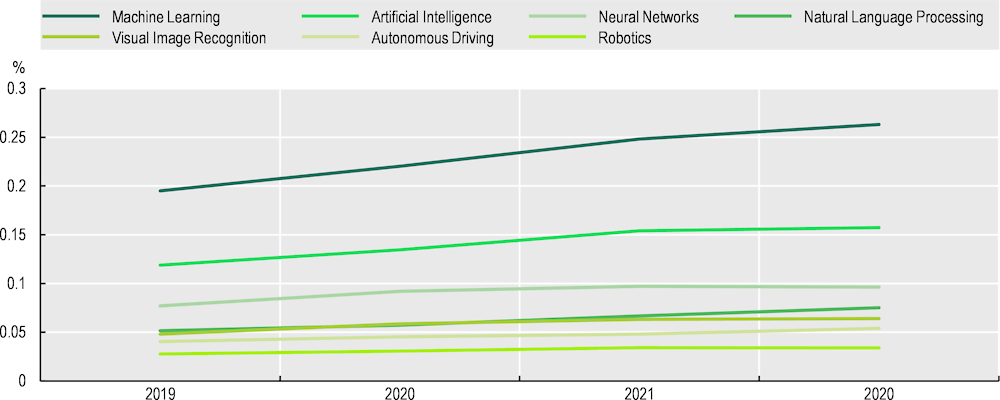
Note: The figure shows the percentage of online vacancies requiring AI skills relative to all postings, by skill cluster and year, which is the total number of online vacancies requiring AI skills relative to all online vacancies. Examples of skills assigned to the different skill clusters are provided in Table 8.1, while a full overview of which AI skills are assigned to which cluster is provided in Borgonovi et al. (2023[1]). Positions requiring AI skills are positions in which at least two generic AI skills or at least one AI‑specific skill were required [see Borgonovi et al. (2023[1]) on generic and specific skills].
Source: Calculations based on Lightcast (2022[35]), Ligthcast™, https://lightcast.io/ (accessed December 2022), in Borgonovi et al. (2023[1]), ”Emerging trends in AI skill demand across 14 OECD countries”, https://doi.org/10.1787/7c691b9a-en.
Figure 8.7 shows the relative demand for the top three of seven skill clusters mentioned in postings requiring AI skills in each country between 2019 and 2022. On average, over the period and across the 14 countries with available data, 34% of online vacancies requiring AI skills required skills from the Machine Learning cluster, followed by 21% requiring skills related to the AI cluster and 14% requiring skills related to the Neural Networks cluster.
Across all countries, the Machine Learning skill cluster ranked highest, ranging from 39% in the United States to 29% in France. In 11 out of 14 countries, skills related to the AI cluster were the second most frequently requested skill cluster, with the highest shares observed in New Zealand (27%) and the lowest in Sweden (14%). In Switzerland, Germany, and France, the share of AI-related postings requiring AI skills is smaller than the share requiring Neural Networks. However, France and Germany lead the distribution of postings requiring Neural Networks skills, with 18% and 17%, respectively.
Figure 8.7. Top three skill clusters demanded in postings requiring AI skills, selected countries, 2019-22
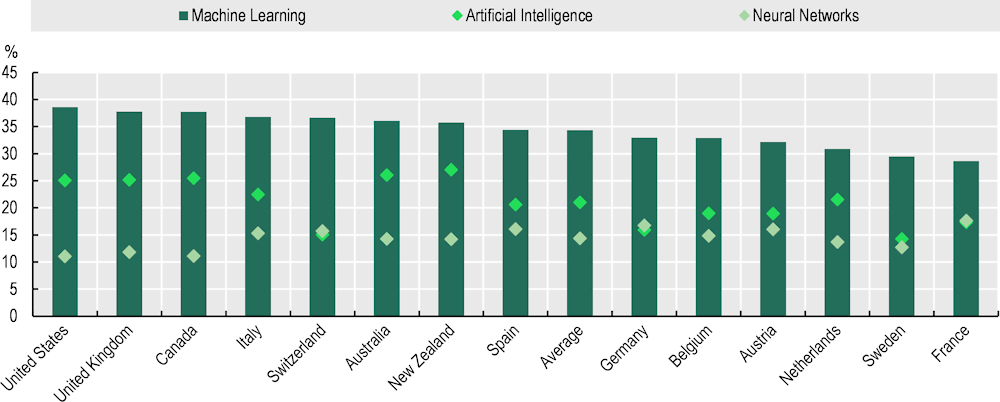
Note: The figure shows the percentage of online vacancies requiring AI skills for specific skill clusters, for the top three skill clusters, by country, which is the total number of online vacancies requiring specific skill clusters relative to the total number of online vacancies requiring AI skills. Examples of skills assigned to the different skill clusters are provided in Table 8.1, while a full overview of which AI skills are assigned to which cluster is provided in Borgonovi et al. (2023[1]). Positions requiring AI skills are positions in which at least two generic AI skills or at least one AI-specific skill were required [see Borgonovi et al. (2023[1]) on generic and specific skills]. Countries are sorted in descending order by percentage of postings requiring Machine Learning skills. Average refers to the average across countries with available data.
Source: Calculations based on Lightcast (2022[35]), Lightcast™, https://lightcast.io/ (accessed December 2022), in Borgonovi et al. (2023[1]), ”Emerging trends in AI skill demand across 14 OECD countries”, https://doi.org/10.1787/7c691b9a-en.
Examining the type of skills required in online postings advertising positions requiring AI skills indicates that two skill clusters are especially prevalent over the 2019‑22 time period (Figure 8.6) and across countries (Figure 8.7): Machine Learning and AI. Nonetheless, Figure 8.8 suggests that when other skill clusters are considered, countries vary with respect to the relative demand for skills in the less-demanded clusters. In particular, whereas Figure 8.6 showed that two skill clusters have, compared to all other clusters, relatively low shares on average across countries in 2022 (Autonomous Driving [0.05%] and Robotics [0.03%]), Figure 8.8 indicates that the share of postings requiring skills related to the Autonomous Driving cluster was comparatively high in France (where 19% of online vacancies advertising positions requiring AI skills require skills in this cluster) and Sweden (where 17% of online vacancies advertising positions requiring AI skills require skills in this cluster). This was very low in New Zealand (where only 3.7% of online vacancies advertising positions requiring AI skills require skills in this cluster). For the Robotics cluster, demand was comparatively strong in the Netherlands (13%), followed by Sweden (9%), and lowest in New Zealand and Spain (1.5%).
Figure 8.8. AI skill cluster distribution, by country, 2019-22
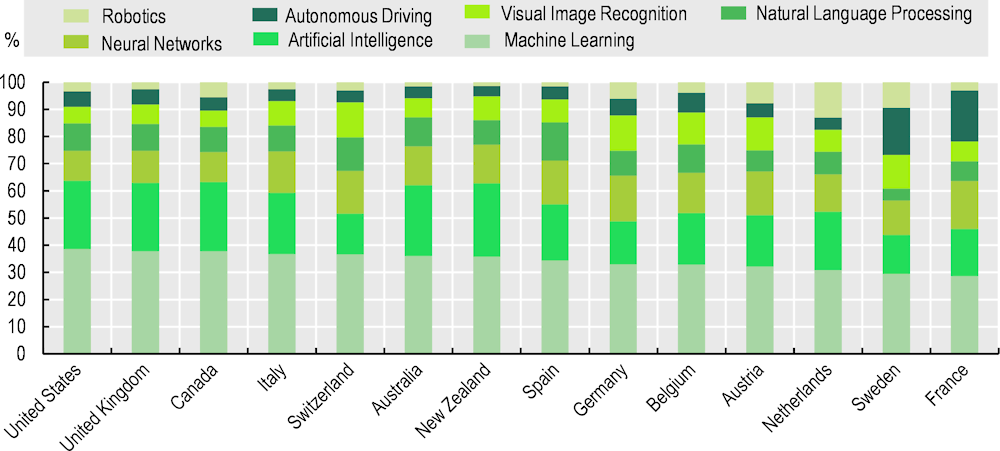
Note: The figure shows the percentage of positions requiring specific skill clusters relative to the total number of AI jobs averaged across 2019‑22, by country. Examples of skills assigned to the different skill clusters are provided in Table 8.1, while a full overview of which AI skills are assigned to which cluster is provided in Borgonovi et al. (2023[1]). Positions requiring AI skills are positions in which at least two generic AI skills or at least one AI-specific skill were required [see Borgonovi et al. (2023[1]) on generic and specific skills]. Countries are sorted in descending order by percentage of postings requiring Machine Learning skills.
Source: Calculations based on Lightcast (2022[35]), Lightcast™, https://lightcast.io/ (accessed December 2022), in Borgonovi et al. (2023[1]), ”Emerging trends in AI skill demand across 14 OECD countries”, https://doi.org/10.1787/7c691b9a-en.
8.3.2. AI job postings rarely mention ethical decision making
AI systems have the potential to support and improve decision making and perform complex analytical tasks, but at the same time, they pose unique ethical challenges. The Recommendation on Artificial Intelligence – the first intergovernmental standard on AI – was adopted by the OECD Council at the Ministerial level on 22 May 2019 (OECD, 2019[36]). The Recommendation aims to foster innovation and trust in AI by promoting the responsible stewardship of trustworthy AI while ensuring respect for human rights and democratic values. Complementing existing OECD standards in areas such as privacy, digital security risk management and responsible business conduct, the Recommendation focuses on AI-specific issues and sets a standard that is implementable and sufficiently flexible to stand the test of time in this rapidly evolving field. In June 2019, at the Osaka Summit, Group of Twenty (G20) Leaders welcomed the G20 AI Principles drawn from the OECD Recommendation (OECD, 2019[36]).
The Recommendation identifies five complementary values-based principles for the responsible stewardship of trustworthy AI and calls on AI actors to promote and implement them: 1) inclusive growth, sustainable development and well-being; 2) human-centred values and fairness; 3) transparency and explainability; 4) robustness, security and safety; and 5) accountability.
Alongside strong regulatory frameworks, ensuring the implementation of the OECD AI Principles requires AI developers and users to embed ethical considerations into the design, development, adaptation and ultimate use of AI systems.
Despite the adoption of the OECD AI Principles and growing concerns about the importance of the ethical risks associated with AI development and use when prospective employers advertise positions for people to develop and adapt AI systems in their work, they only rarely mention ethical decision making.
Figure 8.9 shows the share of online AI job postings for positions involving tasks related to developing and utilising AI systems. It specifically focuses on the presence of keywords associated with AI ethics, trustworthy AI, responsible AI or ethical AI. Results indicate that in the majority of countries, less than 1% of all vacancies mentioned keywords associated with AI ethics, trustworthy AI, responsible AI or ethical AI. However, between 2019 and 2022, the share of positions mentioning keywords related to ethics in AI increased sharply in the majority of countries.
For example, in the United States in 2019, only 0.1% of all online job postings for AI professionals mentioned any keyword associated with ethics in AI in vacancies requiring prospective workers to possess skills related to AI development and use. This increased to 0.5% in 2022. Similarly, in Germany, the share of AI positions advertised requiring ethics in AI increased from less than 0.05% in 2019 to 0.4% in 2022; in the United Kingdom, this increased from around 0.1% in 2019 to 0.4% in 2022 and in Spain, it increased from less than 0.1% in 2019 to 0.3% in 2022.
Figure 8.9. AI job postings mentioning keywords related to ethics, 2019-22
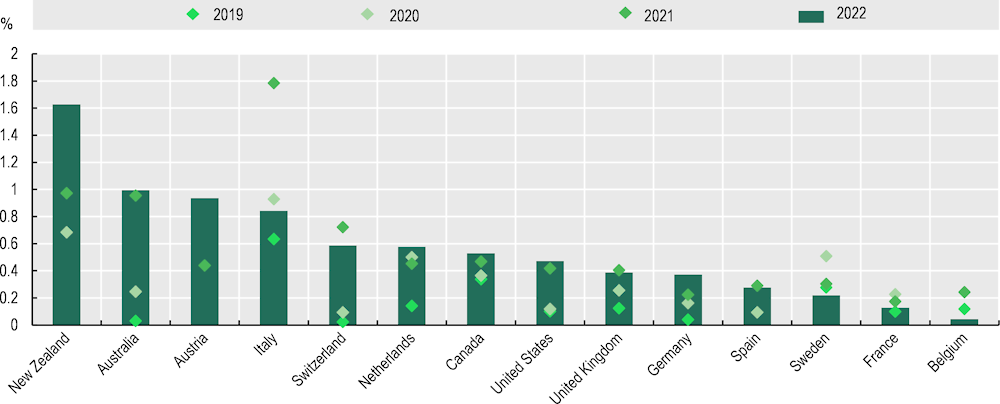
Note: The figure shows the percentage of AI job postings mentioning AI ethics-related keywords. The AI keywords used were “ethical artificial intelligence”, “ethical ai”, “ai ethics”, “ethics of artificial intelligence”, “responsible ai”, “responsible artificial intelligence”, “trustworthy AI” and “trustworthy artificial intelligence” as well as similar terms in the official languages spoken in the different countries.
Source: Calculations based on Lightcast (2022[35]), Lightcast™, https://lightcast.io/ (accessed December 2022), in Borgonovi et al. (2023[1]), ”Emerging trends in AI skill demand across 14 OECD countries”, https://doi.org/10.1787/7c691b9a-en.
8.3.3. The demand for AI professionals among large employers of AI talent (top AI employers)
This section focuses on a subset of AI employers in the United States, the country with the highest demand for AI talent in online job postings among countries with available data. More specifically, the section considers the demand for workers with AI skills among the top ten employers within each industry, which make up around 43% of all AI postings (just under 315 000) in the United States in 2022. Similar to the previous analyses, this section explores differences in the number of vacancies for positions requiring AI skills across sectors, occupations and skills. However, here, the hiring patterns are further distinguished by employer type.
In particular, AI online vacancies posted by the top ten AI employers (hereafter, “top AI”) are compared to the remaining AI postings posted by other AI employers (hereafter, “other AI”). The top ten AI employers are the ten employers in each industry that posted the largest share of vacancies on line for workers with the skills needed to perform AI tasks. To put any potential differences into perspective, the analyses also extend to postings not mentioning any AI-related skills, i.e. not looking for any AI talent (hereafter, “non‑AI”), hence postings stemming from top AI, other AI or non-AI employers.
Levels of concentration of AI job postings by top AI employers across industries
ICT, Professional Services, Manufacturing, and Finance and Insurance are among the industries with the highest share of AI vacancies posted by the top ten AI employers, as shown in Figure 8.10 in the United States. Figure 8.10 shows that the share of postings requiring AI skills by top employers relative to postings by other AI employers is higher among industries where overall AI vacancy shares are comparatively low. For example, although around 3% of vacancies in the US Information industry require AI skills, only around 46% of those are posted by the top ten AI employers. Instead, in Agriculture, around 1.4% of postings require AI skills, but almost 90% are posted by the top ten AI employers.
Figure 8.10. Share of AI vacancies posted by US top AI and other AI employers, by industry, 2022
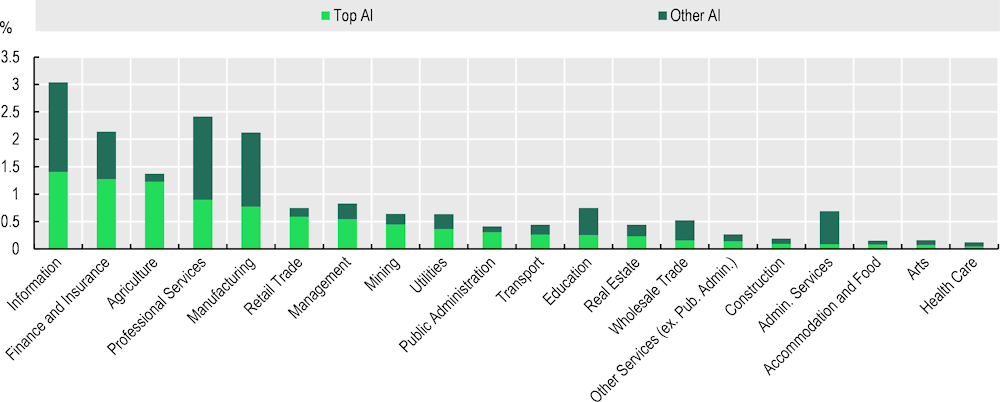
Note: The figure shows the percentage of online vacancies requiring AI skills that are posted by the top ten AI (light green) and other AI (dark green) employers, by industry in the United States, which is the total number of online vacancies requiring AI skills posted by the top ten AI employers vis-à-vis other AI employers relative to online vacancies posted by all employers in the respective industry. The top ten AI employers are the ten employers in each industry that posted the largest share of vacancies on line for workers with the skills needed to perform AI tasks. Positions requiring AI skills are positions in which at least two generic AI skills or at least one AI-specific skill were required [see Borgonovi et al. (2023[1]) on generic and specific skills]. Industries are sorted in descending order by the percentage of positions requiring AI skills posted by the top ten AI employers.
Source: Calculations based on Lightcast (2022[35]), Lightcast™, https://lightcast.io/ (accessed December 2022), in Borgonovi et al. (2023[1]), ”Emerging trends in AI skill demand across 14 OECD countries”, https://doi.org/10.1787/7c691b9a-en.
Top AI employers demand a mix of technical, socio-emotional and foundational skills
Figure 8.11 focusses on the technical, socio-emotional and foundational skills required by the different AI employer types in the United States. More specifically, Figure 8.11 shows the share of AI online vacancies posted by the top ten AI and other AI employer firms that mention a specific technical (Panel A) or socio‑emotional and foundational (Panel B) skill in their job description, focusing on the 20 skills most frequently demanded in AI job postings by the top ten AI employers. The skill profiles demanded by the top ten AI and other AI employers do not vary substantially, with programming languages accounting for many of the most demanded technical skills, especially Python. This is followed by competencies related to Computer and Data Science, as well as skills associated with the widely adopted cloud and computing service Amazon Web Services (AWS).1
Figure 8.11. Top 20 skills in AI job postings by US top AI employers, 2022
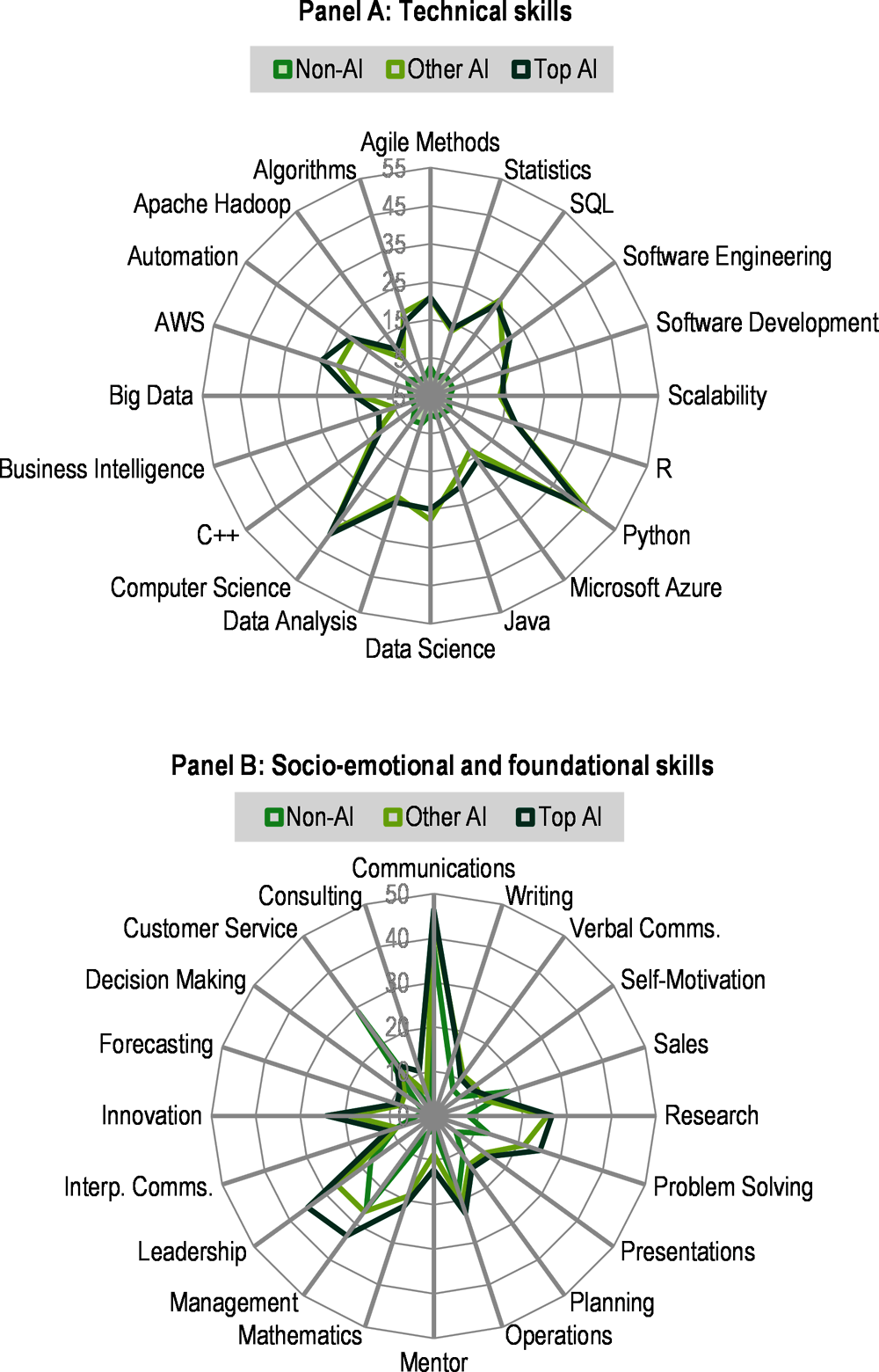
Note: The figure shows the percentage of AI online vacancies for the United States, mentioning a specific technical (Panel A) or socio-emotional and foundational (Panel B) skill, distinguishing vacancies posted by the top ten AI and other AI employers. It also presents the percentage of the remaining non-AI online vacancies. The top ten AI employers are the ten firms in each industry that posted the largest share of vacancies online for workers with the skills needed to perform AI tasks. Therefore, for both the top ten AI and other AI employers, the share is defined as their total number of AI vacancies requiring a specific skill relative to their total number of AI vacancies; hence, the shares across all skills can exceed 100%. The figure displays only the top 20 skills in each panel based on the share of AI jobs posted by the top ten AI employers. The underlying data to the figure for technical and soft skills is provided in Borgonovi et al. (2023[1]). Positions requiring AI skills are positions in which at least two generic AI skills or at least one AI-specific skill were required [see Borgonovi et al. (2023[1]) on generic and specific skills].
Source: Calculations based on Lightcast (2022[35]), Lightcast™, https://lightcast.io/ (accessed December 2022), in Borgonovi et al. (2023[1]), ”Emerging trends in AI skill demand across 14 OECD countries”, https://doi.org/10.1787/7c691b9a-en.
Compared to other AI firms, a larger share of the top AI employers requires skills related to AWS, Business Intelligence, Microsoft Azure, Apache Hadoop, Java, Software Engineering, Data Analysis, and Big Data. Unsurprisingly, none of these skills is particularly evident in non-AI online vacancies, i.e. vacancies not requiring any AI-related skills.
By contrast, the frequency with which socio-emotional and foundational skills appear in AI and non-AI online vacancies is more similar. In particular, Communication skills are very common in postings across the board. However, more AI-related online vacancies, compared to non-AI postings, demand Leadership and Management skills, as well as Innovation, Research, Problem Solving and Mentorship, reflecting the need for AI workers to be endowed with a broad skill mix, including technical as well as socio-emotional and creativity-related skills. In fact, except for Customer Service and skills related to Sales, employers demand either of the remaining socio-emotional and foundational skills presented in Figure 8.11 more frequently in AI than non-AI postings.
In particular, top ten AI employers put relatively more emphasis on Leadership, Mentorship and Management skills – as well as Innovation and Problem-Solving skills – than other AI employers. Compared to other AI firms, the top ten firms also more frequently request competencies related to Customer Service, Forecasting, Operations, Planning or Maths. Interestingly, although different types of Communication skills are highly demanded across all online vacancies, top AI employers demand Interpersonal Communication and Presentation skills more often.
8.4. Attitudes and dispositions needed to make the most of generative AI systems
The previous section details the set of skills that are required of workers engaged in the development, maintenance and adoption of AI technologies. However, the impact of AI on economies and societies will also depend on the set of skills, attitudes and dispositions of broader populations who may use products and technologies enabled and supported by AI. The French writer François Gaston, duc de Lévis, suggested that people should be judged by the quality of their questions rather than the quality of their answers (duc de Lévis, 1810[37]). Generative AI systems reduce the time and effort required to generate output based on existing knowledge, i.e. to produce answers. However, the quality of the outputs produced by generative AI systems depends on the quality of the prompts humans develop, the problems they prioritise and how such problems are framed. Furthermore, how the outputs produced by AI are used depends on how humans interpret them. In other words, at least in the near term, realising the potential of generative AI will depend on individuals being able to generate high-quality prompts – i.e. asking good questions – and being able to evaluate the quality of the answers and interpret them in ways that make them applicable to real-life problems and situations. Finally, responsible use of AI requires human users to be ready to discard the output of AI systems and take an alternative course of action.
Decision making over the if, when, and how outputs of AI systems are used to perform different tasks clearly requires individuals to possess the content knowledge needed to evaluate AI’s output. At the same time, strong content knowledge must be accompanied by the belief in the superiority of one’s own knowledge and skills over those of the AI being used, a prerequisite for individuals to assume responsibility for making decisions over the use of AI’s outputs. This belief requires people to have a high level of confidence and self-efficacy in their abilities in general and their preferred course of action in specific contexts. It also requires them to be willing to make mistakes when choosing to discard input provided by AI systems which, ex post, might have proved right. At the same time, the attitudes and behaviours of managerial structures in firms will also require new accountability practices with the aim of fostering the empowerment of workers in relation to the use of AI.
A potential paradox arises from certain attributes that define tasks where AI systems are unable to replace humans in the foreseeable future. This paradox stems from the discomfort experienced by many individuals and organisations when operating in situations where their comparative advantage lies, i.e. situations with only little prior evidence and that reflect cultural and contextual nuances. Rather than seeing such uncertain situations as opportunities, individuals currently view them as threats. Psychological research suggests that for many people, the toll associated with the experience of losses is larger than the benefit associated with the experience of gains, and, as a result, faced with the prospect of failure, they are more likely to settle for a suboptimal course of action from a “rational perspective” to avoid experiencing losses (Kahneman and Tversky, 1979[38]).
8.4.1. Most people in OECD countries consider the term “risk” more as danger than opportunity
In statistics, the term “risk” refers to the probability that an event will occur. Therefore, risk refers to the known level of uncertainty that certain events will occur and is neutral, i.e. does not have positive or negative connotations. Nonetheless, Figure 8.12 suggests that, except for Latvia, more than one in two adults in OECD countries perceive the term “risk” as denoting danger. In Mexico, Colombia, Spain and Costa Rica, over 80% of adults perceived “risk” to indicate danger, whereas in Croatia, Slovenia, Lithuania, Bulgaria, Germany, Estonia, Poland, Austria, Hungary and Greece, between 50% and 60% did.
Figure 8.12. Perception of “risk” as a threat or an opportunity in selected countries, 2019
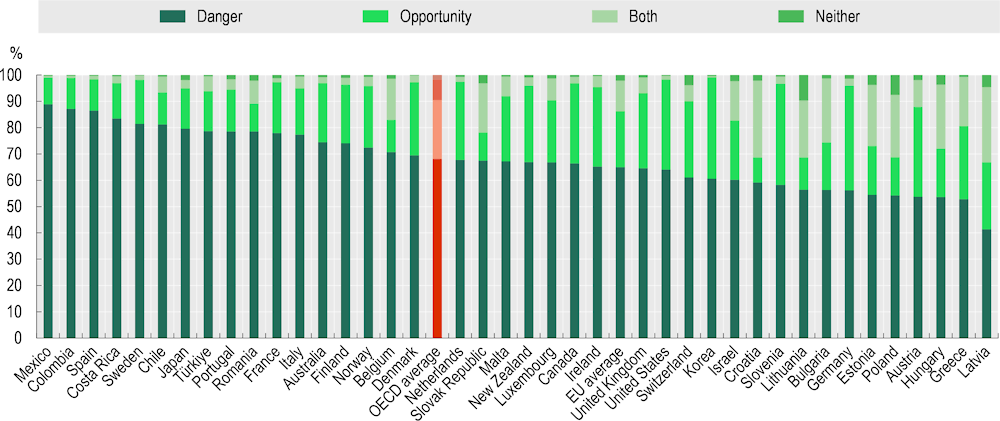
Note: The figure shows the percentage of adults who respond “opportunity, “danger”, “both”, or “neither” to the following question, ”When you hear the word ‘risk’, do you think more about opportunity or danger?”. Countries are sorted in descending order by the percentage of adults reporting perceiving risk as a danger.
Source: World Risk Poll (2019[39]), The Lloyd’s Register Foundation World Risk Poll Report 2019, https://wrp.lrfoundation.org.uk/.
Individuals’ perception of the word “risk” as associated with danger differs across socio-economic characteristics. In particular, on average, across OECD countries, 64% of men but 72% of women reported that they associate the word “risk” with danger rather than opportunity, a difference of 8 percentage points (Figure 8.13). Similarly, across OECD countries, perceptions of risk as reflecting danger were more prevalent among lower-income than higher-income groups. For example, among the poorest 20% of the population in each country, 70% reported that the word “risk” indicates danger, whereas 65% of the 20% richest did. By contrast, differences in perceptions of risk as reflecting danger were less pronounced across levels of educational attainment: on average, 69% of individuals without a tertiary qualification reported that the word risk indicates danger rather than opportunity, while among those with a tertiary-level qualification, 66% did.
Figure 8.13. Perceptions of risk as a danger, by gender, income and educational attainment, OECD average, 2019
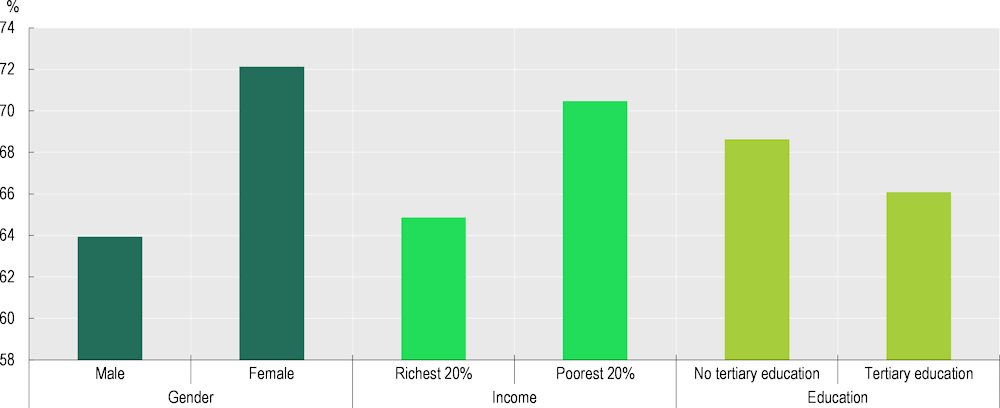
Note: The figure shows the percentage of adults who respond “danger” to the following question, ”When you hear the word ‘risk’, do you think more about opportunity or danger?”, by gender, income and educational attainment.
Source: World Risk Poll (2019[39]), The Lloyd’s Register Foundation World Risk Poll Report 2019, https://wrp.lrfoundation.org.uk/.
8.4.2. Many young people fear failure, and a fear of failure is especially strong among girls
Fear of failure characterises the tendency of individuals to avoid making mistakes when performing a task or an activity because failure is perceived as shameful (Borgonovi and Han, 2020[40]; McGregor and Elliot, 2005[41]). The level of fear is determined by the perceived level of risk of failing (i.e. how hard a task is) and by the costs associated with failure (which can include the missed opportunity of benefiting from success as well as social stigma and judgement and other negative consequences that can arise from failure (Lazarus, 1991[42]; Warr, 2000[43]). Failure avoidance can lead individuals to limit their choices and take fewer risks than optimal given their abilities. The literature indicates that fear of failure is higher among women than among men (McGregor and Elliot, 2005[41]) and that women are more likely to experience negative outcomes when they fear failure (Wach et al., 2015[44]). These findings are consistent with research indicating that women respond less positively to competitive environments (Croson and Gneezy, 2009[45]; Niederle and Vesterlund, 2010[46]), tend to be more risk-averse (Fisk, 2018[47]) and have lower levels of self-efficacy and self-concept than males with similar levels of achievement (Goldman and Penner, 2014[48]).
To the extent that organisations responding to advances in AI and robotics will require individuals to perform a larger number of tasks that entail a high degree of uncertainty, managers will have to develop metrics of success that reflect the inherent uncertainty in outcomes despite the level of effort and the proficiency of workers. At the same time, as a precondition of success, workers will have to develop an appreciation of failure and failing that is free from stigma and grow accustomed to engaging with harder and less predictable tasks and situations.
Figure 8.14 suggests that, on average, across OECD countries, 73% of 15-year-old students report that failure makes them doubt their talent. In Japan, 87% of 15-year-old students indicated that failure made them doubt their talent; in the Slovak Republic, this figure was 84%; in Korea, 83%; in Ireland and Denmark, 82%. By contrast, 56% of 15-year-old students in the Netherlands and Austria indicated that failure made them doubt their talent; this figure was 50% in Germany.
Figure 8.14. Students’ fear of failure in selected countries, 2018
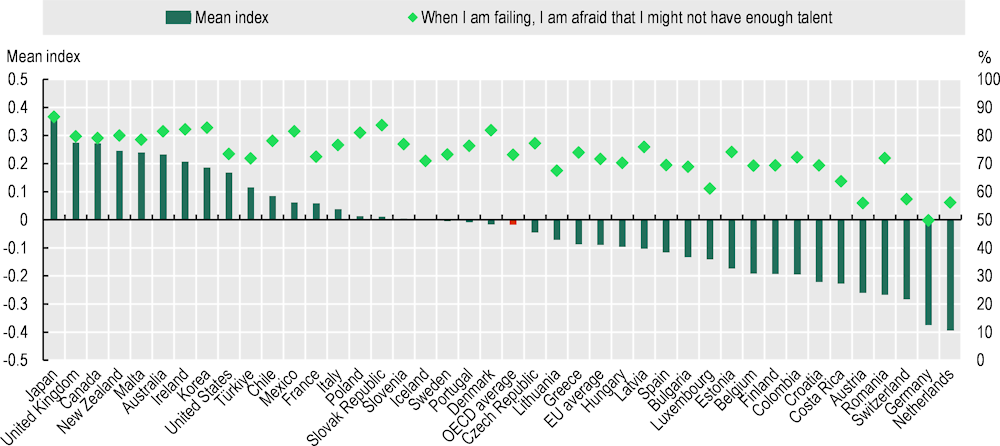
Note: The figure shows the mean of students’ general fear of failure, which consists of three items: “When I am failing, I worry about what others think of me”, “When I am failing, I am afraid that I might not have enough talent”, and “When I am failing, this makes me doubt my plans for the future” (left y-axis). The percentage of students who agree and strongly agree with the statement, “When I am failing, this makes me doubt my plans for the future”, is provided on the right y-axis. Countries are sorted in descending order by the mean index of students’ general fear of failure.
Source: OECD (2018[49]), PISA database 2018, https://www.oecd.org/pisa/data/2018database/.
In line with previous empirical evidence on adult populations, Figure 8.15 reveals that girls are considerably more likely than boys to express a fear of failure. For example, on average across OECD countries, 67% of boys, but 77% of girls, indicate that when failing, they worry about what others will think of them; 65% of boys, but 81% of girls, indicate that when failing, they worry that they might not have enough talent; and 45% of boys, but 61% of girls, indicate that failing makes them doubt their plans for the future. By contrast, there are no differences in fear of failure across students with and without at least one parent with a tertiary‑level qualification. For example, on average across OECD countries, 73% of 15-year-old students with, and 72% of students without, a tertiary-educated parent indicate that when failing, they worry about what others will think of them; 73% indicate that when failing, they worry that they might not have enough talent; and 54% and 53% indicate that failing makes them doubt their plans for the future.
Figure 8.15. Students’ fear of failure in selected countries, by gender and parental education, 2018
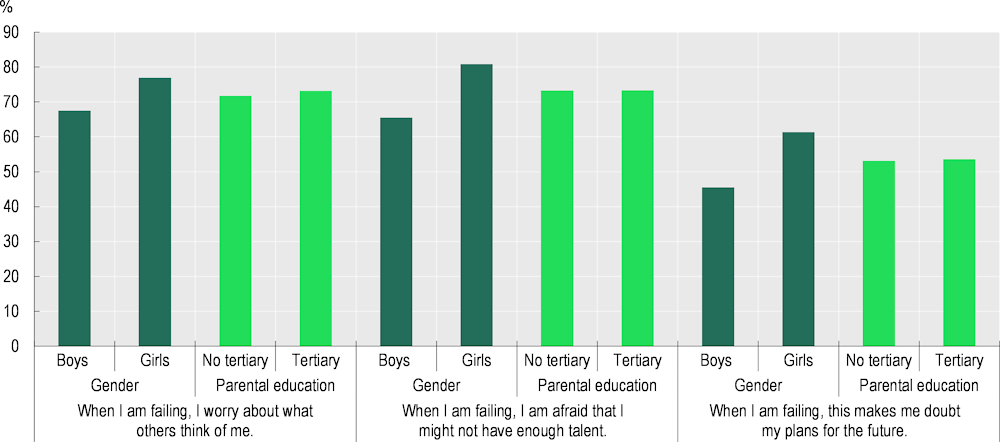
Note: The figure shows the percentage of students who “agree” or “strongly agree” with the statements, “When I am failing, I worry about what others think of me”, “When I am failing, I am afraid that I might not have enough talent”, and “When I am failing, this makes me doubt my plans for the future”, by gender and parental education.
Source: OECD (2018[49]), PISA database 2018, https://www.oecd.org/pisa/data/2018database/.
8.5. Conclusions
Humans tend to anthropomorphise, i.e. to “ascribe” human traits to non-human entities and to engage with them as if these were human (Festerling and Siraj, 2021[50]). Despite this tendency, robotics and AI systems, including large language models such as GPT4, should be recognised for what they are: tools created by humans that, at their core, combine in complex ways, work previously developed by other humans (Lanier, 2023[51]). This means that whereas machines excel at summarising and identifying patterns in the work previously conducted by other humans, they are instruments in support of human decision making and do not yet have an independent level of autonomy.
Evaluating the role of skills policies in supporting responsible AI development and deployment and developing effective policies as a result rests on a better understanding of: 1) the demand for workers involved in the development and deployment of AI, 2) the skills demanded of them, 3) the attitudes and dispositions that shape human decision making, and 4) the attitudes which may be affected by large-scale deployment of generative AI. As highlighted by the findings regarding the hopes and concerns expressed by populations regarding the potential impact of AI on people’s opportunities, it is essential to ensure that the talent engaged in AI development and deployment possesses not only the technical skills required for their work but also the ability to foster the development of responsible and ethical AI systems.
Results presented in this chapter indicate that, even following a marked increase in the demand for professionals with AI skills, AI-related online vacancies represent only a small share of all job vacancies that were posted on line in the 14 countries analysed in 2019 to 2022. In particular, the share of AI-related online job vacancies was highest in the United States in 2022, where AI-related vacancies represented 0.84% of all vacancies posted on line. On average, across the countries in the sample, shares of online vacancies requiring AI skills were 0.30% in 2019 and 0.40% in 2022. The fact that only a small share of new positions advertised on line required individuals involved in the development and deployment of AI, coupled with the major impact AI systems can have, suggests that a relatively small number of people have the potential to exert a profound impact on the global economy and human societies.
Some skills – notably those related to Machine Learning – appear particularly pervasive among AI-related job postings. Indeed, across all countries, between 29% (in France) and 39% (in the United States) of all AI job postings require skills related to Machine Learning. Skills related to Autonomous Driving and Robotics appear, on average, less demanded, possibly reflecting their more industry-specific nature. They also exhibit a high degree of cross-country variation, possibly reflecting the relative importance of different sectors in different countries and their likely evolution, given industry-specific trends and industrial investment decisions.
A set of analyses examined differences in skills requirements among different firms in the United States, where the AI sector has been comparatively large for the longest. Results reveal that top AI firms tend to demand some technical skills more intensively and require Leadership and Management skills more frequently, as well as Innovation and Problem-Solving skills. This reflects the importance such companies place on their employees having a broad skill mix. The hiring decisions of top ten AI firms may anticipate trends in skills requirements in the broader economy as AI permeates a broader set of firms.
Despite a growing awareness of the importance of ethical considerations in the development of AI, only a very small minority of firms advertising positions requesting prospective employees to possess AI skills mentioned ethics and responsible AI in online vacancies. By 2022, only 0.3% of AI positions advertised in Canada, 0.5% in the United Kingdom and 0.4% in the United States requested skills related to ethics in AI. This suggests that despite strong commitments on the part of countries and stated intentions on the part of AI development firms, ethics in AI is not yet prioritised in hiring decisions. These considerations should be prioritised alongside considerations over the lack of diversity of the AI workforce and the implications this has for the AI systems that are being developed.
A final set of analyses illustrated variations across countries and by socio-economic groups in the attitudes adults and youngsters have with respect to risk and failure. Attitudes and dispositions related to decision making under uncertainty are likely to shape how AI will be adopted by people and organisations worldwide; their ability to adopt AI as a useful instrument and aid in independent decision making; and willingness to engage in tasks in which humans are at a comparative advantage over AI systems, i.e. situations that are data poor and marred with a high degree of uncertainty. Results reveal that large sections of OECD populations hold negative attitudes towards risk and uncertainty and have high levels of fear of failure, especially women and girls. These results are concerning because they may be an early signal that women might be less ready to engage in tasks in which AI tools perform poorly and to override AI algorithms in their decision-making processes as AI outputs improve in quality and AI systems become widely adopted. These findings should be considered alongside results indicating a lack of representativeness among AI workers (Green and Lamby, 2023[28]) and findings highlighted in Chapter 5 of this report on boys’ higher levels of overconfidence. They should also be considered in relation to societal perceptions that boys/men are more likely than girls/women to be endowed with exceptional talent (Leslie et al., 2015[52]; Zhao et al., 2022[53]) and the fact that such perceptions allow boys and men to experience failure as a possible precursor of success rather than evidence of underlying (lack of) potential.
Analysing the human element behind advances in AI makes it possible to assess the scale and diversity of the demand for AI jobs and skills. Such analysis may help in designing interventions to promote the diffusion of AI throughout the economy, particularly among employers and sectors that have yet to fully benefit from its potential. The adoption of AI encompasses both the utilisation of AI services, such as ChatGPT or Amazon (widely used services in OECD countries), which leverage AI in their service provision, as well as the active integration and customisation of AI algorithms in production and service delivery, as seen, for example, in the development of an online dating app. Such analyses are also a useful reminder that humans drive technical developments in AI diffusion and adoption and, as such, AI developments can ultimately be the subject of human decision making.
References
[26] Acemoglu, D. et al. (2022), “Artificial intelligence and jobs: Evidence from online vacancies”, Journal of Labor Economics, Vol. 40/S1, pp. S293-S340, https://doi.org/10.1086/718327.
[13] Agrawal, A., J. Gans and A. Goldfarb (2022), Power and Prediction: The Disruptive Economics of Artificial Intelligence, Harvard Business Review Press.
[27] Alekseeva, L. et al. (2021), “The demand for AI skills in the labor market”, Labour Economics, Vol. 71, p. 102002, https://doi.org/10.1016/j.labeco.2021.102002.
[30] Alekseeva, L. et al. (2020), “AI adoption and firm performance: Management versus IT”, SSRN Electronic Journal, https://doi.org/10.2139/ssrn.3677237.
[19] American Federation of Labor and Congress of Industrial Organizations (AFL-CIO) (2023), The State of Labor Unions Is Strong, https://aflcio.org/sites/default/files/2023-08/GBAO%20AFL-CIO%20Labor%20Day%20Poll%20Memo.pdf (accessed on 19 October 2023).
[22] Andrews, D., C. Criscuolo and P. Gal (2016), “The best versus the rest: The global productivity slowdown, divergence across firms and the role of public policy”, OECD Productivity Working Papers, No. 5, OECD Publishing, Paris, https://doi.org/10.1787/63629cc9-en.
[10] Arntz, M., T. Gregory and U. Zierahn (2016), “The risk of automation for jobs in OECD countries: A comparative analysis”, OECD Social, Employment and Migration Working Papers, No. 189, OECD Publishing, Paris, https://doi.org/10.1787/5jlz9h56dvq7-en.
[3] Autor, D., F. Levy and R. Murnane (2003), “The skill content of recent technological change: An empirical exploration”, The Quarterly Journal of Economics, Vol. 118/4, pp. 1279-1333, https://doi.org/10.1162/003355303322552801.
[31] Babina, T. et al. (2020), “Artificial intelligence, firm growth, and industry concentration”, SSRN Electronic Journal, https://doi.org/10.2139/ssrn.3651052.
[11] Bessen, J. (2016), “How computer automation affects occupations: Technology, jobs, and skills”, Boston University School of Law, Law and Economics Research Paper, Vol. 15-49, https://scholarship.law.bu.edu/cgi/viewcontent.cgi?article=1811&context=faculty_scholarship.
[20] Bi, W. et al. (2019), “Artificial intelligence in cancer imaging: Clinical challenges and applications”, CA: A Cancer Journal for Clinicians, https://doi.org/10.3322/caac.21552.
[1] Borgonovi, F. et al. (2023), Emerging trends in AI skill demand across 14 OECD countries, https://doi.org/10.1787/7c691b9a-en.
[40] Borgonovi, F. and S. Han (2020), “Gender disparities in fear of failure among 15‐year‐old students: The role of gender inequality, the organisation of schooling and economic conditions”, Journal of Adolescence, Vol. 86/1, pp. 28-39, https://doi.org/10.1016/j.adolescence.2020.11.009.
[21] Borgonovi, F., J. Hervé and H. Seitz (2023), “Not lost in translation: The implications of machine translation technologies for language professionals and for broader society”, OECD Social, Employment and Migration Working Papers, No. 291, OECD Publishing, Paris, https://doi.org/10.1787/e1d1d170-en.
[6] Brynjolfsson, E., D. Rock and C. Syverson (2017), Artificial Intelligence and the Modern Productivity Paradox: A Clash of Expectations and Statistics, National Bureau of Economic Research, Cambridge, MA, https://doi.org/10.3386/w24001.
[25] Calvino, F. and L. Fontanelli (2023), “A portrait of AI adopters across countries: Firm characteristics, assets’ complementarities and productivity”, OECD Science, Technology and Industry Working Papers, No. 2023/02, OECD Publishing, Paris, https://doi.org/10.1787/0fb79bb9-en.
[23] Calvino, F. et al. (2022), “Identifying and characterising AI adopters: A novel approach based on big data”, OECD Science, Technology and Industry Working Papers, No. 2022/06, OECD Publishing, Paris, https://doi.org/10.1787/154981d7-en.
[45] Croson, R. and U. Gneezy (2009), “Gender differences in preferences”, Journal of Economic Literature, Vol. 47/2, pp. 448-474, https://doi.org/10.1257/jel.47.2.448.
[24] Dernis, H. et al. (2023), “Identifying artificial intelligence actors using online data”, OECD Science, Technology and Industry Working Papers, No. 2023/01, OECD Publishing, Paris, https://doi.org/10.1787/1f5307e7-en.
[37] duc de Lévis, P. (1810), Maximes et réflections sur différents sujets de morale et de politique.
[50] Festerling, J. and I. Siraj (2021), “Anthropomorphizing technology: A conceptual review of anthropomorphism research and how it relates to children’s engagements with digital voice assistants”, Integrative Psychological and Behavioral Science, Vol. 56/3, pp. 709-738, https://doi.org/10.1007/s12124-021-09668-y.
[47] Fisk, S. (2018), “Who’s on top? Gender differences in risk-taking produce unequal outcomes for high-ability women and men”, Social Psychology Quarterly, Vol. 81/3, pp. 185-206, https://doi.org/10.1177/0190272518796512.
[7] Georgieff, A. and R. Hyee (2021), “Artificial intelligence and employment: New cross-country evidence”, OECD Social, Employment and Migration Working Papers, No. 265, OECD Publishing, Paris, https://doi.org/10.1787/c2c1d276-en.
[48] Goldman, A. and A. Penner (2014), “Exploring international gender differences in mathematics self-concept”, International Journal of Adolescence and Youth, Vol. 21/4, pp. 403-418, https://doi.org/10.1080/02673843.2013.847850.
[28] Green, A. and L. Lamby (2023), “The supply, demand and characteristics of the AI workforce across OECD countries”, OECD Social, Employment and Migration Working Papers, No. 287, OECD Publishing, Paris, https://doi.org/10.1787/bb17314a-en.
[4] Ikenaga, T. and R. Kambayashi (2016), “Task polarization in the Japanese labor market: Evidence of a long-term trend”, Industrial Relations: A Journal of Economy and Society, Vol. 55/2, pp. 267-293, https://doi.org/10.1111/irel.12138.
[38] Kahneman, D. and A. Tversky (1979), “Prospect theory: An analysis of decision under risk”, Econometrica, Vol. 47/2, p. 263, https://doi.org/10.2307/1914185.
[18] Lane, M., M. Williams and S. Broecke (2023), “The impact of AI on the workplace: Main findings from the OECD AI surveys of employers and workers”, OECD Social, Employment and Migration Working Papers, No. 288, OECD Publishing, Paris, https://doi.org/10.1787/ea0a0fe1-en.
[51] Lanier, J. (2023), There Is No A.I - There are ways of controlling the new technology—but first we have to stop mythologizing it, https://www.newyorker.com/science/annals-of-artificial-intelligence/there-is-no-ai (accessed on 24 April 2023).
[12] Lassébie, J. and G. Quintini (2022), “What skills and abilities can automation technologies replicate and what does it mean for workers?: New evidence”, OECD Social, Employment and Migration Working Papers, No. 282, OECD Publishing, Paris, https://doi.org/10.1787/646aad77-en.
[42] Lazarus, R. (1991), Emotion and Adaptation, Oxford University Press, New York, NY.
[52] Leslie, S. et al. (2015), “Expectations of brilliance underlie gender distributions across academic disciplines”, Science, Vol. 347/6219, pp. 262-265, https://doi.org/10.1126/science.1261375.
[34] Lightcast (2023), New Skills Sought for Data Scientists, Machine Learning Engineers, Curriculum Writers in Past 12 Months, https://lightcast.io/resources/blog/generative-ai-10-19-2023 (accessed on 23 October 2023).
[35] Lightcast (2022), Lightcast™, https://lightcast.io/ (accessed on 5 December 2022).
[32] Manca, F. (2023), “Six questions about the demand for artificial intelligence skills in labour markets”, OECD Social, Employment and Migration Working Papers, No. 286, OECD Publishing, Paris, https://doi.org/10.1787/ac1bebf0-en.
[41] McGregor, H. and A. Elliot (2005), “The shame of failure: Examining the link between fear of failure and shame”, Personality and Social Psychology Bulletin, Vol. 31/2, pp. 218-231, https://doi.org/10.1177/0146167204271420.
[46] Niederle, M. and L. Vesterlund (2010), “Explaining the gender gap in math test scores: The role of competition”, Journal of Economic Perspectives, Vol. 24/2, pp. 129-144, https://doi.org/10.1257/jep.24.2.129.
[8] OECD (2023), Is Education Losing the Race with Technology?: AI’s Progress in Maths and Reading, Educational Research and Innovation, OECD Publishing, Paris, https://doi.org/10.1787/73105f99-en.
[2] OECD (2023), OECD Employment Outlook 2023: Artificial Intelligence and the Labour Market, OECD Publishing, Paris, https://doi.org/10.1787/08785bba-en.
[9] OECD (2023), “Putting AI to the test: How does the performance of GPT and 15-year-old students in PISA compare?”, OECD Education Spotlights, No. 6, OECD Publishing, Paris, https://doi.org/10.1787/2c297e0b-en.
[14] OECD (2021), AI and the Future of Skills, Volume 1: Capabilities and Assessments, Educational Research and Innovation, OECD Publishing, Paris, https://doi.org/10.1787/5ee71f34-en.
[36] OECD (2019), Recommendation of the Council on Artificial Intelligence, OECD Publishing, Paris, https://legalinstruments.oecd.org/en/instruments/oecd-legal-0449.
[49] OECD (2018), PISA database 2018, https://www.oecd.org/pisa/data/2018database/.
[16] OECD (forthcoming), AI and the Future of Skills, Volume 2: Methods for evaluating AI capabilities.
[29] Samek, L., M. Squicciarini and E. Cammeraat (2021), “The human capital behind AI: Jobs and skills demand from online job postings”, OECD Science, Technology and Industry Policy Papers, No. 120, OECD Publishing, Paris, https://doi.org/10.1787/2e278150-en.
[5] Spitz‐Oener, A. (2006), “Technical change, job tasks, and rising educational demands: Looking outside the wage structure”, Journal of Labor Economics, Vol. 24/2, pp. 235-270, https://doi.org/10.1086/499972.
[33] Squicciarini, M. and H. Nachtigall (2021), “Demand for AI skills in jobs: Evidence from online job postings”, OECD Science, Technology and Industry Working Papers, No. 2021/03, OECD Publishing, Paris, https://doi.org/10.1787/3ed32d94-en.
[15] Violante, G. (2008), “Skill-Biased Technical Change”, in The New Palgrave Dictionary of Economics, Palgrave Macmillan UK, London, https://doi.org/10.1057/978-1-349-95121-5_2388-1.
[44] Wach, F. et al. (2015), “Sex differences in secondary school achievement – The contribution of self-perceived abilities and fear of failure”, Learning and Instruction, Vol. 36, pp. 104-112, https://doi.org/10.1016/j.learninstruc.2015.01.005.
[43] Warr, M. (2000), “Fear of Crime in the United States: Avenues for Research and Policy”, Criminal Justice, Vol. 4, pp. 451-489, https://www.ncjrs.gov/criminal_justice2000/vol_4/04i.pdf (accessed on 23 May 2023).
[17] World Risk Poll (2021), World Risk Poll 2021: A Digital World - Perceptions of Risk from AI and Misuse of Personal Data, https://wrp.lrfoundation.org.uk/LRF_2021_report_a-digtial-world-ai-and-personal-data_online_version.pdf.
[39] World Risk Poll (2019), The Lloyd’s Register Foundation World Risk Poll Report 2019, https://wrp.lrfoundation.org.uk/.
[53] Zhao, S. et al. (2022), “The acquisition of the gender‐brilliance stereotype: Age trajectory, relation to parents’ stereotypes, and intersections with race/ethnicity”, Child Development, Vol. 93/5, https://doi.org/10.1111/cdev.13809.
Note
← 1. AWS stands as a comprehensive suite of services offered by Amazon and provides businesses with the digital infrastructure to manage data, execute applications, and perform a myriad of other tasks via the internet, bypassing the need for physical servers.