The quality of the distributional results largely depends on the quality and alignment of the micro data and the national accounts totals. Furthermore, assumptions may play an important role, for example in imputing for any missing elements, closing gaps between the micro and macro data, and linking data across different data sets. In this light, it is important to closely assess the consistency and plausibility of the results at the end of the compilation process. This chapter provides an overview of checks that may be conducted in this regard.
OECD Handbook on the Compilation of Household Distributional Results on Income, Consumption and Saving in Line with National Accounts Totals
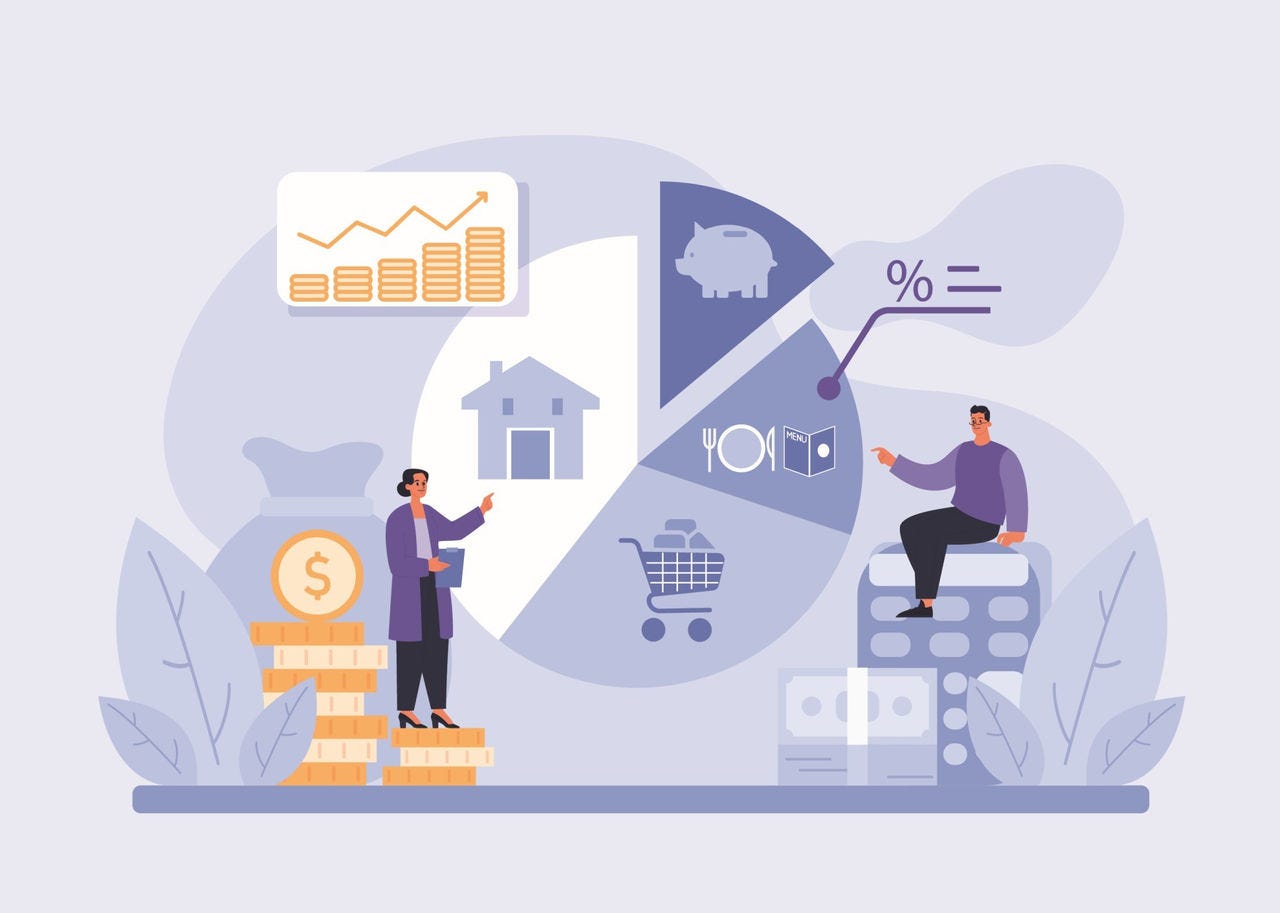
12. Consistency and plausibility checks
Abstract
12.1. Introduction
The previous chapters have explained how national accounts data may be combined with micro data to arrive at distributional results on income, consumption and saving in line with national accounts. It is clear that the quality of these distributional results will be highly dependent on the quality and the alignment of these two sources of information, the size of the items for which micro data is lacking and for which compilers have to rely on assumptions, and the quality of the matching of data across different data sets to arrive at homogenous results for households or household groups. With this in mind, it is important to properly assess the consistency and plausibility of the results at the end of the compilation process. This chapter provides an overview of checks that may be conducted in this respect.
Section 12.2 discusses general approaches to check the consistency and plausibility of the distributional results, both at the level of underlying items and at the level of balancing items. As the distributional results are the outcome of a step-by-step approach, many issues may underlie possible inconsistencies and implausibilities in the results. For that reason, it is important to have insight into how these results have been constructed and how different assumptions in the compilation process (e.g. regarding the allocation of micro-macro gaps or the allocation of imputed items across the distribution) may have led to different results. Section 12.3 presents reconciliation tables that may provide this type of insight. The chapter ends with some conclusions in Section 12.4.
12.2. Checking consistency and plausibility of distributional results
This section discusses approaches that may be used to check the consistency and plausibility of the distributional results. This concerns specific checks that can be applied to certain items due to direct or indirect links with other items as well as general checks that can be applied to all items and aggregates in the DNA work.
12.2.1. Checking internal consistency of distributional results
A first check is to look at the consistency of the distributional results for specific items that have a direct or indirect link to other items in the DNA work. Direct links may result from the setup of the System of National Accounts (SNA) in which some items should be identical by definition (e.g. employers’ actual social contributions received by households as part of their compensation of employees (D121R) and paid by households as part of their social contributions (D611P) are equal by definition) and in which other items may constitute an input in another component in the framework (e.g. property income attributed to non-life insurance policyholders as received by households (D441AR) forms part of insurance premiums paid by households (D71P)).
Table 12.1 provides an overview of direct conceptual links between income and consumption items inherent to the setup of the SNA. The table presents the conceptual link and includes a short explanation. Please refer to the relevant sections on the related items in Chapters 10 and 11 for more information on these conceptual links. Compilers should make sure that these links are respected in compiling distributional results for the relevant items. If they encounter any inconsistencies, they should check where this inconsistency stems from (see also Section 12.3) and try to solve it on the basis of the underlying information.
Table 12.1. Direct links between variables in the system of national accounts
Overview of the conceptual links between income and consumption items in the system of national accounts.
Conceptual link |
Explanation |
|
---|---|---|
Employers’ actual social contributions |
D121R = D611P |
These contributions are presented as being paid by employers to their employees as part of compensation of employees which are then paid by these employees into social insurance schemes (see §8.83 of the 2008 SNA). |
Employers’ imputed social contributions |
D122R = D612P |
These contributions are presented as being paid by employers to their employees as part of compensation of employees which are then paid by these employees into social insurance schemes (see §8.84 of the 2008 SNA). |
Property income received, attributed to non-life insurance policyholders |
D71P ≥ D441AR |
The investment income on reserves related to non-life insurance policies is treated as income to policyholders which is then treated as paid back to the insurance corporation as premium supplement (see §7.142 of the 2008 SNA. |
Investment income payable on pension entitlements |
D614P ≥ D442R |
The investment income payable on pension entitlements is treated as being received by policy holders in the form of property income which is then treated as paid back to the pension fund as social contribution supplement (see §8.86 of the 2008 SNA). |
FISIM |
CP1261 ≤ D41R_FISIM + |D41P_FISIM| |
The amount of FISIM as recorded as correction item for property income should be allocated to intermediate consumption and final consumption for the relevant households. This means that the sum of FISIM recorded as final consumption (CP1261) and as intermediate consumption (reflected in lower values of B2 and B3) should equal the amount of FISIM recorded as correction item in property income at the level of the household (or household group). As a consequence, the consumption item cannot exceed the total amount of FISIM at the household level. |
Change in net equity of households in pension funds |
D8 = D61Ppensions – D62Rpensions |
The adjustment for the change in pension entitlements is derived on the basis of a formula which should hold at the level of the household and consequently also at the level of household groups (see §9.24 of the 2008 SNA). |
Social insurance scheme service charges |
CP125 ≥ D61xP |
Social insurance scheme service charges are presented as a correction item in D61 as they are not part of the social contribution but concern (indirect) payments insurance services. As this consumption item also includes services charges for other types of insurance, the consumption item should at least be equal to the social insurance scheme service charge (see also §8.82 of the 2008 SNA). |
Net lending/net borrowing |
Saving + net capital transfers + net capital accumulation = net lending/net borrowing from financial accounts |
Net lending/net borrowing as derived from the income and capital account should equal net lending/net borrowing as derived from the financial account (see §11.1 of the 2008 SNA). |
In addition to links between specific items, the setup of the system of national accounts also ensures consistency between resources and uses at the level of the various sectors and subsectors in the system. This identity is usually checked by confronting net lending/net borrowing as derived from the current and capital account with that from the financial account. These results should be identical but may differ in practice due to the use of different data sources and different compilation techniques. Any inconsistencies will be reflected in “statistical discrepancies”. The smaller these discrepancies, the better the alignment between the current, capital and financial accounts, and the higher the plausibility of the underlying results.
For this reason, if distributional information is also available for the capital1 and the financial accounts, it is recommended to confront these results with the results derived for income and consumption at the level of the household or the household group. If these show discrepancies, this may point to issues in the underlying data. This check is particularly important as results for various countries showed large negative saving rates for low-income household groups. Whereas this may reflect economic reality, it may also point to weaknesses in the compilation process. Confronting these results with distributional information from the capital and financial accounts may provide more insight into the plausibility of these results. Box 12.1 describes the issue of negative saving in more detail.
Finally, direct checks can be performed on the fact that several items cannot become negative,2 while others can assume negative values by definition. More specifically, in the DNA template, the following items on the income side can assume negative values as a result of the compilation process: operating surplus (B2R),3 mixed income (B3R),4 net property income (D4N), reinvested earnings on foreign direct investment (D43R), balance of primary incomes (B5), net other current transfers (D7N), net non-life insurance claims minus premiums (D72R-D71P), net miscellaneous current transfers (D75N), transfers between resident households (D75x), disposable income (B6) and adjusted disposable income (B7). On the consumption side, it concerns items that may be affected by second-hand trade, such as purchases of vehicles (CP071). Finally, in deriving results for saving, it has to be borne in mind that the adjustment for the change in pension entitlements (D8) and gross saving (B8) may also be negative. When looking at the template, it is also important to note that, as discussed in Section 10.5.1, FISIM related to interest paid (D41P) should be reported with a negative sign. All other items in the template should be reported with a positive sign, regardless of the fact whether they positively or negatively contribute to the balancing items.
In addition to direct links, compilers may also focus on the consistency and plausibility of the distributional results by looking at indirect links between certain items. In that regard, some items may be expected to correlate with others from an economic point of view. Current taxes on income may for example be expected to correlate with primary income. Furthermore, property income items will usually be closely linked to the assets owned and liabilities owed by the specific households or household groups. Table 12.2 provides several examples of indirect links. It will depend on country-specific characteristics how strong these links are and on data availability (e.g. distributional information on wealth will not be available in all countries) and quality (e.g. some types of assets or liabilities may also suffer from large gaps between micro and macro results) which checks may be performed. Furthermore, compilers may also identify other indirect links that may be relevant at the national level.
Although no immediate conclusions can be derived with regard to (in)correctness of some of the distributional results on the basis of these indirect links, they may often provide insight into their plausibility. Particularly in case historical distributional results show strong correlation between certain items, which is no longer present (or less strong) in the current results, this may point to an issue in the data. The same goes if two related items move in opposite directions. Furthermore, if other countries show a strong correlation between specific items whereas this is not the case for the country at stake, this may also raise questions whether there is a specific explanation for this. It does not necessarily imply an error in the data, but it may trigger the compiler to try to assess the rationale underlying this difference.
Table 12.2. Indirect links between variables
Overview of indirect links between items that may provide insight into possible implausibilities in the distributional results.
Economic link |
Explanation |
|
---|---|---|
Link between wages and salaries and employers’ actual social contributions |
D121R versus D11R |
Often social contributions will be linked to wages and salaries of employees. It may not always concern a fixed percentage, but at least a correlation may be expected. Information may be available at the country-level on the exact link. |
Link between financial intermediation services and interest receipts and payments |
D41R_FISIM versus D41R and D41P_FISIM versus D41P |
FISIM implies that the actual interest received from and actual interest paid to financial corporations includes a correction for the service provided by the financial corporation. As a consequence, there is a link between the actual interest flows and the amount of FISIM. |
Link between taxes paid and primary income |
D5P versus B5 |
Current taxes on income and wealth will relate to the income received by households. It may depend on specific tax rules in a country how the two relate and which specific items should be taken into consideration, but at least it is expected that this item will relate to primary income received by households. |
Link between operating surplus from housing services produced and consumption of these housing services |
B2R1 versus CP042 |
Housing services produced by homeowners are consumed by the same households. They also constitute the main input for deriving operating surplus from owner-occupied dwellings (B2R1). This is obtained by deducting intermediate costs from these housing services. As a consequence, the distribution of the consumption of imputed rentals on housing is usually closely aligned with the distribution of operating surplus from owner-occupied dwellings. |
Link between property income flows and financial stock data |
D41R versus interest-bearing assets (AF.2, AF.3, AF.4 and AF.8) D41P versus interest-bearing liabilities (AF.4 and AF.8) D42R versus equity holdings (AF.51) D441AR versus non-life insurance technical reserves (AF.61) D441BR versus life insurance technical reserves (AF.62) D442R versus pension entitlements (AF.63) D443R versus investment fund shares (AF.52) |
Property income is the income receivable by the owner of a financial asset or a natural resource in return for providing this asset to another unit. The return may often depend on the type of underlying asset, but it is expected that there is also a strong correlation between the amount of the assets and the income received on these assets. This holds for a range of property income flows received and paid by households. |
Link between net social contributions received, social benefits paid, and mixed income |
D61R and D62P versus B3R3 |
Net social contributions received and social benefits paid by households relate to their role as unincorporated enterprises which run their own social insurance scheme for their employees. As a consequence, there is a link between these amounts and the households that include an unincorporated enterprise. An indication of the latter is formed by the amount of mixed income recorded for a specific household or household group, although there will not be a perfect correlation between the two. |
Link between non-life insurance premiums and non-life insurance technical reserves |
D71P versus non-life insurance technical reserves (AF.61) |
It may be expected that the amount of non-life insurance technical reserves will depend on the amount of non-life insurance premiums that are paid by policy holders. As a consequence, the two may be expected to be correlated. |
Box 12.1. Plausibility of negative saving rates
Negative saving rates have proved to be a common area of focus when evaluating the plausibility of distributional results. In that regard, several countries have reported negative saving rates for the lowest income quintile and some even for the second and/or third income quintile. These results may be viewed with some scepticism as such a situation cannot be maintained over a longer period of time at the individual household level. In that respect, these outcomes may be regarded as statistical artefacts, resulting from the methodology to derive the distributional results, possibly relating to errors in the underlying micro or macro data, incorrect allocation of imputed items (including the imputation for transactions between households), and/or incorrect linking of data across data sources. On the other hand, there may also be plausible economic explanations for negative saving rates for specific groups of households.
First of all, it is important to note that a negative saving rate for a specific household group does not necessarily mean that each household in that group reports negative saving. It just reflects the average for the group as a whole. In that regard, it would be interesting to have more information on the number of households within the group that report negative saving. Also information on the median saving rate would provide useful information. Furthermore, more granular breakdowns into other socio-demographic characteristics (such as household type, education level and age of the head of the household) may provide more insight in specific groups that report negative saving.
Secondly, it is important to keep in mind that household groups, particularly when clustering according to income, may often not consist of the same households over time. For example, if self-employed households experience an unproductive business year and do not earn their normal annual income, they will be grouped in a lower income group in a given period. In future periods they may be re-grouped in a higher income group when their income situation returns to normal. This will also be the case for households that experience temporary unemployment. Furthermore, one would expect upwards movement in income group over time for certain types of households that may be found in the lower income groups in the current recording period such as single-student households. Thus, the fact that the income groups are not fixed over time could provide an explanation for the existence of negative saving for certain income groups for a longer period of time.
Looking at the plausibility of negative saving at the level of individual households, there may also be a number of possible explanations. First of all, as mentioned above, they may concern temporary negative saving for households that became (partly) unemployed or self-employed households that experienced an unproductive business year. Furthermore, a temporary negative saving may be explained by a large purchase for a household which may be financed by disposing assets or by obtaining consumer credit. Information from the financial accounts may provide more insight in that regard. There is also a wealth of economic literature on households’ saving and consumption behaviour related to their position in the life cycle which can provide explanation for negative saving results. This field of study has been guided by two main macro-economic theories, i.e. the Life Cycle Hypothesis (Modigliani and Brumberg, 1954[1]) and the Permanent Income Hypothesis (Friedman, 1957[2]).
The Life Cycle Hypothesis states that individuals plan their consumption on the basis of their entire lifetime such that consumption can remain relatively stable across all periods of their lives. When individuals are young, they accumulate debt as they consume more than they earn and then afterwards when they find employment, they can begin to reduce their debts and accumulate savings for retirement where they will use up their accumulated assets. In this manner, saving over a lifetime would follow an inverted “U”-shaped pattern. Negative saving rates for specific household groups can thus be explained by the presence of single-student households or households drawing on pensions.
The Permanent Income Hypothesis explains that a person’s consumption is based both on their current income as well as their expected future income. This means that when there is a temporary change in income, the adjustment to consumption will be smoothed out over time. According to this hypothesis one would predict that a household suffering from a large negative income shock in a given period would maintain the same level of consumption as in previous periods, possibly leading to temporary negative saving.
In response to the impact of the position of a person or household in its lifecycle on its income and saving levels, it is often argued that consumption may provide more insight into the real level of inequality in a country. Only looking at income inequality on the basis of the results of a specific year would not incorporate the position of households in their life cycle and not reflect inequality on the basis of the permanent income of households, therewith very likely overstating inequality (Fisher, Johnson and Smeeding, 2012[3]).
12.2.2. Analysing changes over time
In analysing the plausibility of distributional results, compilers can also look at changes over time. For this purpose, one may focus on changes in the absolute values or in the distribution of specific items or aggregates. Both may show remarkable changes which may point to issues in the data. For example, a sharp increase or decrease in the absolute value of a specific item for a household group will probably raise questions, whereas this will also be the case when the relative distribution shows a significant change in comparison with previous years. The plausibility of such changes will also depend on the specific item. Some items may be expected to show more fluctuation over time (e.g. mixed income and distributed income of corporations) than others (e.g. operating surplus and compensation of employees).
When analysing the plausibility of changes over time at the level of household groups, one has to be aware that changes may be caused by changes at the level of the underlying households or by changes in the composition of these groups. For example, households that were classified in the lowest income group in a specific year need not necessarily be classified in the same group in the following year. Ideally, more information is available on dynamics between household groups over time. This may for example be done on the basis of panel information which may enable following the same household over time. An alternative is to look at more granular breakdowns of household groups, for example further breaking down income groups into household type. Also the underlying socio-demographic information may provide more insight into changes in the stability of the composition of specific household groups over time.
As was the case with the indirect links (as explained in the previous subsection), no immediate conclusions can be drawn on the basis of this analysis with regard to inconsistencies in the distributional results. It will depend on the underlying reasons for these changes, whether it may indeed point to implausible results. For that reason, it is important to look at dynamics between household groups over time (as explained above), but also to have more insight into (changes in) the computation of these results over time. Analysis of changes in the contributions of the underlying micro data, the imputations for missing elements and alignment of the micro data to the national accounts totals over time may show the main underlying reason for the change in the distributional results at the aggregated level, also providing more insight into the plausibility of these results. This is explained in more detail in the next section.
12.2.3. Making cross-country comparisons
A third approach to obtain more insight into the plausibility of distributional results is to compare them with results from other countries. Although results are likely to differ across countries because of country-specific characteristics, such a comparison may provide useful insight in how comparable or different distributions are for specific items from those in other countries. Particularly for items for which no micro data is available or for which gaps between the micro and macro data show to be relatively large and for which compilers may have had to rely on several assumptions to derive the distributional results, such a comparison may prove very helpful. In making these cross-country comparisons, it is recommended to look for countries that are deemed most comparable. Furthermore, in case of any differences it may also be important to assess how the results in other countries have been derived.
In making cross-country comparisons, one can look at the distributional results for the various items and aggregates. It is recommended to compare results at the most granular level of detail possible as this is expected to provide the most reliable comparison (bearing in mind that the share of the various items in the aggregates may differ across countries).
In addition to comparing the distributional results, one may also compare the socio-demographic composition of the various household groups across countries. On the one hand, this may provide insight into the plausibility of the allocation of households to the various household groups and, on the other hand, it will provide more information on whether distributional results for specific household groups may expected to be comparable across countries. If the socio-demographic compositions of household groups show to be very different, their results are less likely to be comparable.
Finally, one may also compare the composition of (adjusted) disposable income and actual final consumption in terms of their underlying components of household groups across countries. It may for example show the share of compensation of employees in disposable income for the various income quintiles across countries or the percentage of income that is spent on food and non-alcoholic beverages by various income groups across countries. It may be interesting to see how comparable or different these compositions are across countries, particularly for those countries for which household groups may be deemed comparable.
Again, cross-country comparisons may not directly point to incorrect distributional results but may show where additional analysis may be needed to check the plausibility of the results. It may trigger the compiler to try to assess the underlying rationale for these differences.
12.3. Obtaining insight into possible reasons for implausible results
If questions arise about the plausibility of some of the distributional results, it is important to look at the underlying composition of these results. This will provide insight to what extent these results have been driven by underlying micro data (including any corrections for conceptual and classification differences), imputations for missing items, and the alignment of the micro data to the national accounts totals. The robustness of the distributional outcomes will then depend on the size and the reliability of each of these factors. This will also provide insight to what extent different decisions in the process may lead to different distributional results. For example, if the assumptions to allocate imputed amounts to underlying households are deemed to be relatively weak, it may be assessed whether a different allocation may lead to more plausible results. When looking at results for the aggregates, it also has to be borne in mind that these may have been influenced by linking of data across different data sources. Therefore, the quality of this matching should also be assessed in checking the robustness of the distributional results.
12.3.1. Assessing the composition of the distributional results
A first step in obtaining more insight into the robustness of the distributional results is to look at their composition in terms of underlying micro data (including corrections for conceptual and classification differences), imputation for missing items, and the alignment of the micro data to the national accounts totals. A general overview as presented in Section 7.2 may already provide insight to what extent these different components have contributed to the overall distributional results and how this compares to other countries and how it develops over time. More detailed overviews focusing on the composition of the results for the underlying household groups will provide more insight into how the allocation of these components affect the distributional results and how different decisions in the compilation process may lead to different outcomes. These more detailed overviews are the focus of this subsection.
Table 12.3 presents a template to show the composition of the results for a specific item across household groups, broken down into the contributions by micro data (including corrections for conceptual and classification differences), the imputation for missing items, and the alignment of the micro data to the national accounts totals. It has to be borne in mind that most of these contributions will be positive (e.g. if an item is missing and should be imputed), but that they may also be negative (e.g. if the micro aggregate exceeds the national accounts aggregate). For that reason, the template separately distinguishes positive and negative contributions. Depending on whether the micro data fall short or exceed the national accounts total and on how the gap is allocated to underlying households, the alignment of the micro-macro gap will be recorded as positive or negative contribution. Please note that the template only shows the contributions of micro data, imputations and the alignment of micro and macro data, but depending on the amount of underlying information further details may be included in the template, such as separate corrections for conceptual and classification differences and further breakdowns of the imputations made for various missing elements.
Table 12.3. Template to show the composition of a specific item
Q1 |
Q2 |
Q3 |
Q4 |
Q5 |
|
---|---|---|---|---|---|
NA aligned total |
… |
… |
… |
… |
… |
Positive contribution |
|||||
Micro data |
… |
… |
… |
… |
… |
Imputation |
… |
… |
… |
… |
… |
Positive alignment of micro-macro gap |
… |
… |
… |
… |
… |
Negative contribution |
|||||
Negative alignment of micro-macro gap |
… |
… |
… |
… |
… |
Whereas these tables can be used to assess the composition of the various items in terms of micro data, imputations and alignments, it can also be used to assess the composition of the main aggregates. In that case, the results for the underlying items should be added up to arrive at the compositional results for the totals, bearing in mind that some items will positively contribute to the aggregates whereas others may contribute negatively.
Table 12.4 provides an example of the composition of adjusted disposable income on the basis of its underlying contributions. A distinction has been made into positive and negative contributions, both broken down into micro data, imputations and alignment of the micro and macro data. The micro data reported under the positive contributions show the sum of all the micro data that positively contribute to adjusted disposable income, such as the micro data underlying compensation of employees, interest received, distributed income of corporations received and current transfers received. The micro data under the negative contributions show all the micro data that negatively contribute to adjusted disposable income, such as the micro data underlying interest paid, current on income and wealth, and current transfers paid. Whereas this will usually be relatively straightforward for micro data and for imputed items, this may be less straightforward for the alignment of micro data to national accounts totals. Whether this should be recorded as positive or negative contribution will depend on whether the micro aggregate exceeds or falls short of the national accounts total and whether the item itself positively or negatively contributes to the aggregate. For example, if the micro aggregate for interest received exceeds the national accounts total, the alignment of the micro-macro gap will negatively contribute to adjusted disposable income. But the same will be the case when the micro data underlying taxes paid fall short of the national accounts totals. Compilers should be aware of this issue to properly account for the positive and negative contributions of the various underlying components.
Table 12.4. Example of the composition of an item on the basis of its underlying contributors
Composition of adjusted disposable income as result of underlying micro data, imputations and alignment of micro-macro gaps.
Adjusted disposable income |
Q1 |
Q2 |
Q3 |
Q4 |
Q5 |
---|---|---|---|---|---|
NA aligned total |
165 |
185 |
245 |
250 |
295 |
Positive contributions (+) |
|||||
Micro data |
200 |
250 |
300 |
350 |
400 |
Imputation |
40 |
35 |
40 |
28 |
30 |
Alignment of micro-macro gap |
15 |
20 |
30 |
22 |
25 |
Negative contributions (-) |
|||||
Micro data |
60 |
80 |
90 |
110 |
120 |
Imputation |
20 |
20 |
20 |
20 |
20 |
Alignment of micro-macro gap |
10 |
20 |
15 |
20 |
20 |
The composition of (aggregated) items may also be presented graphically. Figure 12.1 provides an example for the composition of adjusted disposable income. It presents distributional results for the five quintiles, showing the positive and negative components, broken down into underlying micro data, imputations and alignments.5
Figure 12.1. Example of composition of distributional results
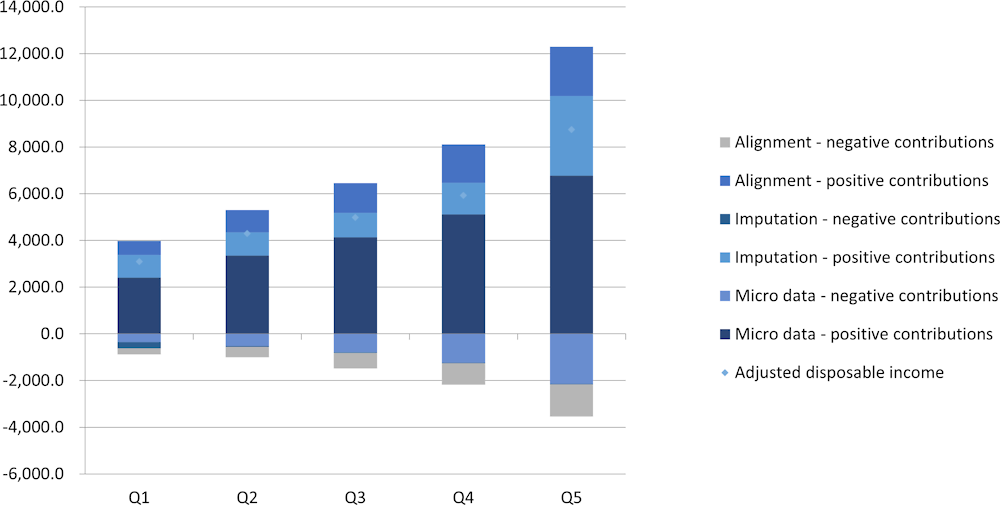
When analysing the results, the contributions can be assessed at an aggregated level, such as presented in Table 12.4 and Figure 12.1. The positive and negative contributions may be further broken down into underlying components. This may provide further insight into which items have the largest contributions to aggregates for specific household groups. Furthermore, it may provide more insight in how different allocations for some specific items for which the allocations are deemed to be less reliable, may affect the distributional results. For example, allocating a larger part of a micro-macro gap to lower income households will change the overall distribution and may at the same time increase the plausibility of the overall results.
By carefully assessing the margins of error surrounding the components contributing to the distributional results, one may derive the margins surrounding these outcomes and assess how these may be affected by different allocations. The initial allocation may already be the result of a careful analysis considering all available (direct or indirect) information, but there may still be some margin surrounding these allocations. This will be particularly true for allocations of micro-macro gaps in case no information is available on what may have been causing the remaining gap.
In assessing possible margins of error in relation to the allocation of the remaining micro-macro gaps, one has to look at sensible alternatives for allocating the gap. In this regard, the proportional allocation may be assumed as a rather reliable way of closing any final micro-macro gap under the assumption that any remaining gap is likely related to more generic issues that will be affecting households across the distribution in rather similar ways.6 However, slightly different allocations could be considered. For that reason, it may be useful to conduct sensitivity analyses, starting from the underlying micro data and slightly adjusting the weights in comparison to a proportional allocation (i.e. assigning relatively larger amounts to some household groups at the expense of others), which would respect the initial distribution to a large extent and be in line with the assumption that any remaining gap is likely due to more generic factors.7
For any other components, compilers may assess the reliability of their applied allocation and assess what plausible alternatives could be envisaged. For example, for items for which direct micro data is missing, compilers may assess the reliability of the proxy applied to arrive at the relevant distributions. In some cases, the allocation has been based on an item that closely links to the target variable, leaving very small margins of error. In other cases, the proxy may have been much weaker, leaving more uncertainty around the results.
The same applies to the matching of the results across data sets. Compilers should try to conduct sensitivity analyses to check how the results may change when applying slightly different assumptions for the linking or matching. In some cases, this may have a very small impact on the results (i.e. when applying direct linking on the basis of identifiers), but the impact may be larger when the matching relies on specific assumptions (i.e. in case of statistical matching or matching at the aggregated level).8
12.3.2. Checking plausibility of the national accounts data
Although it is not mentioned as one of the direct contributors to the distributional results, national accounts totals may have a large impact on the data, as they constitute the benchmark for the distributional results. In that regard, they feed into the alignment of the micro data to the national accounts totals. Especially in case of large micro-macro gaps, it is important to assess the quality of the national accounts totals, as was explained in Chapter 7 For that purpose, it would be helpful to have more insight in how the national accounts result has been derived, obtaining more information on the main underlying data source(s) (e.g. whether it has been based on direct information on the household sector, on counterparty sector information or whether it has been derived as a residual) and on changes that may have been applied in the course of the compilation to arrive at the final national accounts result. This information would help in assessing the reliability and possible margins of error surrounding the results.
12.3.3. Checking plausibility of the micro data
In analysing the consistency and plausibility of the results, it is also important to assess the quality of the underlying micro data. Usually, these data will already have undergone extensive checks as part of the compilation of the relevant micro statistics, but additional checks may be performed in the process of compiling distributional results in line with national accounts totals. For example, some of the checks as described in Section 12.2 may not have been performed yet at the micro level, as it concerns confronting data from multiple data sources that may not have been available at the level of the individual statistics. A specific example is the confrontation of income and consumption results at the micro level, which often can only be done by combining information from different data sets. Furthermore, compilers can check the consistency of micro data over time, look at comparisons of results within and across household groups, and look at the distribution of the results within the micro source. Such checks may provide insight into the plausibility of the data included in the various data sources. However, it has to be borne in mind that inconsistencies or implausibilities after linking data across multiple data sources may also be due to incorrect linking of the data. Thus, in case inconsistencies or implausible results are found at the micro level after linking data from multiple data sets, both the quality of the linking procedure and the underlying micro data should be carefully assessed.
12.3.4. Checking plausibility of imputations
It is also important to assess the reliability of the amounts and the allocation for items that are missing in the micro data. In some cases, these may be derived on the basis of auxiliary information, but in other cases, they may have been derived to a large extent on the basis of assumptions. In the latter cases, it is important to assess how different assumptions would affect the results and whether that would lead to more plausible results, either for the item itself or for the aggregated items. As imputations may relate to various elements that may be missing in the micro data, such as missing parts of the population, missing elements in relation to the national accounts items, underground economy and illegal and informal activities, ideally the amounts and their allocations should be assessed separately, as they may have different distributions and may rely on different types of underlying information.
12.3.5. Checking plausibility of aligning micro-macro gaps
The alignment of the gaps between micro and macro data may account for a large part of the distributional results. For that reason, it is important to check the plausibility of this allocation. In some cases, auxiliary information may be available on the basis of which these gaps can be allocated to the most probable underlying causes and for their allocation to the relevant households, but if this is not the case, it should be assessed whether the allocation that has been applied indeed leads to the most plausible results for the relevant item and for the aggregates. In case different allocations lead to more plausible results and also make sense from a technical and economic point of view, compilers may decide to apply an alternative allocation. In that regard, it is also important to take note of underlying reasons distinguished by other countries to explain any micro-macro gaps for specific items and how they have dealt with these. Furthermore, it is important to monitor the size and allocation of these gaps over time.
12.3.6. Checking plausibility of linking or matching data across data sources
Finally, the quality of the matching of data across different data sources should be checked. It will depend on the specific technique used (see Chapter 8) how one may best check for this plausibility. If linking or matching is done at the micro level, the plausibility of the matching results can be checked at the micro level, for example by looking at consistency between items from different data sets that have direct or indirect links or by looking at the consistency between income and consumption (and possibly wealth). If these checks show implausible results, this could point to errors in the micro data or to errors in matching the data. In that case, both the quality of the matching procedure as the underlying micro data should be carefully assessed. Such a confrontation may also be applied when matching data at the aggregated level. Furthermore, in that case, one may also look at the fitness of underlying socio-demographic characteristics of the groups after linking. For example, if the characteristics of the first income group according to data sources on income are completely different from those derived on the basis of data sources on consumption, this may point either to errors in the underlying data (i.e. in the income and/or consumption data or in the reported socio-demographic information) or in the matching across data sources. It would in that case be best to liaise with the micro experts to retrieve the most likely cause for the implausible results and to solve this in the best possible way.
12.4. Conclusions
This chapter has presented several ways to check the consistency and plausibility of the distributional results and provided guidance to assess possible causes for any inconsistencies or implausible results. As has become clear from this chapter, it will often be difficult to draw firm conclusions on errors in the (processing of the) data, but these checks may point to possible issues in the compilation process. This should help compilers to carefully assess the inputs and assumptions used to arrive at these results and to check whether alternative decisions would be possible that may lead to more plausible results. Of course, this does not imply that compilers may just work towards any specific outcome, as they should carefully assess to what extent different assumptions may indeed be possible and valid from a technical, economic and historic point of view. In that regard, compilers should always account for any changes in the data or in the assumptions that may change the distributional results and to explain their rationale from a technical and economic perspective.
References
[3] Fisher, J., D. Johnson and T. Smeeding (2012), “Inequality of Income and Consumption: Measuring the Trends in Inequality from 1985-2010 for the Same Individuals”, http://www.iariw.org/papers/2012/FisherPaper.pdf (accessed on 29 September 2017).
[2] Friedman, M. (1957), A Theory of the Consumption Function, Princeton University Press, Princeton.
[1] Modigliani, F. and R. Brumberg (1954), “Utility analysis and the consumption function: An interpretation of cross-section data”, in Post-Keynesian Economics, Ruthers University Press New Brunswick New Jersey, London.
Notes
← 1. The EG DNA has been investigating possibilities to include information on capital transfers received (D9R) and paid (D9P), gross capital formation (P5) and acquisitions less disposals of non-produced assets (NP) to arrive at results up until net lending/net borrowing.
← 2. In some cases, negative values may still show up for these items in the micro data, for example related to repayments of wages and taxes. Theoretically, these repayments should lead to a correction to the relevant item in the period in which the “incorrect” amount was recorded, but in practice, this may not always be feasible.
← 3. This also holds for its underlying components, operating surplus from owner occupied dwellings (B2R1) and operating surplus from leasing of dwellings (B2R2).
← 4. This also holds for mixed income excluding underground and own account production (B3R3). Furthermore, in theory it may also hold for own account production (B3R1) and underground production (B3R2), although this is not very likely in practice.
← 5. The breakdown into micro data and alignments is derived on the basis of the assumption of proportional adjustment of the gaps between the micro and macro aggregates for each individual component.
← 6. One could also apply assumptions that the gap is due to very specific reasons only involving a limited group of households, but this may be less likely. For example, it could be assumed that the gap for a specific item is fully due to underreporting by households at the lower end of the income distribution. However, as these may consist of very different types of households, it would seem very unlikely that the underreporting would only apply to this specific group of households and that there would be no issues for similar types of households elsewhere in the distribution. Furthermore, assigning the full micro-macro gap to a very limited group of households may imply assigning relatively large values to specific households in comparison to their initially reported data (possibly also assigning large values to households that did not report any values for a specific item to start with), with possibly large impacts on their ranking in the overall distribution. This will be much smaller when keeping closer to the initially reported data and assuming more generic causes for the remaining gaps.
← 7. The EG DNA is currently developing a more specific proposal on how to conduct sensible sensitivity analyses on the basis of these assumptions, as part of the development of a more elaborated quality framework.
← 8. The EG DNA is looking into the development of standard sensitivity analyses to accompany the results as part of the new quality framework.